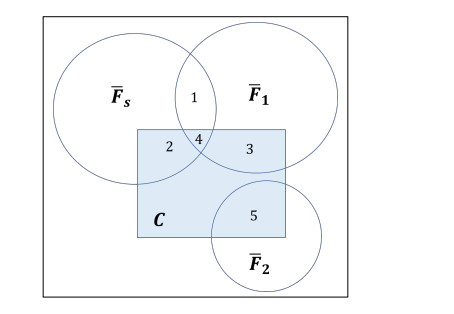
Citation: Omar A. M. Salem, Feng Liu, Ahmed Sobhy Sherif, Wen Zhang, Xi Chen. Feature selection based on fuzzy joint mutual information maximization[J]. Mathematical Biosciences and Engineering, 2021, 18(1): 305-327. doi: 10.3934/mbe.2021016
[1] | Ming Meng, Luyang Dai, Qingshan She, Yuliang Ma, Wanzeng Kong . Crossing time windows optimization based on mutual information for hybrid BCI. Mathematical Biosciences and Engineering, 2021, 18(6): 7919-7935. doi: 10.3934/mbe.2021392 |
[2] | Philip A. Warrick, Emily F. Hamilton . Information theoretic measures of perinatal cardiotocography synchronization. Mathematical Biosciences and Engineering, 2020, 17(3): 2179-2192. doi: 10.3934/mbe.2020116 |
[3] | Han-Yu Lin, Tung-Tso Tsai, Hong-Ru Wu, Miao-Si Ku . Secure access control using updateable attribute keys. Mathematical Biosciences and Engineering, 2022, 19(11): 11367-11379. doi: 10.3934/mbe.2022529 |
[4] | Miin-Shen Yang, Wajid Ali . Fuzzy Gaussian Lasso clustering with application to cancer data. Mathematical Biosciences and Engineering, 2020, 17(1): 250-265. doi: 10.3934/mbe.2020014 |
[5] | Muhammad Akram, Ahmad N. Al-Kenani, Anam Luqman . Degree based models of granular computing under fuzzy indiscernibility relations. Mathematical Biosciences and Engineering, 2021, 18(6): 8415-8443. doi: 10.3934/mbe.2021417 |
[6] | Ansheng Ye, Xiangbing Zhou, Kai Weng, Yu Gong, Fang Miao, Huimin Zhao . Image classification of hyperspectral remote sensing using semi-supervised learning algorithm. Mathematical Biosciences and Engineering, 2023, 20(6): 11502-11527. doi: 10.3934/mbe.2023510 |
[7] | Yufeng Qian . Exploration of machine algorithms based on deep learning model and feature extraction. Mathematical Biosciences and Engineering, 2021, 18(6): 7602-7618. doi: 10.3934/mbe.2021376 |
[8] | Muhammad Akram, Ayesha Khan, Uzma Ahmad, José Carlos R. Alcantud, Mohammed M. Ali Al-Shamiri . A new group decision-making framework based on 2-tuple linguistic complex q-rung picture fuzzy sets. Mathematical Biosciences and Engineering, 2022, 19(11): 11281-11323. doi: 10.3934/mbe.2022526 |
[9] | Yuting Zhu, Wenyu Zhang, Junjie Hou, Hainan Wang, Tingting Wang, Haining Wang . The large-scale group consensus multi-attribute decision-making method based on probabilistic dual hesitant fuzzy sets. Mathematical Biosciences and Engineering, 2024, 21(3): 3944-3966. doi: 10.3934/mbe.2024175 |
[10] | Jiahui Wen, Haitao Gan, Zhi Yang, Ran Zhou, Jing Zhao, Zhiwei Ye . Mutual-DTI: A mutual interaction feature-based neural network for drug-target protein interaction prediction. Mathematical Biosciences and Engineering, 2023, 20(6): 10610-10625. doi: 10.3934/mbe.2023469 |
Recently, classification systems have a wide range in many fields such as text classification, intrusion detection, bio-informatics, and image retrieval [1]. Unfortunately, a huge amount of data which may include irrelevant or redundant features is one of the main challenges of these systems. The negative effect of these undesirable features reduces the classification performance [2]. For this reason, reducing the number of features by finding an effective subset of features is an important task in classification systems [2]. Feature reduction has two techniques: feature selection and feature extraction [3]. Both reduce a high-dimensional dataset into a representative feature subset of low-dimensional. Feature extraction is effective when the original features fail to discriminate the classes [4], but it requires extra computation. Moreover, it changes the true meaning of the original features. In contrast, the feature selection preserves the true meaning of the selected features, which is important for some classification systems [5]. Furthermore, the result of FS is more understandable for domain experts [6].
FS tries to find the best feature subset which represents the dataset well and improves the performance of classification systems [7]. It can be classified into three approaches [6]: wrapper, embedded, and filter. According to an evaluation strategy, wrapper and embedded are called classifier-dependent approaches, while filter is called classifier-independent approach [8]. In this paper, we use the filter approach according to its advantages over wrapper or embedded approaches in terms of efficiency, simplicity, scalability, practicality, and classifier-independently [6,9]. Filter approach is a pre-processing task which finds the highly ranked features to be the input of classification systems [7,10]. There are two criteria to rank features: feature relevance, and feature redundancy [11]. Feature relevance is related to how features discriminate different classes, while feature redundancy is related to how features share the same information of each other [12]. To define these criteria, filter approach uses many weighting functions which rank features based on their significance [10] such as correlation [13], mutual information (MI) [14]. MI overcomes the weakness of correlation, whereas, correlation is suitable only for linear relationship and numerical features [1]. MI is suitable for any kind of relationship such as linear and non-linear. Moreover, MI deals with both numerical and categorical features [1].
Although MI has been widely used in many methods to find the best feature subset that maximizes the relevancy between the candidate feature and class label, and minimizes the redundancy between the candidate feature and pre-selected features [15]. The main limitations of these methods are: (1) difficult to indicate the best candidate features with the same new classification information [16], (2) difficult to deal with continuous features without information loss [17], and (3) consider the inner-class information only [18]. In this paper, we integrate fuzzy concept with mutual information to propose a new FS method called Fuzzy Joint Mutual Information Maximization (FJMIM). The fuzzy concept helps the proposed method to exploit all possible information of data where it can deal with any numerical data and extract the inner and outer-class information. Moreover, the objective function of FJMIM can overcome the feature overestimation problem which happens when the candidate feature be completely correlated with some of pre-selected features and does not depend on the majority of the subset at the same time [8].
The rest of this paper is organized as follows: Section 2 presents the basic measures of fuzzy information theory. Then, we present the proposed method in section 3. After that, the experiment design was presented in section 4, followed by the results and discussion in section 5. Finally, section 6 concludes the paper.
For the purpose of measuring the significance of features, information theory introduced many information measures such as entropy, and mutual information. To enhance these measures, fuzzy concept is used to estimate new extensions of information measures based on fuzzy equivalence relations such as fuzzy entropy, and fuzzy mutual information [19,20]. Fuzzy entropy measures the average amount of uncertainty of fuzzy relation in order to estimate its discriminative power, while fuzzy mutual information measures the shared amount of information between two fuzzy relations. In the following, we present the basic measures of fuzzy information theory:
Given a dataset D=F∪C, where F is a set of n features, and C is the class label. Let ˉF={a1,a2,…..,am} be a feature of m samples, where ˉF∈F. Let S is the feature subset with d of selected features, and the remaining set is {F−S}, where ˉFf∈F−S and ˉFs∈S. Based on the fuzzy equivalence relation RˉF on ˉF, the feature ˉF can be represented by the relation matrix M(RˉF).
M(RˉF)=(r11r12…r1mr21……r2m…………rm1rm2…rmm) | (2.1) |
where rij=RˉF(ai,aj) is the fuzzy equivalence relation between two samples ai and aj.
In this paper, the used fuzzy equivalence relation between two elements ai and aj is defined as [21]:
RˉF(ai,aj)=exp−‖ai−aj‖ | (2.2) |
Fuzzy equivalence class of sample ai on RˉF can be defined as:
[ai]RˉF=[ri1]a1+[ri2]a2+⋯+[rim]am | (2.3) |
Fuzzy entropy of feature ¯F1 based on fuzzy equivalence relation is defined as:
H(ˉF)=1mm∑i=1logm|[ai]RˉF| | (2.4) |
where |[ai]RˉF|=∑mi=1rij.
Let ¯F1 and ¯F2 be two features of F, fuzzy joint entropy of ¯F1 and ¯F2 is defined as:
H(¯F1,¯F2)=H(R¯F1,R¯F2)=1mm∑i=1logm|[ai]R¯F1∩[ai]R¯F2| | (2.5) |
Fuzzy conditional entropy of ¯F1 given ¯F2 is defined as
H(¯F1|¯F2)=H(R¯F1|R¯F2)=1mm∑i=1log|[ai]R¯F2||[ai]R¯F1∩[ai]R¯F2| | (2.6) |
Fuzzy Mutual information between two features ¯F1 and ¯F2 is defined as:
I(¯F1;¯F2)=I(R¯F1;R¯F2)=1mm∑i=1logm|[ai]R¯F1∩[ai]R¯F2||[ai]R¯F1.[ai]R¯F2| | (2.7) |
Fuzzy conditional mutual information between feature ¯F1 and ¯F2 given class C is defined as:
I(¯F1;¯F2|C)=H(¯F1|C)+H(¯F2|C)−H(¯F1,¯F2|C) | (2.8) |
Fuzzy joint mutual information between two features ¯F1, ¯F2 and class C is defined as:
I(¯F1,¯F2;C)=I(¯F1;C)+I(¯F2;C|¯F1) | (2.9) |
Fuzzy interaction information between among ¯F1, ¯F2 and C is defined as:
I(¯F1;¯F2;C)=I(¯F1;C)+I(¯F2;C)−I(¯F1,¯F2;C) | (2.10) |
In this section, we presented the general theoretical frameworks of different feature selection methods based on mutual information. Then, we studied the limitation of previous work. Finally, we introduced the proposed method.
Brown et al. [22] studied the exist feature selection methods based on MI and analyzed the different criteria to propose the following theoretical framework of these methods.
J(ˉFf)=I(ˉFf;C)−β∑ˉFs∈SI(ˉFf;ˉFs)+γ∑ˉFs∈SI(ˉFf;ˉFs|C) | (3.1) |
This framework is a linear combination of three terms: relevance, redundancy, and conditional that measures the individual predictive power of the feature, the unconditional relation, and the class-conditional relation, respectively. The criteria of different feature selection based on MI depends on the value of β and γ. MIM (β=γ=0) [23] is the simplest FS method based on MI. It considers only the relevance relation only. However, It may suffer from the redundant features. MIFS (γ=0) [24] introduced two criteria to estimate the feature relevance and redundancy. An extension of MIFS, called MIFS-U [24] is proposed to improve the redundancy term of MIFS by considering the uniform distribution of the information. However, Both MIFS and MIFS-U still require an input parameter β. To avoid this limitation, MRMR (β=1|S|, γ=0) [25] introduced the mean of the redundancy term as automatic value to the input parameter (β). JMI (β=γ=1|S|) [26] extended MRMR to extract the benefit of conditional term. In addition, Brown et al. [22] introduced also a similar non-linear framework to represent some methods as CMIM method [27]. According to [22], CMIM can be written as:
Jcmim=minˉFs∈S[I(ˉFf;C|ˉFs)]=I(ˉFf;C)−maxˉFs∈S[I(ˉFf;ˉFs)−I(ˉFf;ˉFs|C)] | (3.2) |
The reason of the non-linear relation on CMIM returns to the using of max operation. Similar to CMIM, JMIM [8] introduces a non-linear relation as follows:
Jjmim=minˉFs∈S[I(ˉFs;C)+I(ˉFf;C|ˉFs)]=I(ˉFf;C)−maxˉFs∈S[I(ˉFf;ˉFs)−I(ˉFf;ˉFs|C)−I(ˉFs;C)] | (3.3) |
Although MI has been widely used in many feature selection methods such as MIFS [24], JMI [26], mRMR [25], DISR [28], IGFS [29], NMIFS [30] and MIFS-ND [31]. These methods suffer from the overestimation of the feature significance problem [8]. For this reason, Bennasar et al. [8] proposed JMIM method to address the overestimation of the feature significance problem. However, it may fail to select the best candidate features if they have the same new classification information. To illustrate this problem, Figure 1 shows the FS scenario, where ¯F1 and ¯F2 are two candidate features, ¯Fs is the pre-selected feature subset, and C is the class label. ¯F1 is partially redundant with ¯Fs, while ¯F2 is independent to ¯Fs. Suppose that ¯F1 and ¯F2 have the same new classification information I(¯F1;C|¯Fs) = (area 3) and I(¯F2;C|¯Fs) = (area 5) respectively. In this case, JMIM may fail to indicate the best feature where I(¯F1,¯Fs;C) and I(¯F1,¯Fs;C) are equal.
Unfortunately, JMIM also shares some limitations with the previous methods. Firstly, it can not directly estimate MI between continuous features [17]. To address this limitation, there are two methods were introduced. One is to estimate MI based on Parzen window [18], but it is inefficient in high-dimensional feature spaces with spare samples [17]. Moreover, its performance depends on the used window function which requires a window width parameter [32]. The other one is to discretize continuous features before estimating MI [33], but it may cause information loss [34]. Secondly, JMIM depends only on inner-class information without considering outer-class information [18].
Motivated by the previous limitation of JMIM, we proposed a new FS method, called Fuzzy Joint Mutual Information Maximization (FJMIM). Both of FJMIM and JMIM depends on "maximum of the minimum" approach. The main difference is that JMIM maximize the joint mutual information of the candidate feature and pre-selected feature subset with class, whereas FJMIM maximizes the joint mutual information of the candidate feature and pre-selected feature subset with class without considering the class-relevant redundancy. To illustrate the difference in Figure 1, JMIM depends on the union of areas 2, 4 and 3, while FJMIM depends on the union of areas 2 and 3. The proposed method discarded the class-relevant redundancy (area 4) because it can reduce the predictive ability of the feature subset when a candidate feature is selected [15]. On the other hand, integrating fuzzy concept with MI has many benefits. Firstly, using a fuzzy concept helps to deal directly with continuous features. Furthermore, it enables MI to take the advantages of inner and outer-class information [35]. Moreover, FS methods based on fuzzy concept are more robust toward any change of the data than methods based on probability concept [36].
According to FJMIM, the candidate feature ˉFf must satisfy the following condition
ˉFf=argmaxˉFf∈F−S(minˉFs∈S(I(ˉFf,ˉFs;C)−I(ˉFf;ˉFs;C))) | (3.4) |
FJMIM also can be written according to the non-linear framework as follows:
ˉFf=2∗I(ˉFf;C)−maxˉFs∈S[I(ˉFf;ˉFs)−I(C;ˉFs)−I(ˉFf;ˉFs|C)−I(ˉFf;C|ˉFs)] | (3.5) |
The proposed method can be summarized to find the best feature subset of size d as follows:
Input: F is a set of n features, C is the class label, and d is the number of selected features.
Step 1: Initialize the empty selected feature subset S.
Step 2: Update the selected feature set S and the feature set F.
Step 2.1: Compute I(ˉF;C) for all ˉF in the feature set F.
Step 2.2: Add the feature ˉF that maximizes I(ˉF;C) to the selected feature set S.
Step 2.3: Remove the feature ˉF from the feature set F.
Step 3: Repeat until |S|=d
Step 3.1: Add the feature ˉF that satisfies
argmaxˉFf∈F−S(minˉFs∈S(I(ˉFf,ˉFs;C)−I(ˉFf;ˉFs;C)))
to the selected feature set S.
Step 3.2: Remove the feature ˉF from the feature set F.
Output: Return the selected feature set S.
Success of FS methods depends on different criteria such as classification performance, and stability [11]. Consequently, we design the experiment based on these criteria (Figure 2). To clarify our improvement, we compared our proposed method FJMIM, with four conventional methods (CMIM [27], JMI [26], QPFS [37], Relief [38]) and five state-of-the-art methods (CMIM3 [39], JMI3 [39], JMIM [8], MIGM [40], and WRFS [41]). The compared methods can be divided into two groups: FS based on fuzzy concept and FS based on probability concept. For the methods which depend on probability concept, data discretization is required as a pre-processing step prior to the FS process. So, the continuous features are transformed into ten bins using EqualWidth discretization [42]. Then, we selected feature subset from all methods based on threshold which is defined as the median position of the ranked features (or the nearest integer position when the number of ranked features is even).
FS plays an important role to improve the classification performance. There are many measures to evaluate classification performance such as classification accuracy, precision, F-measure, area-under-ROC (AUC), and area-under-PRC (AUCPR) [43]. To clarify our improvement, popular classifiers were used in this study such as Naive Bayes (NB), Support Vector Machine (SVM), and 3-Nearest Neighbors (KNN) [44]. The average classification performance measures were computed by 10-fold cross-validation approach [45].
Another important evaluation criterion for FS is stability. FS stability measures the impact of any change in the input data on FS result [46]. In this study, we measure the impact of noise on the selected feature subset. Firstly, we produce the noise using standard deviation and the normal distribution of each feature [47]. Then, we injected 10% of the data by adding noise. After that, we repeated this step ten times. Each time produces a different sequence of selected features. Finally, we computed the stability of each method using Kuncheva stability index [48].
Our experiment was conducted using 13 datasets from UCI machine learning repository [49]. Table 1 presents a brief description about these datasets.
No. | Datasets | Instances | Features | Classes |
1 | Acute Inflammations | 120 | 6 | 2 |
2 | Arrhythmia | 452 | 279 | 13 |
3 | Blogger | 100 | 6 | 2 |
4 | Diabetic Retinopathy Debrecen (DRD) | 1151 | 20 | 2 |
5 | Hayes-Roth | 160 | 5 | 3 |
6 | Indian Liver Patient Dataset (ILPD) | 583 | 10 | 2 |
7 | Lenses | 24 | 4 | 3 |
8 | Lymphography | 148 | 18 | 4 |
9 | Congressional Voting Records (CVR) | 435 | 16 | 2 |
10 | Sonar | 208 | 60 | 2 |
11 | Thoracic Surgery | 470 | 17 | 2 |
12 | Wilt | 4889 | 6 | 2 |
13 | Zoo | 101 | 17 | 7 |
1) Accuracy: A paired two-tailed t-test is employed between FJMIM and other compared methods. The notations ([=], [+], and [-]) indicate the statistically significant (5%) that the proposed method (equals, wins, and losses) other methods. According to NB classifier, FJMIM achieved the maximum average accuracy with score 78.02%, while Relief achieved the minimum average accuracy with score 75.34% (Table 2). The proposed method outperformed compared methods in the range from 0.06 to 2.68%. In SVM classifier, FJMIM outperformed other methods with score 80.03%, while Relief achieved the minimum average accuracy with score 77.33% (Table 3). The proposed method outperformed compared methods in the range from 0.69 to 2.7%. Similarly with KNN classifier, FJMIM kept the maximum average accuracy by 81.45%, while Relief achieved the minimum average accuracy with score 77.98% (Table 4). The proposed method outperformed compared methods in the range from 0.57 to 3.47%. Across all datasets, Figure 3(a) shows the distribution of the average accuracy values of all used classifiers. In a detailed box-plot, the box represents upper and lower quartiles, while the black circle represents the median. The box-plot confirms that FJMIM is more consistent and outperformed other compared methods. Figure 3(b) shows the average accuracy of the three used classifiers. FJMIM achieved the best accuracy, followed by QPFS, JMI3, both of JMI and CMIM, both of CMIM3 and JMIM, MIGM, WRFS, and Relief respectively. The proposed method outperformed compared methods in the range from 0.6 to 2.9%.
Dataset | CMIM | CMIM3 | JMI | JMI3 | JMIM | MIGM | QPFS | Relief | WRFS | FJMIM |
Acute Inflammations | 100±0[=] | 100±0[=] | 100±0[=] | 100±0[=] | 100±0[=] | 99.75±2.5[=] | 99.75±2.5[=] | 100±0[=] | 90.83±8.5[+] | 100±0 |
Arrhythmia | 60.64±7.01[=] | 59.22±6.17[=] | 59.42±6.49[=] | 59.58±6.58[=] | 60.62±6.93[=] | 58.98±6.39[=] | 63.7±6.57[-] | 64.94±6.33[-] | 59.31±6.3[=] | 59.74±6.43 |
Blogger | 61.9±10.7[=] | 61.9±10.7[=] | 61.9±10.7[=] | 64.9±10.2[=] | 61.9±10.7[=] | 61.9±10.7[=] | 61.9±10.7[=] | 65.4±7.84[=] | 66.1±9.09[=] | 65.9±10.26 |
DRD | 57.59±4.95[=] | 60.66±4.69[-] | 60.3±5.04[-] | 60.6±4.58[-] | 57.59±4.95[=] | 60.3±5.04[-] | 61.19±4.49[-] | 60.61±5.06[-] | 57.47±4.98[=] | 57.56±4.83 |
Hayes-Roth | 45.81±10.77[=] | 45.81±10.77[=] | 45.81±10.77[=] | 45.81±10.77[=] | 45.81±10.77[=] | 45.81±10.77[=] | 49.37±10.53[=] | 46.31±11.48[=] | 45.81±10.77[=] | 49.37±10.53 |
ILPD | 68.99±5.68[=] | 67.33±5.87[=] | 68.99±5.68[=] | 67.33±5.87[=] | 68.99±5.68[=] | 68.99±5.68[=] | 68.73±5.64[=] | 64.96±6.45[+] | 70.11±6.74[=] | 69.54±5.75 |
Lenses | 86.83±21.88[=] | 86.83±21.88[=] | 86.83±21.88[=] | 86.83±21.88[=] | 86.83±21.88[=] | 86.83±21.88[=] | 86.83±21.88[=] | 54.83±30.73[+] | 86.83±21.88[=] | 86.83±21.88 |
Lymphography | 79.02±10.57[=] | 82.05±9.59[=] | 79.02±10.57[=] | 77.93±10.87[=] | 78.27±10.98[=] | 77.25±11.38[=] | 79.02±10.57[=] | 82.61±9.77[=] | 76.91±11.64[+] | 81.5±10.29 |
CVR | 94.3±3.15[=] | 94.32±3.12[=] | 93.75±3.57[=] | 93.75±3.57[=] | 93.75±3.57[=] | 93.75±3.57[=] | 94.32±3.26[=] | 93.82±3.28[=] | 93.52±3.18[=] | 93.75±3.57 |
Sonar | 75.05±8.33[=] | 75.42±9.08[=] | 75.75±8.85[=] | 76.23±9.99[=] | 72.2±9.93[=] | 72.14±9.54[+] | 76.42±8.69[=] | 74.25±8.33[=] | 77.91±8.74[=] | 77±8.98 |
Thoracic Surgery | 83.83±3.1[=] | 83.38±3.09[=] | 83.38±3.09[=] | 83.85±2.24[=] | 83.38±3.09[=] | 84.64±1.83[=] | 83.55±3.08[=] | 83.06±2.77[=] | 83.85±2.74[=] | 83.38±3.09 |
Wilt | 94.61±0.06[=] | 94.61±0.06[=] | 94.61±0.06[=] | 94.61±0.06[=] | 94.61±0.06[=] | 94.61±0.06[=] | 94.61±0.06[=] | 94.61±0.06[=] | 94.61±0.06[=] | 94.61±0.06 |
Zoo | 95.15±5.74[=] | 96.05±5.6[=] | 96.03±5.66[=] | 96.03±5.66[=] | 96.03±5.66[=] | 96.93±5.42[=] | 94.08±6.6[=] | 94.08±6.6[=] | 92.96±7.05[=] | 95.05±5.87 |
Average | 77.21 | 77.51 | 77.37 | 77.50 | 76.92 | 77.07 | 77.96 | 75.34 | 76.63 | 78.02 |
Dataset | CMIM | CMIM3 | JMI | JMI3 | JMIM | MIGM | QPFS | Relief | WRFS | FJMIM |
Acute Inflammations | 98.33±3.35[=] | 99.67±1.64[=] | 98.33±3.35[=] | 98.33±3.35[=] | 98.33±3.35[=] | 99.42±2.44[=] | 99.42±2.44[=] | 100±0[+] | 89.92±9.5[+] | 99.42±2.44 |
Arrhythmia | 66.09±6.81[=] | 64.08±6.11[=] | 65.01±5.96[=] | 65.7±6.23[=] | 66.14±6.61[=] | 65.23±6.39[=] | 66.82±6.2[=] | 67.22±6.05[=] | 65.23±6.15[=] | 67.11±6.7 |
Blogger | 68±4.02[=] | 67.7±4.89[=] | 68±4.02[=] | 67.4±5.62[=] | 68±4.02[=] | 68±4.02[=] | 67.7±4.89[=] | 67.7±4.89[=] | 68±4.02[=] | 67.7±4.89 |
DRD | 68.03±3.92[=] | 67.55±4.07[=] | 67.15±3.85[=] | 67.03±3.95[=] | 68.03±3.92[=] | 67.19±3.8[=] | 65.1±4.33[+] | 61.91±4.51[+] | 67.7±4[=] | 67.96±4.23 |
Hayes-Roth | 49.94±12.83[=] | 49.94±12.83[=] | 49.94±12.83[=] | 49.94±12.83[=] | 49.94±12.83[=] | 49.94±12.83[=] | 52±11.61[=] | 48.75±13.21[=] | 49.94±12.83[=] | 52±11.61 |
ILPD | 71.36±0.73[=] | 71.36±0.73[=] | 71.36±0.73[=] | 71.36±0.73[=] | 71.36±0.73[=] | 71.36±0.73[=] | 71.36±0.73[=] | 71.36±0.73[=] | 71.36±0.73[=] | 71.36±0.73 |
Lenses | 76.17±22.13[=] | 76.17±22.13[=] | 76.17±22.13[=] | 76.17±22.13[=] | 76.17±22.13[=] | 76.17±22.13[=] | 76.17±22.13[=] | 54.5±30.42[+] | 76.17±22.13[=] | 76.17±22.13 |
Lymphography | 81.57±10.06[=] | 80.56±9.09[=] | 81.42±10.15[=] | 84.55±8.84[=] | 82.02±10.48[=] | 79.06±10.44[+] | 81.57±10.11[=] | 80.3±9[=] | 74.25±11.45[+] | 85.5±8.3 |
CVR | 94.09±3.33[=] | 94.46±3.37[=] | 94.3±3.34[=] | 94.32±3.32[=] | 94.3±3.34[=] | 94.3±3.34[=] | 94±3.44[=] | 94.43±3.41[=] | 94.37±3.41[=] | 94.3±3.34 |
Sonar | 79.34±8.37[=] | 80.48±8.05[=] | 80.18±8.33[=] | 78.46±8.44[=] | 80.54±8.43[=] | 76.93±8.71[=] | 79.91±7.73[=] | 82.1±8.07[=] | 77.41±8.41[=] | 80.67±7.88 |
Thoracic Surgery | 85.11±0[=] | 85.11±0[=] | 85.11±0[=] | 85.11±0[=] | 85.11±0[=] | 85.11±0[=] | 85.11±0[=] | 85.11±0[=] | 85.11±0[=] | 85.11±0 |
Wilt | 97.62±0.57[+] | 97.97±0.49[=] | 97.97±0.49[=] | 97.97±0.49[=] | 97.62±0.57[+] | 97.97±0.49[=] | 94.61±0.06[+] | 97.97±0.49[=] | 97.62±0.57[+] | 97.98±0.49 |
Zoo | 95.75±5.12[=] | 93.88±6.72[=] | 89.39±5.72[+] | 89.49±5.64[+] | 89.49±5.64[+] | 94.06±6.9[=] | 95.27±5.85[=] | 93.89±6.96[=] | 90.49±7.96[+] | 95.06±6.2 |
Average | 79.34 | 79.15 | 78.79 | 78.91 | 79.00 | 78.83 | 79.16 | 77.33 | 77.51 | 80.03 |
Dataset | CMIM | CMIM3 | JMI | JMI3 | JMIM | MIGM | QPFS | Relief | WRFS | FJMIM |
Acute Inflammations | 99.5±1.99[=] | 100±0[=] | 99.5±1.99[=] | 99.5±1.99[=] | 99.5±1.99[=] | 100±0[=] | 100±0[=] | 100±0[=] | 99.67±1.64[=] | 100±0 |
Arrhythmia | 58.27±5.17[+] | 57.63±5.17[+] | 57.79±5.34[+] | 58.32±5.08[+] | 58.94±5.13[=] | 57.67±5.21[+] | 60.73±4.64[=] | 65.13±4.43[-] | 58.39±5.22[+] | 61.11±5.23 |
Blogger | 72.1±11.31[=] | 72.1±11.31[=] | 72.1±11.31[=] | 78.8±10.18[=] | 72.1±11.31[=] | 72.1±11.31[=] | 72.1±11.31[=] | 70.3±11.41[=] | 76.1±12.05[=] | 78.6±11.37 |
DRD | 64.3±4.19[=] | 62.62±5.29[=] | 63.69±4.58[=] | 61.96±4.68[=] | 64.3±4.19[=] | 63.58±4.56[=] | 60.47±4.64[+] | 63.51±4.63[=] | 64.06±4.4[=] | 64.7±4.23 |
Hayes-Roth | 59.19±10.41[=] | 59.19±10.41[=] | 59.19±10.41[=] | 59.19±10.41[=] | 59.19±10.41[=] | 59.19±10.41[=] | 63.12±11.32[=] | 57.56±10.82[=] | 59.19±10.41[=] | 63.12±11.32 |
ILPD | 67.16±5.41[=] | 68.22±5.06[=] | 67.54±5.44[=] | 68.22±5.06[=] | 67.16±5.41[=] | 67.3±5.42[=] | 68.74±5.01[=] | 67.84±5.56[=] | 66.66±5.25[=] | 69.15±5.62 |
Lenses | 86.83±21.88[=] | 86.83±21.88[=] | 86.83±21.88[=] | 86.83±21.88[=] | 86.83±21.88[=] | 86.83±21.88[=] | 86.83±21.88[=] | 54.17±30.46[+] | 86.83±21.88[=] | 86.83±21.88 |
Lymphography | 84.61±7.58[=] | 72.61±9.14[+] | 84.61±7.58[=] | 84.16±9.46[=] | 80.99±9.49[=] | 76.62±8.87[=] | 84.61±7.58[=] | 81.44±9.42[=] | 79.74±9.36[=] | 78.87±8.35 |
CVR | 93.63±3.43[=] | 94.09±3.33[=] | 95.26±3.01[=] | 95.26±3.01[=] | 95.26±3.01[=] | 95.26±3.01[=] | 94.14±3.37[=] | 93.67±3.5[=] | 94.09±2.95[=] | 95.26±3.01 |
Sonar | 85.09±7.43[=] | 83.51±7.68[=] | 87.74±7.51[=] | 85.53±8.83[=] | 82.7±9.06[=] | 82.4±7.72[+] | 86.15±7.24[=] | 87.95±6.78[=] | 84.17±7.73[=] | 87.65±7.27 |
Thoracic Surgery | 80.96±3.45[=] | 81.38±3.53[=] | 81.38±3.53[=] | 83.45±3.05[=] | 81.38±3.53[=] | 82.64±3.28[=] | 83.89±2.95[=] | 82.68±3.08[=] | 84.85±0.97[-] | 81.38±3.53 |
Wilt | 97.69±0.57[+] | 98.12±0.55[=] | 98.12±0.55[=] | 98.12±0.55[=] | 97.69±0.57[+] | 98.12±0.55[=] | 94.44±0.52[+] | 98.12±0.55[=] | 97.69±0.57[+] | 98.12±0.55 |
Zoo | 91.9±7.21[=] | 92.06±7.35[=] | 92.05±7.24[=] | 92.05±7.24[=] | 92.05±7.24[=] | 91.16±7.48[=] | 91.42±6.94[=] | 91.42±6.94[=] | 90.29±7.45[+] | 94.08±6.6 |
Average | 80.09 | 79.10 | 80.45 | 80.88 | 79.85 | 79.45 | 80.51 | 77.98 | 80.13 | 81.45 |
2) Precision: Figure 4 shows the precision results of NB, SVM, KNN, and their average. FJMIM achieved the highest precision, while Relief achieved the lowest precision. The proposed method outperformed other methods in the range from 0.6 to 12.7% based on NB, from 0.3 to 3.3% based on SVM, and from 2 to 8.2% based on KNN. According to the average of all classifiers, FJMIM achieved the highest precision by 71.2%, while Relief kept the lowest precision by 63.1%. The second-best method achieved by CMIM3, followed by JMI3, QPFS, JMI, JMIM, MIGM, CMIM and WRFS. The results of precision distribution are inconsistent as shown in Figure 5. Both FJMIM and QPFS achieved the best distribution. FJMIM achieved the highest upper quartile and median, while QPFS achieved the highest lower quartile and median. In addition, FJMIM shares the highest upper quartile with JMI, JMIM and MIGM.
3) F-measure: Figure 6 shows the F-measure results of the three used classifiers and their average. FJMIM achieved the highest F-measure by 79.8, 71.5 and 84% on NB, SVM and KNN respectively. Relief achieved the lowest F-measure on NB and SVM, while WRFS achieved the lowest score on SVM. The proposed method outperformed other methods in the range from 0.3 to 16.6% based on NB, from 0.1 to 1.4% based on SVM, and from 0.6 to 15.2% based on KNN. According to the average of all classifiers, FJMIM achieved the highest precision by 78.4% and outperformed other methods in the range from 2.5 to 10.8%. The second-best method achieved by JMI3, followed by QPFS, CMIM3, JMI, JMIM, CMIM, MIGM, WRFS and Relief. Figure 7 shows the distribution of F-measure across all datasets. The box-plot confirms the outperformance of FJMIM compared to other methods.
4) AUC: Figure 8 shows the AUC results of the used classifiers and their average. FJMIM achieved the highest AUC on NB and KNN by 83.9 and 85.2%, while it achieved the second-best score on SVM by 74.8%. On the other hand, Relief achieved the lowest AUC on all classifiers. Although MIGM achieved the best AUC on SVM, FJMIM outperformed on the average of all classifiers. The proposed method outperformed other methods in the range from 0.4 to 4.2%. As shown in the box-plot (Figure 9), the proposed method achieved the highest lower quartile and median values. On the other hand, JMI achieved also the highest lower quartile, while CMIM achieved the highest upper quartile.
5) AUCPR: The highest AUCPR was achieved by both FJMIM and CMIM3 using NB, MIGM using SVM, and FJMIM using KNN (Figure 10). On the other hand, Relief achieved the lowest AUCPR using all classifiers. According to the average of all classifiers, FJMIM achieved the best AUCPR by 81.6%, while Relief kept the lowest AUCPR by 77.2%. The proposed method outperformed other methods in the range from 0.3 to 4.4%. The second-best AUCPR was achieved by CMIM3, followed by MIGM, both QPFS and JMIM, both CMIM and JMI, and both JMI3 and WRFS. Figure 11 shows the distribution of AUCPR across all datasets. It's obvious that CMIM achieved the highest median, lower, and upper quartiles, while FJMIM achieved the highest median, upper quartile.
Figure 12(a) shows the stability of the used FS methods on all datasets. It's obvious that FJMIM is more consistent and stable compared to all other methods. Figure 12(b) confirms the stability of the compared method. FJMIM achieved the highest average stability by 87.8%. The proposed method outperformed other methods in the range from 6.6 to 43%. JMI achieved the second-best position by 81.2%, while Relief achieved the lowest stability by 44.3%.
More detailed results are presented in the appendix section. According to previous results, It is obvious that FJMIM achieves the best results in most measures. This is expected because our proposed method addresses the feature overestimation problem and handle the candidate feature problem well. Moreover, it avoids the discretization step. Another reason is the advantages of both inner and outer class information which FJMIM depends on it. This information helps the proposed method to be more robust toward the noise. On the other hand, the other compared methods are close to FJMIM than Relief that achieved the lowest result. This is because all compared method except Relief depends on mutual information as the proposed method to estimate the significant of features.
In this paper, we propose a new FS method, called, Fuzzy Joint Mutual Information Maximization (FJMIM). The proposed method depends on integrating an improved JMIM objective function with fuzzy concept. The benefits of our proposed method include: 1) The ability to deal directly with discrete and continuous features. 2) The suitability to handle any kind of relations between features such as linear and non-linear relation. 3) The ability to take the advantages of inner and outer class information. 4) The robustness toward the noise. 5) The ability to select the most significant feature subset and avoiding the undesirable features.
To confirm the effectiveness of FJMIM, 13 benchmark datasets have been used to evaluate the proposed method in the term of classification performance (accuracy, precision, F-measure, AUC, and AUCPR) and feature selection stability. According to nine conventional and state-of-the-art feature selection methods, the proposed method achieved promising improvement on feature selection in the terms of classification performance and stability.
In future work, we plan to extend the proposed method to cover multi-label classification problem. Moreover, we plan to study the effectiveness of imbalanced data on the proposed method.
This research has been supported by the National Natural Science Foundation (61572368).
The authors declare no conflict of interest.
Tables A1–A6 show some numerical results of Figures 3(b), 4, 6, 8, 10 and 12(b), while Figures A1–A4 show the statistical results (mean ± standard deviation) of some datasets (DRD, Sonar, Wilt and Zoo) by precision, F-measure, AUC and AUCPR, respectively.
FS method | Average |
CMIM | 78.9 |
CMIM3 | 78.6 |
JMI | 78.9 |
JMI3 | 79.1 |
JMIM | 78.6 |
MIGM | 78.4 |
QPFS | 79.2 |
Relief | 76.9 |
WRFS | 78.1 |
FJMIM | 79.8 |
FS method | NB | SVM | KNN | Average |
CMIM | 0.702 | 0.641 | 0.704 | 0.682 |
CMIM3 | 0.742 | 0.641 | 0.705 | 0.696 |
JMI | 0.705 | 0.640 | 0.713 | 0.686 |
JMI3 | 0.712 | 0.636 | 0.723 | 0.690 |
JMIM | 0.701 | 0.642 | 0.707 | 0.683 |
MIGM | 0.701 | 0.637 | 0.708 | 0.682 |
QPFS | 0.712 | 0.636 | 0.711 | 0.686 |
Relief | 0.621 | 0.612 | 0.661 | 0.631 |
WRFS | 0.698 | 0.632 | 0.708 | 0.679 |
FJMIM | 0.748 | 0.645 | 0.743 | 0.712 |
FS method | NB | SVM | KNN | Average |
CMIM | 0.722 | 0.712 | 0.818 | 0.750 |
CMIM3 | 0.795 | 0.712 | 0.752 | 0.753 |
JMI | 0.724 | 0.712 | 0.823 | 0.753 |
JMI3 | 0.729 | 0.712 | 0.834 | 0.759 |
JMIM | 0.719 | 0.714 | 0.821 | 0.751 |
MIGM | 0.722 | 0.710 | 0.818 | 0.750 |
QPFS | 0.732 | 0.712 | 0.825 | 0.756 |
Relief | 0.632 | 0.708 | 0.688 | 0.676 |
WRFS | 0.716 | 0.701 | 0.826 | 0.748 |
FJMIM | 0.798 | 0.715 | 0.840 | 0.784 |
FS method | NB | SVM | KNN | Average |
CMIM | 0.835 | 0.733 | 0.812 | 0.793 |
CMIM3 | 0.836 | 0.748 | 0.844 | 0.809 |
JMI | 0.837 | 0.734 | 0.815 | 0.795 |
JMI3 | 0.831 | 0.729 | 0.805 | 0.788 |
JMIM | 0.836 | 0.742 | 0.812 | 0.796 |
MIGM | 0.832 | 0.754 | 0.807 | 0.798 |
QPFS | 0.838 | 0.710 | 0.807 | 0.785 |
Relief | 0.804 | 0.707 | 0.801 | 0.771 |
WRFS | 0.831 | 0.732 | 0.806 | 0.789 |
FJMIM | 0.839 | 0.748 | 0.852 | 0.813 |
FS method | NB | SVM | KNN | Average |
CMIM | 0.848 | 0.708 | 0.798 | 0.785 |
CMIM3 | 0.862 | 0.715 | 0.862 | 0.813 |
JMI | 0.850 | 0.708 | 0.797 | 0.785 |
JMI3 | 0.837 | 0.705 | 0.800 | 0.781 |
JMIM | 0.852 | 0.713 | 0.797 | 0.787 |
MIGM | 0.857 | 0.724 | 0.827 | 0.803 |
QPFS | 0.857 | 0.708 | 0.797 | 0.787 |
Relief | 0.842 | 0.685 | 0.791 | 0.772 |
WRFS | 0.850 | 0.697 | 0.795 | 0.781 |
FJMIM | 0.862 | 0.718 | 0.869 | 0.816 |
FS method | Stability |
CMIM | 72.6 |
CMIM3 | 67.3 |
JMI | 81.2 |
JMI3 | 75.2 |
JMIM | 75.9 |
MIGM | 66.2 |
QPFS | 71.7 |
Relief | 44.3 |
WRFS | 66.6 |
FJMIM | 87.8 |
[1] |
L. T. Vinh, S. Lee, Y. Park, B. J. d'Auriol, A novel feature selection method based on normalized mutual information, Appl. Intell., 37 (2012), 100-120. doi: 10.1007/s10489-011-0315-y
![]() |
[2] |
J. R. Vergara, P. A. Estévez, A review of feature selection methods based on mutual information, Neural Comput. Appl., 24 (2014), 175-186. doi: 10.1007/s00521-013-1368-0
![]() |
[3] | I. K. Fodor, A survey of dimension reduction techniques, Lawrence Livermore National Lab, CA (US), 2002. |
[4] |
H. X. Li, L. D. Xu, Feature space theory—a mathematical foundation for data mining, Knowl. Based Syst., 14 (2001), 253-257. doi: 10.1016/S0950-7051(01)00103-4
![]() |
[5] |
R. Thawonmas, S. Abe, A novel approach to feature selection based on analysis of class regions, IEEE Trans. Syst. Man Cybern. Syst., 27 (1997), 196-207. doi: 10.1109/3477.558798
![]() |
[6] |
Y. Saeys, I. Inza, P. Larrañaga, A review of feature selection techniques in bioinformatics, Bioinformatics, 23 (2007), 2507-2517. doi: 10.1093/bioinformatics/btm344
![]() |
[7] | I. Guyon, A. Elisseeff, An introduction to variable and feature selection, J. Mach. Learn. Res., 3 (2003), 1157-1182. |
[8] |
M. Bennasar, Y. Hicks, R. Setchi, Feature selection using joint mutual information maximisation, Expert Syst. Appl., 42 (2015), 8520-8532. doi: 10.1016/j.eswa.2015.07.007
![]() |
[9] |
Q. Hu, D. Yu, Z. Xie, Information-preserving hybrid data reduction based on fuzzy-rough techniques, Pattern Recognit. Lett., 27 (2006), 414-423. doi: 10.1016/j.patrec.2005.09.004
![]() |
[10] | C. Lazar, J. Taminau, S. Meganck, D. Steenhoff, A. Coletta, C. Molter, et al., A survey on filter techniques for feature selection in gene expression microarray analysis, IEEE/ACM Trans. Comput. Biol. Bioinf., 9 (2012), 1106-1119. |
[11] |
G. Chandrashekar, F. Sahin, A survey on feature selection methods, Comput. Electr. Eng., 40 (2014), 16-28. doi: 10.1016/j.compeleceng.2013.11.024
![]() |
[12] |
O. A. Salem, L. Wang, Fuzzy mutual information feature selection based on representative samples, Int. J. Software Innovation, 6 (2018), 58-72. doi: 10.4018/IJSI.2018010105
![]() |
[13] |
D. Mo, S. H. Huang, Feature selection based on inference correlation, Intell. Data Anal., 15 (2011), 375-398. doi: 10.3233/IDA-2010-0473
![]() |
[14] |
R. Steuer, J. Kurths, C. O. Daub, J. Weise, J. Selbig, The mutual information: detecting and evaluating dependencies between variables, Bioinformatics, 18 (2002), S231-S240. doi: 10.1093/bioinformatics/18.suppl_2.S231
![]() |
[15] |
J. Wang, J. M. Wei, Z. Yang, S. Q. Wang, Feature selection by maximizing independent classification information, IEEE Trans. Knowl. Data Eng., 29 (2017), 828-841. doi: 10.1109/TKDE.2017.2650906
![]() |
[16] |
F. Macedo, M. R. Oliveira, A. Pacheco, R. Valadas, Theoretical foundations of forward feature selection methods based on mutual information, Neurocomputing, 325 (2019), 67-89. doi: 10.1016/j.neucom.2018.09.077
![]() |
[17] |
D. Yu, S. An, Q. Hu, Fuzzy mutual information based min-redundancy and max-relevance heterogeneous feature selection, Int. J. Comput. Intell. Syst., 4 (2011), 619-633. doi: 10.1080/18756891.2011.9727817
![]() |
[18] |
J. Liang, K. Chin, C. Dang, R. C. Yam, A new method for measuring uncertainty and fuzziness in rough set theory, Int. J. Gen. Syst., 31 (2002), 331-342. doi: 10.1080/0308107021000013635
![]() |
[19] | Z. Li, P. Zhang, X. Ge, N. Xie, G. Zhang, C. F. Wen, Uncertainty measurement for a fuzzy relation information system, IEEE Trans. Fuzzy Syst., 27 (2019), 2338-2352. |
[20] |
C. Wang, Y. Huang, M. Shao, D. Chen, Uncertainty measures for general fuzzy relations, Fuzzy Sets Syst., 360 (2019), 82-96. doi: 10.1016/j.fss.2018.07.006
![]() |
[21] |
Y. Li, K. Qin, X. He, Some new approaches to constructing similarity measures, Fuzzy Sets Syst., 234 (2014), 46-60. doi: 10.1016/j.fss.2013.03.008
![]() |
[22] | G. Brown, A new perspective for information theoretic feature selection, Artif. Intell. Stat., 2009, 49-56. |
[23] | D. D. Lewis, Feature selection and feature extract ion for text categorization, Speech and Natural Language: Proceedings of a Workshop Held at Harriman, New York, 1992, 23-26. |
[24] |
R. Battiti, Using mutual information for selecting features in supervised neural net learning, IEEE Trans. Neural Netw. Learn. Syst., 5 (1994), 537-550. doi: 10.1109/72.298224
![]() |
[25] |
H. Peng, F. Long, C. Ding, Feature selection based on mutual information criteria of max-dependency, max-relevance, and min-redundancy, IEEE Trans. Pattern Anal. Mach. Intell., 27 (2005), 1226-1238. doi: 10.1109/TPAMI.2005.159
![]() |
[26] | H. Yang, J. Moody, Feature selection based on joint mutual information, Proc. Int. ICSC Symp. Adv. Intell. Data Anal., 1999, 22-25. |
[27] | F. Fleuret, Fast binary feature selection with conditional mutual information, J. Mach. Learn. Res., 5 (2004), 1531-1555. |
[28] | P. E. Meyer, G. Bontempi, On the use of variable complementarity for feature selection in cancer classification, Workshops on applications of evolutionary computation, Springer, Berlin, Heidelberg, 2006, 91-102. |
[29] | A. El Akadi, A. El Ouardighi, D. Aboutajdine, A powerful feature selection approach based on mutual information, Int. J. Comput. Sci. Network Secur., 8 (2008), 116. |
[30] |
P. A. Estévez, M. Tesmer, C. A. Perez, J. M. Zurada, Normalized mutual information feature selection, IEEE Trans. Neural Networks, 20 (2009), 189-201. doi: 10.1109/TNN.2008.2005601
![]() |
[31] |
N. Hoque, D. Bhattacharyya, J. K. Kalita, Mifs-nd: a mutual information-based feature selection method, Expert Syst. Appl., 41 (2014), 6371-6385. doi: 10.1016/j.eswa.2014.04.019
![]() |
[32] |
G. Herman, B. Zhang, Y. Wang, G. Ye, F. Chen, Mutual information-based method for selecting informative feature sets, Pattern Recognit., 46 (2013), 3315-3327. doi: 10.1016/j.patcog.2013.04.021
![]() |
[33] |
J. Y. Ching, A. K. Wong, K. C. C. Chan, Class-dependent discretization for inductive learning from continuous and mixed-mode data, IEEE Trans. Pattern Anal. Mach. Intell., 17 (1995), 641-651. doi: 10.1109/34.391407
![]() |
[34] |
Q. Shen, R. Jensen, Selecting informative features with fuzzy-rough sets and its application for complex systems monitoring, Pattern Recognit., 37 (2004), 1351-1363. doi: 10.1016/j.patcog.2003.10.016
![]() |
[35] |
J. Zhao, Z. Zhang, C. Han, Z. Zhou, Complement information entropy for uncertainty measure in fuzzy rough set and its applications, Soft Comput., 19 (2015), 1997-2010. doi: 10.1007/s00500-014-1387-5
![]() |
[36] |
H.-M. Lee, C.-M. Chen, J.-M. Chen, Y.-L. Jou, An efficient fuzzy classifier with feature selection based on fuzzy entropy, IEEE Trans. Syst. Man Cybern. Syst., 31 (2001), 426-432. doi: 10.1109/3477.931536
![]() |
[37] | I. Rodriguez-Lujan, R. Huerta, C. Elkan, C. S. Cruz, Quadratic programming feature selection, J. Mach. Learn. Res., 11 (2010), 1491-1516. |
[38] | K. Kira, L. A. Rendell, The feature selection problem: Traditional methods and a new algorithm, Aaai, 2 (1992), 129-134. |
[39] |
K. Sechidis, L. Azzimonti, A. Pocock, G. Corani, J. Weatherall, G. Brown, Efficient feature selection using shrinkage estimators, Mach. Learn., 108 (2019), 1261-1286. doi: 10.1007/s10994-019-05795-1
![]() |
[40] |
X. Wang, B. Guo, Y. Shen, C. Zhou, X. Duan, Input feature selection method based on feature set equivalence and mutual information gain maximization, IEEE Access, 7 (2019), 151525-151538. doi: 10.1109/ACCESS.2019.2948095
![]() |
[41] | P. Zhang, W. Gao, G. Liu, Feature selection considering weighted relevancy, Appl. Intell., 1-11. |
[42] | S. Garcia, J. Luengo, J. A. Sáez, V. Lopez, F. Herrera, A survey of discretization techniques: Taxonomy and empirical analysis in supervised learning, IEEE Trans. Knowl. Data Eng., 25 (2012), 734-750. |
[43] | A. Tharwat, Classification assessment methods, Appl. Comput. Inform., 2020. |
[44] | M. Allahyari, S. Pouriyeh, M. Assefi, S. Safaei, E. D. Trippe, J. B. Gutierrez, et al., A brief survey of text mining: Classification, clustering and extraction techniques, preprint, arXiv: 1707.02919. |
[45] | R. Kohavi, A study of cross-validation and bootstrap for accuracy estimation and model selection, Ijcai, 14 (1995), 1137-1145. |
[46] | S. Nogueira, G. Brown, Measuring the stability of feature selection, Joint European conference on machine learning and knowledge discovery in databases, Springer, Cham, 2016,442-457. |
[47] | Y. S. Tsai, U. C. Yang, I. F. Chung, C. D. Huang, A comparison of mutual and fuzzy-mutual information-based feature selection strategies, 2013 IEEE international conference on fuzzy systems (FUZZ-IEEE), IEEE, 2013, 1-6. |
[48] | L. I. Kuncheva, A stability index for feature selection, Artificial intelligence and applications, 2007,421-427. |
[49] | D. Dua, C. Graff, UCI machine learning repository, 2017. Available from: http://archive.ics.uci.edu/ml. |
1. | Lin Sun, Shanshan Si, Jing Zhao, Jiucheng Xu, Yaojin Lin, Zhiying Lv, Feature selection using binary monarch butterfly optimization, 2023, 53, 0924-669X, 706, 10.1007/s10489-022-03554-9 | |
2. | Amjad Qtaish, Malik Braik, Dheeb Albashish, Mohammad T. Alshammari, Abdulrahman Alreshidi, Eissa Jaber Alreshidi, Enhanced coati optimization algorithm using elite opposition-based learning and adaptive search mechanism for feature selection, 2024, 1868-8071, 10.1007/s13042-024-02222-3 | |
3. | Xi-Ao Ma, Hao Xu, Yi Liu, Justin Zuopeng Zhang, Class-specific feature selection using fuzzy information-theoretic metrics, 2024, 136, 09521976, 109035, 10.1016/j.engappai.2024.109035 | |
4. | Lei Xiao, 2024, Evaluation Model Construction Based on 'Three-dimensional' Data Fusion in Cross-cultural Blended Course, 9798400710360, 11, 10.1145/3686424.3686426 | |
5. | Pedro J. Gutiérrez-Diez, Jorge Alves-Antunes, Stock market uncertainty determination with news headlines: A digital twin approach, 2023, 9, 2473-6988, 1683, 10.3934/math.2024083 | |
6. | Ah. E. Hegazy, B. Hafiz, M. A. Makhlouf, Omar A. M. Salem, Optimizing medical data classification: integrating hybrid fuzzy joint mutual information with binary Cheetah optimizer algorithm, 2025, 28, 1386-7857, 10.1007/s10586-025-05102-9 |
No. | Datasets | Instances | Features | Classes |
1 | Acute Inflammations | 120 | 6 | 2 |
2 | Arrhythmia | 452 | 279 | 13 |
3 | Blogger | 100 | 6 | 2 |
4 | Diabetic Retinopathy Debrecen (DRD) | 1151 | 20 | 2 |
5 | Hayes-Roth | 160 | 5 | 3 |
6 | Indian Liver Patient Dataset (ILPD) | 583 | 10 | 2 |
7 | Lenses | 24 | 4 | 3 |
8 | Lymphography | 148 | 18 | 4 |
9 | Congressional Voting Records (CVR) | 435 | 16 | 2 |
10 | Sonar | 208 | 60 | 2 |
11 | Thoracic Surgery | 470 | 17 | 2 |
12 | Wilt | 4889 | 6 | 2 |
13 | Zoo | 101 | 17 | 7 |
Dataset | CMIM | CMIM3 | JMI | JMI3 | JMIM | MIGM | QPFS | Relief | WRFS | FJMIM |
Acute Inflammations | 100±0[=] | 100±0[=] | 100±0[=] | 100±0[=] | 100±0[=] | 99.75±2.5[=] | 99.75±2.5[=] | 100±0[=] | 90.83±8.5[+] | 100±0 |
Arrhythmia | 60.64±7.01[=] | 59.22±6.17[=] | 59.42±6.49[=] | 59.58±6.58[=] | 60.62±6.93[=] | 58.98±6.39[=] | 63.7±6.57[-] | 64.94±6.33[-] | 59.31±6.3[=] | 59.74±6.43 |
Blogger | 61.9±10.7[=] | 61.9±10.7[=] | 61.9±10.7[=] | 64.9±10.2[=] | 61.9±10.7[=] | 61.9±10.7[=] | 61.9±10.7[=] | 65.4±7.84[=] | 66.1±9.09[=] | 65.9±10.26 |
DRD | 57.59±4.95[=] | 60.66±4.69[-] | 60.3±5.04[-] | 60.6±4.58[-] | 57.59±4.95[=] | 60.3±5.04[-] | 61.19±4.49[-] | 60.61±5.06[-] | 57.47±4.98[=] | 57.56±4.83 |
Hayes-Roth | 45.81±10.77[=] | 45.81±10.77[=] | 45.81±10.77[=] | 45.81±10.77[=] | 45.81±10.77[=] | 45.81±10.77[=] | 49.37±10.53[=] | 46.31±11.48[=] | 45.81±10.77[=] | 49.37±10.53 |
ILPD | 68.99±5.68[=] | 67.33±5.87[=] | 68.99±5.68[=] | 67.33±5.87[=] | 68.99±5.68[=] | 68.99±5.68[=] | 68.73±5.64[=] | 64.96±6.45[+] | 70.11±6.74[=] | 69.54±5.75 |
Lenses | 86.83±21.88[=] | 86.83±21.88[=] | 86.83±21.88[=] | 86.83±21.88[=] | 86.83±21.88[=] | 86.83±21.88[=] | 86.83±21.88[=] | 54.83±30.73[+] | 86.83±21.88[=] | 86.83±21.88 |
Lymphography | 79.02±10.57[=] | 82.05±9.59[=] | 79.02±10.57[=] | 77.93±10.87[=] | 78.27±10.98[=] | 77.25±11.38[=] | 79.02±10.57[=] | 82.61±9.77[=] | 76.91±11.64[+] | 81.5±10.29 |
CVR | 94.3±3.15[=] | 94.32±3.12[=] | 93.75±3.57[=] | 93.75±3.57[=] | 93.75±3.57[=] | 93.75±3.57[=] | 94.32±3.26[=] | 93.82±3.28[=] | 93.52±3.18[=] | 93.75±3.57 |
Sonar | 75.05±8.33[=] | 75.42±9.08[=] | 75.75±8.85[=] | 76.23±9.99[=] | 72.2±9.93[=] | 72.14±9.54[+] | 76.42±8.69[=] | 74.25±8.33[=] | 77.91±8.74[=] | 77±8.98 |
Thoracic Surgery | 83.83±3.1[=] | 83.38±3.09[=] | 83.38±3.09[=] | 83.85±2.24[=] | 83.38±3.09[=] | 84.64±1.83[=] | 83.55±3.08[=] | 83.06±2.77[=] | 83.85±2.74[=] | 83.38±3.09 |
Wilt | 94.61±0.06[=] | 94.61±0.06[=] | 94.61±0.06[=] | 94.61±0.06[=] | 94.61±0.06[=] | 94.61±0.06[=] | 94.61±0.06[=] | 94.61±0.06[=] | 94.61±0.06[=] | 94.61±0.06 |
Zoo | 95.15±5.74[=] | 96.05±5.6[=] | 96.03±5.66[=] | 96.03±5.66[=] | 96.03±5.66[=] | 96.93±5.42[=] | 94.08±6.6[=] | 94.08±6.6[=] | 92.96±7.05[=] | 95.05±5.87 |
Average | 77.21 | 77.51 | 77.37 | 77.50 | 76.92 | 77.07 | 77.96 | 75.34 | 76.63 | 78.02 |
Dataset | CMIM | CMIM3 | JMI | JMI3 | JMIM | MIGM | QPFS | Relief | WRFS | FJMIM |
Acute Inflammations | 98.33±3.35[=] | 99.67±1.64[=] | 98.33±3.35[=] | 98.33±3.35[=] | 98.33±3.35[=] | 99.42±2.44[=] | 99.42±2.44[=] | 100±0[+] | 89.92±9.5[+] | 99.42±2.44 |
Arrhythmia | 66.09±6.81[=] | 64.08±6.11[=] | 65.01±5.96[=] | 65.7±6.23[=] | 66.14±6.61[=] | 65.23±6.39[=] | 66.82±6.2[=] | 67.22±6.05[=] | 65.23±6.15[=] | 67.11±6.7 |
Blogger | 68±4.02[=] | 67.7±4.89[=] | 68±4.02[=] | 67.4±5.62[=] | 68±4.02[=] | 68±4.02[=] | 67.7±4.89[=] | 67.7±4.89[=] | 68±4.02[=] | 67.7±4.89 |
DRD | 68.03±3.92[=] | 67.55±4.07[=] | 67.15±3.85[=] | 67.03±3.95[=] | 68.03±3.92[=] | 67.19±3.8[=] | 65.1±4.33[+] | 61.91±4.51[+] | 67.7±4[=] | 67.96±4.23 |
Hayes-Roth | 49.94±12.83[=] | 49.94±12.83[=] | 49.94±12.83[=] | 49.94±12.83[=] | 49.94±12.83[=] | 49.94±12.83[=] | 52±11.61[=] | 48.75±13.21[=] | 49.94±12.83[=] | 52±11.61 |
ILPD | 71.36±0.73[=] | 71.36±0.73[=] | 71.36±0.73[=] | 71.36±0.73[=] | 71.36±0.73[=] | 71.36±0.73[=] | 71.36±0.73[=] | 71.36±0.73[=] | 71.36±0.73[=] | 71.36±0.73 |
Lenses | 76.17±22.13[=] | 76.17±22.13[=] | 76.17±22.13[=] | 76.17±22.13[=] | 76.17±22.13[=] | 76.17±22.13[=] | 76.17±22.13[=] | 54.5±30.42[+] | 76.17±22.13[=] | 76.17±22.13 |
Lymphography | 81.57±10.06[=] | 80.56±9.09[=] | 81.42±10.15[=] | 84.55±8.84[=] | 82.02±10.48[=] | 79.06±10.44[+] | 81.57±10.11[=] | 80.3±9[=] | 74.25±11.45[+] | 85.5±8.3 |
CVR | 94.09±3.33[=] | 94.46±3.37[=] | 94.3±3.34[=] | 94.32±3.32[=] | 94.3±3.34[=] | 94.3±3.34[=] | 94±3.44[=] | 94.43±3.41[=] | 94.37±3.41[=] | 94.3±3.34 |
Sonar | 79.34±8.37[=] | 80.48±8.05[=] | 80.18±8.33[=] | 78.46±8.44[=] | 80.54±8.43[=] | 76.93±8.71[=] | 79.91±7.73[=] | 82.1±8.07[=] | 77.41±8.41[=] | 80.67±7.88 |
Thoracic Surgery | 85.11±0[=] | 85.11±0[=] | 85.11±0[=] | 85.11±0[=] | 85.11±0[=] | 85.11±0[=] | 85.11±0[=] | 85.11±0[=] | 85.11±0[=] | 85.11±0 |
Wilt | 97.62±0.57[+] | 97.97±0.49[=] | 97.97±0.49[=] | 97.97±0.49[=] | 97.62±0.57[+] | 97.97±0.49[=] | 94.61±0.06[+] | 97.97±0.49[=] | 97.62±0.57[+] | 97.98±0.49 |
Zoo | 95.75±5.12[=] | 93.88±6.72[=] | 89.39±5.72[+] | 89.49±5.64[+] | 89.49±5.64[+] | 94.06±6.9[=] | 95.27±5.85[=] | 93.89±6.96[=] | 90.49±7.96[+] | 95.06±6.2 |
Average | 79.34 | 79.15 | 78.79 | 78.91 | 79.00 | 78.83 | 79.16 | 77.33 | 77.51 | 80.03 |
Dataset | CMIM | CMIM3 | JMI | JMI3 | JMIM | MIGM | QPFS | Relief | WRFS | FJMIM |
Acute Inflammations | 99.5±1.99[=] | 100±0[=] | 99.5±1.99[=] | 99.5±1.99[=] | 99.5±1.99[=] | 100±0[=] | 100±0[=] | 100±0[=] | 99.67±1.64[=] | 100±0 |
Arrhythmia | 58.27±5.17[+] | 57.63±5.17[+] | 57.79±5.34[+] | 58.32±5.08[+] | 58.94±5.13[=] | 57.67±5.21[+] | 60.73±4.64[=] | 65.13±4.43[-] | 58.39±5.22[+] | 61.11±5.23 |
Blogger | 72.1±11.31[=] | 72.1±11.31[=] | 72.1±11.31[=] | 78.8±10.18[=] | 72.1±11.31[=] | 72.1±11.31[=] | 72.1±11.31[=] | 70.3±11.41[=] | 76.1±12.05[=] | 78.6±11.37 |
DRD | 64.3±4.19[=] | 62.62±5.29[=] | 63.69±4.58[=] | 61.96±4.68[=] | 64.3±4.19[=] | 63.58±4.56[=] | 60.47±4.64[+] | 63.51±4.63[=] | 64.06±4.4[=] | 64.7±4.23 |
Hayes-Roth | 59.19±10.41[=] | 59.19±10.41[=] | 59.19±10.41[=] | 59.19±10.41[=] | 59.19±10.41[=] | 59.19±10.41[=] | 63.12±11.32[=] | 57.56±10.82[=] | 59.19±10.41[=] | 63.12±11.32 |
ILPD | 67.16±5.41[=] | 68.22±5.06[=] | 67.54±5.44[=] | 68.22±5.06[=] | 67.16±5.41[=] | 67.3±5.42[=] | 68.74±5.01[=] | 67.84±5.56[=] | 66.66±5.25[=] | 69.15±5.62 |
Lenses | 86.83±21.88[=] | 86.83±21.88[=] | 86.83±21.88[=] | 86.83±21.88[=] | 86.83±21.88[=] | 86.83±21.88[=] | 86.83±21.88[=] | 54.17±30.46[+] | 86.83±21.88[=] | 86.83±21.88 |
Lymphography | 84.61±7.58[=] | 72.61±9.14[+] | 84.61±7.58[=] | 84.16±9.46[=] | 80.99±9.49[=] | 76.62±8.87[=] | 84.61±7.58[=] | 81.44±9.42[=] | 79.74±9.36[=] | 78.87±8.35 |
CVR | 93.63±3.43[=] | 94.09±3.33[=] | 95.26±3.01[=] | 95.26±3.01[=] | 95.26±3.01[=] | 95.26±3.01[=] | 94.14±3.37[=] | 93.67±3.5[=] | 94.09±2.95[=] | 95.26±3.01 |
Sonar | 85.09±7.43[=] | 83.51±7.68[=] | 87.74±7.51[=] | 85.53±8.83[=] | 82.7±9.06[=] | 82.4±7.72[+] | 86.15±7.24[=] | 87.95±6.78[=] | 84.17±7.73[=] | 87.65±7.27 |
Thoracic Surgery | 80.96±3.45[=] | 81.38±3.53[=] | 81.38±3.53[=] | 83.45±3.05[=] | 81.38±3.53[=] | 82.64±3.28[=] | 83.89±2.95[=] | 82.68±3.08[=] | 84.85±0.97[-] | 81.38±3.53 |
Wilt | 97.69±0.57[+] | 98.12±0.55[=] | 98.12±0.55[=] | 98.12±0.55[=] | 97.69±0.57[+] | 98.12±0.55[=] | 94.44±0.52[+] | 98.12±0.55[=] | 97.69±0.57[+] | 98.12±0.55 |
Zoo | 91.9±7.21[=] | 92.06±7.35[=] | 92.05±7.24[=] | 92.05±7.24[=] | 92.05±7.24[=] | 91.16±7.48[=] | 91.42±6.94[=] | 91.42±6.94[=] | 90.29±7.45[+] | 94.08±6.6 |
Average | 80.09 | 79.10 | 80.45 | 80.88 | 79.85 | 79.45 | 80.51 | 77.98 | 80.13 | 81.45 |
FS method | Average |
CMIM | 78.9 |
CMIM3 | 78.6 |
JMI | 78.9 |
JMI3 | 79.1 |
JMIM | 78.6 |
MIGM | 78.4 |
QPFS | 79.2 |
Relief | 76.9 |
WRFS | 78.1 |
FJMIM | 79.8 |
FS method | NB | SVM | KNN | Average |
CMIM | 0.702 | 0.641 | 0.704 | 0.682 |
CMIM3 | 0.742 | 0.641 | 0.705 | 0.696 |
JMI | 0.705 | 0.640 | 0.713 | 0.686 |
JMI3 | 0.712 | 0.636 | 0.723 | 0.690 |
JMIM | 0.701 | 0.642 | 0.707 | 0.683 |
MIGM | 0.701 | 0.637 | 0.708 | 0.682 |
QPFS | 0.712 | 0.636 | 0.711 | 0.686 |
Relief | 0.621 | 0.612 | 0.661 | 0.631 |
WRFS | 0.698 | 0.632 | 0.708 | 0.679 |
FJMIM | 0.748 | 0.645 | 0.743 | 0.712 |
FS method | NB | SVM | KNN | Average |
CMIM | 0.722 | 0.712 | 0.818 | 0.750 |
CMIM3 | 0.795 | 0.712 | 0.752 | 0.753 |
JMI | 0.724 | 0.712 | 0.823 | 0.753 |
JMI3 | 0.729 | 0.712 | 0.834 | 0.759 |
JMIM | 0.719 | 0.714 | 0.821 | 0.751 |
MIGM | 0.722 | 0.710 | 0.818 | 0.750 |
QPFS | 0.732 | 0.712 | 0.825 | 0.756 |
Relief | 0.632 | 0.708 | 0.688 | 0.676 |
WRFS | 0.716 | 0.701 | 0.826 | 0.748 |
FJMIM | 0.798 | 0.715 | 0.840 | 0.784 |
FS method | NB | SVM | KNN | Average |
CMIM | 0.835 | 0.733 | 0.812 | 0.793 |
CMIM3 | 0.836 | 0.748 | 0.844 | 0.809 |
JMI | 0.837 | 0.734 | 0.815 | 0.795 |
JMI3 | 0.831 | 0.729 | 0.805 | 0.788 |
JMIM | 0.836 | 0.742 | 0.812 | 0.796 |
MIGM | 0.832 | 0.754 | 0.807 | 0.798 |
QPFS | 0.838 | 0.710 | 0.807 | 0.785 |
Relief | 0.804 | 0.707 | 0.801 | 0.771 |
WRFS | 0.831 | 0.732 | 0.806 | 0.789 |
FJMIM | 0.839 | 0.748 | 0.852 | 0.813 |
FS method | NB | SVM | KNN | Average |
CMIM | 0.848 | 0.708 | 0.798 | 0.785 |
CMIM3 | 0.862 | 0.715 | 0.862 | 0.813 |
JMI | 0.850 | 0.708 | 0.797 | 0.785 |
JMI3 | 0.837 | 0.705 | 0.800 | 0.781 |
JMIM | 0.852 | 0.713 | 0.797 | 0.787 |
MIGM | 0.857 | 0.724 | 0.827 | 0.803 |
QPFS | 0.857 | 0.708 | 0.797 | 0.787 |
Relief | 0.842 | 0.685 | 0.791 | 0.772 |
WRFS | 0.850 | 0.697 | 0.795 | 0.781 |
FJMIM | 0.862 | 0.718 | 0.869 | 0.816 |
FS method | Stability |
CMIM | 72.6 |
CMIM3 | 67.3 |
JMI | 81.2 |
JMI3 | 75.2 |
JMIM | 75.9 |
MIGM | 66.2 |
QPFS | 71.7 |
Relief | 44.3 |
WRFS | 66.6 |
FJMIM | 87.8 |
No. | Datasets | Instances | Features | Classes |
1 | Acute Inflammations | 120 | 6 | 2 |
2 | Arrhythmia | 452 | 279 | 13 |
3 | Blogger | 100 | 6 | 2 |
4 | Diabetic Retinopathy Debrecen (DRD) | 1151 | 20 | 2 |
5 | Hayes-Roth | 160 | 5 | 3 |
6 | Indian Liver Patient Dataset (ILPD) | 583 | 10 | 2 |
7 | Lenses | 24 | 4 | 3 |
8 | Lymphography | 148 | 18 | 4 |
9 | Congressional Voting Records (CVR) | 435 | 16 | 2 |
10 | Sonar | 208 | 60 | 2 |
11 | Thoracic Surgery | 470 | 17 | 2 |
12 | Wilt | 4889 | 6 | 2 |
13 | Zoo | 101 | 17 | 7 |
Dataset | CMIM | CMIM3 | JMI | JMI3 | JMIM | MIGM | QPFS | Relief | WRFS | FJMIM |
Acute Inflammations | 100±0[=] | 100±0[=] | 100±0[=] | 100±0[=] | 100±0[=] | 99.75±2.5[=] | 99.75±2.5[=] | 100±0[=] | 90.83±8.5[+] | 100±0 |
Arrhythmia | 60.64±7.01[=] | 59.22±6.17[=] | 59.42±6.49[=] | 59.58±6.58[=] | 60.62±6.93[=] | 58.98±6.39[=] | 63.7±6.57[-] | 64.94±6.33[-] | 59.31±6.3[=] | 59.74±6.43 |
Blogger | 61.9±10.7[=] | 61.9±10.7[=] | 61.9±10.7[=] | 64.9±10.2[=] | 61.9±10.7[=] | 61.9±10.7[=] | 61.9±10.7[=] | 65.4±7.84[=] | 66.1±9.09[=] | 65.9±10.26 |
DRD | 57.59±4.95[=] | 60.66±4.69[-] | 60.3±5.04[-] | 60.6±4.58[-] | 57.59±4.95[=] | 60.3±5.04[-] | 61.19±4.49[-] | 60.61±5.06[-] | 57.47±4.98[=] | 57.56±4.83 |
Hayes-Roth | 45.81±10.77[=] | 45.81±10.77[=] | 45.81±10.77[=] | 45.81±10.77[=] | 45.81±10.77[=] | 45.81±10.77[=] | 49.37±10.53[=] | 46.31±11.48[=] | 45.81±10.77[=] | 49.37±10.53 |
ILPD | 68.99±5.68[=] | 67.33±5.87[=] | 68.99±5.68[=] | 67.33±5.87[=] | 68.99±5.68[=] | 68.99±5.68[=] | 68.73±5.64[=] | 64.96±6.45[+] | 70.11±6.74[=] | 69.54±5.75 |
Lenses | 86.83±21.88[=] | 86.83±21.88[=] | 86.83±21.88[=] | 86.83±21.88[=] | 86.83±21.88[=] | 86.83±21.88[=] | 86.83±21.88[=] | 54.83±30.73[+] | 86.83±21.88[=] | 86.83±21.88 |
Lymphography | 79.02±10.57[=] | 82.05±9.59[=] | 79.02±10.57[=] | 77.93±10.87[=] | 78.27±10.98[=] | 77.25±11.38[=] | 79.02±10.57[=] | 82.61±9.77[=] | 76.91±11.64[+] | 81.5±10.29 |
CVR | 94.3±3.15[=] | 94.32±3.12[=] | 93.75±3.57[=] | 93.75±3.57[=] | 93.75±3.57[=] | 93.75±3.57[=] | 94.32±3.26[=] | 93.82±3.28[=] | 93.52±3.18[=] | 93.75±3.57 |
Sonar | 75.05±8.33[=] | 75.42±9.08[=] | 75.75±8.85[=] | 76.23±9.99[=] | 72.2±9.93[=] | 72.14±9.54[+] | 76.42±8.69[=] | 74.25±8.33[=] | 77.91±8.74[=] | 77±8.98 |
Thoracic Surgery | 83.83±3.1[=] | 83.38±3.09[=] | 83.38±3.09[=] | 83.85±2.24[=] | 83.38±3.09[=] | 84.64±1.83[=] | 83.55±3.08[=] | 83.06±2.77[=] | 83.85±2.74[=] | 83.38±3.09 |
Wilt | 94.61±0.06[=] | 94.61±0.06[=] | 94.61±0.06[=] | 94.61±0.06[=] | 94.61±0.06[=] | 94.61±0.06[=] | 94.61±0.06[=] | 94.61±0.06[=] | 94.61±0.06[=] | 94.61±0.06 |
Zoo | 95.15±5.74[=] | 96.05±5.6[=] | 96.03±5.66[=] | 96.03±5.66[=] | 96.03±5.66[=] | 96.93±5.42[=] | 94.08±6.6[=] | 94.08±6.6[=] | 92.96±7.05[=] | 95.05±5.87 |
Average | 77.21 | 77.51 | 77.37 | 77.50 | 76.92 | 77.07 | 77.96 | 75.34 | 76.63 | 78.02 |
Dataset | CMIM | CMIM3 | JMI | JMI3 | JMIM | MIGM | QPFS | Relief | WRFS | FJMIM |
Acute Inflammations | 98.33±3.35[=] | 99.67±1.64[=] | 98.33±3.35[=] | 98.33±3.35[=] | 98.33±3.35[=] | 99.42±2.44[=] | 99.42±2.44[=] | 100±0[+] | 89.92±9.5[+] | 99.42±2.44 |
Arrhythmia | 66.09±6.81[=] | 64.08±6.11[=] | 65.01±5.96[=] | 65.7±6.23[=] | 66.14±6.61[=] | 65.23±6.39[=] | 66.82±6.2[=] | 67.22±6.05[=] | 65.23±6.15[=] | 67.11±6.7 |
Blogger | 68±4.02[=] | 67.7±4.89[=] | 68±4.02[=] | 67.4±5.62[=] | 68±4.02[=] | 68±4.02[=] | 67.7±4.89[=] | 67.7±4.89[=] | 68±4.02[=] | 67.7±4.89 |
DRD | 68.03±3.92[=] | 67.55±4.07[=] | 67.15±3.85[=] | 67.03±3.95[=] | 68.03±3.92[=] | 67.19±3.8[=] | 65.1±4.33[+] | 61.91±4.51[+] | 67.7±4[=] | 67.96±4.23 |
Hayes-Roth | 49.94±12.83[=] | 49.94±12.83[=] | 49.94±12.83[=] | 49.94±12.83[=] | 49.94±12.83[=] | 49.94±12.83[=] | 52±11.61[=] | 48.75±13.21[=] | 49.94±12.83[=] | 52±11.61 |
ILPD | 71.36±0.73[=] | 71.36±0.73[=] | 71.36±0.73[=] | 71.36±0.73[=] | 71.36±0.73[=] | 71.36±0.73[=] | 71.36±0.73[=] | 71.36±0.73[=] | 71.36±0.73[=] | 71.36±0.73 |
Lenses | 76.17±22.13[=] | 76.17±22.13[=] | 76.17±22.13[=] | 76.17±22.13[=] | 76.17±22.13[=] | 76.17±22.13[=] | 76.17±22.13[=] | 54.5±30.42[+] | 76.17±22.13[=] | 76.17±22.13 |
Lymphography | 81.57±10.06[=] | 80.56±9.09[=] | 81.42±10.15[=] | 84.55±8.84[=] | 82.02±10.48[=] | 79.06±10.44[+] | 81.57±10.11[=] | 80.3±9[=] | 74.25±11.45[+] | 85.5±8.3 |
CVR | 94.09±3.33[=] | 94.46±3.37[=] | 94.3±3.34[=] | 94.32±3.32[=] | 94.3±3.34[=] | 94.3±3.34[=] | 94±3.44[=] | 94.43±3.41[=] | 94.37±3.41[=] | 94.3±3.34 |
Sonar | 79.34±8.37[=] | 80.48±8.05[=] | 80.18±8.33[=] | 78.46±8.44[=] | 80.54±8.43[=] | 76.93±8.71[=] | 79.91±7.73[=] | 82.1±8.07[=] | 77.41±8.41[=] | 80.67±7.88 |
Thoracic Surgery | 85.11±0[=] | 85.11±0[=] | 85.11±0[=] | 85.11±0[=] | 85.11±0[=] | 85.11±0[=] | 85.11±0[=] | 85.11±0[=] | 85.11±0[=] | 85.11±0 |
Wilt | 97.62±0.57[+] | 97.97±0.49[=] | 97.97±0.49[=] | 97.97±0.49[=] | 97.62±0.57[+] | 97.97±0.49[=] | 94.61±0.06[+] | 97.97±0.49[=] | 97.62±0.57[+] | 97.98±0.49 |
Zoo | 95.75±5.12[=] | 93.88±6.72[=] | 89.39±5.72[+] | 89.49±5.64[+] | 89.49±5.64[+] | 94.06±6.9[=] | 95.27±5.85[=] | 93.89±6.96[=] | 90.49±7.96[+] | 95.06±6.2 |
Average | 79.34 | 79.15 | 78.79 | 78.91 | 79.00 | 78.83 | 79.16 | 77.33 | 77.51 | 80.03 |
Dataset | CMIM | CMIM3 | JMI | JMI3 | JMIM | MIGM | QPFS | Relief | WRFS | FJMIM |
Acute Inflammations | 99.5±1.99[=] | 100±0[=] | 99.5±1.99[=] | 99.5±1.99[=] | 99.5±1.99[=] | 100±0[=] | 100±0[=] | 100±0[=] | 99.67±1.64[=] | 100±0 |
Arrhythmia | 58.27±5.17[+] | 57.63±5.17[+] | 57.79±5.34[+] | 58.32±5.08[+] | 58.94±5.13[=] | 57.67±5.21[+] | 60.73±4.64[=] | 65.13±4.43[-] | 58.39±5.22[+] | 61.11±5.23 |
Blogger | 72.1±11.31[=] | 72.1±11.31[=] | 72.1±11.31[=] | 78.8±10.18[=] | 72.1±11.31[=] | 72.1±11.31[=] | 72.1±11.31[=] | 70.3±11.41[=] | 76.1±12.05[=] | 78.6±11.37 |
DRD | 64.3±4.19[=] | 62.62±5.29[=] | 63.69±4.58[=] | 61.96±4.68[=] | 64.3±4.19[=] | 63.58±4.56[=] | 60.47±4.64[+] | 63.51±4.63[=] | 64.06±4.4[=] | 64.7±4.23 |
Hayes-Roth | 59.19±10.41[=] | 59.19±10.41[=] | 59.19±10.41[=] | 59.19±10.41[=] | 59.19±10.41[=] | 59.19±10.41[=] | 63.12±11.32[=] | 57.56±10.82[=] | 59.19±10.41[=] | 63.12±11.32 |
ILPD | 67.16±5.41[=] | 68.22±5.06[=] | 67.54±5.44[=] | 68.22±5.06[=] | 67.16±5.41[=] | 67.3±5.42[=] | 68.74±5.01[=] | 67.84±5.56[=] | 66.66±5.25[=] | 69.15±5.62 |
Lenses | 86.83±21.88[=] | 86.83±21.88[=] | 86.83±21.88[=] | 86.83±21.88[=] | 86.83±21.88[=] | 86.83±21.88[=] | 86.83±21.88[=] | 54.17±30.46[+] | 86.83±21.88[=] | 86.83±21.88 |
Lymphography | 84.61±7.58[=] | 72.61±9.14[+] | 84.61±7.58[=] | 84.16±9.46[=] | 80.99±9.49[=] | 76.62±8.87[=] | 84.61±7.58[=] | 81.44±9.42[=] | 79.74±9.36[=] | 78.87±8.35 |
CVR | 93.63±3.43[=] | 94.09±3.33[=] | 95.26±3.01[=] | 95.26±3.01[=] | 95.26±3.01[=] | 95.26±3.01[=] | 94.14±3.37[=] | 93.67±3.5[=] | 94.09±2.95[=] | 95.26±3.01 |
Sonar | 85.09±7.43[=] | 83.51±7.68[=] | 87.74±7.51[=] | 85.53±8.83[=] | 82.7±9.06[=] | 82.4±7.72[+] | 86.15±7.24[=] | 87.95±6.78[=] | 84.17±7.73[=] | 87.65±7.27 |
Thoracic Surgery | 80.96±3.45[=] | 81.38±3.53[=] | 81.38±3.53[=] | 83.45±3.05[=] | 81.38±3.53[=] | 82.64±3.28[=] | 83.89±2.95[=] | 82.68±3.08[=] | 84.85±0.97[-] | 81.38±3.53 |
Wilt | 97.69±0.57[+] | 98.12±0.55[=] | 98.12±0.55[=] | 98.12±0.55[=] | 97.69±0.57[+] | 98.12±0.55[=] | 94.44±0.52[+] | 98.12±0.55[=] | 97.69±0.57[+] | 98.12±0.55 |
Zoo | 91.9±7.21[=] | 92.06±7.35[=] | 92.05±7.24[=] | 92.05±7.24[=] | 92.05±7.24[=] | 91.16±7.48[=] | 91.42±6.94[=] | 91.42±6.94[=] | 90.29±7.45[+] | 94.08±6.6 |
Average | 80.09 | 79.10 | 80.45 | 80.88 | 79.85 | 79.45 | 80.51 | 77.98 | 80.13 | 81.45 |
FS method | Average |
CMIM | 78.9 |
CMIM3 | 78.6 |
JMI | 78.9 |
JMI3 | 79.1 |
JMIM | 78.6 |
MIGM | 78.4 |
QPFS | 79.2 |
Relief | 76.9 |
WRFS | 78.1 |
FJMIM | 79.8 |
FS method | NB | SVM | KNN | Average |
CMIM | 0.702 | 0.641 | 0.704 | 0.682 |
CMIM3 | 0.742 | 0.641 | 0.705 | 0.696 |
JMI | 0.705 | 0.640 | 0.713 | 0.686 |
JMI3 | 0.712 | 0.636 | 0.723 | 0.690 |
JMIM | 0.701 | 0.642 | 0.707 | 0.683 |
MIGM | 0.701 | 0.637 | 0.708 | 0.682 |
QPFS | 0.712 | 0.636 | 0.711 | 0.686 |
Relief | 0.621 | 0.612 | 0.661 | 0.631 |
WRFS | 0.698 | 0.632 | 0.708 | 0.679 |
FJMIM | 0.748 | 0.645 | 0.743 | 0.712 |
FS method | NB | SVM | KNN | Average |
CMIM | 0.722 | 0.712 | 0.818 | 0.750 |
CMIM3 | 0.795 | 0.712 | 0.752 | 0.753 |
JMI | 0.724 | 0.712 | 0.823 | 0.753 |
JMI3 | 0.729 | 0.712 | 0.834 | 0.759 |
JMIM | 0.719 | 0.714 | 0.821 | 0.751 |
MIGM | 0.722 | 0.710 | 0.818 | 0.750 |
QPFS | 0.732 | 0.712 | 0.825 | 0.756 |
Relief | 0.632 | 0.708 | 0.688 | 0.676 |
WRFS | 0.716 | 0.701 | 0.826 | 0.748 |
FJMIM | 0.798 | 0.715 | 0.840 | 0.784 |
FS method | NB | SVM | KNN | Average |
CMIM | 0.835 | 0.733 | 0.812 | 0.793 |
CMIM3 | 0.836 | 0.748 | 0.844 | 0.809 |
JMI | 0.837 | 0.734 | 0.815 | 0.795 |
JMI3 | 0.831 | 0.729 | 0.805 | 0.788 |
JMIM | 0.836 | 0.742 | 0.812 | 0.796 |
MIGM | 0.832 | 0.754 | 0.807 | 0.798 |
QPFS | 0.838 | 0.710 | 0.807 | 0.785 |
Relief | 0.804 | 0.707 | 0.801 | 0.771 |
WRFS | 0.831 | 0.732 | 0.806 | 0.789 |
FJMIM | 0.839 | 0.748 | 0.852 | 0.813 |
FS method | NB | SVM | KNN | Average |
CMIM | 0.848 | 0.708 | 0.798 | 0.785 |
CMIM3 | 0.862 | 0.715 | 0.862 | 0.813 |
JMI | 0.850 | 0.708 | 0.797 | 0.785 |
JMI3 | 0.837 | 0.705 | 0.800 | 0.781 |
JMIM | 0.852 | 0.713 | 0.797 | 0.787 |
MIGM | 0.857 | 0.724 | 0.827 | 0.803 |
QPFS | 0.857 | 0.708 | 0.797 | 0.787 |
Relief | 0.842 | 0.685 | 0.791 | 0.772 |
WRFS | 0.850 | 0.697 | 0.795 | 0.781 |
FJMIM | 0.862 | 0.718 | 0.869 | 0.816 |
FS method | Stability |
CMIM | 72.6 |
CMIM3 | 67.3 |
JMI | 81.2 |
JMI3 | 75.2 |
JMIM | 75.9 |
MIGM | 66.2 |
QPFS | 71.7 |
Relief | 44.3 |
WRFS | 66.6 |
FJMIM | 87.8 |