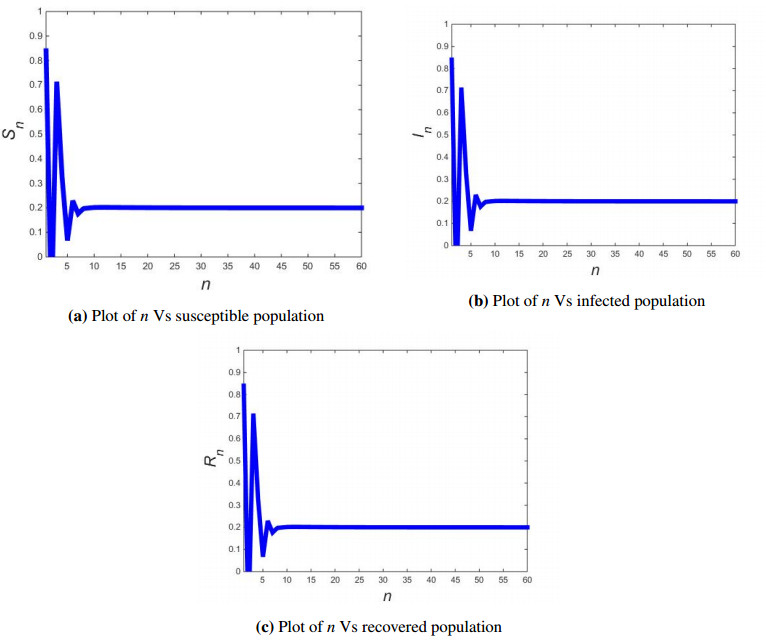
The local dynamics with different topological classifications, bifurcation analysis and chaos control in a discrete-time COVID-19 epidemic model are investigated in the interior of R3+. It is proved that discrete-time COVID-19 epidemic model has boundary equilibrium solution for all involved parameters, but it has an interior equilibrium solution under definite parametric condition. Then by linear stability theory, local dynamics with different topological classifications are investigated about boundary and interior equilibrium solutions of the discrete-time COVID-19 epidemic model. Further for the discrete-time COVID-19 epidemic model, existence of periodic points and convergence rate are also investigated. It is also investigated the existence of possible bifurcations about boundary and interior equilibrium solutions, and proved that there exists no flip bifurcation about boundary equilibrium solution. Moreover, it is proved that about interior equilibrium solution there exists hopf and flip bifurcations, and we have studied these bifurcations by utilizing explicit criterion. Next by feedback control strategy, chaos in the discrete COVID-19 epidemic model is also explored. Finally numerically verified theoretical results.
Citation: A. Q. Khan, M. Tasneem, M. B. Almatrafi. Discrete-time COVID-19 epidemic model with bifurcation and control[J]. Mathematical Biosciences and Engineering, 2022, 19(2): 1944-1969. doi: 10.3934/mbe.2022092
[1] | Hongfan Lu, Yuting Ding, Silin Gong, Shishi Wang . Mathematical modeling and dynamic analysis of SIQR model with delay for pandemic COVID-19. Mathematical Biosciences and Engineering, 2021, 18(4): 3197-3214. doi: 10.3934/mbe.2021159 |
[2] | Tao Chen, Zhiming Li, Ge Zhang . Analysis of a COVID-19 model with media coverage and limited resources. Mathematical Biosciences and Engineering, 2024, 21(4): 5283-5307. doi: 10.3934/mbe.2024233 |
[3] | Cheng-Cheng Zhu, Jiang Zhu . Spread trend of COVID-19 epidemic outbreak in China: using exponential attractor method in a spatial heterogeneous SEIQR model. Mathematical Biosciences and Engineering, 2020, 17(4): 3062-3087. doi: 10.3934/mbe.2020174 |
[4] | Pannathon Kreabkhontho, Watchara Teparos, Thitiya Theparod . Potential for eliminating COVID-19 in Thailand through third-dose vaccination: A modeling approach. Mathematical Biosciences and Engineering, 2024, 21(8): 6807-6828. doi: 10.3934/mbe.2024298 |
[5] | Salma M. Al-Tuwairqi, Sara K. Al-Harbi . Modeling the effect of random diagnoses on the spread of COVID-19 in Saudi Arabia. Mathematical Biosciences and Engineering, 2022, 19(10): 9792-9824. doi: 10.3934/mbe.2022456 |
[6] | Sarita Bugalia, Jai Prakash Tripathi, Hao Wang . Mathematical modeling of intervention and low medical resource availability with delays: Applications to COVID-19 outbreaks in Spain and Italy. Mathematical Biosciences and Engineering, 2021, 18(5): 5865-5920. doi: 10.3934/mbe.2021295 |
[7] | Yue Deng, Siming Xing, Meixia Zhu, Jinzhi Lei . Impact of insufficient detection in COVID-19 outbreaks. Mathematical Biosciences and Engineering, 2021, 18(6): 9727-9742. doi: 10.3934/mbe.2021476 |
[8] | Shishi Wang, Yuting Ding, Hongfan Lu, Silin Gong . Stability and bifurcation analysis of $ SIQR $ for the COVID-19 epidemic model with time delay. Mathematical Biosciences and Engineering, 2021, 18(5): 5505-5524. doi: 10.3934/mbe.2021278 |
[9] | Xinyu Liu, Zimeng Lv, Yuting Ding . Mathematical modeling and stability analysis of the time-delayed $ SAIM $ model for COVID-19 vaccination and media coverage. Mathematical Biosciences and Engineering, 2022, 19(6): 6296-6316. doi: 10.3934/mbe.2022294 |
[10] | Chenxi Dai, Jing Yang, Kaifa Wang . Evaluation of prevention and control interventions and its impact on the epidemic of coronavirus disease 2019 in Chongqing and Guizhou Provinces. Mathematical Biosciences and Engineering, 2020, 17(4): 2781-2791. doi: 10.3934/mbe.2020152 |
The local dynamics with different topological classifications, bifurcation analysis and chaos control in a discrete-time COVID-19 epidemic model are investigated in the interior of R3+. It is proved that discrete-time COVID-19 epidemic model has boundary equilibrium solution for all involved parameters, but it has an interior equilibrium solution under definite parametric condition. Then by linear stability theory, local dynamics with different topological classifications are investigated about boundary and interior equilibrium solutions of the discrete-time COVID-19 epidemic model. Further for the discrete-time COVID-19 epidemic model, existence of periodic points and convergence rate are also investigated. It is also investigated the existence of possible bifurcations about boundary and interior equilibrium solutions, and proved that there exists no flip bifurcation about boundary equilibrium solution. Moreover, it is proved that about interior equilibrium solution there exists hopf and flip bifurcations, and we have studied these bifurcations by utilizing explicit criterion. Next by feedback control strategy, chaos in the discrete COVID-19 epidemic model is also explored. Finally numerically verified theoretical results.
In December 2019, Wuhan city in China—home to 11 million people—first came to the global spotlight with the reports of a pneumonia-like disease. Initially it was suspected that certain animals such as bats were its origin. Later on, however, with an unexpected surge of the corona cases all over the world, it was declared as a serious infectious pandemic which spread from one infected person to another through direct or indirect contact. This severe acute respiratory syndrome, later called COVID-19, is actually caused by a novel Coronavirus SARS-CoV-2.
Besides, SARS-CoV-2, there have also been identified at least six other kinds of coronavirus that originate from animals. Most of them cause common cold which, in certain cases, can prove a potential threat to human health. Each infected person is estimated to pass the phenomenon on to three other people. It lasts approximately between 1–14 days. The common symptoms include: sore throat, fever, general weakness, shortness of breath, cough, pain etc. There are particular groups of people among whom the risk factor becomes too higher. They include old men and women, obese guys, chain smokers, those fighting hypertension and cancer patients. Prevention seems to be the only sure cure out there.
When it initiated in China nearly two years ago, the COVID-19 was limited only to the city of Wuhan. With the progress of time, however, it first spread in the whole country and then within months reached almost every corner of the world. It happened largely due to the careless of the masses. As a result, most government were forced to impose such strict regulations as lockdown. Maintaining social distancing too could be a very viral step to curb the spread of the virus which is generally reliant upon other people to reach the healthy segment of the society.
The second wave of this contagious coronavirus was another reminder that it's over. As we learn through media, the death rate was once again on the rise. In Pakistan and Azad Kashmir (just like the rest of the world), all educational institutions were closed for two or more months. The reason was simple. People didn't take the threat seriously and, as a result, its spread couldn't be stopped. Nations globally need to learn their lessons quickly. Without taking all the precautionary measures, we won't be able to fight COVID-19. On the other hand, as compared to second wave, the third and forth waves of coronavirus were not so serious and rate of new cases controlled.
Various vaccines are continuously being tested throughout the world. Most of them have reportedly proven successful too. This, however, seems to be time consuming task. It isn't simple to first agree on a single vaccine and then make it simultaneously available across all the continents. Therefore, we, the people, are left with no other option than taking care of ourselves and near and dear ones. Kids, being less immune, are particularly prone to catch most ailments. If there's a strict parental control, it will not just keep their own families safe, but the whole society at large will also be in a better position to defy this looming health danger.
In the meanwhile, many chemists, biologists, doctors and mathematicians are trying to study the behavior of coronavirus. On the mathematical side, there are few models already discovered, to study it's behavior. Some of them are: SIR (Susceptible, Infected and Recovered) model, SIQR (Susceptible, Infectious, Quarantined and Recovered) model, SEIR (Susceptible, Exposed, Infected and Recovered) model, and SIRS (Susceptible, Infected and Recovered, Susceptible) model. For instance, Zou et al. [1] suggested the following epidemic model (SuEIR), which is alternative to the SEIR model by considering the unreported COVID-19 cases and instructed by machine learning algorithms based on the reported historical data, for forecasting the propagation of COVID-19 comprising a lot of authentic and casualty cases at national levels in the United States:
dSdt=−β(I+E)SN, dEdt=β(I+E)SN−σE, dIdt=μσE−γI, dRdt=γI, | (1.1) |
where β is the exposure rate between the susceptible and infected people, σ expresses the ratio of cases in the revealed sections that are either confirmed as infectious or dead/recovered without authentication, μ is the rate of realization of the infected cases, and γ represents the alteration rate between the sections I and R. Ray et al. [2] estimated the real-time application of an open, collective ensemble to predict deaths attributable to COVID-19 in the United States. Shea et al. [3] explored multiple COVID-19 mathematical models to comprehend the sources of widespread COVID-19 disease. Nadim et al. [4] studied the stability analysis of the following compartmental epidemic model of COVID-19 to forecast and control the upsurge:
dSdt=Π−S(βI+rQβQ+rAβA+rJβJ)N−μS,dEdt=S(βI+rQβQ+rAβA+rJβJ)N−(γ1+k1+μ)E,dQdt=γ1E−(k2+σ1+μ)J,dAdt=pK1E−(σ2+μ)A,dIdt=(1−p)k1E−(γ2+σ3+μ)I,dJdt=k2Q+γ2I−(δ+σ4+μ)J,dRdt=σ1Q+σ2A+σ3I+σ4J−μR, | (1.2) |
where Π is recuitment rate, β is transmission rate. rQ, rA and rJ are modification factors for quarantined, asymptomatic and isolated respectively. γ1 is the rate at which the exposed individuals are diminished by quarantine, and γ2 is the rate at which the symptomatic individuals are diminished by isolation. k1 and k2 are rates at which exposed become infected, quarantined individuals are isolated, respectively. p is the proportion of asymptomatic individuals. σ1, σ2, σ3, σ4 are respectively the recovery rates from quarantined, asymptomatic, symptomatic and isolated individuals. δ and μ are the diseases induced mortality rate and natural death rate, respectively. Li et al. [5] developed a SEIQR COVID-19 model, described by the system of difference equation:
St+1=St−βStEt+β1StItNt,Et+1=Et+βStEt+β1StItNt−δEt,It+1=It+δEt−mIt,Qt+1=Qt+mIt−γQt,Rt+1=Rt+γQt,Nt=St+Et+It+Qt+Rt, | (1.3) |
where human host incubation period is 1δ days. Infectious cases at the rate m revert to confirmed cases, and recovered rate is γ. Susceptible humans acquire COVID-19 through direct contact with exposed cases and infected cases at rates βStEtNt and β1StItNt, respectively. Tian et al. [6] investigated the spread and control of COVID-19 using a data set that included case reports, human movement, and public health interventions. Sun et al. [7] presented the following model to show the propagation of COVID-19 in Wuhan:
dSdt=−β′1SE+β′2SIN, dEdt=β′1SE+β′2SIN−δE, dIdt=δE−m1I, dQdt=m1I−γQ, dRdt=γQ, | (1.4) |
where N, S, E, I, Q, R are total, susceptible, exposed, infected, confirmed and removed populations, respectively. β′1 and β′2 are infection coefficients under lockdown, and m1 is confirmation rate depends on the rickness of medical resources. δ is transform rate from the exposed population to infected population, and γ is transform rate from the confirmed population to the removed population. In 2021, Tesfaya et al. [8] suggested the following continuous-time stochastic COVID-19 epidemic model with jump-diffusion derived by both Gaussian and non-Gaussian noises:
dSdt=Λ−βSI−νS+σR, dIdt=βSI−(ν+γ)I, dRdt=γI−(ν+σ)R, | (1.5) |
where S, I and R respectively denote susceptible, infected and recovered populations, whereas joining rate of people to susceptible class through migration or birth is Λ, β is the rate at which susceptible class tends to infected one, ν is due to coronavirus death, recovery rate is γ, and σ is the rate of deteriorate in health.
Motivated from the aforementioned studies, the purpose of this study is to investigate the dynamical characteristics of COVID-19 epidemic model, which is discrete analogue of continuous-time stochastic COVID-19 epidemic model (1.5), by Euler-forward formula. It is noted here that by applying Euler-forward formula the continuous-time stochastic COVID-19 epidemic model (1.5) takes the following form:
St+1−Sth=Λ−βStIt−νSt+σRt, It+1−Ith=βStIt−(ν+γ)It, Rt+1−Rth=γIt−(ν+σ)Rt. | (1.6) |
After simplification, model (1.6) takes the following form:
Sn+1=hΛ+(1−νh)Sn−βhSnIn+hσRn, In+1=(1−νh−γh)In+hβSnIn,Rn+1=(1−hν−hσ)Rn+hγIn, | (1.7) |
with h is step size and t is denoted by n. It is also important to note that in the proposed work we study dynamical characteristics of discrete-time COVID-19 epidemic model (1.7), which is counter part of epidemic model (1.5), because discrete model gives more efficient computational results as compared to continuous once.
In the subsequent Section existence of equilibrium solutions of discrete COVID-19 epidemic model (1.7) is explored. In Section 3 we have constructed linearized form of discrete COVID-19 epidemic model (1.7) whereas Section 4 is about the exploration of local behavior of COVID-19 epidemic model (1.7) at equilibrium solutions. In Section 5 periodic points with period-n of COVID-19 epidemic model (1.7) is investigated. The convergence rate for COVID-19 epidemic model (1.7) is studied in Section 6. In detailed bifurcation analysis about equilibrium solutions of discrete COVID-19 epidemic model (1.7) is given in Section 7. Section 8 is about the study of chaos control of COVID-19 epidemic model (1.7). Numerical verifications of theoretical results are given in Section 9. The concluding remarks are given in Section 10.
In R3+, existence of equilibrium solutions of COVID-19 epidemic model (1.7) is studied in this Section, as follows:
Lemma 2.1. For existence results regarding equilibrium solutions of discrete COVID-19 epidemic model (1.7), following statements hold:
(i) ∀ h, β, Λ, ν, σ, γ>0, discrete COVID-19 epidemic model (1.7) has boundary equilibrium solution: ES00(Λν,0,0);
(ii) If β>ν(ν+γ)Λ then discrete COVID-19 epidemic model (1.7) has interior equilibrium solution:
E+SIR(ν+γβ,(ν+σ)(βΛ−ν2−νγ)β(ν2+(σ+γ)ν),γ(βΛ−ν2−νγ)β(ν2+(σ+γ)ν)). |
Proof. If equilibrium solution of COVID-19 epidemic model (1.7) is ESIR(S,I,R) then
S=hΛ+(1−νh)S−βhSI+hσR, I=(1−νh−γh)I+hβSI, R=(1−hν−hσ)R+hγI. | (2.1) |
It is noted that for ES00(Λν,0,0) algebraic system (2.1) satisfied identically. Thus one can obtain that the boundary solution of discrete COVID-19 epidemic model (1.7) is ES00(Λν,0,0). For the interior equilibrium solution of discrete COVID-19 epidemic model (1.7), one need to solve following algebraic system simultaneously:
(βI+ν)S=Λ+σR, ν+γ=βS, (ν+σ)R=γI. | (2.2) |
2nd equation of (2.2) yields
S=ν+γβ. | (2.3) |
Using Eq (2.3) in 1st equation of (2.2) one has
(βI+ν)ν+γβ=Λ+σR. | (2.4) |
From 3rd equation of (2.2) one has
R=γIν+σ. | (2.5) |
From Eqs (2.4) and (2.5) one has
I=(ν+σ)(βΛ−ν2−νγ)β(ν2+(σ+γ)ν). | (2.6) |
Using Eq (2.6) in Eq (2.5) one has
R=γ(βΛ−ν2−νγ)β(ν2+(σ+γ)ν). | (2.7) |
Finally, from Eqs (2.3), (2.6) and (2.7) it can be concluded that if β>ν(ν+γ)Λ then interior equilibrium solution of discrete COVID-19 epidemic model (1.7) is E+SIR(ν+γβ,(ν+σ)(βΛ−ν2−νγ)β(ν2+(σ+γ)ν),γ(βΛ−ν2−νγ)β(ν2+(σ+γ)ν)). Moreover it is important here to mention that if β>ν(ν+γ)Λ, i.e., βΛν(ν+γ)>1 then discrete COVID-19 epidemic model (1.7) has interior equilibrium solution, and hence basic reproductive number is R0=βΛν(ν+γ).
In the present Section, linearized form of discrete COVID-19 epidemic model (1.7) about equilibrium solution ESIR(S,I,R) is explored. The linearized form of discrete COVID-19 epidemic model (1.7) about equilibrium solution ESIR(S,I,R) under the map
(f,g,h)↦(Sn+1,In+1,Rn+1), | (3.1) |
is
Ψn+1=J|ESIR(S,I,R)Ψn, | (3.2) |
where
J|ESIR(S,I,R)=(1−νh−βhI−βhSσhβhI1−νh−γh+βhS00γh1−νh−σh), | (3.3) |
and
f=hΛ+(1−νh)S−βhSI+hσR, g=(1−νh−γh)I+hβSI, h=(1−hν−hσ)R+hγI. | (3.4) |
Local dynamic behavior of COVID-19 epidemic model (1.7) about equilibrium solutions: ES00(Λν,0,0) and E+SIR(ν+γβ,(ν+σ)(βΛ−ν2−νγ)β(ν2+(σ+γ)ν),γ(βΛ−ν2−νγ)β(ν2+(σ+γ)ν)) is explored in this section.
About ES00(Λν,0,0), Eq (3.3) becomes
J|ES00(Λν,0,0)=(1−νh−βΛhνσh01−hν−hγ+βΛhν00hγ1−hν−hσ), | (4.1) |
with characteristic roots are
λ1=1−νh, λ2=1−νh−γh+βΛhν, λ3=1−νh−σh. | (4.2) |
From Eq (4.2) and by stability theory [9,10,11,12], one can conclude local dynamic behavior of discrete COVID-19 epidemic model (1.7) about ES00(Λν,0,0) as follows.
Lemma 4.1. For local dynamic behavior of COVID-19 epidemic model (1.7) about ES00(Λν,0,0), following statements hold:
(i) ES00(Λν,0,0) of discrete COVID-19 model (1.7) is a sink if
0<ν<min{2h,2h−σ} and ν(νh+γh−2)hΛ<β<ν(ν+γ)Λ, | (4.3) |
with
σ<2h and ν>2h−γ; | (4.4) |
(ii) ES00(Λν,0,0) of discrete COVID-19 model (1.7) is a source if (4.4) holds and additionally
ν>max{2h,2h−σ} and β<ν(νh+γh−2)hΛ; | (4.5) |
(iii) ES00(Λν,0,0) of discrete COVID-19 model (1.7) is a saddle if (4.4) hold and additionally
ν>max{2h,2h−σ} and ν(νh+γh−2)hΛ<β<ν(ν+γ)Λ, | (4.6) |
or
2h−σ<ν<2h and ν(νh+γh−2)hΛ<β<ν(ν+γ)Λ, | (4.7) |
or
0<ν<min{2h,2h−σ} and β<ν(νh+γh−2)hΛ, | (4.8) |
or
2h−σ<ν<2h and β<ν(νh+γh−2)hΛ; | (4.9) |
(iv) ES00(Λν,0,0) of discrete COVID-19 model (1.7) is non-hyperbolic if
ν=2h, | (4.10) |
or
β=ν(νh+γh−2)hΛ, | (4.11) |
or
ν=2h−σ. | (4.12) |
Proof. (i) By stability theory ES00(Λν,0,0) of discrete COVID-19 model (1.7) is a sink if eigenvalues of J|ES00(Λν,0,0) which are depicted in (4.2) satisfying |λ1,2,3|<1. So if |λ1|=|1−νh|<1, |λ2|=|1−νh−γh+βΛhν|<1 and |λ3|=|1−νh−σh|<1 then straightforward manipulation implies that ES00(Λν,0,0) of discrete COVID-19 model (1.7) is a sink if 0<ν<min{2h,2h−σ}, ν(νh+γh−2)hΛ<β<ν(ν+γ)Λ with σ<2h and ν>2h−γ. In similarly way one can prove conclusions (ii)-(iv).
In order to find local dynamic behavior of discrete COVID-19 epidemic model (1.7) about interior equilibrium solution: E+SIR(ν+γβ,(ν+σ)(βΛ−ν2−νγ)β(ν2+(σ+γ)ν),γ(βΛ−ν2−νγ)β(ν2+(σ+γ)ν)) following theorem is utilized which shows the fact that all roots of the characteristic equation of J|E+SIR(ν+γβ,(ν+σ)(βΛ−ν2−νγ)β(ν2+(σ+γ)ν),γ(βΛ−ν2−νγ)β(ν2+(σ+γ)ν)) whose absolute value less than one ([13], Theorem 1.2.3).
Theorem 4.2. The necessary and sufficient conditions for roots of following third-degree polynomial
P(λ)=λ3+H1λ2+H2λ+H3, | (4.13) |
satisfying |λ1,2,3|<1 are
|H1+H3|<1+H2, |H1−3H3|<3−H2, H23+H2−H3H1<1. | (4.14) |
Lemma 4.3. E+SIR(ν+γβ,(ν+σ)(βΛ−ν2−νγ)β(ν2+(σ+γ)ν),γ(βΛ−ν2−νγ)β(ν2+(σ+γ)ν)) of discrete COVID-19 epidemic model (1.7) is a stable if
|H1+H3|<1+H2, |H1−3H3|<3−H2, H23+H2−H3H1<1, | (4.15) |
where
H1=−3+hν+hσ+βΛhν+βΛhσ−νγσhγν+ν2+σν,H2=1ν2+σν+γν[3γν−2βΛh(ν+σ)+3ν2+βΛν2h2+βΛσνh2−γν2h−ν3h+2νγσh−ν2γσh2−h2(γ+ν)(−βΛ+ν2+νγ)(ν+σ)+βΛνσh2+βΛσ2h2−γνσh−ν2σh−νγσ2h2+3σν−ν2hσ−σ2hν−h(σ+ν)(γν+ν2+σν)],H3=1ν2+σν+γν[βΛνh+βΛσh−γν−ν2(1+βΛh2)−βΛσνh2+γν2h−νγσh+ν3h+ν2h2γσ−h2(γ+σ)(ν+σ)(−βΛ+ν2+νγ)(−1+hν+σh)−βΛσh2(ν+σ)+γνσh+ν2σh+νγσ2h2−h3σγ(ν+σ)(βΛ−ν2−νγ)−σν(1−νh−σh)]. | (4.16) |
Proof. About E+SIR(ν+γβ,(ν+σ)(βΛ−ν2−νγ)β(ν2+(σ+γ)ν),γ(βΛ−ν2−νγ)β(ν2+(σ+γ)ν)), Eq (3.3) becomes
J|E+SIR=(ν2+σν+γν−βΛνh−βΛσh+νγσhν2+σν+γν−h(ν+γ)σhh(ν+σ)(βΛ−ν2−νγ)ν2+σν+γν100γh1−hν−hσ). | (4.17) |
The characteristic polynomial of J|E+SIR(ν+γβ,(ν+σ)(βΛ−ν2−νγ)β(ν2+(σ+γ)ν),γ(βΛ−ν2−νγ)β(ν2+(σ+γ)ν)) about interior equilibrium solution E+SIR(ν+γβ,(ν+σ)(βΛ−ν2−νγ)β(ν2+(σ+γ)ν),γ(βΛ−ν2−νγ)β(ν2+(σ+γ)ν)) of discrete COVID-19 epidemic model (1.7) is
P(λ)=λ3+H1λ2+H2λ+H3, | (4.18) |
where H1,H2 and H3 are depicted in Eq (4.16). Now Theorem 4.2 implies that interior equilibrium solution E+SIR(ν+γβ,(ν+σ)(βΛ−ν2−νγ)β(ν2+(σ+γ)ν),γ(βΛ−ν2−νγ)β(ν2+(σ+γ)ν)) of discrete COVID-19 epidemic model (1.7) is a sink if |H1+H3|<1+H2, |H1−3H3|<3−H2 and H23+H2−H3H1<1.
Motivated from existing study [14], in this section it is explored that equilibrium solutions: ES00(Λν,0,0) and E+SIR(ν+γβ,(ν+σ)(βΛ−ν2−νγ)β(ν2+(σ+γ)ν),γ(βΛ−ν2−νγ)β(ν2+(σ+γ)ν)) of discrete COVID-19 epidemic model (1.7) are periodic points with period-n.
Theorem 5.1. ES00(Λν,0,0) and E+SIR(ν+γβ,(ν+σ)(βΛ−ν2−νγ)β(ν2+(σ+γ)ν),γ(βΛ−ν2−νγ)β(ν2+(σ+γ)ν)) of discrete COVID-19 epidemic model (1.7) are periodic points of prime period-1.
Proof. From model (1.7) one denotes
P(S,I,R):=(f(S,I,R),g(S,I,R),h(S,I,R)), | (5.1) |
where f(S,I,R), g(S,I,R) and h(S,I,R) are depicted in Eq (3.4). From Eq (5.1) the computation yields
P|ES00(Λν,0,0)=ES00(Λν,0,0),P|E+SIR(ν+γβ,(ν+σ)(βΛ−ν2−νγ)β(ν2+(σ+γ)ν),γ(βΛ−ν2−νγ)β(ν2+(σ+γ)ν))=E+SIR(ν+γβ,(ν+σ)(βΛ−ν2−νγ)β(ν2+(σ+γ)ν),γ(βΛ−ν2−νγ)β(ν2+(σ+γ)ν)). | (5.2) |
Therefore Eq (5.2) implies that ES00(Λν,0,0) and E+SIR(ν+γβ,(ν+σ)(βΛ−ν2−νγ)β(ν2+(σ+γ)ν),γ(βΛ−ν2−νγ)β(ν2+(σ+γ)ν)) of discrete COVID-19 epidemic model (1.7) are periodic points of prime period-1.
Theorem 5.2. Equilibrium solution: ES00(Λν,0,0) of discrete COVID-19 epidemic model (1.7) is a periodic point of period-n.
Proof. From Eq (5.1) we have
P2=((1−νh)f(S,I,R)+hΛ− βhf(S,I,R)g(S,I,R)+hσh(S,I,R), (1−νh−γh)g(S,I,R)+ βhf(S,I,R)g(S,I,R), (1−hν−hσ)h(S,I,R)+hγg(S,I,R))⇒P2|ES00(Λν,0,0)=ES00(Λν,0,0),P3=((1−νh)f2(S,I,R)+hΛ− βhf2(S,I,R)g2(S,I,R) +hσh2(S,I,R), (1−νh−γh)g2(S,I,R)+ βhf2(S,I,R)g2(S,I,R), (1−hν−hσ)h2(S,I,R)+hγg2(S,I,R))⇒P3|ES00(Λν,0,0)=ES00(Λν,0,0),⋮Pn=((1−νh)fn−1(S,I,R)+ hΛ−βhfn−1(S,I,R)gn−1(S,I,R) +hσhn−1(S,I,R), (1−νh−γh)gn−1(S,I,R)+ βhfn−1(S,I,R)gn−1(S,I,R), (1−hν−hσ)hn−1(S,I,R)+hγgn−1(S,I,R))⇒Pn|ES00(Λν,0,0)=ES00(Λν,0,0). | (5.3) |
Equation (5.3) implies that equilibrium solution:ES00(Λν,0,0) of discrete COVID-19 epidemic model (1.7) is a periodic point of period-n.
Theorem 5.3. Equilibrium solution: E+SIR(ν+γβ,(ν+σ)(βΛ−ν2−νγ)β(ν2+(σ+γ)ν),γ(βΛ−ν2−νγ)β(ν2+(σ+γ)ν)) of discrete COVID-19 epidemic model (1.7) is a periodic point of period-n.
Proof. From Eq (5.3) the following computation yields the required statement:
P2|E+SIR(ν+γβ,(ν+σ)(βΛ−ν2−νγ)β(ν2+(σ+γ)ν),γ(βΛ−ν2−νγ)β(ν2+(σ+γ)ν))=E+SIR(ν+γβ,(ν+σ)(βΛ−ν2−νγ)β(ν2+(σ+γ)ν),γ(βΛ−ν2−νγ)β(ν2+(σ+γ)ν)),P3|E+SIR(ν+γβ,(ν+σ)(βΛ−ν2−νγ)β(ν2+(σ+γ)ν),γ(βΛ−ν2−νγ)β(ν2+(σ+γ)ν))=E+SIR(ν+γβ,(ν+σ)(βΛ−ν2−νγ)β(ν2+(σ+γ)ν),γ(βΛ−ν2−νγ)β(ν2+(σ+γ)ν)),⋮Pn|E+SIR(ν+γβ,(ν+σ)(βΛ−ν2−νγ)β(ν2+(σ+γ)ν),γ(βΛ−ν2−νγ)β(ν2+(σ+γ)ν))=E+SIR(ν+γβ,(ν+σ)(βΛ−ν2−νγ)β(ν2+(σ+γ)ν),γ(βΛ−ν2−νγ)β(ν2+(σ+γ)ν)). |
Convergence rate of discrete COVID-19 epidemic model (1.7) is studied for the completeness of this Section.
Theorem 6.1. If {(Sn,In,Rn)} is a positive solution of discrete COVID-19 epidemic model (1.7) such that limn→∞{(Sn,In,Rn)}=ESIR(S,I,R) then
φn=(φ1nφ2nφ3n), | (6.1) |
satisfying the following mathematical relation:
limn→∞n√||φn||=|λ1,2,3J|ESIR(S,I,R)|,limn→∞||φn+1||||φn||=|λ1,2,3J|ESIR(S,I,R)|. | (6.2) |
Proof. It is recalled that if {(Sn,In,Rn)} is a positive solution of COVID-19 epidemic model (1.7) such that limn→∞{(Sn,In,Rn)}=ESIR(S,I,R), then in order for error terms one has
Sn+1−S=(1−νh−βhI)(Sn−S)−βhSn(In−I)+hσ(Rn−R),In+1−I=hβI(Sn−S)+(1−νh−γh+hβSn)(In−I),Rn+1−R=hγ(In−I)+(1−hν−hσ)(Rn−R). | (6.3) |
Set
φ1n=Sn−S, φ2n=In−I, φ3n=Rn−R. | (6.4) |
In view of Eq (6.4), Eq (6.3) becomes:
φ1n+1=α11φ1n+α12φ2n+α13φ3n,φ2n+1=α21φ1n+α22φ2n,φ3n+1=α32φ2n+α33φ3n, | (6.5) |
where
α11=1−νh−βhI,α12=−βhSn,α13=hσ,α21=hβI,α22=1−νh−γh+hβSn,α32=hγ,α33=1−hν−hσ. | (6.6) |
From Eq (6.6), one has
limn→∞α11=1−νh−βhI,limn→∞α12=−βhS,limn→∞α13=hσ,limn→∞α21=hβI,limn→∞α22=1−νh−γh+hβS,limn→∞α32=hγ,limn→∞α33=1−hν−hσ, | (6.7) |
that is
α11=1−νh−βhI+σ11,α12=−βhS+σ12,α13=hσ+σ13,α21=hβI+σ21,α22=1−νh−γh+hβS+σ22,α32=hγ+σ32,α33=1−hν−hσ+σ33, | (6.8) |
where σ11,σ12,σ13,σ21,σ22,σ32,σ33→0 as n→∞. In view of existing literature [15], one has the following error system:
φn+1=(A+Bn)φn, | (6.9) |
where A=J|ESIR(S,I,R) and Bn=(σ11σ12σ13σ21σ2200σ32σ33). Therefore one has the following limiting system of error terms
(φ1n+1φ2n+1φ3n+1)=(1−νh−βhI−βhShσhβI1−νh−hγ+hβS00hγ1−hν−hσ)(φ1nφ2nφ3n), | (6.10) |
which is same as linearized system of discrete COVID-19 epidemic model (1.7) about ESIR(S,I,R). Particularly Eq (6.10) implies that
(φ1n+1φ2n+1φ3n+1)=(1−νh−βΛhνσh01−hν−hγ+βΛhν00hγ1−hν−hσ)(φ1nφ2nφ3n), | (6.11) |
and
(φ1n+1φ2n+1φ3n+1)=(ν2+σν+γν−βΛνh−βΛσh+νγσhν2+σν+γν−h(ν+γ)σhh(ν+σ)(βΛ−ν2−νγ)ν2+σν+γν100γh1−hν−hσ)(φ1nφ2nφ3n), | (6.12) |
which are same as respective linearized system obtained at equilibrium solution ES00(Λν,0,0) and E+SIR(ν+γβ,(ν+σ)(βΛ−ν2−νγ)β(ν2+(σ+γ)ν),γ(βΛ−ν2−νγ)β(ν2+(σ+γ)ν)) of the discrete COVID-19 epidemic model (1.7).
The bifurcation analysis about ES00(Λν,0,0) and E+SIR(ν+γβ,(ν+σ)(βΛ−ν2−νγ)β(ν2+(σ+γ)ν),γ(βΛ−ν2−νγ)β(ν2+(σ+γ)ν)) of the discrete COVID-19 epidemic model (1.7) are explored deeply in the section by bifurcation theory [16,17].
From (4.2) the computation yields λ1|(4.10)=−1 but λ2,3=−1−γh+βΛh22,−1−σh≠1 or −1, which implies that discrete COVID-19 epidemic model (1.7) may undergo flip bifurcation if (Λ,β,γ,ν,σ,h) located in the set:
F|ES00(Λν,0,0)={(Λ,β,γ,ν,σ,h):ν=2h}. | (7.1) |
The following Theorem guarantees the fact that if (Λ,β,γ,ν,σ,h)∈F|ES00(Λν,0,0) then discrete COVID-19 epidemic model (1.7) does not undergo flip bifurcation.
Theorem 7.1. If (Λ,β,γ,ν,σ,h)∈F|ES00(Λν,0,0) then discrete COVID-19 epidemic model (1.7) does not undergo flip bifurcation.
Proof. Since discrete COVID-19 epidemic model (1.7) is invariant with respect to I=R=0. Therefore in order to determine bifurcation, discrete COVID-19 epidemic model (1.7) is restricted on I=R=0, where it becomes
Sn+1=(1−νh)Sn+hΛ. | (7.2) |
From Eq (7.2) one denotes the map
f(S):=(1−νh)S+hΛ. | (7.3) |
Now if ν=ν∗=2h and S=S∗=Λν then from Eq (7.3) one gets
∂f∂S|ν=ν∗=2h, S=S∗=Λν=−1, | (7.4) |
∂f∂ν|ν=ν∗=2h, S=S∗=Λν=−hΛν≠0, | (7.5) |
and
∂2f∂S2|ν=ν∗=2h, S=S∗=Λν=0. | (7.6) |
It is noted that the condition obtained in Eq (7.6) violates the non-degenerate condition for the existence of flip bifurcation and hence one can say that discrete COVID-19 epidemic model (1.7) does not undergo flip bifurcation if (Λ,β,γ,ν,σ,h)∈F|ES00(Λν,0,0).
By utilizing explicit criterion (without finding eigenvalues), we will explore hopf and flip bifurcations by choosing h as a bifurcation parameter about E+SIR(ν+γβ,(ν+σ)(βΛ−ν2−νγ)β(ν2+(σ+γ)ν),γ(βΛ−ν2−νγ)β(ν2+(σ+γ)ν)) of discrete COVID-19 epidemic model (1.7) in this section.
By using following explicit criterion[18], hopf bifurcation for the discrete COVID-19 epidemic model (1.7) about E+SIR(ν+γβ,(ν+σ)(βΛ−ν2−νγ)β(ν2+(σ+γ)ν),γ(βΛ−ν2−νγ)β(ν2+(σ+γ)ν)) is explored.
Lemma 7.2. Consider the following n-dimensional discrete dynamical system:
Xn+1=fh(Xn), | (7.7) |
where h∈R is considered as a bifurcation parameter. Moreover characteristic polynomial of J|X about X of n-dimensional discrete dynamical system, which is depicted in system (7.7), is
P(λ)=λn+H1λn−1+H2λn−2+⋯+Hn. | (7.8) |
Now considering the determinants: Δ±0(h)=1, Δ±1(h),⋯,Δ±n(h), which can be expressed as
Δ±j(h)=|(1H1H2⋯Hj−101H1⋯Hj−2001⋯Hj−3⋯⋯⋯⋯⋯000⋯1)±(Hn−j+1Hn−j+2⋯Hn−1HnHn−j+2Hn−j+3⋯Hn0⋯⋯⋯⋯⋯Hn−1Hn⋯00Hn0⋯00)|, | (7.9) |
where j=1,⋯,n. Furthermore, hopf bifurcation occurs at critical value h=h0 if following parametric conditions hold:
Γ1: Eigenvalue assignment: Ph0(1)>0, (−1)nPh0(−1)>0, Δ−n−1(h0)=0, Δ+n−1(h0)>0, Δ±j(h0)>0 where j=n−3,n−5,⋯,1 (or 2), when n is even (or odd, respectively).
Γ2: Transversality condition: ddhΔ−n−1(h0)≠0.
Γ3: Nonresonance condition: cos(2πl)≠1−0.5Ph(1)Δ−n−3(h0)Δ+n−2(h0) or resonance condition cos(2πl)=1−0.5Ph(1)Δ−n−3(h0)Δ+n−2(h0), where l=3,4,⋯.
Theorem 7.3. If
1−H2+H3(H1−H3)=0,1+H2−H3(H1+H3)>0,1+H1+H2+H3>0,1−H1+H2−H3>0,ddh(1−H2+H3(H1−H3))|h=h0≠0,cos2πl≠1−1+H1+H2+H32(1+H3), l=3,4,⋯, | (7.10) |
then about E+SIR(ν+γβ,(ν+σ)(βΛ−ν2−νγ)β(ν2+(σ+γ)ν),γ(βΛ−ν2−νγ)β(ν2+(σ+γ)ν)) discrete COVID-19 epidemic model (1.7) undergoes a hopf bifurcation at a critical value h0 where H1,H2,H3 are depicted in Eq (4.16) and h0 is the real root of 1−H2(h)+H3(h)(H1(h)−H3(h))=0.
Proof. By utilizing Lemma 7.2 for n=3, one gets:
Δ−2(h)=1−H2+H3(H1−H3)=0,Δ+2(h)=1+H2−H3(H1+H3)>0,Ph(1)=1+H1+H2+H3>0,(−1)3Ph(−1)=1−H1+H2−H3>0,ddh(Δ−2(h))|h=h0=ddh(1−H2+H3(H1−H3))|h=h0≠0. | (7.11) |
Finally
1−0.5Ph(1)Δ−0(h)Δ+1(h)=1−1+H1+H2+H32(1+H3). |
By using following explicit criterion [18,19], flip bifurcation for the discrete COVID-19 epidemic model (1.7) about E+SIR(ν+γβ,(ν+σ)(βΛ−ν2−νγ)β(ν2+(σ+γ)ν),γ(βΛ−ν2−νγ)β(ν2+(σ+γ)ν)) by choosing h as a bifurcation parameter is explored.
Lemma 7.4. Consider the system (7.7) with h∈R is a bifurcation parameter. Moreover, characteristic polynomial of J|X about X of system (7.7) is of the form, which is depicted in Eq (7.8). Now considering the determinants: Δ±0(h)=1, Δ±1(h),⋯,Δ±n(h), which are depicted in Eq (7.9) and j=1,⋯,n. Furthermore flip bifurcation occurs at critical value h=h0 if following parametric conditions hold:
Γ1: Eigenvalue assignment: Ph0(−1)=0, Ph0(1)>0, Δ±n−1(h0)>0, Δ±j(h0)>0 where j=n−3,n−5,⋯,1 (or 2), when n is even (or odd, respectively).
Γ2: Transversality condition: n∑i=1(−1)n−iH′in∑i=1(−1)n−i(n−i+1)Hi−1≠0 where H′i represents the derivative w.r.t h at h=h0.
Theorem 7.5. If
1−H2+H3(H1−H3)>0,1+H2−H3(H1+H3)>0,1+H1+H2+H3>0,1−H1+H2−H3=0,1±H3>0,H′1−H′2+H′33−2H1+H2≠0, | (7.12) |
then about E+SIR(ν+γβ,(ν+σ)(βΛ−ν2−νγ)β(ν2+(σ+γ)ν),γ(βΛ−ν2−νγ)β(ν2+(σ+γ)ν)) discrete COVID-19 epidemic model (1.7) undergoes a flip bifurcation at a critical value h0, where h0 is the real root of 1−H1(h)+H2(h)−H3(h)=0.
Proof. By utilizing Lemma 7.4 for n=3, one gets:
Δ−2(h)=1−H2+H3(H1−H3)>0,Δ+2(h)=1+H2−H3(H1+H3)>0,Ph0(1)=1+H1+H2+H3>0,Ph0(−1)=1−H1+H2−H3=0,Δ±j=1±H3>0,3∑i=1(−1)3−iH′i3∑i=1(−1)3−i(3−i+1)Hi−1=H′1−H′2+H′33−2H1+H2≠0. | (7.13) |
In this Section, feedback control strategy is utilized in order to study chaos in discrete COVID-19 epidemic model (1.7) about interior equilibrium solution: E+SIR(ν+γβ,(ν+σ)(βΛ−ν2−νγ)β(ν2+(σ+γ)ν),γ(βΛ−ν2−νγ)β(ν2+(σ+γ)ν)). By utilizing feedback control strategy, discrete COVID-19 epidemic model (1.7) takes the form
Sn+1=hΛ+(1−νh)Sn−βhSnIn+hσRn+δ(Sn−S),In+1=(1−νh−γh)In+hβSnIn+δ(In−I),Rn+1=(1−hν−hσ)Rn+hγIn+δ(Rn−R), | (8.1) |
with δ is chosen as the control parameter. The JC|E+SIR(ν+γβ,(ν+σ)(βΛ−ν2−νγ)β(ν2+(σ+γ)ν),γ(βΛ−ν2−νγ)β(ν2+(σ+γ)ν)) about interior equilibrium solution E+SIR(ν+γβ,(ν+σ)(βΛ−ν2−νγ)β(ν2+(σ+γ)ν),γ(βΛ−ν2−νγ)β(ν2+(σ+γ)ν)) of controlled discrete COVID-19 epidemic model (8.1) is
J|E+SIR=(1+νγσh−βΛh(ν+σ)ν2+σν+γν+δ−h(ν+γ)σhh(ν+σ)(βΛ−ν2−νγ)ν2+σν+γν1+δ00γh1−hν−hσ+δ), | (8.2) |
the characteristic polynomial of JC|E+SIR(ν+γβ,(ν+σ)(βΛ−ν2−νγ)β(ν2+(σ+γ)ν),γ(βΛ−ν2−νγ)β(ν2+(σ+γ)ν)) about interior equilibrium solution E+SIR(ν+γβ,(ν+σ)(βΛ−ν2−νγ)β(ν2+(σ+γ)ν),γ(βΛ−ν2−νγ)β(ν2+(σ+γ)ν)) is
P(λ)=λ3+H∗1λ2+H∗2λ+H∗3, | (8.3) |
where
H∗1=βhΛ(σ+ν)+ν{γ(−3−3δ+hν)+(σ+ν)(−3−3δ+h(σ+ν))}ν(γ+σ+ν),H∗2=1+4δ+3δ2−2hδσ−hν−2hδν−h2(γ+ν)(σ+ν)(−βΛ+ν(γ+ν))ν(γ+σ+ν)+{2+2δ−h(σ+ν)}{−βhΛ(σ+ν)+ν(γ+σ+hγσ+ν)}ν(γ+σ+ν),H∗3=−1+δ−hνν(γ+σ+ν)[βhΛ(σ+ν){−1−δ+h(γ+σ+ν)}+ν{−h2γ2(σ+ν)−(σ+ν)(1+δ+hσ)(−1−δ+h(σ+ν))+γ(1+δ(2+δ)−h2(σ+ν)(σ+2ν))}]. | (8.4) |
Based on linear stability theory the local dynamics of controlled discrete COVID-19 epidemic model (8.1) about E+SIR(ν+γβ,(ν+σ)(βΛ−ν2−νγ)β(ν2+(σ+γ)ν),γ(βΛ−ν2−νγ)β(ν2+(σ+γ)ν)) can be stated as following Lemma:
Lemma 8.1. E+SIR(ν+γβ,(ν+σ)(βΛ−ν2−νγ)β(ν2+(σ+γ)ν),γ(βΛ−ν2−νγ)β(ν2+(σ+γ)ν)) of controlled discrete COVID-19 epidemic model (8.1) is a sink if
|H∗1+H∗3|<1+H∗2, |H∗1−3H∗3|<3−H∗2, H∗32+H∗2−H∗3H∗1<1, | (8.5) |
where H∗1,H∗2 and H∗3 are depicted in Eq (8.4).
Proof. Since JC|E+SIR(ν+γβ,(ν+σ)(βΛ−ν2−νγ)β(ν2+(σ+γ)ν),γ(βΛ−ν2−νγ)β(ν2+(σ+γ)ν)) about interior equilibrium solution E+SIR(ν+γβ,(ν+σ)(βΛ−ν2−νγ)β(ν2+(σ+γ)ν),γ(βΛ−ν2−νγ)β(ν2+(σ+γ)ν)) of controlled discrete COVID-19 epidemic model (8.1) has characteristics polynomial which is depicted in model (8.3). By Theorem 4.2 E^+_{SIR}\left(\frac{\nu+\gamma}{\beta}, \frac{\left(\nu+\sigma\right) \left(\beta \Lambda-{\nu}^2-\nu \gamma\right)}{\beta \left({\nu}^2+\left(\sigma+\gamma\right) \nu\right)}, \frac{\gamma \left(\beta \Lambda-{\nu}^2-\nu \gamma\right)}{\beta \left({\nu}^2+\left(\sigma+\gamma\right) \nu\right)}\right) of controlled discrete COVID-19 epidemic model (8.1) is a sink if |\mathcal{H}_1^{*}+\mathcal{H}_3^{*}| < 1+\mathcal{H}_2^{*} , |\mathcal{H}_1^{*}-3\mathcal{H}_3^{*}| < 3-\mathcal{H}_2^{*} and {\mathcal{H}_3^{*}}^2+\mathcal{H}_2^{*}-\mathcal{H}_3^{*}\mathcal{H}_1^{*} < 1 where \mathcal{H}_1^{*} , \mathcal{H}_2^{*} and \mathcal{H}_3^{*} are depicted in Eq (8.4).
Theoretical results are illustrated numerically in this section. In this regard, following cases are presented to discuss the correctness of obtained theoretical results about equilibrium solutions for discrete COVID-19 epidemic model (1.7) :
Case Ⅰ: If h = 0.565, \ \beta = 3, \ \Lambda = 0.4, \ \nu = 0.2, \ \sigma = 2, \ \gamma = 0.4 then from Eq (4.13) computation yields \left|\mathcal{H}_1 + \mathcal{H}_3\right| = 0.0791966397692303 < 1+\mathcal{H}_2 = 0.3493437692307695 , \left|\mathcal{H}_1-3\mathcal{H}_3\right| = 3.512871619153845 < 3-\mathcal{H}_2 = 3.6506562307692305 and \mathcal{H}_3^2 + \mathcal{H}_2 - \mathcal{H}_3\mathcal{H}_1 = 0.8910931323439 < 1 , which implies that equilibrium solution E^+_{SIR}\left(0.2, 1.5230769230769232, 0.27692307692307694\right) of discrete COVID-19 epidemic model (1.7) is a sink. In this case plots for discrete COVID-19 epidemic model (1.7) with initial values (S_0, I_0, R_0) = (0.84, 0.84, 0.0082) are drawn in Figure 1 which show that interior equilibrium solution E^+_{SIR}\left(0.2, 1.5230769230769232, 0.27692307692307694\right) is a sink.
Case Ⅱ: Now in this case it is proved that at h = 0.9645432692307686 , discrete COVID-19 epidemic model (1.7) undergoes a hopf bifurcation if \beta = 3, \ \Lambda = 0.4, \ \nu = 0.2, \ \sigma = 0.42, \ \gamma = 4.5 and h\in[0.1, 1.9] with initial values (S_0, I_0, R_0) = (0.94, 0.00084, 0.000782) . If \beta = 3, \ \Lambda = 0.4, \ \nu = 0.2, \ \sigma = 0.42, \ \gamma = 4.5 and h = 0.9645432692307686 then from Eq (4.18) one gets:
\begin{equation} \lambda^3-2.057234309269832\lambda^2 +2.008979566986039\lambda-0.807091346153846 = 0, \end{equation} | (9.1) |
whose roots are \lambda_{1, 2} = 0.625071481557993\pm0.7805675133791408\iota, \lambda_3 = 0.8070913461538463 where |\lambda_{1, 2}| = |0.625071481557993\pm0.7805675133791408\iota| = 1 . This implies that for said parametric values the eigenvalues criterion for the existence of hopf bifurcation holds, and hence discrete COVID-19 epidemic model (1.7) may undergo hopf bifurcation. In the rest of simulation, it is proved that discrete COVID-19 epidemic model (1.7) must undergo hopf bifurcation. For instance, if \beta = 3, \ \Lambda = 0.4, \ \nu = 0.2, \ \sigma = 0.42, \ \gamma = 4.5 and h = 0.9645432692307686 then from Eq (7.10) the computation yields
\begin{eqnarray} \begin{split} 1 -\mathcal{H}_2 +\mathcal{H}_3(\mathcal{H}_1 -\mathcal{H}_3)& = 0, \\ 1 + \mathcal{H}_2 - \mathcal{H}_3(\mathcal{H}_1 + \mathcal{H}_3)& = 0.6972071179271455 > 0, \\ 1 + \mathcal{H}_1 + \mathcal{H}_2 + \mathcal{H}_3& = 0.14465391156236085 > 0, \\ 1 - \mathcal{H}_1 + \mathcal{H}_2 - \mathcal{H}_3& = 5.8733052224097175 > 0, \\ \frac{d}{dh}\left(1 - \mathcal{H}_2 + \mathcal{H}_3(\mathcal{H}_1 - \mathcal{H}_3)\right)|_{h = 0.9645432692307686}& = 0.499543845441214\neq 0, \\ 1- \frac{1 + \mathcal{H}_1 + \mathcal{H}_2 + \mathcal{H}_3}{2(1+\mathcal{H}_3)}& = 0.6250714815579933. \end{split} \end{eqnarray} | (9.2) |
Moreover \cos\frac{2\pi}{l} = 0.6250714815579933 implies l = \pm7.0158253519640486 . Thus from Eq (9.2) all conditions of Theorem 7.3 hold and hence it can be concluded that discrete COVID-19 epidemic model (1.7) undergoes hopf bifurcation. So hopf bifurcation diagram and maximum lypunov exponents are drawn in Figure 2. Finally some phase portraits for discrete COVID-19 epidemic model (1.7) are also plotted for certain values of h with initial values (S_0, I_0, R_0) = (0.94, 0.00084, 0.000782) in Figure 3.
Case Ⅲ: Now in this case it is proved that at h = 0.3882629532979648 , discrete COVID-19 epidemic model (1.7) undergoes a flip bifurcation if \beta = 3, \ \Lambda = 0.4, \ \nu = 0.2, \ \sigma = 0.2, \ \gamma = 0.043 and h\in[0.01, 1.5] with initial values (S_0, I_0, R_0) = (0.4, 0.6, 0.7) . If \beta = 3, \ \Lambda = 0.4, \ \nu = 0.2, \ \sigma = 0.2, \ \gamma = 0.043 and h = 0.3882629532979648 , then from Eq (4.18) one gets:
\begin{equation} \lambda^3-0.7487760229319358\lambda^2 -0.9865217321810046\lambda+0.7622542907509305 = 0, \end{equation} | (9.3) |
whose roots are \lambda_{1} = -1 but \lambda_{2, 3} = 0.8264286135915285, 0.922347409340407\not = 1\ \mathrm{or} \ -1 . This implies that for said parametric values the eigenvalues criterion for the existence of flip bifurcation holds, and hence discrete COVID-19 epidemic model (1.7) may undergo flip bifurcation. In the rest of simulation it is proved that discrete COVID-19 epidemic model (1.7) must undergo flip bifurcation. For instance if \beta = 3, \ \Lambda = 0.4, \ \nu = 0.2, \ \sigma = 0.2, \ \gamma = 0.043 and h = 0.3882629532979648 , then from Eq (7.12) the computation yields
\begin{eqnarray} \begin{split} 1 -\mathcal{H}_2 +\mathcal{H}_3(\mathcal{H}_1 -\mathcal{H}_3)& = 0.8347323921215153 > 0, \\ 1 + \mathcal{H}_2 - \mathcal{H}_3(\mathcal{H}_1 + \mathcal{H}_3)& = 0.0032044003420764813 > 0, \\ 1 + \mathcal{H}_1 + \mathcal{H}_2 + \mathcal{H}_3& = 0.026956535637990164 > 0, \\ 1 - \mathcal{H}_1 + \mathcal{H}_2 - \mathcal{H}_3& = 0, \\ 1+\mathcal{H}_3& = 1.7622542907509304 > 0, \\ 1-\mathcal{H}_3& = 0.23774570924906946 > 0, \\ \frac{\mathcal{H}_1^{'} - \mathcal{H}_2^{'} + \mathcal{H}_3^{'}}{3-2\mathcal{H}_1+\mathcal{H}_2}& = -1.0314039405572908\neq0. \end{split} \end{eqnarray} | (9.4) |
Thus from Eq (9.4) all conditions of Theorem 7.5 hold and hence it can be concluded that discrete COVID-19 epidemic model (1.7) undergoes flip bifurcation. So flip bifurcation diagrams and maximum lypunov exponents are drawn in Figure 4. Finally, phase portraits for discrete COVID-19 epidemic model (1.7) are also plotted for h = 0.38, 0.383 with initial values (S_0, I_0, R_0) = (0.4, 0.6, 0.7) in Figure 5.
Case Ⅳ: The numerical simulation will be provided in order to verify result of Lemma 8.1 for controlled discrete COVID-19 epidemic model (8.1). If h = 0.5643, \ \beta = 3, \ \Lambda = 0.22, \ \nu = 0.2, \ \sigma = 2, \ \gamma = 0.43, \delta = 0.22 then from Eq (8.5) computation yields |\mathcal{H}_1^{*}+\mathcal{H}_3^{*}| = 1.9887516175217854 < 1+\mathcal{H}_2^{*} = 2.062885326736867, |\mathcal{H}_1^{*}-3\mathcal{H}_3^{*}| = 1.4779003281575345 < 3-\mathcal{H}_2^{*} = 1.937114673263 and {\mathcal{H}_3^{*}}^2+\mathcal{H}_2^{*}-\mathcal{H}_3^{*}\mathcal{H}_1^{*} = 0.8415173747084458 < 1 which implies that interior equilibrium solution of controlled discrete COVID-19 epidemic model (8.1) is a sink. In this case plots for discrete COVID-19 epidemic model (1.7) with initial values (S_0, I_0, R_0) = (0.84, 0.84, 0.0082) are drawn in Figure 6, which show that interior equilibrium solution is a sink.
Case Ⅴ: Finally, we will fit real data, obtained from published materials for three different countries France, Italy and United Kingdom, to our under consideration discrete epidemic model (1.7) in order for the effectiveness of our mathematical analysis regarding stability. The collected real data is depicted in Table 1 whereas corresponding dynamical analysis of discrete epidemic model (1.7) of France, Italy and the United Kingdom are respectively given in Figures 7–9. In Figure 7(a) the plot of susceptible population show a curve that represent the number of susceptible individuals increase rapidly with the passage of time. After peak, the number of susceptible individuals decrease and attain a steady state whereas Figure 7(b) shows a curve that represent the infection rate is sharply increase with the passage of time and reach to its maximum level. Finally, Figure 7(c) represent that curve goes upward slowly which means that the number of recovered individuals were small initially due to less medical care and facilities but with the passage of time the recovery rate increases due to proper medication and vaccination. Similar results can be interpreted for real data plotted in Figures 8 and 9 for Italy and United Kingdom, respectively.
Parameter | Interpretation | France | Italy | United Kingdom | Source |
\Lambda | Rate in S through migration | 0.21 | 0.21 | 0.21 | Estimated |
\beta | Rate to join S to I | 0.926 | 0.571 | 0.999 | [20,21] |
\nu | Death rate due to virus | 3.3616\times 10^{-5} | 3.2612\times 10^{-5} | 3.2929\times 10^{-5} | [23] |
\gamma | Recovery rate | 0.0735 | 0.076 | 0.0714 | [21,22] |
\sigma | Rate of deteriorate in health | 0.0249 | 0.0338 | 0.0294 | [23] |
h | Step size | 0.2 | 0.2 | 0.2 | Estimated |
Beginning from the COVID-19, different mathematicians have proposed mathematical models to predict the cause of COVID-19 pandemic starting from Wuhan city of China in December 2019. For instance, few newly proposed mathematical models regarding COVID-19 pandemic are quoted in references [1,2,3,4,5,6,7]. In the references [1,2,3,4,5,6,7] and more study indicates that different authors predict the case of this disease by mathematical models representing systems of differential or difference equations, and moreover based on date analysis and mathematical analysis authors have explored the effect of lockdown and medical resources on the COVID-19 transformation in Wuhan city of China. So motivation from aforementioned studies, in this paper we have explored local dynamical properties, bifurcation and control in a discrete-time COVID-19 epidemic model in \mathbb{R}_+^3 . Algebraically, it is proved that discrete COVID-19 epidemic model (1.7) has boundary equilibrium solution: E_{S00}\left(\frac{\Lambda}{\nu}, 0, 0\right) \ \forall \ h, \ \beta, \ \Lambda, \ \nu, \ \sigma, \ \gamma > 0 but it has has interior equilibrium solution: E^+_{SIR}\left(\frac{\nu+\gamma}{\beta}, \frac{\left(\nu+\sigma\right) \left(\beta \Lambda-{\nu}^2-\nu \gamma\right)}{\beta \left({\nu}^2+\left(\sigma+\gamma\right) \nu\right)}, \frac{\gamma \left(\beta \Lambda-{\nu}^2-\nu \gamma\right)}{\beta \left({\nu}^2+\left(\sigma+\gamma\right) \nu\right)}\right) if \beta > \frac{\nu \left(\nu+\gamma\right)}{\Lambda} . Further local dynamical characteristics with topological classifications about equilibrium solutions E_{S00}\left(\frac{\Lambda}{\nu}, 0, 0\right) and E^+_{SIR}\left(\frac{\nu+\gamma}{\beta}, \frac{\left(\nu+\sigma\right) \left(\beta \Lambda-{\nu}^2-\nu \gamma\right)}{\beta \left({\nu}^2+\left(\sigma+\gamma\right) \nu\right)}, \frac{\gamma \left(\beta \Lambda-{\nu}^2-\nu \gamma\right)}{\beta \left({\nu}^2+\left(\sigma+\gamma\right) \nu\right)}\right) of discrete COVID-19 epidemic model (1.7) are explored. It is investigated that E_{S00}\left(\frac{\Lambda}{\nu}, 0, 0\right) of discrete COVID-19 epidemic model (1.7) is a sink if 0 < \nu < \min\left\{\frac{2}{h}, \frac{2}{h}-\sigma\right\} \ \ \mathrm{and} \ \ \frac{\nu (\nu h+\gamma h-2)}{h \Lambda} < \beta < \frac{\nu (\nu+\gamma)}{\Lambda} with \sigma < \frac{2}{h}\ \ \mathrm{and}\ \ \nu > \frac{2}{h}-\gamma ; source if (4.4) holds and additionally \nu > \max\left\{\frac{2}{h}, \frac{2}{h}-\sigma\right\} \ \ \mathrm{and} \ \beta < \frac{\nu (\nu h+\gamma h-2)}{h \Lambda} ; saddle if (4.4) holds and additionally \nu > \max\left\{\frac{2}{h}, \frac{2}{h}-\sigma\right\} \ \ \mathrm{and} \ \frac{\nu (\nu h+\gamma h-2)}{h \Lambda} < \beta < \frac{\nu (\nu+\gamma)}{\Lambda}, or \frac{2}{h}-\sigma < \nu < \frac{2}{h}\ \mathrm{and} \ \ \frac{\nu (\nu h+\gamma h-2)}{h \Lambda} < \beta < \frac{\nu (\nu+\gamma)}{\Lambda}, or 0 < \nu < \min\left\{\frac{2}{h}, \frac{2}{h}-\sigma\right\}\ \ \mathrm{and} \ \beta < \frac{\nu (\nu h+\gamma h-2)}{h \Lambda}, or \frac{2}{h}-\sigma < \nu < \frac{2}{h}\ \mathrm{and} \ \ \beta < \frac{\nu (\nu h+\gamma h-2)}{h \Lambda} ; non-hyperbolic if \nu = \frac{2}{h}, or \beta = \frac{\nu (\nu h+\gamma h-2)}{h \Lambda}, or \nu = \frac{2}{h}-\sigma , and moreover interior equilibrium solution E^+_{SIR}\left(\frac{\nu+\gamma}{\beta}, \frac{\left(\nu+\sigma\right) \left(\beta \Lambda-{\nu}^2-\nu \gamma\right)}{\beta \left({\nu}^2+\left(\sigma+\gamma\right) \nu\right)}, \frac{\gamma \left(\beta \Lambda-{\nu}^2-\nu \gamma\right)}{\beta \left({\nu}^2+\left(\sigma+\gamma\right) \nu\right)}\right) of discrete COVID-19 epidemic model (1.7) is a sink if \left|\mathcal{H}_1 + \mathcal{H}_3\right| < 1+\mathcal{H}_2, \ \ \left|\mathcal{H}_1-3\mathcal{H}_3\right| < 3-\mathcal{H}_2, \ \ \mathcal{H}_3^2 + \mathcal{H}_2 - \mathcal{H}_3\mathcal{H}_1 < 1 where \mathcal{H}_1, \mathcal{H}_2 and \mathcal{H}_3 are depicted in Eq (4.16). It is shown that E_{S00}\left(\frac{\Lambda}{\nu}, 0, 0\right) and E^+_{SIR}\left(\frac{\nu+\gamma}{\beta}, \frac{\left(\nu+\sigma\right) \left(\beta \Lambda-{\nu}^2-\nu \gamma\right)}{\beta \left({\nu}^2+\left(\sigma+\gamma\right) \nu\right)}, \frac{\gamma \left(\beta \Lambda-{\nu}^2-\nu \gamma\right)}{\beta \left({\nu}^2+\left(\sigma+\gamma\right) \nu\right)}\right) of discrete COVID-19 epidemic model (1.7) are periodic points of period- n . We have also studied convergence rate for discrete COVID-19 epidemic model (1.7). Further, in order to understand dynamics of discrete COVID-19 epidemic model (1.7) deeply, we have studied the possible bifurcation scenarios. It is proved that about E_{S00}\left(\frac{\Lambda}{\nu}, 0, 0\right) there exist no flip bifurcation if (\Lambda, \beta, \gamma, \nu, \sigma, h)\in \mathcal{F}|_{E_{S00}\left(\frac{\Lambda}{\nu}, 0, 0\right)} = \left\{(\Lambda, \beta, \gamma, \nu, \sigma, h):\nu = \frac{2}{h}\right\} , but discrete COVID-19 epidemic model (1.7) undergoes both hopf and flip bifurcations about E^+_{SIR}\left(\frac{\nu+\gamma}{\beta}, \frac{\left(\nu+\sigma\right) \left(\beta \Lambda-{\nu}^2-\nu \gamma\right)}{\beta \left({\nu}^2+\left(\sigma+\gamma\right) \nu\right)}, \frac{\gamma \left(\beta \Lambda-{\nu}^2-\nu \gamma\right)}{\beta \left({\nu}^2+\left(\sigma+\gamma\right) \nu\right)}\right) by choosing h as bifurcation parameter. We have studied hopf and flip bifurcations about E^+_{SIR}\left(\frac{\nu+\gamma}{\beta}, \frac{\left(\nu+\sigma\right) \left(\beta \Lambda-{\nu}^2-\nu \gamma\right)}{\beta \left({\nu}^2+\left(\sigma+\gamma\right) \nu\right)}, \frac{\gamma \left(\beta \Lambda-{\nu}^2-\nu \gamma\right)}{\beta \left({\nu}^2+\left(\sigma+\gamma\right) \nu\right)}\right) of discrete COVID-19 epidemic model (1.7) by utilizing explicit criterion. By feedback control strategy, chaos in discrete COVID-19 epidemic model (1.7) about E^+_{SIR}\left(\frac{\nu+\gamma}{\beta}, \frac{\left(\nu+\sigma\right) \left(\beta \Lambda-{\nu}^2-\nu \gamma\right)}{\beta \left({\nu}^2+\left(\sigma+\gamma\right) \nu\right)}, \frac{\gamma \left(\beta \Lambda-{\nu}^2-\nu \gamma\right)}{\beta \left({\nu}^2+\left(\sigma+\gamma\right) \nu\right)}\right) is also explored. For controlled system (8.1) it is proved that E^+_{SIR}\left(\frac{\nu+\gamma}{\beta}, \frac{(\nu+\sigma) (\beta \Lambda-{\nu}^2-\nu \gamma)}{\beta ({\nu}^2+(\sigma+\gamma) \nu)}, \frac{\gamma (\beta \Lambda-{\nu}^2-\nu \gamma)}{\beta ({\nu}^2+(\sigma+\gamma) \nu)}\right) is a sink if |\mathcal{H}_1^{*}+\mathcal{H}_3^{*}| < 1+\mathcal{H}_2^{*}, \ |\mathcal{H}_1^{*}-3\mathcal{H}_3^{*}| < 3-\mathcal{H}_2^{*} and {\mathcal{H}_3^{*}}^2+\mathcal{H}_2^{*}-\mathcal{H}_3^{*}\mathcal{H}_1^{*} < 1 , where \mathcal{H}_1^{*}, \mathcal{H}_2^{*} and \mathcal{H}_3^{*} are depicted in Eq (8.4). Finally numerically verified theoretical results.
This research is partially supported by the Higher Education Commission of Pakistan.
The authors declare that they have no conflicts of interest regarding the publication of this paper.
[1] | D. Zou, L. Wang, P. Xu, J. Chen, W. Zhang, Q. Gu, Epidemic model guided machine learning for COVID-19 forecasts in the United States, preprint, medRxiv, (2020). doi: 10.1101/2020.05.24.20111989. |
[2] | E. L. Ray, N. Wattanachit, J. Niemi, A. H. Kanji, K. House, E. Y. Cramer, et al., Ensemble forecasts of coronavirus disease 2019 (COVID-19) in the US, preprint, medRxiv, (2020). doi: 10.1101/2020.08.19.20177493. |
[3] | K. Shea, R. K. Borchering, W. J. Probert, E. Howerton, T. L. Bogich, S. Li, et al., COVID-19 reopening strategies at the county level in the face of uncertainty: multiple models for outbreak decision support, preprint, medRxiv, (2020). doi: 10.1101/2020.11.03.20225409. |
[4] |
S. S. Nadim, I. Ghosh, J. Chattopadhyay, Short-term predictions and prevention strategies for COVID-19: a model-based study. Appl. Math. Comput., 404 (2021), 126251. doi: 10.1016/j.amc.2021.126251. doi: 10.1016/j.amc.2021.126251
![]() |
[5] |
M. T. Li, G. Q. Sun, J. Zhang, Y. Zhao, X. Pei, L. Li, et al., Analysis of COVID-19 transmission in Shanxi Province with discrete time imported cases, Math. Biosci. Eng., 17 (2020), 3710–3720. doi: 10.3934/mbe.2020208. doi: 10.3934/mbe.2020208
![]() |
[6] |
H. Tian, Y. Liu, Y. Li, C. H. Wu, B. Chen, M. U. Kraemer, et al., An investigation of transmission control measures during the first 50 days of the COVID-19 epidemic in China, Science, 368 (2020), 638–642. doi: 10.1126/science.abb6105. doi: 10.1126/science.abb6105
![]() |
[7] |
G. Q. Sun, S. F. Wang, M. T. Li, L. Li, J. Zhang, W. Zhang, et al., Transmission dynamics of COVID-19 in Wuhan, China: effects of lockdown and medical resources, Nonlinear Dyn., 101 (2020), 1981–1993. doi: 10.1007/s11071-020-05770-9. doi: 10.1007/s11071-020-05770-9
![]() |
[8] |
A. Tesfaya, T. Saeed, A. Zeb, D. Tesfay, A. Khalafa, J. Brannanc, Dynamics of a stochastic COVID-19 epidemic model with jump-diffusion, Adv. Differ. Equ., 2021 (2021), 1–18. doi: 10.1186/s13662-021-03396-8. doi: 10.1186/s13662-021-03396-8
![]() |
[9] | E. A. Grove, G. Ladas, Periodicities in nonlinear difference equations, Chapman and Hall/CRC, 2004. |
[10] | A. Wikan, Discrete dynamical systems: with an introduction to discrete optimization problems, Bookboon, 2013. |
[11] | S. N. Elaydi, An introduction to difference equations, Springer-Verlag, 1996. |
[12] | M. R. Kulenovic, G. Ladas, Dynamics of second order rational difference equations: with open problems and conjectures, Chapman and Hall/CRC, 2001. |
[13] | E. Camouzis, G. Ladas, Dynamics of third-order rational difference equations with open problems and conjectures, CRC Press, 2007. |
[14] | W. B. Zhang, Discrete dynamical systems, bifurcations and chaos in economics, Elsevier, 2006. |
[15] |
M. Pituk, More on Poincare's and Perron's theorems for difference equations, J. Differ. Equations Appl., 8 (2002), 201-216. doi: 10.1080/10236190211954. doi: 10.1080/10236190211954
![]() |
[16] | J. Guckenheimer, P. Holmes, Nonlinear oscillations, dynamical systems and bifurcation of vector fields New York, Springer-Verlag, 1983. |
[17] | Y. A. Kuznetsov, Elements of applied bifurcation theorey, 3rd edition, Springer-Verlag, 2004. |
[18] |
G. Wen, Criterion to identify hopf bifurcations in maps of arbitrary dimension, Phys. Rev. E, 72 (2005), 026201. doi: 10.1103/PhysRevE.72.026201. doi: 10.1103/PhysRevE.72.026201
![]() |
[19] |
S. Yao, New bifurcation critical criterion of Flip-Neimark-Sacker bifurcations for two-parameterized family of-dimensional discrete systems, Discrete Dyn. Nat. Soc., 2012 (2012), 1–12. doi: 10.1155/2012/264526. doi: 10.1155/2012/264526
![]() |
[20] |
S. Liu, M. Liu, Dynamic analysis of a stochastic SEQIR model and application in the COVID-19 pandemic, Discrete Dyn. Nat. Soc., 2021 (2021). doi: 10.1155/2021/6125064. doi: 10.1155/2021/6125064
![]() |
[21] |
R. Forien, G. Pang, È. Pardoux, Estimating the state of the COVID-19 epidemic in France using a model with memory, R. Soc. Open Sci., 8 (2021), 202327. doi: 10.1098/rsos.202327. doi: 10.1098/rsos.202327
![]() |
[22] |
M. Gatto, E. Bertuzzo, L. Mari, S. Miccoli, L. Carraro, R. Casagrandi, et al., Spread and dynamics of the COVID-19 epidemic in Italy: Effects of emergency containment measures, Proc. Natl. Acad. Sci., 117 (2020), 10484–10491. doi: 10.1073/pnas.2004978117. doi: 10.1073/pnas.2004978117
![]() |
[23] | Life expectancy, Available from: https://www.worldometers.info/population. |
1. | Abderrahmane Abbes, Adel Ouannas, Nabil Shawagfeh, Giuseppe Grassi, The effect of the Caputo fractional difference operator on a new discrete COVID-19 model, 2022, 39, 22113797, 105797, 10.1016/j.rinp.2022.105797 | |
2. | Yaping Wang, Yuanfu Shao, Chuanfu Chai, Dynamics of a predator-prey model with fear effects and gestation delays, 2023, 8, 2473-6988, 7535, 10.3934/math.2023378 | |
3. | Muhammad Farman, Khadija Jamil, Changjin Xu, Kottakkaran Sooppy Nisar, Ayesha Amjad, Fractional order forestry resource conservation model featuring chaos control and simulations for toxin activity and human-caused fire through modified ABC operator, 2025, 227, 03784754, 282, 10.1016/j.matcom.2024.07.038 | |
4. | Haneche Nabil, Hamaizia Tayeb, The impact of the Caputo fractional difference operator on the dynamical behavior of a discrete-time SIR model for influenza A virus, 2024, 99, 0031-8949, 115269, 10.1088/1402-4896/ad8703 | |
5. | Fatao Wang, Ruizhi Yang, Yining Xie, Jing Zhao, Hopf bifurcation in a delayed reaction diffusion predator-prey model with weak Allee effect on prey and fear effect on predator, 2023, 8, 2473-6988, 17719, 10.3934/math.2023905 | |
6. | Chaoxiong Du, Wentao Huang, Hopf bifurcation problems near double positive equilibrium points for a class of quartic Kolmogorov model, 2023, 8, 2473-6988, 26715, 10.3934/math.20231367 | |
7. | Sarker Md. Sohel Rana, Md. Jasim Uddin, P. K. Santra, G. S. Mahapatra, A. K. Alomari, Chaotic Dynamics and Control of a Discrete‐Time Chen System, 2023, 2023, 1024-123X, 10.1155/2023/7795246 | |
8. | Abdul Qadeer Khan, Tania Akhtar, Adil Jhangeer, Muhammad Bilal Riaz, Codimension-two bifurcation analysis at an endemic equilibrium state of a discrete epidemic model, 2024, 9, 2473-6988, 13006, 10.3934/math.2024634 | |
9. | Zhao Li, Shan Zhao, Bifurcation, chaotic behavior and solitary wave solutions for the Akbota equation, 2024, 9, 2473-6988, 22590, 10.3934/math.20241100 | |
10. | Aziz Khan, Thabet Abdeljawad, Mahmoud Abdel-Aty, D.K. Almutairi, Digital analysis of discrete fractional order cancer model by artificial intelligence, 2025, 118, 11100168, 115, 10.1016/j.aej.2025.01.036 | |
11. | Pshtiwan Othman Mohammed, On the delta Mittag-Leffler functions and its application in monotonic analysis, 2025, 465, 03770427, 116565, 10.1016/j.cam.2025.116565 |
Parameter | Interpretation | France | Italy | United Kingdom | Source |
\Lambda | Rate in S through migration | 0.21 | 0.21 | 0.21 | Estimated |
\beta | Rate to join S to I | 0.926 | 0.571 | 0.999 | [20,21] |
\nu | Death rate due to virus | 3.3616\times 10^{-5} | 3.2612\times 10^{-5} | 3.2929\times 10^{-5} | [23] |
\gamma | Recovery rate | 0.0735 | 0.076 | 0.0714 | [21,22] |
\sigma | Rate of deteriorate in health | 0.0249 | 0.0338 | 0.0294 | [23] |
h | Step size | 0.2 | 0.2 | 0.2 | Estimated |
Parameter | Interpretation | France | Italy | United Kingdom | Source |
\Lambda | Rate in S through migration | 0.21 | 0.21 | 0.21 | Estimated |
\beta | Rate to join S to I | 0.926 | 0.571 | 0.999 | [20,21] |
\nu | Death rate due to virus | 3.3616\times 10^{-5} | 3.2612\times 10^{-5} | 3.2929\times 10^{-5} | [23] |
\gamma | Recovery rate | 0.0735 | 0.076 | 0.0714 | [21,22] |
\sigma | Rate of deteriorate in health | 0.0249 | 0.0338 | 0.0294 | [23] |
h | Step size | 0.2 | 0.2 | 0.2 | Estimated |