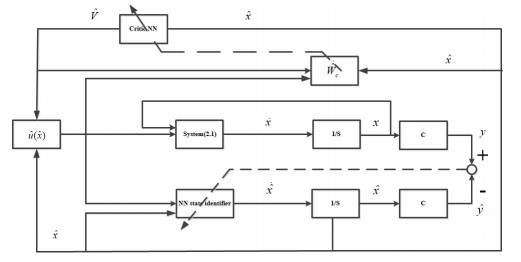
Citation: Michael J. Hamilton, Matthew D. Young, Silvia Sauer, Ernest Martinez. The interplay of long non-coding RNAs and MYC in cancer[J]. AIMS Biophysics, 2015, 2(4): 794-809. doi: 10.3934/biophy.2015.4.794
[1] | Yuhang Yao, Jiaxin Yuan, Tao Chen, Xiaole Yang, Hui Yang . Distributed convex optimization of bipartite containment control for high-order nonlinear uncertain multi-agent systems with state constraints. Mathematical Biosciences and Engineering, 2023, 20(9): 17296-17323. doi: 10.3934/mbe.2023770 |
[2] | Zichen Wang, Xin Wang . Fault-tolerant control for nonlinear systems with a dead zone: Reinforcement learning approach. Mathematical Biosciences and Engineering, 2023, 20(4): 6334-6357. doi: 10.3934/mbe.2023274 |
[3] | Vladimir Djordjevic, Hongfeng Tao, Xiaona Song, Shuping He, Weinan Gao, Vladimir Stojanovic . Data-driven control of hydraulic servo actuator: An event-triggered adaptive dynamic programming approach. Mathematical Biosciences and Engineering, 2023, 20(5): 8561-8582. doi: 10.3934/mbe.2023376 |
[4] | Dongxiang Gao, Yujun Zhang, Libing Wu, Sihan Liu . Fixed-time command filtered output feedback control for twin-roll inclined casting system with prescribed performance. Mathematical Biosciences and Engineering, 2024, 21(2): 2282-2301. doi: 10.3934/mbe.2024100 |
[5] | Na Zhang, Jianwei Xia, Tianjiao Liu, Chengyuan Yan, Xiao Wang . Dynamic event-triggered adaptive finite-time consensus control for multi-agent systems with time-varying actuator faults. Mathematical Biosciences and Engineering, 2023, 20(5): 7761-7783. doi: 10.3934/mbe.2023335 |
[6] | Yuhan Su, Shaoping Shen . Adaptive predefined-time prescribed performance control for spacecraft systems. Mathematical Biosciences and Engineering, 2023, 20(3): 5921-5948. doi: 10.3934/mbe.2023256 |
[7] | K. Renee Fister, Jennifer Hughes Donnelly . Immunotherapy: An Optimal Control Theory Approach. Mathematical Biosciences and Engineering, 2005, 2(3): 499-510. doi: 10.3934/mbe.2005.2.499 |
[8] | Tianqi Yu, Lei Liu, Yan-Jun Liu . Observer-based adaptive fuzzy output feedback control for functional constraint systems with dead-zone input. Mathematical Biosciences and Engineering, 2023, 20(2): 2628-2650. doi: 10.3934/mbe.2023123 |
[9] | Siyu Li, Shu Li, Lei Liu . Fuzzy adaptive event-triggered distributed control for a class of nonlinear multi-agent systems. Mathematical Biosciences and Engineering, 2024, 21(1): 474-493. doi: 10.3934/mbe.2024021 |
[10] | Yong Xiong, Lin Pan, Min Xiao, Han Xiao . Motion control and path optimization of intelligent AUV using fuzzy adaptive PID and improved genetic algorithm. Mathematical Biosciences and Engineering, 2023, 20(5): 9208-9245. doi: 10.3934/mbe.2023404 |
The research on optimal control for nonlinear systems plays a significant role in industry and military fields. Due to the influence of the environment and the limitations of the engineering system, it is very tough to maximize or minimize the performance index of the controlled system in practical. Therefore, optimization problem is a difficult problem in current control field, and has gradually been the focus of attention. The optimal control problems of nonlinear systems are finally transformed into the solutions of Hamilton-Jacobi-Bellman (HJB) partial differential equations.
However, because the HJB equation is a nonlinear partial differential equation, it is difficult to obtain an analytical solution. Therefore, how to obtain the analytical solution of HJB equation then realize the optimization performance index of the system, is the key points to resolve the optimization issue.
In order to avoid the problems encountered in solving HJB equation, Kalman [1] proposed the inverse-optimal-based control method for the first time. On the basis of [1], Freeman and Kokotovic [2] studied inverse optimization control of nonlinear systems. The basic idea of inverse optimization control is not to minimize the cost function by designing the controller, but to minimize the cost function by designing the appropriate control Lyapunov function(CLF). Therefore, the solution of HJB equation comes down to seeking the CLF of the controlled system, thus avoiding the shortcoming of directly solving HJB equation.
Besides, aiming at the above problems, Bellman [3] proposed the theory of dynamic programming (DP). However, the issue of "dimension disaster" would be caused in the process of DP control design, that is, the complexity of space storage and computation increases exponentially with the increase of the dimension of control vectors and states. Therefore, in order to overcome the phenomenon of "dimension disaster" in the process of optimal control design, an adaptive optimal control design method combining NNs is proposed by Werbos [4], which is called RL or adaptive/ approximate dynamic programming (ADP). In the control field, RL can effectively solve the "dimension disaster" problem in DP. In [5], Werbos retrospected the classic econometric approach and proposed a robust method. In [6], Werbos defined a more limited design called "brain-like intelligent control", it discusses the brain as a member of intelligent control, which implies a property to be sought in future research.
Since then, inspired by [4], large amounts of optimal control methods via APD have been developed, see [7,8]. Among them, for continuous-time (CT) systems, in offline situations, Abu-Khalaf and Lewis [9] presented an offline algorithm via RL to solve the optimal control issue of CT nonlinear systems. Since the offline control algorithms cannot be adopted to adjust online in real time, thus, to overcome this disadvantage, Vamvoudak and Lewis [10] proposed an online adaptive method via policy iteration. In [11], Li et al. investigated the Lyapunov stability problem for impulsive systems via event-triggered impulsive control. In [12], Li et al. considerd a class of nonlinear impulsive systems with delayed impulses, based on impulsive control theory and the ideas of average dwell-time (ADT), a set of Lyapunov-based sufficient conditions for globally exponential stability were obtained. However, we need to know the accurate knowledge of CT nonlinear systems in [9,10]. Since nonlinear systems usually contain uncertain nonlinear functions, it it difficult to acquire the analytical solution of the HJB equation.
In order to solve this problem, by choosing an appropriate cost function to reflect uncertainty regulation, the authors in [13] proposed a robust optimization controller design strategy based on an online strategy iterative algorithm for a class of continuous nonlinear systems with nonlinearities. Zhang et al. [14] designed a new data-driven robust identified optimization tracking controller via the acquired data-driven model for a kind of nonlinear CT systems.
Consider a class of affine nonlinear system as:
$ ˙x(t)=g(x(t))u(t)+f(x(t)),x(0)=x0 $
|
(2.1) |
where $ f(x) $ and $ g(x) $ are the uncertain smooth functions, which satisfy that $ f(0) = 0 $, $ g(0) = 0 $. $ u $ is the control input, $ x\in \textbf{R}^{n} $ is the state vector. For the above system, some adaptive optimization control strategies have been proposed.
In [15], for a class of affine nonlinear CT systems with unknown internal dynamics, Liu and Wang et al. developed an online method based on ADP, which constructed a critic neural network to facilitate the solution of the modified HJB equation. In [16], Liu et al. developed an online optimization control algorithm for CT affine nonlinear systems with infinite horizon cost. And in [17], Wen and Chen et al. studied an adaptive optimized tracking control method via RL algorithm and NNs.
For [16,17], value function is selected as:
$ V(z)=∫∞tr(z(r),u(z))dτ $
|
(2.2) |
where $ r(z, u) = z^{T}(t)Q(x)z(t) + u^{T}u $ is the value function, and $ Q(x) = q(x)q^{T}(x)\in \textbf{R}^{n\times n} $ is a positive definite matrix. The HJB equation is defined as:
$ H(z,u,Vz)=VTz(z)z(t)+r(z,u)=VTz(f(x)+g(x)u−yd(t))+zTQ(x)z(t)+uTu $
|
(2.3) |
where $ V_{z}\in \textbf{R}^{n} $ is the partial gradient of $ V_{z} $, $ y_{d}(t)\in \textbf{R}_{n} $ is the ideal tracking trajectory, $ z(t) = x(t)-y_{d}(t) $ is the tracking error. When considering the input constraint control and saturation constraints control issue, Liu and Yang developed a robust optimal adaptive optimal control method via RL for a kind of uncertain nonlinear systems. In [18,19], there exists a symmetric definite matrix $ Q $, and the value function is selected as:
$ V(x(t))=∫∞t[xTQx+ϖ(u)]ds,(s≥t) $
|
(2.4) |
where $ \varpi(u) $ is positive. For the sake of solving the constraint control issue, define $ \varpi(u) $ as:
$ ϖ(u)=2κ∫u0(ψ−1(υ/κ))TRdv=2κm∑i=1∫u0(ψ−1(υi/κ))TRidv $
|
(2.5) |
where $ R = diag[r_{1}, \cdots, r_{n}] $ with $ r_{i} > 0 $, $ (i = 1, \cdots, m) $, $ \psi(\cdot) $ is a bounded one-to-one function with $ |\psi(\cdot)|\leq1 $, $ \psi\in \textbf{R}^{m} $, $ \psi^{-1} = (\psi^{-1})^{T} $, $ \psi^{-1}(\upsilon/\kappa) = [\psi^{-1}(\upsilon_{1}/\kappa), \cdots, \psi^{-1}(\upsilon_{m}/\kappa)]^{T} $, $ u(x)\in \Xi $, $ \Xi = \{{u|u\in \textbf{R}^{m}, |u_{i}|\leq \kappa, i = 1, 2, \cdots, m}\} $, $ \kappa > 0 $ is a constant. Define the HJB equation and the value function as:
$ H(x,Vx,u)=VTx(f(x)+g(x)u)+r(x,u) $
|
(2.6) |
where $ V_{x}\in \textbf{R}^{n} $ is the partial derivative of $ V(x) $ with respect to $ x $.
In the previous article, since there exist the unknown nonlinear functions $ f(x) $ and $ g(x) $, the analytic solution of the equation cannot be received when resolving the HJB equation. Because of their properties and fault tolerance, attributes of nonlinearity, adaptivity, the identified solution of the HJB equation can be obtained symmetric via NNs.
Because the system (2.1) contains unknown dynamics, we can identify the system for receiving the optimal control. In [20], Yang et al. presented identifier-actor-critic (IAC) structure, where the actor NN is carried out control actions, and critic NN is employed to estimated these actions, and then returns the evaluations to actor, and the dynamics of uncertain system robust dynamic can be approximate by NN identifiers. From system (2.1), we have that:
$ ˙x=g(x)u+f(x)=g(x)u+Ax+ϝ(x) $
|
(2.7) |
where $ A\in \textbf {R}^{n\times n} $ is a certain constant matrix, $ \digamma(x) = f(x)-Ax $.
A NN is applied to identify $ \digamma(x) $ as follows:
$ ϝ(x)=WT1σ(x)+ε1(x) $
|
(2.8) |
where $ \varepsilon_{1}(x)\in \textbf {R}^{n} $ is the NN function reestablishment error, $ \sigma(x) $ is the activation function, $ W_{1}^{T}\in \textbf {R}^{n\times n} $ is the NN weight. By using (2.8), (2.7) can be developed by:
$ ˙x(t)=g(x)u+ε1(x)+Ax+WT1σ(x) $
|
(2.9) |
The NN identifier is designed as:
$ ˙ˆx(t)=g(ˆx)u+v(t)+Aˆx+ˆWT1σ(ˆx) $
|
(2.10) |
where $ \hat{x}\in \textbf{R}^{n} $ is the identifier NN state, $ \hat{W}_{1}\in \textbf{R}^{n\times n} $ is weight estimation, and $ v(t) $ is the robust feedback term.
The optimal value function can be expressed by NN as:
$ V∗(ˆx)=WTϕ(ˆx)+εv(ˆx) $
|
(2.11) |
The optimal control can be expressed by NN as:
$ u∗(ˆx)=−12R−1gT(ˆx)(ϕ′(ˆx)TW+ε′v(ˆx)T) $
|
(2.12) |
where $ \varepsilon_{v}(\cdot)\in R $ is the function reestablishment error, $ \phi(\hat{x}) = [\phi_{1}(\hat{x}), \phi_{2}(\hat{x}), \cdots, \phi_{N}(\hat{x})]^{T}\in \textbf{R}^{N} $, $ \phi'(\hat{x}) = \frac{\bigtriangleup \partial \phi(\hat{x})}{\partial \hat{x}} $ and $ W\in \textbf{R}^{N} $ are uncertain desired NN weights, $ N $ is the number of neurons.
The critic-actor $ \hat{V}(\hat{x}) $ and $ \hat{u} $, which can learn the optimization value function and adjust the optimization control online, is expressed as:
$ ˆV(ˆx)=ˆWTcϕ(ˆx) $
|
(2.13) |
$ ˆu(ˆx)=−12R−1gT(ˆx)ϕ′T(ˆx)^Wa $
|
(2.14) |
where $ \hat{W}_{c}(t)\in \textbf{R}^{N} $ and $ \hat{W}_{a}(t)\in \textbf{R}^{N} $ estimate the ideal weights of the critic-actor NNs. Whereas the system dynamics are estimated online by using the identification error $ \tilde{x}(t) = x(t)-\hat{x}(t) $. The overall planning diagram of the control algorithm is given in Figure 1.
Besides Bhasin et al. [21] proposed an online adaptive solution via RL for the unbounded optimization control nonlinear systems with CT uncertain problem. The advantage of using the IAC structure is that the learning of critics, actors, and identifiers is successive and simultaneous, removing the knowledge of system drift dynamics.
However, the above proposed control design algorithm for the affine nonlinear systems cannot be used to solve the optimal control issues for unmatching condition nonlinear systems, because it cannot guarantee the optimization of each subsystem.
The above research methods on affine nonlinear systems cannot be applied to nonlinear systems with unmatching conditions and the optimality of each subsystem can not be guaranteed. In order to solve the problem of unmatching conditions, we used the backstepping technology, which can also optimize each subsystem.
Consider the following strict feedback nonlinear systems as:
$ {˙xi=fi(¯xi)+xi+1,i=1,2,⋯,n−1˙xn=fn(¯xn)+uy=x1 $
|
(3.1) |
where $ u $ and $ y $ are the control input and output, $ x $ is the state, $ \bar{x_{i}} = [x_{1}, x_{2}, \cdots, x_{i}] $, is the system state vector. $ f_{i}(\cdot) $ is the uncertain nonlinear function, which satisfies $ f(0) = 0 $.
In 1995, Kristic [22] firstly proposed the backstepping technology. The design idea of the backstepping algorithm is as follows: for systems that satisfy strict feedback control structures, via the backstepping algorithm, the Lyapunov function and controller are constructed in a systematic way. Then, for each subsystem, local Lyapunov function and intermediate control function are designed successively until the design of the whole controller is completed.
For the sake of solving the control issue for unmatching nonlinear systems (3.1), Wen et al. [23] first proposed an optimized backstepping control technology, under the backstepping framework, we can ensure that each subsystem can be optimized. Based on [23], for a kind of nonlinear large-scale systems with strict-feedback structure, Tong et al. [24] proposed the fuzzy decentralized adaptive optimal control, and used FLS to identify the uncertain nonlinear function of the systems. And in [25], for a quarter of the car active electric suspension systems, Li et al. addressed the output-feedback adaptive NN optimization control issue.
Because there are unknown nonlinear functions, the updating laws and learning laws designed for the above systems are very complex. In order to solve this problem, in [26], Wen et al. proposed a simplified RL algorithm, which generates a negative gradient of a simple positive function from the partial derivative of HJB equation, and derives a new law from the negative gradient.
Define the Hamiltonian's approximation error as:
$ E=H(ˆz,u,ˆV∗ˆz)−H(z,u∗,V∗ˆz)=H(ˆz,u,ˆV∗ˆz) $
|
(3.2) |
where $ V_{\hat{z}}^{*}(\hat{z}) $ is the gradient of $ V^{*}(\hat{z}) $, $ u^{*} $ is the optimal control. Since $ V_{\hat{z}}^{*}(\hat{z}) $ and $ u^{*} $ contain the unknown part $ V_{\hat{z}}^{0}(\hat{z}) $, which can be approximated on a compact set by NNs as:
$ V0ˆz(ˆz)=Θ∗TVφV(ˆz)+εV(ˆz) $
|
(3.3) |
Since $ \Theta_{V}^{*} $ is an uncertain constant vector, it is not available in practical control, RL algorithm is implemented by both critic-actor NNs.
The learning law of critic NN is designed as:
$ ˙ˆΘVc(t)=−kcφV(ˆz)φTV(ˆz)ˆΘVc(t) $
|
(3.4) |
where $ k_{c} $ is the critic network learning rate, $ \hat{\Theta}_{Vc}(t) $ is the critic NN weight.
The learning law of actor NN is designed as:
$ ˙ˆΘVa(t)=−φV(ˆz)φTV(ˆz)(ka(ˆΘVa(t)−ˆΘVc(t))+kcˆΘVc(t)) $
|
(3.5) |
where $ k_{a} $ is the actor network learning rate, $ \hat{\Theta}_{Va}(t) $ is the actor NN weight, $ k_{a} > k_{c} > 0 $.
In accordance with the above description, the optimal solution $ {{\hat{\alpha}}}(\hat{z}) $ is supposed to meet $ E(t) = H(\hat{z}, u, \hat{V}_{\hat{z}}^{*})\rightarrow 0 $.
If $ H(\hat{z}, u, \hat{V}_{\hat{z}}^{*}) $ is held and exist the unique solution, then it is equivalent to the following equation holds:
$ ∂H(ˆz,u,ˆV∗ˆz)∂ˆΘVa=φVφTV(ˆΘTVa(t)−ˆΘTVc(t))=0 $
|
(3.6) |
The positive definite function is designed as:
$ P(t)=(ˆΘVa(t)−ˆΘVc(t))T(ˆΘVa(t)−ˆΘVc(t)) $
|
(3.7) |
Clearly, the Eq (3.6) is the equivalent to $ P(t) = 0 $. Since $ \frac{\partial P(t)}{\partial \hat{\Theta}_{Va}(t)} = -\frac{\partial P(t)}{{\partial \hat{\Theta}_{Vc}}(t)} = 2(\hat{\Theta}_{Va}(t)-\hat{\Theta}_{Vc}(t)) $, we can get
$ ∂P(t)dt=∂P(t)∂ˆΘVc(t)⋅ˆΘVc(t)+∂P(t)∂ˆΘVa(t)⋅ˆΘVa(t)=−kc∂P(t)∂ˆΘVc(t)φVφTVˆΘTVc(t)−∂P(t)∂ˆΘVc(t)φVφTV[ka(ˆΘVa(t)−ˆΘVc(t))+kcˆΘVc(t)]=−ka2∂P(t)∂ˆΘa(t)φVφTV∂P(t)∂ˆΘa(t)≤0 $
|
(3.8) |
In [27], for nonlinear lithium battery systems, Pei et al. addressed adaptive NN output feedback optimization control problem, and the stability of the nonlinear lithium battery is proved.
On the basis of [26], under the frame of backstepping control, some simplified-based adaptive optimization control algorithms have been proposed, which require construct all intermediate control functions and the actual control function of backstepping to be the optimization controls, hence, RL is performed in each subsystem (see Figure 2).
In [28], Wen et al. addressed optimization control method for nonlinear strict-feedback systems with unknown functions. In [29], for second-order unknown nonlinear multiagent systems, Lan et al. proposed a distributed time-varying optimization formation protocol based on an adaptive NN state observer. In [30], Xiao et al. addressed the distributed optimization containment control issue for multiple nonholonomic mobile robots differential game.
It is worth mentioning that system states usually need to be confined within some preselected compact sets due to the physical limitations of actual systems. For real systems, in [31], Jiang and Lou considered the input-to-state stability (ISS) of delayed systems with bounded-delay impulses. In [32], for a hydraulic servo actuator (HSA) with sensor faults, Vladimir and Ljubisa investigated the mechanism for the fault estimation (FE) problem. However, methods in [29,30] could not solve the actual constraint problem. To solve this problem, various state-constrained control methodologies is discussed.
Aiming at strict-feedback nonlinear systems, which contain immeasurable states and internal dynamics, Li et al. [33] proposed an output-feedback adaptive NN optimization control design. Under the backstepping control design, there will be coupling terms or cross terms at each step, which will lead to that each subsystem is not optimal. Therefore, state constraints should be introduced to make the coupling terms bounded to ensure that each subsystem is optimal. And all the states are limited in the compact sets, that is, $ |x_{i}| < k_{ci} $, where $ k_{ci} > 0 $.
The neoteric barrier optimization performance index functions for subsystems are designed to ensure that the system state does not violate the constraint bounds and achieves the optimization control objective, which is selected as:
$ J(z(t))=limτ→∞1τ∫τtq(z((t),α(z)))dz $
|
(3.9) |
where $ \tau $ is the terminal time, $ q(z, \alpha) = \xi log[k_{b}^{4}/(k_{b}^{4}-z^{4})]+r(\alpha)^{2} $, $ \xi > 0 $ is a constant, $ \alpha $ is the intermediate control function, The following Hamiltonian can be derived as:
$ H(ˆz,u,ˆV∗ˆz)=ξlogk4b(k4b−z4)+r(α)2+dV∗(z)dz(α∗+g(x)−yr) $
|
(3.10) |
According to the algorithm presented in [34], for power systems with stochastic character, Li et al. designed the adaptive NN optimal tracking control to resolve the issue of state constraints and uncertain nonlinear dynamics. In [35], Li et al. put forward an adaptive NN optimized output-feedback control method to solve the issue of unknown nonlinear dynamics and input saturation. In [36], for uncertain nonlinear systems with time-varying full state constraints, input saturation and unknown control direction, Wu and Xie employed asymmetric barrier Lyapunov functions, the auxiliary subsystem and the Nussbaum gain technique.
Based on the above published works, some adaptive optimal control methods via backstepping control have also been applied to practical systems, for example, see [37,38]. In [37], Li et al. presented an adaptive NN optimized control strategy for full vehicle active suspension system. And in [38], Li et al. studied adaptive optimal formation control approach for second-order stochastic multi-agent system, which contains unknown nonlinear dynamics.
Based on the inverse optimization control method in [1], Ezal et al. [38] proposed a new robust backstepping inverse optimal control design, which achieved both local optimization and global inverse optimization. For a class of nonlinear uncertain strict feedback systems, Li et al. [39] designed adaptive fuzzy inverse optimization control by establishing an equivalent system and an auxiliary system.
System (2.1) can be rewritten as the following nonlinear system:
$ ˙x=G(x)u+F(x)+q(x) $
|
(3.11) |
where $ u\in \textbf{R} $ is the control input, $ x $ is the state vector, $ x = 0 $ is the equilibrium point of system. $ q(x) $ is an uncertain bounded function vector, $ G(x) $ and $ F(x) $ are smooth function vectors.
Define $ \gamma $ is a class $ K_{\infty} $ function, then the derivative of $ \gamma $ exists and it is also a class $ K_{\infty} $ function. An auxiliary system is constructed for the nonlinear system (2.1):
$ ˙x=lγ(2|LΔV|R(x))×R−2(x)(LΔV)T(LΔV)2+F(x)+G(x)u $
|
(3.12) |
where $ V(x) $ is the control Lyapunov function. $ L\vartriangle V = \partial\vartriangle V/\partial x $, $ L_{F}V_{n} = \partial V_{n}/\partial xF(x) $, $ L_{G}V_{n} = \partial V_{n}/\partial xG(x) $.
The cost functional is selected as:
$ J(u)=supd∈D{limt→∞[∫t0(l(x))+uTRu−γ(d))dτ+E(x)]} $
|
(3.13) |
where $ D $ is a set of locally bounded functions of $ x $, $ R(x) $ is matrix-valued function, which satisfied that $ R(x) = R(x)^{T} > 0 $. $ E(x) $ and $ l(x) $ are positive definite radially unbounded functions.
The fuzzy adaptive inverse optimization control structure is shown in Figure 3.
And in [40], Li et al. studied a fuzzy inverse optimization fuzzy adaptive output feedback control method based on observer for a class of nonlinear strict feedback systems. In [41], Lu et al. addressed a fuzzy adaptive inverse optimization control issue, and a switching inverse optimization controller is constructed by using a single parameter learning mechanism, which confirmed that the method guarantees the input-to-state stability of the control systems.
Inspired by the above theory, the inverse optimization theory is also widely applied to some practical systems. In [42], for vehicle active suspension system with unknown nonlinear dynamics, Li et al. designed an adaptive fuzzy inverse optimal control method via state observer. Long et al. [43] proposed an inverse optimal fuzzy adaptive control approach for the system of flexible spacecraft system with fault-free actuator, which is subjected to input saturation, uncertain parameter and external disturbances.
In addition, people hope the practical engineering will reach the stable in finite time, and use the less control energy when achieving the satisfactory performance indicators simultaneously. Thus, how to achieve the effective balance between control quality and control energy has become a hot research issue. In [44], for nonlinear impulsive systems, Li and Ho studied the problem of finite-time stability (FTS). In [45], Li and Yang developed the Lyapunov–Razumikhin method for finite-time stability (FTS) and finite-time contractive stability (FTCS) of time-delay systems. In [46], via the power integral control approach and backstepping control method, Yang designed a semi-global real finite time controller. Then, according to the basic idea of inverse optimization, an appropriate objective functional is constructed, and the constructed objective functional is minimized by adjusting the parameters of semi-global real finite time controller.
Consider the Lyapunov function as follows:
$ V1=[r12v−τ]x(2v−τ)r1+12¯ω12 $
|
(3.14) |
where $ \bar{\omega_{1}} = \omega_{1}^{*}-\hat{\omega_{1}} $, $ \hat{\omega_{1}} $ is the estimation of the unknown parameter $ \omega_{1}^{*} $, $ v = max\{{r_{1}, p_{1}r_{2}}\} $, $ j = 1, 2, \cdots, n $, $ p_{j}r_{j+1} = r_{j}+\tau $, $ \upsilon = \mathop{max}\limits_{1\leq j\leq n} \{r_{j}, p_{j}r_{j+1}\} $, $ j = 1, 2, \cdots, n $. $ r_{j} $ and $ p_{j} $ is the ratio of two positive odd numbers. $ r_{1} = 1 $, $ \tau $ is the design parameter.
Based on [46], for a class of interlinked nonlinear systems with powers of positive odd rational numbers, Li et al. [47] developed a series of homogeneous controllers, which are capable of guaranteeing the local finite-time stability of the closed-loop systems by using the adding one power integrator approach and backstepping technique.
Most of the existing optimization finite-time control methods are limited by complicated design and updating process, which vastly affect the ideal property of optimization finite-time control. In order to solve this issue, in [48], Lu et al. first proposed an immediate fuzzy adaptive inverse optimization approach to receive a switching-type inverse optimization controller and a one parameter learning mechanism. The inverse optimal stabilization is solvable, and there exists a matrix-valued function $ P(x) $, which satisfied that $ P(x) = P(x)^{T} > 0 $, then the cost function is defined as:
$ J(u)=limt→∞∫t0[L(x)+ℏ(|P(x)12u)]dτ} $
|
(3.15) |
where $ \hbar $ and its derivative $ \hbar' $ are $ K_{\infty} $ functions, $ L(x) $ is positive functions, $ u(x) $ is away from the origin in succession with $ u(x) = 0 $.
For a kind of robotic manipulator system, which contains uncertain dynamics and input saturation, the authors in [49] proposed a fixed-time trajectory tracking control approach based on RL. For the sake of guaranteeing that $ e_{1} $ and $ e_{2} $ convergence to diminutive neighborhood around $ 0 $ in a uniformly bounded convergence time $ T_{s} $, where $ T_{s} $ stands alone with the original states. A noval nonsingular fixed-time fast terminal sliding mode is proposed as:
$ s=K(e1e1)+sigυ1(e2) $
|
(3.16) |
where $ Ke_{1} = diag[{k_{e11}, k_{e12}, \cdots, k_{e1n}}] $ is a diagonal matrix. $ k_{e1i}, i = 1, 2, \cdots, n $, are designed as:
$ ke1i=(α|e1i|p−1/(kυ1)+β|e1i|g−1/(kυ1))kυ1 $
|
(3.17) |
where $ p $ and $ g $ are positive scalars with $ gk > 1 $ and $ 1/\upsilon_{1} < pk < 1 $, $ \alpha > 0 $, $ \beta > 0 $, $ k > 1 $, $ \upsilon_{1} > 1 $.
Obviously, the above developed control method can effectively solve the finite/fixed-time optimal control problems and can make the minimize the cost function. Besides, in [50], Hu et al. considered the fixed-time stability of delayed neural networks with impulsive perturbations.
It can be seen from this review that optimization control design for unknown nonlinear systems via RL and ADP has been diffusely studied in control area and has achieved fruitful results. The origin and the development of optimization algorithms have been introduced, the research results of optimization control of affine nonlinear systems have been summarized. Then, under the frame of backstepping control, the adaptive optimal control, finite-time inverse optimal control, constraint control have also been described for strict-feedback nonlinear systems. At the same time, we have summarized the applications development of adaptive optimization control methods. In addition, as a novel hot issue in this field, finite/fixed-time optimal control via backstepping and RL/ADP for nonlinear systems have attracted considerable attentions, both theory and practical applications also need to be further studied in the future.
This work was supported by the National Natural Science Foundation of China under Grant No. 61822307.
The authors declare there is no conflict of interest.
[1] |
Volders PJ, Verheggen K, Menschaert G, et al. (2015) An update on LNCipedia: a database for annotated human lncRNA sequences. Nucleic Acids Res 43: D174-180. doi: 10.1093/nar/gku1060
![]() |
[2] | Amati B, Frank SR, Donjerkovic D, et al. (2001) Function of the c-Myc oncoprotein in chromatin remodeling and transcription. Biochim Biophys Acta 1471: M135-145. |
[3] |
Bretones G, Delgado MD, Leon J (2015) Myc and cell cycle control. Biochim Biophys Acta 1849: 506-516. doi: 10.1016/j.bbagrm.2014.03.013
![]() |
[4] | Dang CV (2013) MYC, metabolism, cell growth, and tumorigenesis. Cold Spring Harb Perspect Med 3. |
[5] |
McMahon SB (2014) MYC and the control of apoptosis. Cold Spring Harb Perspect Med 4: a014407. doi: 10.1101/cshperspect.a014407
![]() |
[6] | Vennstrom B, Sheiness D, Zabielski J, et al. (1982) Isolation and characterization of c-myc, a cellular homolog of the oncogene (v-myc) of avian myelocytomatosis virus strain 29. J Virol 42: 773-779. |
[7] |
Zheng GX, Do BT, Webster DE, et al. (2014) Dicer-microRNA-Myc circuit promotes transcription of hundreds of long noncoding RNAs. Nat Struct Mol Biol 21: 585-590. doi: 10.1038/nsmb.2842
![]() |
[8] |
Winkle M, van den Berg A, Tayari M, et al. (2015) Long noncoding RNAs as a novel component of the Myc transcriptional network. FASEB J 29: 2338-2346. doi: 10.1096/fj.14-263889
![]() |
[9] |
Xiang JF, Yang L, Chen LL (2015) The long noncoding RNA regulation at the MYC locus. Curr Opin Genet Dev 33: 41-48. doi: 10.1016/j.gde.2015.07.001
![]() |
[10] |
Rinn JL, Chang HY (2012) Genome regulation by long noncoding RNAs. Annu Rev Biochem 81: 145-166. doi: 10.1146/annurev-biochem-051410-092902
![]() |
[11] |
Kapranov P, Cawley SE, Drenkow J, et al. (2002) Large-scale transcriptional activity in chromosomes 21 and 22. Science 296: 916-919. doi: 10.1126/science.1068597
![]() |
[12] |
Rinn JL, Euskirchen G, Bertone P, et al. (2003) The transcriptional activity of human Chromosome 22. Genes Dev 17: 529-540. doi: 10.1101/gad.1055203
![]() |
[13] |
Guttman M, Garber M, Levin JZ, et al. (2010) Ab initio reconstruction of cell type-specific transcriptomes in mouse reveals the conserved multi-exonic structure of lincRNAs. Nat Biotechnol 28: 503-510. doi: 10.1038/nbt.1633
![]() |
[14] |
Cabili MN, Trapnell C, Goff L, et al. (2011) Integrative annotation of human large intergenic noncoding RNAs reveals global properties and specific subclasses. Genes Dev 25: 1915-1927. doi: 10.1101/gad.17446611
![]() |
[15] |
Wu J, Okada T, Fukushima T, et al. (2012) A novel hypoxic stress-responsive long non-coding RNA transcribed by RNA polymerase III in Arabidopsis. RNA Biol 9: 302-313. doi: 10.4161/rna.19101
![]() |
[16] |
Derrien T, Johnson R, Bussotti G, et al. (2012) The GENCODE v7 catalog of human long noncoding RNAs: analysis of their gene structure, evolution, and expression. Genome Res 22: 1775-1789. doi: 10.1101/gr.132159.111
![]() |
[17] |
Gardini A, Shiekhattar R (2015) The many faces of long noncoding RNAs. FEBS J 282: 1647-1657. doi: 10.1111/febs.13101
![]() |
[18] |
Wilusz JE, Spector DL (2010) An unexpected ending: noncanonical 3' end processing mechanisms. RNA 16: 259-266. doi: 10.1261/rna.1907510
![]() |
[19] |
Zhang Y, Yang L, Chen LL (2014) Life without A tail: new formats of long noncoding RNAs. Int J Biochem Cell Biol 54: 338-349. doi: 10.1016/j.biocel.2013.10.009
![]() |
[20] |
Peart N, Sataluri A, Baillat D, et al. (2013) Non-mRNA 3' end formation: how the other half lives. Wiley Interdiscip Rev RNA 4: 491-506. doi: 10.1002/wrna.1174
![]() |
[21] | Ravasi T, Suzuki H, Pang KC, et al. (2006) Experimental validation of the regulated expression of large numbers of non-coding RNAs from the mouse genome. Genome Res 16: 11-19. |
[22] |
Djebali S, Davis CA, Merkel A, et al. (2012) Landscape of transcription in human cells. Nature 489: 101-108. doi: 10.1038/nature11233
![]() |
[23] |
He S, Liu S, Zhu H (2011) The sequence, structure and evolutionary features of HOTAIR in mammals. BMC Evol Biol 11: 102. doi: 10.1186/1471-2148-11-102
![]() |
[24] |
Brown JA, Bulkley D, Wang J, et al. (2014) Structural insights into the stabilization of MALAT1 noncoding RNA by a bipartite triple helix. Nat Struct Mol Biol 21: 633-640. doi: 10.1038/nsmb.2844
![]() |
[25] |
Brown JA, Valenstein ML, Yario TA, et al. (2012) Formation of triple-helical structures by the 3'-end sequences of MALAT1 and MENbeta noncoding RNAs. Proc Natl Acad Sci U S A 109: 19202-19207. doi: 10.1073/pnas.1217338109
![]() |
[26] |
Smith MA, Gesell T, Stadler PF, et al. (2013) Widespread purifying selection on RNA structure in mammals. Nucleic Acids Res 41: 8220-8236. doi: 10.1093/nar/gkt596
![]() |
[27] |
Somarowthu S, Legiewicz M, Chillon I, et al. (2015) HOTAIR forms an intricate and modular secondary structure. Mol Cell 58: 353-361. doi: 10.1016/j.molcel.2015.03.006
![]() |
[28] |
Mortimer SA, Kidwell MA, Doudna JA (2014) Insights into RNA structure and function from genome-wide studies. Nat Rev Genet 15: 469-479. doi: 10.1038/nrg3681
![]() |
[29] | Ding Y, Tang Y, Kwok CK, et al. (2014) In vivo genome-wide profiling of RNA secondary structure reveals novel regulatory features. Nature 505: 696-700. |
[30] |
Kertesz M, Wan Y, Mazor E, et al. (2010) Genome-wide measurement of RNA secondary structure in yeast. Nature 467: 103-107. doi: 10.1038/nature09322
![]() |
[31] |
Lucks JB, Mortimer SA, Trapnell C, et al. (2011) Multiplexed RNA structure characterization with selective 2'-hydroxyl acylation analyzed by primer extension sequencing (SHAPE-Seq). Proc Natl Acad Sci U S A 108: 11063-11068. doi: 10.1073/pnas.1106501108
![]() |
[32] |
Seetin MG, Kladwang W, Bida JP, et al. (2014) Massively parallel RNA chemical mapping with a reduced bias MAP-seq protocol. Methods Mol Biol 1086: 95-117. doi: 10.1007/978-1-62703-667-2_6
![]() |
[33] |
Underwood JG, Uzilov AV, Katzman S, et al. (2010) FragSeq: transcriptome-wide RNA structure probing using high-throughput sequencing. Nat Methods 7: 995-1001. doi: 10.1038/nmeth.1529
![]() |
[34] |
Aviran S, Trapnell C, Lucks JB, et al. (2011) Modeling and automation of sequencing-based characterization of RNA structure. Proc Natl Acad Sci U S A 108: 11069-11074. doi: 10.1073/pnas.1106541108
![]() |
[35] | Rouskin S, Zubradt M, Washietl S, et al. (2014) Genome-wide probing of RNA structure reveals active unfolding of mRNA structures in vivo. Nature 505: 701-705. |
[36] |
Novikova IV, Hennelly SP, Sanbonmatsu KY (2013) Tackling structures of long noncoding RNAs. Int J Mol Sci 14: 23672-23684. doi: 10.3390/ijms141223672
![]() |
[37] |
Fatica A, Bozzoni I (2014) Long non-coding RNAs: new players in cell differentiation and development. Nat Rev Genet 15: 7-21. doi: 10.1038/nri3777
![]() |
[38] |
Batista PJ, Chang HY (2013) Long noncoding RNAs: cellular address codes in development and disease. Cell 152: 1298-1307. doi: 10.1016/j.cell.2013.02.012
![]() |
[39] |
Batista PJ, Chang HY (2013) Cytotopic localization by long noncoding RNAs. Curr Opin Cell Biol 25: 195-199. doi: 10.1016/j.ceb.2012.12.001
![]() |
[40] |
Han X, Yang F, Cao H, et al. (2015) Malat1 regulates serum response factor through miR-133 as a competing endogenous RNA in myogenesis. FASEB J 29: 3054-3064. doi: 10.1096/fj.14-259952
![]() |
[41] | Yoon JH, Abdelmohsen K, Kim J, et al. (2013) Scaffold function of long non-coding RNA HOTAIR in protein ubiquitination. Nat Commun 4: 2939. |
[42] |
McHugh CA, Chen CK, Chow A, et al. (2015) The Xist lncRNA interacts directly with SHARP to silence transcription through HDAC3. Nature 521: 232-236. doi: 10.1038/nature14443
![]() |
[43] |
Deng K, Guo X, Wang H, et al. (2014) The lncRNA-MYC regulatory network in cancer. Tumour Biol 35: 9497-9503. doi: 10.1007/s13277-014-2511-y
![]() |
[44] |
Beroukhim R, Mermel CH, Porter D, et al. (2010) The landscape of somatic copy-number alteration across human cancers. Nature 463: 899-905. doi: 10.1038/nature08822
![]() |
[45] | Colombo T, Farina L, Macino G, et al. (2015) PVT1: a rising star among oncogenic long noncoding RNAs. Biomed Res Int 2015: 304208. |
[46] |
Walz S, Lorenzin F, Morton J, et al. (2014) Activation and repression by oncogenic MYC shape tumour-specific gene expression profiles. Nature 511: 483-487. doi: 10.1038/nature13473
![]() |
[47] |
Carramusa L, Contino F, Ferro A, et al. (2007) The PVT-1 oncogene is a Myc protein target that is overexpressed in transformed cells. J Cell Physiol 213: 511-518. doi: 10.1002/jcp.21133
![]() |
[48] | Tseng YY, Moriarity BS, Gong W, et al. (2014) PVT1 dependence in cancer with MYC copy-number increase. Nature 512: 82-86. |
[49] |
Zanke BW, Greenwood CM, Rangrej J, et al. (2007) Genome-wide association scan identifies a colorectal cancer susceptibility locus on chromosome 8q24. Nat Genet 39: 989-994. doi: 10.1038/ng2089
![]() |
[50] |
Tenesa A, Farrington SM, Prendergast JG, et al. (2008) Genome-wide association scan identifies a colorectal cancer susceptibility locus on 11q23 and replicates risk loci at 8q24 and 18q21. Nat Genet 40: 631-637. doi: 10.1038/ng.133
![]() |
[51] |
Tomlinson I, Webb E, Carvajal-Carmona L, et al. (2007) A genome-wide association scan of tag SNPs identifies a susceptibility variant for colorectal cancer at 8q24.21. Nat Genet 39: 984-988. doi: 10.1038/ng2085
![]() |
[52] |
Nissan A, Stojadinovic A, Mitrani-Rosenbaum S, et al. (2012) Colon cancer associated transcript-1: a novel RNA expressed in malignant and pre-malignant human tissues. Int J Cancer 130: 1598-1606. doi: 10.1002/ijc.26170
![]() |
[53] |
Ahmadiyeh N, Pomerantz MM, Grisanzio C, et al. (2010) 8q24 prostate, breast, and colon cancer risk loci show tissue-specific long-range interaction with MYC. Proc Natl Acad Sci U S A 107: 9742-9746. doi: 10.1073/pnas.0910668107
![]() |
[54] |
Kim T, Cui R, Jeon YJ, et al. (2014) Long-range interaction and correlation between MYC enhancer and oncogenic long noncoding RNA CARLo-5. Proc Natl Acad Sci U S A 111: 4173-4178. doi: 10.1073/pnas.1400350111
![]() |
[55] |
Pomerantz MM, Ahmadiyeh N, Jia L, et al. (2009) The 8q24 cancer risk variant rs6983267 shows long-range interaction with MYC in colorectal cancer. Nat Genet 41: 882-884. doi: 10.1038/ng.403
![]() |
[56] |
Sur IK, Hallikas O, Vaharautio A, et al. (2012) Mice lacking a Myc enhancer that includes human SNP rs6983267 are resistant to intestinal tumors. Science 338: 1360-1363. doi: 10.1126/science.1228606
![]() |
[57] |
Tuupanen S, Turunen M, Lehtonen R, et al. (2009) The common colorectal cancer predisposition SNP rs6983267 at chromosome 8q24 confers potential to enhanced Wnt signaling. Nat Genet 41: 885-890. doi: 10.1038/ng.406
![]() |
[58] |
Xiang JF, Yin QF, Chen T, et al. (2014) Human colorectal cancer-specific CCAT1-L lncRNA regulates long-range chromatin interactions at the MYC locus. Cell Res 24: 513-531. doi: 10.1038/cr.2014.35
![]() |
[59] |
Deng L, Yang SB, Xu FF, et al. (2015) Long noncoding RNA CCAT1 promotes hepatocellular carcinoma progression by functioning as let-7 sponge. J Exp Clin Cancer Res 34: 18. doi: 10.1186/s13046-015-0136-7
![]() |
[60] |
He X, Tan X, Wang X, et al. (2014) C-Myc-activated long noncoding RNA CCAT1 promotes colon cancer cell proliferation and invasion. Tumour Biol 35: 12181-12188. doi: 10.1007/s13277-014-2526-4
![]() |
[61] |
Yang F, Xue X, Bi J, et al. (2013) Long noncoding RNA CCAT1, which could be activated by c-Myc, promotes the progression of gastric carcinoma. J Cancer Res Clin Oncol 139: 437-445. doi: 10.1007/s00432-012-1324-x
![]() |
[62] |
Ling H, Spizzo R, Atlasi Y, et al. (2013) CCAT2, a novel noncoding RNA mapping to 8q24, underlies metastatic progression and chromosomal instability in colon cancer. Genome Res 23: 1446-1461. doi: 10.1101/gr.152942.112
![]() |
[63] | Kim T, Jeon YJ, Cui R, et al. (2015) Role of MYC-regulated long noncoding RNAs in cell cycle regulation and tumorigenesis. J Natl Cancer Inst 107. |
[64] | Ye Z, Zhou M, Tian B, et al. (2015) Expression of lncRNA-CCAT1, E-cadherin and N-cadherin in colorectal cancer and its clinical significance. Int J Clin Exp Med 8: 3707-3715. |
[65] |
Alaiyan B, Ilyayev N, Stojadinovic A, et al. (2013) Differential expression of colon cancer associated transcript1 (CCAT1) along the colonic adenoma-carcinoma sequence. BMC Cancer 13: 196. doi: 10.1186/1471-2407-13-196
![]() |
[66] | Kim T, Cui R, Jeon YJ, et al. (2015) MYC-repressed long noncoding RNAs antagonize MYC-induced cell proliferation and cell cycle progression. Oncotarget. |
[67] |
Prensner JR, Chen W, Han S, et al. (2014) The long non-coding RNA PCAT-1 promotes prostate cancer cell proliferation through cMyc. Neoplasia 16: 900-908. doi: 10.1016/j.neo.2014.09.001
![]() |
[68] |
Yamamura S, Saini S, Majid S, et al. (2012) MicroRNA-34a modulates c-Myc transcriptional complexes to suppress malignancy in human prostate cancer cells. PLoS One 7: e29722. doi: 10.1371/journal.pone.0029722
![]() |
[69] |
Siemens H, Jackstadt R, Hunten S, et al. (2011) miR-34 and SNAIL form a double-negative feedback loop to regulate epithelial-mesenchymal transitions. Cell Cycle 10: 4256-4271. doi: 10.4161/cc.10.24.18552
![]() |
[70] |
Benassi B, Flavin R, Marchionni L, et al. (2012) MYC is activated by USP2a-mediated modulation of microRNAs in prostate cancer. Cancer Discov 2: 236-247. doi: 10.1158/2159-8290.CD-11-0219
![]() |
[71] |
Poliseno L, Salmena L, Zhang J, et al. (2010) A coding-independent function of gene and pseudogene mRNAs regulates tumour biology. Nature 465: 1033-1038. doi: 10.1038/nature09144
![]() |
[72] |
Salmena L, Poliseno L, Tay Y, et al. (2011) A ceRNA hypothesis: the Rosetta Stone of a hidden RNA language? Cell 146: 353-358. doi: 10.1016/j.cell.2011.07.014
![]() |
[73] |
Ge X, Chen Y, Liao X, et al. (2013) Overexpression of long noncoding RNA PCAT-1 is a novel biomarker of poor prognosis in patients with colorectal cancer. Med Oncol 30: 588. doi: 10.1007/s12032-013-0588-6
![]() |
[74] |
Chung S, Nakagawa H, Uemura M, et al. (2011) Association of a novel long non-coding RNA in 8q24 with prostate cancer susceptibility. Cancer Sci 102: 245-252. doi: 10.1111/j.1349-7006.2010.01737.x
![]() |
[75] |
Hung CL, Wang LY, Yu YL, et al. (2014) A long noncoding RNA connects c-Myc to tumor metabolism. Proc Natl Acad Sci U S A 111: 18697-18702. doi: 10.1073/pnas.1415669112
![]() |
[76] |
Chu C, Qu K, Zhong FL, et al. (2011) Genomic maps of long noncoding RNA occupancy reveal principles of RNA-chromatin interactions. Mol Cell 44: 667-678. doi: 10.1016/j.molcel.2011.08.027
![]() |
[77] | Pickard MR, Williams GT (2015) Molecular and Cellular Mechanisms of Action of Tumour Suppressor GAS5 LncRNA. Genes (Basel) 6: 484-499. |
[78] |
Hu G, Lou Z, Gupta M (2014) The long non-coding RNA GAS5 cooperates with the eukaryotic translation initiation factor 4E to regulate c-Myc translation. PLoS One 9: e107016. doi: 10.1371/journal.pone.0107016
![]() |
[79] |
Mourtada-Maarabouni M, Pickard MR, Hedge VL, et al. (2009) GAS5, a non-protein-coding RNA, controls apoptosis and is downregulated in breast cancer. Oncogene 28: 195-208. doi: 10.1038/onc.2008.373
![]() |
[80] |
Pickard MR, Mourtada-Maarabouni M, Williams GT (2013) Long non-coding RNA GAS5 regulates apoptosis in prostate cancer cell lines. Biochim Biophys Acta 1832: 1613-1623. doi: 10.1016/j.bbadis.2013.05.005
![]() |
[81] |
Sun M, Jin FY, Xia R, et al. (2014) Decreased expression of long noncoding RNA GAS5 indicates a poor prognosis and promotes cell proliferation in gastric cancer. BMC Cancer 14: 319. doi: 10.1186/1471-2407-14-319
![]() |
[82] | Tu ZQ, Li RJ, Mei JZ, et al. (2014) Down-regulation of long non-coding RNA GAS5 is associated with the prognosis of hepatocellular carcinoma. Int J Clin Exp Pathol 7: 4303-4309. |
[83] | Li LJ, Zhu JL, Bao WS, et al. (2014) Long noncoding RNA GHET1 promotes the development of bladder cancer. Int J Clin Exp Pathol 7: 7196-7205. |
[84] |
Yang F, Xue X, Zheng L, et al. (2014) Long non-coding RNA GHET1 promotes gastric carcinoma cell proliferation by increasing c-Myc mRNA stability. FEBS J 281: 802-813. doi: 10.1111/febs.12625
![]() |
[85] |
Lemm I, Ross J (2002) Regulation of c-myc mRNA decay by translational pausing in a coding region instability determinant. Mol Cell Biol 22: 3959-3969. doi: 10.1128/MCB.22.12.3959-3969.2002
![]() |
[86] | Weidensdorfer D, Stohr N, Baude A, et al. (2009) Control of c-myc mRNA stability by IGF2BP1-associated cytoplasmic RNPs. RNA 15: 104-115. |
[87] |
Matouk IJ, DeGroot N, Mezan S, et al. (2007) The H19 non-coding RNA is essential for human tumor growth. PLoS One 2: e845. doi: 10.1371/journal.pone.0000845
![]() |
[88] |
Matouk IJ, Raveh E, Abu-lail R, et al. (2014) Oncofetal H19 RNA promotes tumor metastasis. Biochim Biophys Acta 1843: 1414-1426. doi: 10.1016/j.bbamcr.2014.03.023
![]() |
[89] | Jiang X, Yan Y, Hu M, et al. (2015) Increased level of H19 long noncoding RNA promotes invasion, angiogenesis, and stemness of glioblastoma cells. J Neurosurg: 1-8. |
[90] |
Lottin S, Adriaenssens E, Dupressoir T, et al. (2002) Overexpression of an ectopic H19 gene enhances the tumorigenic properties of breast cancer cells. Carcinogenesis 23: 1885-1895. doi: 10.1093/carcin/23.11.1885
![]() |
[91] |
Luo M, Li Z, Wang W, et al. (2013) Long non-coding RNA H19 increases bladder cancer metastasis by associating with EZH2 and inhibiting E-cadherin expression. Cancer Lett 333: 213-221. doi: 10.1016/j.canlet.2013.01.033
![]() |
[92] |
Ma C, Nong K, Zhu H, et al. (2014) H19 promotes pancreatic cancer metastasis by derepressing let-7's suppression on its target HMGA2-mediated EMT. Tumour Biol 35: 9163-9169. doi: 10.1007/s13277-014-2185-5
![]() |
[93] |
Zhang EB, Han L, Yin DD, et al. (2014) c-Myc-induced, long, noncoding H19 affects cell proliferation and predicts a poor prognosis in patients with gastric cancer. Med Oncol 31: 914. doi: 10.1007/s12032-014-0914-7
![]() |
[94] |
Barsyte-Lovejoy D, Lau SK, Boutros PC, et al. (2006) The c-Myc oncogene directly induces the H19 noncoding RNA by allele-specific binding to potentiate tumorigenesis. Cancer Res 66: 5330-5337. doi: 10.1158/0008-5472.CAN-06-0037
![]() |
[95] |
Shi Y, Wang Y, Luan W, et al. (2014) Long non-coding RNA H19 promotes glioma cell invasion by deriving miR-675. PLoS One 9: e86295. doi: 10.1371/journal.pone.0086295
![]() |
[96] |
Kallen AN, Zhou XB, Xu J, et al. (2013) The imprinted H19 lncRNA antagonizes let-7 microRNAs. Mol Cell 52: 101-112. doi: 10.1016/j.molcel.2013.08.027
![]() |
[97] |
Yan L, Zhou J, Gao Y, et al. (2015) Regulation of tumor cell migration and invasion by the H19/let-7 axis is antagonized by metformin-induced DNA methylation. Oncogene 34: 3076-3084. doi: 10.1038/onc.2014.236
![]() |
[98] |
Liao LM, Sun XY, Liu AW, et al. (2014) Low expression of long noncoding XLOC_010588 indicates a poor prognosis and promotes proliferation through upregulation of c-Myc in cervical cancer. Gynecol Oncol 133: 616-623. doi: 10.1016/j.ygyno.2014.03.555
![]() |
[99] |
Mestdagh P, Fredlund E, Pattyn F, et al. (2010) An integrative genomics screen uncovers ncRNA T-UCR functions in neuroblastoma tumours. Oncogene 29: 3583-3592. doi: 10.1038/onc.2010.106
![]() |
[100] |
Atmadibrata B, Liu PY, Sokolowski N, et al. (2014) The novel long noncoding RNA linc00467 promotes cell survival but is down-regulated by N-Myc. PLoS One 9: e88112. doi: 10.1371/journal.pone.0088112
![]() |
[101] |
Tee AE, Ling D, Nelson C, et al. (2014) The histone demethylase JMJD1A induces cell migration and invasion by up-regulating the expression of the long noncoding RNA MALAT1. Oncotarget 5: 1793-1804. doi: 10.18632/oncotarget.1785
![]() |
[102] | Liu PY, Erriquez D, Marshall GM, et al. (2014) Effects of a novel long noncoding RNA, lncUSMycN, on N-Myc expression and neuroblastoma progression. J Natl Cancer Inst 106. |
[103] |
Vadie N, Saayman S, Lenox A, et al. (2015) MYCNOS functions as an antisense RNA regulating MYCN. RNA Biol 12: 893-899. doi: 10.1080/15476286.2015.1063773
![]() |
[104] |
Stanton BR, Perkins AS, Tessarollo L, et al. (1992) Loss of N-myc function results in embryonic lethality and failure of the epithelial component of the embryo to develop. Genes Dev 6: 2235-2247. doi: 10.1101/gad.6.12a.2235
![]() |
[105] | Stanton BR, Parada LF (1992) The N-myc proto-oncogene: developmental expression and in vivo site-directed mutagenesis. Brain Pathol 2: 71-83. |