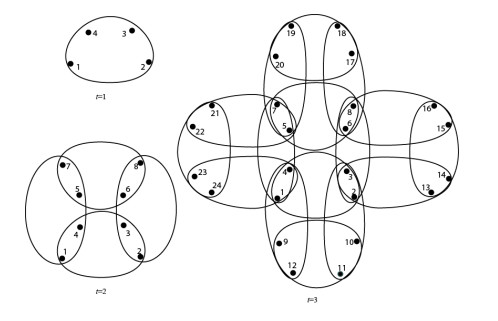
Fractal is a common feature of many deterministic complex networks. The complex networks with fractal features have interesting structure and good performance. The network based on hypergraph is named hypernetwork. In this paper, we construct a hypernetwork model with fractal properties, and obtain its topological properties. Moreover, according to the exact controllability theory, we obtain the node controllability and the hyperedge controllability of the fractal hypernetwork. The simulation results show that the measure of hyperedge controllability is smaller than that of node in the fractal hypernetwork. In addition, We compare the controllability of three types of hypernetwork, which are easier to control by their hyperedges. It is shown the fractal hypernetwork constructed in this paper has the best controllability. Because of the good controllability of our fractal hypernetwork model, it is suitable for the topology structure of many real systems.
Citation: Xiujuan Ma, Fuxiang Ma, Jun Yin. A fractal hypernetwork model with good controllability[J]. AIMS Mathematics, 2021, 6(12): 13758-13773. doi: 10.3934/math.2021799
[1] | Baoxing Zhang, Yunkun Zhang, Yuanyuan Xie . Generating irregular fractals based on iterated function systems. AIMS Mathematics, 2024, 9(5): 13346-13357. doi: 10.3934/math.2024651 |
[2] | Muhammad Farman, Ali Akgül, Kottakkaran Sooppy Nisar, Dilshad Ahmad, Aqeel Ahmad, Sarfaraz Kamangar, C Ahamed Saleel . Epidemiological analysis of fractional order COVID-19 model with Mittag-Leffler kernel. AIMS Mathematics, 2022, 7(1): 756-783. doi: 10.3934/math.2022046 |
[3] | Muhammad Farman, Ali Akgül, Sameh Askar, Thongchai Botmart, Aqeel Ahmad, Hijaz Ahmad . Modeling and analysis of fractional order Zika model. AIMS Mathematics, 2022, 7(3): 3912-3938. doi: 10.3934/math.2022216 |
[4] | Huda Alsaud, Muhammad Owais Kulachi, Aqeel Ahmad, Mustafa Inc, Muhammad Taimoor . Investigation of SEIR model with vaccinated effects using sustainable fractional approach for low immune individuals. AIMS Mathematics, 2024, 9(4): 10208-10234. doi: 10.3934/math.2024499 |
[5] | Muhammad Farman, Aqeel Ahmad, Ali Akgül, Muhammad Umer Saleem, Kottakkaran Sooppy Nisar, Velusamy Vijayakumar . Dynamical behavior of tumor-immune system with fractal-fractional operator. AIMS Mathematics, 2022, 7(5): 8751-8773. doi: 10.3934/math.2022489 |
[6] | Chatthai Thaiprayoon, Jutarat Kongson, Weerawat Sudsutad . Dynamics of a fractal-fractional mathematical model for the management of waste plastic in the ocean with four different numerical approaches. AIMS Mathematics, 2025, 10(4): 8827-8872. doi: 10.3934/math.2025405 |
[7] | A. M. A. El-Sayed, H. H. G. Hashem, Sh. M. Al-Issa . A comprehensive view of the solvability of non-local fractional orders pantograph equation with a fractal-fractional feedback control. AIMS Mathematics, 2024, 9(7): 19276-19298. doi: 10.3934/math.2024939 |
[8] | Cemil Tunç, Alireza Khalili Golmankhaneh . On stability of a class of second alpha-order fractal differential equations. AIMS Mathematics, 2020, 5(3): 2126-2142. doi: 10.3934/math.2020141 |
[9] | Obaid Algahtani, Sayed Saifullah, Amir Ali . Semi-analytical and numerical study of fractal fractional nonlinear system under Caputo fractional derivative. AIMS Mathematics, 2022, 7(9): 16760-16774. doi: 10.3934/math.2022920 |
[10] | Hasib Khan, Jehad Alzabut, Anwar Shah, Sina Etemad, Shahram Rezapour, Choonkil Park . A study on the fractal-fractional tobacco smoking model. AIMS Mathematics, 2022, 7(8): 13887-13909. doi: 10.3934/math.2022767 |
Fractal is a common feature of many deterministic complex networks. The complex networks with fractal features have interesting structure and good performance. The network based on hypergraph is named hypernetwork. In this paper, we construct a hypernetwork model with fractal properties, and obtain its topological properties. Moreover, according to the exact controllability theory, we obtain the node controllability and the hyperedge controllability of the fractal hypernetwork. The simulation results show that the measure of hyperedge controllability is smaller than that of node in the fractal hypernetwork. In addition, We compare the controllability of three types of hypernetwork, which are easier to control by their hyperedges. It is shown the fractal hypernetwork constructed in this paper has the best controllability. Because of the good controllability of our fractal hypernetwork model, it is suitable for the topology structure of many real systems.
Since the term "fractal" was proposed by Mandelbrot [1], many researchers have been observed the fractal features in natural systems, such as crystals, plants, chemical molecules, rivers, and more [1]. Researchers model the real systems with fractal characteristics as fractal networks based on the graph theory [2,3,4,5,6,7,8,9]. And they have been found many interesting structural properties and dynamic behaviors in fractal networks [2,3,4,5,6,7,8,9]. Moreover, many researchers focus on how the fractal structure affects the performance of network, and have been obtained many important results [9,10,11,34].
In the field of complex networks, more and more researchers found that many real systems cannot be represented by networks based on the graph theory [12,13,14,15,16,17,18,19,20,21]. Hypergraph theory provide a powerful tool to solve such problems [22]. Many researchers have tended to represent more complex systems by hypergraphs [12,13,14,15,16,17,18,19,20,21,23,24,25,26,27,28]. They construct many hypernetwork models to describe the real complex systems. There are many real systems with fractal features in nature, which are more suitable modeled by fractal hypernetworks. Such as, the fractal plant systems and the fractal power systems. Because of the random structure of irregular hypernetworks, it is difficult to obtain the relationship between the structure and the performances in the hypernetworks, such as scale-free hypernetwork [12,14,27] and random hypernetwork [26]. However, in fractal hypernetworks, it is easier to obtain the exact results of properties, such as the node number, the hyperedge number, the hyperdegree distribution, and so on.
Controlling is one of the hot issues in complex systems [10,29,30,31,32,33,34]. The controllability is closely related to the dynamic behavior of the network [31,32,33]. For example, in the spread of rumors, if a few spreaders are controlled, the rumors will be controlled. A machine can run automatically only by controlling one switch. A complex system is controllable if imposing appropriate external signals on some elements, the system can be driven from any initial state to any final state in finite time [29,30]. It is important to control a hypernetwork system. For instance, the node represents the Wechat user and the hyperedge represents the Wechat group in Wechat user hypernetwork. The information is spread quickly in Wechat group. If we can control a few Wechat groups, we can control the spread of the information. Yuan studied the controllability of fractal networks based on graph and obtained some important results [10]. The controllability of fractal hypernetworks has not been studied yet. By analysis the controllability of the fractal hypernetworks, we will obtain how the fractal structure affects the controllability of the fractal hypernetworks.
In this paper, we construct a fractal hypernetwork model, which is named 2k uniform (1, 3) flower hypernetwork. In Section 2, the algorithm is proposed to construct the fractal hypernetwork model, and its topological properties are analyzed. In Section 3, we analyze the node controllability and the hyperedge controllability of the fractal (1, 3) flower hypernetwork by the exact controllability theory [29]. In Section 4, we compare the controllability of three hypernetworks. The last section concludes this paper.
In this section, the algorithm for constructing the fractal (1, 3) flower hypernetwork is proposed. Moreover, we analyze some topological properties of the fractal hypernetwork.
In ref. [5], researchers proposed a (1, 3) flower network model with fractal features based on graph. The fractal (1, 3) flower network has small-world characteristics, and its node degree distribution obey power law distribution. Inspired by the fractal (1, 3) flower network, we construct a 2k uniform (1, 3) flower hypernetwork model with fractal features.
Let HF2k(t) be the fractal 2k uniform (1, 3) flower hypernetwork after t time steps. The existing hyperedges at time t-1 are named as old hyperedges, and the hyperedges generated at time t are named as new hyperedges. Let N2k(t) and E2k(t) be the node number and the hyperedge number of HF2k(t), respectively. An iterative algorithm for constructing 2k uniform (1, 3) flower hypernetwork is as follows:
Step 1: at the initial time t = 1, the hypernetwork HF2k(1) has one hyperedge with 2k nodes, where N2k(1) = 2k, E2k(1) = 1.
Step 2: at time t = 2, the hypernetwork HF2k(2) is an evolution hypernetwork from HF2k(1) by adding 2k new nodes and 3 new hyperedges. One hyperedge contains 2k new nodes, other two hyperedges are consisted of k new nodes and k old nodes, respectively, where N2k(2) = 4k, E2k(2) = 4. In other words, each old hyperedge generates three new hyperedges.
Step 3: at time t (t > 2), the fractal hypernetwork HF2k(t) is an evolution hypernetwork from HF2k(t-1) by adding 2kE2k(t-1) new nodes and 3E2k(t-1) new hyperedges according to Step 2, then N2k(t) = 2kE2k(t-1)+N2k(t-1), E2k(t) = 4E2k(t-1).
Figure 1 shows the iterative processes of 2k uniform (1, 3) flower hypernetwork with fractal features from time 1 to time 3.
The 2k uniform (1, 3) flower hypernetwork is a fractal hypernetwork. According to the construction process, we obtain some topological properties of 2k uniform (1, 3) flower hypernetwork.
According to the iteration algorithm and Mathematical Induction method, after t iterations, it is easy to get (1) and (2).
N2k(t)=2k4t−1+23 | (1) |
E2k(t)=4t−1 | (2) |
According to (2), the hyperedge number of 2k uniform (1, 3) flower hypernetwork is just related to iteration time t. Table 1 shows the node number and the hyperedge number of the HF2k(t). For k = 2, the node number of 4-uniform (1, 3) flower hypernetwork is N4(t)=44t−1+23=4t+83, and the hyperedge number is E4(t)=4t−1
time | 1 | 2 | 3 | …… | s |
node number | 2k | 4k | 12k | …… | 2k4s−1+23 |
hyperedge number | 1 | 4 | 16 | …… | 4s−1 |
Let dt−12k(i) be the hyperdegree of node i at time t-1 of 2k uniform (1, 3) flower hypernetwork and Δdt2k(i) be the hyperdegree increment of node i from time t-1 to time t. Obviously, d12k(i)=1, Based on the iteration algorithm, if the node i is contained in dt−12k(i) old hyperedges at time t-1, then the hyperdegree of the node i will be increase dt−12k(i) at time t, which means that Δdt2k(i)=dt−12k(i). Furthermore, each old hyperedge will generate 3 new hyperedges and 2k new nodes at time t. The hyperdegree of new nodes is 2 at time t > 1, namely dt2k(j)=2, where j is a new node which is generated at time t. The hyperdegree of the old node i at time t is
dt2k(i)=dt−12k(i)+Δdt2k(i)=dt−12k(i)+dt−12k(i)=2dt−12k(i) | (3) |
The hyperdegrees of nodes in 2k uniform (1, 3) flower hypernetwork are related with the time of nodes being generated. The hyperdegrees of 2k nodes which are generated at time 1 are
dt2k(i)=2dt−12k(i)=...=2×2t−2d12k(i)=2t−1d12k(i)=2t−1,i=1,2,...,2k. |
The number of nodes joined to HF2k(t) at time s is N2k(s)−N2k(s−1)=2k4s−2. And the hyperdegrees of these nodes are
dt2k(i)=2dt−12k(i)=2×2t−s−1ds2k(i)=2t−s+1(2⩽s⩽t),i=1,2,...,2k4s−2 | (4) |
Let P(dt2k) be the hyperdegree distribution of 2k uniform (1, 3) flower hypernetwork, N(dt2k) be the number of nodes with hyperdegree dt2k at time t, then P(dt2k)=N(dt2k)N2k(t).
By (4), we have 2t−s+1=dt2k, then s=t−log2dt2k+1, N(dt2k)=2k4t−log2dt2k−1, N2k(t)=2k4t−1+23. So, the hyperdegree distribution of 2k uniform (1, 3) flower hypernetwork is represented by (5).
P(dt2k)=34t+84t−log2dt2k,dt2k=2,22,...,2t−2,2t−1 | (5) |
Figure 2 shows the hyperdegree distribution of 4-uniform (1, 3) flower hypernetwork at time 7, which follows the power law distribution.
According to the exact controllability theory [29] we analyze the node controllability and the hyperedge controllability of 2k uniform (1, 3) flower hypernetwork with fractal features in this section.
The following expression describes the controllability of network with N nodes,
˙X=Ax+Bu | (6) |
In (6), the states of N nodes are represented by the vector x = (x1, x2, …, xN)T, and A is the N-order coupling square matrix of network. The vector u = (µ1, µ2, …, µm)T represents the m controllers, and B is the input matrix of size N×m. To completely control the network, we need to choose suitable B and u. For minimizing the number of signals added to driver nodes, a proper matrix B should be designed. The matrix B corresponds to the minimum number of input signals imposed on a minimum set of driver nodes. The controllability of complex network systems is defined by the fraction of driver nodes. A complex network system is controllable if the fraction of driver nodes is less than 1 [29,30].
Let ND be the minimum driver node set (MDS) [29,30]. According to the results of Yuan [29] and Liu [30], ND is the key to measure the controllability of complex network system, it is clearly that
ND≡min{rank(B)}. | (7) |
Yuan et.al [29] proved that for an arbitrary network, the minimum number of ND is determined by the maximum geometric multiplicity μ(λi) of the eigenvalue λi of A:
ND≡max{μ(λi)}, | (8) |
where λi=(i=1,2,...,l) are the distinct eigenvalues of A. If the coupling matrix A is symmetric, then the geometric multiplicity and the algebraic multiplicity of A are equal. In an undirected network, ND is determined by the maximum algebraic multiplicity of δ(λi) of λi:
ND≡max{δ(λi)}. | (9) |
According to (8) and (9), we can calculate ND of all networks.
In ref. [30], nD denotes the measure of controllability, which is defined as follow:
nD=NDN. | (10) |
According (10), a network is more controllable if it has smaller nD, which means that the network is controllable if nD < 1.
In this subsection, we put forward the methods to analyze the node controllability and the hyperedge controllability of hypernetwork, respectively.
The node controllability in hypernetwork is similar to common network.
For a hypernetwork system with N nodes, if inputted suitable signals on a proper subset of nodes, the hypernetwork system can be driven from initial state to final state in finite time, then the hypernetwork system can be controlled by nodes. The minimal set of the proper node subset is called the minimum driven node set of hypernetwork (MDHS). Let HND be the number of the minimum driven node set of a hypernetwork, and HnD be the node controllability measure of the hypernetwork, where HnD=HNDN.
We propose a method to analyzing the node controllability of hypernetwork based on the 2- section graph of the hypergraph [22].
Let H = (V, E) be a hypergraph with N nodes, its 2-section graph is a common graph, written as [H]2. The vertex set of [H]2 is the vertex set of H. If two nodes are contained in the same hyperedge then they are connected by an edge in 2-section graph [22]. Figure 3 shows a hypergraph and its 2-section graph.
Let [HF2k(t)]2 be the 2-section graph of HF2k(t). Figure 4 shows [HF4(t)]2 of HF4(t) at time t (t = 1, 2, 3).
Inspired by [18], we can obtain the node controllability of 2k uniform (1, 3) flower hypernetwork by analyzing the node controllability in its 2-section graph network.
According the exact controllability theory [29], we give the analytical process of node controllability of 2k uniform (1, 3) flower hypernetwork as follows.
Let AHF(t) be the adjacency matrix of [HF2k(t)]2. And let HND be the number of minimum driven node set at time t. The node controllability measure of HF2k(t) at time t is written as HnD(t). Moreover, let δ(λi(t)) be the maximum algebraic multiplicity of the eigenvalue λi for AHF(t).
By (9) and (10), we have HND(t)=max{δ(λi(t))}, and HnD(t)=HND(t)N2k(t).
Figure 5 shows the curve of HND as a function of evolution time t, which shows that the minimum number of driven nodes HND increases with the nodes increasing. Figure 6 shows the curve of HnD over time t, which shows that the measure of node controllability HnD<1 at all times. Therefore, the fractal 2k uniform (1, 3) flower hypernetwork is a node controllable hypernetwork.
For a hypernetwork system with E hyperedges, if the hypernetwork system, which is imposed suitable external signals on the proper hyperedge subset, can be driven from any initial state to any final state, then the hypernetwork system can be controlled by hyperedges. The minimal set of the proper hyperedge subset is called the minimum driven hyperedge set of hypernetwork (MDHES). In a hypernetwork, let HED be minimum number of the driven hyperedges and HeD denote the measure of hyperedge controllability, then HeD=HEDE.
We give a method to analyze the hyperedge controllability based on the line graph of hypergraph [22]. Let H = (V, E) be a hypergraph with N nodes and E hyperedges. The line graph of H is a graph, written as L(H), where the vertex set of L(H) is the hyperedge set E of H. If the intersection of two hyperedges in H is not empty, then their corresponding vertices are connected by an edge in L(H) [22]. Let L(HF2k(t)) be the line graph of HF2k(t).
Figure 7 shows a hypergraph and its line graph. Figure 8 shows L(HF4(t)) of HF4(t) at time t (t = 1, 2, 3).
We can obtain the hyperedge controllability of a hypernetwork by analyzing the node controllability of its line graph. We give the analytical process of the hyperedge controllability for HF4(t) based on the exact controllability theory [29] as follows.
Let ALHF(t) be the adjacency matrix of L(HF2k(t)), HED(t) be the number of minimum driven hyperedge set at time t. The hyperedge controllability measure of HF2k(t) at time t is written as HeD(t). Moreover, let δ(λi(t)) be the maximum algebraic multiplicity of the eigenvalue λi for ALHF(t).
By (9) and (10), we have HED(t)=max{δ(λi(t))}, and HeD(t)=HED(t)E2k(t).
Figure 9 shows the curve of HED as a function of evolution time t. The result shows that the minimum number of driven hyperedges HED increases with the number of hyperedges increasing. Figure 10 shows the curve of the measure of hyperedge controllability HeD as a function of evolution time t. The result shows that the measure of hyperedge controllability HeD<1 in 4-uniform (1, 3) flower hypernetwork. The fractal 4-uniform (1, 3)-flower hypernetwork is a hyperedge controllable hypernetwork.
We compare the measures of the node controllability and the hyperedge controllability of 4-uniform (1, 3) flower hypernetwork with fractal feasures, which is drawn in Figure 11. As a result, the measure of hyperedge controllability is smaller than that of node controllability. It indicates that 4-uniform (1, 3) flower hypernetwork can be better controlled by its hyperedges.
We design the simulation experiment to compare the controllability of 4-uniform (1, 3) flower hypernetwork with two hypernetworks which are 4-uniform linear hypertree network [35] and 4-uniform BA hypernetwork [12].
In ref. [35], the authors give a hypernetwork model with fractal features based on hypergraph. It is named k-uniform linear hypertree network. The 4-uniform linear hypertree network can be construct in an iterative way [35]. Figure 12 shows the iterative processes of 4-uniform linear hypertree network from time 1 to time 3.
Figure 13 shows that the difference of node controllability measures between 4-uniform (1, 3) flower hypernetwork and 4-uniform linear hypertree network. These hypernetworks are both fractal hypernetworks. The results of Figure 13 indicate that 4-uniform (1, 3) flower hypernetwork has better node controllability than 4-uniform linear hypertree network.
Figure 14 shows that the hyperedge controllability measure of 4-uniform (1, 3) flower hypernetwork is smaller than 4-uniform linear hypertree network from time 2 to 7. Therefore, 4-uniform (1, 3) flower hypernetwork has better hyperedge controllability than 4-uniform linear hypertree network.
Figure 15 shows the node controllability measures of 4-uniform (1, 3) flower hypernetwork and 4-uniform BA hypernetwork. The results indicates that 4-uniform (1, 3) flower hypernetwork has better node controllability than 4-uniform BA hypernetwork.
Figure 16 shows the hyperedge controllability measures of 4-uniform (1, 3) flower hypernetwork and 4 uniform BA hypernetwork. The results indicate that 4-uniform (1, 3) flower hypernetwork has smaller measure of the hyperedge controllability than 4-uniform BA hypernetwork. In other words, the fractal 4-uniform (1, 3) flower has better hyperedge controllability.
In Figure 17, we compare the node controllability and the hyperedge controllability of three hypernetworks. We see that the node controllability measure of fractal 4-uniform (1, 3) flower hypernetwork is the smallest, so is the hyperedge controllability measure. It indicates that the fractal 4-uniform (1, 3) flower hypernetwork has better controllability than other two hypernetworks.
Figure 17 shows that the hyperedge controllability measures of three hypernetworks are smaller than their node controllability measures. It indicates that three hypernetworks are easier to control by their hyperedges
In this paper, we construct a fractal (1, 3) flower hypernetwork model. We obtain the mathematical expressions of its topological properties, including the node number, the hyperedge number, the node hyperdegree and the node hyperdegree distribution. In particular, we analyze the node controllability and the hyperedge controllability of the fractal (1, 3) flower hypernetwork. The simulation results show that the controllability measures of node and hyperedge are both less than 1. It indicates that the fractal (1, 3) flower hypernetwork is controllable by nodes or hyperedges. Moreover, the fractal (1, 3) flower hypernetwork has better controllability than fractal linear hypertree network and BA hypernetwork. We compare the node controllability and the hyperedge controllability of three hypernetworks. The results show that three hypernetworks can be controlled easily by hyperedges. Because of the good controllability of fractal (1, 3) flower hypernetwork model, it suitable as the topology structure for some real systems with fractal features, such as, transmission system, communications system, power system, LAN, and so on.
This work is supported by Natural Science Foundation of Qinghai Province in China (No. 2019-ZJ-7012) and the National Natural Science Foundation of China (Nos. 11801296, 61603206).
All authors have no conflicts of interest to declare.
[1] |
B. B. Mandelbrot, The fractal geometry of nature, Am. J. Phys., 51 (1983), 286-286. doi: 10.1119/1.13295
![]() |
[2] | T. Vicsek, Fractal models for diffusion controlled aggregation, J. Phys. A. Math. Gen., 16 (1983), L647. |
[3] |
A. Lakhtakia, V. K. Varadanet, R Messier, V. V. Varadan, Generalisations and randomisation of the plane Koch curve, J. Phys. A: Phys. Gen., 20 (1987), 3537-3541. doi: 10.1088/0305-4470/20/11/052
![]() |
[4] | S. Klavžar, U. Milutinović, Graphs s(n, k) and a variant of the tower of hanoi problem, Czech. Math. J., 47 (1997), 95-104. |
[5] | H. D. Rozenfeld, S. Havlin, D. Ben-Avraham, Fractal and transfractal recursive scale-free nets, New J. Phys., 9 (2006), 1-15. |
[6] |
Z. Z. Zhang, B. Wu, H. J. Zhang, S. G. Zhou, Determining global mean-first-passage time of random walks on vicsek fractals using eigenvalues of laplacian matrices, Phys. Rev. E, 81 (2010), 031118. doi: 10.1103/PhysRevE.81.031118
![]() |
[7] |
C. Song, S. Havlin, H. A. Makse, Self-similarity of complex networks, Nature, 433 (2005), 392-395. doi: 10.1038/nature03248
![]() |
[8] |
C. Song, S. Havlin, H. A. Makse, Origins of fractality in the growth of complex networks, Nat. Phys., 2 (2006), 275-281. doi: 10.1038/nphys266
![]() |
[9] | J. Aguirre, R. L. Viana, M. A. F. Sanjuán, Fractal structures in nonlinear dynamics, Rev. Mod. Phys., 81 (2009), 333-386. |
[10] |
J. W. Li, Z. Z. Yuan, Y. Fan, W. X. Wang, Z. R. Di, Controllability of fractal networks: An analytical approach, EPL, 105 (2014), 58001-58006. doi: 10.1209/0295-5075/105/58001
![]() |
[11] | Z. Zhang, S. Zhou, T. Zou, L. Chen, J. Guan, Different thresholds of bond percolation in scale-free networks with identical degree sequence, Phys. Rev. E., 79 (2009), 03110-03117. |
[12] | J. W. Wang, L. L. Rong, Q. H. Deng, J. Y. Zhang, Evolving hypernetwork model, Eur. Phys. J. B, 77 (2010), 493-498. |
[13] | Z. K. Zhang, C. Liu, A hypergraph model of social tagging networks, J. Stat. Mech., 10 (2010), P10005-P1007. |
[14] | F. Hu, H. X. Zhao, X. J. Ma, An evolving hypernetwork model and its properties, Sci. Sini. Phys., 1 (2013), 16-22. |
[15] | F. H. Wang, J. L. Guo, A. Z. Shen, Q. Suo, Evolving hypernetwork model based on WeChat user relations, Comput. Sci., 11 (2015), 1-14. |
[16] | J. G. Liu, G. Y. Yang, Z. L. Hu, A Knowledge Generation model via the hypernetwork, PLoS One, 9 (2014), e89746-e89748. |
[17] | J. Huang, C. Chen, F. Ye, W. Hu, Z. Zheng, Nonuniform hyper-network embedding with dual mechanism, ACM Trans. Inf. Syst., 38 (2020), 1-18. |
[18] | X. J. Ma, H. X. Zhao, F. Hu, Cascading failure analysis in hyper-network based on the hypergraph, Acta Phys. Sin., 65 (2016), 370-379. |
[19] |
X. J. Ma, F. X. Ma, J. Yin, H. X. Zhao, Cascading failures of k uniform hyper-network based on the hyper adjacent matrix, Physica A, 510 (2018), 281-289. doi: 10.1016/j.physa.2018.06.122
![]() |
[20] |
Z. Wu, J. Duan, X. Fu, Synchronization of an evolving complex hyper-network, Appl. Math. Model., 38 (2014), 2961-2968. doi: 10.1016/j.apm.2013.11.009
![]() |
[21] |
G. Y. Yang, Z. L. Hu, J. G. Liu, Knowledge diffusion in the collaboration hypernetwork, Physica A, 419 (2015), 429-436. doi: 10.1016/j.physa.2014.10.012
![]() |
[22] | A. Bretto, Hypergraph Theory: An Introduction, Berlin: Springer Science Business Media, 2013. |
[23] |
S. G. Aksoy, C. Joslyn, C. Ortizmarrero, B. Praggastis, E. Purvine, Hypernetworks science via high-order hypergraph walk, EPJ Data Sci., 9 (2020), 2-34. doi: 10.1140/epjds/s13688-019-0214-8
![]() |
[24] |
Z. Zhou, Z. Jin, J. Jin, H. Song, Emergence of scaling in evolving hypernetworks, Physica A, 546 (2020), 123765. doi: 10.1016/j.physa.2019.123765
![]() |
[25] |
H. Yin, X. Jiang, Z. Wang, Exploring the dynamic growth mechanism of social networks using evolutionary hypergraph, Physica A, 544 (2020), 122545. doi: 10.1016/j.physa.2019.122545
![]() |
[26] | F. Hu, J. L. Guo, F. X. Li, X. H. Zhao, Hypernetwork models based on random hypergraphs, Int. J. Mod. Phys. C, 30 (2019), 122545. |
[27] |
A. Z. Shen, J. L. Guo, Q. Suo, Study of the variable growth hypernetworks influence on the scaling law, Chaos Soliton. Fractal., 97 (2017), 84-89. doi: 10.1016/j.chaos.2017.02.009
![]() |
[28] |
F. Hu, L. Ma, X. X. Zhan, Y. Zhou, Z. K. Zhang, The aging effect in evolving scientific citation networks, Scientometrics, 126 (2021), 4297-4309. doi: 10.1007/s11192-021-03929-8
![]() |
[29] | Z. Z. Yuan, C. Zhao, Z. Di, W. X. Wang, Y. C. Lai, Exact controllability of complex networks, Nat. Commun., 4 (2013), 1-9. |
[30] | Y. Y. Liu, J. J. Slotine, A. L. Barabási, Controllability of complex networks, Nature, 473 (2011), 167-173. |
[31] | T. Jia, Y. Y. Liu, E. Csoka, M. Pósfai, J. J. Slotine, A. L. Barabási, Emergence of bimodality in controlling complex networks, Nat. Commun., 4 (2013), 1-6. |
[32] | M. Pósfai, Y. Y. Liu, J. J. Slotine, A. L. Barabási, Effect of correlations on network controllability, Sci. Rep., 3 (2013), 1-7. |
[33] | S. Nie, X. Wang, H. Zhang, Q. Li, B. Wang, Robustness of Controllability for Networks Based on Edge-Attack, PLoS One, 9 (2014), e89066. |
[34] | X. Ma, H. Zhao, B. Wang, Controllability of deterministic networks with the identical degree sequence, PloS One, 10 (2015), e0127545. |
[35] |
W. H. Wang, Y. S. Xue, On the r-uniform linear hypertrees with extremal Estrada indices, Appl. Math. Com., 377 (2020), 125144. doi: 10.1016/j.amc.2020.125144
![]() |
1. | Li Luo, Fuzhong Nian, Yuanlin Cui, Fangfang Li, Fractal information dissemination and clustering evolution on social hypernetwork, 2024, 34, 1054-1500, 10.1063/5.0228903 |
time | 1 | 2 | 3 | …… | s |
node number | 2k | 4k | 12k | …… | 2k4s−1+23 |
hyperedge number | 1 | 4 | 16 | …… | 4s−1 |