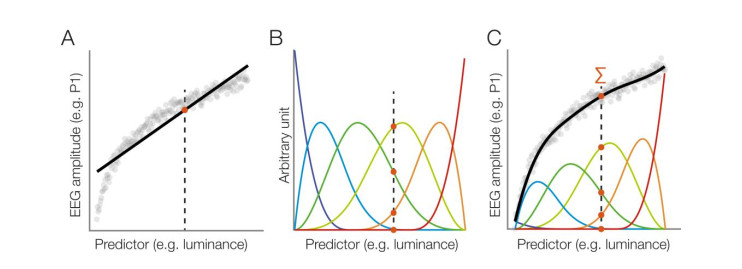
The paper uses the panel smooth conversion fractional-order system model to divide the mechanism of financial development in high-tech industries into two systems. At the same time, the article uses a continuous state to connect these two extreme situations to investigate the nonlinear characteristics of linear feedback control that inhibit the impact of financial development on high-tech industries. Studies have shown that financial development under a low system has a significant role in promoting high-tech industries. On the other hand, financial development under the high-level system has a restraining effect on high-tech industries. There are dual-threshold characteristics between the scale of financial development and the development of high-tech industries. Firstly, the scale of financial development should be controlled within [0.553, 0.840]. Secondly, there is a single threshold between the level of financial development and the development of high-tech industries, and the level of financial development should be controlled within 0.756.
Citation: Xiaoguang Luo, Jiaying Feng, Steven Formaneck. The influence of linear feedback control suppression under the calculation of fractional financial system on the upgrading of high-tech industries[J]. Mathematical Biosciences and Engineering, 2021, 18(5): 5114-5124. doi: 10.3934/mbe.2021260
[1] | Vladimir Djordjevic, Hongfeng Tao, Xiaona Song, Shuping He, Weinan Gao, Vladimir Stojanovic . Data-driven control of hydraulic servo actuator: An event-triggered adaptive dynamic programming approach. Mathematical Biosciences and Engineering, 2023, 20(5): 8561-8582. doi: 10.3934/mbe.2023376 |
[2] | Chaoyue Wang, Zhiyao Ma, Shaocheng Tong . Adaptive fuzzy output-feedback event-triggered control for fractional-order nonlinear system. Mathematical Biosciences and Engineering, 2022, 19(12): 12334-12352. doi: 10.3934/mbe.2022575 |
[3] | Junlin Zhu, Hua Wang, Lin Miao, Zitong Yu . Case study of financial leasing model driven by fuzzy logic control for alternative fuel vehicles operation. Mathematical Biosciences and Engineering, 2023, 20(1): 894-912. doi: 10.3934/mbe.2023041 |
[4] | H. T. Banks, Cammey E. Cole, Paul M. Schlosser, Hien T. Tran . Modeling and optimal regulation of erythropoiesis subject to benzene intoxication. Mathematical Biosciences and Engineering, 2004, 1(1): 15-48. doi: 10.3934/mbe.2004.1.15 |
[5] | Juanjuan Tian, Li Li . Research on artificial intelligence of accounting information processing based on image processing. Mathematical Biosciences and Engineering, 2022, 19(8): 8411-8425. doi: 10.3934/mbe.2022391 |
[6] | Zichen Wang, Xin Wang . Fault-tolerant control for nonlinear systems with a dead zone: Reinforcement learning approach. Mathematical Biosciences and Engineering, 2023, 20(4): 6334-6357. doi: 10.3934/mbe.2023274 |
[7] | Filippo Cacace, Valerio Cusimano, Alfredo Germani, Pasquale Palumbo, Federico Papa . Closed-loop control of tumor growth by means of anti-angiogenic administration. Mathematical Biosciences and Engineering, 2018, 15(4): 827-839. doi: 10.3934/mbe.2018037 |
[8] | Maria Conceição A. Leite, Yunjiao Wang . Multistability, oscillations and bifurcations in feedback loops. Mathematical Biosciences and Engineering, 2010, 7(1): 83-97. doi: 10.3934/mbe.2010.7.83 |
[9] | Zhen Yu, Sheng-Huang Lin, Chia-Ching Cho, Changping Chen . Performance management algorithm of financial shared service center based on Internet of Things public cloud privacy protection. Mathematical Biosciences and Engineering, 2023, 20(7): 12510-12528. doi: 10.3934/mbe.2023557 |
[10] | Wei Zou, Yanxia Shen, Lei Wang . Design of robust fuzzy iterative learning control for nonlinear batch processes. Mathematical Biosciences and Engineering, 2023, 20(11): 20274-20294. doi: 10.3934/mbe.2023897 |
The paper uses the panel smooth conversion fractional-order system model to divide the mechanism of financial development in high-tech industries into two systems. At the same time, the article uses a continuous state to connect these two extreme situations to investigate the nonlinear characteristics of linear feedback control that inhibit the impact of financial development on high-tech industries. Studies have shown that financial development under a low system has a significant role in promoting high-tech industries. On the other hand, financial development under the high-level system has a restraining effect on high-tech industries. There are dual-threshold characteristics between the scale of financial development and the development of high-tech industries. Firstly, the scale of financial development should be controlled within [0.553, 0.840]. Secondly, there is a single threshold between the level of financial development and the development of high-tech industries, and the level of financial development should be controlled within 0.756.
Regarding the relationship and research on the high-tech financial support industry in the western region and the financial support development in the western region, the academic circles generally have the following views. A traditional view is that the scale and efficiency of the development of financial support industries in the western region are positively correlated with the development of high-tech financial support industries. Therefore, the western region's financial system and structural reforms should be promoted and the economy endogenous. The growth path is undoubtedly an essential key to developing high-tech industries and finance in the western region. Foreign scholars generally pointed out that when banks or other financial institutions provide high-tech financial support to customers of high-tech financial support industries in the western region. The financial development scale and efficiency of high-tech industries will inevitably increase significantly. Positive or negative, and at the same time, the level of backward bank credit and financial support has adversely affected the export development of high-tech products in the western region. Some experts and scholars have studied the influence and effect of bank credit finance support on emerging industries and financial development from the bank credit capital market and other capital financial support markets. They pointed out the two aspects of the market demand and characteristics of the emerging industries in the western region that affect financial development [1].
The research on the high-tech industry and financial development in academia generally have the following views. One point of view is that the scale and efficiency of financial development are positively correlated with high-tech industries. Therefore, promoting the reform of the financial system and taking the path of endogenous growth is the key to the development of high-tech industries. Foreign scholars pointed out that when banks or financial institutions provide financial support to high-tech industries, the development efficiency of high-tech industries will increase significantly. Another view is that the scale of financial development in the eastern region has a significantly positive impact on high-tech industries. Still, its impact on the central region is not prominent, and its impact on the western region is significantly harmful. At the same time, the backward level of financial development has adversely affected the export of high-tech products. Some scholars study the effect of financial support on developing emerging industries from the credit market and capital market. They pointed out that the demand-side factors of the development of emerging industries include market size and customer demand characteristics. Some scholars analyze fiscal incentive policies and preferential tax policies, property rights markets, high-tech industry clusters, and industry clusters. They pointed out that fiscal incentive policies can stimulate the output growth of high-tech industries more than preferential tax policies. The property right market can activate venture capital, lead venture capital, and bring vitality to the financing and mergers and acquisitions of high-tech enterprises. The more backward the region's financial market is connected with the accumulation of high-tech industries, the more significant the efficiency of industrial innovation will be.
In the research mentioned above on high-tech industries, some scholars believe that the impact of financial development on high-tech industries is positively correlated. Another part of scholars believes that the impact of financial development on high-tech industries is not apparent in the central region. The western region even has a negative impact. What these scholars have in common is that they all use linear models as tools. Therefore, we fully consider the nonlinear effect between financial development and high-tech industries, further enriching the relevant research on my country's high-tech industries.
Firstly, it is based on the general production function, and secondly, the Cobb-Douglas linear production function is introduced into the model. The main idea is to use part of the theory of fractional differential equations. Finally, the model is further expanded into a multi-factor input nonlinear smooth conversion model, as shown in Figure 1. The general form of the production function, including labor, capital, and financial development, is
Y=A(t)f(K,L,E) | (1) |
Among them, Y represents total output, A represents technological progress, the factor input of labor is L, the factor input of capital is K, and the financial development is E. Taking the derivative of Eq (1) concerning time t, we get:
dYdt=dAdt×YA+Yf(∂f∂K×∂K∂t+∂f∂L×∂L∂t+∂f∂E×∂E∂t) | (2) |
Divide both ends of the above formula by Y and get:
ZY=ZA+∂f∂K×KfZK+∂f∂L×LfZL+∂f∂E×EfZE | (3) |
The above formula ZY,ZA,ZK,ZL,ZE represent output growth rate dY/dtY, technological progress rate dA/dtY, capital input growth rate dK/dtY, labor input growth rate dL/dtY, and financial growth rate dE/dtY. The general C-D production function based on the functions of technological innovation, capital and labor, and financial development are as follows
Y=AKaLβEφ | (4) |
α represents the elasticity of capital relative to output, that is, the contribution of capital in output; β is the elasticity of labor relative to output, that is, the contribution of labor in output; ϕ represents the elasticity of financial development relative to output, that is the contribution rate of financial development in output. Among them, α+β+ϕ=1 represents the constant return to scale. Substituting formula (4) into formula (2) to sort out. Figure 2 shows the elasticity curve of capital-output versus labor payment.
zY=ZA+α×ZK+β×ZL+φ×ZE | (5) |
Equation (5) concludes that technological innovation, capital input, labor input increase, and financial development will stimulate the increase of output of strategic emerging industries; conversely, if technology declines, the degree of financial development decreases, or labor and capital input decrease. It will also slow down the growth rate of high-tech industry output. To prevent the problem of heteroscedasticity in the research process, the logarithmic process is carried out, then formula (5) can be rewritten as:
ln ZY=ln ZA+αln ZK+βln ZL+φln ZE | (6) |
Further, the above model is extended to multi-element inputs, and the following formula is obtained:
ln y=n∑i=1ciln xi+ui | (7) |
On the one hand, financial development can expand the production scale of high-tech industries and increase output and provide continuous financial support for expanding production. On the other hand, sufficient funds often stimulate technological innovation and further promote the increase of the output value of high-tech industries. However, excessive financial development will harm the high-tech industry. First, financial supervision is challenging to keep up with the level of financial development. Many regulatory deficiencies have led to the accumulation of vulnerabilities and ultimately lead to an internal crisis in the high-tech industry. It is conducive to the development of the industry; secondly, financial support depends on the financing ability of the high-tech industry with a particular leverage effect. Suppose the high-tech industry is overly dependent on financial support once a link is wrong. In that case, it will lead to disasters for the entire industry, and financial support may even become a crisis. Finally, the high-tech industries under excessive financial development are prone to bubbles, creating false prosperity. The bubbles will eventually burst when they accumulate to a certain extent, bringing a catastrophic blow to the high-tech industries. Therefore, there is more than a simple positive correlation between the high-tech industry and financial development; therefore, the traditional linear model cannot accurately examine the relationship between the two. The author uses the nonlinear model as a tool to study the second Nonlinear effects between the participants. In reality, there are three forms of nonlinear mechanism conversion models, namely Markov, threshold regression, and smooth conversion models. The first two conversion models assume that the time series jump from one machine to another, and the mechanism of action is one Mutation process. In fact, with the changes in the scale of financial development and the level of financial development, high-tech transformations are often a long and gradual process. The panel smooth conversion model is as follows,
ln yit=ui+n∑i=1ciln xitr∑j=1dj(n∑i=1ln xit)×Gj(γj,aj;sjit)+εit | (8) |
yit represents the explained variable xit represents the explanatory variable ci and di represents the parameter vector, ui represents the fixed effect of the individual, εit is the residual, Gi represents the smooth transfer function, and its value is between 0 and 1; the parameter yi is the transfer function in The conversion speed between 0 and 1. The parameter ai represents the position parameter of the conversion, that is, the threshold value at which the conversion occurs, and sij refers to the conversion variable, which can be one of the explanatory variables or the time trend. The conversion function is Logistic Formula: G(γ,a;sit)={1+exp[−γ∏(sit−aj)]}−1 When the transfer function G = 0, the model is in the low system; when G = 1, the model is in the high system, because the value of the transfer function is between 0 and 1, the mode switches between the low system and the high system. It can be seen that the smooth transition model not only explains the two extreme cases at the same time but also connects the two extreme cases with a continuous state.
(1) Fixed capital investment K. The fixed capital stock is used to replace the capital flow, and the perpetual inventory method is usually used to calculate the fixed capital stock:
Kt=(1−δ)Kt−1+It | (9) |
Kt represents the stock of fixed assets of each province and city in the high-tech industry in period t, It refers to the fixed asset investment flow of the high-tech industry in period t, δ is the capital depreciation rate, and δ is 9.6%. The general range of parameter t is 1-12.
(2) Human capital investment L. At present, human capital is still the central core economic driving force for the economic and social development of high-tech industries. It has more potential and space for its appreciation than ordinary social material labor capital. Similarly, human capital must also have the ability to allocate labor resources and adjust the enterprise itself rationally. Develop business strategy and another adaptability; therefore, the use and investment of human capital to the economic and social contribution of high-tech industries cannot be ignored. We can use the formula of average labor input and years of higher education to express average human capital input. Assume that the number of years of primary school education is 6 years. The number of years of junior high school education is 9 years. High school is 12a. The university is 16a. We multiply the respective proportions of the number of educated persons in elementary school, junior high school, high school, and university by the corresponding number of years and sum them to get the average number of years of education. The paper uses this to express human capital investment.
(3) Financial Development E. We use the ratio of the deposit and loan balance of financial institutions to GDP and the ratio of total stock market value to GDP in various regions. Using principal component analysis synthesizes a financial development scale e1 indicator as a proxy variable to measure financial development. The article uses two indicators: the ratio of deposits to loan balances of regional financial institutions and the ratio of stock trading volume to stocks' market value in circulation. We use principal component analysis to synthesize a financial development level e2 as another proxy variable to measure financial development [2].
(4) Industry output value Y. The leading conversion index coefficient for analyzing and measuring the characteristics of the service industry and the total output value of the service industry is y. The industry comprehensive output value index is used to measure the sum of the primary high-tech product services and main production materials engaged in the operation and development of the high-tech service industry in a specific period in China. It can objectively reflect and directly show the principal high-tech economic value and industrial achievements of China's primary means of production engaged in high-tech service industries and technical service production activities. The comprehensive conversion price coefficient based on the value of the GDP price index of the developed countries and developed regions in China over the years, after the comprehensive conversion of the value, can form the unchanged high-tech industrial product of the previous year the conversion base period. The price is shown in Table 1 below.
Variable | Sample / Individual standard | Standard error | Mean | Min | Max |
lny | 372 | 1.978 | 6.131 | 1.191 | 10.354 |
lnk | 372 | 1.957 | 3.682 | −3.506 | 7.573 |
lnl | 372 | 1.709 | 11.453 | 6.966 | 15.169 |
lne1 | 372 | 0.317 | 0.228 | −0.447 | 1.414 |
lne2 | 372 | 0.216 | 0.351 | −0.084 | 1.065 |
The research data comes from the "Statistical Yearbooks, " "China High-tech Industry Statistical Yearbooks, " "China Demographic Yearbooks, " and "China Financial Statistics Yearbooks" of various provinces and cities. In addition, because the relationship between financial development and high-tech industries is not a simple linear relationship, financial development is selected as a conversion variable. Financial development is usually measured by the scale and level of financial development, and the scale and level of financial development are used as conversion variables. Establish the following two-panel smooth transition empirical models [3]:
Taking financial development scale e1 as the proxy variable to measure financial development, analyses the nonlinear effect of financial development on the impact of high-tech industries:
ln yit=ui+c1ln kit+c2ln lit+c3ln e1+(d1ln kit+d2ln lit+d3ln e1)G(ln e1,γ,α)+εit | (10) |
Using financial development level e2 as a proxy variable to measure financial development, analyses the nonlinear effects of financial development on high-tech industries:
ln yit=ui+c1ln kit+c2ln lit+c3ln e2+(d1ln kit+d2ln lit+d3ln e2)G(ln e2,γ,α)+εit | (11) |
In the above formula, I represents different provinces, subscript t represents different years, γ represents the speed of conversion, ci represents the parameter of the linear part, di is the parameter of the nonlinear part, α is the threshold value, and G is the transfer function. The transfer function represents the mechanism of the transformation of the impact of financial development on high-tech industries. If the value of the transfer function is about 0, the impact of financial development on the high-tech industry obeys the low system. If the value of the transfer function is about 1, the impact of financial development on high-tech industries the influence of the industry obeys the low system. If the value of the transfer function is between 0 and 1, the influence of financial development on the high-tech industry obeys the mixed mechanism [4].
The problem of non-stationarity often exists in economic data. Before studying the problem, the data needs to be tested for unit root. The unit root process of panel data includes the unit root process in the same and different roots. Considering these two cases, use LLC Inspection and IPS inspection, as shown in Table 2.
Variable | IPS inspection | LLC inspection | ||
Statistic | prob | Statistic | prob | |
lny | −1.769 | 0.017 | −10.786 | 0.000 |
d(lny) | −2.335 | 0.000 | 13.335 | 0.000 |
lnk | −1.714 | 0.121 | −10.313 | 0.000 |
d(lnk) | −2.542 | 0.000 | −16.429 | 0.000 |
lnl | −1.262 | 0.909 | −7.336 | 0.002 |
d(lnl) | −2.383 | 0.000 | −14.768 | 0.000 |
lne1 | −1.356 | 0.791 | −7.413 | 0.035 |
d(lne1) | −1.720 | 0.000 | −9.227 | 0.000 |
lne2 | −1.539 | 0.420 | −9.289 | 0.093 |
d(lne2) | −1.743 | 0.002 | −8.963 | 0.000 |
According to the requirement of establishing a panel smooth transition model (PSTR) proposed by scholars, it is necessary to test the heterogeneity of panel data. To avoid identification problems, the conversion function G[ln eit,γ,α] is subjected to a first-order Taylor expansion to construct an auxiliary model:
ln yit=ui+ρ0xit+ρ1xitsit+⋅⋅⋅+ρmxitsmit+εit | (12) |
xit represents (ln kit+ln lit+ln eit), sln kit represents the conversion variable, m represents the number of positional parameters and initially assumes m = 3. The null hypothesis of the heterogeneity test using the auxiliary function is set as a linear model, that is, H0 is ρ1+ρ2+ρ3=0. If the heterogeneity test accepts the null hypothesis, it means that there is no nonlinear relationship between financial development and high-tech industries. On the contrary, if Rejecting the original hypothesis shows at least one position parameter PSTR model. It shows that the panel smooth conversion model can accurately represent the nonlinear characteristics between financial and high-tech industries. Accordingly, the results of the heterogeneity test using auxiliary functions are shown in Table 3.
Model | Conversion variable | H0: ρ1 = ρ2 = ρ3 = 0 | Conclusion | |
F-statistics | Prob | |||
Model (a) | Financial development scale | 5.345 | 0.000 | Reject the null hypothesis |
Model (b) | Financial development efficiency | 3.933 | 0.001 | Reject the null hypothesis |
In Table 3, the F statistic of the two models is greater than the critical value at the 1% significance level, indicating that the null hypothesis ρ1+ρ2+ρ3=0 is rejected. The model is a PSTR model. That is, there is a nonlinear mechanism conversion between financial development and high-tech industries. It shows that it is reasonable to estimate PSTR using the two conversion variables of financial development scale and financial development level.
Null hypothesis | Financial development scale | Financial development level | ||
F-statistic | Prob | F-statistic | Prob | |
ρ3 = 0 | 4.465 | 0.055 | 7.324 | 0.001 |
ρ2 = 0/ρ3 = 0 | 6.195 | 0.001 | 4.099 | 0.030 |
ρ1 = 0 = ρ2 = ρ3 = 0 | 2.567 | 0.040 | 0.172 | 0.840 |
The nonlinear least-squares method (NLS) is used to iteratively estimate the nonlinear equations of a model (a) and model (b). The estimation results are shown in Table 5.
Null hypothesis | Model (a) | Model (b) | ||
coef | Std | coef | Std | |
c | 0.667 | 0.158 | 0.633 | 0.053 |
c2 | 0.278 | 0.163 | 0.328 | 0.032 |
c3 | 0.346 | 0.045 | 0.416 | 0.339 |
d1 | −0.220 | 0.256 | −0.113 | 0.074 |
d2 | 0.432 | 0.139 | 0.243 | 0.233 |
d3 | −0.532 | 0.227 | −0.665 | 0.053 |
Position parameter a | 0.553 | 0.756 | ||
Conversion speed | 0.867 | 1.322 | ||
R2 | 0.998 | 0.955 | ||
Number of observations | 372 | 372 |
The coefficients of the two model variables in Table 5 are statistically significant, indicating that the scale and level of financial development and the development of high-tech industries in various provinces and cities have noticeable nonlinear effects. According to the estimated results:
(1) Explain the scale of financial technology industry development. The optimal number of location parameters of the financial model (a) is 2. The location parameters are fitted as a1 = 0.553, a2 = 0.840. The optimal degree of location parameter fitting is 0.998, indicating the development of the financial technology industry in the foreign system. The equal and positive impact of the scale of other high-tech industries on the development of other high-tech industries is a characteristic of nonlinear economics with double thresholds. The scale of the development of the financial technology industry in a particular province or city is 0.553 < e1 < 0.84. the equal and positive impact model of developing the financial technology industry on other high-tech industries is intermediate. The equal and positive value of the transfer function is about 0 [5]. The elastic coefficients of capital input, labor cost input, and the development of other financial technology industries relative to the output value of the external system and the development of high-tech industries are taken as 0.667, 0.278 and 0.335. It shows that the development of the financial technology industry positively impacts developing other high-tech industries. When the value of the development scale of the financial technology industry of a particular country or province is e1 < 0.553 or the value is e1 > 0.84, the model is under the external system. The value of the transfer function is equal to and close to 1, respectively, the development of the financial technology industry Relative to other high-tech industries, the elasticity coefficient of the development output value changes from 0.346 transfer function of the intermediate system to −0.186 (0.346-0.532) of the external system. The conversion speed γ = 0.867 shows that the conversion speed between the financial development and the high-tech industry according to the conversion function mechanism is relatively slow. The estimation result of Model 1 shows prominent nonlinear characteristics between financial development and high-tech industries. The scale of financial development should be controlled within the interval of [0.553, 0.840]. Otherwise, both low-level financial development and high-level financial development will inhibit the development of high-tech industries.
(2) Reflects the level of rapid development of the financial economy. With the rapid development of the financial economy relative to other high-tech industries' input and output value, the financial-economic elasticity growth rate coefficient increased from 0.416 in the low system to −0.249 (0.416-0.665) in the high system. The conversion speed γ = 1.322, indicating that the current development of the financial industry and other high-tech industries can be converted according to the mechanism of the conversion function interaction is faster than model (a). The estimation results relative to model (b) also indicate that there should be apparent nonlinear conversion characteristics between the current financial industry development and other high-tech industries. At this time, the current financial industry development level should be strictly controlled below 0.756. Excessive nonlinear financial industry development will harm the marketization and rapid development of other high-tech industries [6].
The development of excessive finance plays an essential role in developing and suppressing the high-tech crisis magnified industry. First of all, the excessive financial development of high-tech industries mostly depends on a kind of financing control ability of the high-tech industry itself [7]. This catastrophic financing control ability itself contains a kind of leverage crisis amplification effect, which hides substantial financial risks. As we all know, the leveraged crisis amplification effect itself refers to a critical link of financial supervision errors, which will inevitably trigger a series of chain reactions in the industry. So this leveraged crisis suppression and amplification effect will inevitably cause incompetence to the development of China's high-tech industries. An economic disaster to save. Secondly, the degree of financial development requires that the risk is stabilized within a specific limit. Once the degree of excessive financial industry development requires the risk to exceed this specific limit, the problems and drawbacks of insufficient financial supervision capabilities will inevitably be further highlighted and exposed [8] and will be further exposed. As a result, intensify and accumulate crises within the high-tech industry. Finally, China's high-tech crisis magnified industries under excessive financial development is likely to produce economic bubbles in a short period, resulting in a false and prosperous macroeconomic scene. The accumulation of bubbles, to a certain extent, will eventually lead to the collapse of the bubbles, which will give China's high-tech industries. Thus, development has brought a disastrous economic blow [9].
Using the empirical results of the panel smooth transition model to test the nonlinear smooth transition relationship between strategic financial development and strategic high-tech industries, it proves that this nonlinear smooth transition relationship between the development of financial technology industries and strategic high-tech industries is mainly. It is a smooth transition from the traditional low system (or intermediate system) to the high system (or foreign system) based on the smooth transfer function. The test results show that due to the dual development threshold characteristics of the gap between the scale of financial development and the rapid development of strategic high-tech industries, the gap between the development and scale of the financial technology industry should be strictly controlled [0.553, 0.840]. Otherwise, whether the development of a strategic financial technology industry with a relatively low degree of marketization or the development of a strategic financial technology industry with a high degree of marketization will directly inhibit the rapid development of other strategic financial emerging industries. Due to the development of the financial technology industry, there is a difference in single development threshold characteristics between the development and strategic high-tech industries. Therefore, the gap between the development scale and level of the strategic financial technology industry should be strictly controlled within 0.756. Once the development level exceeds 0.756, increasing strategic finance support will not effectively promote the rapid development of strategic high-tech industries. Still, it may have adverse effects on the economy.
All authors declare that there is no conflict of interest in this paper.
[1] | V. Seshadri, On the choice of optimum gain for linear feedback control systems, IETE J. Res., 13 (2015), 184-189. |
[2] |
S. Wu, Internet public informatioan text data mining and intelligence influence analysis for user intent understanding, J. Intell. Fuzzy Syst., 38 (2020), 487-494. doi: 10.3233/JIFS-179423
![]() |
[3] |
T. Lei, W. Li, J. Wang, J. Zhao, Research on the characteristic of automotive failure diagnosis based on complex networks, Open Mech. Eng. J., 9 (2015), 508-513. doi: 10.2174/1874155X01509010508
![]() |
[4] | T. Dean, D. A. Griffith, R. J. Calantone, New product creativity: understanding contract specificity in new product introductions, J. Mark., 80 (2016), 39-58. |
[5] |
Y. Liu, E. Pavlovskaia, M. Wiercigroch, Z. Peng, Forward and backward motion control of a vibro-impact capsule system, Int. J. Nonlinear Mech., 70 (2015), 30-46. doi: 10.1016/j.ijnonlinmec.2014.10.009
![]() |
[6] |
J. Cui, H. Jo, M. G. Velasquez, The influence of christian religiosity on managerial decisions concerning the environment, J. Bus. Ethics, 132 (2015), 203-231. doi: 10.1007/s10551-014-2306-5
![]() |
[7] |
S.Wu, Research on the application of spatial partial differential equation in user oriented information mining, Alexandria Eng. J., 59 (2020), 2193-2199. doi: 10.1016/j.aej.2020.01.047
![]() |
[8] | Z. Lu, K. Li, H. Ding, L. Chen, Nonlinear energy harvesting based on a modified snap-through mechanism, Appl. Math. Nonlinear Sci., 12 (2019), 132-134. |
[9] | J. Ruiz-Fernández, M. Benlloch, N. López, Influence of seasonal factors in the earned value of construction, Appl. Math. Nonlinear Sci., 3 (2019), 45-46. |
Variable | Sample / Individual standard | Standard error | Mean | Min | Max |
lny | 372 | 1.978 | 6.131 | 1.191 | 10.354 |
lnk | 372 | 1.957 | 3.682 | −3.506 | 7.573 |
lnl | 372 | 1.709 | 11.453 | 6.966 | 15.169 |
lne1 | 372 | 0.317 | 0.228 | −0.447 | 1.414 |
lne2 | 372 | 0.216 | 0.351 | −0.084 | 1.065 |
Variable | IPS inspection | LLC inspection | ||
Statistic | prob | Statistic | prob | |
lny | −1.769 | 0.017 | −10.786 | 0.000 |
d(lny) | −2.335 | 0.000 | 13.335 | 0.000 |
lnk | −1.714 | 0.121 | −10.313 | 0.000 |
d(lnk) | −2.542 | 0.000 | −16.429 | 0.000 |
lnl | −1.262 | 0.909 | −7.336 | 0.002 |
d(lnl) | −2.383 | 0.000 | −14.768 | 0.000 |
lne1 | −1.356 | 0.791 | −7.413 | 0.035 |
d(lne1) | −1.720 | 0.000 | −9.227 | 0.000 |
lne2 | −1.539 | 0.420 | −9.289 | 0.093 |
d(lne2) | −1.743 | 0.002 | −8.963 | 0.000 |
Model | Conversion variable | H0: ρ1 = ρ2 = ρ3 = 0 | Conclusion | |
F-statistics | Prob | |||
Model (a) | Financial development scale | 5.345 | 0.000 | Reject the null hypothesis |
Model (b) | Financial development efficiency | 3.933 | 0.001 | Reject the null hypothesis |
Null hypothesis | Financial development scale | Financial development level | ||
F-statistic | Prob | F-statistic | Prob | |
ρ3 = 0 | 4.465 | 0.055 | 7.324 | 0.001 |
ρ2 = 0/ρ3 = 0 | 6.195 | 0.001 | 4.099 | 0.030 |
ρ1 = 0 = ρ2 = ρ3 = 0 | 2.567 | 0.040 | 0.172 | 0.840 |
Null hypothesis | Model (a) | Model (b) | ||
coef | Std | coef | Std | |
c | 0.667 | 0.158 | 0.633 | 0.053 |
c2 | 0.278 | 0.163 | 0.328 | 0.032 |
c3 | 0.346 | 0.045 | 0.416 | 0.339 |
d1 | −0.220 | 0.256 | −0.113 | 0.074 |
d2 | 0.432 | 0.139 | 0.243 | 0.233 |
d3 | −0.532 | 0.227 | −0.665 | 0.053 |
Position parameter a | 0.553 | 0.756 | ||
Conversion speed | 0.867 | 1.322 | ||
R2 | 0.998 | 0.955 | ||
Number of observations | 372 | 372 |
Variable | Sample / Individual standard | Standard error | Mean | Min | Max |
lny | 372 | 1.978 | 6.131 | 1.191 | 10.354 |
lnk | 372 | 1.957 | 3.682 | −3.506 | 7.573 |
lnl | 372 | 1.709 | 11.453 | 6.966 | 15.169 |
lne1 | 372 | 0.317 | 0.228 | −0.447 | 1.414 |
lne2 | 372 | 0.216 | 0.351 | −0.084 | 1.065 |
Variable | IPS inspection | LLC inspection | ||
Statistic | prob | Statistic | prob | |
lny | −1.769 | 0.017 | −10.786 | 0.000 |
d(lny) | −2.335 | 0.000 | 13.335 | 0.000 |
lnk | −1.714 | 0.121 | −10.313 | 0.000 |
d(lnk) | −2.542 | 0.000 | −16.429 | 0.000 |
lnl | −1.262 | 0.909 | −7.336 | 0.002 |
d(lnl) | −2.383 | 0.000 | −14.768 | 0.000 |
lne1 | −1.356 | 0.791 | −7.413 | 0.035 |
d(lne1) | −1.720 | 0.000 | −9.227 | 0.000 |
lne2 | −1.539 | 0.420 | −9.289 | 0.093 |
d(lne2) | −1.743 | 0.002 | −8.963 | 0.000 |
Model | Conversion variable | H0: ρ1 = ρ2 = ρ3 = 0 | Conclusion | |
F-statistics | Prob | |||
Model (a) | Financial development scale | 5.345 | 0.000 | Reject the null hypothesis |
Model (b) | Financial development efficiency | 3.933 | 0.001 | Reject the null hypothesis |
Null hypothesis | Financial development scale | Financial development level | ||
F-statistic | Prob | F-statistic | Prob | |
ρ3 = 0 | 4.465 | 0.055 | 7.324 | 0.001 |
ρ2 = 0/ρ3 = 0 | 6.195 | 0.001 | 4.099 | 0.030 |
ρ1 = 0 = ρ2 = ρ3 = 0 | 2.567 | 0.040 | 0.172 | 0.840 |
Null hypothesis | Model (a) | Model (b) | ||
coef | Std | coef | Std | |
c | 0.667 | 0.158 | 0.633 | 0.053 |
c2 | 0.278 | 0.163 | 0.328 | 0.032 |
c3 | 0.346 | 0.045 | 0.416 | 0.339 |
d1 | −0.220 | 0.256 | −0.113 | 0.074 |
d2 | 0.432 | 0.139 | 0.243 | 0.233 |
d3 | −0.532 | 0.227 | −0.665 | 0.053 |
Position parameter a | 0.553 | 0.756 | ||
Conversion speed | 0.867 | 1.322 | ||
R2 | 0.998 | 0.955 | ||
Number of observations | 372 | 372 |