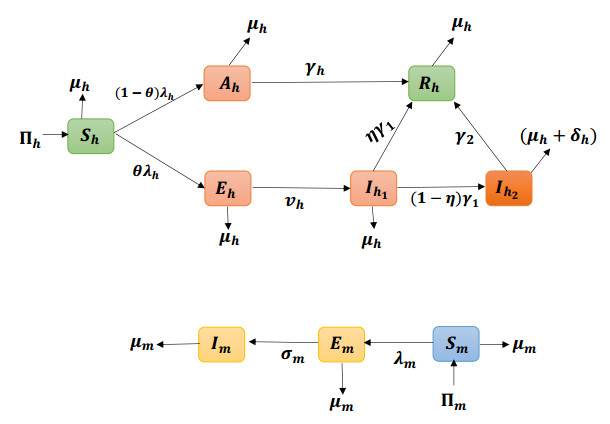
Citation: Viktorija Dičpinigaitienė, Lina Novickytė. Application of systemic risk measurement methods: A systematic review and meta-analysis using a network approach[J]. Quantitative Finance and Economics, 2018, 2(4): 798-820. doi: 10.3934/QFE.2018.4.798
[1] | Eunha Shim . Optimal dengue vaccination strategies of seropositive individuals. Mathematical Biosciences and Engineering, 2019, 16(3): 1171-1189. doi: 10.3934/mbe.2019056 |
[2] | Hyun Mo Yang, André Ricardo Ribas Freitas . Biological view of vaccination described by mathematical modellings: from rubella to dengue vaccines. Mathematical Biosciences and Engineering, 2019, 16(4): 3195-3214. doi: 10.3934/mbe.2019159 |
[3] | Vanessa Steindorf, Sergio Oliva, Jianhong Wu . Cross immunity protection and antibody-dependent enhancement in a distributed delay dynamic model. Mathematical Biosciences and Engineering, 2022, 19(3): 2950-2984. doi: 10.3934/mbe.2022136 |
[4] | Haitao Song, Dan Tian, Chunhua Shan . Modeling the effect of temperature on dengue virus transmission with periodic delay differential equations. Mathematical Biosciences and Engineering, 2020, 17(4): 4147-4164. doi: 10.3934/mbe.2020230 |
[5] | Bo Zheng, Lihong Chen, Qiwen Sun . Analyzing the control of dengue by releasing Wolbachia-infected male mosquitoes through a delay differential equation model. Mathematical Biosciences and Engineering, 2019, 16(5): 5531-5550. doi: 10.3934/mbe.2019275 |
[6] | Guangming Qiu, Zhizhong Yang, Bo Deng . Backward bifurcation of a plant virus dynamics model with nonlinear continuous and impulsive control. Mathematical Biosciences and Engineering, 2024, 21(3): 4056-4084. doi: 10.3934/mbe.2024179 |
[7] | Wei You, Jie Ren, Qimin Zhang . Finite-time contraction stability of a stochastic reaction-diffusion dengue model with impulse and Markov switching. Mathematical Biosciences and Engineering, 2023, 20(9): 16978-17002. doi: 10.3934/mbe.2023757 |
[8] | Biao Tang, Weike Zhou, Yanni Xiao, Jianhong Wu . Implication of sexual transmission of Zika on dengue and Zika outbreaks. Mathematical Biosciences and Engineering, 2019, 16(5): 5092-5113. doi: 10.3934/mbe.2019256 |
[9] | Saima Akter, Zhen Jin . Simulations and fractional modeling of dengue transmission in Bangladesh. Mathematical Biosciences and Engineering, 2023, 20(6): 9891-9922. doi: 10.3934/mbe.2023434 |
[10] | Zuohuan Zheng, Kaihua Wang, Daiyu Yang, Feifei Yin, Dingwei Sun, Weiyan Yu, Jialun Lin, Ying Liu, Changkuan Chen, Zehui Yang, Biao Wu . Factors affecting the transmission of dengue fever in Haikou city in 2019. Mathematical Biosciences and Engineering, 2023, 20(9): 16045-16059. doi: 10.3934/mbe.2023716 |
Dengue virus (DENV), a flavivirus primarily transmitted to human via a bites of an infected mosquitoes of the genus Aedes (i.e., Aedes aegypti or Aedes albopictus) [1,2,3,4,5,6,7,8,9,10]. This species is capable of transmitting other mosquito-borne diseases such as Chikungunya, Zika, Yellow fever [3,11,12,13,14]. The DENV is endemic in at least 100 countries in the tropical and subtropical regions of the world, with nearly 4 billion people at risk [7,15]. It is second to malaria amongst the deadly mosquito-borne diseases with fast-spreading ability [7], with an estimate of 390 million new infections of which about 96 million are clinical, up to 1 million are severe cases (i.e., dengue hemorrhagic fever/dengue shock syndrome also known as DHF/DSS), and more than 20 thousand deaths every year globally [6,7,16]. The DHF/DSS have a high mortality risk with death occurring within 24hrs after the onset of shock [17,18,19]).
The DENV are of four serotypes (i.e., DENV-1, DENV-2, DENV-3 and DENV-4) with low cross-immunity among each other which can result in secondary infection after an infection with one serotype has happened [7]. An individual infected with one serotype can be infected with different serotypes about a half year later [3], yet there is no proof of reinfection with the same serotype. DEN infection of all serotypes can cause three distinct syndromes: classic dengue fever, DHF and DSS [3].
The epidemiological cycle occurs as follows. Female Aedes aegypti mosquitoes are infected by the blood meal from biting an infectious human during the viremic stage of the infection. After a period of time called the extrinsic incubation period (EIP), the mosquitoes become infectious [20]. The range of the extrinsic incubation period lies between 4 to 10 days [20]. The infected mosquito does not have immunity and can transmit the virus throughout its lifespan [3,7,20]. An infectious mosquito can transmit the virus to a susceptible individual via bites [21], after a period of time called the intrinsic incubation period (IIP), which varies from 4 to 7 days [20], the virus can evolve from classical dengue fever to the secondary cases of dengue infection which may lead to DHF or DSS [17]. The manifestation of the symptoms varies between 3 to 7 days [3], which corresponds roughly to the infectious period. Thereafter, the individual develops the life-long immunity against the same serotype of the virus [3].
The prevention against DENV is limited to controlling mechanisms applied on the mosquito, because the vaccine is not yet available (even though there is a vaccine called dengvaxia, which is still under clinical trial, released by Sanofi Pasteur in 2015 which was approved by morethan 10 countries [7,22]. The vaccine efficacy varies by the serotypes of the DENV (i.e., 54.7 % for DENV-1, 43.0 % for DENV-2, 71.6 % for DENV-3, and 76.9 % for DENV-4 [7,23]). To describe the overall transmission dynamics of the DENV, we developed a mathematical model that takes into account the female adult mosquito coupled with the human population to analyses and explain the transmission dynamics of the DENV epidemics in Taiwan in 2014–2015.
A number of mathematical modeling studies have been carried out to gain insight into the DENV transmission dynamics in human and/or mosquito populations [3,4,5,6,11,24,25]. Garba et al. [6] developed a deterministic model for the DENV transmission within human and mosquito populations, and extended the model to incorporate an imperfect vaccine against the virus, and find that both models exhibited backward bifurcation phenomenon, and the courses have been explained in detail by Gumel [26]. Yang and Ferreiri [3] proposed a model to describe the transmission dynamics of the DENV in human and mosquito populations, they incorporated aquatic stages (i.e., eggs, larvae, pupae) in the mosquito population, and calculated the basic reproduction number to be less than one (which indicate that the disease can dies out in time). Yang et al. [24] designed a simple model in order to assess the effect of temperature on the population of the mosquito by using the temperature controlled experiments. Yang et al. [25] extended Yang et al. [24] by including human compartments and updated most of the parameters to assess the effect of temperature on the risk of the DENV outbreaks. Yang [5] developed and analyzed a model encompassing four quiescence stages of the mosquito, and used the model to assess the influence of the quiescent eggs on the transmission dynamics of the mosquito.
In the current study, we focus on the design and analysis of a model, which extends some of the aforementioned studies, for assessing the transmission dynamics of the DENV in human and mosquito populations. Our model includes both the human and mosquito populations, and incorporates asymptomatic (Ah) and severe cases (those with DHF/DSS) of the DENV infection (Ih2) in human population. We performed model fitting by using the plug-and-play inference framework for the human population, and our results contribute to better understanding of the transmission dynamics of the DENV epidemics in Taiwan in 2014-15 and provide useful guidelines for the design of control strategies in the future.
The paper is organized as follows. The model is formulated in section 2 and analyzed in section 3. Sensitivity analysis results of the full model is also given in section 4. Finally numerical analysis of the human only sub-model is performed with a plug-and-play inference framework in section 5.
The total human population at time t, denoted by Nh(t), is divided into six compartments of susceptible, Sh(t), exposed (infected but not infectious individuals that can progress to Ih1 class), Eh(t), asymptomatically infected, Ah(t) (i.e., expose individuals that can progress and becomes infectious without showing any clinical symptoms, which are lumped together for computational conveniences), symptomatically infected, Ih1(t), symptomatically infected with severe DENV (DHF/DSS), Ih2(t), and recovered, Rh(t), humans. Hence,
Nh(t)=Sh(t)+Eh(t)+Ah(t)+Ih1(t)+Ih2(t)+Rh(t). |
The total mosquito population at time t, denoted by Nm(t), is split into susceptible Sm(t), exposed (infected but not infectious mosquitoes) Em(t) and symptomatic (infectious mosquitoes) Im(t) classes. Hence,
Nm(t)=Sm(t)+Em(t)+Im(t). |
The model for the DENV transmission in the human and mosquito populations is given by the following deterministic ordinary differential equations (ODE) systems (2.1). The flow diagram of the model (2.1) is depicted in Figure 1. The state variables and parameters of the model are described in Tables 1 and 2, respectively.
dShdt=Πh−λhSh−μhSh,dEhdt=θλhSh−(vh+μh)Eh,dAhdt=(1−θ)λhSh−(γh+μh)Ah,dIh1dt=vhEh−(γ1+μh)Ih1,dIh2dt=(1−η)γ1Ih1−(δh+γ2+μh)Ih2,dRhdt=ηγ1Ih1+γ2Ih2+γhAh−μhRh,dSmdt=Πm−λmSm−μmSm,dEmdt=λmSm−(σm+μm)Em,dImdt=σmEm−μmIm. | (2.1) |
Variable | Interpretation |
Nh(t) | Total population of humans |
Sh(t) | Population of susceptible humans |
Eh(t) | Population of humans exposed to DENV |
Ah(t) | Population of asymptomatic humans |
Ih1(t) | Population of humans with clinical symptoms of the DENV |
Ih2(t) | Population of humans with severe clinical symptoms of the DENV |
Rh(t) | Population of humans recovered from the DENV |
Nm(t) | Total population of adult female mosquitoes |
Sm(t) | population of susceptible adult mosquitoes |
Em(t) | population of exposed adult mosquitoes |
Im(t) | population of infectious adult mosquitoes |
Parameter | Interpretation/Description |
Πh/Πm | Recruitment rate of humans/mosquitoes |
μh | Natural death rate of humans |
μm | Death rate of female adult mosquitoes |
βh | Transmission probability from infectious mosquitoes to susceptible humans |
βm | Transmission probability from infectious humans to susceptible mosquitoes |
a | Mosquito biting rate |
b | Maximum number of bites a human can receive per unit time |
θ | Fraction of infected humans that are exposed |
vh | Progression rate of exposed humans to infectious humans with clinical symptoms |
η | Fraction of infectious humans that will not get a severe symptom |
γh, γ1, γ2 | Recovery rate of infectious humans from Ah, Ih1, Ih2, respectively |
δh | Disease-induced death rate of humans |
σm | Progression rate of exposed mosquitoes to the infectious mosquitoes |
ρ | Reporting rate to actual case ratio |
m | Average mosquito to human ratio |
Here, the infection rate for humans (λh) and vectors (λm) are given by
λh=abβhImNh,andλm=abβm(Ah+Ih1+Ih2)Nh. | (2.2) |
In model (2.1), Πh is the recruitment rate for human by birth, λh is the infection rate of susceptible human from infectious mosquitoes, where a is the mosquito biting rate, b is the maximum number of bites a human can receive per unit time, βh is the transmission probability from infected mosquitoes to the susceptible humans while βm is transmission probability from an infectious humans to the susceptible mosquitoes per bite, and μh is the natural death rate of human. The term θ is the symptomatic ratio, and (1−θ) remains the fraction of asymptomatic ratio. The parameter vh measures the rate at which an individuals in the Eh class develop a primary clinical symptoms of the virus, while η is a fraction of humans who will not get a severe DENV, δh accounts for the disease-induced death rate of humans from the Ih2 class, and γi(i=0,1,2) measures the rate of recovery of individual from Ah, Ih1, and Ih2, respectively.
The susceptible adult mosquitoes acquire the DENV at a rate λm, this population is decreased by natural death at a rate μm. Finally, σm is a progression rate of the DENV from the Em(t) class to the Im(t). It is also worth stating that the model (2.1) accounts for the conservation law of mosquito bites. Thus, the infection rates, λh and λm, are normalized by the total host population, that is Nh(t) [27].
The basic properties of the model (2.1) will now be explored. Consider the following equations for the rate of change of the total human N′h(t) and mosquito N′m(t) populations
N′h(t)=dNhdt=Πh−μhNh−δhIh2⩽Πh−μhNh, | (2.3) |
and
N′m(t)=dNmdt=Πm−μmNm, | (2.4) |
here, the prime, ′, represent a differentiation with respect to time, t.
Furthermore, consider the region,
Ω={(Sh,Eh,Ah,Ih1,Ih2,Rh,Sm,Em,Im)∈R9+:Nh⩽Πhμh,Nm⩽Πmμm}. |
It can be shown by solving for Nh and Nm in Eqs (2.3)-(2.4) so that all solutions of the system starting in the region Ω will remain in Ω for all time t with t⩾0. Thus, the region Ω is positively-invariant, and it is sufficient to consider solutions restricted in Ω. In this region, the usual existence, uniqueness and continuation results hold for the system (2.1) [28,29].
The disease-free equilibrium (DFE) of the model (2.1) obtained at steady state is given by
E0=(S0h,E0h,A0h,I0h1,I0h2,R0h,S0m,E0m,I0m)=(Πhμh,0,0,0,0,0,Πmμm,0,0). |
Using the next generation matrix method [30], we obtained the associated reproduction number of the model (2.1), denoted by R0=ρ(FV−1). The term ρ represents the function to find the spectral radius of the next generation matrix, G=FV−1. The matrices F (for the new infection terms) and V (for the remaining transition terms), associated with the model (2.1), are given by
F=[00000θabβh00000(1−θ)abβh0000000000000abβmS0mNhabβmS0mNhabβmS0mNh00000000], | (3.1) |
V=[n1000000n20000−vh0n300000−(1−η)γ1n4000000n500000−σmμm], | (3.2) |
where n1=vh+μh, n2=γh+μh, n3=γ1+μh, n4=δh+γ2+μh, and n5=σm+μm. Therefore, the basic reproduction number, R0, is given by
R0=Rh⋅Rm, | (3.3) |
where
Rh=√abβhμhΠh⋅[θvhn1n3+θ(1−η)γ1vhn1n3n4+(1−θ)n2], | (3.4) |
and
Rm=√abβmσmS0mn5μm. | (3.5) |
R0 interpretation: The threshold quantity R0 is ecologically and epidemiologically interpreted as follows.
ⅰ. abβhNh accounts for the number of new infected human hosts caused by an infected mosquito over its expected infectious period,
ⅱ. vhn1 is the probability that an exposed human survives the exposed stage and move to the infectious stage, Ih1,
ⅲ. 1n3 is the average duration in the infectious stage,
ⅳ. (1−η)γ1n4 is the probability that an infectious human survives the infectious stage (including severe stage of the DENV) and move to the recovered class,
ⅴ. (1−θ)n2 is the probability that an infected human move to the asymptomatic class,
ⅵ. abβmσmn5S0mμm accounts for the possible number of new infected mosquito caused by an infected human hosts over its exposed infectious period.
The theorem 3.1 below follows the Theorem 2 of Ref [30].
Theorem 3.1. The DFE, E0, of the model (2.1), is locally-asymptotically stable (LAS) in Ω if R0<1, but unstable if R0>1.
The endemic equilibrium (EE) for the system (2.1), which is
E∗=(S∗h,E∗h,A∗h,I∗h1,I∗h2,R∗h,S∗m,E∗m,I∗m), |
in terms of the forces of infection, λ∗h and λ∗m, is given by
S∗h=Πhλh+μh,E∗h=θλhΠhn1(λh+μh),A∗h=(1−θ)λhΠhn2(λh+μh),I∗h1=vhθλhΠhn1n3(λh+μh),I∗h2=(1−η)γ1vhθλhΠhn1n3n4(λh+μh),R∗h=Πhλhμh(λh+μh)((1−θ)γhn2+ηγ1vhθn1n3+γ1γ2(1−η)vhθn1n3n4),S∗m=Πmλm+μm,E∗m=Πmλmn5(λm+μm)andI∗m=σmΠmλmn5μm(λm+μm). | (3.6) |
Substituting the above equilibrium points (equation (3.6)) into equation (2.2), we have
Aλ2h+Bλh+C=0, | (3.7) |
where
A=Πh(σm+μm)μm(−abβmμhθvhn2n4−abβmμhγ1θvhn2+abβmμhγ1θvhn2η−abβmμh(1−θ)n1n3n4−μmθμhn2n3n4−μm(1−θ)μhn1n3n4−μmθvhμhn2n4−μmγ1θvhμhn2+μmγ1θvhμhn2η−μmγh(1−θ)n1n3n4−μmηγ1vhθn2n4−μmγ1γ2vhθn2+μmγ1γ2vhθn2η)(−θμhn2n3n4−(1−θ)μhn1n3n4−θvhμhn2n4−γ1θvhμhn2+γ1θvhμhn2η−γh(1−θ)n1n3n4−ηγ1vhθn2n4−γ1γ2vhθn2+γ1γ2vhθn2η), |
B=n4n2n1μh(−μm(σm+μm)[(((((−2n3−2vh)n4+2γ1vh(−1+η))n2+2n1n3n4)μh−2vh(n4η−γ2(−1+η))γ1n2+2γhn1n3n4)θ−2n1n3n4(μh+γh))μm+a(((−n4+γ1(−1+η))vhn2+n1n3n4)θ−n1n3n4)μhbβm]Πh+a2σm(((−n4+γ1(−1+η))vhn2+n1n3n4)θ−n1n3n4)μhΠmb2βmβh)n3,andC=(1−R20). |
Therefore, one can obtain the positive EE of the model (2.1) by simplifying the quadratic equation (3.7), and substituting only the positive values of the λh into the EE points. The occurrence of the quadratic equation (3.7) shows that the backward bifurcation (BB) for the model (2.1) exists.
Hence, the following Theorem 3.2 is established.
Theorem 3.2. The model (2.1) has
i) a EE if C<0⇔R0>1;
ii) a unique EE if B<0 and C=0 or B2−4AB=0;
iii) two EEs if B<0, C>0 and B2−4AC>0; or
iv) no EE otherwise.
The existence of the phenomenon of the BB in the model (2.1) highlights a co-existence between a stable disease free-equilibrium (DFE) and a stable endemic equilibrium (EE) even if the basic reproduction number (R0) is less than unity, this makes the disease control more difficult. Furthermore, the disease eradication would no longer depends on the basic concept of R0, i.e., when R0<1, the disease dies out in time; and while R0>1, the disease persists in the community. Although the BB has been firstly shown to exist in the DENV from the work of Garba et al. [6] and its courses has been explained extensively by Gumel [26]. In this study, our analysis suggests that the BB can be removed if the R0 can be equal to one (i.e., R0=1), so that equation (3.7) will be Aλh+B=0 which is linear equation.
The following Theorem 3.3 is claimed.
Theorem 3.3. The endemic equilibrium (EE), E∗, is globally-asymptotically stable (GAS) in Ω when R0>1 provided that
(1−λhλ∗h)(1−Ih1λ∗hI∗h1λh)⩾0,(1−λhλ∗h)(1−Ih2λ∗hI∗h2λh)⩾0,(1−λmλ∗m)(1−Imλ∗mI∗mλm)⩾0,and(Ih1I∗h1−lnIh1Ih1+lnAhAh+AhA∗h)⩽0. | (3.8) |
The proof of the above Theorem 3.3 is given in Appendix 7, and the Figure 2 is used to demonstrate the analytical result obtained.
Parameter | Baseline [Range] | Units | Source |
Πh | 2.5 [1,5] | person~day−1 | [6] |
Πm | 5000 [1000,6000] | Day−1 | estimated from [6] |
μh | 3.9×10−5 [3.6×10−5, 4.0×10−5] | Day−1 | [12] |
μm | 0.05714 [0.01,0.1] | Day−1 | [11] |
βh | 0.75 [0.1,0.95] | Day−1 | [6] |
βm | 0.75 [0.1,0.95] | Day−1 | [6] |
a | 0.4997 [0.1,1] | Day−1 | [13,14] |
b | 0.4 [0.1,1] | Day−1 | [13] |
θ | 0.18 [0.1,0.6] | Dimensionless | [31] |
vh | 0.0666 [0,1] | Day−1 | [6,32] |
η | 0.1 [0.05,0.15] | Dimensionless | [19,33] |
γh | 0.1428 [0.1,0.2] | Day−1 | [13] |
γ1 | 0.2 [0.1428,0.3] | Day−1 | [13] |
γ2 | 0.05 [0.0333,0.07143] | Day−1 | [13] |
δh | 10−3 [10−4,2×10−3] | Day−1 | [6] |
σm | 0.5 [0,1] | Day−1 | [6] |
m | 10 [1,20] | Dimensionless | assumed |
Following the previous studies [13,14,34,35], we adopted the partial ranked correlation coefficient (PRCC) for sensitivity analysis. The PRCC of the R0 and IAR of the model (2.1) is estimated. The sensitivity analysis results in Figure 3 suggest the top three ranked parameters (i.e., a, b and Πm) are prioritized in controlling the DENV epidemics in Taiwan.
The analyses in this section will be carried out for the special scenario of the model (2.1) in the absence of mosquito compartments (i.e., a human only sub-model). We assumed that there is no birth and death rate (Πh=μh=0), and no disease-induced mortality rate (δh=0) in a short period. We also assumed that the effective contact rate of the simplified version of the model to be β=β(t)=ab⋅m(t), where m(t) is the time-dependent mosquito to human ratio. This setting allows us to fit the "SIR" based model to a vector-borne disease under the "absence of vector" model structure. The term of the product of (abm) is equivalent to the standard Ross-Macdonald malaria model [36].
The equivalent (simplified) model without mosquito compartment together with the above assumptions is given by
dShdt=−βIh1ShNh,dEhdt=θβIh1ShNh−vhEh,dIh1dt=vhEh−γ1Ih1,dIh2dt=(1−η)γ1Ih1−γ2Ih2,dAhdt=(1−θ)βIh1ShNh−γhAh,dRhdt=ηγ1Ih1+γ2Ih2+γhAh, | (5.1) |
where the basic reproduction ratio of the simplified model (5.1) is given by R0(t)=βθγ1.
The weekly number of cases of the i-th week is
Zi=∫weekiργ1Ih1dt, | (5.2) |
where the term ρ denotes a constant reporting rate of the DENV cases. The Zi denotes the theoretical weekly DENV cases yield from the model (5.1).
We model the observed (reported) DENV cases, Ci for the i-th week during the study period, as a partially observed Markov process (POMP, also know as hidden Markov model, HMM). Instead of implementing Poisson-distributed priors [34], all Cis are assumed to follow over-dispersed Poisson distributions according to the theoretical modelling (Eq (5.2)) outputs, Zis. Since the rate of Poisson distribution is a Gamma random variable, the observed weekly number of people who are confirmed DENV infections (Ci of the i-th week) is a random sample from a Negative-binomial (NB) distribution. Therefore,
Ci∼NB(mean=Zi,variance=Zi(1+ψZi)), | (5.3) |
where ψ is the over-dispersion parameter for NB distribution under estimation. In this model, we set Li(⋅) be the likelihood function for the week, which is the "probability" of Ci, given the real cases from simulations Zi under the NB distribution [37,38]. In this section, the model simulations are conducted by using the R (version 3.4.1) package "POMP" [39].
The overall log-likelihood, l, for the whole-time series is
l(Θ)=T∑i=1ln[Li(Ci∣C0,...,Ci−1;Θ)], | (5.4) |
where Θ is the parameter vector under estimation. The term T denotes the total number of weeks during the study period. We apply the iterated filtering algorithm with the plug-and-play likelihood-based inference framework to estimate the maximum likelihood estimates (MLE) of Θ, (see [37,40,41,42,43,44,45,46]. We use the fixed-time-step Euler-multinomial algorithm to simulate the ODE system (5.1) [14,34,37,38,40], We compared different models using the small-sample-size corrected Akaike's Information Criterion (AICc) [44] as a measurement of the trade-off between model complexity and the goodness-of-fit. The AICc is given by
AICc=−2l(ˆΘ)+2k+2k(k+1)N−k−1, | (5.5) |
where N is the number of data points and k is the number of free parameters.
The (simplified) epidemic model (5.1) is fitted to the dengue cases time series in Kaohsiung and Tainan cities in 2014–2016. We proposed the time-dependent basic reproduction number (i.e., R0(t)) driven by variable mosquito abundance and dengue transmission rate (e.g., mosquito biting rate, etc). Here, we compared two numerical approaches to fit the epidemic data and reconstruct the R0(t) series, namely,
● the cubic spline reconstruction approach, and
● the square wave reconstruction approach.
The cubic spline approach is defined from the previous studies [14,47]. We used pq to denote the number of nodes in the cubic spline of R0(t) to be reconstructed. We tested different possible pq's with the aim to find the value of pq that leads to the lowest AICc. We further restrict that Kaohsiung and Tainan shared exactly the same cubic spline function of R0(t). Hence, the two cities were fitted simultaneously with the same set of parameter estimates. For the cubic spline approach, the fitting is conducted with completely the same time series of R0(t) (i.e., same timing, scale, and all settings are the same) for both cities. And we find the goodness-of-fit (in term of the MLL) can still be improved for both cities. Even though the spline can yield a relatively flexible (in term of the degree of freedom of the parameters to be estimated) R0(t) reconstruction outcome, the fittings are still not satisfied. Thus, we speculate a non-synchronized R0(t) would be more reasonable. The fitting results of the R0(t) reconstructed by the cubic spline function are shown in Figure 4, and the parameter estimates are summarized in Table 5. The square wave is defined as a baseline transmission rate plus a constant additional value for each year. The additional values are different for both Kaohsiung and Tainan in the same year, and also vary in different years. To explore the possible non-synchronization of R0(t)'s in Kaohsiung and Tainan cities, we further allow the changing time of the square wave to have different estimates in the two cities. Thus, the reconstructed R0(t)s in Kaohsiung and Tainan are expected to have different shape which indicates that the R0(t)'s of the two cities are not perfectly synchronized. In Tainan, we fix the time of the occurrence of the R0(t) high values (i.e., with the additional value) to be May 1 and October 31 in both 2014 and 2015. In Kaohsiung, our estimates are that the high values of R0(t) occurred between March 16 and October 31 in 2014, and between May 1 and November 25 in 2015. These time shifts are crucial to achieve good fitting outcomes in Kaohsiung city. Thus, this finding indicates that the DENV outbreaks in Kaohsiung turned on early in 2014, and turned off late in 2015. For the square-wave approach, the fitting is conducted with similar series of R0(t)'s for the two cities. The timing (of change) and the scale of R0(t)'s were slightly different. Although this approach is less flexible as the spline approach (i.e., with 4 degrees of freedom decreased), it leads to better fitting results with MLL improved by 10 units. And together, the AIC improves (i.e., decreases) by 24 units. This indicates that the non-synchronized square-wave approach is a strong and significant improvement from the synchronized spline approach. The fitting results of R0(t) reconstructed by the square wave function are shown in Figure 5, and the parameter estimates are summarized in Table 6. Therefore, for the spline versus square-wave approach, the non-synchronized square-wave approach has better goodness-of-fit (i.e., higher MLL) with less flexibility (i.e., less degree of freedom). In other words, the non-synchronized square-wave approach has better fitting performance (in term of the AIC). Hence, we conclude that non-synchronized R0(t)'s were likely to occur in the two cities.
Our (simplified) DENV epidemic model well-explained the temporal patterns of the DENV outbreaks in the two cities in Taiwan under biologically reasonable conditions (i.e., set of model parameters). We discovered that the epidemics in the two cites can be reconstructed by similar transmission series (i.e., R0(t)), and also estimated with the same model structure. The square wave approach not only reduced the number of free parameters by 4, but also increased the log likelihood by 10 units. Hence, its AICc decreases roughly by 24 units from the cubic spline reconstruction, which is a significant improvement.
Although we find that the estimated transmission rates exhibited similar seasonal patterns in Kaohsiung and Tainan, they are neither completely synchronized between the two cities despite their proximity, nor periodically in-phase perfectly. If they were completely synchronized or perfectly periodic, the cubic spline approach would identify such a pattern reflected by an equivalent level of goodness-of-fit as in the square wave approach. We conclude that the time lags between the seasonal waves of the transmission rates (R0(t)) in the two cities are likely to occurred. Other than the pathogenic factors that can causes the phase difference in the transmissibility [48], these time lags could be due to the heterogeneity in the local conditions in Kaohsiung and Tainan cities.
We formulated and analyzed a mathematical model to study the transmission dynamics of the dengue outbreaks in Kaohsiung and Tainan cities in Taiwan in 2014–2015. We showed that the model (2.1) has two equilibria, of which the disease-free equilibrium (DFE) is locally asymptotically stable (LAS) whenever the basic reproduction number (R0<1), and unstable if the R0>1. And the endemic equilibrium (EE) is globally asymptotically stable (GAS) in the region of attraction, Ω, whenever the R0>1 (numerical examples, see Figure 2, is used to demonstrate the analytical results obtained). Our analysis shows the existence of backward bifurcation (BB) phenomenon of the model (2.1), a situation where a stable disease free-equilibrium co-exists with a stable endemic equilibrium even when the basic reproduction number (R0) is less than one, which makes the disease control even more difficult and is no longer dependent on the basic concept of the R0 (i.e., R0<1 the disease dies out in time, while R0>1 the disease persist to the community). In this paper, the analysis suggest that the backward bifurcation can be removed when the basic reproduction number is equal to one (i.e., R0=1).
We employed the plug-and-play statistical inference framework on the special case of the model (2.1) in the absence of mosquito. We fitted the simplified model (5.1) to the weekly number of cases in the Kaohsiung and Tainan Cities in Taiwan (obtained from the National Infectious Disease Statistics System [49], see Figures. 4 and 5). Our (simplified) dengue epidemic model is able to explained the temporal patterns of the dengue outbreaks in the two cities in Taiwan under biologically reasonable conditions (i.e., set of model parameters). We obtained that the epidemics in the two cites can be reconstructed by the same transmission series (i.e., R0(t)) and also estimated with the same model structure. This findings suggests the similarity of the dengue outbreak in a two different places during the same period of time, which could be due to the similar demographic and/or meteorological conditions. The proposed two reconstruction approaches presents (roughly) equivalent goodness-of-fit in terms of the values of the log-likelihood. However, the AICc improves (decreases) roughly by 24 units from the cubic spline to the square wave reconstruction. We also found that despite the proximity in Kaohsiung and Tainan, the estimated transmission rates were neither completely synchronized, nor periodically in-phase perfectly in the two cities. The time lags between the seasonal waves of the transmission rates in the two cities were likely to occur. These time lags could be due to the differences in their local conditions. Furthermore, we show that the non-synchronized square-wave approach has better goodness-of-fit (i.e., higher MLL) with less flexibility (i.e., less degree of freedom). In other words, the non-synchronized square-wave approach has a better fitting performance (in term of the AIC) than the cubic spline approach. Hence, we concluded that non-synchronized R0(t)s were likely to occur in the two cities.
The sensitivity analysis results shows the top three ranked parameters (i.e., a, b and Πm), that is, the mosquito biting rate, the maximum number of bites a human can receive per unit time, and the mosquito recruitment rate are the key parameters to be prioritized for controlling the Taiwan dengue outbreaks. This further suggests that proper sanitation of mosquito breeding sites and avoiding the mosquito bites are the key control measures to future dengue outbreaks in Taiwan.
List of Abbreviations
We listed the abbreviations used in Table 4.
abbreviation | explanation / full name |
AICc | corrected Akaike's Information Criterion |
BB | Backward bifurcation |
CI | Confidence interval |
DENV | Degue virus |
DENV-1 | Dengue virus serotype 1 |
DENV-2 | Dengue virus serotype 2 |
DENV-3 | Dengue virus serotype 3 |
DENV-4 | Dengue virus serotype 4 |
DFE | Disease-free equilibrium |
DHF | Dengue hemorrhagic fever |
DSS | Dengue shock syndrome |
EE | Endemic equilibrium |
EIP | Extrinsic incubation period |
GAS | Globally-asymptotically stable |
HMM | Hidden Markov Model |
IIP | Intrinsic incubation period |
LAS | Locally-asymptotically stable |
MLE | Maximum likelihood estimates |
MLL | Maximum log-likelihood |
NB | Negative binomial (distribution) |
ODE | Ordinary differential equation |
POMP | partially observed Markov process |
PRCC | Partial rank correlation coefficient |
Zhen Jin is partly supported by Shanxi Scientific and Technology Innovation Team (201705D15111172 & 201805D131012-1). We thank reviewers for helpful comments.
The authors declare that they have no conflict of interest in this paper.
Proof. Define a Lyapunov function as follows
V(t)=J1(Sh−S∗h−S∗hlnShS∗h)+J2(Eh−E∗h−E∗hlnEhE∗h)+J3(Ah−A∗h−A∗hlnAhA∗h)+J4(Ih1−I∗h1−I∗h1lnIh1I∗h1)+J5(Ih2−I∗h2−I∗h2lnIh2I∗h2)+K1(Sm−S∗m−S∗mlnSmS∗m)+K2(Em−E∗m−E∗mlnEmE∗m)+K3(Im−I∗m−I∗mlnImI∗m), | (A1-1) |
where J1=2, J2=11−θ, J3=1(θ), J4=λ∗hS∗hvhE∗h, J5=λ∗hS∗h(1−η)γ1I∗h1, K1=K2=1, and K3=λ∗mS∗mσmE∗m.
Thus, the Lyapunov derivative computed along solutions of the system (2.1) is given by
˙V(t)=J1(1−S∗hSh)˙Sh+J2(1−A∗hAh)˙Ah+J3(1−E∗hEh)˙Eh+J4(1−I∗h1Ih1)˙Ih1+J5(1−I∗h2Ih2)˙Ih2+K1(1−S∗mSm)˙Sm+K2(1−E∗mEm)˙Em+K3(1−I∗mIm)˙Im. | (A1-2) |
Hence,
˙V(t)=−J1μh(Sh−S∗h)2Sh+J1λ∗hS∗h[1−λhShλ∗hS∗h−S∗hSh+λhλ∗h]+J2(1−θ)λ∗hS∗h[λhShλ∗hS∗h−AhA∗h−A∗hλhShAhλ∗hS∗h+1]+J3θλ∗hS∗h[λhShλ∗hS∗h−EhE∗h−E∗hλhShEhλ∗hS∗h+1]+J4vhE∗m[EhE∗h−Ih1I∗h1−I∗h1EhIh1E∗h+1]+J5(1−η)γ1Ihh1∗[Ih1I∗h1−Ih2I∗h2−I∗h2Ih1Ih2I∗h1+1]+−K1μm(Sm−S∗m)2Sm+K1λ∗mS∗m[1−λmSmλ∗mS∗m−S∗mSm+λmλ∗m]+K2λ∗mS∗m[λmSmλ∗mS∗m−EmE∗m−E∗mλmSmEmλ∗mS∗m+1]+K3σmE∗m[EmE∗m−ImI∗m−I∗mEmImE∗m+1]. | (A1-3) |
Following [50,51], the function v(x)=1−x+lnx, if x>0, it leads to v(x)⩽0. And if x=1, we have v(x)=0. Thus, x−1⩾ln(x) for any x>0. Then, we also have that
λ∗hS∗h[2−S∗hSh−EhE∗h−λhShE∗hλ∗hS∗hEh+λhλ∗h]=λ∗hS∗h[−(1−λhλ∗h)(1−Ih1λ∗hI∗λh)+3−S∗hSh−λhShE∗hλ∗hS∗hEh−Ih1λ∗I∗h1λh−EhE∗h+Ih1I∗h1]⩽λ∗hS∗h[−(S∗hSh−1)−(λhShE∗hλ∗hS∗hEh−1)−(Ih1λ∗hI∗h1λh1−1)−EhE∗h+Ih1I∗h1]⩽λ∗hS∗h[−ln(S∗hShλhShE∗hλ∗S∗hEhIh1λ∗hI∗h1λh)−EhE∗h+Ih1I∗h1]=λ∗hS∗h[Ih1I∗h1−ln(Ih1I∗h1)+ln(EhE∗h)−EhE∗h]. | (A1-4) |
Similarly, we have
λ∗hS∗h[2−S∗hSh−AhA∗h−λhShA∗hλ∗hS∗hAh+λhλ∗h]⩽λ∗hS∗h[Ih2I∗h2−ln(Ih2I∗h2)+ln(AhA∗h)−AhA∗h], | (A1-5) |
and
λ∗mS∗m[2−S∗mSm−EmE∗m−λmSmE∗mλ∗mS∗mEm+λmλ∗m]⩽λ∗mS∗m[ImI∗m−ln(ImI∗m)+ln(EmE∗m)−EmE∗m]. | (A1-6) |
Furthermore, we have that
λ∗hS∗h(EhE∗h−Ih1I∗h1−I∗h1EhIh1E∗h+1)⩽λ∗hS∗h(EhE∗h−ln(EhE∗h)+ln(Ih1I∗h1)−Ih1I∗h1), | (A1-7) |
and also
λ∗hS∗h(Ih1I∗h1−Ih2I∗h2−I∗h2Ih1Ih2I∗h1+1)⩽λ∗hS∗h(Ih1I∗h1−ln(Ih1I∗h1)+ln(Ih2I∗h2)−Ih2I∗h2), | (A1-8) |
as well as
λ∗mS∗m(EmE∗m−ImI∗m−I∗mEmImE∗m+1)⩽λ∗mS∗m(EmE∗m−ln(EmE∗m)+ln(ImI∗m)−ImI∗m). | (A1-9) |
Hence, the equations (A1-1)-(A1-9) together with condition (3.8) ensure that dVdt⩽0. Furthermore, the strict inequality dVdt=0 holds only for Sh=S∗h, Eh=E∗h, Ah=A∗h, Ih1=I∗h1, Ih2=I∗h2, Rh=R∗h, Sm=S∗m Em=E∗m and Im=I∗m. Thus, the endemic equilibrium state E∗ is the only positive invariant set to the system (2.1) contained entirely in {(Sh,Eh,Ah,Ih1,Ih2,Rh,Sm,Em,Im)∈Ω:Sh=S∗h,Eh=E∗h,Ah=A∗h,Ih1=I∗h1,Ih2=I∗h2,Rh=R∗h,Sm=S∗m,Em=E∗m,Im=I∗m\bigamma}. Therefore, it follows from the LaSalle's invariance principle [52] that every solutions to the equations in (A1-2) with initial conditions in Ω converge to stable endemic equilibrium point, E∗, as t→∞. Hence, the positive endemic equilibrium is globally asymptotically stable.
Parameter | Value (Kaohsiung) | Value (Tainan) | Source |
Sh(0) | 0.93212 | 0.8774 | estimated |
Eh(0) | 3.229552×10−6 | 3.241949×10−6 | estimated |
Ah(0) | 3.229552×10−6 | 3.241949×10−6 | estimated |
Ih1(0) | 3.229552×10−6 | 3.241949×10−6 | estimated |
Ih1(0) | 3.229552×10−6 | 3.241949×10−6 | estimated |
Rh(0) | 0.0688 | 0.1226 | estimated |
β | 1×10−20 | 1×10−20 | estimated |
ρ | 0.0631 | 0.0631 | [53,54,55] |
nm | 9 | 9 | estimated |
Parameter | Value (Kaohsiung) | Value (Tainan) | Source |
Sh(0) | 0.9483 | 0.9161 | estimated |
Eh(0) | 2.773339×10−6 | 3.24338×10−6 | estimated |
Ah(0) | 2.773339×10−6 | 3.24338×10−6 | estimated |
Ih1(0) | 2.773339×10−6 | 3.24338×10−6 | estimated |
Ih1(0) | 2.773339×10−6 | 3.24338×10−6 | estimated |
Rh(0) | 0.0517 | 0.0839 | estimated |
β | 1.9986 | 1.9986 | estimated |
ρ | 0.0837 | 0.0837 | estimated |
[1] |
Abdymomunov A (2013) Regime-switching measure of systemic financial stress. Ann Finance 9: 455–470. doi: 10.1007/s10436-012-0194-1
![]() |
[2] |
Acharya V, Engle R, Pierret D (2014) Testing macroprudential stress tests: The risk of regulatory risk weights. J Monetary Econ 65: 36–53. doi: 10.1016/j.jmoneco.2014.04.014
![]() |
[3] | Adrian T, Brunnermeier MK (2011) CoVaR. NBER, Working Paper, 17454. |
[4] | Aikman D, Kiley M, Lee SJ, et al. (2017) Mapping heat in the U.S. financial system. J Banking Financ 81: 36–64. |
[5] |
Aldasoro I, Angeloni I (2015) Input-output-based measures of systemic importance. Quant Financ 15: 589–606. doi: 10.1080/14697688.2014.968194
![]() |
[6] | Anginer D, Demirguc-Kunt A, Zhu M (2014) How does competition affect bank systemic risk? J Financ Int 23: 1–26. |
[7] |
Baek S, Cursio JD, Cha SY (2015) Nonparametric Factor Analytic Risk Measurement in Common Stocks in Financial Firms: Evidence from Korean Firms. Asia-Pac J Financ Stud 44: 497–536. doi: 10.1111/ajfs.12098
![]() |
[8] |
Baglioni A, Cherubini U (2013) Marking-to-market government guarantees to financial systems-Theory and evidence for Europe. J Int Money and Financ 32: 990–1007. doi: 10.1016/j.jimonfin.2012.08.004
![]() |
[9] | Banulescu GD, Dumitrescu EI (2015) Which are the SIFIs? A Component Expected Shortfall approach to systemic risk. J Banking Financ 50: 575–588. |
[10] | Barth JR, Wihlborg C (2017) Too big to fail: Measures, remedies, and consequences for efficiency and stability. Financ Mark Inst Instrum 26: 175–245. |
[11] |
Battaglia F, Gallo A (2013) Securitization and systemic risk: An empirical investigation on Italian banks over the financial crisis. Int Rev Financ Anal 30: 274–286. doi: 10.1016/j.irfa.2013.03.002
![]() |
[12] | Battaglia F, Gallo A (2017) Strong boards, ownership concentration and EU banks' systemic risk-taking: Evidence from the financial crisis. J Int Financ Mark 46: 128–146. |
[13] | Benoit S (2014) Where is the system? Int Econ 138: 1–27. |
[14] | Berger D, Pukthuanthong K (2012) Market fragility and international market crashes. J Financ Econ 105: 565–580. |
[15] | Bernal O, Gnabo JY, Guilmin G (2014) Assessing the contribution of banks, insurance and other financial services to systemic risk. J Banking Financ 47: 270–287. |
[16] |
Bernardi M, Maruotti A, Petrella L (2017) Multiple risk measures for multivariate dynamic heavy-tailed models. J Empirical Financ 43: 1–32. doi: 10.1016/j.jempfin.2017.04.005
![]() |
[17] |
Bisias D, Flood M, Lo AW, et al. (2012) A survey of systemic risk analytics. Annu Rev Financ Econ 4: 255–296. doi: 10.1146/annurev-financial-110311-101754
![]() |
[18] |
Bluhm M, Krahnen JP (2014) Systemic risk in an interconnected banking system with endogenous asset markets. J Financ Stab 13: 75–94. doi: 10.1016/j.jfs.2014.04.002
![]() |
[19] | Borri N (2017) Local currency systemic risk. Emerging Mark Rev 34: 1–13. |
[20] | Cai J, Eidam F, Saunders A, et al. (2018) Syndication, interconnectedness, and systemic risk. J Financ Stab 34: 105–120. |
[21] |
Cambón MI, Estévez L (2016) A Spanish Financial Market Stress Index (FMSI). Span Rev Financ Econ 14: 23–41. doi: 10.1016/j.srfe.2016.01.002
![]() |
[22] | Castro C, Ferrari S (2014) Measuring and testing for the systemically important financial institutions. J Empirical Financ 25: 1–14. |
[23] | Cerchiello P, Giudici P (2016) Big data analysis for financial risk management. J Big Data 3: 18. |
[24] |
Cerchiello P, Giudici P, Nicola G (2017) Twitter data models for bank risk contagion. Neurocomputing 264: 50–56. doi: 10.1016/j.neucom.2016.10.101
![]() |
[25] | Chakroun MA, Gallali MI (2017) Contribution of Islamic banks to systemic risk. Int J Banking Accounting 8: 52–92. |
[26] | Chang CW, Li X, Lin EMH, et al. (2017) Systemic risk, interconnectedness, and non-core activities in Taiwan insurance industry. Int Rev Econ Financ 55: 1–12. |
[27] | Cheng F, Wellman MP (2017) Accounting for strategic response in an agent-based model of financial regulation. Acm Conf Econ Comput 2017: 187–204. |
[28] | Chiu WC, Peña JI, Wang CW (2015) Measuring Systemic Risk: Common Factor Exposures and Tail Dependence Effects. Eur Financ Manage 21: 833–866. |
[29] | Cipra T, Hendrych R (2017) Systemic risk in financial risk regulation. Czech J Econ Financ 67: 15–38. |
[30] | Conciarelli A (2014) A New macroprudential tool to assess sources of financial risks: Implied-systemic cost of risks. Int J Financ Econ 19: 74–88. |
[31] | Derbali A (2017) Systemic Risk in the Chinese Financial System: Measuring and Ranking. Chin Econ 50: 34–58. |
[32] | Derbali A, Hallara S (2016) Systemic risk of European financial institutions: Estimation and ranking by the Marginal Expected Shortfall. Res Int Bus Financ 37: 113–134. |
[33] | Dhar V (2013) Data science and prediction. Commun ACM 56: 64–73. |
[34] | Cesare AD, Stork PA, De Vries CG (2012) Risk measures for autocorrelated hedge fund returns. J Financ Econ 13: 868–895. |
[35] | Drakos AA, Kouretas GP (2013) Measuring Systemic Risk in Emerging Markets Using CoVaR. Emerging Markets and the Global Economy: A Handbook, 271–307. |
[36] |
Drakos AA, Kouretas GP (2015) Bank ownership, financial segments and the measurement of systemic risk: An application of CoVaR. Int Rev Econ Financ 40: 127–140. doi: 10.1016/j.iref.2015.02.010
![]() |
[37] | Drehmann M, Tarashev N (2013) Measuring the systemic importance of interconnected banks. J Financ Int 22: 586–607. |
[38] | Foggitt GM, Heymans A, Vuuren GWV, et al. (2017) Measuring the systemic risk in the South African banking sector. South Afr J Econ Manage Sci 20: 1–9. |
[39] |
Garciadeandoain C, Kremer M (2017) Beyond spreads: Measuring sovereign market stress in the euro area. Econ Lett 159: 153–156. doi: 10.1016/j.econlet.2017.06.042
![]() |
[40] | Gauthier C, Lehar A, Souissi M (2012) Macroprudential capital requirements and systemic risk. J Financ Int 21: 594–618. |
[41] |
Girardi G, Ergün AT (2013) Systemic risk measurement: Multivariate GARCH estimation of CoVaR. J Banking Financ 37: 3169–3180. doi: 10.1016/j.jbankfin.2013.02.027
![]() |
[42] |
Giudici P, Parisi L (2017) Sovereign risk in the Euro area: A multivariate stochastic process approach. Quant Financ 17: 1995–2008. doi: 10.1080/14697688.2017.1357968
![]() |
[43] | González-Hermosillo B, Hesse H (2011) Global Market Conditions and Systemic Risk. J Emerging Mark Financ 10: 227–252. |
[44] | Gramlich D, Oet MV, Ong SJ (2017) The contributions to systemic stress of financial interactions between the US and Europe. Eur J Financ 23: 1176–1196. |
[45] | Gray DF, Malone SW (2012) Sovereign and financial-sector risk: Measurement and interactions. Annu Rev Financ Econ 4: 297–312. |
[46] | Hałaj G, Kok C (2013) Assessing interbank contagion using simulated networks. Comput Manage Sci 10: 157–186. |
[47] | Härdle WK, Wang W, Yu L (2016) TENET: Tail–event driven NETwork risk. J Econ 192: 499–513. |
[48] |
Hespeler F, Loiacono G (2017) Monitoring systemic risk in the hedge fund sector. Quant Financ 17: 1859–1883. doi: 10.1080/14697688.2017.1357969
![]() |
[49] |
Hmissi B, Bejaoui A, Snoussi W (2017) On identifying the domestic systemically important banks: The case of Tunisia. Res Int Bus Financ 42: 1343–1354. doi: 10.1016/j.ribaf.2017.07.071
![]() |
[50] |
Huang X, Zhou H, Zhu H (2009) A framework for assessing the systemic risk of major financial institutions. J Banking Financ 33: 2036–2049. doi: 10.1016/j.jbankfin.2009.05.017
![]() |
[51] |
Huang X (2012) Systemic risk contributions. J Financ Serv Res 42: 55–83. doi: 10.1007/s10693-011-0117-8
![]() |
[52] | IJtsma P, Spierdijk L, Shaffer S (2017) The concentration-stability controversy in banking: New evidence from the EU-25. J Financ Stab 33: 273–284. |
[53] | Irresberger F, Bierth C, Weiß GNF (2017) Size is everything: Explaining SIFI designations. Rev Financi Econ 32: 7–19. |
[54] | Jobst AA (2014) Measuring systemic risk-adjusted liquidity (SRL)-a model approach. J Banking Financ 45: 270–287. |
[55] |
Jondeau E, Khalilzadeh A (2017) Collateralization, leverage, and stressed expected loss. J Financ Stab 33: 226–243. doi: 10.1016/j.jfs.2017.01.005
![]() |
[56] | Kanno M (2015a) Assessing systemic risk using interbank exposures in the global banking system. J Financ Stab 20: 105–130. |
[57] | Kanno M (2015b) The network structure and systemic risk in the Japanese interbank market. Jpn World Econ 36: 102–112. |
[58] |
Kanno M (2016) The network structure and systemic risk in the global non-life insurance market. Insur Math Econ 67: 38–53. doi: 10.1016/j.insmatheco.2015.12.004
![]() |
[59] | Karimalis EN, Nomikos NK (2017) Measuring systemic risk in the European banking sector: A copula CoVaR approach. Eur J Financ 24: 1–38. |
[60] | Khiari W, Nachnouchi J (2017) Banks' systemic risk in the Tunisian context: Measures and Determinants. Res Int Bus Financ. |
[61] | Kleinow J, Horsch A, Garcia-Molina M (2017a) Factors driving systemic risk of banks in Latin America. J Econ Financ 41: 211–234. |
[62] | Kleinow J, Moreira F, Strobl S, et al. (2017b) Measuring systemic risk: A comparison of alternative market-based approaches. Financ Res Lett 21: 40–46. |
[63] | Klinger T, Teplý P (2014) Systemic risk of the global banking system-an agent-based network model approach. Prague Econ Pap 23: 24–41. |
[64] | Klinger T, Teplý P (2016) The nexus between systemic risk and sovereign crises. Czech J Econ Financ 66: 50–69. |
[65] | Kreis Y, Leisen DPJ (2016) Systemic risk in a structural model of bank default linkages. J Financ Stab, 1–46. |
[66] | Kubinschi M, Barnea D (2016) Systemic risk impact on economic growth-The case of the CEE countries. Rom J Econ Forecast 19: 79–94. |
[67] | Kupiec PH, Ramirez CD (2013) Bank failures and the cost of systemic risk: Evidence from 1900 to 1930. J Financ Int 22: 285–307. |
[68] | Kupiec P, Güntay L (2016) Testing for Systemic Risk Using Stock Returns. J Financ Serv Res 49: 203–227. |
[69] |
Kurowski ŁK, Rogowicz K (2017) Negative interest rates as systemic risk event. Financ Res Lett 22: 153–157. doi: 10.1016/j.frl.2017.04.001
![]() |
[70] |
Lee JH, Ryu J, Tsomocos D (2013) Measures of systemic risk and financial fragility in Korea. Ann Financ 9: 757–786. doi: 10.1007/s10436-012-0218-x
![]() |
[71] | Lee J, Lee DH, Yun SG (2016) Systemic Risk on Trade Credit Systems: With the Tangible Interconnectedness. Comput Econ 51: 1–16. |
[72] |
Leroy A, Lucotte Y (2017) Is there a competition–stability trade–off in European banking? J Int Financ Mark 46: 199–215. doi: 10.1016/j.intfin.2016.08.009
![]() |
[73] | Li F, Perez-Saiz H (2018) Measuring systemic risk across financial market infrastructures. J Financ Stab 34: 1–11. |
[74] |
Liao S, Sojli E, Tham WW (2015) Managing systemic risk in The Netherlands. Int Rev Econ Financ 40: 231–245. doi: 10.1016/j.iref.2015.02.012
![]() |
[75] |
López-Espinosa G, Moreno A, Rubia A, et al. (2015) Systemic risk and asymmetric responses in the financial industry. J Banking Financ 58: 471–485. doi: 10.1016/j.jbankfin.2015.05.004
![]() |
[76] |
López-Espinosa G, Rubia A, Valderrama L, et al. (2013) Good for one, bad for all: Determinants of individual versus systemic risk. J Financ Stab 9: 287–299. doi: 10.1016/j.jfs.2013.05.002
![]() |
[77] |
Ma Y, Chen Y (2014) Financial imbalance index as a new early warning indicator: Methods and applications in the Chinese economy. China World Econ 22: 64–86. doi: 10.1111/cwe.12092
![]() |
[78] | Martinez-Jaramillo S, Alexandrova-Kabadjova B, Bravo-Benitez B, et al. (2014) An empirical study of the Mexican banking system's network and its implications for systemic risk. J Econ Dyn Control 40: 242–265. |
[79] | Martínez-Jaramillo S, Pérez OP, Embriz FA, et al. (2010) Systemic risk, financial contagion and financial fragility. J Econ Dyn Control 34: 2358–2374. |
[80] |
Mayordomo S, Rodriguez-Moreno M, Peña JI (2014) Derivatives holdings and systemic risk in the U.S. banking sector. J Banking Financ 45: 84–104. doi: 10.1016/j.jbankfin.2014.03.037
![]() |
[81] |
Mendonça HF, Silva RBD (2018) Effect of banking and macroeconomic variables on systemic risk: An application of Δ COVAR for an emerging economy. North Am J Econ Financ 43: 141–157. doi: 10.1016/j.najef.2017.10.011
![]() |
[82] | Mensah JO, Premaratne G (2017) Systemic interconnectedness among Asian Banks. Jpn World Econ 41: 17–33. |
[83] | Mezei J, Sarlin P (2016) Aggregating expert knowledge for the measurement of systemic risk. Decis Support Syst 88: 38–50. |
[84] |
Mezei J, Sarlin P (2018) RiskRank: Measuring interconnected risk. Econ Modell 68: 41–50. doi: 10.1016/j.econmod.2017.04.016
![]() |
[85] | Milne A (2014) Distance to default and the financial crisis. J Financ Stab 12: 26–36. |
[86] | Nucera F, Lucas A, et al. (2017) Do negative interest rates make banks less safe? Econ Lett 159: 112–115. |
[87] |
Patro DK, Qi M, Sun X (2013) A simple indicator of systemic risk. J Financ Stab 9: 105–116. doi: 10.1016/j.jfs.2012.03.002
![]() |
[88] |
Pederzoli C, Torricelli C (2017) Systemic risk measures and macroprudential stress tests: An assessment over the 2014 EBA exercise. Ann Financ 13: 237–251. doi: 10.1007/s10436-017-0294-z
![]() |
[89] | Popescu A, Turcu C (2014) Systemic sovereign risk in Europe: An MES and CES approach. Work Pap 124: 899–925. |
[90] |
Popescu A, Turcu C (2017) Sovereign debt and systemic risk in the eurozone: A macroeconomic perspective. Econ Modell 67: 275–284. doi: 10.1016/j.econmod.2016.12.032
![]() |
[91] |
Reboredo JC, Ugolini A (2015) Systemic risk in European sovereign debt markets: A CoVaR-copula approach. J Int Money Financ 51: 214–244. doi: 10.1016/j.jimonfin.2014.12.002
![]() |
[92] | Reboredo JC, Ugolini A (2016) Systemic risk of Spanish listed banks: A vine copula CoVaR approach [Riesgo sistémico de los bancos españoles cotizados: Una aproximación CoVaR con cópulas vine]. Span J Financ Accounting 45: 1–31. |
[93] |
Rönnqvist S, Sarlin P (2015) Bank networks from text: Interrelations, centrality and determinants. Quant Financ 15: 1619–1635. doi: 10.1080/14697688.2015.1071076
![]() |
[94] | Rösch D, Scheule H (2016) The role of loan portfolio losses and bank capital for Asian financial system resilience. Pac-Basin Financ J 40: 289–305. |
[95] |
Sedunov J (2016) What is the systemic risk exposure of financial institutions? J Financ Stab 24: 71–87. doi: 10.1016/j.jfs.2016.04.005
![]() |
[96] | Sheu HJ, Cheng CL (2012) Systemic risk in Taiwan stock market. J Bus Econ Manage 13: 895–914. |
[97] |
Siebenbrunner C, Sigmund M, Kerbl S (2017) Can bank-specific variables predict contagion effects? Quant Financ 17: 1805–1832. doi: 10.1080/14697688.2017.1357974
![]() |
[98] |
Silva TC, Souza SRSD, Tabak BM (2017) Monitoring vulnerability and impact diffusion in financial networks. J Econ Dyn Control 76: 109–135. doi: 10.1016/j.jedc.2017.01.001
![]() |
[99] |
Souza SRSD, Silva TC, Tabak BM, et al. (2016) Evaluating systemic risk using bank default probabilities in financial networks. J Econ Dyn Control 66: 54–75. doi: 10.1016/j.jedc.2016.03.003
![]() |
[100] |
Staum J (2012) Systemic risk components and deposit insurance premia. Quant Financ 12: 651–662. doi: 10.1080/14697688.2012.664942
![]() |
[101] | Stolbov M, Shchepeleva M (2017) Systemic risk in Europe: Deciphering leading measures, common patterns and real effects. Ann Financ 14: 1–43. |
[102] |
Strobl S (2016) Stand-alone vs systemic risk-taking of financial institutions. J Risk Financ 17: 374–389. doi: 10.1108/JRF-05-2016-0064
![]() |
[103] |
Trabelsi N, Naifar N (2017) Are Islamic stock indexes exposed to systemic risk? Multivariate GARCH estimation of CoVaR. Res Int Bus Financ 42: 727–744. doi: 10.1016/j.ribaf.2017.07.013
![]() |
[104] |
Xu S, In F, Forbes C, et al. (2017) Systemic risk in the European sovereign and banking system. Quant Financ 17: 633–656. doi: 10.1080/14697688.2016.1205212
![]() |
[105] |
Yao Y, Li J, Zhu X, et al. (2017) Expected default based score for identifying systemically important banks. Econ Modell 64: 589–600. doi: 10.1016/j.econmod.2017.04.023
![]() |
[106] |
Yun J, Moon H (2014) Measuring systemic risk in the Korean banking sector via dynamic conditional correlation models. Pac-Basin Financ J 27: 94–114. doi: 10.1016/j.pacfin.2014.02.005
![]() |
1. | Salihu S. Musa, Shi Zhao, Daozhou Gao, Qianying Lin, Gerardo Chowell, Daihai He, Mechanistic modelling of the large-scale Lassa fever epidemics in Nigeria from 2016 to 2019, 2020, 493, 00225193, 110209, 10.1016/j.jtbi.2020.110209 | |
2. | Qianying Lin, Salihu S. Musa, Shi Zhao, Daihai He, Modeling the 2014–2015 Ebola Virus Disease Outbreaks in Sierra Leone, Guinea, and Liberia with Effect of High- and Low-risk Susceptible Individuals, 2020, 82, 0092-8240, 10.1007/s11538-020-00779-y | |
3. | Shi Zhao, Salihu S. Musa, Jay T. Hebert, Peihua Cao, Jinjun Ran, Jiayi Meng, Daihai He, Jing Qin, Modelling the effective reproduction number of vector-borne diseases: the yellow fever outbreak in Luanda, Angola 2015–2016 as an example, 2020, 8, 2167-8359, e8601, 10.7717/peerj.8601 | |
4. | Akhil Kumar Srivastav, Pankaj Kumar Tiwari, Mini Ghosh, Modeling the impact of early case detection on dengue transmission: deterministic vs. stochastic, 2020, 0736-2994, 1, 10.1080/07362994.2020.1804403 | |
5. | Abdullahi Yusuf, Bahar Acay, Umar Tasiu Mustapha, Mustafa Inc, Dumitru Baleanu, Mathematical modeling of pine wilt disease with Caputo fractional operator, 2021, 143, 09600779, 110569, 10.1016/j.chaos.2020.110569 | |
6. | Salihu Sabiu Musa, Shi Zhao, Daihai He, Chao Liu, The Long-Term Periodic Patterns of Global Rabies Epidemics Among Animals: A Modeling Analysis, 2020, 30, 0218-1274, 2050047, 10.1142/S0218127420500479 | |
7. | Salihu Sabiu Musa, Sania Qureshi, Shi Zhao, Abdullahi Yusuf, Umar Tasiu Mustapha, Daihai He, Mathematical modeling of COVID-19 epidemic with effect of awareness programs, 2021, 6, 24680427, 448, 10.1016/j.idm.2021.01.012 | |
8. | Rahul Subramanian, Victoria Romeo-Aznar, Edward Ionides, Claudia T. Codeço, Mercedes Pascual, Predicting re-emergence times of dengue epidemics at low reproductive numbers: DENV1 in Rio de Janeiro, 1986–1990, 2020, 17, 1742-5689, 20200273, 10.1098/rsif.2020.0273 | |
9. | Yongli Cai, Shi Zhao, Yun Niu, Zhihang Peng, Kai Wang, Daihai He, Weiming Wang, Modelling the effects of the contaminated environments on tuberculosis in Jiangsu, China, 2021, 508, 00225193, 110453, 10.1016/j.jtbi.2020.110453 | |
10. | Daihai He, Shi Zhao, Qianying Lin, Salihu S. Musa, Lewi Stone, Christopher M. Barker, New estimates of the Zika virus epidemic attack rate in Northeastern Brazil from 2015 to 2016: A modelling analysis based on Guillain-Barré Syndrome (GBS) surveillance data, 2020, 14, 1935-2735, e0007502, 10.1371/journal.pntd.0007502 | |
11. | G. Udhaya Sankar, C. Ganesa Moorthy, 2020, chapter 4, 9781799821977, 64, 10.4018/978-1-7998-2197-7.ch004 | |
12. | Salihu Sabiu Musa, Shi Zhao, Nafiu Hussaini, Abdulrazaq Garba Habib, Daihai He, Mathematical modeling and analysis of meningococcal meningitis transmission dynamics, 2020, 13, 1793-5245, 2050006, 10.1142/S1793524520500060 | |
13. | Salihu Sabiu Musa, Shi Zhao, Nafiu Hussaini, Salisu Usaini, Daihai He, Dynamics analysis of typhoid fever with public health education programs and final epidemic size relation, 2021, 10, 25900374, 100153, 10.1016/j.rinam.2021.100153 | |
14. | Clarisse Lins de Lima, Ana Clara Gomes da Silva, Cecilia Cordeiro da Silva, Giselle Machado Magalhães Moreno, Abel Guilhermino da Silva Filho, Anwar Musah, Aisha Aldosery, Livia Dutra, Tercio Ambrizzi, Iuri Valério Graciano Borges, Merve Tunali, Selma Basibuyuk, Orhan Yenigün, Tiago Lima Massoni, Kate Jones, Luiza Campos, Patty Kostkova, Wellington P. dos Santos, 2022, Chapter 17, 978-3-030-79752-2, 299, 10.1007/978-3-030-79753-9_17 | |
15. | Umar T. Mustapha, Salihu S. Musa, Muhammad A. Lawan, Aliyu Abba, Evren Hincal, Musa D. Mohammed, Bashir D. Garba, Rabiu B. Yunus, Shehu A. Adamu, Daihai He, Mathematical modeling and analysis of schistosomiasis transmission dynamics, 2021, 12, 1793-9623, 2150021, 10.1142/S1793962321500215 | |
16. | Salihu S. Musa, Isa A. Baba, Abdullahi Yusuf, Tukur A. Sulaiman, Aliyu I. Aliyu, Shi Zhao, Daihai He, Transmission dynamics of SARS-CoV-2: A modeling analysis with high-and-moderate risk populations, 2021, 26, 22113797, 104290, 10.1016/j.rinp.2021.104290 | |
17. | Bilgen Kaymakamzade, Evren Hincal, Nezihal Gokbulut, Tamer Sanlidag, 2022, Chapter 8, 978-3-030-96681-2, 65, 10.1007/978-3-030-96682-9_8 | |
18. | Javier Armando Gutierrez, Karina Laneri, Juan Pablo Aparicio, Gustavo Javier Sibona, Meteorological indicators of dengue epidemics in non-endemic Northwest Argentina, 2022, 7, 24680427, 823, 10.1016/j.idm.2022.10.004 | |
19. | Afeez Abidemi, Hammed Olawale Fatoyinbo, Joshua Kiddy K. Asamoah, Sishu Shankar Muni, 2022, Evaluation of the Efficacy of Wolbachia Intervention on Dengue Burden in a Population: A Mathematical Insight, 978-1-6654-9501-1, 1618, 10.1109/DASA54658.2022.9765106 | |
20. | Emmanuelle Sylvestre, Clarisse Joachim, Elsa Cécilia-Joseph, Guillaume Bouzillé, Boris Campillo-Gimenez, Marc Cuggia, André Cabié, Victor S. Santos, Data-driven methods for dengue prediction and surveillance using real-world and Big Data: A systematic review, 2022, 16, 1935-2735, e0010056, 10.1371/journal.pntd.0010056 | |
21. | RUBAYYI T. ALQAHTANI, ABDULLAHI YUSUF, DEVELOPMENT AND ANALYSIS OF A SEIR MODEL FOR COVID-19 EPIDEMIC WITH VACCINATION AND NONSINGULAR KERNEL, 2022, 30, 0218-348X, 10.1142/S0218348X22400400 | |
22. | Salihu S. Musa, Abdullahi Yusuf, Emmanuel A. Bakare, Zainab U. Abdullahi, Lukman Adamu, Umar T. Mustapha, Daihai He, Unravelling the dynamics of Lassa fever transmission with differential infectivity: Modeling analysis and control strategies, 2022, 19, 1551-0018, 13114, 10.3934/mbe.2022613 | |
23. | Abdurrahman Abdulhamid, Nafiu Hussaini, Salihu S. Musa, Daihai He, Mathematical analysis of Lassa fever epidemic with effects of environmental transmission, 2022, 35, 22113797, 105335, 10.1016/j.rinp.2022.105335 | |
24. | Ziyue Yuan, Salihu Sabiu Musa, Shu-Chien Hsu, Clara Man Cheung, Daihai He, Post pandemic fatigue: what are effective strategies?, 2022, 12, 2045-2322, 10.1038/s41598-022-13597-0 | |
25. | Ziyue Yuan, Shu-Chien Hsu, Clara Man Cheung, Vahid Asghari, Effectiveness of Interventions for Controlling COVID-19 Transmission between Construction Workers and Their Close Contacts, 2022, 38, 0742-597X, 10.1061/(ASCE)ME.1943-5479.0001033 | |
26. | Mohammed M. Al-Shomrani, Salihu S. Musa, Abdullahi Yusuf, Unfolding the Transmission Dynamics of Monkeypox Virus: An Epidemiological Modelling Analysis, 2023, 11, 2227-7390, 1121, 10.3390/math11051121 | |
27. | Xin-You Meng, Chong-Yang Yin, DYNAMICS OF A DENGUE FEVER MODEL WITH UNREPORTED CASES AND ASYMPTOMATIC INFECTED CLASSES IN SINGAPORE, 2020, 2023, 13, 2156-907X, 782, 10.11948/20220111 | |
28. | Alhassan Ibrahim, Usa Wannasingha Humphries, Parinya Sa Ngiamsunthorn, Isa Abdullahi Baba, Sania Qureshi, Amir Khan, Modeling the dynamics of COVID-19 with real data from Thailand, 2023, 13, 2045-2322, 10.1038/s41598-023-39798-9 | |
29. | Abdoulaye Sow, Cherif Diallo, Hocine Cherifi, Benny Yong, Interplay between vaccines and treatment for dengue control: An epidemic model, 2024, 19, 1932-6203, e0295025, 10.1371/journal.pone.0295025 | |
30. | Nawaf L. Alsowait, Mohammed M. Al-Shomrani, Ismail Abdulrashid, Salihu S. Musa, Synergistic Impact of Active Case Detection and Early Hospitalization for Controlling the Spread of Yellow Fever Outbreak in Nigeria: An Epidemiological Modeling and Optimal Control Analysis, 2024, 12, 2227-7390, 3817, 10.3390/math12233817 |
Variable | Interpretation |
Nh(t) | Total population of humans |
Sh(t) | Population of susceptible humans |
Eh(t) | Population of humans exposed to DENV |
Ah(t) | Population of asymptomatic humans |
Ih1(t) | Population of humans with clinical symptoms of the DENV |
Ih2(t) | Population of humans with severe clinical symptoms of the DENV |
Rh(t) | Population of humans recovered from the DENV |
Nm(t) | Total population of adult female mosquitoes |
Sm(t) | population of susceptible adult mosquitoes |
Em(t) | population of exposed adult mosquitoes |
Im(t) | population of infectious adult mosquitoes |
Parameter | Interpretation/Description |
Πh/Πm | Recruitment rate of humans/mosquitoes |
μh | Natural death rate of humans |
μm | Death rate of female adult mosquitoes |
βh | Transmission probability from infectious mosquitoes to susceptible humans |
βm | Transmission probability from infectious humans to susceptible mosquitoes |
a | Mosquito biting rate |
b | Maximum number of bites a human can receive per unit time |
θ | Fraction of infected humans that are exposed |
vh | Progression rate of exposed humans to infectious humans with clinical symptoms |
η | Fraction of infectious humans that will not get a severe symptom |
γh, γ1, γ2 | Recovery rate of infectious humans from Ah, Ih1, Ih2, respectively |
δh | Disease-induced death rate of humans |
σm | Progression rate of exposed mosquitoes to the infectious mosquitoes |
ρ | Reporting rate to actual case ratio |
m | Average mosquito to human ratio |
Parameter | Baseline [Range] | Units | Source |
Πh | 2.5 [1,5] | person~day−1 | [6] |
Πm | 5000 [1000,6000] | Day−1 | estimated from [6] |
μh | 3.9×10−5 [3.6×10−5, 4.0×10−5] | Day−1 | [12] |
μm | 0.05714 [0.01,0.1] | Day−1 | [11] |
βh | 0.75 [0.1,0.95] | Day−1 | [6] |
βm | 0.75 [0.1,0.95] | Day−1 | [6] |
a | 0.4997 [0.1,1] | Day−1 | [13,14] |
b | 0.4 [0.1,1] | Day−1 | [13] |
θ | 0.18 [0.1,0.6] | Dimensionless | [31] |
vh | 0.0666 [0,1] | Day−1 | [6,32] |
η | 0.1 [0.05,0.15] | Dimensionless | [19,33] |
γh | 0.1428 [0.1,0.2] | Day−1 | [13] |
γ1 | 0.2 [0.1428,0.3] | Day−1 | [13] |
γ2 | 0.05 [0.0333,0.07143] | Day−1 | [13] |
δh | 10−3 [10−4,2×10−3] | Day−1 | [6] |
σm | 0.5 [0,1] | Day−1 | [6] |
m | 10 [1,20] | Dimensionless | assumed |
abbreviation | explanation / full name |
AICc | corrected Akaike's Information Criterion |
BB | Backward bifurcation |
CI | Confidence interval |
DENV | Degue virus |
DENV-1 | Dengue virus serotype 1 |
DENV-2 | Dengue virus serotype 2 |
DENV-3 | Dengue virus serotype 3 |
DENV-4 | Dengue virus serotype 4 |
DFE | Disease-free equilibrium |
DHF | Dengue hemorrhagic fever |
DSS | Dengue shock syndrome |
EE | Endemic equilibrium |
EIP | Extrinsic incubation period |
GAS | Globally-asymptotically stable |
HMM | Hidden Markov Model |
IIP | Intrinsic incubation period |
LAS | Locally-asymptotically stable |
MLE | Maximum likelihood estimates |
MLL | Maximum log-likelihood |
NB | Negative binomial (distribution) |
ODE | Ordinary differential equation |
POMP | partially observed Markov process |
PRCC | Partial rank correlation coefficient |
Parameter | Value (Kaohsiung) | Value (Tainan) | Source |
Sh(0) | 0.93212 | 0.8774 | estimated |
Eh(0) | 3.229552×10−6 | 3.241949×10−6 | estimated |
Ah(0) | 3.229552×10−6 | 3.241949×10−6 | estimated |
Ih1(0) | 3.229552×10−6 | 3.241949×10−6 | estimated |
Ih1(0) | 3.229552×10−6 | 3.241949×10−6 | estimated |
Rh(0) | 0.0688 | 0.1226 | estimated |
β | 1×10−20 | 1×10−20 | estimated |
ρ | 0.0631 | 0.0631 | [53,54,55] |
nm | 9 | 9 | estimated |
Parameter | Value (Kaohsiung) | Value (Tainan) | Source |
Sh(0) | 0.9483 | 0.9161 | estimated |
Eh(0) | 2.773339×10−6 | 3.24338×10−6 | estimated |
Ah(0) | 2.773339×10−6 | 3.24338×10−6 | estimated |
Ih1(0) | 2.773339×10−6 | 3.24338×10−6 | estimated |
Ih1(0) | 2.773339×10−6 | 3.24338×10−6 | estimated |
Rh(0) | 0.0517 | 0.0839 | estimated |
β | 1.9986 | 1.9986 | estimated |
ρ | 0.0837 | 0.0837 | estimated |
Variable | Interpretation |
Nh(t) | Total population of humans |
Sh(t) | Population of susceptible humans |
Eh(t) | Population of humans exposed to DENV |
Ah(t) | Population of asymptomatic humans |
Ih1(t) | Population of humans with clinical symptoms of the DENV |
Ih2(t) | Population of humans with severe clinical symptoms of the DENV |
Rh(t) | Population of humans recovered from the DENV |
Nm(t) | Total population of adult female mosquitoes |
Sm(t) | population of susceptible adult mosquitoes |
Em(t) | population of exposed adult mosquitoes |
Im(t) | population of infectious adult mosquitoes |
Parameter | Interpretation/Description |
Πh/Πm | Recruitment rate of humans/mosquitoes |
μh | Natural death rate of humans |
μm | Death rate of female adult mosquitoes |
βh | Transmission probability from infectious mosquitoes to susceptible humans |
βm | Transmission probability from infectious humans to susceptible mosquitoes |
a | Mosquito biting rate |
b | Maximum number of bites a human can receive per unit time |
θ | Fraction of infected humans that are exposed |
vh | Progression rate of exposed humans to infectious humans with clinical symptoms |
η | Fraction of infectious humans that will not get a severe symptom |
γh, γ1, γ2 | Recovery rate of infectious humans from Ah, Ih1, Ih2, respectively |
δh | Disease-induced death rate of humans |
σm | Progression rate of exposed mosquitoes to the infectious mosquitoes |
ρ | Reporting rate to actual case ratio |
m | Average mosquito to human ratio |
Parameter | Baseline [Range] | Units | Source |
Πh | 2.5 [1,5] | person~day−1 | [6] |
Πm | 5000 [1000,6000] | Day−1 | estimated from [6] |
μh | 3.9×10−5 [3.6×10−5, 4.0×10−5] | Day−1 | [12] |
μm | 0.05714 [0.01,0.1] | Day−1 | [11] |
βh | 0.75 [0.1,0.95] | Day−1 | [6] |
βm | 0.75 [0.1,0.95] | Day−1 | [6] |
a | 0.4997 [0.1,1] | Day−1 | [13,14] |
b | 0.4 [0.1,1] | Day−1 | [13] |
θ | 0.18 [0.1,0.6] | Dimensionless | [31] |
vh | 0.0666 [0,1] | Day−1 | [6,32] |
η | 0.1 [0.05,0.15] | Dimensionless | [19,33] |
γh | 0.1428 [0.1,0.2] | Day−1 | [13] |
γ1 | 0.2 [0.1428,0.3] | Day−1 | [13] |
γ2 | 0.05 [0.0333,0.07143] | Day−1 | [13] |
δh | 10−3 [10−4,2×10−3] | Day−1 | [6] |
σm | 0.5 [0,1] | Day−1 | [6] |
m | 10 [1,20] | Dimensionless | assumed |
abbreviation | explanation / full name |
AICc | corrected Akaike's Information Criterion |
BB | Backward bifurcation |
CI | Confidence interval |
DENV | Degue virus |
DENV-1 | Dengue virus serotype 1 |
DENV-2 | Dengue virus serotype 2 |
DENV-3 | Dengue virus serotype 3 |
DENV-4 | Dengue virus serotype 4 |
DFE | Disease-free equilibrium |
DHF | Dengue hemorrhagic fever |
DSS | Dengue shock syndrome |
EE | Endemic equilibrium |
EIP | Extrinsic incubation period |
GAS | Globally-asymptotically stable |
HMM | Hidden Markov Model |
IIP | Intrinsic incubation period |
LAS | Locally-asymptotically stable |
MLE | Maximum likelihood estimates |
MLL | Maximum log-likelihood |
NB | Negative binomial (distribution) |
ODE | Ordinary differential equation |
POMP | partially observed Markov process |
PRCC | Partial rank correlation coefficient |
Parameter | Value (Kaohsiung) | Value (Tainan) | Source |
Sh(0) | 0.93212 | 0.8774 | estimated |
Eh(0) | 3.229552×10−6 | 3.241949×10−6 | estimated |
Ah(0) | 3.229552×10−6 | 3.241949×10−6 | estimated |
Ih1(0) | 3.229552×10−6 | 3.241949×10−6 | estimated |
Ih1(0) | 3.229552×10−6 | 3.241949×10−6 | estimated |
Rh(0) | 0.0688 | 0.1226 | estimated |
β | 1×10−20 | 1×10−20 | estimated |
ρ | 0.0631 | 0.0631 | [53,54,55] |
nm | 9 | 9 | estimated |
Parameter | Value (Kaohsiung) | Value (Tainan) | Source |
Sh(0) | 0.9483 | 0.9161 | estimated |
Eh(0) | 2.773339×10−6 | 3.24338×10−6 | estimated |
Ah(0) | 2.773339×10−6 | 3.24338×10−6 | estimated |
Ih1(0) | 2.773339×10−6 | 3.24338×10−6 | estimated |
Ih1(0) | 2.773339×10−6 | 3.24338×10−6 | estimated |
Rh(0) | 0.0517 | 0.0839 | estimated |
β | 1.9986 | 1.9986 | estimated |
ρ | 0.0837 | 0.0837 | estimated |