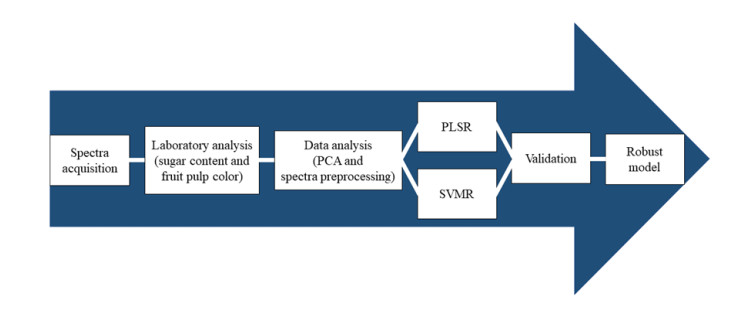
The development of the global model is an important part of research involving the quality prediction of agricultural commodities using visible/near-infrared (Vis/NIR) spectroscopy due to its efficiency and effectiveness. The Vis/NIR was used in this study to develop a global model and to evaluate the sugar content and pulp color, which are the main determinants of ripeness and quality of melons. Furthermore, it also provides a comparison between linear and nonlinear regression using partial least squares regression (PLSR) and support vector machine regression (SVMR), respectively. The model accuracy was determined by ratio of performance to deviation (RPD). The results showed that there were good model accuracy values in some parameters, such as SSC (2.14), glucose (1.59), sucrose (2.31), a* (2.97), and b* (2.49), while the fructose (1.35) and L* (1.06) modeling showed poor prediction accuracy. The best model for SSC was developed using PLSR, while that of fructose, glucose, sucrose, L*, a*, and b* were obtained from SVMR. Therefore, Vis/NIR spectroscopy can be used as an alternative method to monitor sugar content and pulp color of a melon, but with some limitations, such as the low accuracy in predicting certain variables, such as the L* and fructose.
Citation: Kusumiyati Kusumiyati, Yuda Hadiwijaya, Wawan Sutari, Agus Arip Munawar. Global model for in-field monitoring of sugar content and color of melon pulp with comparative regression approach[J]. AIMS Agriculture and Food, 2022, 7(2): 312-325. doi: 10.3934/agrfood.2022020
[1] | Nur Laylah, S. Salengke, Amran Laga, Supratomo Supratomo . Effects of the maturity level and pod conditioning period of cocoa pods on the changes of physicochemical properties of the beans of Sulawesi 2 (S2) cocoa clone. AIMS Agriculture and Food, 2023, 8(2): 615-636. doi: 10.3934/agrfood.2023034 |
[2] | Sirajuddin, Geeta Chauhan, Pramod K Nanda, Annada Das, Serlene Tomar, Arun K Das . Low-calorie and carrot pulp incorporated shrikhand from low-fat cow milk: Optimization and quality evaluation. AIMS Agriculture and Food, 2024, 9(1): 220-236. doi: 10.3934/agrfood.2024013 |
[3] | Saima Latif, Muhammad Sohaib, Sanaullah Iqbal, Muhammad Hassan Mushtaq, Muhammad Tauseef Sultan . Comparative evaluation of nutritional composition, phytochemicals and sensorial attributes of lyophilized vs conventionally dried Grewia asiatica fruit pulp powder. AIMS Agriculture and Food, 2025, 10(1): 247-265. doi: 10.3934/agrfood.2025013 |
[4] | Cíntia Sorane Good Kitzberger, David Pot, Pierre Marraccini, Luiz Filipe Protasio Pereira, Maria Brígida dos Santos Scholz . Flavor precursors and sensory attributes of coffee submitted to different post-harvest processing. AIMS Agriculture and Food, 2020, 5(4): 700-714. doi: 10.3934/agrfood.2020.4.700 |
[5] | Shahindokht Bassiri-Jahromi, Aida Doostkam . Comparative evaluation of bioactive compounds of various cultivars of pomegranate (Punica granatum) in different world regions. AIMS Agriculture and Food, 2019, 4(1): 41-55. doi: 10.3934/agrfood.2019.1.41 |
[6] | Stefano Puccio, Anna Perrone, Giuseppe Sortino, Giuseppe Gianguzzi, Carla Gentile, Vittorio Farina . Yield, pomological characteristics, bioactive compounds and antioxidant activity of Annona cherimola Mill. grown in mediterranean climate. AIMS Agriculture and Food, 2019, 4(3): 592-603. doi: 10.3934/agrfood.2019.3.592 |
[7] | Marcelo Augusto de Carvalho, Cíntia Sorane Good Kitzberger, Altamara Viviane de Souza Sartori, Marta de Toledo Benassi, Maria Brígida dos Santos Scholz, Clandio Medeiros da Silva . Free choice profiling sensory analysis and principal component analysis as tools to support an apple breeding program. AIMS Agriculture and Food, 2020, 5(4): 769-784. doi: 10.3934/agrfood.2020.4.769 |
[8] | Sapta Raharja, Yogi Purna Rahardjo, Samsudin, Khaswar Syamsu . Aroma precursor enhancing in dried cocoa beans fermentation using enzyme and heat addition. AIMS Agriculture and Food, 2023, 8(2): 674-686. doi: 10.3934/agrfood.2023037 |
[9] | Naima Belguedj, Ghayth Rigane, Ridha Ben Salem, Khodir Madani . Conventional and eco-friendly aqueous extraction methods of date palm fruit compounds: Optimization, comparison, characterization of the date pulp extract and value-added potential. AIMS Agriculture and Food, 2025, 10(1): 218-246. doi: 10.3934/agrfood.2025012 |
[10] | Heni Purwaningsih, Kristamtini, Pamudji Rahardjo, Bangun Prajanto Nusantoro, Erni Apriyati, Siti Dewi Indrasari, Rubiyo . The effect of different storage times and methods on the chemical and organoleptic properties of white cooked rice and black cooked rice. AIMS Agriculture and Food, 2022, 7(1): 184-196. doi: 10.3934/agrfood.2022012 |
The development of the global model is an important part of research involving the quality prediction of agricultural commodities using visible/near-infrared (Vis/NIR) spectroscopy due to its efficiency and effectiveness. The Vis/NIR was used in this study to develop a global model and to evaluate the sugar content and pulp color, which are the main determinants of ripeness and quality of melons. Furthermore, it also provides a comparison between linear and nonlinear regression using partial least squares regression (PLSR) and support vector machine regression (SVMR), respectively. The model accuracy was determined by ratio of performance to deviation (RPD). The results showed that there were good model accuracy values in some parameters, such as SSC (2.14), glucose (1.59), sucrose (2.31), a* (2.97), and b* (2.49), while the fructose (1.35) and L* (1.06) modeling showed poor prediction accuracy. The best model for SSC was developed using PLSR, while that of fructose, glucose, sucrose, L*, a*, and b* were obtained from SVMR. Therefore, Vis/NIR spectroscopy can be used as an alternative method to monitor sugar content and pulp color of a melon, but with some limitations, such as the low accuracy in predicting certain variables, such as the L* and fructose.
Melon is one of the most popular fruits in the world, which is widely cultivated in tropical and subtropical countries. Furthermore, it is a non-climacteric fruit since its ripening process only occurs while the fruit is still on the tree. Melon does not ripen after harvesting unlike what is seen in other fruits, such as bananas or papayas. Subsequently, ripeness has been found to be the best measure of the quality of most fruit commodities in comparison to other external properties, such as the peel shape or color, which cannot ascertain the sugar content based on the soluble solids content (SSC), fructose, glucose, and sucrose. Traditional methods for determining the SSC, fructose, glucose, and sucrose in melons are destructive, time-consuming, and unsuitable for large-scale data acquisition or field use to assess the internal quality of the fruit on a tree in real-time.
The potential development of non-destructive methods could enable the rapid and repeated analysis of large quantities on fruit samples for a variety of purposes. Various studies have been reported regarding the use of this non-destructive technology to predict water content and SSC of various commodities in the Cucurbitaceae family [1,2,3]. Additionally, this technology could be potentially used for concurrent collection of data based on different quality indices to allow the development of postharvest protocol and to separate the fruits based on internal quality [4,5,6,7].
According to the various types of technology developed in the last few decades, visible/near-infrared (Vis/NIR) spectroscopy is a promising non-destructive method for determining quality standards in agricultural products [8]. The main focus of Vis/NIR development is the prediction and monitoring of various internal quality traits in fruits while on the tree or after harvesting [9]. Furthermore, this technology was selected to determine the sugar content in melons by the presence of important wavelengths in the visible/near-infrared region since it is safe, fast, and non-destructive. The implementation of Vis/NIR in the field allows farmers to determine the quality of fruits in trees, which eases the decision making before harvest time. Also, this procedure can be used to sort large samples in order to improve efficiency and make decisions in real-time on each distribution chain [10].
Several studies on the development of the Vis/NIR model for different varieties of single fruit species has shown that this method increases the robustness and accuracy of the developed calibration model [11,12]. Additionally, it is more preferred to use this global model in real-time since its development involves the determination of the quality attributes of melons using various varieties. The development of a single Vis/NIR model is intriguing due to the various melon varieties in Indonesia. Therefore, this study aims to (1) develop a global Vis/NIR model for predicting the sugar content and pulp color in 3 melon varieties; and (2) to apply two different chemometric approaches, which includes the partial least squares regression (PLSR) and the support vector machine regression (SVMR) as a linear regression and non-linear regression method, respectively, in order to obtain an accurate Vis/NIR model. There has been no comparative study of the two methods for monitoring the quality of melon pulps with thick pulp, even though these two methods were used to predict the quality attributes of agricultural products.
The sample used in this study consisted of three melon varieties in order to produce a reliable and accurate global regression model. A total of 135 melons were used, with 45 samples from each variety, such as Linius, Ignazio, and Dalmatian melon varieties, which were harvested from hydroponic gardens in Garut, Indonesia. A total of two-thirds of the total sample was used for calibration model development (90 samples), while the remaining third was used for the prediction set (45 samples) to test the previously developed calibration model. The 90 samples of the calibration set were selected from 30 samples of each variety, whereas the prediction set picked an amount of 15 samples per variety.
The handheld NirVana AG410 (Integrated Spectronics Pty, Ltd, Australia) was used to acquire Vis-NIR spectra with a wavelength range of 381-1065 nm and spectral sampling intervals of 3 nm. The absorbance spectra of 135 whole melon samples were acquired during the acquisition using 6 data points for each. The melon sample spectrum was calculated by averaging the values of six data collection points on the same fruit. Each fruit was numbered and labelled at the six spectral collection points immediately following the acquisition of the spectra for each variety. A Vis/NIR spectrometer was used to scan the fruit parts, which were then used to collect reference data (sugar content and color). To minimize the effect of temperature variations on the performance of the resulting model, all spectra acquisition and reference analysis were performed on the same day at a temperature of 27 ℃ (room temperature).
The pulp color reference data was measured after the sample has been destructed. L*, a*, and b* values were used to describe the pulp color and were measured with a CM-600d spectrophotometer (Konica Minolta, INC, Japan). L* denotes the brightness value, a* denotes the green-red color gradation, and b* denotes the blue-yellow color gradation. At a later stage, the mean value of the six measurements in each sample was used as reference data for modelling.
The fruit was peeled, cut into small pieces, sliced and blended for 5 minutes on high speed to form a 15 mL sample of melon juice, which was then transferred to a centrifuge test tube. It was also centrifuged for 7 minutes at 4000 rpm. The centrifuge supernatant was dropped on the refractometer to obtain the sample's SSC value, which was expressed as a percentage Brix [13]. Each sample was measured three times, and the average of the three measurements was calculated. The fructose, glucose, and sucrose content were determined using high-performance liquid chromatography (Shimadzu, LC 20AT Prominence, Japan) and the procedure described by Damayanti et al. [14] and Saad et al. [15].
The calibration model was created using Vis/NIR spectra and reference data on seven quality attributes: SSC, fructose, glucose, sucrose, L*, a*, and b*. The Unscrambler X 10.4 (Camo Software AS, Oslo, Norway) was used for data analysis, including spectra preprocessing and model development. To improve the calibration model's accuracy, the original spectra were preprocessed using several methods, including standard normal variate (SNV), multiplicative scatter correction (MSC), and second derivative Savitzky-Golay (dg2). In general, preprocessing spectra help detect and correct anomalous spectra in calibration datasets, so the developed model is more accurate. SNV and MSC are popular preprocessing methods for normalizing spectra. Both methods are used to reduce the light scattering and additive effects. SNV eliminates the multiplicative effect by centering and scaling of the original individual spectra, while MSC requires the reference spectrum that is free of scattering effects. The reference spectrum is usually employed from the average of sample data. Lastly, dg2 is effective to reduce peak overlap and linear baseline slope variations. In spectroscopic analysis, dg2 is mostly run to detect the quality attributes with low concentration. Figure 1 depicts an overview of the Vis/NIR modelling procedure. The best model for each quality attribute is selected based on the highest coefficient of determination calculation on calibration set (R2cal) and prediction set (R2pred), as well as the lowest root mean squares error of calibration set (RMSEC) and root mean squares error of prediction set (RMSEP). The results of calibration modelling involving partial least squares regression (PLSR) and support vector machine regression (SVMR), which act as chemometric algorithms in model development, yield R2cal and RMSEC. R2pred and RMSEP, on the other hand, are obtained by applying the regression equation derived from the calibration model development, which is then used to predict unknown samples. The ratio of performance to deviation (RPD) is calculated to aid in the selection of the best predictive model. RPD is calculated by dividing the standard deviation ratio in the prediction set by the RMSEP. The recommended RPD for agricultural applications is greater than 3, an RPD value between 2-3 indicates good predictive ability, 1.5-2 indicates moderate predictive ability but still needs improvement, and less than 1.5 indicates poor predictive ability [16,17].
The results of laboratory measurements related to the quality of the three melon varieties are shown in Table 1. Dalmatian had the highest mean sugar content, including SSC, fructose, glucose, and sucrose, followed by Ignazio and Linius (Table 1). Additionally, the three varieties had different color values (L*, a*, and b*). This is due to the orange pulp of the Linius melon, the white pulp of the Ignazio melon, and the green pulp of the Dalmatian melon. Because the three varieties were combined during the modelling stage, each quality attribute has a wide range, namely SSC (2.70-12.60%), fructose (1.81-7.02%), glucose (1.68-12.13%), sucrose (0.02-15.84%), L* (29.85-74.68), a* (−7.70-27.27), and b* (9.57-46.42). Furthermore, this study aimed to create a global model that can be applied to a wide range of melon varieties. Therefore, a wide range of data on quality attributes certainly aids in a better modelling process [18,19].
Quality attributes | Variety | Minimum | Maximum | Mean | Standard deviation |
SSC (%) | Linius | 2.70 | 8.80 | 4.74 | 1.21 |
Ignazio | 7.00 | 10.90 | 8.56 | 1.00 | |
Dalmatian | 6.20 | 12.60 | 9.29 | 1.53 | |
Fructose (%) | Linius | 1.81 | 4.18 | 3.06 | 0.69 |
Ignazio | 3.05 | 5.95 | 3.95 | 0.63 | |
Dalmatian | 2.99 | 7.02 | 4.49 | 0.84 | |
Glucose (%) | Linius | 1.68 | 6.80 | 3.79 | 1.20 |
Ignazio | 5.28 | 9.41 | 6.79 | 0.98 | |
Dalmatian | 4.33 | 12.13 | 7.16 | 1.57 | |
Sucrose (%) | Linius | 0.02 | 3.30 | 0.84 | 0.77 |
Ignazio | 2.83 | 11.00 | 6.18 | 1.88 | |
Dalmatian | 1.73 | 15.84 | 6.19 | 3.23 | |
L* | Linius | 29.85 | 68.41 | 57.36 | 8.23 |
Ignazio | 41.47 | 74.68 | 59.63 | 7.62 | |
Dalmatian | 31.16 | 69.96 | 54.55 | 8.30 | |
a* | Linius | 12.44 | 27.27 | 19.18 | 3.52 |
Ignazio | −1.15 | 8.37 | 1.62 | 2.51 | |
Dalmatian | −7.70 | 9.13 | −1.84 | 4.15 | |
b* | Linius | 23.23 | 46.42 | 35.66 | 4.84 |
Ignazio | 9.57 | 22.99 | 14.08 | 3.63 | |
Dalmatian | 13.48 | 41.32 | 23.79 | 5.92 |
Figure 2 depicts the results of the principal component analysis evaluation (PCA). The reference data for Linius sugar content were separated from Ignazio and Dalmatian group (Figure 2(a)), which had an overlapping data distribution and indicated similarity in sugar content. However, Dalmatian has a higher sugar content than Ignazio based on the average value. The total variation in data that can be explained by three PCs is 82% (PC1), 15% (PC2), and 2% (PC3). The scatter plot for color data that includes L*, a*, and b* in Figure 2(b) yielded values of 67%, 25%, and 8% for PC1, PC2, and PC3, respectively, with a total of 100% data variation. Unlike the sugar content scatter plot, the PCA analysis results on color data show three large groups representing each variety, with no overlapping data distribution between varieties (Figure 2(b)).
The dataset contains 135 spectra from three different melon varieties. The Vis/NIR spectra in the 381-1065 nm wavelength range contain detailed information about the sample's chemical constituents. Figure 3(a) describes the example of spectrum of each variety, while Figure 3(b) displays the first two PCs of loadings plot extracted from the all acquisition of calibration set. Each variety has its own characteristics, which makes developing general quantitative models for melons difficult. Therefore, it is important to interpret the Vis/NIR spectra before the modelling stage. The original spectra revealed that the peaks at 480, 500, and 640 nm corresponded to color pigment absorptions [20,21]. Conversely, the peaks at 950 and 975 nm are the water and sugar content absorptions [22,23]. However, it is still important to optimize the original spectra to clarify the chemical information of the sample. The Vis/NIR modeling in this research was conducted to predict the quality attributes quantitatively. There is the need to eliminate the noise produced by the spectrum, as well as other irrelevant data based on the quality attributes [24,25].
Chemometric techniques in Vis/NIR modelling are broadly classified into two types: linear regression approaches and nonlinear regression approaches. PLSR is the most commonly used approach in the application of linear regression to build a calibration model on Vis/NIR data. However, PLSR has limitations in finding correlations between Vis/NIR spectral data and quality attributes in some situations. This condition occurs when the predictor and response variables have a nonlinear relationship, which can also occur when there are significant data variations in calibration modelling [26]. Therefore, this problem can be solved using a nonlinear regression approach, specifically SVMR, which has a similar sample distribution with PLSR. Additionally, the calibration set contains a broader range of data than the prediction set since it aims to develop an accurate model.
As described in the methods section, the SNV, MSC, and dg2 methods were used to process all of the original spectra (Figure 4). Each preprocessing spectrum was evaluated on all quality attributes (Table 2). Previous study by Wu et al. [27] reported that the use of SNV and MSC yielded highest accuracy over other spectra preprocessing for sugar content quantification in pears. Moreover, a study conducted by Xie et al. [28] regarding the prediction of glucose, fructose, and sucrose in bayberry juice concluded that second derivative preprocessing method generated the preferable result compared to the first derivative and the original spectra. Hence, this led to the application of SNV, MSC, and dg2 in our study. The combination of PLSR and dg2 was found to be the optimal model for modelling SSC, sucrose, and b*. PLSR + SNV produced the most reliable model for fructose, L*, and a* prediction. On the other hand, the PLSR + original spectra combination produced the best results for the quality attribute of glucose levels. By incorporating the analyzed quality attribute data (variable Y), PLSR converts the spectral data (variable X) to a new variable (principal components), and then performs multiple linear regression on the principal component and variable Y [29]. The results of modelling with PLSR indicate that a global regression model for monitoring melon quality can be developed successfully. The following challenge is to develop a model capable of higher prediction accuracy.
Quality attributes | Model development | Calibration | Prediction (Validation) | RPD | ||
R2cal | RMSEC | R2pred | RMSEP | |||
SSC | PLSR + dg2 | 0.82 | 1.00 | 0.78 | 1.02 | 2.14 |
SVMR + dg2 | 0.90 | 0.73 | 0.75 | 1.09 | 2.00 | |
Fructose | PLSR + SNV | 0.37 | 0.76 | 0.39 | 0.66 | 1.26 |
SVMR + dg2 | 0.61 | 0.62 | 0.46 | 0.62 | 1.35 | |
Glucose | PLSR + Original | 0.56 | 1.34 | 0.60 | 1.16 | 1.57 |
SVMR + dg2 | 0.63 | 1.24 | 0.61 | 1.15 | 1.59 | |
Sucrose | PLSR + dg2 | 0.69 | 1.90 | 0.76 | 1.44 | 2.04 |
SVMR + dg2 | 0.76 | 1.76 | 0.81 | 1.27 | 2.31 | |
L* | PLSR + SNV | 0.02 | 8.47 | 0.10 | 7.27 | 1.04 |
SVMR + Original | 0.14 | 8.41 | 0.13 | 7.11 | 1.06 | |
a* | PLSR+ SNV | 0.90 | 3.07 | 0.87 | 3.36 | 2.82 |
SVMR + dg2 | 0.93 | 2.50 | 0.88 | 3.19 | 2.97 | |
b* | PLSR + dg2 | 0.78 | 4.74 | 0.80 | 4.20 | 2.26 |
SVMR + dg2 | 0.87 | 3.71 | 0.84 | 3.81 | 2.49 | |
Note: SSC: soluble solids content; PLSR: partial least squares regression; SVMR: support vector machine regression; SNV: standard normal variate; MSC: multiplicative scatter correction; R2cal: coefficient of determination in calibration set; RMSEC: root mean squares error of calibration; R2pred: coefficient of determination in prediction set; RMSEP: root mean squares error of prediction; RPD: ratio of performance to deviation. |
SVMR was also applied to the same data to compare its predictive accuracy with PLSR. Furthermore, SVMR can be expected to be a good candidate for this problem because of its ability to find nonlinear relationships and the good data generalizability [30,31]. The best spectra preprocessing for SSC quality attributes, fructose, glucose, sucrose, a*, and b* in SVMR modeling are shown by dg2 (Table 2). The dg2 method was quite effective in interpreting the low concentration of quality attributes since it could increase the spectral resolution [32]. On the other hand, SVMR + original spectra show the best calibration and prediction in L* modeling. SSC, glucose, sucrose, a*, and b* resulted in a reliable model to be applied in monitoring fruit quality in the field. This is indicated by the RPD values of 2.00 (SSC), 1.59 (glucose), 2.31 (sucrose), 2.97 (a*), and 2.49 (b*). However, SVMR showed poor predictive accuracy similar to the PLSR approach on the quality attributes of fructose and L* (Figure 5). In both models, only 1.35 (fructose) and 1.06 (L*) RPD values were obtained. This is due to the limited range of Vis/NIR wavelengths to penetrate thick-pulped melons hence the fructose and L* modeling results are less accurate (RPD < 1.5). Another cause is the variability of the physical characteristics and particle size of the sample which affects the linearity of the data.
Generally, this study aimed to promote SVMR as a viable candidate for spectral data regression. Theoretically, SVMR is advantageous for spectra regression because it is capable of performing both linear and nonlinear regression on a set of data efficiently. Basically, PLSR and SVMR can be used to predict global melon fruit quality using Vis/NIR spectroscopy [33,34]. The optimal model should exhibit the highest R2cal, R2pred, and RPD values. However, the error value of the model should be kept to a minimum (RMSEC and RMSEP). The error value of the SVMR calibration model is less than that of the PLSR. These results suggest that SVMR produces a more accurate model when dealing with variations in the data of the calibration set. This is also supported by the fact that SVMR produces a better calibration model than PLSR, as shown by the higher R2cal value on all quality attributes. On the other hand, the prediction set produced inconsistent results, which showed that the PLSR outperformed SVMR in terms of R2pred in SSC prediction. Additionally, the RMSEP of SVMR was significantly higher in SSC prediction but the reason for the increased RMSEP was still unknown. This often occurs in over-fitting models [35,36,37]. Conversely, SVMR produces a lower RMSEP than PLSR in other scenarios and was found to be more effective at establishing relationships between Vis/NIR spectra and complex patterns of quality attributes. However, this does not always imply that SVMR is the best method. The use of linear regression is usually preferred over nonlinear regression because of its simplicity. Additionally, certain nonlinear regression problems, such as SSC prediction in this study, can be solved using linear regression methods. SVMR may be used in place of PLSR to predict the sugar content and pulp color of melon in a non-destructive manner, especially the three varieties of melon that are prevalent in Indonesia.
The results showed that visible near-infrared (Vis/NIR) spectroscopy can be used to predict the sugar content and pulp color of melon using a global model. Also, the resulting model showed a good accuracy based on the quality attributes of SSC, glucose, sucrose, a*, b*. However, the RPD values based on the quality attributes of fructose and L* were 1.35 and 1.06, which were below the minimum recommended values for field application. The use of support vector machine regression (SVMR) could improve the accuracy of the model on the quality attributes of fructose, glucose, sucrose, L*, a*, and b*.
The authors are grateful to Yusuf Eka Maulana and Ine Elisa Putri as research assistants for sharing and supporting this study.
The authors declare no conflict of interest.
[1] | Kusumiyati, Hadiwijaya Y, Putri IE, et al. (2021) Multi-product calibration model for soluble solids and water content quantification in Cucurbitaceae family, using visible/near-infrared spectroscopy. Heliyon 7: e07677. https://doi.org/10.1016/j.heliyon.2021.e07677 |
[2] |
Kusumiyati K, Hadiwijaya Y, Putri IE, et al. (2021) Enhanced visible/near-infrared spectroscopic data for prediction of quality attributes in Cucurbitaceae commodities. Data Brief 39: 107458. https://doi.org/10.1016/j.dib.2021.107458 doi: 10.1016/j.dib.2021.107458
![]() |
[3] |
Hadiwijaya Y, Kusumiyati K, Munawar AA (2020) Penerapan teknologi visible-near infrared spectroscopy untuk prediksi cepat dan simultan kadar air buah melon (Cucumis melo L.) golden. Agroteknika 3: 67-74. https://doi.org/10.32530/agroteknika.v3i2.83 doi: 10.32530/agroteknika.v3i2.83
![]() |
[4] | Hadiwijaya Y, Kusumiyati K, Munawar AA (2020) Prediksi total padatan terlarut buah melon golden menggunakan vis-swnirs dan analisis multivariat. J Penelit Saintek 25: 103-114. |
[5] |
Mancini M, Mazzoni L, Gagliardi F, et al. (2020) Application of the non-destructive NIR technique for the evaluation of strawberry fruits quality parameters. Foods 9: 441. https://doi.org/10.3390/foods9040441 doi: 10.3390/foods9040441
![]() |
[6] |
Gao Q, Wang ML, Guo YY, et al. (2019) Comparative analysis of non-destructive prediction model of soluble solids content for malus micromalus makino based on near-infrared spectroscopy. IEEE Access 7: 128064-128075. https://doi.org/10.1109/ACCESS.2019.2939579. doi: 10.1109/ACCESS.2019.2939579
![]() |
[7] |
Alhamdan AM, Atia A (2017) Non-destructive method to predict Barhi dates quality at different stages of maturity utilising near-infrared (NIR) spectroscopy. Int J Food Prop 20: 2950-2959. https://doi.org/10.1080/10942912.2017.1387794 doi: 10.1080/10942912.2017.1387794
![]() |
[8] |
Kusumiyati K, Hadiwijaya Y, Putri IE (2019) Non-destructive classification of fruits based on vis-nir spectroscopy and principal component analysis. J Biodjati 4: 89-95. https://doi.org/10.15575/biodjati.v4i1.4389 doi: 10.15575/biodjati.v4i1.4389
![]() |
[9] |
Kusumiyati K, Hadiwijaya Y, Suhandy D, et al. (2021) Prediction of water content and soluble solids content of 'manalagi' apples using near infrared spectroscopy. IOP Conf Ser Earth Environ Sci 922: 012062. https://doi.org/10.1088/1755-1315/922/1/012062 doi: 10.1088/1755-1315/922/1/012062
![]() |
[10] |
Sánchez MT, Torres I, De La Haba MJ, et al. (2014) First steps to predicting pulp colour in whole melons using near-infrared reflectance spectroscopy. Biosyst Eng 123: 12-18. https://doi.org/10.1016/j.biosystemseng.2014.04.010 doi: 10.1016/j.biosystemseng.2014.04.010
![]() |
[11] |
Li M, Han DH, Liu W (2019) Non-destructive measurement of soluble solids content of three melon cultivars using portable visible/near infrared spectroscopy. Biosyst Eng 188: 31-39. https://doi.org/10.1016/j.biosystemseng.2019.10.003 doi: 10.1016/j.biosystemseng.2019.10.003
![]() |
[12] |
Wang JH, Wang J, Chen Z, et al. (2017) Development of multi-cultivar models for predicting the soluble solid content and firmness of European pear (Pyrus communis L.) using portable vis-NIR spectroscopy. Postharvest Biol Tec 129: 143-151. https://doi.org/10.1016/j.postharvbio.2017.03.012 doi: 10.1016/j.postharvbio.2017.03.012
![]() |
[13] |
Kusumiyati, Hadiwijaya Y, Putri IE, et al. (2019) Water content prediction of 'crystal' guava using visible-near infrared spectroscopy and chemometrics approach. IOP Conf Ser Earth Environ Sci 393: 012099. https://doi.org/10.1088/1755-1315/393/1/012099 doi: 10.1088/1755-1315/393/1/012099
![]() |
[14] | Damayanti S, Permana B, Weng CC (2012) Determination of sugar content in fruit juices using high performance liquid chromatography. Acta Pharm Indones 37: 139-145. |
[15] | Saad WMM, Salin NSM, Ramzi AS, et al. (2020) Identification and quantification of fructose, glucose and sucrose in watermelon peel juice. Malaysian J Anal Sci 24: 382-389. |
[16] |
Kawamura K, Tsujimoto Y, Rabenarivo M, et al. (2017) Vis-NIR spectroscopy and PLS regression with waveband selection for estimating the total C and N of paddy soils in Madagascar. Remote Sens-Basel 9: 1081. https://doi.org/10.3390/rs9101081 doi: 10.3390/rs9101081
![]() |
[17] |
Nicolaï BM, Beullens K, Bobelyn E, et al. (2007) Nondestructive measurement of fruit and vegetable quality by means of NIR spectroscopy: A review. Postharvest Biol Tec 46: 99-118. https://doi.org/10.1016/j.postharvbio.2007.06.024 doi: 10.1016/j.postharvbio.2007.06.024
![]() |
[18] |
Rambo MKD, Ferreira MMC, Amorim EP (2016) Multi-product calibration models using NIR spectroscopy. Chemometr Intell Lab 151: 108-114. https://doi.org/10.1016/j.chemolab.2015.12.013 doi: 10.1016/j.chemolab.2015.12.013
![]() |
[19] |
Clavaud M, Roggo Y, Dégardin K, et al. (2017) Global regression model for moisture content determination using near-infrared spectroscopy. Eur J Pharm Biopharm 119: 343-352. https://doi.org/10.1016/j.ejpb.2017.07.007 doi: 10.1016/j.ejpb.2017.07.007
![]() |
[20] |
Kusumiyati K, Putri IE, Munawar AA (2021) Model prediksi kadar air buah cabai rawit domba (Capsicum frutescens L.) menggunakan spektroskopi ultraviolet visible near infrared. Agro Bali Agric J 4: 15-22. https://doi.org/10.37637/ab.v4i1.615 doi: 10.37637/ab.v4i1.615
![]() |
[21] |
Putri IE, Kusumiyati K, Munawar AA (2021) Penerapan algoritma diskriminasi menggunakan metode principal component analysis (PCA) dan Vis-SWNIR spectroscopy pada buah cabai rawit domba berbagai tingkat kematangan. SINTECH J 4: 40-46. https://doi.org/10.31598/sintechjournal.v4i1.680 doi: 10.31598/sintechjournal.v4i1.680
![]() |
[22] |
Kusumiyati, Hadiwijaya Y, Putri IE (2018) Determination of water content of intact sapodilla using near infrared spectroscopy. IOP Conf Ser Earth Environ Sci 207: 012047. https://doi.org/10.1088/1755-1315/207/1/012047 doi: 10.1088/1755-1315/207/1/012047
![]() |
[23] |
Kusumiyati, Hadiwijaya Y, Putri IE, et al. (2020) Rapid and non-destructive prediction of total soluble solids of guava fruits at various storage periods using handheld near-infrared instrument, IOP Conf Ser Earth Environ Sci 458: 012022. https://doi.org/10.1088/1755-1315/458/1/012022 doi: 10.1088/1755-1315/458/1/012022
![]() |
[24] |
Kusumiyati K, Putri IE, Munawar AA, et al. (2022) A data fusion model to merge the spectra data of intact and powdered cayenne pepper for the fast inspection of antioxidant properties. Sustainability 14: 201. https://doi.org/10.3390/su14010201 doi: 10.3390/su14010201
![]() |
[25] |
Rinnan Å, Berg FVD, Engelsen SB (2009) Review of the most common pre-processing techniques for near-infrared spectra. TrAC-Trend Anal Chem 28: 1201-1222. https://doi.org/10.1016/j.trac.2009.07.007 doi: 10.1016/j.trac.2009.07.007
![]() |
[26] | Igne B, Drennen JK, Anderson CA (2014) Improving near-infrared prediction model robustness with support vector machine regression: A pharmaceutical tablet assay example. Appl Spectrosc 68: 1348-1356. https://doi.org/10.1366%2F14-07486 |
[27] |
Wu X, Li GL, He FY (2021) Nondestructive analysis of internal quality in pears with a self-made near-infrared spectrum detector combined with multivariate data processing. Foods 10: 1315. https://doi.org/10.3390/foods10061315 doi: 10.3390/foods10061315
![]() |
[28] |
Xie LJ, Ye XQ, Liu DH, et al. (2009) Quantification of glucose, fructose and sucrose in bayberry juice by NIR and PLS. Food Chem 114: 1135-1140. https://doi.org/10.1016/j.foodchem.2008.10.076 doi: 10.1016/j.foodchem.2008.10.076
![]() |
[29] | Cui CH, Fearn T (2017) Comparison of partial least squares regression, least squares support vector machines, and Gaussian process regression for a near infrared calibration. J Near Infrared Spec 25: 5-14. https://doi.org/10.1177%2F0967033516678515 |
[30] |
Sarkar S, Basak JK, Moon BE, et al. (2020) A comparative study of PLSR and SVM-R with various preprocessing techniques for the quantitative determination of soluble solids content of hardy kiwi fruit by a portable Vis/NIR spectrometer. Foods 9: 1078. https://doi.org/10.3390/foods9081078 doi: 10.3390/foods9081078
![]() |
[31] |
Skolik P, Morais CLM, Martin FL, et al. (2019) Determination of developmental and ripening stages of whole tomato fruit using portable infrared spectroscopy and Chemometrics. BMC Plant Biol 19: 1-15. https://doi.org/10.1186/s12870-019-1852-5 doi: 10.1186/s12870-019-1852-5
![]() |
[32] |
Cen HY, He Y (2007) Theory and application of near infrared reflectance spectroscopy in determination of food quality. Trends Food Sci Tech 18: 72-83. https://doi.org/10.1016/j.tifs.2006.09.003 doi: 10.1016/j.tifs.2006.09.003
![]() |
[33] |
Xie LJ, Ying YB (2009) Use of near-infrared spectroscopy and least-squares support vector machine to determine quality change of tomato juice. J Zhejiang Univ-Sci B 10: 465-471. https://doi.org/10.1631/jzus.B0820299 doi: 10.1631/jzus.B0820299
![]() |
[34] |
Khurnpoon L, Sirisomboon P (2018) Rapid evaluation of the texture properties of melon (Cucumis melo L. Var. reticulata cv. Green net) using near infrared spectroscopy. J Texture Stud 49: 387-394. https://doi.org/10.1111/jtxs.12329 doi: 10.1111/jtxs.12329
![]() |
[35] |
Zhang YY, Nock JF, Al Shoffe Y, et al. (2019) Non-destructive prediction of soluble solids and dry matter contents in eight apple cultivars using near-infrared spectroscopy. Postharvest Biol Tec 151: 111-118. https://doi.org/10.1016/j.postharvbio.2019.01.009 doi: 10.1016/j.postharvbio.2019.01.009
![]() |
[36] |
Schoot M, Kapper C, van Kollenburg GH, et al. (2020) Investigating the need for preprocessing of near-infrared spectroscopic data as a function of sample size. Chemometr Intell Lab 204: 104105. https://doi.org/10.1016/j.chemolab.2020.104105 doi: 10.1016/j.chemolab.2020.104105
![]() |
[37] |
Fernández-Novales J, Garde-Cerdán T, Tardáguila J, et al. (2019) Assessment of amino acids and total soluble solids in intact grape berries using contactless Vis and NIR spectroscopy during ripening. Talanta 199: 244-253. https://doi.org/10.1016/j.talanta.2019.02.037 doi: 10.1016/j.talanta.2019.02.037
![]() |
1. | Kusumiyati Kusumiyati, Rosalinda Julian Syifa, Farida Farida, Effect of various varieties and dosage of potassium fertilizer on growth, yield, and quality of red chili (Capsicum annuum L.), 2022, 7, 2391-9531, 948, 10.1515/opag-2022-0151 | |
2. | Kusumiyati Kusumiyati, Yonathan Asikin, Machine learning-based prediction of total phenolic and flavonoid in horticultural products, 2023, 8, 2391-9531, 10.1515/opag-2022-0163 | |
3. | Kusumiyati Kusumiyati, Ine Elisa Putri, Jajang Sauman Hamdani, Diding Suhandy, Real-Time Detection of the Nutritional Compounds in Green ‘Ratuni UNPAD’ Cayenne Pepper, 2022, 8, 2311-7524, 554, 10.3390/horticulturae8060554 | |
4. | Kusumiyati Kusumiyati, Ine Elisa Putri, Comparison of color spectrophotometer and Vis/NIR spectroscopy on assessing natural pigments of cucumber applied with different ethephon concentrations, 2023, 9, 24058440, e22564, 10.1016/j.heliyon.2023.e22564 | |
5. | Kusumiyati Kusumiyati, Grace Pratiwi Manurung, Jajang Sauman Hamdani, Growth, yield, and secondary metabolite responses of three shallot cultivars at different watering intervals, 2024, 9, 2391-9531, 10.1515/opag-2022-0255 | |
6. | Kusumiyati Kusumiyati, Eizo Taira, Yusuf Eka Maulana, Near-infrared Spectroscopy for Direct Investigation of Quality Compounds in Capsicum spp (Capsicum annuum L. and Capsicum frutescens L.), 2024, 18, 1874-3315, 10.2174/0118743315289226240122063646 | |
7. | L. Marinoni, T. M. P. Cattaneo, M. Vanoli, S. Barzaghi, Real-time monitoring of solar drying of melon slices with a portable NIR spectrometer: a preliminary approach, 2023, 249, 1438-2377, 2151, 10.1007/s00217-023-04285-y | |
8. | Kusumiyati Kusumiyati, Ine Elisa Putri, Yuda Hadiwijaya, Agitha Kartika, Yusuf Eka Maulana, Wawan Sutari, Ravi Pandiselvam, Quality Assurance of Total Carotenoids and Quercetin in Marigold Flowers (Tagetes erecta L.) as Edible Flowers, 2025, 2025, 2356-7015, 10.1155/ijfo/3277288 |
Quality attributes | Variety | Minimum | Maximum | Mean | Standard deviation |
SSC (%) | Linius | 2.70 | 8.80 | 4.74 | 1.21 |
Ignazio | 7.00 | 10.90 | 8.56 | 1.00 | |
Dalmatian | 6.20 | 12.60 | 9.29 | 1.53 | |
Fructose (%) | Linius | 1.81 | 4.18 | 3.06 | 0.69 |
Ignazio | 3.05 | 5.95 | 3.95 | 0.63 | |
Dalmatian | 2.99 | 7.02 | 4.49 | 0.84 | |
Glucose (%) | Linius | 1.68 | 6.80 | 3.79 | 1.20 |
Ignazio | 5.28 | 9.41 | 6.79 | 0.98 | |
Dalmatian | 4.33 | 12.13 | 7.16 | 1.57 | |
Sucrose (%) | Linius | 0.02 | 3.30 | 0.84 | 0.77 |
Ignazio | 2.83 | 11.00 | 6.18 | 1.88 | |
Dalmatian | 1.73 | 15.84 | 6.19 | 3.23 | |
L* | Linius | 29.85 | 68.41 | 57.36 | 8.23 |
Ignazio | 41.47 | 74.68 | 59.63 | 7.62 | |
Dalmatian | 31.16 | 69.96 | 54.55 | 8.30 | |
a* | Linius | 12.44 | 27.27 | 19.18 | 3.52 |
Ignazio | −1.15 | 8.37 | 1.62 | 2.51 | |
Dalmatian | −7.70 | 9.13 | −1.84 | 4.15 | |
b* | Linius | 23.23 | 46.42 | 35.66 | 4.84 |
Ignazio | 9.57 | 22.99 | 14.08 | 3.63 | |
Dalmatian | 13.48 | 41.32 | 23.79 | 5.92 |
Quality attributes | Model development | Calibration | Prediction (Validation) | RPD | ||
R2cal | RMSEC | R2pred | RMSEP | |||
SSC | PLSR + dg2 | 0.82 | 1.00 | 0.78 | 1.02 | 2.14 |
SVMR + dg2 | 0.90 | 0.73 | 0.75 | 1.09 | 2.00 | |
Fructose | PLSR + SNV | 0.37 | 0.76 | 0.39 | 0.66 | 1.26 |
SVMR + dg2 | 0.61 | 0.62 | 0.46 | 0.62 | 1.35 | |
Glucose | PLSR + Original | 0.56 | 1.34 | 0.60 | 1.16 | 1.57 |
SVMR + dg2 | 0.63 | 1.24 | 0.61 | 1.15 | 1.59 | |
Sucrose | PLSR + dg2 | 0.69 | 1.90 | 0.76 | 1.44 | 2.04 |
SVMR + dg2 | 0.76 | 1.76 | 0.81 | 1.27 | 2.31 | |
L* | PLSR + SNV | 0.02 | 8.47 | 0.10 | 7.27 | 1.04 |
SVMR + Original | 0.14 | 8.41 | 0.13 | 7.11 | 1.06 | |
a* | PLSR+ SNV | 0.90 | 3.07 | 0.87 | 3.36 | 2.82 |
SVMR + dg2 | 0.93 | 2.50 | 0.88 | 3.19 | 2.97 | |
b* | PLSR + dg2 | 0.78 | 4.74 | 0.80 | 4.20 | 2.26 |
SVMR + dg2 | 0.87 | 3.71 | 0.84 | 3.81 | 2.49 | |
Note: SSC: soluble solids content; PLSR: partial least squares regression; SVMR: support vector machine regression; SNV: standard normal variate; MSC: multiplicative scatter correction; R2cal: coefficient of determination in calibration set; RMSEC: root mean squares error of calibration; R2pred: coefficient of determination in prediction set; RMSEP: root mean squares error of prediction; RPD: ratio of performance to deviation. |
Quality attributes | Variety | Minimum | Maximum | Mean | Standard deviation |
SSC (%) | Linius | 2.70 | 8.80 | 4.74 | 1.21 |
Ignazio | 7.00 | 10.90 | 8.56 | 1.00 | |
Dalmatian | 6.20 | 12.60 | 9.29 | 1.53 | |
Fructose (%) | Linius | 1.81 | 4.18 | 3.06 | 0.69 |
Ignazio | 3.05 | 5.95 | 3.95 | 0.63 | |
Dalmatian | 2.99 | 7.02 | 4.49 | 0.84 | |
Glucose (%) | Linius | 1.68 | 6.80 | 3.79 | 1.20 |
Ignazio | 5.28 | 9.41 | 6.79 | 0.98 | |
Dalmatian | 4.33 | 12.13 | 7.16 | 1.57 | |
Sucrose (%) | Linius | 0.02 | 3.30 | 0.84 | 0.77 |
Ignazio | 2.83 | 11.00 | 6.18 | 1.88 | |
Dalmatian | 1.73 | 15.84 | 6.19 | 3.23 | |
L* | Linius | 29.85 | 68.41 | 57.36 | 8.23 |
Ignazio | 41.47 | 74.68 | 59.63 | 7.62 | |
Dalmatian | 31.16 | 69.96 | 54.55 | 8.30 | |
a* | Linius | 12.44 | 27.27 | 19.18 | 3.52 |
Ignazio | −1.15 | 8.37 | 1.62 | 2.51 | |
Dalmatian | −7.70 | 9.13 | −1.84 | 4.15 | |
b* | Linius | 23.23 | 46.42 | 35.66 | 4.84 |
Ignazio | 9.57 | 22.99 | 14.08 | 3.63 | |
Dalmatian | 13.48 | 41.32 | 23.79 | 5.92 |
Quality attributes | Model development | Calibration | Prediction (Validation) | RPD | ||
R2cal | RMSEC | R2pred | RMSEP | |||
SSC | PLSR + dg2 | 0.82 | 1.00 | 0.78 | 1.02 | 2.14 |
SVMR + dg2 | 0.90 | 0.73 | 0.75 | 1.09 | 2.00 | |
Fructose | PLSR + SNV | 0.37 | 0.76 | 0.39 | 0.66 | 1.26 |
SVMR + dg2 | 0.61 | 0.62 | 0.46 | 0.62 | 1.35 | |
Glucose | PLSR + Original | 0.56 | 1.34 | 0.60 | 1.16 | 1.57 |
SVMR + dg2 | 0.63 | 1.24 | 0.61 | 1.15 | 1.59 | |
Sucrose | PLSR + dg2 | 0.69 | 1.90 | 0.76 | 1.44 | 2.04 |
SVMR + dg2 | 0.76 | 1.76 | 0.81 | 1.27 | 2.31 | |
L* | PLSR + SNV | 0.02 | 8.47 | 0.10 | 7.27 | 1.04 |
SVMR + Original | 0.14 | 8.41 | 0.13 | 7.11 | 1.06 | |
a* | PLSR+ SNV | 0.90 | 3.07 | 0.87 | 3.36 | 2.82 |
SVMR + dg2 | 0.93 | 2.50 | 0.88 | 3.19 | 2.97 | |
b* | PLSR + dg2 | 0.78 | 4.74 | 0.80 | 4.20 | 2.26 |
SVMR + dg2 | 0.87 | 3.71 | 0.84 | 3.81 | 2.49 | |
Note: SSC: soluble solids content; PLSR: partial least squares regression; SVMR: support vector machine regression; SNV: standard normal variate; MSC: multiplicative scatter correction; R2cal: coefficient of determination in calibration set; RMSEC: root mean squares error of calibration; R2pred: coefficient of determination in prediction set; RMSEP: root mean squares error of prediction; RPD: ratio of performance to deviation. |