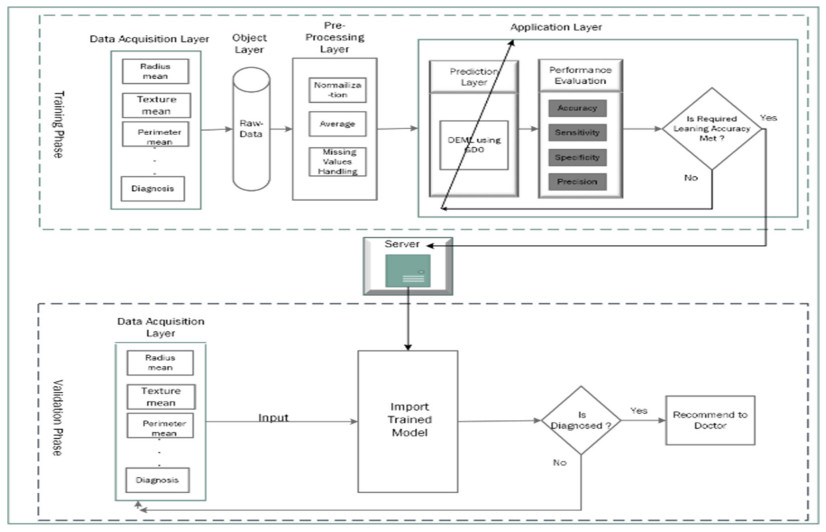
Cancer is a manifestation of disorders caused by the changes in the body's cells that go far beyond healthy development as well as stabilization. Breast cancer is a common disease. According to the stats given by the World Health Organization (WHO), 7.8 million women are diagnosed with breast cancer. Breast cancer is the name of the malignant tumor which is normally developed by the cells in the breast. Machine learning (ML) approaches, on the other hand, provide a variety of probabilistic and statistical ways for intelligent systems to learn from prior experiences to recognize patterns in a dataset that can be used, in the future, for decision making. This endeavor aims to build a deep learning-based model for the prediction of breast cancer with a better accuracy. A novel deep extreme gradient descent optimization (DEGDO) has been developed for the breast cancer detection. The proposed model consists of two stages of training and validation. The training phase, in turn, consists of three major layers data acquisition layer, preprocessing layer, and application layer. The data acquisition layer takes the data and passes it to preprocessing layer. In the preprocessing layer, noise and missing values are converted to the normalized which is then fed to the application layer. In application layer, the model is trained with a deep extreme gradient descent optimization technique. The trained model is stored on the server. In the validation phase, it is imported to process the actual data to diagnose. This study has used Wisconsin Breast Cancer Diagnostic dataset to train and test the model. The results obtained by the proposed model outperform many other approaches by attaining 98.73 % accuracy, 99.60% specificity, 99.43% sensitivity, and 99.48% precision.
Citation: Muhammad Bilal Shoaib Khan, Atta-ur-Rahman, Muhammad Saqib Nawaz, Rashad Ahmed, Muhammad Adnan Khan, Amir Mosavi. Intelligent breast cancer diagnostic system empowered by deep extreme gradient descent optimization[J]. Mathematical Biosciences and Engineering, 2022, 19(8): 7978-8002. doi: 10.3934/mbe.2022373
[1] | Jian-xue Tian, Jue Zhang . Breast cancer diagnosis using feature extraction and boosted C5.0 decision tree algorithm with penalty factor. Mathematical Biosciences and Engineering, 2022, 19(3): 2193-2205. doi: 10.3934/mbe.2022102 |
[2] | Chunmei He, Hongyu Kang, Tong Yao, Xiaorui Li . An effective classifier based on convolutional neural network and regularized extreme learning machine. Mathematical Biosciences and Engineering, 2019, 16(6): 8309-8321. doi: 10.3934/mbe.2019420 |
[3] | Jiajia Jiao, Xiao Xiao, Zhiyu Li . dm-GAN: Distributed multi-latent code inversion enhanced GAN for fast and accurate breast X-ray image automatic generation. Mathematical Biosciences and Engineering, 2023, 20(11): 19485-19503. doi: 10.3934/mbe.2023863 |
[4] | Bo An . Construction and application of Chinese breast cancer knowledge graph based on multi-source heterogeneous data. Mathematical Biosciences and Engineering, 2023, 20(4): 6776-6799. doi: 10.3934/mbe.2023292 |
[5] | Juan Zhou, Xiong Li, Yuanting Ma, Zejiu Wu, Ziruo Xie, Yuqi Zhang, Yiming Wei . Optimal modeling of anti-breast cancer candidate drugs screening based on multi-model ensemble learning with imbalanced data. Mathematical Biosciences and Engineering, 2023, 20(3): 5117-5134. doi: 10.3934/mbe.2023237 |
[6] | Vinh Huy Chau . Powerlifting score prediction using a machine learning method. Mathematical Biosciences and Engineering, 2021, 18(2): 1040-1050. doi: 10.3934/mbe.2021056 |
[7] | Sushovan Chaudhury, Kartik Sau, Muhammad Attique Khan, Mohammad Shabaz . Deep transfer learning for IDC breast cancer detection using fast AI technique and Sqeezenet architecture. Mathematical Biosciences and Engineering, 2023, 20(6): 10404-10427. doi: 10.3934/mbe.2023457 |
[8] | Qun Xia, Yangmei Cheng, Jinhua Hu, Juxia Huang, Yi Yu, Hongjuan Xie, Jun Wang . Differential diagnosis of breast cancer assisted by S-Detect artificial intelligence system. Mathematical Biosciences and Engineering, 2021, 18(4): 3680-3689. doi: 10.3934/mbe.2021184 |
[9] | Anastasia-Maria Leventi-Peetz, Kai Weber . Probabilistic machine learning for breast cancer classification. Mathematical Biosciences and Engineering, 2023, 20(1): 624-655. doi: 10.3934/mbe.2023029 |
[10] | Chelsea Harris, Uchenna Okorie, Sokratis Makrogiannis . Spatially localized sparse approximations of deep features for breast mass characterization. Mathematical Biosciences and Engineering, 2023, 20(9): 15859-15882. doi: 10.3934/mbe.2023706 |
Cancer is a manifestation of disorders caused by the changes in the body's cells that go far beyond healthy development as well as stabilization. Breast cancer is a common disease. According to the stats given by the World Health Organization (WHO), 7.8 million women are diagnosed with breast cancer. Breast cancer is the name of the malignant tumor which is normally developed by the cells in the breast. Machine learning (ML) approaches, on the other hand, provide a variety of probabilistic and statistical ways for intelligent systems to learn from prior experiences to recognize patterns in a dataset that can be used, in the future, for decision making. This endeavor aims to build a deep learning-based model for the prediction of breast cancer with a better accuracy. A novel deep extreme gradient descent optimization (DEGDO) has been developed for the breast cancer detection. The proposed model consists of two stages of training and validation. The training phase, in turn, consists of three major layers data acquisition layer, preprocessing layer, and application layer. The data acquisition layer takes the data and passes it to preprocessing layer. In the preprocessing layer, noise and missing values are converted to the normalized which is then fed to the application layer. In application layer, the model is trained with a deep extreme gradient descent optimization technique. The trained model is stored on the server. In the validation phase, it is imported to process the actual data to diagnose. This study has used Wisconsin Breast Cancer Diagnostic dataset to train and test the model. The results obtained by the proposed model outperform many other approaches by attaining 98.73 % accuracy, 99.60% specificity, 99.43% sensitivity, and 99.48% precision.
Breast cancer is the second most common cancer in women after skin cancer [1]. According to WHO statistics, about 7.8 million women were diagnosed with breast cancer by the end of 2020 [2]. It is getting more ubiquitous with each passing day. Resultantly, it is expediting the mortality rate all over world. Moreover, it has become the second greatest cause of mortality among women [3]. This threatening trend can be countered if the lumps are detected earlier [4]. In the early screening of breast cancer diagnosis, the mammogram is extensively used due to its comparatively inexpensive characteristics [5]. During the diagnosis process, factors like radiologist experience and activeness play a vital role [6,7]. Breast cancer is caused by the irregular growth of cells in the breast. It starts as cells begin to develop in an unbalanced fashion [8]. Cancer-free breast tissue may expand unusually, but it does not expand beyond the breast. A healthcare provider must test any breast lump or shift to see whether it is benign or malignant? A tumor can be benign (non-cancerous) or malignant (cancerous). Benign cells are universal. Further, they grow rapidly and do not infiltrate neighboring tissues or spread to other body parts. Malignant tumors, on the other hand, are cancerous in character. Malignant cells, if left untreated, ultimately go beyond the initial tumor's area and extend their reach to the other parts of the body [9].
Plethora of imaging systems have been developed to diagnose and cure the breast cancer. Besides, many models meant for the earlier detection of this menace have been suggested by the experts. Moreover, many studies have been carried out [10,11] to enhance the diagnosis accuracy of this threat. Data mining has proved very useful in extracting the important data from a large set of data [12]. Further, the varied techniques of this discipline have been extensively exploited in the discovery of many diseases. Moreover, the techniques like machine learning (ML), statistical analysis, data warehouse, fuzzy systems, databases, and neural networks have been employed in the prediction and diagnosis of various types of cancer [13]. Additionally, deep models helped a lot to the academicians and practitioners while they grappled the intricacies in the real-world training [14,15,16]. In the training of ML and deep learning (DL) models, the gradient descent optimization method is crucial. In recent years, many new variant algorithms [17,18,19,20] have been developed to improve it further. Machine learning is a branch of computer science that make computers to comprehend the tasks without explicitly teaching them to do so [21,22,23]. Introducing a cost function to machine learning and data mining enables the machines to discover appropriate weights for outcomes [24]. Optimization finds the function parameters in a way that the solution of the problem becomes simpler. Many machine learning methods are plagued by this problem [25,26].
Gradient descent is applied for optimization of multiple loss functions like Support Vector Machine (SVM), and Logistic Regression (LR) methods [27]. Gradient descent optimization-based techniques for binary classifications have produced better accuracy for the detection of diseases. These diseases, in turn, are based on certain parameters which provide the necessary basis to the research for carrying out breast cancer detection [28]. Deep learning is an offshoot of ML techniques whose primary thrust is on the acquisition of data models rather than job-specific algorithms [29]. Common classical diagnosis methods are not delivering the way the current era needs. So, more accurate and reliable breast cancer diagnosis techniques are required to thwart the rising numbers of death of women [30]. The objective of this study is to build a deep learning-based model for breast cancer diagnosis that has a better prediction rate with minimum complexities. To develop such a diagnostic system, deep extreme machine learning using gradient descent optimization technique is being proposed in this work. DEGDO based model consists of two major phases; first is the training and the second one is the validation phase. There are three major layers in the training phase. The data acquisition layer gets the data to be used in training that is, in turn, taken from some source or test reports. Data acquisition layer stores that data in the object layer where it is in raw form. Data in the object layer can contain noise which is needed to be removed. Preprocessing layer processes the raw data in order to remove noise and to handle missing values. The application layer is the main part of the training phase which consists of two sub layers, i.e., predication layer and the evaluation layer. The prediction layer holds the actual DEGDO to predict the disease and then the result is evaluated by the evaluation layer based on certain evaluation parameters. Moreover, accuracy is measured and get it compared with the required accuracy. If it meets the training criterion, training process ends and the trained model is stored on the server. In case, training criterion is not met, the required accuracy model is retrained. This process continues unless it obtains the required accuracy. In the validation phase, data is provided to the trained model imported from the server and the result of the diagnosis is predicted. To carry out the experiments, a dataset has been taken from UCI Machine Learning Repository for the proposed research. This dataset is based on 569 instances with 32 attributes for each record [31]. The proposed model predicts the diagnostic status as positive or negative based on the provided set of parameters.
The current study is geared towards the enhancement of the prognosis accuracy of the breast cancer detection. Moreover, it introduces a more reliable classification method using deep extreme with gradient descent optimization technique resulting in a higher diagnostic accuracy rate. Apart from that, this work also presents a comparative study of the state-of-the-art methods on the same dataset. Ten different evaluation metrics have been employed to demonstrate the utility, effectiveness and authenticity of the proposed work. These metrics span accuracy, specificity, sensitivity, positive predicted value (PPV), negative predictive value (NPV), false-positive rate (FPR), false-negative rate (FNR), false discovery rate (FDR), F-score (F1) and Matthews correlation coefficient (MCC). Moreover, the area under the curve (AUC) from the receiver operating characteristic (ROC) curve has also been used for the evaluation of the proposed model. Besides, both the split and K-fold cross-validation have also been applied.
Rest of the article has been formatted like this. Section 2 summarizes the relevant literature. Section 3 describes the proposed model and the procedure for conducting a detailed evaluation about the Breast cancer like malignant or benign. Details of the dataset used for research have been given in the Section 4. Section 5 sheds the necessary light on the experimental results, discussion and the ensuing findings. Finally, the paper has been concluded in the last Section 6.
The conventional approach to cancer diagnosis rests upon a technique called colloquially a "gold standard" which entails three screenings: clinical assessment, radiographic, and pathology [32]. The traditional approach, rooted in regression, shows the existence of malignancy. Whereas, the state-of-the-art machine learning methods and algorithms are based on model development. A model is created to predict previously unknown data. As this model is sparked with a list of parameters depending upon the nature of the problem, it generates the required outcome through the twin processes of training and testing [33]. Pre-processing, feature selection or extraction, and classification are the three major processes used in the machine learning [34].
Breast cancer is the second leading cause of death after the lung cancer. 8% of women throughout their lifetime are diagnosed with breast cancer. Machine learning techniques have been frequently employed to categorize the breast cancer. Researches carried out to diagnose breast cancer with the help of KNN classifier offered better accuracy (97.5%) with a lower error rate compared to its Naive Bayes counterpart (96.19%) [35]. Detection of breast cancer with the help of algorithms has become a pronounced medical problem in the current era. Diagnosis of early breast cancer is, no doubt, a key to the survival of a patient. This study showed how a decision tree algorithm, along with some other approaches, was used to construct a real breast cancer diagnosis model for a clinical and a systematic treatment. The experimental results demonstrated the viability and do-ability of the proposed concept. The effectiveness of the decision tree technique for the detection of breast cancer has been studied and shown through the experiments [36]. Besides, a combination of K-means and K-Support Vector Machine (K-SVM) algorithms was developed for extracting valuable information which could be utilized to diagnose the tumor. Moreover, K-means algorithm has been used for the detection of the secret/hidden patterns of the cancerous cells. Each tumor membership was measured and viewed as a new trait in these patterns. Apart from that, a support vector machine was employed to acquire a novel classifier in order to distinguish between benign and malignant tumors. The proposed technique raised the accuracy of Wisconsin diagnostic breast cancer results to the mark of 97.38%. Findings given in [37] not only reflected the potential of the recommended solution for the diagnosis of breast cancer but it also indicated the lower cost of time during preparation phase.
To identify the hematoxylin and eosin-stained-breast biopsy photos using the convolutional neural network, the researcher utilized a deep-learning-based method and obtained 83.30% accuracy [38]. Moreover, the Sequential Minimum Optimization (SMO) and K Nearest Neighbor Classification Algorithms (IBk) were used for the breast cancer estimation using certain ensemble techniques. Apart from that, the dataset used for the experiments purpose consisted of 683 records with 9 parameters for each record. Moreover, Weka data mining tool was used for this research, which used cross-validation (K Fold) technique to determine the accuracy of the proposed method for breast cancer diagnosis. Additionally, the SMO achieved 96.19% accuracy while IBk reached to the mark of 95.90% for breast cancer detection [39]. Breast cancer data was collected from Iranian Centre on Breast Cancer (ICBC). The results were evaluated by the researchers through an array of validation metrics like specificity, and sensitivity of DT (C4.5), ANN, and SVM-based models. The ensuring results showed t accuracy hat SVM, with an accuracy of 95.70%, was the best predictive algorithm for breast cancer screening [40]. Apart from that, using the ADTree, J48, and CART algorithms taking digital files in DOCOM format, the Indian Breast Cancer Center Adyar, Chennai's breast cancer dataset was examined to build a model for breast cancer diagnosis. The dataset used by the researchers was in the CSV format. In this work, three different data mining (DM) algorithms were used to investigate the accuracy of prediction. The findings out of this study revealed that the CART algorithm is more suitable than the others as CART achieved an accuracy of 98.5% while ADTree and DT J48 achieved accuracies of 97.7 and 98.1% respectively [41]. Moreover, researchers performed a comparative study on NB, RF, LR, ANN-MLP, and KNN based models for breast cancer diagnosis. Apart from that, UCI data was used to diagnose breast cancer by taking the top ten different parameters from the dataset. Each algorithm was applied to the dataset to test the output of each model. The accuracies of the instances identified by KNN, NB, and RF were 72.3, 71.6 and 69.5% in a respective fashion. While, the accuracies of LR and ANN-MLP remained just 68.8 and 64.6% respectively [42]. In Nigeria, breast cancer is a very common disease. Further, no diagnosis is available for such a heterogeneous disease in Nigeria. Dataset having 17 attributes was taken by LASUTH, Nigerian Cancer Registry. The NB probabilistic method was applied for controlling the dependent group count on the probabilistic model. Top to bottom greedy checks on training data were implemented in the decision tree J48. J48 came out to be the most suitable procedure to predict and diagnose breast cancer as its accuracy was 94.20% while NB obtained an accuracy of just 82.60% [43].
The deep learning approaches including multiple kernel/activation functions like maxout, tanh, and exprectifier to diagnose breast cancer on infected cells, were applied. Moreover, a comparison of different ML techniques like NB, DT, SVM, and RF was carried out on the Wisconsin dataset. It was shown that the highest accuracy of 96.99% was obtained by Exponential Rectifier Linear Unit activation function - a deep learning algorithm in order to diagnose breast cancer [44]. Apart from the breast cancer diagnosis dataset, DT, NB, and KNN were also introduced to build a model for diagnosing the breast cancer. In this study, researchers utilized the original Wisconsin dataset. The results indicated that the accuracy of the NB classifier reached to the mark of 95.99% which was higher than those of DT and KNN algorithms [45]. Moreover, comparative analyses of different nonlinear supervised learning models like MLP, KNN, SVM, CAR Tree and Gaussian NB have been carried out for the detection of breast cancer. Comparative analysis for the said methods was the main theme of the research for efficient breast cancer identification. Apart from that, prediction accuracy for every algorithm was independently calculated using the Wisconsin breast cancer dataset. Moreover, for performance analysis of algorithms, a cross-validation (K-Fold) approach has been applied. MLP produced 96.70% accuracy for breast cancer detection which was higher among all [46]. To predict the restorative emergence of breast cancer, a data mining-based model was developed. Additionally, there were two major methods for this model namely Extreme Learning Machine (ELM) and the Bat algorithm. The prejudices and random weights were generated using the Bat algorithm. MATLAB software was used for carrying out experiments on the Wisconsin breast cancer dataset with certain selected attributes. Besides, the coefficient correlation approach was used for attributes selection. ELM and Bat algorithms have been employed to predict whether the breast cancer was recurring or non-recurring. To verify the consistency of research at various training levels, tanh and sigmoid activation functions were applied. When tanh was used as an activation function, 93.75% accuracy was recorded with a minimum error rate (RMSE = 0.30) [47]. The greedy search algorithm was proposed to build a diagnostic system to predict breast cancer diagnosis.
For the selection of features that are important ranging from the broad set of data to the trivial ones, SVM with Constrained Search Sequential Floating Forward Search (CSSFFS) is used. For this experiment, the dataset was compiled from the WDBC machine learning database. Researchers used the cross-validation (K-Fold) technique to establish results for CSSFFS with SVM. The main purpose of using SVM was to eliminate irrelevant features. Using the CSSFFS method, with some top attributes, accuracy was enhanced up to 98.25%. RBF network produced a decent accuracy of 93.60% when all the attributes were taken into consideration [48]. Besides, a deep learning-based automated mammography processing technique was employed for estimating the patients' risk of getting breast cancer [49]. The automatic classification was conducted for the region containing the cancerous part in images of the breast. Grasshopper optimization algorithm and CNNs were used in their proposed research. Their research model was able to manage 93% accuracy [50]. Moreover, breast cancer detection with the help of histogram images using deep learning CNN was carried out by the authors. This study showed reasonable results by obtaining an 86.60% accurate detection rate [51]. In a yet another work, a deep feature-based model was used for the breast mass categorization which, in turn, employed CNNs and decision trees [52]. A Patch-based LeNet, a U-Net, and transfer learning techniques were employed using a pre-trained FCN-AlexNet in order to identify lesions in breast ultrasound images [53]. A CNN which is pre-trained and its learned parameters were transferred to some other CNN for classification of mitoses obtained a 0.80 F-Score [54]. In another work, ELM was used to categorize breast tumor characteristics and its results were compared to the SVM classifier [55]. By training CNN with a huge quantity of time series data, [56] used CNN for the risk prediction of breast cancer. Based on 420 mammography time series data, [57] utilized deep neural networks to forecast the probability of breast cancer in near future. Moreover, [58] abstracted breast tumor representations using CNN and subsequently categorized the tumors as malignant or benign. Because features may influence both the efficacy and efficiency of a breast CAD system, [59] proposed an image retrieval system utilizing Zernike Moments (ZMs) to get the required features. Apart from that, machine learning and deep leaning optimization are popular techniques which have the potential to get used in the diverse fields like price controlling in medical domain, agriculture, business intelligence etc. Moreover, Chun-Hui He' iteration algorithms can also be used for optimization [60,61].
Deep extreme gradient descent optimization-based model comprises of two stages: training and validation. Moreover, the data acquisition layer, the pre-processing layer, and the application layer are the three levels that make up the training phase. Additionally, the data acquisition layer collects data from some source and stores it in raw form which is used as a database in future. This raw data may contain noisy values as well since it is transmitted through an online link from the source to the acquisition layer. Apart from that, pre-processing layer deals with missing values and eliminate noise from the given data. Moreover, the moving average technique is employed to approximate the missing data. Further, normalization addresses the problem of noise. As pre-processing is completed, the application layer starts its work. The application layer comprises of two sub-layers: prediction and performance evaluation. Deep extreme gradient descent optimization method is used in the prediction layer. Moreover, the performance evaluation layer assesses the predictive model's performance in terms of the validation metrics like accuracy, sensitivity, specificity, precision, and miss rate. As the required learning criterion is met, the trained model is stored on the server which, of course, is used in a later phase. Apart from that, the validation phase uses the data acquisition layer which provides data as an input to the trained model. This trained model is, in turn, imported from the server to predict the disease. Figure 1 demonstrates the methodological diagram of the proposed model based on deep extreme gradient descent optimization.
Deep extreme learning machine has been applied in the varied fields. Since conventional artificial neural network needs more samples so it consumes more time for learning. Besides, it can produce over-fit results [62]. The deep extreme learning machine is extensively used for regression and classification problems in different fields. Its learning rate is better and computational complexity is much lower than that of traditional artificial neural networks. The structure of a deep extreme learning machine model consists of three layers namely the input layer, multiple hidden layers, and the output layer [63]. Extreme learning was firstly idealized by [64].
Figure 2 shows the diagrammatical model for the proposed system based on DEGDO, where ip denotes the nodes of the input layer; hidden layer nodes are represented by h, and ODP shows the output layer node.
A mathematical representation of the filter of moving average is given in Eq (1) [77].
P[x]=1G∑G−1T=0u(x+T) | (1) |
Here u represents inputs; P denotes output, and the point of moving average is denoted by G. To increase the predictive ability and to improve the training process of the machine learning model, dataset is standardized for the interval [0, 1] with the help of Eq (2) [77,78].
C=ux−uminumax−umin;x=1,2,3…N | (2) |
At first taking training [A,B]=[av,bv](x=1,2,...,R) and input A=[av1,av2,av3...avr] samples and B=[b11,b12,b13,...,b1r] as target matrix, has been taken from training samples then matrices A and B, which can be presented as provided in Eqs (3) and (4) respectively [77,78,79]. While A and B are considered the dimensions of the input and output matrix. The extreme learning machine is utilized to adjust the weights between the input and the hidden layers. Considering the Cth as the input layer node and lth as the hidden layer node, the weights between them are represented by CE1 as given in Eq (5) [80]. Where Matrix A represents as input features; B as target matrix, C as Input layer node, weights between Input and hidden layer and D as weights between hidden neurons and output layer neurons.
A=[a11a12…a1va21a22…a2va31a32…a2v...aE1aE2…aEv] | (3) |
B=[b11b12…b1vb21b22…b2vb31b32…b2v...bE1bE2…bEv] | (4) |
C=[c11c12…c1vc21c22…c2vc31c32…c2v...cE1cE2…cEv] | (5) |
D=[d11d12…d1vd21d22…d2vd31d32…d2v...dE1dE2…dEv] | (6) |
Furthermore, Eq (7) shows the randomly selected biases for the hidden layer nodes by Extreme Learning Machine [81,82]. A function represented as f(x) is a network activation function preferred by Extreme Machine Learning. Eq (8) shows the resulting matrix given in the data acquisition layer. Equation (9) represents the column vector of S i.e., the resulting matrix [60,77,80].
B=[b1,b2,b3…bE]′ | (7) |
H=[h1,h2,h3…hz]x×y | (8) |
h1=[h1jh2jT3j⋮Txj]=[∑∝q=1Ml1f(ωlαq+bl)∑∝q=1Ml2f(ωlαq+bl)∑∝q=1Ml3f(ωlαq+bl)⋮∑∝q=1Mlyf(ωlαq+bl)](q=1,2,3,…,y) | (9) |
Z is the hidden layer outcome and transposition of H has been denoted by H′. Equation (11) shows the calculations of matrix β's weighted values by using the least square method [79,81].
Zβ=H′ | (10) |
β=Z+H′ | (11) |
To increase the overall stability of the network, β regularization term has been utilized [65].
Deep learning has become a topnotch research niche for the scientists due to the marvelous it has. Minimum four input layers and one output layer is needed for a system to qualify as a deep learning system [64]. Neurons present in different layers of deep learning networks are trained with different parameters based on the result of the previous layer. Besides, a deep learning network bears immense promise to process extensive datasets. In order to capture the positive and outstanding features of both ELM and DL, the proposed work is utilizing a deep extreme gradient descent optimization-based approach. The proposed model is based on a deep extreme learning machine with gradient descent that consists of one input layer, six hidden layers, and one output layer. Moreover, the input layer contains sixteen (16) neurons. Besides, each of the hidden layers consists of sixteen (16) neurons as well. Whereas, the output layer consists of only one (1) neuron. For the selection of the hidden layer's number of nodes, a test and error scheme is applied. The output of 2nd hidden layer is obtained as [77,81]:
Zg=Hβ+g;g=1,2,3,4…,6 | (12) |
where β+ represents the general inverse of the β matrix. Equation (11) can be helpful for obtaining values for the 2nd hidden layer [77,80].
f(WgZ+Bg)=Zg | (13) |
Four parameters are present in Eq (13). Among them, Wg represents the weight of the first 2 hidden layers. In this layer, the first neurons preference is shown by Z. Bg is the measured first hidden layer's output and Zg as the second hidden layer's projected output [78,79].
WFG=f−1(g)F+G | (14) |
F+G represents the inverse of FG. Moreover, to calculate Eq (5), f(x) has been used as an activation function [80,81]. Therefore, f(x),the activation function is corrected to revise the second hidden layer's outcome given below.
Zg+1=f(WFGZG) |
Such as WFGZG=Qhg+1
Zg+1=f(Qhg+1) | (15) |
As per Eq (16), weighted matrix β between the 2nd and 3rd layers has been updated [80]. Z+g+1 is an inverted form of Zg+1. Equation (17) provides the result of the estimated layer [80,81].
βg+1=Z+g+1H | (16) |
H+β is the inverse of the weight matrix μg+1. Then matrix FWFG = [Bg+1, Wg+1] is set by a deep extreme learning machine. Equations (10) and (11) help to achieve the output of the further layer [77,78,79].
f(x)=11+e−x | (17) |
The back-propagation algorithm provides weight initialization, feed-forward, error back-propagation, and updating weights and bias. f(x)=sigmoid(x) being an activation function is present on hidden layers of every neuron. The hidden layer and sigmoid input function of DELM can be composed through this method [80,81];
ErrorBP=12∑n(aon−ton)2 | (18) |
to = desired output t
ao = measured or calculated output
Equation (18) shows the error's back-propagation. Adjustment of weights is required to minimize the overall error [77,78,79,80,81]. Equation (19) presents the output layer's rate of weight change [77,78,79,80,81].
ΔZhd=6m,n∝∂E∂Zhd=6 | (19) |
where m = 1, 2, 3... 10 (Neurons) and n = output layer
ΔZhd=6m,n=−constant∂E∂Zhd=6 | (20) |
Applying the chain rule on Eq (20) generates Eq (21) [79,80].
ΔZhd=6m,n=−constant∂E∂aohdn×∂aohdn∂QhZhdn×∂QhZhdn∂Zhdn | (21) |
After simplification of Eq (21) it can be written as [80,82]:
ΔZhd=6m,n=constant(ton−aon)×(aohdn(1−aohdn)×aohdn) |
Through aotoZ6
ΔZhd=6m,n=constantρnaohdn | (22) |
The following method shows calculation to find out the proper weight change to hidden weight [80,82]. This is considered more complex because weighted links can become a reason for errors at every node.
Through Z6toZ1orZk
where k = 5, 4, 3, 2, 1
ΔZhdm,k∝−[∑n∂E∂aohdn×∂aohdn∂QhZhdn×∂Zhdn∂Zhdk]×∂aohdk∂QhZhdk×∂QhZhdk∂Zhdm,k |
ΔZhdm,k=−E[∑n∂E∂aohdn×∂aohdn∂QhZhdn×∂Zhdk∂aohdk]×∂aohdk∂Zhdk×∂QhZhdk∂Zhdm,k |
ΔZhdm,k=E[∑n(ton−aohdn)×aohdk(1−aohdn)×Zk,n]×aohdn(1−aohdn)×(Lm,k) |
ΔZhdm,k=E[∑n(ton−aohdn)×aohdk(1−aohdn)×Zk,n]×aohdn(1−aohdn)×(Lm,k) |
ΔZhdm,k=E[∑n(ton−aohdn)×aohdk(1−aohdn)×Zk,n]×aohdk(1−aohdk)×(Lm,k) |
ΔZhdm,k=E[ρk(Lm,k)] |
where,
ρk=[∑nρn(Zhdk,n)]×aohdk(1−aohdk) |
Modifying weight and bias among output & hidden layer is presented in Eq (23) where ∇Zm,n represent the gradient descent w.r.t Zm,n [77,79,80,81,82].
ΔZhd=6m,n(u)=ΔZhd=6m,n(u)+τ∇Zhd=6m,n | (23) |
Modifying weight and bias among input & hidden layers is present in Eq (24) where ∇Zm,n is gradient descent w.r.t Zm,n[77,79,80,81,82].
ΔZhdm,k(u)=ΔZhdm,k(u+1)+τ∇Zhdm,n | (24) |
τ represent the key to finding local minima because it gives the step size for finding local minima.
Materials and methods applied in this study are described below.
In this research, one dataset has been used for the experimentation. This dataset is accessible from the UCI Learning Repository. Apart from that, Cleveland data package was used for training, testing, and validating the prediction of breast cancer. Besides, the Wisconsin Breast Cancer Diagnostic (WBCD) dataset [66] is open to the public for analysis and research. This data set includes 32 human and biological characteristics. Further, the selection of features plays a vital role in the classification outcomes [67]. An increase in the performance and decrease in the time complexity of machine learning can be achieved through the appropriate selection of features [68]. Top 16 features have been selected using uni-variate and recursive feature selection strategies. Besides, data has been distributed among two classes (Positive and Negative). There are 355 healthy (Negative) samples and 214 diseased (Positive) samples. The selected features of data collection specifications are shown in the Table 1.
Sr. No. | Attributes | Symbol | Type |
1 | Mean of the concave sections of the contour's severity | concavity_mean | Numeric |
2 | The average of distances between the center and the peripheral points | radius_mean | Numeric |
3 | The mean value for the severity of concave sections of the contour that is the worst or the greatest | concavity_worst | Numeric |
4 | Area | area_se | Numeric |
5 | Gray-scale standard deviation | Texture | Numeric |
6 | Worst symmetry | symmetry_worst | Numeric |
7 | arithmetic mean of the regional variance in radius lengths | smoothness_mean | Numeric |
8 | The standard error for the severity of concave contour segments | concavity_se | Numeric |
9 | The mean value that is the worst or the greatest for local variance in radius lengths | smoothness_worst | Numeric |
10 | The worst or biggest number for the mean of "coastline approximation"-1 | fractal_dimension_worst | Numeric |
11 | The standard error for approximating the coastline-1 | fractal_dimension_se | Numeric |
12 | Symmetry mean | symmetry_ mean | Numeric |
13 | arithmetic mean for "coastline approximation"-1 | fractal_dimension_mean | Numeric |
14 | Symmetry se | symmetry_se | Numeric |
15 | standard inaccuracy in radius lengths due to local variation | smoothness_se | Numeric |
16 | standard inaccuracy for the standard deviation of gray-scale values | texture_se | Numeric |
An array of performance evaluation metrics has been developed to evaluate the performance of machine learning algorithms. Out of this array, frequently used metrics are accuracy (which evaluates accuracy rate (Acc)), specificity (Sp), precision (Pres), sensitivity (Sn), F-Measure, Negative Predicted Value (NPV), False Discovery Rate (FDR), False Positive Rate (FPR), False Negative Rate (FNR) and Mathew Co-relation Co-efficient (MCC), which assesses steadiness employing false positives, true negative, false negatives, and false positives values. These criteria are as follows:
Using Eq (25), accuracy can be calculated as given under [83]: -
Accuracy(Acc)=TrP+TrNTrp+TrN+Fap+FaN | (25) |
Using Eq (26), sensitivity/recall can be calculated as given under [83,84,85]: -
Sensitivity/Recall(Sn)=TrPTrP+FaN | (26) |
Using Eq (27), specificity can be calculated as given under [83,84]: -
Specificity(Sp)=TrNTrN+FaP | (27) |
Using Eq (28), Precision(PPV) can be calculated as given under [85]: -
Precision(PPV)=TrPTrP+FaP | (28) |
Using Eq (29), NPV can be calculated as given under [85]: -
NegativePredictedValue(NPV)=TrNTrN+FaN | (29) |
Using Eq (30), FPR can be calculated as given under [84,85]: -
FalsePositiveRate(FPR)=FaPTrN+FaP | (30) |
Using Eq (31), FDR can be calculated as given under [83,84]: -
FalseDiscoveryRate(FDR)=FaPTrP+FaP | (31) |
Using Eq (32), FNR can be calculated as given under [83,84]: -
FalseNegativeRate(FNR)=FaNTrP+FaN | (32) |
Using Eq (33), F1-Score can be calculated as given under [83,84]: -
F1=2×TrP(2×TrP+FaN+FaP) | (33) |
Using Eq (34) MCC can be calculated as given under: -
MCC=(TrP×TrN−FaP×FaN)sqrt((TrP+FaP)×(TrP+FaN)×(TrN+FaP)×(TrN+FaN)) | (34) |
The receiver operating curve method is used to quantify and analyze the connection between a binary classifier's sensitivity and specificity. Sensitivity quantifies the percentage of properly classified positives; specificity quantifies the percentage of properly classified negatives [69,83,84].
AUC is the measurement of the area that is entirely covered by the ROC curve and it varies between 0 to 1. If a classification model produces a 100% accuracy rate then the AUC for that model comes out to be 1. In case, classification model gives 100% wrong classification results then the value of AUC calculates to be 0.
In split validation, data is divided into certain train and test ratios. In the proposed research, dataset has been divided into different sets of train-test ratios.
K-Fold cross-validation has also been employed to test the proposed model by plugging the different values in K. We have used K = 2 to K = 10 for K-Fold cross-validation to compute the average values but we have incorporated 10 folds cross-validation for the proposed model.
The MSE analysis shows up to what extent the model has learned how much impact it is casting on the outcome. The machine's efficiency requires error minimization. The discrepancy between the intended and the actual output is measured as the mean square error. Besides, MSE, RMSR, MAE values for the training, testing, and validation phases were recorded against the different epochs.
Varied experiments have been carried out to demonstrate the classification performance of the proposed model. Moreover, WBCD dataset has been used to train, test, and validate the model. Both the Split and K-Fold cross-validation techniques have been employed to validate the DEGDO base model. Besides, different train-test ratio groups have been set up and used like 50-50, 60-40, 70-30, and 80-20. Performance results produced with different train-test ratio groups are given in the Table 3. Apart from that, K-Fold cross-validation has also been carried out using the various values of K, K = 2 to K-10, for instance. Moreover, average performance produced by the different count of folds is shown in the Figure 3.
In the proposed model, multiple classifiers have been used to compare the performance of the proposed model DEGDO with other state-of-the-art methods. Moreover, multiple performance evaluation metrics have been used to check the proposed model for different classifiers like AUC-ROC, MSE, RMSE, MAE, Acc, Sp, PPV, Sn, F-Measure, NPV, FDR, FPR, FNR, and Matthew Co-relation Co-efficient (MCC). Table 2 shows the performance assessment of the Intelligent Breast Cancer Diagnostic System Empowered by Deep Extreme Gradient Descent Optimization with different train-test ratios. Besides, experiments are conducted on the selective features as well as on all the available features. Results produced with selective features are shown in the Figures 3 and 4. Apart from that, results with a complete set of attributes lowered the classification performance when it is compared to the performance carried out with the selective features. Results without selective features are shown in Table 2.
Train-Test Ratio | Acc (%) | Sn (%) | Sp (%) | PPV (%) | NPV (%) | FPR | FDR | FNR | F1 | MCC |
50-50 | 96.66 | 95.28 | 97.48 | 95.73 | 97.21 | 0.0252 | 0.0427 | 0.0472 | 0.9551 | 0.9285 |
60-40 | 97.72 | 97.64 | 97.76 | 96.28 | 98.59 | 0.0224 | 0.0372 | 0.0236 | 0.9696 | 0.9513 |
70-30 | 98.95 | 98.58 | 99.16 | 98.58 | 99.16 | 0.0084 | 0.0142 | 0.0142 | 0.9858 | 0.9774 |
80-20 | 99.12 | 99.06 | 99.16 | 98.59 | 99.44 | 0.0084 | 0.0141 | 0.0094 | 0.9882 | 0.9812 |
The accuracy, sensitivity, and specificity of the Intelligent Breast Cancer Diagnostic System Empowered by DEGDO were measured including classifiers like NB, SVM, K-NN, RF, and ANN. The performance results of the proposed Intelligent Breast Cancer Diagnostic System Empowered by Deep Extreme Gradient Descent Optimization are compared to the state-of-the-art methods like NB, SVM, K-NN, RF, and ANN (Figure 3).
It is found that the classification algorithm performed better with the deep extreme gradient descent optimization-based method. Besides, with the selective attributes for binary classification, the Intelligent Breast Cancer Diagnostic System empowered by DEGDO achieved a maximum accuracy of 98.73%. Apart from that, RF achieved the accuracy of 94.62% after the maximum accuracy of 98.73%. Moreover, Naive Bayes achieved an accuracy of 87.58% which sounds well but falls short of the target. SVM achieved just a 90.25% accuracy, which is better than the other algorithms like K-NN. Additionally, ANN and K-NN achieved accuracies of 85.29 and 83.81% respectively. Given these objective stats, we are justified to assert that the proposed model's accuracy got improved by using the selection of features technique. Moreover, Figure 4 illustrates a schematic comparison of the proposed DEGDO with various state-of-the-art machine learning techniques.
ROC curve generated by different classifiers used in this research is shown in the Figure 4. This figure vividly demonstrates that the proposed model rendered better results. Besides, AUC score for DEGDO, KNN, ANN, SVM, RF and NB are 0.989, 0.838, 0.867, 0.927, 0.948 and 0.876, respectively. X-axis represents the False Positive Rate (FPR) while Y-axis, the True Positive Rate (TPR).
Mean square error results for the training, testing, and validation phases measured by the number of epochs are displayed in Table 3. As the training iterations increase, a linear minimization in MSE, RMSE, and MAE is observed. Moreover, the lowest MSE is attained at 873 training epochs count for the proposed model which is recorded at 0.0569. Furthermore, in the training phase, the lowest obtained MSE was 0.0699 after 873 epochs.
Epochs Count | 0 | 100 | 200 | 300 | 400 | 500 | 600 | 700 | 800 | 873 |
Training Phase | ||||||||||
Mean Square Error (MSE) | 1.665 | 0.2849 | 0.1724 | 0.1298 | 0.1012 | 0.0999 | 0.0842 | 0.0712 | 0.0645 | 0.0599 |
Root Mean Square Error (RMSE) | 1.294 | 0.5464 | 0.4256 | 0.3652 | 0.3199 | 0.2994 | 0.2845 | 0.2689 | 0.2512 | 0.2502 |
Mean Absolute Error (MAE) | 0.943 | 0.3815 | 0.3264 | 0.3054 | 0.2814 | 0.1985 | 0.1845 | 0.1542 | 0.1725 | 0.1311 |
Testing Phase | ||||||||||
Mean Square Error (MSE) | 1.754 | 0.3031 | 0.1852 | 0.1198 | 0.1426 | 0.1287 | 0.0984 | 0.0954 | 0.07425 | 0.0699 |
Root Mean Square Error (RMSE) | 1.2854 | 0.5421 | 0.4157 | 0.3655 | 0.3451 | 0.3356 | 0.3158 | 0.2847 | 0.2485 | 0.0745 |
Mean Absolute Error (MAE) | 0.948 | 0.3548 | 0.2954 | 0.2817 | 0.2465 | 0.2258 | 0.1956 | 0.1785 | 0.1688 | 0.1465 |
Validation Phase | ||||||||||
MSE | 1.841 | 0.3514 | 0.2785 | 0.1545 | 0.1348 | 0.1254 | 0.0998 | 0.0871 | 0.0785 | 0.0721 |
Root Mean Square Error (RMSE) | 1.451 | 0.5812 | 0.5266 | 0.3863 | 0.3598 | 0.3421 | 0.3125 | 0.2706 | 0.2632 | 0.2415 |
Mean Absolute Error (MAE) | 0.987 | 0.4487 | 0.4123 | 0.3458 | 0.2785 | 0.1859 | 0.1481 | 0.1399 | 0.1302 | 0.1298 |
In terms of performance, the proposed approach has been evaluated by comparing it to previously published experimental research models. It has been proven that the proposed approach is far more accurate than the ones published in the past. Table 4 gives a comparison of the accuracies between the proposed model and the other published works.
Reference Method | Model | Accuracy Results (%)a |
[39] | Deep learning SMO, IBK | 96.19, 95.90 |
[43] | J48, Probabilistic NB | 94.20, 82.60 |
[44] | Deep learning ELU, Maxout, Tanh, ReLU Vote (NB + DT + SVM) | 96.99, 96.56, 96.27, 96.55, 96.13 |
[37] | K-SVM | 97.38 |
[48] | CSSFFS (10-FOLD), RBF Network | 98.25, 93.60 |
[70] | BIG-F | 97.10 |
[71] | DLA, EABA | 97.20 |
[72] | LDA & AE-DL | 98.27 |
[73] | DesneNet121 CNN | 98.07 |
[74] | EBL-RBFNN | 98.40 |
[75] | DL-CNN | 95.00 |
[76] | Boosting CN | 98.27 |
Proposed Model | 98.73 |
This article demonstrated a marked rise in the breast cancer detection rates. A range of performance evaluation metrics like AUC-ROC, MSE, RMSE, MAE, Acc, Sp, PPV, Sn, F-Measure, NPV, FDR, FPR, FNR, and Matthew Co-relation Co-efficient (MCC) have been employed to evaluate the proposed model for different classifiers. The proposed model's accuracy, precision, sensitivity, and specificity are much better than many of the ones published in the literature, which, of course, makes this study more pronounced. Apart from that, both split and K-Fold cross-validation have been used to evaluate the performance. Besides, we have taken 10-folds cross-validation as a benchmark for results. The proposed model's accuracy, precision, sensitivity, and specificity rates came out to be 98.73, 99.48, 99.43 and 99.60% respectively. Additionally, the proposed model achieved a 0.989 AUC score. Numerous classifiers like ANN, KNN, NB, RF, and SVM were also applied to the same dataset but the proposed method outperformed all the afore-mentioned classifiers in terms of accuracy, precision, sensitivity, and specificity.
Paradoxically, some limitations plague the proposed model. Firstly, the model is trained and validated on a small dataset. Secondly, the diagnostic process consists of multiple stages ranging from the collection of relevant features to the medical laboratory test reports to feeding it to the proposed model in CSV format which, of course, delays the entire diagnosis.
In the future, this model can be exposed to multiple datasets like TCGA or NCBI GEO databases for better results. Moreover, the fusion technique can also be used to make the proposed model more reliable. Lastly, this model can be synergized with some other feature selections methods to boost its performance.
This research received no external funding.
The authors declare there is no conflict of interest.
[1] |
E. Aličković, A. Subasi, Breast cancer diagnosis using GA feature selection and Rotation Forest, Neural Comput. Appl., 28 (2015), 753–763. https://doi.org/10.1007/s00521-015-2103-9 doi: 10.1007/s00521-015-2103-9
![]() |
[2] | World Health Organization, Breast cancer 2021, 2021. Available from: https://www.who.int/news-room/fact-sheets/detail/breast-cancer. |
[3] |
Y. S. Sun, Z. Zhao, Z. N. Yang, F. Xu, H. J. Lu, Z. Y. Zhu, et al., Risk factors and preventions of breast cancer, Int. J. Biol. Sci., 13 (2017), 1387–1397. https://doi.org/10.7150/ijbs.21635 doi: 10.7150/ijbs.21635
![]() |
[4] |
J. B. Harford, Breast-cancer early detection in low-income and middle-income countries: Do what you can versus one size fits all, Lancet Oncol., 12 (2011), 306–312. https://doi.org/10.1016/s1470-2045(10)70273-4 doi: 10.1016/s1470-2045(10)70273-4
![]() |
[5] |
C. Lerman, M. Daly, C. Sands, A. Balshem, E. Lustbader, T. Heggan, et al., Mammography adherence and psychological distress among women at risk for breast cancer, J. Natl. Cancer Inst., 85 (1993), 1074–1080. https://doi.org/10.1093/jnci/85.13.1074 doi: 10.1093/jnci/85.13.1074
![]() |
[6] |
P. T. Huynh, A. M. Jarolimek, S. Daye, The false-negative mammogram, Radiographics, 18 (1998), 1137–1154. https://doi.org/10.1148/radiographics.18.5.9747612 doi: 10.1148/radiographics.18.5.9747612
![]() |
[7] | M. G. Ertosun, D. L. Rubin, Probabilistic Visual Search for Masses within mammography images using Deep Learning, in 2015 IEEE International Conference on Bioinformatics and Biomedicine (BIBM), (2015), 1310–1315. https://doi.org/10.1109/bibm.2015.7359868 |
[8] | Y. Lu, J. Y. Li, Y. T. Su, A. A. Liu, A review of breast cancer detection in medical images, in 2018 IEEE Visual Communications and Image Processing, (2018), 1–4. https://doi.org/10.1109/vcip.2018.8698732 |
[9] |
J. Ferlay, I. Soerjomataram, R. Dikshit, S. Eser, C. Mathers, M. Rebelo, et al., Cancer incidence and mortality worldwide: Sources, methods and major patterns in Globocan 2012, Int. J. Cancer, 136 (2014), E359–E386. https://doi.org/10.1002/ijc.29210 doi: 10.1002/ijc.29210
![]() |
[10] |
N. Mao, P. Yin, Q. Wang, M. Liu, J. Dong, X. Zhang, et al., Added value of Radiomics on mammography for breast cancer diagnosis: A feasibility study, J. Am. Coll. Radiol., 16 (2019), 485–491. https://doi.org/10.1016/j.jacr.2018.09.041 doi: 10.1016/j.jacr.2018.09.041
![]() |
[11] |
H. Wang, J. Feng, Q. Bu, F. Liu, M. Zhang, Y. Ren, et al., Breast mass detection in digital mammogram based on Gestalt Psychology, J. Healthc. Eng., 2018 (2018), 1–13. https://doi.org/10.1155/2018/4015613 doi: 10.1155/2018/4015613
![]() |
[12] |
S. McGuire, World cancer report 2014, Switzerland: World Health Organization, international agency for research on cancer, Adv. Nutrit. Int. Rev., 7 (2016), 418–419. https://doi.org/10.3945/an.116.012211 doi: 10.3945/an.116.012211
![]() |
[13] |
M. K. Gupta, P. Chandra, A comprehensive survey of Data Mining, Int. J. Comput. Technol., 12 (2020), 1243–1257. https://doi.org/10.1007/s41870-020-00427-7 doi: 10.1007/s41870-020-00427-7
![]() |
[14] | T. Zou, T. Sugihara, Fast identification of a human skeleton-marker model for motion capture system using stochastic gradient descent method, in 2020 8th IEEE RAS/EMBS International Conference for Biomedical Robotics and Biomechatronics (BioRob)., (2020), 181–186. https://doi.org/10.1109/biorob49111.2020.9224442 |
[15] |
A. Reisizadeh, A. Mokhtari, H. Hassani, R. Pedarsani, An exact quantized decentralized gradient descent algorithm, IEEE Trans. Signal Process., 67 (2019), 4934–4947. https://doi.org/10.1109/tsp.2019.2932876 doi: 10.1109/tsp.2019.2932876
![]() |
[16] |
D. Maulud, A. M. Abdulazeez, A review on linear regression comprehensive in machine learning, J. Appl. Sci. Technol. Trends, 1 (2020), 140–147. https://doi.org/10.38094/jastt1457 doi: 10.38094/jastt1457
![]() |
[17] |
D. R. Wilson, T. R. Martinez, The general inefficiency of batch training for gradient descent learning, Neural Networks, 16 (2003) 1429–1451. https://doi.org/10.1016/s0893-6080(03)00138-2 doi: 10.1016/s0893-6080(03)00138-2
![]() |
[18] |
D. Yi, S. Ji, S. Bu, An enhanced optimization scheme based on gradient descent methods for machine learning, Symmetry, 11 (2019), 942. https://doi.org/10.3390/sym11070942 doi: 10.3390/sym11070942
![]() |
[19] |
D. A. Zebari, D. Q. Zeebaree, A. M. Abdulazeez, H. Haron, H. N. Hamed, Improved threshold based and trainable fully automated segmentation for breast cancer boundary and pectoral muscle in mammogram images, IEEE Access, 8 (2020), 203097–203116. https://doi.org/10.1109/access.2020.3036072 doi: 10.1109/access.2020.3036072
![]() |
[20] | D. Q. Zeebaree, H. Haron, A. M. Abdulazeez, D. A. Zebari, Trainable model based on new uniform LBP feature to identify the risk of the breast cancer, in 2019 International Conference on Advanced Science and Engineering (ICOASE), 2019. https://doi.org/10.1109/icoase.2019.8723827 |
[21] |
D. Q. Zeebaree, A. M. Abdulazeez, L. M. Abdullrhman, D. A. Hasan, O. S. Kareem, The prediction process based on deep recurrent neural networks: A Review, Asian J. Comput. Inf. Syst., 10 (2021), 29–45. https://doi.org/10.9734/ajrcos/2021/v11i230259 doi: 10.9734/ajrcos/2021/v11i230259
![]() |
[22] |
D. Q. Zeebaree, A. M. Abdulazeez, D. A. Zebari, H. Haron, H. N. A. Hamed, Multi-level fusion in ultrasound for cancer detection based on uniform LBP features, Comput. Matern. Contin., 66 (2021), 3363–3382. https://doi.org/10.32604/cmc.2021.013314 doi: 10.32604/cmc.2021.013314
![]() |
[23] |
M. Muhammad, D. Zeebaree, A. M. Brifcani, J. Saeed, D. A. Zebari, A review on region of interest segmentation based on clustering techniques for breast cancer ultrasound images, J. Appl. Sci. Technol. Trends, 1 (2020), 78–91. https://doi.org/10.38094/jastt1328 doi: 10.38094/jastt1328
![]() |
[24] |
P. Kamsing, P. Torteeka, S. Yooyen, An enhanced learning algorithm with a particle filter-based gradient descent optimizer method, Neural Comput. Appl., 32 (2020), 12789–12800. https://doi.org/10.1007/s00521-020-04726-9 doi: 10.1007/s00521-020-04726-9
![]() |
[25] |
Y. Hamid, L. Journaux, J. A. Lee, M. Sugumaran, A novel method for network intrusion detection based on nonlinear SNE and SVM, J. Artif. Intell. Soft Comput. Res., 6 (2018), 265. https://doi.org/10.1504/ijaisc.2018.097280 doi: 10.1504/ijaisc.2018.097280
![]() |
[26] | H. Sadeeq, A. M. Abdulazeez, Hardware implementation of firefly optimization algorithm using fpgas, in 2018 International Conference on Advanced Science and Engineering, (2018), 30–35. https://doi.org/10.1109/icoase.2018.8548822 |
[27] | D. P. Hapsari, I. Utoyo, S. W. Purnami, Fractional gradient descent optimizer for linear classifier support vector machine, in 2020 Third International Conference on Vocational Education and Electrical Engineering (ICVEE), (2020), 1–5. |
[28] |
M. S. Nawaz, B. Shoaib, M. A. Ashraf, Intelligent cardiovascular disease prediction empowered with gradient descent optimization, Heliyon, 7 (2021), 1–10. https://doi.org/10.1016/j.heliyon.2021.e06948 doi: 10.1016/j.heliyon.2021.e06948
![]() |
[29] |
Y. Qian, Exploration of machine algorithms based on deep learning model and feature extraction, J. Math. Biosci. Eng., 18 (2021), 7602–7618. https://doi.org/10.3934/mbe.2021376 doi: 10.3934/mbe.2021376
![]() |
[30] |
Z. Wang, M. Li, H. Wang, H. Jiang, Y. Yao, H. Zhang, et al., Breast cancer detection using extreme learning machine based on feature fusion with CNN deep features, IEEE Access, 7 (2019), 105146–105158. https://doi.org/10.1109/access.2019.2892795 doi: 10.1109/access.2019.2892795
![]() |
[31] | UCI Machine Learning Repository, Breast Cancer Wisconsin (Diagnostic) Data Set. Available from: https://archive.ics.uci.edu/ml/datasets/Breast+ Cancer + Wisconsin + (Diagnostic). |
[32] |
R. V. Anji, B. Soni, R. K. Sudheer, Breast cancer detection by leveraging machine learning, ICT Express, 6 (2020), 320–324. https://doi.org/10.1016/j.icte.2020.04.009 doi: 10.1016/j.icte.2020.04.009
![]() |
[33] |
Z. Salod, Y. Singh, Comparison of the performance of machine learning algorithms in breast cancer screening and detection: A Protocol, J. Public Health Res., 8 (2019). https://doi.org/10.4081/jphr.2019.1677 doi: 10.4081/jphr.2019.1677
![]() |
[34] |
Y. Lin, H. Luo, D. Wang, H. Guo, K. Zhu, An ensemble model based on machine learning methods and data preprocessing for short-term electric load forecasting, Energies, 10 (2017), 1186. https://doi.org/10.3390/en10081186 doi: 10.3390/en10081186
![]() |
[35] | M. Amrane, S. Oukid, I. Gagaoua, T. Ensari, Breast cancer classification using machine learning, in 2018 Electric Electronics, Computer Science, Biomedical Engineerings' Meeting (EBBT), (2018), 1–4. https://doi.org/10.1109/ebbt.2018.8391453 |
[36] |
R. Sumbaly, N. Vishnusri, S. Jeyalatha, Diagnosis of breast cancer using decision tree data mining technique, Int. J. Comput. Appl., 98 (2014), 16–24. https://doi.org/10.5120/17219-7456 doi: 10.5120/17219-7456
![]() |
[37] |
B. Zheng, S. W. Yoon, S. S. Lam, Breast cancer diagnosis based on feature extraction using a hybrid of k-means and support vector machine algorithms, Expert Syst. Appl., 41 (2014), 1476–1482. https://doi.org/10.1016/j.eswa.2013.08.044 doi: 10.1016/j.eswa.2013.08.044
![]() |
[38] |
T. Araújo, G. Aresta, E. Castro, J. Rouco, P. Aguiar, C. Eloy, et al., Classification of breast cancer histology images using convolutional neural networks, Plos One, 12 (2017), e0177544. https://doi.org/10.1371/journal.pone.0177544 doi: 10.1371/journal.pone.0177544
![]() |
[39] | S. P. Rajamohana, A. Dharani, P. Anushree, B. Santhiya, K. Umamaheswari, Machine learning techniques for healthcare applications: early autism detection using ensemble approach and breast cancer prediction using SMO and IBK, in Cognitive Social Mining Applications in Data Analytics and Forensics, (2019), 236–251. https://doi.org/10.4018/978-1-5225-7522-1.ch012 |
[40] |
L. G. Ahmad, Using three machine learning techniques for predicting breast cancer recurrence, J. Health Med. Inf., 4 (2013), 10–15. https://doi.org/10.4172/2157-7420.1000124 doi: 10.4172/2157-7420.1000124
![]() |
[41] |
B. Padmapriya, T. Velmurugan, Classification algorithm based analysis of breast cancer data, Int. J. Data Min. Tech. Appl., 5 (2016), 43–49. https://doi.org/10.20894/ijdmta.102.005.001.010 doi: 10.20894/ijdmta.102.005.001.010
![]() |
[42] | S. Bharati, M. A. Rahman, P. Podder, Breast cancer prediction applying different classification algorithm with comparative analysis using Weka, in 2018 4th International Conference on Electrical Engineering and Information & Communication Technology (ICEEiCT), (2018), 581–584. https://doi.org/10.1109/ceeict.2018.8628084 |
[43] |
K. Williams, P. A. Idowu, J. A. Balogun, A. I. Oluwaranti, Breast cancer risk prediction using data mining classification techniques, Trans. Networks Commun., 3 (2015), 17–23. https://doi.org/10.14738/tnc.32.662 doi: 10.14738/tnc.32.662
![]() |
[44] | P. Mekha, N. Teeyasuksaet, Deep learning algorithms for predicting breast cancer based on tumor cells, in 2019 Joint International Conference on Digital Arts, Media and Technology with ECTI Northern Section Conference on Electrical, Electronics, Computer and Telecommunications Engineering (ECTI DAMT-NCON), 2019. https://doi.org/10.1109/ecti-ncon.2019.8692297 |
[45] | C. Shah, A. G. Jivani, Comparison of data mining classification algorithms for breast cancer prediction, in 2013 Fourth International Conference on Computing, Communications and Networking Technologies (ICCCNT), 2013. https://doi.org/10.1109/icccnt.2013.6726477 |
[46] |
A. A. Bataineh, A comparative analysis of nonlinear machine learning algorithms for breast cancer detection, Int. J. Mach. Learn. Comput., 9 (2019), 248–254. https://doi.org/10.18178/ijmlc.2019.9.3.794 doi: 10.18178/ijmlc.2019.9.3.794
![]() |
[47] | M. S. M. Prince, A. Hasan, F. M. Shah, An efficient ensemble method for cancer detection, in 2019 1st International Conference on Advances in Science, Engineering and Robotics Technology (ICASERT), 2019. https://doi.org/10.1109/icasert.2019.8934817 |
[48] |
S. Aruna, A novel SVM based CSSFFS feature selection algorithm for Detecting Breast Cancer, Int. J. Comput., 31 (2011), 14–20. https://doi.org/10.5120/3844-5346 doi: 10.5120/3844-5346
![]() |
[49] |
G. Carneiro, J. Nascimento, A. P. Bradley, Automated analysis of unregistered Multi-View Mammograms with deep learning, IEEE Trans. Med. Imaging, 36 (2017), 2355–2365. https://doi.org/10.1109/tmi.2017.2751523 doi: 10.1109/tmi.2017.2751523
![]() |
[50] |
Z. Sha, L. Hu, B. D. Rouyendegh, Deep learning and optimization algorithms for Automatic Breast Cancer Detection, Int. J. Imaging Syst. Technol., 30 (2020), 495–506. https://doi.org/10.1002/ima.22400 doi: 10.1002/ima.22400
![]() |
[51] |
M. Mahmoud, Breast cancer classification in histopathological images using convolutional neural network, Int. J. Comput. Sci. Appl., 9 (2018), 12–15. https://doi.org/10.14569/ijacsa.2018.090310 doi: 10.14569/ijacsa.2018.090310
![]() |
[52] |
Z. Jiao, X. Gao, Y. Wang, J. Li, A deep feature based framework for Breast Masses classification, Neurocomputing, 197 (2016), 221–231. https://doi.org/10.1016/j.neucom.2016.02.060 doi: 10.1016/j.neucom.2016.02.060
![]() |
[53] |
M. H. Yap, G. Pons, J. Marti, S. Ganau, M. Sentis, R. Zwiggelaar, et al., Automated breast ultrasound lesions detection using convolutional neural networks, IEEE. J. Biomed. Health Inf., 22 (2018), 1218–1226. https://doi.org/10.1109/jbhi.2017.2731873 doi: 10.1109/jbhi.2017.2731873
![]() |
[54] |
N. Wahab, A. Khan, Y. S. Lee, Transfer learning based deep CNN for segmentation and detection of mitoses in breast cancer histopathological images, Microscopy, 68 (2019), 216–233. https://doi.org/10.1093/jmicro/dfz002 doi: 10.1093/jmicro/dfz002
![]() |
[55] |
Z. Wang, G. Yu, Y. Kang, Y. Zhao, Q. Qu, Breast tumor detection in digital mammography based on Extreme Learning Machine, Neurocomputing, 128 (2014), 175–184. https://doi.org/10.1016/j.neucom.2013.05.053 doi: 10.1016/j.neucom.2013.05.053
![]() |
[56] |
Y. Qiu, Y. Wang, S. Yan, M. Tan, S. Cheng, H. Liu, et al., An initial investigation on developing a new method to predict short-term breast cancer risk based on Deep Learning Technology, Comput. Aided. Des., 2016. https://doi.org/10.1117/12.2216275 doi: 10.1117/12.2216275
![]() |
[57] |
X. W. Chen, X. Lin, Big data deep learning: Challenges and perspectives, IEEE Access, 2 (2014), 514–525. https://doi.org/10.1109/access.2014.2325029 doi: 10.1109/access.2014.2325029
![]() |
[58] |
J. Arevalo, F. A. González, R. R. Pollán, J. L. Oliveira, M. A. G. Lopez, Representation learning for mammography mass lesion classification with convolutional neural networks, Comput. Methods Programs Biomed., 127 (2016), 248–257. https://doi.org/10.1016/j.cmpb.2015.12.014 doi: 10.1016/j.cmpb.2015.12.014
![]() |
[59] |
Y. Kumar, A. Aggarwal, S. Tiwari, K. Singh, An efficient and robust approach for biomedical image retrieval using zernike moments, Biomed. Signal Process. Control, 39 (2018), 459–473. https://doi.org/10.1016/j.bspc.2017.08.018 doi: 10.1016/j.bspc.2017.08.018
![]() |
[60] |
K. Kalaiarasi, R. Soundaria, N. Kausar, P. Agarwal, H. Aydi, H. Alsamir, Optimization of the average monthly cost of an EOQ inventory model for deteriorating items in machine learning using Python, Therm. Sci., 25 (2021), 347–358. https://doi.org/10.2298/tsci21s2347k doi: 10.2298/tsci21s2347k
![]() |
[61] |
M. Franulović, K. Marković, A. Trajkovski, Calibration of material models for the human cervical spine ligament behaviour using a genetic algorithm, Facta Univ., Series: Mechan. Eng., 19 (2021) 751. https://doi.org/10.22190/fume201029023f doi: 10.22190/fume201029023f
![]() |
[62] |
M. Fayaz, D. H. Kim, A prediction methodology of energy consumption based on Deep Extreme Learning Machine and comparative analysis in residential buildings, Electronics, 7 (2018), 222. https://doi.org/10.3390/electronics7100222 doi: 10.3390/electronics7100222
![]() |
[63] |
G. B. Huang, D. H. Wang, Y. Lan, Extreme learning machines: A survey, Int. J. Mach. Learn. Cybern., 2 (2011), 107–122. https://doi.org/10.1007/s13042-011-0019-y doi: 10.1007/s13042-011-0019-y
![]() |
[64] |
H. Tang, S. Gao, L. Wang, X. Li, B. Li, S. Pang, A novel intelligent fault diagnosis method for rolling bearings based on Wasserstein generative adversarial network and Convolutional Neural Network under Unbalanced Dataset, Sensors, 21 (2021), 6754. https://doi.org/10.3390/s21206754 doi: 10.3390/s21206754
![]() |
[65] |
J. Wei, H. Liu, G. Yan, F. Sun, Multi-modal deep extreme learning machine for robotic grasping recognition, Proceed. Adapt., Learn. Optim., (2016), 223–233. https://doi.org/10.1007/978-3-319-28373-9_19 doi: 10.1007/978-3-319-28373-9_19
![]() |
[66] |
N. S. Naz, M. A. Khan, S. Abbas, A. Ather, S. Saqib, Intelligent routing between capsules empowered with deep extreme machine learning technique, SN Appl. Sci., 2 (2019), 1–14. https://doi.org/10.1007/s42452-019-1873-6 doi: 10.1007/s42452-019-1873-6
![]() |
[67] |
J. Cai, J. Luo, S. Wang, S. Yang, Feature selection in Machine Learning: A new perspective, Neurocomputing, 300 (2018), 70–79. https://doi.org/10.1016/j.neucom.2017.11.077 doi: 10.1016/j.neucom.2017.11.077
![]() |
[68] |
L. M. Abualigah, A. T. Khader, E. S. Hanandeh, A new feature selection method to improve the document clustering using particle swarm optimization algorithm, J. Comput. Sci., 25 (2018), 456–466. https://doi.org/10.1016/j.jocs.2017.07.018 doi: 10.1016/j.jocs.2017.07.018
![]() |
[69] |
P. A. Flach, ROC analysis, encyclopedia of machine learning and data mining, Encycl. Mach. Learn. Data Min., (2016), 1–8. https://doi.org/10.1007/978-1-4899-7502-7_739-1 doi: 10.1007/978-1-4899-7502-7_739-1
![]() |
[70] |
Q. Wuniri, W. Huangfu, Y. Liu, X. Lin, L. Liu, Z. Yu, A generic-driven wrapper embedded with feature-type-aware hybrid bayesian classifier for breast cancer classification, IEEE Access, 7 (2019), 119931–119942. https://doi.org/10.1109/access.2019.2932505 doi: 10.1109/access.2019.2932505
![]() |
[71] |
J. Zheng, D. Lin, Z. Gao, S. Wang, M. He, J. Fan, Deep Learning assisted efficient ADABOOST algorithm for breast cancer detection and early diagnosis, IEEE Access, 8 (2020), 96946–96954. https://doi.org/10.1109/access.2020.2993536 doi: 10.1109/access.2020.2993536
![]() |
[72] |
X. Zhang, D. He, Y. Zheng, H. Huo, S. Li, R. Chai, et al., Deep learning based analysis of breast cancer using advanced ensemble classifier and linear discriminant analysis, IEEE Access, 8 (2020), 120208–120217. https://doi.org/10.1109/access.2020.3005228 doi: 10.1109/access.2020.3005228
![]() |
[73] |
Y. Yari, T. V. Nguyen, H. T. Nguyen, Deep learning applied for histological diagnosis of breast cancer, IEEE Access, 8 (2020), 162432–162448. https://doi.org/10.1109/access.2020.3021557 doi: 10.1109/access.2020.3021557
![]() |
[74] |
A. H. Osman, H. M. Aljahdali, An effective of ensemble boosting learning method for breast cancer virtual screening using neural network model, IEEE Access, 8 (2020), 39165–39174. https://doi.org/10.1109/access.2020.2976149 doi: 10.1109/access.2020.2976149
![]() |
[75] |
Y. Li, J. Wu, Q. Wu, Classification of breast cancer histology images using multi-size and discriminative patches based on Deep Learning, IEEE Access, 7 (2019), 21400–21408. https://doi.org/10.1109/access.2019.2898044 doi: 10.1109/access.2019.2898044
![]() |
[76] |
D. M. Vo, N. Q. Nguyen, S. W. Lee, Classification of breast cancer histology images using incremental boosting convolution networks, Inf. Sci., 482 (2019), 123–138. https://doi.org/10.1016/j.ins.2018.12.089 doi: 10.1016/j.ins.2018.12.089
![]() |
[77] |
S. Y. Siddiqui, M. A. Khan, S. Abbas, F. Khan, Smart occupancy detection for road traffic parking using deep extreme learning machine, J. K.S.U. Comput. Inf. Sci., 34 (2022), 727–733. https://doi.org/10.1016/j.jksuci.2020.01.016 doi: 10.1016/j.jksuci.2020.01.016
![]() |
[78] |
M. A. Khan, S. Abbas, K. M. Khan, M. A. A. Ghamdi, A. Rehman, Intelligent forecasting model of covid-19 novel coronavirus outbreak empowered with deep extreme learning machine, Comput. Matern. Contin., 64 (2020), 1329–1342. https://doi.org/10.32604/cmc.2020.011155 doi: 10.32604/cmc.2020.011155
![]() |
[79] |
S. Abbas, M. A. Khan, L. E. F. Morales, A. Rehman, Y. Saeed, Modelling, simulation and optimization of power plant energy sustainability for IoT enabled smart cities empowered with deep extreme learning machine, IEEE Access, 8 (2020), 39982–39997. https://doi.org/10.1109/ACCESS.2020.2976452 doi: 10.1109/ACCESS.2020.2976452
![]() |
[80] |
A. Rehman, A. Athar, M. A. Khan, S. Abbas, A. Fatima, M. Zareei, et al., Modelling, simulation, and optimization of diabetes type ii prediction using deep extreme learning machine, J. Ambient Intell. Smart Environ., 12 (2020), 125–138. https://doi.org/10.3233/AIS-200554 doi: 10.3233/AIS-200554
![]() |
[81] |
A. Haider, M. A. Khan, A. Rehman, H. S. Kim, A real-time sequential deep extreme learning machine cybersecurity intrusion detection system, Comput. Matern. Contin., 66 (2021), 1785–1798. https://doi.org/10.32604/cmc.2020.013910 doi: 10.32604/cmc.2020.013910
![]() |
[82] |
M. A. Khan, A. Rehman, K. M. Khan, M. A. A. Ghamdi, S. H. Almotiri, Enhance intrusion detection in computer networks based on deep extreme learning machine, Comput. Matern. Contin., 66 (2021), 467–480. https://doi.org/10.32604/cmc.2020.013121 doi: 10.32604/cmc.2020.013121
![]() |
[83] |
U. Ahmed, G. F. Issa, M. A. Khan, S. Aftab, M. F. Khan, R. A. T. Said, et al., Prediction of diabetes empowered with fused machine learning, IEEE Access, 10 (2022), 8529–8538. https://doi.org/10.1109/ACCESS.2022.3142097 doi: 10.1109/ACCESS.2022.3142097
![]() |
[84] |
S. Y. Siddiqui, A. Haider, T. M. Ghazal, M. A. Khan, I. Naseer, S. Abbas, et al., IoMT cloud-based intelligent prediction of breast cancer stages empowered with deep learning, IEEE Access, 9 (2021), 146478–146491. https://doi.org/10.1109/ACCESS.2021.3123472 doi: 10.1109/ACCESS.2021.3123472
![]() |
[85] |
M. Ahmad, M. Alfayad, S. Aftab, M. A. Khan, A. Fatima, B. Shoaib, et.al., Data and machine learning fusion architecture for cardiovascular disease prediction, Comput. Matern. Contin., 69 (2021), 2717–2731. https://doi.org/10.32604/cmc.2021.019013 doi: 10.32604/cmc.2021.019013
![]() |
1. | Jia Li, Jingwen Shi, Jianrong Chen, Ziqi Du, Li Huang, Self-attention random forest for breast cancer image classification, 2023, 13, 2234-943X, 10.3389/fonc.2023.1043463 | |
2. | Mpho Mokoatle, Vukosi Marivate, Darlington Mapiye, Riana Bornman, Vanessa. M. Hayes, A review and comparative study of cancer detection using machine learning: SBERT and SimCSE application, 2023, 24, 1471-2105, 10.1186/s12859-023-05235-x | |
3. | Ramdas Kapila, Sumalatha Saleti, An efficient ensemble-based Machine Learning for breast cancer detection, 2023, 86, 17468094, 105269, 10.1016/j.bspc.2023.105269 | |
4. | Mana Saleh Al Reshan, Samina Amin, Muhammad Ali Zeb, Adel Sulaiman, Hani Alshahrani, Ahmad Taher Azar, Asadullah Shaikh, Enhancing Breast Cancer Detection and Classification Using Advanced Multi-Model Features and Ensemble Machine Learning Techniques, 2023, 13, 2075-1729, 2093, 10.3390/life13102093 | |
5. | Hari Mohan Rai, Serhii Dashkevych, Joon Yoo, Next-Generation Diagnostics: The Impact of Synthetic Data Generation on the Detection of Breast Cancer from Ultrasound Imaging, 2024, 12, 2227-7390, 2808, 10.3390/math12182808 | |
6. | Hari Mohan Rai, Joon Yoo, A comprehensive analysis of recent advancements in cancer detection using machine learning and deep learning models for improved diagnostics, 2023, 149, 0171-5216, 14365, 10.1007/s00432-023-05216-w | |
7. | Hari Mohan Rai, Cancer detection and segmentation using machine learning and deep learning techniques: a review, 2023, 83, 1573-7721, 27001, 10.1007/s11042-023-16520-5 | |
8. | Hari Mohan Rai, Joon Yoo, Saurabh Agarwal, The Improved Network Intrusion Detection Techniques Using the Feature Engineering Approach with Boosting Classifiers, 2024, 12, 2227-7390, 3909, 10.3390/math12243909 | |
9. | Hari Mohan Rai, Joon Yoo, Serhii Dashkevych, Transformative Advances in AI for Precise Cancer Detection: A Comprehensive Review of Non-Invasive Techniques, 2025, 1134-3060, 10.1007/s11831-024-10219-y |
Sr. No. | Attributes | Symbol | Type |
1 | Mean of the concave sections of the contour's severity | concavity_mean | Numeric |
2 | The average of distances between the center and the peripheral points | radius_mean | Numeric |
3 | The mean value for the severity of concave sections of the contour that is the worst or the greatest | concavity_worst | Numeric |
4 | Area | area_se | Numeric |
5 | Gray-scale standard deviation | Texture | Numeric |
6 | Worst symmetry | symmetry_worst | Numeric |
7 | arithmetic mean of the regional variance in radius lengths | smoothness_mean | Numeric |
8 | The standard error for the severity of concave contour segments | concavity_se | Numeric |
9 | The mean value that is the worst or the greatest for local variance in radius lengths | smoothness_worst | Numeric |
10 | The worst or biggest number for the mean of "coastline approximation"-1 | fractal_dimension_worst | Numeric |
11 | The standard error for approximating the coastline-1 | fractal_dimension_se | Numeric |
12 | Symmetry mean | symmetry_ mean | Numeric |
13 | arithmetic mean for "coastline approximation"-1 | fractal_dimension_mean | Numeric |
14 | Symmetry se | symmetry_se | Numeric |
15 | standard inaccuracy in radius lengths due to local variation | smoothness_se | Numeric |
16 | standard inaccuracy for the standard deviation of gray-scale values | texture_se | Numeric |
Train-Test Ratio | Acc (%) | Sn (%) | Sp (%) | PPV (%) | NPV (%) | FPR | FDR | FNR | F1 | MCC |
50-50 | 96.66 | 95.28 | 97.48 | 95.73 | 97.21 | 0.0252 | 0.0427 | 0.0472 | 0.9551 | 0.9285 |
60-40 | 97.72 | 97.64 | 97.76 | 96.28 | 98.59 | 0.0224 | 0.0372 | 0.0236 | 0.9696 | 0.9513 |
70-30 | 98.95 | 98.58 | 99.16 | 98.58 | 99.16 | 0.0084 | 0.0142 | 0.0142 | 0.9858 | 0.9774 |
80-20 | 99.12 | 99.06 | 99.16 | 98.59 | 99.44 | 0.0084 | 0.0141 | 0.0094 | 0.9882 | 0.9812 |
Epochs Count | 0 | 100 | 200 | 300 | 400 | 500 | 600 | 700 | 800 | 873 |
Training Phase | ||||||||||
Mean Square Error (MSE) | 1.665 | 0.2849 | 0.1724 | 0.1298 | 0.1012 | 0.0999 | 0.0842 | 0.0712 | 0.0645 | 0.0599 |
Root Mean Square Error (RMSE) | 1.294 | 0.5464 | 0.4256 | 0.3652 | 0.3199 | 0.2994 | 0.2845 | 0.2689 | 0.2512 | 0.2502 |
Mean Absolute Error (MAE) | 0.943 | 0.3815 | 0.3264 | 0.3054 | 0.2814 | 0.1985 | 0.1845 | 0.1542 | 0.1725 | 0.1311 |
Testing Phase | ||||||||||
Mean Square Error (MSE) | 1.754 | 0.3031 | 0.1852 | 0.1198 | 0.1426 | 0.1287 | 0.0984 | 0.0954 | 0.07425 | 0.0699 |
Root Mean Square Error (RMSE) | 1.2854 | 0.5421 | 0.4157 | 0.3655 | 0.3451 | 0.3356 | 0.3158 | 0.2847 | 0.2485 | 0.0745 |
Mean Absolute Error (MAE) | 0.948 | 0.3548 | 0.2954 | 0.2817 | 0.2465 | 0.2258 | 0.1956 | 0.1785 | 0.1688 | 0.1465 |
Validation Phase | ||||||||||
MSE | 1.841 | 0.3514 | 0.2785 | 0.1545 | 0.1348 | 0.1254 | 0.0998 | 0.0871 | 0.0785 | 0.0721 |
Root Mean Square Error (RMSE) | 1.451 | 0.5812 | 0.5266 | 0.3863 | 0.3598 | 0.3421 | 0.3125 | 0.2706 | 0.2632 | 0.2415 |
Mean Absolute Error (MAE) | 0.987 | 0.4487 | 0.4123 | 0.3458 | 0.2785 | 0.1859 | 0.1481 | 0.1399 | 0.1302 | 0.1298 |
Reference Method | Model | Accuracy Results (%)a |
[39] | Deep learning SMO, IBK | 96.19, 95.90 |
[43] | J48, Probabilistic NB | 94.20, 82.60 |
[44] | Deep learning ELU, Maxout, Tanh, ReLU Vote (NB + DT + SVM) | 96.99, 96.56, 96.27, 96.55, 96.13 |
[37] | K-SVM | 97.38 |
[48] | CSSFFS (10-FOLD), RBF Network | 98.25, 93.60 |
[70] | BIG-F | 97.10 |
[71] | DLA, EABA | 97.20 |
[72] | LDA & AE-DL | 98.27 |
[73] | DesneNet121 CNN | 98.07 |
[74] | EBL-RBFNN | 98.40 |
[75] | DL-CNN | 95.00 |
[76] | Boosting CN | 98.27 |
Proposed Model | 98.73 |
Sr. No. | Attributes | Symbol | Type |
1 | Mean of the concave sections of the contour's severity | concavity_mean | Numeric |
2 | The average of distances between the center and the peripheral points | radius_mean | Numeric |
3 | The mean value for the severity of concave sections of the contour that is the worst or the greatest | concavity_worst | Numeric |
4 | Area | area_se | Numeric |
5 | Gray-scale standard deviation | Texture | Numeric |
6 | Worst symmetry | symmetry_worst | Numeric |
7 | arithmetic mean of the regional variance in radius lengths | smoothness_mean | Numeric |
8 | The standard error for the severity of concave contour segments | concavity_se | Numeric |
9 | The mean value that is the worst or the greatest for local variance in radius lengths | smoothness_worst | Numeric |
10 | The worst or biggest number for the mean of "coastline approximation"-1 | fractal_dimension_worst | Numeric |
11 | The standard error for approximating the coastline-1 | fractal_dimension_se | Numeric |
12 | Symmetry mean | symmetry_ mean | Numeric |
13 | arithmetic mean for "coastline approximation"-1 | fractal_dimension_mean | Numeric |
14 | Symmetry se | symmetry_se | Numeric |
15 | standard inaccuracy in radius lengths due to local variation | smoothness_se | Numeric |
16 | standard inaccuracy for the standard deviation of gray-scale values | texture_se | Numeric |
Train-Test Ratio | Acc (%) | Sn (%) | Sp (%) | PPV (%) | NPV (%) | FPR | FDR | FNR | F1 | MCC |
50-50 | 96.66 | 95.28 | 97.48 | 95.73 | 97.21 | 0.0252 | 0.0427 | 0.0472 | 0.9551 | 0.9285 |
60-40 | 97.72 | 97.64 | 97.76 | 96.28 | 98.59 | 0.0224 | 0.0372 | 0.0236 | 0.9696 | 0.9513 |
70-30 | 98.95 | 98.58 | 99.16 | 98.58 | 99.16 | 0.0084 | 0.0142 | 0.0142 | 0.9858 | 0.9774 |
80-20 | 99.12 | 99.06 | 99.16 | 98.59 | 99.44 | 0.0084 | 0.0141 | 0.0094 | 0.9882 | 0.9812 |
Epochs Count | 0 | 100 | 200 | 300 | 400 | 500 | 600 | 700 | 800 | 873 |
Training Phase | ||||||||||
Mean Square Error (MSE) | 1.665 | 0.2849 | 0.1724 | 0.1298 | 0.1012 | 0.0999 | 0.0842 | 0.0712 | 0.0645 | 0.0599 |
Root Mean Square Error (RMSE) | 1.294 | 0.5464 | 0.4256 | 0.3652 | 0.3199 | 0.2994 | 0.2845 | 0.2689 | 0.2512 | 0.2502 |
Mean Absolute Error (MAE) | 0.943 | 0.3815 | 0.3264 | 0.3054 | 0.2814 | 0.1985 | 0.1845 | 0.1542 | 0.1725 | 0.1311 |
Testing Phase | ||||||||||
Mean Square Error (MSE) | 1.754 | 0.3031 | 0.1852 | 0.1198 | 0.1426 | 0.1287 | 0.0984 | 0.0954 | 0.07425 | 0.0699 |
Root Mean Square Error (RMSE) | 1.2854 | 0.5421 | 0.4157 | 0.3655 | 0.3451 | 0.3356 | 0.3158 | 0.2847 | 0.2485 | 0.0745 |
Mean Absolute Error (MAE) | 0.948 | 0.3548 | 0.2954 | 0.2817 | 0.2465 | 0.2258 | 0.1956 | 0.1785 | 0.1688 | 0.1465 |
Validation Phase | ||||||||||
MSE | 1.841 | 0.3514 | 0.2785 | 0.1545 | 0.1348 | 0.1254 | 0.0998 | 0.0871 | 0.0785 | 0.0721 |
Root Mean Square Error (RMSE) | 1.451 | 0.5812 | 0.5266 | 0.3863 | 0.3598 | 0.3421 | 0.3125 | 0.2706 | 0.2632 | 0.2415 |
Mean Absolute Error (MAE) | 0.987 | 0.4487 | 0.4123 | 0.3458 | 0.2785 | 0.1859 | 0.1481 | 0.1399 | 0.1302 | 0.1298 |
Reference Method | Model | Accuracy Results (%)a |
[39] | Deep learning SMO, IBK | 96.19, 95.90 |
[43] | J48, Probabilistic NB | 94.20, 82.60 |
[44] | Deep learning ELU, Maxout, Tanh, ReLU Vote (NB + DT + SVM) | 96.99, 96.56, 96.27, 96.55, 96.13 |
[37] | K-SVM | 97.38 |
[48] | CSSFFS (10-FOLD), RBF Network | 98.25, 93.60 |
[70] | BIG-F | 97.10 |
[71] | DLA, EABA | 97.20 |
[72] | LDA & AE-DL | 98.27 |
[73] | DesneNet121 CNN | 98.07 |
[74] | EBL-RBFNN | 98.40 |
[75] | DL-CNN | 95.00 |
[76] | Boosting CN | 98.27 |
Proposed Model | 98.73 |