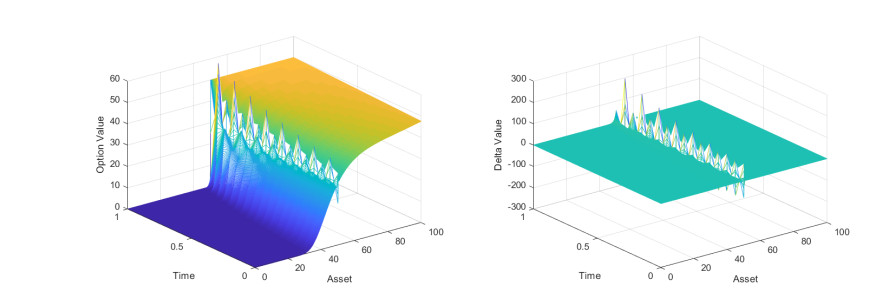
Citation: Rosanna Parlato, Holger Bierhoff. Role of nucleolar dysfunction in neurodegenerative disorders: a game of genes?[J]. AIMS Molecular Science, 2015, 2(3): 211-224. doi: 10.3934/molsci.2015.3.211
[1] | Yiyuan Qian, Haiming Song, Xiaoshen Wang, Kai Zhang . Primal-dual active-set method for solving the unilateral pricing problem of American better-of options on two assets. Electronic Research Archive, 2022, 30(1): 90-115. doi: 10.3934/era.2022005 |
[2] | Yiyuan Qian, Kai Zhang, Jingzhi Li, Xiaoshen Wang . Adaptive neural network surrogate model for solving the implied volatility of time-dependent American option via Bayesian inference. Electronic Research Archive, 2022, 30(6): 2335-2355. doi: 10.3934/era.2022119 |
[3] | Kaiyu Zhang . Sobolev estimates and inverse Hölder estimates on a class of non-divergence variation-inequality problem arising in American option pricing. Electronic Research Archive, 2024, 32(11): 5975-5987. doi: 10.3934/era.2024277 |
[4] | E. A. Abdel-Rehim . The time evolution of the large exponential and power population growth and their relation to the discrete linear birth-death process. Electronic Research Archive, 2022, 30(7): 2487-2509. doi: 10.3934/era.2022127 |
[5] | Shuaikang Wang, Yunzhi Jiang, Yongbin Ge . High-order compact difference methods for solving two-dimensional nonlinear wave equations. Electronic Research Archive, 2023, 31(6): 3145-3168. doi: 10.3934/era.2023159 |
[6] | Li Tian, Ziqiang Wang, Junying Cao . A high-order numerical scheme for right Caputo fractional differential equations with uniform accuracy. Electronic Research Archive, 2022, 30(10): 3825-3854. doi: 10.3934/era.2022195 |
[7] | Yan Dong . Local Hölder continuity of nonnegative weak solutions of inverse variation-inequality problems of non-divergence type. Electronic Research Archive, 2024, 32(1): 473-485. doi: 10.3934/era.2024023 |
[8] | Margarida Camarinha . A natural 4th-order generalization of the geodesic problem. Electronic Research Archive, 2024, 32(5): 3396-3412. doi: 10.3934/era.2024157 |
[9] | Haiyan Song, Fei Sun . A numerical method for parabolic complementarity problem. Electronic Research Archive, 2023, 31(2): 1048-1064. doi: 10.3934/era.2023052 |
[10] | Aya Selmoune, Zhiyuan Liu, Jinwoo Lee . To pay or not to pay? Understanding public acceptance of congestion pricing: A case study of Nanjing. Electronic Research Archive, 2022, 30(11): 4136-4156. doi: 10.3934/era.2022209 |
Assuming that the price of underlying assets satisfies the geometric Brownian motion, the Black-Scholes option pricing model was firstly proposed in 1973 which depends only on the risk-free interest rate and the volatility [1]. The Black-Scholes model quickly attracted a great deal of attention from the communities of both academic researcher and engineer. In order to improve the efficiency of the model and also fit the practical market, a sequence of option pricing models were proposed and studied, for instance, jump-diffusion model [2,3], stochastic volatility model [4,5] and the fractional option pricing models based on Lévy process including of finite moment log stable (FMLS) [6], KoBol [7,8] and CGMY [9] model.
In order to solve the fractional option pricing models based on Lévy process, a number of numerical approaches were proposed and well studied in the past decades. Cont and Voltchkova [10] first presented a finite difference methods for solving the fractional European option pricing model driven by exponential Lévy process and studied the stability and convergence. Then, Cartea and del-Castillo-Negrete [11] rewrote FMLS, CGMY and KoBol option pricing models as a general fractional partial differential equation and studied the shifted Grünwald difference (SGD) formula for the numerical solution. Marom and Momoniat [12] further compared the numerical solutions of three fractional option pricing models based on Lévy process. Chen and Wang [13] developed a numerical scheme with second-order accuracy in both the spatial and time mesh size for pricing European and American option under a geometric Lévy process. Recently, Zhang et al. [14] constructed an implicit numerical scheme with second-order accuracy for FMLS model and used BiCGstab method to solve the discreted linear equations.
As we known, exotic options play important roles and are widely used in the practical finance market [15]. However, it is a great challenge to obtain the solution for traditional numerical methods since the non-smooth payoffs usually lead to serious degradation in the convergence of the numerical schemes and result in inaccurate and discontinuous solution near the strike or barrier. For instance, the well known second-order implicit schemes, Crank-Nicolson method, are prone to spurious oscillations unless the time step size is small enough. To overcome this difficulty, Wade et al. [16] studied the Padé schemes to smooth the Crank-Nicolson scheme to get fourth-order schemes for pricing barrier European option models, see also [15,17,18,19] for the Padé schemes for different exotic option models.
For the fractional exotic options under FMLS model, a class of fourth-order numerical schemes are presented and studied in this paper. We first discretize the fractional option pricing models with weighted and shifted Grünwald difference (WSGD) formula, which is of second-order accuracy in space direction. Then, by making use of the Padé schemes for the time direction, an L-stable and fourth-order accurate scheme is obtained. The convergence of the proposed numerical scheme is proved when the spatial discretization matrix is positive definite and has lower Hessenberg Toeplitz structure, without the assumption of the self-adjoint operator. The proposed method is adapted to be implemented on parallel processors by making use of partial fraction. Numerical experiments on digital option and barrier option are presented to verify the efficiency and accuracy of our numerical schemes.
The structure of this paper is organized as follows. The weighted and shifted Grünwald difference schemes for space discretization is presented in Section 2. Time stepping schemes on Padé approximation and the implementations are presented in Section 3. We then analyze and prove the convergence of the numerical scheme in Section 4. Numerical experiments are given to show the accuracy and efficiency of the proposed schemes in Section 5. Finally, conclusions are drawn in Section 6.
Denote $ S_t $ as the asset price at time $ t $, the FMLS model [6] can be written as follow:
$ ∂V(x,t)∂t+(r−ν)∂V(x,t)∂x+ν⋅−∞DαxV(x,t)=rV(x,t), $
|
(2.1) |
where $ V(x, t) $ is the price of the option at the time $ t $ before the expiry time $ T $, $ x = \ln S_t $, $ _{- \infty}D _ { x } ^ { \alpha } (1 < \alpha < 2) $ is the left Riemann-Liouville derivative [20], $ \nu = - \frac { 1 } { 2 } \sigma ^ { \alpha } \sec \left(\frac { \alpha \pi } { 2 } \right) $, $ S_t $ is the price of underlying asset at time $ t $, $ r $ is the risk free interest rate and $ \sigma $ is the volatility.
In order to solve the FMLS model numerically, we first transform (2.1) into a forward problem by using the transformation $ t^* = T - t $, and then drop $ * $ for simplicity of the notation. Then we truncate the interval of $ x $ to a finite interval $ \left[B_{ d }, B_{ u } \right] $, and consider the following FMLS model for pricing European option
$ ∂V(x,t)∂t=(r−ν)∂V(x,t)∂x+ν⋅BdDαxV(x,t)−rV(x,t),x∈(Bd,Bu),t∈(0,T], $
|
(2.2) |
where the left Riemann-Liouville derivative [20] is defined as
$ BdDαxV(x,t)=1Γ(2−α)d2dx2∫xBdV(η,t)(x−η)α−1dη,1<α<2, $
|
and the initial and boundary conditions are as follows:
$ V(x,0)=v(x),Bd≤x≤Bu,V(Bd,t)=0,V(Bu,t)=B(t),0<t<T. $
|
(2.3) |
Let $ M $ and $ N $ be the number of the uniform discrete points in the space and time direction respectively, $ h = \left(B _ { u } - B _ { d } \right) / M $ and $ \tau = T / N $ be the corresponding step length. Define $ t _ { j } = j \tau (j = 0, 1, 2, \cdots, N), x _ { i } = B _ { d } + i h (i = 0, 1, 2, \cdots, M) $, then the discrete equation can be obtained. We discrete the first order derivative and $ \alpha $ order left Riemann-Liouville fractional derivative by central difference scheme and weighted and shifted Grünwald difference (WSGD) scheme [21] respectively.
The second-order WSGD scheme was first proposed by Tian et al. [21], which is a more general and flexible approach and independent on the changed fractional order. It is further applied into the numerical solution of time fractional sub-diffusion equation [22,23], as well as fractional Black-Schole equation in the FMLS model [14]. Recently Liu et al. [24] developed a class of second-order $ \theta $ schemes based on the WSGD formula for solving the nonlinear fractional cable equation.
Using WSGD scheme, the fractional derivative can be approximated as
$ BdDαxV(xi,t)=1hαi+1∑k=0ω(α)kV(xi−k+1,t)+O(h2), $
|
(2.4) |
where
$ {ω(α)0=α2g(α)0,ω(α)k=α2g(α)k+2−α2g(α)k−1g(α)0=1,g(α)k=(1−α+1k)g(α)k−1,k=1,2,⋯.∑∞k=0g(α)k=0,g(α)k>0,k=0,2,3….g(α)1=−α<0. $
|
(2.5) |
Denote $ V_i^j = V(x_i, t_j), \mathbf { V }_j = \left(V_1^j, V_2^j, \cdots, V_{M-1}^j \right)^T, i = 0, 1, 2, \cdots, M, j = 0, 1, 2, \cdots, N $, then the semidiscretization equation is given by
$ ∂V∂t|(xi,tj)=(r−ν)Vji+1−Vji−12h+νhαi+1∑k=0ω(α)kVji−k+1−rVji, $
|
(2.6) |
where
$ V0i=v(xi),i=1,2,⋯,M−1. $
|
(2.7) |
Denote $ \zeta = \frac { \nu } { h ^ { \alpha } } $, $ \xi = \frac { r - \nu } { 2 h } $, it leads to the following semi-equation
$ ∂V∂t|(xi,tj)+AVj=fj,i=1,2,⋯,M−1, $
|
(2.8) |
where $ \mathbf { A } = r \mathbf { I }-\zeta \mathbf { B } -\xi \mathbf { C} $, $ \mathbf { B } = \left[b _ { i j } \right] _ { (M - 1) \times (M - 1) } $ defined by
$ bij={ω(α)1,i=j,j=1,…,M−1,ω(α)2,i=j+1,j=1,…,M−2,ω(α)0,i=j−1,j=2,…,M−1,ω(α)i−j+1,i−j≥2,j=1,…,M−3,0,otherwise, $
|
(2.9) |
$ \mathbf { C } = {\rm tridiag }\{ - 1, 0, 1 \} $ and $ {f}_j = (0, 0, \cdots, 0, (\xi + \zeta \omega_0^{(\alpha)}) ({ B(t_{j+1})+B(t_{j})}) $. Since both the matrices $ \mathbf{B} $ and $ \mathbf{C} $ are Toeplitz matrices, the matrix $ \mathbf{A} $ is also a Toeplitz matrix [25].
In order to smooth the oscillations caused by the non-smooth payoff functions and improve the accuracy, we construct time stepping schemes with Padé approximation. The discretization (2.8) in space leads to the following system of initial value problem
$ vt+Av=f(t),v(0)=v, $
|
(3.1) |
in a Hilbert space $ X $, where $ v $ denotes the initial condition $ v(x) $ in (2.3). We assume the resolvent set $ \rho (\mathbf{A}) $ (The points $ \lambda $ for which $ \lambda\mathbf{I}-\mathbf{A} $ has a bounded inverse in $ X $ comprise the resolvent set $ \rho (\mathbf{A}) $ of $ \mathbf{A} $) satisfies, for some $ \gamma \in (0, \frac{\pi}{2}) $ [26],
$ ρ(A)⊃ˉΣγ,Σγ:={z∈C:γ<|arg(z)|≤π,z≠0}, $
|
(3.2) |
Also, assume there exists $ C\geq 1 $ such that
$ ‖(zI−A)−1‖≤C|z|−1,z∈Σγ. $
|
(3.3) |
The exact solution of (3.1) satisfies the following recurrence formula
$ v(tj+1)=e−τAv(tj)+τ∫10e−τA(1−η)f(tj+τη)dη, $
|
(3.4) |
where $ \tau = T/N $, $ j = 0, 1, 2, \cdots, N-1 $.
Consider now its discrete analogue of the form
$ vj+1=R(τA)vj+τm′∑i=1Qi(τA)f(tj+siτ), $
|
(3.5) |
where $ \{s_i\}_{i = 1}^{m'}\subset [0, 1] $ are the the distinct numbers selected as integral points to approximate $ {\mathbf { v}(t_{j+1})} $ in formula (3.4).
The time discretization scheme (3.5) is accurate of order $ q $ in time which can be described as follow.
Lemma 3.1. [26] The time discretization scheme $(3.5)$ is accurate of order $ q $ if
$ R(z)=e−z+O(zq+1),as z→0, $
|
(3.6) |
and, for $ 0\leq l\leq q $,
$ m′∑i=1sliQi(z)=l!(−z)l+1(R(z)−l∑j=0(−z)jj!)+O(zq−l),as z→0, $
|
(3.7) |
or, equivalently,
$ m′∑i=1sliQi(z)=∫10sle−z(1−s)ds+O(zq−l),as z→0. $
|
(3.8) |
It is shown in [26] that for the case $ m' = q $ ($ m' $ is the number of quadrature points and $ q $ is the accuracy of the scheme), the conditions of the Lemma 1 can be achieved by choosing the rational functions $ R(z) $ satisfying (3.6), selecting distinct real numbers, by Gaussian Quadrature, $ \{Q_i(z)\}_{i = 1}^q $, and finally solving the system
$ q∑i=1sliQi(z)=l!(−z)l+1(R(z)−l∑j=0(−z)jj!),l=0,1,…,q−1. $
|
(3.9) |
This system (3.9) is of Vandermonde type (whose determinant is not zero), which gives the rational functions $ \{Q_i(z)\}_{i = 1}^q $ as linear combinations of the terms on the right hand side of (3.9).
For the case when the number of quadrature points $ m' $ is less than the order of the scheme $ q $, an alternative formula similar to (3.9) is given in [26]. The accuracy conditions are reformulated by defining
$ Rl(z)=l!(−z)l+1(R(z)−l∑j=0(−z)jj!)−m′∑i=1sliQi(z),l=0,1,…,q−1 $
|
and requiring that
$ Rl(z)=0,asz→0,forl=0,1,…,m′−1, $
|
and a moment condition
$ ∫10p(s)sjds=0,forj=0,…,q−m′−1. $
|
(3.10) |
on the quadrature points, with $ p(s) = \prod\limits_{i = 1}^{m'}\left(s-s_{i}\right) $. The formula to obtain the rational functions $ \{Q_i(z)\}_{i = 1}^q $ in [26] is
$ m′∑i=1sliQi(z)=l!(−z)l+1(R(z)−l∑j=0(−z)jj!),l=0,1,…,m′−1. $
|
(3.11) |
For the rest of this chapter, we will use Padé approximation as $ R(z) $ above to constructe the high-order numerical scheme.
Let $ P_{n, m}(z) $ and $ Q_{n, m}(z) $ be two polynomials of degree $ n $ and $ m $ respectively, the $ (n+m) $ th order rational Padé approximation of the exponential function $ e^{-z} $ can be written as
$ R_{n, m}(z) = \frac{P_{n, m}(z)}{Q_{n, m}(z)}, $ |
where
$ P_{n, m}(z) = \sum\limits_{j = 0}^{n} \frac{(m+n-j)!n!}{(m+n)!j!(n-j)!} (-z)^j, $ |
and
$ Q_{n, m}(z) = \sum\limits_{j = 0}^{m} \frac{(m+n-j)!m!}{(m+n)!j!(m-j)!} z^j. $ |
The Padé approximation $ R_{n, m}(z) $ to the exponential function $ e^{-z} $ is of the order $ (n+m) $.
Definition 3.1. The rational approximation $ R_{n, m}(z) $ of $ e^{-z} $ is said to be $ A $-stable if $ |R_{n, m}(z)| < 1 $ whenever $ \Re(z) < 0 $ and L-stable if in addition $ |R_{n, m}(z)| \rightarrow 0 $ as $ \Re(z) \rightarrow - \infty $.
It is known from [27] that $ R_{n, m}(z) = e^{-z} +O\left(|z|^{m+n+1}\right) $ as $ z \rightarrow 0 $, and we consider the $ L $-stable $ (0, 2m) $-Padé approximations and $ A $-stable $ (m, m) $-Padé approximations [28] for the exponential function $ e^{-z} $.
Here for practical purpose, we are particularly interested to the following $ A $-stable and $ L $-stable Padé approximation of $ e^{-z} $ respectively:
$ R_{2, 2}(z) = \frac{1-\frac{1}{2}z+\frac{1}{12}z^2}{1+\frac{1}{2}z+\frac{1}{12}z^2} \\ $ |
and
$ R_{0, 4}(z) = \frac{1}{1+z+\frac{1}{2}z^2+\frac{1}{6}z^3+\frac{1}{24}z^4}. \\ $ |
Replace the matrix exponential $ e^{-\tau \mathbf{A}} $ by $ (n, m) $ Padé approximation $ R_{n, m}(\tau \mathbf{A}) $, the recurrence relation is approximated by
$ Vj+1=Rn,m(τA)Vj+τ2∑i=1Q(i)n,m(τA)f(tj+siτ),j=0,1,2,⋯,N−1, $
|
(3.12) |
which is the fully discretization of (2.2). The $ \{Q_{n, m}^{(i)}(z)\}_{i = 1}^2 $ are rational functions, which have same denominator as those $ R_{n, m}(z) $ and $ \{s_i\}_{i = 1}^{2} $ are the Gaussian points.
Using the result of equation (3.11), we still have the same accuracy when we choose the corresponding Padé approximation
$ 2∑i=1sliQ(i)n,m(z)=l!(−z)l+1(Rn,m(z)−l∑j=0(−z)jj!),l=0,1, $
|
(3.13) |
which is a linear system in $ Q_{n, m}^{(i)}(z) $ and could be solved easily since the matrix of the coefficients on the left is of Vandermonde's type.
Consider the fourth order $ L $-stable Padé approximation $ R_{0, 4}(z) $ with $ s_1 = \frac{3-\sqrt{3}}{6} $ and $ s_2 = \frac{3+\sqrt{3}}{6} $, the system reduces to
$ Q(1)0,4(z)+Q(2)0,4(z)=−1z(R0,4(z)−1),s1Q(1)0,4(z)+s2Q(2)0,4(z)=1z2(R0,4(z)−1+z). $
|
(3.14) |
Solving the Eq (3.14), it leads to the following fourth order schemes
$ {\mathbf { V }_{j+1}} = R_{0, 4}(\tau \mathbf{A}){\mathbf { V }_{j}} + \tau Q_{0, 4}^{(1)}(\tau \mathbf{A})g(t_j+s_1 \tau) +Q_{0, 4}^{(2)}(\tau \mathbf{A}) f(t_j+s_2 \tau), $ |
where
$ Q(1)0,4(z)=12+(3−√312)z+(2−√324)z2+(1−√348)z31+z+12z2+16z3+124z4,Q(2)0,4(z)=12+(3+√312)z+(2+√324)z2+(1+√348)z31+z+12z2+16z3+124z4. $
|
Both the schemes discussed above require to take inverse of higher order matrix polynomial which can cause computational difficulty due to higher power of matrix $ \mathbf{A} $.
For overcoming this difficulty, Khaliq et al. [29], Gallopoulos and Saad [30] and references therein developed these schemes in a partial fraction decomposition (with complex arithmetic) that allows efficient and accurate computations on serial or parallel machines.
The partial fraction form of the rational functions $ R_{n, m}(z) $ and $ \{Q_{n, m}^{(i)}(z) \}_{i = 1}^{2} $ requires us to consider two cases, $ n < m $ and $ n = m $ for subdiagonal and diagonal Padé schemes respectively.
If $ n < m $, then we have
$ Rn,m(z)=q1∑j=1wjz−ci+2q1+q2∑j=q1+1ℜ(wjz−ci),Q(i)n,m(z)=q1∑j=1wijz−ci+2q1+q2∑j=q1+1ℜ(wijz−ci),i=1,2, $
|
and for the case $ n = m $, the partial fraction form for $ R_{n, m}(z) $ and $ Q_{n, m}^{(i)}(z) $ is given by Gallopoulos and Saad [30]
$ Rn,m(z)=(−1)n+q1∑j=1wjz−ci+2q1+q2∑j=q1+1ℜ(wjz−ci),Q(i)n,m(z)=q1∑j=1wijz−ci+2q1+q2∑j=q1+1ℜ(wijz−ci),i=1,2, $
|
where $ R_{n, m}(z) $ as well as $ Q_{n, m}^{(i)}(z) $ have $ q_1 $ real and $ 2q_2 $ complex pole $ c_{{i}} $ with $ q_1+2q_2 = m $, and $ w_j = \frac{R_{n, m}(c_j)}{Q'_{n, m}(c_j)} $ and $ w_{ij} = \frac{\mathcal{N}^{(i)}_{n, m}(c_j)}{\mathcal{D}^{'(i)}_{n, m}(c_j)} $.
The polynomial $ \mathcal{N}^{(i)}_{n, m}(z) $ and $ \mathcal{D}^{(i)}_{n, m}(z) $ are the numerator and denominator of the function $ Q_{n, m}^{(i)}(z) $ respectively.
The poles and weights for $ R_{n, m}(z) $ and $ Q_{n, m}^{(i)}(z) $ are:
$ q1=0,q2=2,c1=−0.270555768932292+2.50477590436244i,c2=−1.72944423106769−0.888974376121862i,w1=−0.541413348429154+0.248562520866115i,w2=0.541413348429182+1.58885918222330i,w11=−0.295373909958643−0.179575890979879i,w12=0.112361208066424+0.596907381204152i,w21=0.174204307471874−0.023488268401115i,w22=0.508808394420345+0.002507912891072i. $
|
The algorithm becomes
$ \mathbf{V}_{j+1} = 2 \Re(\mathbf{y}_1) + 2 \Re(\mathbf{y}_2), $ |
where
$ (τA−c1I)y1=w1Vj+τw11f(tj+s1τ)+τw21f(tj+s2τ),(τA−c2I)y2=w2Vj+τw12f(tj+s1τ)+τw22f(tj+s2τ),i=1,2, $
|
(3.15) |
which can be solved in parallel on two machines for speedup, or on a serial machine.
In this section, we prove the convergence of the proposed scheme in the case that the spatial discretization matrix $ \mathbf{A} $ is a lower Hessenberg Toeplitz matrix, without the assumption of a self-adjoint operator in [26].
We begin with the proof of the positive definiteness of matrix $ \mathbf{A} $. The matrix $ \mathbf{A} $ is positive definite if and only if its symmetric part $ \mathbf{W} = (\mathbf{A}+\mathbf{A}^T)/2 $ is positive definite [31], which means its eigenvalues are all positive.
Theorem 4.1. Assume the fractional parameter $ \alpha $ satisfying $ 1 < \alpha < 2 $, the matrix $ \mathbf{A} = r \mathbf { I }-\zeta \mathbf { B } -\xi \mathbf { C} $ defined in $(2.8)$ as the following
$ aij={−ζω(α)1+r,i=j,j=1,…,M−1,−ζω(α)2+ξ,i=j+1,j=1,…,M−2,−ζω(α)0−ξ,i=j−1,j=2,…,M−1,−ζω(α)i−j+1,i≥j+2,j=1,…,M−3,0,otherwise, $
|
(4.1) |
is positive definite.
Proof. Consider now the matrix $ \mathbf{W} = [{(-\zeta \mathbf { B } -\xi \mathbf { C})+(-\zeta \mathbf { B } -\xi \mathbf { C})^T}]/2 $ defined by
$ wij={−ζω(α)1,i=j,j=1,…,M−1,−ζ(ω(α)0+ω(α)2)/2,|i−j|=1,−ζω(α)|i−j|+1/2,|i−j|≥2, $
|
(4.2) |
Use Gerschgorin Disk Theorem and note that $ w_{ii} = {\zeta (\alpha+2)(\alpha-1)}/{2} > 0 $, we only need to prove it is row diagonally dominant and column diagonally dominant. It is clear that the $ i $th and the $ (M-i-1) $th rows are the same. Without loss of generality, we choose $ 1 \leq i \leq\lceil \frac{M-1}{2} \rceil $.
For $ i = 1 $, we have
$ |w11|−∑j≠1|w1j|=ζ2[2|ω(α)1|−|ω(α)0+ω(α)2|−M−1∑k=3|ω(α)k|]=ζ2[(1−α4)(α+2)(α−1)−(−α2g(α)2+g(α)2+…+g(α)M−2+α2g(α)M−1)]=ζ2[(α−1)(α2+2)−(g(α)2+…+g(α)M−2+α2g(α)M−1)]>ζ2[(α−1)(α2+2)−∞∑k=2g(α)k]=ζ2[(α−1)(α2+2)−(α−1)]=ζ2(α−1)(α2+1)>0. $
|
For $ i = 2, 3, \ldots, \lceil \frac{M-1}{2} \rceil $, using the properties in (2.5), we have
$ |wii|−∑j≠1|wij|=ζ2[2|ω(α)1|−2|ω(α)0+ω(α)2|−2i∑k=3|ω(α)k|−M−i∑k=i+1|ω(α)k|]=ζ2[(2−α)(α+2)(α−1)2−2i∑k=3|ω(α)k|−M−i∑k=i+1|ω(α)k|]>ζ2[(2−α)(α+2)(α−1)2−2M−i∑k=3|ω(α)k|]=ζ2[(2−α)(α+2)(α−1)2−2(−α2g(α)2+g(α)2+…+g(α)M−2+α2g(α)M−1)]=ζ2[2(α−1)−2M−i∑k=3g(α)k]>ζ2[2(α−1)−2∞∑k=2g(α)k]=ζ2[2(α−1)−2(α−1)]=0. $
|
Therefore, the matrix $ \mathbf{W} $ is row diagonal dominant. Because of its Toeplitz structure, it is column diagonally dominant as well, and thus the matrix $ \mathbf{A} $ is positive definite.
Assume the function $ R(\cdot) $ is the $ L $-stable $ (0, 2m) $-Padé approximation of order $ q $ in (3.6), and $ v $ is the initial condition in (3.1), then the convergence of the scheme is established in the following two theorems.
Theorem 4.2. Assume that $ \mathbf{A} $ defined in $(3.1)$ and satisfying $(3.2)$ and $(3.3)$ is diagonalizable. For the $ R(\cdot) $ and $ q > 0 $ in $(3.6)$, there exists a constant $ C > 0 $ such that for $ n > 1 $
$ ‖(e−tnA−Rn(τA))v‖≤Cτq‖v‖,v∈RM−1. $
|
(4.3) |
Proof. Let $ \mathbf{A} $ have eigenvalues $ \{\lambda_i \}_{i = 1}^{M-1} $ and corresponding orthonormal eigenvectors $ \{w_i \}_{i = 1}^{M-1} $. Suppose $ v = \sum\limits_{j = 1}^{M-1}\alpha_jw_j $, then we have
$ e^{-t_n\mathbf{A}}v = \sum\limits_{j = 1}^{M-1}\alpha_je^{-\lambda_jt_n}w_j, $ |
and
$ R^n(\tau \mathbf{A})v = \sum\limits_{j = 1}^{M-1}\alpha_jR^n(\tau \lambda_j)w_j. $ |
It follows that
$ \| (e^{-t_n\mathbf{A}}-R^n(\tau \mathbf{A}))v \|^2 = \sum\limits_{j = 1}^{M-1}\alpha_j^2|e^{-n\tau \lambda_j}-R^n(\tau \lambda_j) |^2. $ |
Using the identity $ a^n-b^n = (a-b)\sum_{j = 0}^{n-1}a^jb^{n-j-1} $, it follows that
$ |e−nτλj−Rn(τλj)|=|(e−τλj−R(τλj))n−1∑j=0(e−τjλjRn−j−1(τλj))|=|(τλj)q+1n−1∑j=0(e−τjλjRn−j−1(τλj))|. $
|
Without the confusion, we will reuse the constant $ C $ from line to line. From Theorem 4.1 we know that $ \mathbf{A} $ is positive definite, thus $ \Re(\lambda_j) > 0, \; j = 1, 2\ldots, M-1 $. For any integer $ k\geq 0 $ and $ l > 0 $, there exists a constant $ C $ such that
$ |(τλj)ke−τlλj|≤C, $
|
(4.4) |
We also find there exists $ 0 < c < 1 $ such that $ | R(z)|\leq e^{-cz} $ for (0, 4)-Padé scheme, thus we have the following bound
$ |e^{-n\tau \lambda_j}-R^n(\tau \lambda_j) |\leq Cn\tau^{q+1}e^{-c(n-1)\tau\lambda} = C\tau^{q}. $ |
Therefore,
$ \| (e^{-t_n\mathbf{A}}-R^n(\tau \mathbf{A}))v \|^2\leq C\tau^{2q}\sum\limits_{j = 1}^{M-1}\alpha_j^2 = C\tau^{2q}\|v \|^2, $ |
and the proof is complete.
Use the results of Theorem 4.2, and define the spaces $ \dot{H}^{s} = \mathcal{D}\left(\mathbf{A}^{s / 2}\right) $ in [26] with the norm as following
$ |v|_s = (\mathbf{A}^sv, v)^{1/2} = \| \mathbf{A}^{s/2}v \| = \left( \sum\limits_{j = 1}^{M-1}\lambda_j^s(v, w_j)^2 \right)^{1/2}, $ |
we complete the proof of the convergence in the following theorem.
Theorem 4.3. Suppose that $ f^{(l)}(t) \in \dot{H}^{2 q-2 l} $ for $ l < q $ and $ t\geq 0 $, then there exists a constant $ C $ such that
$ ‖vn−v(tn)‖≤Cτq(‖v‖+tnq−1∑l=0sups<tn|f(l)(s)|2q−2l+∫tn0‖f(q)‖ds), $
|
(4.5) |
i.e., the time discretization scheme $(3.5)$ is accurate of order $ q $.
Proof. The error $ \mathcal{E}^{n} = \mathbf{v}^n-\mathbf{v}(t_n) $, for $ n\geq 2 $, can be written as:
$ En=(Rn(τA)−E(tn))v⏟En0+τn−1∑j=0(Rn−j−1(τA)Rkf(tj)−E(tt−j−1)Ikf(tj))⏟Enq, $
|
(4.6) |
where $ E(t) = e^{-t \mathbf{A}} $,
$ \mathcal{I}_kf(t_j) = \int_{0}^{1}E(\tau-s\tau)f(t_j+s\tau)ds, \quad \mathcal{R}_kf(t_j) = \sum\limits_{i = 1}^{m'} Q_i(\tau \mathbf{A}) f(t_j +s_i\tau). $ |
The error term $ \mathcal{E}_0^{n} $ can be approximated by the established result from Theorem 4.2 as follows:
$ ‖En0‖=‖(e−tnA−Rn(τA))v‖≤Cτq‖v‖. $
|
(4.7) |
After inserting the term $ R^{n-j-1}(\tau \mathbf{A})\mathcal{I}_kf(t_j) $ in the error term $ \mathcal{E}_q^{n} $ and rearranging its terms, it can be derived as
$ Enq=τn−1∑j=0(Rn−j−1(τA)−E(tn−j−1))Ikf(tj)⏟En1+τn−1∑j=0Rn−j−1(τA)(Rk−Ik)f(tj)⏟En2. $
|
(4.8) |
Following the approach given in [26], we have the following estimate for $ \mathcal{E}_1^{n} $ and $ \mathcal{E}_2^{n} $,
$ ‖En1‖≤Cτq∫tn0|f|2qds, $
|
(4.9) |
which is bounded by the right hand side of (4.5). Also
$ ‖En2‖≤n−1∑j=0Cτq+1q−1∑j=0|f(l)(tj)|2q−2l+Cτqn−1∑0∫tj+1tj‖f(q)‖ds. $
|
(4.10) |
Since Eq (4.9) can be incorporated into the right hand side of (4.10), we obtain the following estimate for the main scheme:
$ ‖Enq‖≤n−1∑j=0Cτq+1q−1∑j=0|f(l)(tj)|2q−2l+Cτqn−1∑0∫tj+1tj‖f(q)‖ds,≤Cτqtnq−1∑l=0sups<tn|f(l)(s)|2q−2l+Cτq∫tn0‖f(q)‖ds. $
|
(4.11) |
Combining (4.7) and (4.11), it leads to
$ ‖En‖≤‖En0‖+‖Enq‖≤Cτq(‖v‖+tnq−1∑l=0sups<tn|f(l)(s)|2q−2l+∫tn0‖f(q)‖ds), $
|
(4.12) |
which completes the proof.
In this section, numerical performance of different Padé schemes compared with the Crank-Nicolson scheme is given for the numerical solution of both fractional digital options and fractional barrier options.
Since the non-smooth payoffs of fractional exotic options usually result in inaccurate and discontinuous solution, or serious errors when estimating the hedging parameters, e.g., Delta, Vega and Gamma values, we compare the price of the options under different schemes, as well as the Delta [16,32,33], which is the rate of change of the option value with respect to the asset price and can be approximated in the following way:
$ ∂V∂S|Si=1exi⋅∂V∂x|xi≈V(xi+1,t)−V(xi−1,t)2hexi. $
|
The order of the numerical scheme is defined as
$ Order=logτ2τ1‖Vτ2(⋅,0)−V∗(⋅,0)‖‖Vτ1(⋅,0)−V∗(⋅,0)‖, $
|
where $ V_\ast(\cdot, 0) $ denotes the exact solution at $ t = 0 $ and $ V_{\tau}(\cdot, 0) $ denotes the numerical solution with time step $ \tau $ at $ t = 0 $. In our experiments, the exact solution $ V_\ast(\cdot, 0) $ is approximated by the numerical solution using a dense mesh with $ M = N = 8192 $ and we set $ \tau_2/\tau_1 = 2 $ to obtain the convergence order.
Example 5.1. Consider a fractional digital call option pricing model as follows
$ {∂V(x,t)∂t+(r−ν)∂V(x,t)∂x+νBdDαxV(x,t)=rV(x,t),(x,t)∈(Bd,Bu)×(0,T),V(Bd,t)=0,V(Bu,t)=50e−r(T−t),t∈[0,T),V(x,T)=v(x),x∈(Bd,Bu), $
|
where $ \alpha = 1.5, \; r = 0.05, \; \sigma = 0.25, \; B_u = \ln{100}, \; B_d = \ln{0.1}, \; T = 1, \; K = 50 $ and $ \nu = -\frac{1}{2} \sigma^{\alpha} \sec \frac{\alpha \pi}{2} $ where the payoff function is
$ v(x)={50,lnK<x<Bu,25,x=lnK,0,Bd<x<lnK., $
|
where we take the average of playoff at $ x = \ln K $ from mathematical viewpoint to restore the discontinuity in the payoff [15].
In Figures 1–3, the surfaces of the price and the corresponding Delta value of the fractional European digital option are plotted for the Crank-Nicolson, (2, 2)-Padé and (0, 4)-Padé schemes respectively when $ N = 16 $ and $ M = 4096 $.
From Figures 1–3, it is observed that the second-order Crank–Nicolson method suffers from oscillations with non-smooth payoff function while the (0, 4)-Padé scheme can provide reliable and smooth option values and Delta value with little oscillation.
The reason for the oscillation phenomenon at the strike price is that the time discretization grids are coarse [33,34]. One possible remedy is reducing the discrete step size in time direction or local mesh refinement strategy [35,36], which would highly increase the computational time. Here, the (0, 4)-Padé scheme could obtain the best accuracy cheaply and smooth the oscillations with relative less discrete points.
In Tables 1–3, we list the error of the numerical solution in $ L_2 $ and $ L_\infty $ norm, as well as the corresponding order for the Crank-Nicolson scheme, (2, 2)-Padé and (0, 4)-Padé respectively when $ \tau $ is varying and $ M = 8192 $.
$ N $ | $ \tau $ | $ \|V_{\tau}(\cdot, 0)-V_\ast(\cdot, 0)\|_2 $ | Order | $ \|V_{\tau}(\cdot, 0)-V_\ast(\cdot, 0)\|_\infty $ | Order |
8 | 0.12500000 | 51.4645 | *** | 27.0184 | *** |
16 | 0.06250000 | 27.4790 | 0.9052 | 19.2316 | 0.4905 |
32 | 0.03125000 | 10.3013 | 1.4155 | 6.1728 | 1.6395 |
64 | 0.01562500 | 1.0048 | 3.3578 | 4.9171$ \times 10^{-1} $ | 3.6500 |
128 | 0.00781250 | 3.4284$ \times 10^{-3} $ | 8.1952 | 2.2191$ \times 10^{-4} $ | 11.1136 |
$ N $ | $ \tau $ | $ \|V_{\tau}(\cdot, 0)-V_\ast(\cdot, 0)\|_2 $ | Order | $ \|V_{\tau}(\cdot, 0)-V_\ast(\cdot, 0)\|_\infty $ | Order |
8 | 0.12500000 | 31.7954 | *** | 21.3352 | *** |
16 | 0.06250000 | 13.3594 | 1.2510 | 9.0302 | 1.2404 |
32 | 0.03125000 | 2.0437 | 2.7086 | 1.3213 | 2.7728 |
64 | 0.01562500 | 5.1328$ \times 10^{-3} $ | 8.6372 | 2.7977$ \times 10^{-3} $ | 8.8835 |
128 | 0.00781250 | 7.4134$ \times 10^{-8} $ | 16.0793 | 4.8969$ \times 10^{-9} $ | 19.1239 |
$ N $ | $ \tau $ | $ \|V_{\tau}(\cdot, 0)-V_\ast(\cdot, 0)\|_2 $ | Order | $ \|V_{\tau}(\cdot, 0)-V_\ast(\cdot, 0)\|_\infty $ | Order |
8 | 0.12500000 | 1.7217$ \times10^{-2} $ | *** | 1.1203$ \times10^{-3} $ | *** |
16 | 0.06250000 | 1.3686$ \times10^{-3} $ | 3.6530 | 9.0190$ \times10^{-5} $ | 3.6348 |
32 | 0.03125000 | 9.7572$ \times10^{-5} $ | 3.8101 | 8.1591$ \times10^{-6} $ | 3.4665 |
64 | 0.01562500 | 7.0293$ \times10^{-6} $ | 3.7950 | 1.8366$ \times10^{-6} $ | 2.1514 |
128 | 0.00781250 | 7.8313$ \times10^{-7} $ | 3.1661 | 3.8539$ \times10^{-7} $ | 2.2526 |
From Tables 1–3, it is seen that the numerical results of digital option with both (2, 2)-Padé scheme and (0, 4)-Padé scheme have fourth-order accuracy, which is much better than those of the Crank-Nicolson scheme.
In Figure 4, we plot the curves of option price and the corresponding Delta value versus the price of the asset when $ t = 0 $ for the Crank-Nicolson, (2, 2)-Padé and (0, 4)-Padé scheme respectively.
From Figure 4, it is further confirmed that the (0, 4)-Padé scheme can significantly reduce the oscillations of the solution near the strike price and smooth both the price of option and the Delta value, compared with the Crank-Nicolson and (2, 2)-Padé schemes. It is possibly because of (0, 4)-Padé scheme is a high order scheme, so that it can quickly converges to the exact solution with less time layer.
Example 5.2. Consider a fractional barrier put option pricing model as follows
$ {∂V(x,t)∂t+(r−ν)∂V(x,t)∂x+νBdDαxV(x,t)=rV(x,t),(x,t)∈(Bd,Bu)×(0,T),V(Bu,t)=V(Bd,t)=0,t∈[0,T),V(x,T)=v(x),x∈(Bd,Bu), $
|
where $ \alpha = 1.5, \; r = 0.05, \; \sigma = 0.25, \; B_u = \ln{100}, \; B_d = \ln{0.1}, \; T = 1, \; K = 50, \; E = 20 $ and $ \nu = -\frac{1}{2} \sigma^{\alpha} \sec \frac{\alpha \pi}{2} $. The payoff function is
$ v(x)={max{K−ex,0},lnE<x≤Bu,0,Bd<x≤lnE. $
|
In Figures 5–7, the price surfaces of the fractional barrier put option and the corresponding Delta value are plotted for the Crank-Nicolson, (2, 2)-Padé and (0, 4)-Padé schemes respectively when $ N = 16 $ and $ M = 4096 $.
From Figures 5–7, it is observed that the option price and Delta value of the Crank–Nicolson method still suffer from serious oscillations near the barrier while those of the (0, 4)-Padé scheme provide the best approximate results.
It is also seen that though the (2, 2)-Padé scheme makes use of the same interpolation points with the (0, 4)-Padé scheme, the price of option and Delta values of the (2, 2)-Padé scheme still oscillate near the barrier.
Moreover, in Figure 8, we plot the curves of option price and the corresponding Delta value versus the asset price when $ t = 0 $ for the Crank-Nicolson, (2, 2)-Padé and (0, 4)-Padé scheme respectively.
From Figure 8, it is further verified that the (0, 4)-Padé scheme is the best one, which can significantly reduce the oscillations of the option price and the Delta value near the barrier.
In Tables 4–6, the error of the numerical solution in $ L_2 $ and $ L_\infty $ norm, as well as the corresponding order for the Crank-Nicolson, (2, 2)-Padé and (0, 4)-Padé schemes are listed respectively when $ \tau $ is varying and $ M = 8192 $.
$ N $ | $ \tau $ | $ \|V_{\tau}(\cdot, 0)-V_\ast(\cdot, 0)\|_2 $ | Order | $ \|V_{\tau}(\cdot, 0)-V_\ast(\cdot, 0)\|_\infty $ | Order |
8 | 0.12500000 | 33.7205 | *** | 17.8600 | *** |
16 | 0.06250000 | 19.9914 | 0.7542 | 14.8495 | 0.2663 |
32 | 0.03125000 | 8.8330 | 1.1784 | 7.7376 | 0.9405 |
64 | 0.01562500 | 9.9060$ \times 10^{-1} $ | 3.1565 | 7.8221$ \times 10^{-1} $ | 3.3063 |
128 | 0.00781250 | 2.1208$ \times 10^{-3} $ | 8.8676 | 2.3627$ \times 10^{-4} $ | 11.6929 |
$ N $ | $ \tau $ | $ \|V_{\tau}(\cdot, 0)-V_\ast(\cdot, 0)\|_2 $ | Order | $ \|V_{\tau}(\cdot, 0)-V_\ast(\cdot, 0)\|_\infty $ | Order |
8 | 0.12500000 | 22.5121 | *** | 15.7317 | *** |
16 | 0.06250000 | 11.0447 | 1.0274 | 9.6167 | 0.7101 |
32 | 0.03125000 | 1.9755 | 2.4831 | 1.4823 | 2.6977 |
64 | 0.01562500 | 5.2250$ \times 10^{-3} $ | 8.5626 | 3.1998$ \times 10^{-3} $ | 8.8556 |
128 | 0.00781250 | 4.4324$ \times 10^{-8} $ | 16.8470 | 2.9549$ \times 10^{-9} $ | 20.0465 |
$ N $ | $ \tau $ | $ \|V_{\tau}(\cdot, 0)-V_\ast(\cdot, 0)\|_2 $ | Order | $ \|V_{\tau}(\cdot, 0)-V_\ast(\cdot, 0)\|_\infty $ | Order |
8 | 0.12500000 | 1.0414$ \times10^{-2} $ | *** | 6.7754$ \times10^{-4} $ | *** |
16 | 0.06250000 | 8.2617$ \times10^{-4} $ | 3.6559 | 5.4547$ \times10^{-5} $ | 3.6347 |
32 | 0.03125000 | 5.8268$ \times10^{-5} $ | 3.8257 | 3.8767$ \times10^{-6} $ | 3.8146 |
64 | 0.01562500 | 3.8786$ \times10^{-6} $ | 3.9091 | 2.5746$ \times10^{-7} $ | 3.9124 |
128 | 0.00781250 | 3.1080$ \times10^{-7} $ | 3.6415 | 1.5452$ \times10^{-8} $ | 4.0584 |
From Tables 4–6, it is observed that the price of the digital option with both (2, 2)-Padé scheme and (0, 4)-Padé scheme can achieve the fourth-order accuracy, while the results of the (0, 4)-Padé scheme are more accurate than those of the (2, 2)-Padé scheme.
Example 5.3. Consider a fractional double barrier call option pricing model as follows
$ {∂V(x,t)∂t+(r−ν)∂V(x,t)∂x+νBdDαxV(x,t)=rV(x,t),(x,t)∈(Bd,Bu)×(0,T),V(Bu,t)=V(Bd,t)=0,t∈[0,T),V(x,T)=v(x),x∈(Bd,Bu), $
|
where $ \alpha = 1.5, \; r = 0.05, \; \sigma = 0.25, \; B_u = \ln{100}, \; B_d = \ln{0.1}, \; T = 1, \; K = 20, \; E_1 = 40, \; E_2 = 70 $ and $ \nu = -\frac{1}{2} \sigma^{\alpha} \sec \frac{\alpha \pi}{2} $. The payoff function is
$ v(x)={max{ex−K,0},lnE1<x≤lnE2,0,Bd<x≤lnE1,lnE2≤x<Bu. $
|
In Figures 9–11, the price surfaces of the fractional double barrier call option and the corresponding Delta value are drawn for the Crank-Nicolson, (2, 2)-Padé and (0, 4)-Padé schemes respectively with $ N = 16 $ and $ M = 2048 $.
From Figures 9–11, it is observed that both the Crank–Nicolson and (2, 2)-Padé schemes suffer from spurious oscillations near the barrier while (0, 4)-Padé approximation provides reliable option values and smooth Delta value.
In Figure 12, we plot the curves of option price and the corresponding Delta value versus the asset price when $ t = 0 $ for Crank-Nicolson, (2, 2)-Padé and (0, 4)-Padé scheme respectively.
From Figure 12, it is further confirmed that the (0, 4)-Padé scheme can significantly reduce the oscillation of the price of the option near the barrier and smooth the corresponding Delta value.
In Tables 7–9, the error of the numerical solution in $ L_2 $ and $ L_\infty $ norm, as well as the corresponding order for the Crank–Nicolson, (2, 2)-Padé and (0, 4)-Padé schemes are listed respectively when $ \tau $ is varying and $ M = 8192 $.
$ N $ | $ \tau $ | $ \|V_{\tau}(\cdot, 0)-V_\ast(\cdot, 0)\|_2 $ | Order | $ \|V_{\tau}(\cdot, 0)-V_\ast(\cdot, 0)\|_\infty $ | Order |
8 | 0.12500000 | 60.6264 | *** | 29.9147 | *** |
16 | 0.06250000 | 35.9440 | 0.7542 | 24.8172 | 0.2695 |
32 | 0.03125000 | 15.8816 | 1.1784 | 12.9186 | 0.9419 |
64 | 0.01562500 | 1.7811 | 3.1565 | 1.3060 | 3.3062 |
128 | 0.00781250 | 3.6030$ \times10^{-3} $ | 8.9493 | 3.7585$ \times10^{-4} $ | 11.7628 |
$ N $ | $ \tau $ | $ \|V_{\tau}(\cdot, 0)-V_\ast(\cdot, 0)\|_2 $ | Order | $ \|V_{\tau}(\cdot, 0)-V_\ast(\cdot, 0)\|_\infty $ | Order |
8 | 0.12500000 | 40.4761 | *** | 26.3017 | *** |
16 | 0.06250000 | 19.8581 | 1.0273 | 16.0588 | 0.7118 |
32 | 0.03125000 | 3.5519 | 2.4831 | 2.4759 | 2.6974 |
64 | 0.01562500 | 9.3945$ \times10^{-3} $ | 8.5626 | 5.3429$ \times10^{-3} $ | 8.8561 |
128 | 0.00781250 | 7.9967$ \times10^{-8} $ | 16.8421 | 5.0636$ \times10^{-9} $ | 20.0090 |
$ N $ | $ \tau $ | $ \|V_{\tau}(\cdot, 0)-V_\ast(\cdot, 0)\|_2 $ | Order | $ \|V_{\tau}(\cdot, 0)-V_\ast(\cdot, 0)\|_\infty $ | Order |
8 | 0.12500000 | 1.9302$ \times10^{-2} $ | *** | 1.0964$ \times10^{-3} $ | *** |
16 | 0.06250000 | 1.5287$ \times10^{-3} $ | 3.6584 | 8.8458$ \times10^{-5} $ | 3.6316 |
32 | 0.03125000 | 1.0763$ \times10^{-4} $ | 3.8281 | 6.3074$ \times10^{-6} $ | 3.8099 |
64 | 0.01562500 | 7.1519$ \times10^{-6} $ | 3.9117 | 4.3025$ \times10^{-7} $ | 3.8738 |
128 | 0.00781250 | 5.1858$ \times10^{-7} $ | 3.7857 | 3.6863$ \times10^{-8} $ | 3.5449 |
From Tables 7–9, it is seen that the numerical price of the fractional double barrier call option for both (2, 2)-Padé scheme and (0, 4)-Padé scheme can achieve fourth-order accuracy while the numerical results of the Crank–Nicolson scheme only obtains the second-order accuracy. Among the three schemes, the (0, 4)-Padé scheme is the most accurate one, which requires less discrete points than the other two schemes to achieve the same accuracy.
A class of fourth order Padé schemes for pricing fractional exotic options under FMLS model are proposed and studied, which make use of the 2nd-order weighted and shifted Grünwald difference scheme in space direction and the 4th-order Padé schemes in time direction. The convergence of the Padé schemes are proved in detailed under the FMLS model. Numerical experiments on fractional digital option and fractional barrier options are given to verify the 4th-order precision, and show that the (0, 4)-Padé scheme can significantly reduce the oscillations of the solution near the strike price or barrier, smooth the Delta value and save the computational time.
The authors are very grateful to the referees for their constructive comments and valuable suggestions, which greatly improved the original manuscript of the paper. This work is supported by the National Natural Science Foundation of China (No. 11971354).
The authors declare there is no conflicts of interest.
[1] |
Boulon S, Westman BJ, Hutten S, et al. (2010) The nucleolus under stress. Mol Cell 40: 216-227. doi: 10.1016/j.molcel.2010.09.024
![]() |
[2] |
Mayer C, Grummt I (2005) Cellular stress and nucleolar function. Cell Cycle 4: 1036-1038. doi: 10.4161/cc.4.8.1925
![]() |
[3] |
Grummt I (2013) The nucleolus-guardian of cellular homeostasis and genome integrity. Chromosoma 122: 487-497. doi: 10.1007/s00412-013-0430-0
![]() |
[4] |
Rubbi CP, Milner J (2003) Disruption of the nucleolus mediates stabilization of p53 in response to DNA damage and other stresses. Embo J 22: 6068-6077. doi: 10.1093/emboj/cdg579
![]() |
[5] |
Hetman M, Pietrzak M (2012) Emerging roles of the neuronal nucleolus. Trends Neurosci 35: 305-314. doi: 10.1016/j.tins.2012.01.002
![]() |
[6] |
Parlato R, Kreiner G (2013) Nucleolar activity in neurodegenerative diseases: a missing piece of the puzzle? J Mol Med (Berl) 91: 541-547. doi: 10.1007/s00109-012-0981-1
![]() |
[7] |
Haeusler AR, Donnelly CJ, Periz G, et al. (2014) C9orf72 nucleotide repeat structures initiate molecular cascades of disease. Nature 507: 195-200. doi: 10.1038/nature13124
![]() |
[8] | Chan HY (2014) RNA-mediated pathogenic mechanisms in polyglutamine diseases and amyotrophic lateral sclerosis. Front Cell Neurosci 8: 431. |
[9] |
Rohrer JD, Isaacs AM, Mizielinska S, et al. (2015) C9orf72 expansions in frontotemporal dementia and amyotrophic lateral sclerosis. Lancet Neurol 14: 291-301. doi: 10.1016/S1474-4422(14)70233-9
![]() |
[10] |
Kwon I, Xiang S, Kato M, et al. (2014) Poly-dipeptides encoded by the C9orf72 repeats bind nucleoli, impede RNA biogenesis, and kill cells. Science 345: 1139-1145. doi: 10.1126/science.1254917
![]() |
[11] |
Wen X, Tan W, Westergard T, et al. (2014) Antisense proline-arginine RAN dipeptides linked to C9ORF72-ALS/FTD form toxic nuclear aggregates that initiate in vitro and in vivo neuronal death. Neuron 84: 1213-1225. doi: 10.1016/j.neuron.2014.12.010
![]() |
[12] |
Tao Z, Wang H, Xia Q, et al. (2015) Nucleolar stress and impaired stress granule formation contribute to C9orf72 RAN translation-induced cytotoxicity. Hum Mol Genet 24: 2426-2441. doi: 10.1093/hmg/ddv005
![]() |
[13] | Riancho J, Ruiz-Soto M, Villagra NT, et al. (2014) Compensatory Motor Neuron Response to Chromatolysis in the Murine hSOD1(G93A) Model of Amyotrophic Lateral Sclerosis. Front Cell Neurosci 8: 346. |
[14] |
Palanca A, Casafont I, Berciano MT, et al. (2014) Reactive nucleolar and Cajal body responses to proteasome inhibition in sensory ganglion neurons. Biochim Biophys Acta 1842: 848-859. doi: 10.1016/j.bbadis.2013.11.016
![]() |
[15] |
Cong R, Das S, Ugrinova I, et al. (2012) Interaction of nucleolin with ribosomal RNA genes and its role in RNA polymerase I transcription. Nucleic Acids Res 40: 9441-9454. doi: 10.1093/nar/gks720
![]() |
[16] |
Tsoi H, Lau TC, Tsang SY, et al. (2012) CAG expansion induces nucleolar stress in polyglutamine diseases. Proc Natl Acad Sci U S A 109: 13428-13433. doi: 10.1073/pnas.1204089109
![]() |
[17] |
Tsoi H, Chan HY (2013) Expression of expanded CAG transcripts triggers nucleolar stress in Huntington's disease. Cerebellum 12: 310-312. doi: 10.1007/s12311-012-0447-6
![]() |
[18] |
Ross CA, Tabrizi SJ (2011) Huntington's disease: from molecular pathogenesis to clinical treatment. Lancet Neurol 10: 83-98. doi: 10.1016/S1474-4422(10)70245-3
![]() |
[19] |
Lee J, Hwang YJ, Ryu H, et al. (2014) Nucleolar dysfunction in Huntington's disease. Biochim Biophys Acta 1842: 785-790. doi: 10.1016/j.bbadis.2013.09.017
![]() |
[20] |
Kreiner G, Bierhoff H, Armentano M, et al. (2013) A neuroprotective phase precedes striatal degeneration upon nucleolar stress. Cell Death Differ 20: 1455-1464. doi: 10.1038/cdd.2013.66
![]() |
[21] |
Lee J, Hwang YJ, Boo JH, et al. (2011) Dysregulation of upstream binding factor-1 acetylation at K352 is linked to impaired ribosomal DNA transcription in Huntington's disease. Cell Death Differ 18: 1726-1735. doi: 10.1038/cdd.2011.38
![]() |
[22] |
Hwang YJ, Han D, Kim KY, et al. (2014) ESET methylates UBF at K232/254 and regulates nucleolar heterochromatin plasticity and rDNA transcription. Nucleic Acids Res 42: 1628-1643. doi: 10.1093/nar/gkt1041
![]() |
[23] |
Lee J, Hwang YJ, Kim KY, et al. (2013) Epigenetic mechanisms of neurodegeneration in Huntington's disease. Neurotherapeutics 10: 664-676. doi: 10.1007/s13311-013-0206-5
![]() |
[24] |
Ammal Kaidery N, Tarannum S, Thomas B (2013) Epigenetic landscape of Parkinson's disease: emerging role in disease mechanisms and therapeutic modalities. Neurotherapeutics 10: 698-708. doi: 10.1007/s13311-013-0211-8
![]() |
[25] |
Masliah E, Dumaop W, Galasko D, et al. (2013) Distinctive patterns of DNA methylation associated with Parkinson disease: identification of concordant epigenetic changes in brain and peripheral blood leukocytes. Epigenetics 8: 1030-1038. doi: 10.4161/epi.25865
![]() |
[26] |
Healy-Stoffel M, Ahmad SO, Stanford JA, et al. (2013) Altered nucleolar morphology in substantia nigra dopamine neurons following 6-hydroxydopamine lesion in rats. Neurosci Lett 546: 26-30. doi: 10.1016/j.neulet.2013.04.033
![]() |
[27] |
Healy-Stoffel M, Omar Ahmad S, Stanford JA, et al. (2014) Differential effects of intrastriatal 6-hydroxydopamine on cell number and morphology in midbrain dopaminergic subregions of the rat. Brain Res 1574: 113-119. doi: 10.1016/j.brainres.2014.05.045
![]() |
[28] |
Rieker C, Engblom D, Kreiner G, et al. (2011) Nucleolar disruption in dopaminergic neurons leads to oxidative damage and parkinsonism through repression of mammalian target of rapamycin signaling. J Neurosci 31: 453-460. doi: 10.1523/JNEUROSCI.0590-10.2011
![]() |
[29] | Kang H, Shin JH (2014) Repression of rRNA transcription by PARIS contributes to Parkinson's disease. Neurobiol Dis 73C: 220-228. |
[30] |
Vilotti S, Codrich M, Dal Ferro M, et al. (2012) Parkinson's Disease DJ-1 L166P Alters rRNA Biogenesis by Exclusion of TTRAP from the Nucleolus and Sequestration into Cytoplasmic Aggregates via TRAF6. PLoS One 7: e35051. doi: 10.1371/journal.pone.0035051
![]() |
[31] |
Vilotti S, Biagioli M, Foti R, et al. (2012) The PML nuclear bodies-associated protein TTRAP regulates ribosome biogenesis in nucleolar cavities upon proteasome inhibition. Cell Death Differ 19: 488-500. doi: 10.1038/cdd.2011.118
![]() |
[32] |
Iacono D, O'Brien R, Resnick SM, et al. (2008) Neuronal hypertrophy in asymptomatic Alzheimer disease. J Neuropathol Exp Neurol 67: 578-589. doi: 10.1097/NEN.0b013e3181772794
![]() |
[33] |
Pietrzak M, Rempala G, Nelson PT, et al. (2011) Epigenetic Silencing of Nucleolar rRNA Genes in Alzheimer's Disease. PLoS One 6: e22585. doi: 10.1371/journal.pone.0022585
![]() |
[34] |
da Silva AM, Payao SL, Borsatto B, et al. (2000) Quantitative evaluation of the rRNA in Alzheimer's disease. Mech Ageing Dev 120: 57-64. doi: 10.1016/S0047-6374(00)00180-9
![]() |
[35] |
McStay B, Grummt I (2008) The epigenetics of rRNA genes: from molecular to chromosome biology. Annu Rev Cell Dev Biol 24: 131-157. doi: 10.1146/annurev.cellbio.24.110707.175259
![]() |
[36] |
Strohner R, Nemeth A, Jansa P, et al. (2001) NoRC--a novel member of mammalian ISWI-containing chromatin remodeling machines. EMBO J 20: 4892-4900. doi: 10.1093/emboj/20.17.4892
![]() |
[37] |
Mayer C, Schmitz KM, Li J, et al. (2006) Intergenic transcripts regulate the epigenetic state of rRNA genes. Mol Cell 22: 351-361. doi: 10.1016/j.molcel.2006.03.028
![]() |
[38] |
Santoro R, Li J, Grummt I (2002) The nucleolar remodeling complex NoRC mediates heterochromatin formation and silencing of ribosomal gene transcription. Nat Genet 32: 393-396. doi: 10.1038/ng1010
![]() |
[39] | Gu L, Frommel SC, Oakes CC, et al. (2015) BAZ2A (TIP5) is involved in epigenetic alterations in prostate cancer and its overexpression predicts disease recurrence. Nat Genet 47: 22-30. |
[40] |
Wu P, Zuo X, Deng H, et al. (2013) Roles of long noncoding RNAs in brain development, functional diversification and neurodegenerative diseases. Brain Res Bull 97: 69-80. doi: 10.1016/j.brainresbull.2013.06.001
![]() |
[41] |
Santoro R, Schmitz KM, Sandoval J, et al. (2010) Intergenic transcripts originating from a subclass of ribosomal DNA repeats silence ribosomal RNA genes in trans. EMBO Rep 11: 52-58. doi: 10.1038/embor.2009.254
![]() |
[42] |
Wan J, Yourshaw M, Mamsa H, et al. (2012) Mutations in the RNA exosome component gene EXOSC3 cause pontocerebellar hypoplasia and spinal motor neuron degeneration. Nat Genet 44: 704-708. doi: 10.1038/ng.2254
![]() |
[43] |
Bierhoff H, Dammert MA, Brocks D, et al. (2014) Quiescence-induced LncRNAs trigger H4K20 trimethylation and transcriptional silencing. Mol Cell 54: 675-682. doi: 10.1016/j.molcel.2014.03.032
![]() |
[44] |
Evertts AG, Manning AL, Wang X, et al. (2013) H4K20 methylation regulates quiescence and chromatin compaction. Mol Biol Cell 24: 3025-3037. doi: 10.1091/mbc.E12-07-0529
![]() |
[45] |
Sarg B, Koutzamani E, Helliger W, et al. (2002) Postsynthetic trimethylation of histone H4 at lysine 20 in mammalian tissues is associated with aging. J Biol Chem 277: 39195-39201. doi: 10.1074/jbc.M205166200
![]() |
[46] |
Shumaker DK, Dechat T, Kohlmaier A, et al. (2006) Mutant nuclear lamin A leads to progressive alterations of epigenetic control in premature aging. Proc Natl Acad Sci U S A 103: 8703-8708. doi: 10.1073/pnas.0602569103
![]() |
[47] |
Bierhoff H, Schmitz K, Maass F, et al. (2010) Noncoding transcripts in sense and antisense orientation regulate the epigenetic state of ribosomal RNA genes. Cold Spring Harb Symp Quant Biol 75: 357-364. doi: 10.1101/sqb.2010.75.060
![]() |
[48] |
Murayama A, Ohmori K, Fujimura A, et al. (2008) Epigenetic control of rDNA loci in response to intracellular energy status. Cell 133: 627-639. doi: 10.1016/j.cell.2008.03.030
![]() |
[49] |
Donmez G, Outeiro TF (2013) SIRT1 and SIRT2: emerging targets in neurodegeneration. EMBO Mol Med 5: 344-352. doi: 10.1002/emmm.201302451
![]() |
[50] |
Herskovits AZ, Guarente L (2014) SIRT1 in neurodevelopment and brain senescence. Neuron 81: 471-483. doi: 10.1016/j.neuron.2014.01.028
![]() |
[51] |
Li Y, Xu W, McBurney MW, et al. (2008) SirT1 inhibition reduces IGF-I/IRS-2/Ras/ERK1/2 signaling and protects neurons. Cell Metab 8: 38-48. doi: 10.1016/j.cmet.2008.05.004
![]() |
[52] |
Zhang F, Wang S, Gan L, et al. (2011) Protective effects and mechanisms of sirtuins in the nervous system. Prog Neurobiol 95: 373-395. doi: 10.1016/j.pneurobio.2011.09.001
![]() |
[53] |
Smith MR, Syed A, Lukacsovich T, et al. (2014) A potent and selective Sirtuin 1 inhibitor alleviates pathology in multiple animal and cell models of Huntington's disease. Hum Mol Genet 23: 2995-3007. doi: 10.1093/hmg/ddu010
![]() |
[54] | Schnapp A, Pfleiderer C, Rosenbauer H, et al. (1990) A growth-dependent transcription initiation factor (TIF-IA) interacting with RNA polymerase I regulates mouse ribosomal RNA synthesis. EMBO J 9: 2857-2863. |
[55] |
Grewal SS, Evans JR, Edgar BA (2007) Drosophila TIF-IA is required for ribosome synthesis and cell growth and is regulated by the TOR pathway. J Cell Biol 179: 1105-1113. doi: 10.1083/jcb.200709044
![]() |
[56] |
Mayer C, Bierhoff H, Grummt I (2005) The nucleolus as a stress sensor: JNK2 inactivates the transcription factor TIF-IA and down-regulates rRNA synthesis. Genes Dev 19: 933-941. doi: 10.1101/gad.333205
![]() |
[57] |
Hoppe S, Bierhoff H, Cado I, et al. (2009) AMP-activated protein kinase adapts rRNA synthesis to cellular energy supply. Proc Natl Acad Sci U S A 106: 17781-17786. doi: 10.1073/pnas.0909873106
![]() |
[58] |
DuRose JB, Scheuner D, Kaufman RJ, et al. (2009) Phosphorylation of eukaryotic translation initiation factor 2alpha coordinates rRNA transcription and translation inhibition during endoplasmic reticulum stress. Mol Cell Biol 29: 4295-4307. doi: 10.1128/MCB.00260-09
![]() |
[59] |
Nguyen le XT, Mitchell BS (2013) Akt activation enhances ribosomal RNA synthesis through casein kinase II and TIF-IA. Proc Natl Acad Sci U S A 110: 20681-20686. doi: 10.1073/pnas.1313097110
![]() |
[60] | Kiryk A, Sowodniok K, Kreiner G, et al. (2013) Impaired rRNA synthesis triggers homeostatic responses in hippocampal neurons. Front Cell Neurosci 7: 207. |
[61] |
Shamsi F, Parlato R, Collombat P, et al. (2014) A genetic mouse model for progressive ablation and regeneration of insulin producing beta-cells. Cell Cycle 13: 3948-3957. doi: 10.4161/15384101.2014.952176
![]() |
[62] |
Parlato R, Kreiner G, Erdmann G, et al. (2008) Activation of an endogenous suicide response after perturbation of rRNA synthesis leads to neurodegeneration in mice. J Neurosci 28: 12759-12764. doi: 10.1523/JNEUROSCI.2439-08.2008
![]() |
[63] |
Domanskyi A, Geissler C, Vinnikov IA, et al. (2011) Pten ablation in adult dopaminergic neurons is neuroprotective in Parkinson's disease models. FASEB J 25: 2898-2910. doi: 10.1096/fj.11-181958
![]() |
[64] |
Yuan X, Zhou Y, Casanova E, et al. (2005) Genetic inactivation of the transcription factor TIF-IA leads to nucleolar disruption, cell cycle arrest, and p53-mediated apoptosis. Mol Cell 19: 77-87. doi: 10.1016/j.molcel.2005.05.023
![]() |
[65] |
Erickson JD, Bazan NG (2013) The nucleolus fine-tunes the orchestration of an early neuroprotection response in neurodegeneration. Cell Death Differ 20: 1435-1437. doi: 10.1038/cdd.2013.107
![]() |
[66] |
Plotkin JL, Day M, Peterson JD, et al. (2014) Impaired TrkB receptor signaling underlies corticostriatal dysfunction in Huntington's disease. Neuron 83: 178-188. doi: 10.1016/j.neuron.2014.05.032
![]() |
[67] |
Lee JH, Tecedor L, Chen YH, et al. (2015) Reinstating aberrant mTORC1 activity in Huntington's disease mice improves disease phenotypes. Neuron 85: 303-315. doi: 10.1016/j.neuron.2014.12.019
![]() |
[68] |
Ravikumar B, Vacher C, Berger Z, et al. (2004) Inhibition of mTOR induces autophagy and reduces toxicity of polyglutamine expansions in fly and mouse models of Huntington disease. Nat Genet 36: 585-595. doi: 10.1038/ng1362
![]() |
[69] |
Hamdane N, Stefanovsky VY, Tremblay MG, et al. (2014) Conditional inactivation of Upstream Binding Factor reveals its epigenetic functions and the existence of a somatic nucleolar precursor body. PLoS Genet 10: e1004505. doi: 10.1371/journal.pgen.1004505
![]() |
1. | Ming-Kai Wang, Cheng Wang, Jun-Feng Yin, A second-order ADI method for pricing options under fractional regime-switching models, 2023, 18, 1556-1801, 647, 10.3934/nhm.2023028 | |
2. | M. YOUSUF, A. Q. M. KHALIQ, PRICING AMERICAN OPTION USING A MODIFIED FRACTIONAL BLACK–SCHOLES MODEL UNDER MULTI-STATE REGIME SWITCHING, 2023, 26, 0219-0249, 10.1142/S021902492350019X | |
3. | Xiaofeng Guo, Jianyu Pan, Approximate inverse preconditioners for linear systems arising from spatial balanced fractional diffusion equations, 2023, 8, 2473-6988, 17284, 10.3934/math.2023884 |
$ N $ | $ \tau $ | $ \|V_{\tau}(\cdot, 0)-V_\ast(\cdot, 0)\|_2 $ | Order | $ \|V_{\tau}(\cdot, 0)-V_\ast(\cdot, 0)\|_\infty $ | Order |
8 | 0.12500000 | 51.4645 | *** | 27.0184 | *** |
16 | 0.06250000 | 27.4790 | 0.9052 | 19.2316 | 0.4905 |
32 | 0.03125000 | 10.3013 | 1.4155 | 6.1728 | 1.6395 |
64 | 0.01562500 | 1.0048 | 3.3578 | 4.9171$ \times 10^{-1} $ | 3.6500 |
128 | 0.00781250 | 3.4284$ \times 10^{-3} $ | 8.1952 | 2.2191$ \times 10^{-4} $ | 11.1136 |
$ N $ | $ \tau $ | $ \|V_{\tau}(\cdot, 0)-V_\ast(\cdot, 0)\|_2 $ | Order | $ \|V_{\tau}(\cdot, 0)-V_\ast(\cdot, 0)\|_\infty $ | Order |
8 | 0.12500000 | 31.7954 | *** | 21.3352 | *** |
16 | 0.06250000 | 13.3594 | 1.2510 | 9.0302 | 1.2404 |
32 | 0.03125000 | 2.0437 | 2.7086 | 1.3213 | 2.7728 |
64 | 0.01562500 | 5.1328$ \times 10^{-3} $ | 8.6372 | 2.7977$ \times 10^{-3} $ | 8.8835 |
128 | 0.00781250 | 7.4134$ \times 10^{-8} $ | 16.0793 | 4.8969$ \times 10^{-9} $ | 19.1239 |
$ N $ | $ \tau $ | $ \|V_{\tau}(\cdot, 0)-V_\ast(\cdot, 0)\|_2 $ | Order | $ \|V_{\tau}(\cdot, 0)-V_\ast(\cdot, 0)\|_\infty $ | Order |
8 | 0.12500000 | 1.7217$ \times10^{-2} $ | *** | 1.1203$ \times10^{-3} $ | *** |
16 | 0.06250000 | 1.3686$ \times10^{-3} $ | 3.6530 | 9.0190$ \times10^{-5} $ | 3.6348 |
32 | 0.03125000 | 9.7572$ \times10^{-5} $ | 3.8101 | 8.1591$ \times10^{-6} $ | 3.4665 |
64 | 0.01562500 | 7.0293$ \times10^{-6} $ | 3.7950 | 1.8366$ \times10^{-6} $ | 2.1514 |
128 | 0.00781250 | 7.8313$ \times10^{-7} $ | 3.1661 | 3.8539$ \times10^{-7} $ | 2.2526 |
$ N $ | $ \tau $ | $ \|V_{\tau}(\cdot, 0)-V_\ast(\cdot, 0)\|_2 $ | Order | $ \|V_{\tau}(\cdot, 0)-V_\ast(\cdot, 0)\|_\infty $ | Order |
8 | 0.12500000 | 33.7205 | *** | 17.8600 | *** |
16 | 0.06250000 | 19.9914 | 0.7542 | 14.8495 | 0.2663 |
32 | 0.03125000 | 8.8330 | 1.1784 | 7.7376 | 0.9405 |
64 | 0.01562500 | 9.9060$ \times 10^{-1} $ | 3.1565 | 7.8221$ \times 10^{-1} $ | 3.3063 |
128 | 0.00781250 | 2.1208$ \times 10^{-3} $ | 8.8676 | 2.3627$ \times 10^{-4} $ | 11.6929 |
$ N $ | $ \tau $ | $ \|V_{\tau}(\cdot, 0)-V_\ast(\cdot, 0)\|_2 $ | Order | $ \|V_{\tau}(\cdot, 0)-V_\ast(\cdot, 0)\|_\infty $ | Order |
8 | 0.12500000 | 22.5121 | *** | 15.7317 | *** |
16 | 0.06250000 | 11.0447 | 1.0274 | 9.6167 | 0.7101 |
32 | 0.03125000 | 1.9755 | 2.4831 | 1.4823 | 2.6977 |
64 | 0.01562500 | 5.2250$ \times 10^{-3} $ | 8.5626 | 3.1998$ \times 10^{-3} $ | 8.8556 |
128 | 0.00781250 | 4.4324$ \times 10^{-8} $ | 16.8470 | 2.9549$ \times 10^{-9} $ | 20.0465 |
$ N $ | $ \tau $ | $ \|V_{\tau}(\cdot, 0)-V_\ast(\cdot, 0)\|_2 $ | Order | $ \|V_{\tau}(\cdot, 0)-V_\ast(\cdot, 0)\|_\infty $ | Order |
8 | 0.12500000 | 1.0414$ \times10^{-2} $ | *** | 6.7754$ \times10^{-4} $ | *** |
16 | 0.06250000 | 8.2617$ \times10^{-4} $ | 3.6559 | 5.4547$ \times10^{-5} $ | 3.6347 |
32 | 0.03125000 | 5.8268$ \times10^{-5} $ | 3.8257 | 3.8767$ \times10^{-6} $ | 3.8146 |
64 | 0.01562500 | 3.8786$ \times10^{-6} $ | 3.9091 | 2.5746$ \times10^{-7} $ | 3.9124 |
128 | 0.00781250 | 3.1080$ \times10^{-7} $ | 3.6415 | 1.5452$ \times10^{-8} $ | 4.0584 |
$ N $ | $ \tau $ | $ \|V_{\tau}(\cdot, 0)-V_\ast(\cdot, 0)\|_2 $ | Order | $ \|V_{\tau}(\cdot, 0)-V_\ast(\cdot, 0)\|_\infty $ | Order |
8 | 0.12500000 | 60.6264 | *** | 29.9147 | *** |
16 | 0.06250000 | 35.9440 | 0.7542 | 24.8172 | 0.2695 |
32 | 0.03125000 | 15.8816 | 1.1784 | 12.9186 | 0.9419 |
64 | 0.01562500 | 1.7811 | 3.1565 | 1.3060 | 3.3062 |
128 | 0.00781250 | 3.6030$ \times10^{-3} $ | 8.9493 | 3.7585$ \times10^{-4} $ | 11.7628 |
$ N $ | $ \tau $ | $ \|V_{\tau}(\cdot, 0)-V_\ast(\cdot, 0)\|_2 $ | Order | $ \|V_{\tau}(\cdot, 0)-V_\ast(\cdot, 0)\|_\infty $ | Order |
8 | 0.12500000 | 40.4761 | *** | 26.3017 | *** |
16 | 0.06250000 | 19.8581 | 1.0273 | 16.0588 | 0.7118 |
32 | 0.03125000 | 3.5519 | 2.4831 | 2.4759 | 2.6974 |
64 | 0.01562500 | 9.3945$ \times10^{-3} $ | 8.5626 | 5.3429$ \times10^{-3} $ | 8.8561 |
128 | 0.00781250 | 7.9967$ \times10^{-8} $ | 16.8421 | 5.0636$ \times10^{-9} $ | 20.0090 |
$ N $ | $ \tau $ | $ \|V_{\tau}(\cdot, 0)-V_\ast(\cdot, 0)\|_2 $ | Order | $ \|V_{\tau}(\cdot, 0)-V_\ast(\cdot, 0)\|_\infty $ | Order |
8 | 0.12500000 | 1.9302$ \times10^{-2} $ | *** | 1.0964$ \times10^{-3} $ | *** |
16 | 0.06250000 | 1.5287$ \times10^{-3} $ | 3.6584 | 8.8458$ \times10^{-5} $ | 3.6316 |
32 | 0.03125000 | 1.0763$ \times10^{-4} $ | 3.8281 | 6.3074$ \times10^{-6} $ | 3.8099 |
64 | 0.01562500 | 7.1519$ \times10^{-6} $ | 3.9117 | 4.3025$ \times10^{-7} $ | 3.8738 |
128 | 0.00781250 | 5.1858$ \times10^{-7} $ | 3.7857 | 3.6863$ \times10^{-8} $ | 3.5449 |
$ N $ | $ \tau $ | $ \|V_{\tau}(\cdot, 0)-V_\ast(\cdot, 0)\|_2 $ | Order | $ \|V_{\tau}(\cdot, 0)-V_\ast(\cdot, 0)\|_\infty $ | Order |
8 | 0.12500000 | 51.4645 | *** | 27.0184 | *** |
16 | 0.06250000 | 27.4790 | 0.9052 | 19.2316 | 0.4905 |
32 | 0.03125000 | 10.3013 | 1.4155 | 6.1728 | 1.6395 |
64 | 0.01562500 | 1.0048 | 3.3578 | 4.9171$ \times 10^{-1} $ | 3.6500 |
128 | 0.00781250 | 3.4284$ \times 10^{-3} $ | 8.1952 | 2.2191$ \times 10^{-4} $ | 11.1136 |
$ N $ | $ \tau $ | $ \|V_{\tau}(\cdot, 0)-V_\ast(\cdot, 0)\|_2 $ | Order | $ \|V_{\tau}(\cdot, 0)-V_\ast(\cdot, 0)\|_\infty $ | Order |
8 | 0.12500000 | 31.7954 | *** | 21.3352 | *** |
16 | 0.06250000 | 13.3594 | 1.2510 | 9.0302 | 1.2404 |
32 | 0.03125000 | 2.0437 | 2.7086 | 1.3213 | 2.7728 |
64 | 0.01562500 | 5.1328$ \times 10^{-3} $ | 8.6372 | 2.7977$ \times 10^{-3} $ | 8.8835 |
128 | 0.00781250 | 7.4134$ \times 10^{-8} $ | 16.0793 | 4.8969$ \times 10^{-9} $ | 19.1239 |
$ N $ | $ \tau $ | $ \|V_{\tau}(\cdot, 0)-V_\ast(\cdot, 0)\|_2 $ | Order | $ \|V_{\tau}(\cdot, 0)-V_\ast(\cdot, 0)\|_\infty $ | Order |
8 | 0.12500000 | 1.7217$ \times10^{-2} $ | *** | 1.1203$ \times10^{-3} $ | *** |
16 | 0.06250000 | 1.3686$ \times10^{-3} $ | 3.6530 | 9.0190$ \times10^{-5} $ | 3.6348 |
32 | 0.03125000 | 9.7572$ \times10^{-5} $ | 3.8101 | 8.1591$ \times10^{-6} $ | 3.4665 |
64 | 0.01562500 | 7.0293$ \times10^{-6} $ | 3.7950 | 1.8366$ \times10^{-6} $ | 2.1514 |
128 | 0.00781250 | 7.8313$ \times10^{-7} $ | 3.1661 | 3.8539$ \times10^{-7} $ | 2.2526 |
$ N $ | $ \tau $ | $ \|V_{\tau}(\cdot, 0)-V_\ast(\cdot, 0)\|_2 $ | Order | $ \|V_{\tau}(\cdot, 0)-V_\ast(\cdot, 0)\|_\infty $ | Order |
8 | 0.12500000 | 33.7205 | *** | 17.8600 | *** |
16 | 0.06250000 | 19.9914 | 0.7542 | 14.8495 | 0.2663 |
32 | 0.03125000 | 8.8330 | 1.1784 | 7.7376 | 0.9405 |
64 | 0.01562500 | 9.9060$ \times 10^{-1} $ | 3.1565 | 7.8221$ \times 10^{-1} $ | 3.3063 |
128 | 0.00781250 | 2.1208$ \times 10^{-3} $ | 8.8676 | 2.3627$ \times 10^{-4} $ | 11.6929 |
$ N $ | $ \tau $ | $ \|V_{\tau}(\cdot, 0)-V_\ast(\cdot, 0)\|_2 $ | Order | $ \|V_{\tau}(\cdot, 0)-V_\ast(\cdot, 0)\|_\infty $ | Order |
8 | 0.12500000 | 22.5121 | *** | 15.7317 | *** |
16 | 0.06250000 | 11.0447 | 1.0274 | 9.6167 | 0.7101 |
32 | 0.03125000 | 1.9755 | 2.4831 | 1.4823 | 2.6977 |
64 | 0.01562500 | 5.2250$ \times 10^{-3} $ | 8.5626 | 3.1998$ \times 10^{-3} $ | 8.8556 |
128 | 0.00781250 | 4.4324$ \times 10^{-8} $ | 16.8470 | 2.9549$ \times 10^{-9} $ | 20.0465 |
$ N $ | $ \tau $ | $ \|V_{\tau}(\cdot, 0)-V_\ast(\cdot, 0)\|_2 $ | Order | $ \|V_{\tau}(\cdot, 0)-V_\ast(\cdot, 0)\|_\infty $ | Order |
8 | 0.12500000 | 1.0414$ \times10^{-2} $ | *** | 6.7754$ \times10^{-4} $ | *** |
16 | 0.06250000 | 8.2617$ \times10^{-4} $ | 3.6559 | 5.4547$ \times10^{-5} $ | 3.6347 |
32 | 0.03125000 | 5.8268$ \times10^{-5} $ | 3.8257 | 3.8767$ \times10^{-6} $ | 3.8146 |
64 | 0.01562500 | 3.8786$ \times10^{-6} $ | 3.9091 | 2.5746$ \times10^{-7} $ | 3.9124 |
128 | 0.00781250 | 3.1080$ \times10^{-7} $ | 3.6415 | 1.5452$ \times10^{-8} $ | 4.0584 |
$ N $ | $ \tau $ | $ \|V_{\tau}(\cdot, 0)-V_\ast(\cdot, 0)\|_2 $ | Order | $ \|V_{\tau}(\cdot, 0)-V_\ast(\cdot, 0)\|_\infty $ | Order |
8 | 0.12500000 | 60.6264 | *** | 29.9147 | *** |
16 | 0.06250000 | 35.9440 | 0.7542 | 24.8172 | 0.2695 |
32 | 0.03125000 | 15.8816 | 1.1784 | 12.9186 | 0.9419 |
64 | 0.01562500 | 1.7811 | 3.1565 | 1.3060 | 3.3062 |
128 | 0.00781250 | 3.6030$ \times10^{-3} $ | 8.9493 | 3.7585$ \times10^{-4} $ | 11.7628 |
$ N $ | $ \tau $ | $ \|V_{\tau}(\cdot, 0)-V_\ast(\cdot, 0)\|_2 $ | Order | $ \|V_{\tau}(\cdot, 0)-V_\ast(\cdot, 0)\|_\infty $ | Order |
8 | 0.12500000 | 40.4761 | *** | 26.3017 | *** |
16 | 0.06250000 | 19.8581 | 1.0273 | 16.0588 | 0.7118 |
32 | 0.03125000 | 3.5519 | 2.4831 | 2.4759 | 2.6974 |
64 | 0.01562500 | 9.3945$ \times10^{-3} $ | 8.5626 | 5.3429$ \times10^{-3} $ | 8.8561 |
128 | 0.00781250 | 7.9967$ \times10^{-8} $ | 16.8421 | 5.0636$ \times10^{-9} $ | 20.0090 |
$ N $ | $ \tau $ | $ \|V_{\tau}(\cdot, 0)-V_\ast(\cdot, 0)\|_2 $ | Order | $ \|V_{\tau}(\cdot, 0)-V_\ast(\cdot, 0)\|_\infty $ | Order |
8 | 0.12500000 | 1.9302$ \times10^{-2} $ | *** | 1.0964$ \times10^{-3} $ | *** |
16 | 0.06250000 | 1.5287$ \times10^{-3} $ | 3.6584 | 8.8458$ \times10^{-5} $ | 3.6316 |
32 | 0.03125000 | 1.0763$ \times10^{-4} $ | 3.8281 | 6.3074$ \times10^{-6} $ | 3.8099 |
64 | 0.01562500 | 7.1519$ \times10^{-6} $ | 3.9117 | 4.3025$ \times10^{-7} $ | 3.8738 |
128 | 0.00781250 | 5.1858$ \times10^{-7} $ | 3.7857 | 3.6863$ \times10^{-8} $ | 3.5449 |