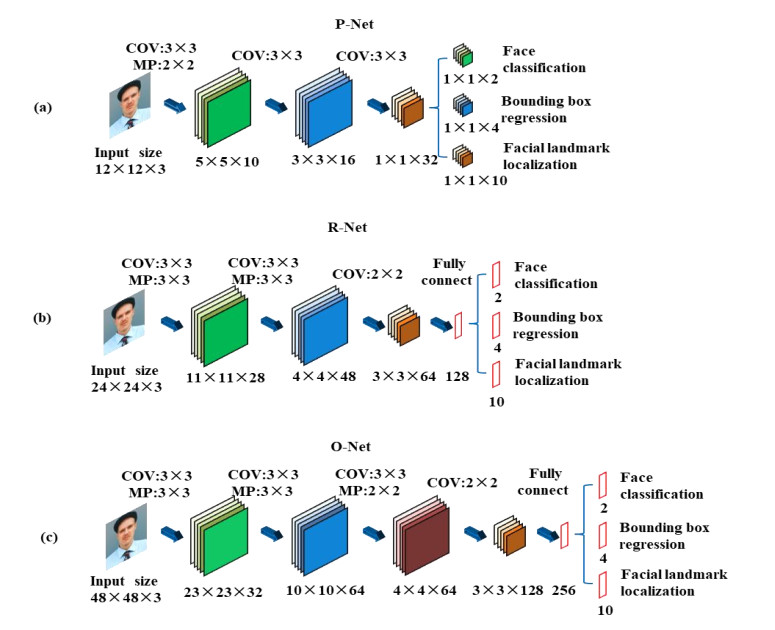
Citation: Wei Zhang, Sheng Cao, Jessica L. Martin, Joachim D. Mueller, Louis M. Mansky. Morphology and ultrastructure of retrovirus particles[J]. AIMS Biophysics, 2015, 2(3): 343-369. doi: 10.3934/biophy.2015.3.343
[1] | Jing Zhang, Haoliang Zhang, Ding Lang, Yuguang Xu, Hong-an Li, Xuewen Li . Research on rainy day traffic sign recognition algorithm based on PMRNet. Mathematical Biosciences and Engineering, 2023, 20(7): 12240-12262. doi: 10.3934/mbe.2023545 |
[2] | Yongmei Ren, Xiaohu Wang, Jie Yang . Maritime ship recognition based on convolutional neural network and linear weighted decision fusion for multimodal images. Mathematical Biosciences and Engineering, 2023, 20(10): 18545-18565. doi: 10.3934/mbe.2023823 |
[3] | Hong Qi, Guanglei Zhang, Heming Jia, Zhikai Xing . A hybrid equilibrium optimizer algorithm for multi-level image segmentation. Mathematical Biosciences and Engineering, 2021, 18(4): 4648-4678. doi: 10.3934/mbe.2021236 |
[4] | Shikai Wang, Heming Jia, Xiaoxu Peng . Modified salp swarm algorithm based multilevel thresholding for color image segmentation. Mathematical Biosciences and Engineering, 2020, 17(1): 700-724. doi: 10.3934/mbe.2020036 |
[5] | Xiao Ma, Xuemei Luo . Finger vein recognition method based on ant colony optimization and improved EfficientNetV2. Mathematical Biosciences and Engineering, 2023, 20(6): 11081-11100. doi: 10.3934/mbe.2023490 |
[6] | Shuai Cao, Biao Song . Visual attentional-driven deep learning method for flower recognition. Mathematical Biosciences and Engineering, 2021, 18(3): 1981-1991. doi: 10.3934/mbe.2021103 |
[7] | Sakorn Mekruksavanich, Wikanda Phaphan, Anuchit Jitpattanakul . Epileptic seizure detection in EEG signals via an enhanced hybrid CNN with an integrated attention mechanism. Mathematical Biosciences and Engineering, 2025, 22(1): 73-105. doi: 10.3934/mbe.2025004 |
[8] | Jinhua Zeng, Xiulian Qiu, Shaopei Shi . Image processing effects on the deep face recognition system. Mathematical Biosciences and Engineering, 2021, 18(2): 1187-1200. doi: 10.3934/mbe.2021064 |
[9] | Jing Wang, Jiaohua Qin, Xuyu Xiang, Yun Tan, Nan Pan . CAPTCHA recognition based on deep convolutional neural network. Mathematical Biosciences and Engineering, 2019, 16(5): 5851-5861. doi: 10.3934/mbe.2019292 |
[10] | Basem Assiri, Mohammad Alamgir Hossain . Face emotion recognition based on infrared thermal imagery by applying machine learning and parallelism. Mathematical Biosciences and Engineering, 2023, 20(1): 913-929. doi: 10.3934/mbe.2023042 |
In recent years, artificial intelligence technique has advanced rapidly [1,2,3]. Biometric recognition [4,5,6], which includes face recognition, voice recognition, fingerprint recognition, iris recognition, eye pattern recognition, etc. will occupy a very important position in the field of artificial intelligence in the future. At present, technologies such as smart cards based on radio frequency identification, second-generation ID cards, and user passwords are mostly used in identification, and biometrics will gradually occupy an important market share.
Due to the superiority of biometrics, it is widely used in bank payment, securities, transportation, e-commerce, airport subway entrance access control, attendance, and criminal investigation by public security and judicial departments [7,8,9]. Major enterprises, institutions, companies, and government agencies have established their own biometric-based access control systems and attendance systems to improve the informatization and intelligence of management, greatly improve management efficiency, and effectively liberate the labor force [10,11,12,13].
Face recognition technology started relatively late, but the technology related to face recognition [37] has developed rapidly, and has achieved remarkable results in recognition accuracy, etc., and related technical achievements have attracted worldwide attention. Due to the lack of prior knowledge of face images, large illumination changes, complex backgrounds, and variable face angles, the demand for face images is large, expression changes are large, and face occlusion leads to low accuracy of face recognition.
Deep learning applications often use convolutional neural networks to achieve image processing and recognition with high efficiency and accuracy [14,15,16,17]. Facial images are highly structured images. Combining with prior facial knowledge is a very popular method in face recognition.
Dong et al. proposed an image super-resolution method based on deep convolutional neural network [18], which realizes the mapping from the low-resolution end to the high-resolution end of the image, and extends the traditional super-resolution method based on coding coefficients. Since then, the research of neural network combined with image super-resolution has continued to deepen. Kim et al. [19] proposed the use of very deep convolutional neural network on the basis of VGG network to improve the super-resolution accuracy by using multiple filters in the neural network, and realize the use of image context information.
Yu et al. proposed a transforming and distinguishing neural network [20] for the serious problems of multi-posture and degradation, and solving the problem of multi-posture and image misalignment. As the depth of the network increases, the features gradually disappear during the transmission process. In response to this problem, the multi-scale residual network image super-resolution (MSRN) algorithm [21] uses the combination of local multi-scale features and global features to maximize utilizing the features of low-resolution images; the problem of feature disappearance during transmission is solved. There are various existing algorithms and deep learning models [38,39,40] in which not only can they facilitate face recognition, but also perform human activity recognition and motion prediction.
In this paper, we propose a face detection and recognition model based on multi-task convolutional neural network (MTCNN) [22,23,24]. The recognition model combined with deep learning has a high accuracy rate, and has a shorter recognition time, which can reduce the waste of human resources.
MTCNN implements the face area detection and face key point detection together, and its subject framework is similar to cascade. The whole can be divided into a three-layer network structure of Proposal Network (P-Net), Refine Network (R-Net), and Output Network (O-Net) [25,26]. It is a multi-task neural network model for face detection tasks which mainly uses three cascaded networks and the idea of candidate boxes plus classifiers.
The three cascaded networks are P-Net for quickly generating candidate windows, R-Net for filtering and selecting high-precision candidate windows, and O-Net for generating final bounding boxes and face key points. And many convolutional neural network models that deal with image problems; the model also uses image pyramids, border regression, non-maximum suppression and other technologies. The network structure of P-Net, R-Net and O-Net is shown in Figure 1.
To balance computational expenses and performance, MTCNN avoids the huge performance consumption caused by traditional ideas such as sliding windows and classifiers, we first use a small model to generate a candidate frame for the target area with a certain possibility, and then use a more complex model for fine classification. Then we return the higher-precision area box, and let this step be executed recursively. The network structure of MTCNN is presented by Figure 2.
R-CNN [27] draws on the idea of sliding window and adopts the scheme of area recognition. The specific identification scheme is the first step. Given an input image, extract 2000 independent candidate regions from the image; the second step is to use CNN to extract a fixed-length feature vector for each region; the third step, we use SVM to classify each area. Figure 3 shows the process of R-CNN in the recognition of faces.
Faster R-CNN [28,29] creatively uses the convolutional network to generate the suggestion frame by itself, and shares the convolutional network with the target detection network, so that the number of suggestion frames is reduced from about 2000 to 300. This framework can even be combined with nonlinear anisotropic diffusion filtering and other morphological methods to perform denoising of image. To ensure excellent face recognition, the noise and the other unrelated background areas can be removed. The process of Faster R-CNN to recognize faces is shown in Figure 4.
The commonly used kernel functions [30] include linear kernel functions [31,32], Gaussian kernel functions [33,34], and other similar algorithms. One of the most commonly used is the Gaussian kernel function, which can map feature data to infinite dimensions.
The linear kernel function is mainly used in the case of linear separability, which can achieve a good classification effect. The mathematical expression of the linear kernel function is shown in Eq (1). There are few parameters in the function, so the computation rate is very fast, and the dimension of its input space is the same as the dimension of the feature space, which is suitable for the first attempt in the classification task.
$ K\left(X, Y\right) = \left({X}^{T}Y+C\right) $ | (1) |
In Eq (1), X and Y represent eigenvectors, and C represents a constant.
The Gaussian kernel function can map the sample from the input space to the higher-dimensional feature space, and it can achieve good results regardless of the sample size. When the classification task cannot determine which kernel function to use, the Gaussian kernel function is the most widely used one of the kernel functions. The mathematical expression of the Gaussian kernel function is shown in Eq (2).
$ K\left(X, Y\right) = exp\left(-\frac{{‖X-Y‖}^{2}}{2{\sigma }^{2}}\right) $ | (2) |
In particular, σ in Eq (2) is the width parameter of the function, which controls the radial range of the function.
We collect 2500 face images for our face recognition research in this paper. During the test, the data set is divided into the original image data set and the test data set according to the parity position. The two images at the corresponding positions of the two data sets are the comparison objects, as shown in Figure 5. Then, we apply the algorithm of this paper to these two data sets, and extract the images out in turn. Table 1 show the gender ratio and picture size of the two data sets.
Characteristics | Original image data set | Test data set |
Male: Female | 10: 15 | 13: 12 |
Image size | 1897×1897 | 1897×1897 |
The high-resolution face image reconstructed after the algorithm processing requires a certain measurement standard to examine the performance of the algorithm. The earlier evaluation standard was subjective evaluation through naked eye observation. This method is simple and direct. The objective evaluation calculates the similarity between the synthesized image and the original image, and has a specific value to measure the reconstruction result of the image. Compared with the subjective evaluation, its advantage is that the comparison result is more concise and accurate. At present, the commonly used objective evaluation methods include Peak Signal-to-Noise Ratio (PSNR) and Structural Similarity Index Measurement (SSIM) [35,36].
PSNR is an image quality evaluation method based on the error between corresponding pixels. It is the most common image quality evaluation index, and its expression is as Eq (3).
$ PSNR = 10{\mathrm{log}}_{10}\left(\frac{{\left({2}^{b}-1\right)}^{2}}{MSE}\right) $ | (3) |
In Eq (3), b is the number of bits of the pixel, which is usually 8. The unit of PSNR is decibel (dB). The larger the value means higher quality of the reconstructed image. Mean Square Error (MSE) represents the mean square error between images. The symbol h represents the height of the image, and w represents the width of the image. The expression is shown in Eq (4).
$ MSE = \frac{1}{h\times w}\sum _{i = 1}^{h}\sum _{j = 1}^{w}{\left[{X}_{1}\left(i, j\right)-{X}_{2}\left(i, j\right)\right]}^{2} $ | (4) |
However, because the human perception of an area will be affected by the surrounding area and other reasons, this method does not take into account the human visual characteristics, but only calculates the difference between pixels, so the result of the peak signal-to-noise ratio evaluation method will appear to be different from that of humans.
The value of SSIM is a value between 0 and 1. The larger the value of SSIM, explant the smaller the difference between both photos of the faces. Therefore, the larger the value of SSIM replies the better the image reconstruction quality. The SSIM method measures image similarity from three aspects: brightness (L), contrast (C), and structure (S). The expression of SSIM is shown in Eq (5).
$ SSIM\left(X, Y\right) = L\left(X, Y\right)\times C\left(X, Y\right)\times S\left(X, Y\right) $ | (5) |
SSIM simulates the human perception of changes in image information, uses the image average to model the image brightness, the image standard deviation to model the image contrast, and the image covariance to model the image structure, which makes up for the shortcomings of the PSNR method.
Receiver operating characteristic (ROC) curve is a comprehensive indicator that reflects the sensitivity and specificity of continuous variables. Each point on the ROC curve reflects the susceptibility to the same signal stimulus.
The abscissa is false positive rate (FPR), that is, the proportion of all negative samples that are predicted to be positive but actually negative. The larger the FPR, the positive prediction the more negative classes in the class. The computational FPR is as Eq (6).
$ FPR = \frac{FP}{TN+FN} $ | (6) |
The ordinate is true positive rate (TPR), the proportion of all positive samples that are predicted to be positive and actually positive. The larger the TPR stands the more actual positive classes in the predicted positive class. The computational TPR is as Eq (7).
$ \mathrm{T}\mathrm{P}\mathrm{R} = \frac{TP}{TP+FP} $ | (7) |
In Eqs (6) and (7), True Positive (TP), the prediction is a positive sample and the actual number of features is also a positive sample. False Positive (FP), the number of features predicted to be a positive sample and actually a negative sample. True Negative (TN) is predicted to be a negative sample and is actually the number of features of a negative sample. False Negative (FN), the number of features predicted to be negative samples and actually positive samples.
In order to better recognize the face image, this paper implements the Gaussian kernel function as the mapping function in the model. In order to achieve the best results, we need to adjust three parameters, which are the local constraint parameter λ, the kernel function similarity parameter σ, and the high resolution layer constraint parameter k.
We fix the value of k and the kernel function similarity parameter σ, and set the value range of λ to be 0.01 to 0.12. The mean value of PSNR and mean value of SSIM as the evaluation criteria change with the value of λ as shown in Figure 6(a), (b). As shown in the figure, when λ = 0.08, the average PSNR of MTCNN and Faster R-CNN is the largest, and when λ = 0.08, the average PSNR of R-CNN is the largest. The average value of SSIM reaches its peak. Since the difference between the two values is very small, the value of λ is selected here as 0.08.
We fix the values of k and λ, and set the value of the kernel function similarity parameter σ from 200 to 1000. The average value of PSNR and the average value of SSIM as the evaluation criteria change with the value of σ as shown in Figure 6(c), (d). We find that when the σ value is 400, the average PSNR of the three models reaches the maximum, while the average SSIM reaches the peak at σ = 400 and 500. Combining the two objective evaluation criteria, we choose σ = 400.
We set the values of λ and the kernel function similarity parameter σ, and set the value of the high-resolution layer error term parameter k to 0.01 to 0.10. As the evaluation criteria, the mean value of PSNR and mean value of SSIM change with the value of k as shown in Figure 6(e), (f). It can be clearly seen from the following line chart that both the mean PSNR and the mean SSIM peak when k takes 0.05, so we determine the optimal value of k to be 0.05.
From the optimization parameters, we know that when our parameters are set to λ as 0.08, σ as 400 and k as 0.05, the three models are in the optimal state. Table 2 shows the average values of PSNR and SSIM of the three models.
Model | PSNR (dB) | SSIM |
MTCNN | 36.245 | 0.954 |
R-CNN | 35.005 | 0.927 |
Faster R-CNN | 35.305 | 0.938 |
From Table 2, the mean PSNR and SSIM pertaining to the MTCNN are better than R-CNN and Faster R-CNN. The average PSNR of our method is 1.24 dB higher than that of R-CNN and 0.94 dB higher than that of Faster R-CNN. The average SSIM of MTCNN is 10.3% higher than R-CNN and 8.7% higher than Faster R-CNN.
Figure 7 shows the ROC curves of MTCNN, R-CNN and Faster R-CNN. The Area Under Curve (AUC) of MTCNN is 97.56%, the AUC of R-CNN is 91.24%, and the AUC of Faster R-CNN is 92.01%. MTCNN has the best overall face recognition performance. For detection of faces, MTCNN still has the best effect.
In actual scenes, the acquired face images are usually of poor resolution and low-quality, which is caused by a variety of reasons: first, the location of the surveillance camera is high, the shooting range is large, and the target face image is small; second, the monitoring The device is limited by storage space and highly compresses video images, so the image loses detailed information; third, external environments such as rainy weather and poor lighting will further reduce the quality of the captured images. In response to these problems, the face recognition technology combined with convolutional neural network realizes its practical application value.
Although this paper has conducted considerable research worthy of investigation, and performed exploration on learning-based image classification methods, there are still significant limitations that need to be addressed. Despite the sound conclusion of this research in this field, there are still some issues worthy of attention and future implementation of other relevant networks is required for a thorough analysis.
With the continuous deepening of research on convolutional neural networks, vector-based convolution and pooling processing have been fully studied. In fact, in a network, we can apply the Riemannian manifold geometry to the data in the middle layer for processing. This pooling and iterative process in the form of a matrix can have a positive effect on the final output of the network.
MTCNN mainly uses three cascaded networks, and uses the idea of candidate box plus classifier to execute fast and efficient face recognition. Among the three evaluation indicators, MTCNN has the best overall face recognition performance, and for defective faces, MTCNN still has the best effect as well as performance.
The technology based on MTCNN has a good development prospect, which greatly improves the accuracy of face recognition. While improving accuracy, it also improves the security of the image recognition system.
The authors declare no conflict of interest.
This research is funded by the National Natural Science Foundation of China (No. 62006102).
[1] |
Ganser-Pornillos BK, Yeager M, Pornillos O (2012) Assembly and architecture of HIV. Adv Exp Med Biol 726: 441-465. doi: 10.1007/978-1-4614-0980-9_20
![]() |
[2] | Sundquist WI, Krausslich HG (2012) HIV-1 assembly, budding, and maturation. Cold Spring Harb Perspect Med 2: a006924. |
[3] | Borggren M, Jansson M (2015) The Evolution of HIV-1 Interactions with Coreceptors and Mannose C-Type Lectin Receptors. Prog Mol Biol Transl Sci 129C: 109-140. |
[4] |
Miyauchi K, Kim Y, Latinovic O, et al. (2009) HIV enters cells via endocytosis and dynamin-dependent fusion with endosomes. Cell 137: 433-444. doi: 10.1016/j.cell.2009.02.046
![]() |
[5] |
Moulard M, Decroly E (2000) Maturation of HIV envelope glycoprotein precursors by cellular endoproteases. Biochim Biophys Acta 1469: 121-132. doi: 10.1016/S0304-4157(00)00014-9
![]() |
[6] |
Low JT, Garcia-Miranda P, Mouzakis KD, et al. (2014) Structure and dynamics of the HIV-1 frameshift element RNA. Biochemistry 53: 4282-4291. doi: 10.1021/bi5004926
![]() |
[7] |
Stephenson JD, Li H, Kenyon JC, et al. (2013) Three-dimensional RNA structure of the major HIV-1 packaging signal region. Structure 21: 951-962. doi: 10.1016/j.str.2013.04.008
![]() |
[8] |
Watts JM, Dang KK, Gorelick RJ, et al. (2009) Architecture and secondary structure of an entire HIV-1 RNA genome. Nature 460: 711-716. doi: 10.1038/nature08237
![]() |
[9] | Swanstrom R, Wills JW (1997) Synthesis, Assembly, and Processing of Viral Proteins. In: Coffin JM HS, Varmus HE, editor. Retroviruses. Cold Spring Harbor, New York: Cold Spring Harbor Laboratory Press. |
[10] |
Checkley MA, Luttge BG, Freed EO (2011) HIV-1 envelope glycoprotein biosynthesis, trafficking, and incorporation. J Mol Biol 410: 582-608. doi: 10.1016/j.jmb.2011.04.042
![]() |
[11] |
Postler TS, Desrosiers RC (2013) The tale of the long tail: the cytoplasmic domain of HIV-1 gp41. J Virol 87: 2-15. doi: 10.1128/JVI.02053-12
![]() |
[12] |
Bharat TA, Davey NE, Ulbrich P, et al. (2012) Structure of the immature retroviral capsid at 8 A resolution by cryo-electron microscopy. Nature 487: 385-389. doi: 10.1038/nature11169
![]() |
[13] | Maldonado JO, Martin JL, Mueller JD, et al. (2014) New insights into retroviral Gag-Gag and Gag-membrane interactions. Front Microbiol 5: 302. |
[14] | Sonnino S, Prinetti A (2013) Membrane domains and the “lipid raft” concept. Curr Med Chem 20: 4-21. |
[15] |
Levin JG, Mitra M, Mascarenhas A, et al. (2010) Role of HIV-1 nucleocapsid protein in HIV-1 reverse transcription. RNA Biol 7: 754-774. doi: 10.4161/rna.7.6.14115
![]() |
[16] |
Jiang J, Aiken C (2007) Maturation-dependent human immunodeficiency virus type 1 particle fusion requires a carboxyl-terminal region of the gp41 cytoplasmic tail. J Virol 81: 9999-10008. doi: 10.1128/JVI.00592-07
![]() |
[17] |
Wyma DJ, Jiang J, Shi J, et al. (2004) Coupling of human immunodeficiency virus type 1 fusion to virion maturation: a novel role of the gp41 cytoplasmic tail. J Virol 78: 3429-3435. doi: 10.1128/JVI.78.7.3429-3435.2004
![]() |
[18] | Aiken C, Zhang P (2013) HIV-1 Maturation. In: Freed EO, editor. Advances in HIV-1 Assembly and Release: Springer. |
[19] |
Forshey BM, von Schwedler U, Sundquist WI, et al. (2002) Formation of a human immunodeficiency virus type 1 core of optimal stability is crucial for viral replication. J Virol 76: 5667-5677. doi: 10.1128/JVI.76.11.5667-5677.2002
![]() |
[20] |
Ganser-Pornillos BK, Yeager M, Sundquist WI (2008) The structural biology of HIV assembly. Curr Opin Struct Biol 18: 203-217. doi: 10.1016/j.sbi.2008.02.001
![]() |
[21] |
Bush DL, Vogt VM (2014) In Vitro Assembly of Retroviruses. Annual Reviews in Virology 1: 561-580. doi: 10.1146/annurev-virology-031413-085427
![]() |
[22] |
Briggs JA (2013) Structural biology in situ--the potential of subtomogram averaging. Curr Opin Struct Biol 23: 261-267. doi: 10.1016/j.sbi.2013.02.003
![]() |
[23] |
Merk A, Subramaniam S (2013) HIV-1 envelope glycoprotein structure. Curr Opin Struct Biol 23: 268-276. doi: 10.1016/j.sbi.2013.03.007
![]() |
[24] |
Briggs JA, Simon MN, Gross I, et al. (2004) The stoichiometry of Gag protein in HIV-1. Nat Struct Mol Biol 11: 672-675. doi: 10.1038/nsmb785
![]() |
[25] |
Butan C, Winkler DC, Heymann JB, et al. (2008) RSV capsid polymorphism correlates with polymerization efficiency and envelope glycoprotein content: implications that nucleation controls morphogenesis. J Mol Biol 376: 1168-1181. doi: 10.1016/j.jmb.2007.12.003
![]() |
[26] |
Fuller SD, Wilk T, Gowen BE, et al. (1997) Cryo-electron microscopy reveals ordered domains in the immature HIV-1 particle. Curr Biol 7: 729-738. doi: 10.1016/S0960-9822(06)00331-9
![]() |
[27] |
Kingston RL, Olson NH, Vogt VM (2001) The organization of mature Rous sarcoma virus as studied by cryoelectron microscopy. J Struct Biol 136: 67-80. doi: 10.1006/jsbi.2001.4423
![]() |
[28] |
Yeager M, Wilson-Kubalek EM, Weiner SG, et al. (1998) Supramolecular organization of immature and mature murine leukemia virus revealed by electron cryo-microscopy: implications for retroviral assembly mechanisms. Proc Natl Acad Sci U S A 95: 7299-7304. doi: 10.1073/pnas.95.13.7299
![]() |
[29] |
Carlson LA, Briggs JA, Glass B, et al. (2008) Three-dimensional analysis of budding sites and released virus suggests a revised model for HIV-1 morphogenesis. Cell Host Microbe 4: 592-599. doi: 10.1016/j.chom.2008.10.013
![]() |
[30] |
Briggs JA, Johnson MC, Simon MN, et al. (2006) Cryo-electron microscopy reveals conserved and divergent features of gag packing in immature particles of Rous sarcoma virus and human immunodeficiency virus. J Mol Biol 355: 157-168. doi: 10.1016/j.jmb.2005.10.025
![]() |
[31] |
Briggs JA, Riches JD, Glass B, et al. (2009) Structure and assembly of immature HIV. Proc Natl Acad Sci U S A 106: 11090-11095. doi: 10.1073/pnas.0903535106
![]() |
[32] |
Keller PW, Huang RK, England MR, et al. (2013) A two-pronged structural analysis of retroviral maturation indicates that core formation proceeds by a disassembly-reassembly pathway rather than a displacive transition. J Virol 87: 13655-13664. doi: 10.1128/JVI.01408-13
![]() |
[33] |
Wright ER, Schooler JB, Ding HJ, et al. (2007) Electron cryotomography of immature HIV-1 virions reveals the structure of the CA and SP1 Gag shells. EMBO J 26: 2218-2226. doi: 10.1038/sj.emboj.7601664
![]() |
[34] |
de Marco A, Davey NE, Ulbrich P, et al. (2010) Conserved and variable features of Gag structure and arrangement in immature retrovirus particles. J Virol 84: 11729-11736. doi: 10.1128/JVI.01423-10
![]() |
[35] |
Datta SA, Temeselew LG, Crist RM, et al. (2011) On the role of the SP1 domain in HIV-1 particle assembly: a molecular switch? J Virol 85: 4111-4121. doi: 10.1128/JVI.00006-11
![]() |
[36] |
Liang C, Hu J, Russell RS, et al. (2002) Characterization of a putative alpha-helix across the capsid-SP1 boundary that is critical for the multimerization of human immunodeficiency virus type 1 gag. J Virol 76: 11729-11737. doi: 10.1128/JVI.76.22.11729-11737.2002
![]() |
[37] |
Briggs JA, Wilk T, Welker R, et al. (2003) Structural organization of authentic, mature HIV-1 virions and cores. EMBO J 22: 1707-1715. doi: 10.1093/emboj/cdg143
![]() |
[38] |
Ganser BK, Li S, Klishko VY, et al. (1999) Assembly and analysis of conical models for the HIV-1 core. Science 283: 80-83. doi: 10.1126/science.283.5398.80
![]() |
[39] |
Heymann JB, Butan C, Winkler DC, et al. (2008) Irregular and Semi-Regular Polyhedral Models for Rous Sarcoma Virus Cores. Comput Math Methods Med 9: 197-210. doi: 10.1080/17486700802168106
![]() |
[40] |
Cao S, Maldonado JO, Grigsby IF, et al. (2015) Analysis of human T-cell leukemia virus type 1 particles using cryo-electron tomography. J Virol 89: 2430-2435. doi: 10.1128/JVI.02358-14
![]() |
[41] |
Li S, Hill CP, Sundquist WI, et al. (2000) Image reconstructions of helical assemblies of the HIV-1 CA protein. Nature 407: 409-413. doi: 10.1038/35030177
![]() |
[42] |
Thomas D, Schultz P, Steven AC, et al. (1994) Mass analysis of biological macromolecular complexes by STEM. Biol Cell 80: 181-192. doi: 10.1111/j.1768-322X.1994.tb00929.x
![]() |
[43] |
Fogarty KH, Zhang W, Grigsby IF, et al. (2011) New insights into HTLV-1 particle structure, assembly, and Gag-Gag interactions in living cells. Viruses 3: 770-793. doi: 10.3390/v3060770
![]() |
[44] |
Johnson J, Chen Y, Mueller JD (2010) Characterization of brightness and stoichiometry of bright particles by flow-fluorescence fluctuation spectroscopy. Biophys J 99: 3084-3092. doi: 10.1016/j.bpj.2010.08.057
![]() |
[45] |
Parker SD, Wall JS, Hunter E (2001) Analysis of Mason-Pfizer monkey virus Gag particles by scanning transmission electron microscopy. J Virol 75: 9543-9548. doi: 10.1128/JVI.75.19.9543-9548.2001
![]() |
[46] | Vogt VM, Simon MN (1999) Mass determination of rous sarcoma virus virions by scanning transmission electron microscopy. J Virol 73: 7050-7055. |
[47] |
Yu F, Joshi SM, Ma YM, et al. (2001) Characterization of Rous sarcoma virus Gag particles assembled in vitro. J Virol 75: 2753-2764. doi: 10.1128/JVI.75.6.2753-2764.2001
![]() |
[48] |
Chen Y, Wu B, Musier-Forsyth K, et al. (2009) Fluorescence fluctuation spectroscopy on viral-like particles reveals variable gag stoichiometry. Biophys J 96: 1961-1969. doi: 10.1016/j.bpj.2008.10.067
![]() |
[49] |
Grigsby IF, Zhang W, Johnson JL, et al. (2010) Biophysical analysis of HTLV-1 particles reveals novel insights into particle morphology and Gag stochiometry. Retrovirology 7: 75. doi: 10.1186/1742-4690-7-75
![]() |
[50] |
Lanman J, Lam TT, Emmett MR, et al. (2004) Key interactions in HIV-1 maturation identified by hydrogen-deuterium exchange. Nat Struct Mol Biol 11: 676-677. doi: 10.1038/nsmb790
![]() |
[51] |
Briggs JA, Watson BE, Gowen BE, et al. (2004) Cryoelectron microscopy of mouse mammary tumor virus. J Virol 78: 2606-2608. doi: 10.1128/JVI.78.5.2606-2608.2004
![]() |
[52] |
Forster F, Medalia O, Zauberman N, et al. (2005) Retrovirus envelope protein complex structure in situ studied by cryo-electron tomography. Proc Natl Acad Sci U S A 102: 4729-4734. doi: 10.1073/pnas.0409178102
![]() |
[53] |
Briggs JA, Grunewald K, Glass B, et al. (2006) The mechanism of HIV-1 core assembly: insights from three-dimensional reconstructions of authentic virions. Structure 14: 15-20. doi: 10.1016/j.str.2005.09.010
![]() |
[54] |
Hamann MV, Mullers E, Reh J, et al. (2014) The cooperative function of arginine residues in the Prototype Foamy Virus Gag C-terminus mediates viral and cellular RNA encapsidation. Retrovirology 11: 87. doi: 10.1186/s12977-014-0087-7
![]() |
[55] |
Roux KH, Taylor KA (2007) AIDS virus envelope spike structure. Curr Opin Struct Biol 17: 244-252. doi: 10.1016/j.sbi.2007.03.008
![]() |
[56] |
Liu J, Bartesaghi A, Borgnia MJ, et al. (2008) Molecular architecture of native HIV-1 gp120 trimers. Nature 455: 109-113. doi: 10.1038/nature07159
![]() |
[57] |
Zanetti G, Briggs JA, Grunewald K, et al. (2006) Cryo-electron tomographic structure of an immunodeficiency virus envelope complex in situ. PLoS Pathog 2: e83. doi: 10.1371/journal.ppat.0020083
![]() |
[58] |
Harris A, Borgnia MJ, Shi D, et al. (2011) Trimeric HIV-1 glycoprotein gp140 immunogens and native HIV-1 envelope glycoproteins display the same closed and open quaternary molecular architectures. Proc Natl Acad Sci U S A 108: 11440-11445. doi: 10.1073/pnas.1101414108
![]() |
[59] |
Meyerson JR, Tran EE, Kuybeda O, et al. (2013) Molecular structures of trimeric HIV-1 Env in complex with small antibody derivatives. Proc Natl Acad Sci U S A 110: 513-518. doi: 10.1073/pnas.1214810110
![]() |
[60] |
Tran EE, Borgnia MJ, Kuybeda O, et al. (2012) Structural mechanism of trimeric HIV-1 envelope glycoprotein activation. PLoS Pathog 8: e1002797. doi: 10.1371/journal.ppat.1002797
![]() |
[61] |
White TA, Bartesaghi A, Borgnia MJ, et al. (2011) Three-dimensional structures of soluble CD4-bound states of trimeric simian immunodeficiency virus envelope glycoproteins determined by using cryo-electron tomography. J Virol 85: 12114-12123. doi: 10.1128/JVI.05297-11
![]() |
[62] |
White TA, Bartesaghi A, Borgnia MJ, et al. (2010) Molecular architectures of trimeric SIV and HIV-1 envelope glycoproteins on intact viruses: strain-dependent variation in quaternary structure. PLoS Pathog 6: e1001249. doi: 10.1371/journal.ppat.1001249
![]() |
[63] |
Loving R, Wu SR, Sjoberg M, et al. (2012) Maturation cleavage of the murine leukemia virus Env precursor separates the transmembrane subunits to prime it for receptor triggering. Proc Natl Acad Sci U S A 109: 7735-7740. doi: 10.1073/pnas.1118125109
![]() |
[64] |
Sjoberg M, Wu SR, Loving R, et al. (2014) Furin cleavage of the Moloney murine leukemia virus Env precursor reorganizes the spike structure. Proc Natl Acad Sci U S A 111: 6034-6039. doi: 10.1073/pnas.1317972111
![]() |
[65] |
Wu SR, Sjoberg M, Wallin M, et al. (2008) Turning of the receptor-binding domains opens up the murine leukaemia virus Env for membrane fusion. EMBO J 27: 2799-2808. doi: 10.1038/emboj.2008.187
![]() |
[66] |
Zhu P, Liu J, Bess J, Jr., et al. (2006) Distribution and three-dimensional structure of AIDS virus envelope spikes. Nature 441: 847-852. doi: 10.1038/nature04817
![]() |
[67] | Schur FK, Hagen WJ, Rumlova M, et al. (2015) Structure of the immature HIV-1 capsid in intact virus particles at 8.8 A resolution. . Nature 517: 505-508. |
[68] |
Pettersen EF, Goddard TD, Huang CC, et al. (2004) UCSF Chimera--a visualization system for exploratory research and analysis. J Comput Chem 25: 1605-1612. doi: 10.1002/jcc.20084
![]() |
[69] |
Zhao G, Perilla JR, Yufenyuy EL, et al. (2013) Mature HIV-1 capsid structure by cryo-electron microscopy and all-atom molecular dynamics. Nature 497: 643-646. doi: 10.1038/nature12162
![]() |
[70] |
Cardone G, Purdy JG, Cheng N, et al. (2009) Visualization of a missing link in retrovirus capsid assembly. Nature 457: 694-698. doi: 10.1038/nature07724
![]() |
[71] |
Hyun JK, Radjainia M, Kingston RL, et al. (2010) Proton-driven assembly of the Rous Sarcoma virus capsid protein results in the formation of icosahedral particles. J Biol Chem 285: 15056-15064. doi: 10.1074/jbc.M110.108209
![]() |
[72] |
Ganser-Pornillos BK, Cheng A, Yeager M (2007) Structure of full-length HIV-1 CA: a model for the mature capsid lattice. Cell 131: 70-79. doi: 10.1016/j.cell.2007.08.018
![]() |
[73] |
Bartesaghi A, Merk A, Borgnia MJ, et al. (2013) Prefusion structure of trimeric HIV-1 envelope glycoprotein determined by cryo-electron microscopy. Nat Struct Mol Biol 20: 1352-1357. doi: 10.1038/nsmb.2711
![]() |
[74] |
Lyumkis D, Julien JP, de Val N, et al. (2013) Cryo-EM structure of a fully glycosylated soluble cleaved HIV-1 envelope trimer. Science 342: 1484-1490. doi: 10.1126/science.1245627
![]() |
[75] |
Bartesaghi A, Lecumberry F, Sapiro G, et al. (2012) Protein secondary structure determination by constrained single-particle cryo-electron tomography. Structure 20: 2003-2013. doi: 10.1016/j.str.2012.10.016
![]() |
[76] | Schur FK, Hagen WJ, de Marco A, et al. (2013) Determination of protein structure at 8.5A resolution using cryo-electron tomography and sub-tomogram averaging. . J Struct Biol 184: 394-400. |
[77] | Tang C, Ndassa Y, Summers MF (2002) Structure of the N-terminal 283-residue fragment of the immature HIV-1 Gag polyprotein. Nat Struct Biol 9: 537-543. |
[78] |
Pornillos O, Ganser-Pornillos BK, Kelly BN, et al. (2009) X-ray structures of the hexameric building block of the HIV capsid. Cell 137: 1282-1292. doi: 10.1016/j.cell.2009.04.063
![]() |
[79] |
Macek P, Chmelik J, Krizova I, et al. (2009) NMR structure of the N-terminal domain of capsid protein from the mason-pfizer monkey virus. J Mol Biol 392: 100-114. doi: 10.1016/j.jmb.2009.06.029
![]() |
[80] |
Bharat TA, Castillo Menendez LR, Hagen WJ, et al. (2014) Cryo-electron microscopy of tubular arrays of HIV-1 Gag resolves structures essential for immature virus assembly. Proc Natl Acad Sci U S A 111: 8233-8238. doi: 10.1073/pnas.1401455111
![]() |
[81] |
Mao Y, Wang L, Gu C, et al. (2012) Subunit organization of the membrane-bound HIV-1 envelope glycoprotein trimer. Nat Struct Mol Biol 19: 893-899. doi: 10.1038/nsmb.2351
![]() |
[82] |
Mao Y, Wang L, Gu C, et al. (2013) Molecular architecture of the uncleaved HIV-1 envelope glycoprotein trimer. Proc Natl Acad Sci U S A 110: 12438-12443. doi: 10.1073/pnas.1307382110
![]() |
[83] | Cohen J Structural biology. Is high-tech view of HIV too good to be true? Science 341: 443-444. |
[84] | Henderson R Avoiding the pitfalls of single particle cryo-electron microscopy: Einstein from noise. Proc Natl Acad Sci U S A 110: 18037-18041. |
[85] | Mao Y, Castillo-Menendez LR, Sodroski JG Reply to Subramaniam, van Heel, and Henderson: Validity of the cryo-electron microscopy structures of the HIV-1 envelope glycoprotein complex. Proc Natl Acad Sci U S A 110: E4178-4182. |
[86] |
Frank GA, Narayan K, Bess JW, Jr., et al. (2015) Maturation of the HIV-1 core by a non-diffusional phase transition. Nat Commun 6: 5854. doi: 10.1038/ncomms6854
![]() |
[87] | van Heel M Finding trimeric HIV-1 envelope glycoproteins in random noise. Proc Natl Acad Sci U S A 110: E4175-4177. |
[88] | Mammano F, Ohagen A, Hoglund S, et al. (1994) Role of the major homology region of human immunodeficiency virus type 1 in virion morphogenesis. J Virol 68: 4927-4936. |
[89] |
von Schwedler UK, Stray KM, Garrus JE, et al. (2003) Functional surfaces of the human immunodeficiency virus type 1 capsid protein. J Virol 77: 5439-5450. doi: 10.1128/JVI.77.9.5439-5450.2003
![]() |
[90] |
Chu HH, Chang YF, Wang CT (2006) Mutations in the alpha-helix directly C-terminal to the major homology region of human immunodeficiency virus type 1 capsid protein disrupt Gag multimerization and markedly impair virus particle production. J Biomed Sci 13: 645-656. doi: 10.1007/s11373-006-9094-6
![]() |
[91] |
Gamble TR, Yoo S, Vajdos FF, et al. (1997) Structure of the carboxyl-terminal dimerization domain of the HIV-1 capsid protein. Science 278: 849-853. doi: 10.1126/science.278.5339.849
![]() |
[92] |
Ono A, Ablan SD, Lockett SJ, et al. (2004) Phosphatidylinositol (4,5) bisphosphate regulates HIV-1 Gag targeting to the plasma membrane. Proc Natl Acad Sci U S A 101: 14889-14894. doi: 10.1073/pnas.0405596101
![]() |
[93] |
Saad JS, Miller J, Tai J, et al. (2006) Structural basis for targeting HIV-1 Gag proteins to the plasma membrane for virus assembly. Proc Natl Acad Sci U S A 103: 11364-11369. doi: 10.1073/pnas.0602818103
![]() |
[94] |
Fogarty KH, Chen Y, Grigsby IF, et al. (2011) Characterization of cytoplasmic Gag-gag interactions by dual-color z-scan fluorescence fluctuation spectroscopy. Biophys J 100: 1587-1595. doi: 10.1016/j.bpj.2011.02.008
![]() |
[95] |
Lindwasser OW, Resh MD (2004) Human immunodeficiency virus type 1 Gag contains a dileucine-like motif that regulates association with multivesicular bodies. J Virol 78: 6013-6023. doi: 10.1128/JVI.78.11.6013-6023.2004
![]() |
[96] |
Muriaux D, Mirro J, Harvin D, et al. (2001) RNA is a structural element in retrovirus particles. Proc Natl Acad Sci U S A 98: 5246-5251. doi: 10.1073/pnas.091000398
![]() |
[97] |
Poole E, Strappe P, Mok HP, et al. (2005) HIV-1 Gag-RNA interaction occurs at a perinuclear/centrosomal site; analysis by confocal microscopy and FRET. Traffic 6: 741-755. doi: 10.1111/j.1600-0854.2005.00312.x
![]() |
[98] |
Bailey GD, Hyun JK, Mitra AK, et al. (2012) A structural model for the generation of continuous curvature on the surface of a retroviral capsid. J Mol Biol 417: 212-223. doi: 10.1016/j.jmb.2012.01.014
![]() |
[99] |
Mortuza GB, Haire LF, Stevens A, et al. (2004) High-resolution structure of a retroviral capsid hexameric amino-terminal domain. Nature 431: 481-485. doi: 10.1038/nature02915
![]() |
[100] |
Byeon IJ, Meng X, Jung J, et al. (2009) Structural convergence between Cryo-EM and NMR reveals intersubunit interactions critical for HIV-1 capsid function. Cell 139: 780-790. doi: 10.1016/j.cell.2009.10.010
![]() |
[101] |
Yang H, Ji X, Zhao G, et al. (2012) Structural insight into HIV-1 capsid recognition by rhesus TRIM5alpha. Proc Natl Acad Sci U S A 109: 18372-18377. doi: 10.1073/pnas.1210903109
![]() |
[102] |
Zhao G, Ke D, Vu T, et al. (2011) Rhesus TRIM5alpha disrupts the HIV-1 capsid at the inter-hexamer interfaces. PLoS Pathog 7: e1002009. doi: 10.1371/journal.ppat.1002009
![]() |
[103] | Gres AT, Kirby KA, KewalRamani VN, et al. (2015) X-ray crystal structures of native HIV-1 capsid protein reveal conformational variability. Science. |
[104] |
Dalgleish AG, Beverley PC, Clapham PR, et al. (1984) The CD4 (T4) antigen is an essential component of the receptor for the AIDS retrovirus. Nature 312: 763-767. doi: 10.1038/312763a0
![]() |
[105] |
Trkola A, Dragic T, Arthos J, et al. (1996) CD4-dependent, antibody-sensitive interactions between HIV-1 and its co-receptor CCR-5. Nature 384: 184-187. doi: 10.1038/384184a0
![]() |
[106] |
Wyatt R, Sodroski J (1998) The HIV-1 envelope glycoproteins: fusogens, antigens, and immunogens. Science 280: 1884-1888. doi: 10.1126/science.280.5371.1884
![]() |
[107] |
Buzon V, Natrajan G, Schibli D, et al. (2010) Crystal structure of HIV-1 gp41 including both fusion peptide and membrane proximal external regions. PLoS Pathog 6: e1000880. doi: 10.1371/journal.ppat.1000880
![]() |
[108] |
Chan DC, Fass D, Berger JM, et al. (1997) Core structure of gp41 from the HIV envelope glycoprotein. Cell 89: 263-273. doi: 10.1016/S0092-8674(00)80205-6
![]() |
[109] |
Tan K, Liu J, Wang J, et al. (1997) Atomic structure of a thermostable subdomain of HIV-1 gp41. Proc Natl Acad Sci U S A 94: 12303-12308. doi: 10.1073/pnas.94.23.12303
![]() |
[110] |
Weissenhorn W, Dessen A, Harrison SC, et al. (1997) Atomic structure of the ectodomain from HIV-1 gp41. Nature 387: 426-430. doi: 10.1038/387426a0
![]() |
[111] |
Chen L, Kwon YD, Zhou T, et al. (2009) Structural basis of immune evasion at the site of CD4 attachment on HIV-1 gp120. Science 326: 1123-1127. doi: 10.1126/science.1175868
![]() |
[112] |
Huang CC, Lam SN, Acharya P, et al. (2007) Structures of the CCR5 N terminus and of a tyrosine-sulfated antibody with HIV-1 gp120 and CD4. Science 317: 1930-1934. doi: 10.1126/science.1145373
![]() |
[113] |
Huang CC, Tang M, Zhang MY, et al. (2005) Structure of a V3-containing HIV-1 gp120 core. Science 310: 1025-1028. doi: 10.1126/science.1118398
![]() |
[114] |
Huang J, Ofek G, Laub L, et al. (2012) Broad and potent neutralization of HIV-1 by a gp41-specific human antibody. Nature 491: 406-412. doi: 10.1038/nature11544
![]() |
[115] |
Kwong PD, Wyatt R, Robinson J, et al. (1998) Structure of an HIV gp120 envelope glycoprotein in complex with the CD4 receptor and a neutralizing human antibody. Nature 393: 648-659. doi: 10.1038/31405
![]() |
[116] |
McLellan JS, Pancera M, Carrico C, et al. (2011) Structure of HIV-1 gp120 V1/V2 domain with broadly neutralizing antibody PG9. Nature 480: 336-343. doi: 10.1038/nature10696
![]() |
[117] |
Pejchal R, Doores KJ, Walker LM, et al. (2011) A potent and broad neutralizing antibody recognizes and penetrates the HIV glycan shield. Science 334: 1097-1103. doi: 10.1126/science.1213256
![]() |
[118] |
Ward AB, Wilson IA (2015) Insights into the trimeric HIV-1 envelope glycoprotein structure. Trends Biochem Sci 40: 101-107. doi: 10.1016/j.tibs.2014.12.006
![]() |
[119] |
Zhou T, Georgiev I, Wu X, et al. (2010) Structural basis for broad and potent neutralization of HIV-1 by antibody VRC01. Science 329: 811-817. doi: 10.1126/science.1192819
![]() |
[120] |
Zhou T, Xu L, Dey B, et al. (2007) Structural definition of a conserved neutralization epitope on HIV-1 gp120. Nature 445: 732-737. doi: 10.1038/nature05580
![]() |
[121] |
Dutta M, Liu J, Roux KH, et al. (2014) Visualization of retroviral envelope spikes in complex with the V3 loop antibody 447-52D on intact viruses by cryo-electron tomography. J Virol 88: 12265-12275. doi: 10.1128/JVI.01596-14
![]() |
[122] |
Harris AK, Bartesaghi A, Milne JL, et al. (2013) HIV-1 envelope glycoprotein trimers display open quaternary conformation when bound to the gp41 membrane-proximal external-region-directed broadly neutralizing antibody Z13e1. J Virol 87: 7191-7196. doi: 10.1128/JVI.03284-12
![]() |
[123] |
Hu G, Liu J, Taylor KA, et al. (2011) Structural comparison of HIV-1 envelope spikes with and without the V1/V2 loop. J Virol 85: 2741-2750. doi: 10.1128/JVI.01612-10
![]() |
[124] |
Zhu P, Winkler H, Chertova E, et al. (2008) Cryoelectron tomography of HIV-1 envelope spikes: further evidence for tripod-like legs. PLoS Pathog 4: e1000203. doi: 10.1371/journal.ppat.1000203
![]() |
[125] |
Sanders RW, Vesanen M, Schuelke N, et al. (2002) Stabilization of the soluble, cleaved, trimeric form of the envelope glycoprotein complex of human immunodeficiency virus type 1. J Virol 76: 8875-8889. doi: 10.1128/JVI.76.17.8875-8889.2002
![]() |
[126] |
Earl LA, Lifson JD, Subramaniam S (2013) Catching HIV 'in the act' with 3D electron microscopy. Trends Microbiol 21: 397-404. doi: 10.1016/j.tim.2013.06.004
![]() |
[127] |
Lucic V, Rigort A, Baumeister W (2013) Cryo-electron tomography: the challenge of doing structural biology in situ. J Cell Biol 202: 407-419. doi: 10.1083/jcb.201304193
![]() |
[128] |
Jun S, Ke D, Debiec K, et al. (2011) Direct visualization of HIV-1 with correlative live-cell microscopy and cryo-electron tomography. Structure 19: 1573-1581. doi: 10.1016/j.str.2011.09.006
![]() |
[129] | Jun S, Zhao G, Ning J, et al. (2013) Correlative microscopy for 3D structural analysis of dynamic interactions. J Vis Exp. |
[130] |
Majorovits E, Nejmeddine M, Tanaka Y, et al. (2008) Human T-lymphotropic virus-1 visualized at the virological synapse by electron tomography. PLoS One 3: e2251. doi: 10.1371/journal.pone.0002251
![]() |
[131] |
Martin N, Welsch S, Jolly C, et al. (2010) Virological synapse-mediated spread of human immunodeficiency virus type 1 between T cells is sensitive to entry inhibition. J Virol 84: 3516-3527. doi: 10.1128/JVI.02651-09
![]() |
[132] |
Do T, Murphy G, Earl LA, et al. (2014) Three-dimensional imaging of HIV-1 virological synapses reveals membrane architectures involved in virus transmission. J Virol 88: 10327-10339. doi: 10.1128/JVI.00788-14
![]() |
[133] | Drobne D (2013) 3D imaging of cells and tissues by focused ion beam/scanning electron microscopy (FIB/SEM). Methods Mol Biol 950: 275-292. |
[134] |
Risco C, Castro IFd, Sanz-Sánchez L, et al. (2014) Three-Dimensional Imaging of Viral Infections. Annual Review of Virology 1: 453-473. doi: 10.1146/annurev-virology-031413-085351
![]() |
[135] |
Kukulski W, Schorb M, Welsch S, et al. (2011) Correlated fluorescence and 3D electron microscopy with high sensitivity and spatial precision. J Cell Biol 192: 111-119. doi: 10.1083/jcb.201009037
![]() |
[136] |
Sartori A, Gatz R, Beck F, et al. (2007) Correlative microscopy: bridging the gap between fluorescence light microscopy and cryo-electron tomography. J Struct Biol 160: 135-145. doi: 10.1016/j.jsb.2007.07.011
![]() |
[137] |
Zhang P (2013) Correlative cryo-electron tomography and optical microscopy of cells. Curr Opin Struct Biol 23: 763-770. doi: 10.1016/j.sbi.2013.07.017
![]() |
[138] | Woodward CL, Cheng SN, Jensen GJ (2014) Electron cryo-tomography studies of maturing HIV-1 particles reveal the assembly pathway of the viral core. J Virol. |
[139] |
Carlson LA, de Marco A, Oberwinkler H, et al. (2010) Cryo electron tomography of native HIV-1 budding sites. PLoS Pathog 6: e1001173. doi: 10.1371/journal.ppat.1001173
![]() |
[140] |
Dobro MJ, Samson RY, Yu Z, et al. (2013) Electron cryotomography of ESCRT assemblies and dividing Sulfolobus cells suggests that spiraling filaments are involved in membrane scission. Mol Biol Cell 24: 2319-2327. doi: 10.1091/mbc.E12-11-0785
![]() |
[141] |
Effantin G, Dordor A, Sandrin V, et al. (2013) ESCRT-III CHMP2A and CHMP3 form variable helical polymers in vitro and act synergistically during HIV-1 budding. Cell Microbiol 15: 213-226. doi: 10.1111/cmi.12041
![]() |
[142] |
Shen QT, Schuh AL, Zheng Y, et al. (2014) Structural analysis and modeling reveals new mechanisms governing ESCRT-III spiral filament assembly. J Cell Biol 206: 763-777. doi: 10.1083/jcb.201403108
![]() |
[143] |
Van Engelenburg SB, Shtengel G, Sengupta P, et al. (2014) Distribution of ESCRT machinery at HIV assembly sites reveals virus scaffolding of ESCRT subunits. Science 343: 653-656. doi: 10.1126/science.1247786
![]() |
[144] |
de Marco A, Muller B, Glass B, et al. (2010) Structural analysis of HIV-1 maturation using cryo-electron tomography. PLoS Pathog 6: e1001215. doi: 10.1371/journal.ppat.1001215
![]() |
[145] |
de Marco A, Heuser AM, Glass B, et al. (2012) Role of the SP2 domain and its proteolytic cleavage in HIV-1 structural maturation and infectivity. J Virol 86: 13708-13716. doi: 10.1128/JVI.01704-12
![]() |
[146] |
Konnyu B, Sadiq SK, Turanyi T, et al. (2013) Gag-Pol processing during HIV-1 virion maturation: a systems biology approach. PLoS Comput Biol 9: e1003103. doi: 10.1371/journal.pcbi.1003103
![]() |
[147] |
Keller PW, Adamson CS, Heymann JB, et al. (2011) HIV-1 maturation inhibitor bevirimat stabilizes the immature Gag lattice. J Virol 85: 1420-1428. doi: 10.1128/JVI.01926-10
![]() |
[148] |
Benjamin J, Ganser-Pornillos BK, Tivol WF, et al. (2005) Three-dimensional structure of HIV-1 virus-like particles by electron cryotomography. J Mol Biol 346: 577-588. doi: 10.1016/j.jmb.2004.11.064
![]() |
[149] |
Levandovsky A, Zandi R (2009) Nonequilibirum assembly, retroviruses, and conical structures. Phys Rev Lett 102: 198102. doi: 10.1103/PhysRevLett.102.198102
![]() |
[150] |
Yu Z, Dobro MJ, Woodward CL, et al. (2013) Unclosed HIV-1 capsids suggest a curled sheet model of assembly. J Mol Biol 425: 112-123. doi: 10.1016/j.jmb.2012.10.006
![]() |
[151] |
Sougrat R, Bartesaghi A, Lifson JD, et al. (2007) Electron tomography of the contact between T cells and SIV/HIV-1: implications for viral entry. PLoS Pathog 3: e63. doi: 10.1371/journal.ppat.0030063
![]() |
[152] |
Cardone G, Brecher M, Fontana J, et al. (2012) Visualization of the two-step fusion process of the retrovirus avian sarcoma/leukosis virus by cryo-electron tomography. J Virol 86: 12129-12137. doi: 10.1128/JVI.01880-12
![]() |
[153] |
Felts RL, Narayan K, Estes JD, et al. (2010) 3D visualization of HIV transfer at the virological synapse between dendritic cells and T cells. Proc Natl Acad Sci U S A 107: 13336-13341. doi: 10.1073/pnas.1003040107
![]() |
[154] |
Sherer NM, Lehmann MJ, Jimenez-Soto LF, et al. (2007) Retroviruses can establish filopodial bridges for efficient cell-to-cell transmission. Nat Cell Biol 9: 310-315. doi: 10.1038/ncb1544
![]() |
[155] | Pique C, Jones KS (2012) Pathways of cell-cell transmission of HTLV-1. Front Microbiol 3: 378. |
[156] |
Hill CP, Worthylake D, Bancroft DP, et al. (1996) Crystal structures of the trimeric human immunodeficiency virus type 1 matrix protein: implications for membrane association and assembly. Proc Natl Acad Sci U S A 93: 3099-3104. doi: 10.1073/pnas.93.7.3099
![]() |
[157] |
Rao Z, Belyaev AS, Fry E, et al. (1995) Crystal structure of SIV matrix antigen and implications for virus assembly. Nature 378: 743-747. doi: 10.1038/378743a0
![]() |
1. | Manu Shree, Amita Dev, A. K. Mohapatra, 2023, Chapter 56, 978-981-19-6630-9, 807, 10.1007/978-981-19-6631-6_56 | |
2. | Toshiya Akiyama, Kazuyuki Matsumoto, Kyoko Osaka, Ryuichi Tanioka, Feni Betriana, Yueren Zhao, Yoshihiro Kai, Misao Miyagawa, Yuko Yasuhara, Hirokazu Ito, Gil Soriano, Tetsuya Tanioka, Comparison of Subjective Facial Emotion Recognition and “Facial Emotion Recognition Based on Multi-Task Cascaded Convolutional Network Face Detection” between Patients with Schizophrenia and Healthy Participants, 2022, 10, 2227-9032, 2363, 10.3390/healthcare10122363 | |
3. | Chieh-Liang Wu, Shu-Fang Liu, Tian-Li Yu, Sou-Jen Shih, Chih-Hung Chang, Shih-Fang Yang Mao, Yueh-Se Li, Hui-Jiun Chen, Chia-Chen Chen, Wen-Cheng Chao, Deep Learning-Based Pain Classifier Based on the Facial Expression in Critically Ill Patients, 2022, 9, 2296-858X, 10.3389/fmed.2022.851690 | |
4. | Berrimi Fella, Hedli Riadh, Kara-Mohamed Chafia, 2022, Adaptive Diffusion Based Restoration for Noisy Facial Image Recognition, 978-1-7281-8442-5, 487, 10.1109/SETIT54465.2022.9875579 | |
5. | J.A.S.Y. Jayasinghe, Stamos Katsigiannis, Lakmini Malasinghe, 2023, Comparative Study of Face Tracking Algorithms for Remote Photoplethysmography, 979-8-3503-2781-6, 1, 10.1109/ICECET58911.2023.10389182 | |
6. | Jiarui Chen, Wei Li, Peihao Yang, Sheng Li, Baoqin Chen, Fault Diagnosis of Electric Submersible Pumps Using a Three‐Stage Multiscale Feature Transformation Combined with CNN–SVM, 2023, 11, 2194-4288, 10.1002/ente.202201033 | |
7. | Tuotuo Xiong, Ben Wang, Wanyuan Qin, Ling Yang, Yunsheng Ou, Development and validation of a risk prediction model for cage subsidence after instrumented posterior lumbar fusion based on machine learning: a retrospective observational cohort study, 2023, 10, 2296-858X, 10.3389/fmed.2023.1196384 | |
8. | Libo Qiao, Sheng Guan, Wei Wang, 2024, Online learning concentration recognition based on computer vision, 9798400709920, 334, 10.1145/3687311.3687372 | |
9. | Mohamed Gamal, Magdy Shayboub, 2024, Chapter 9, 978-981-97-6713-7, 111, 10.1007/978-981-97-6714-4_9 | |
10. | Arjon Turnip, Adnnia Nafis Qulub Aina, Erwin Sitompul, 2024, Patient Face Recognition for Medical Robots Based on Convolutional Block Attention Module, 979-8-3315-1116-6, 1, 10.1109/CERIA64726.2024.10914760 |
Characteristics | Original image data set | Test data set |
Male: Female | 10: 15 | 13: 12 |
Image size | 1897×1897 | 1897×1897 |
Model | PSNR (dB) | SSIM |
MTCNN | 36.245 | 0.954 |
R-CNN | 35.005 | 0.927 |
Faster R-CNN | 35.305 | 0.938 |