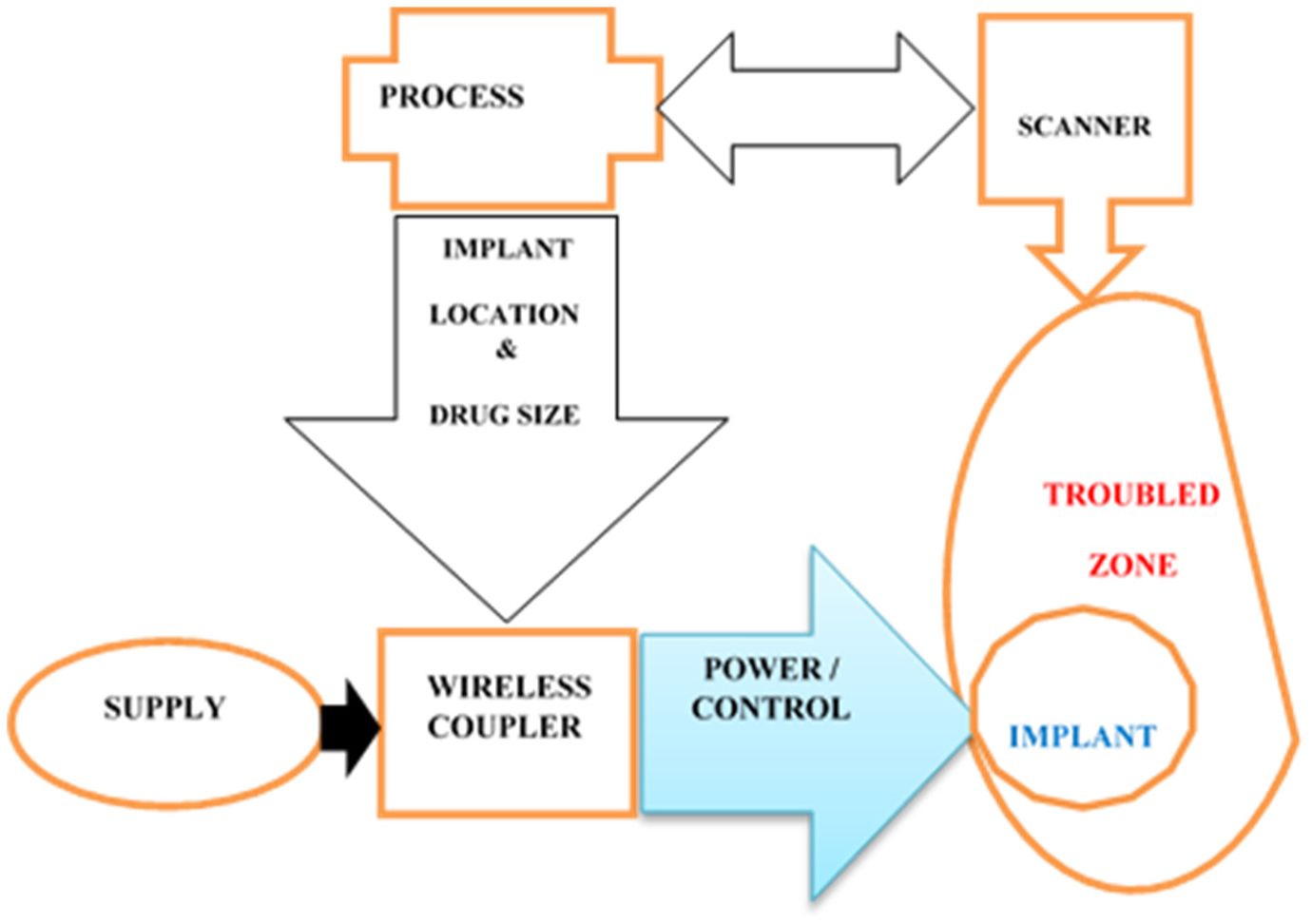
Citation: Adel Razek. Augmented therapeutic tutoring in diligent image-assisted robotic interventions[J]. AIMS Medical Science, 2024, 11(2): 210-219. doi: 10.3934/medsci.2024016
[1] | Masahiro Yasunaga, Shino Manabe, Masaru Furuta, Koretsugu Ogata, Yoshikatsu Koga, Hiroki Takashima, Toshirou Nishida, Yasuhiro Matsumura . Mass spectrometry imaging for early discovery and development of cancer drugs. AIMS Medical Science, 2018, 5(2): 162-180. doi: 10.3934/medsci.2018.2.162 |
[2] | Maribeth Porter, Jillian Harvey, Jennifer K. Gavin, Matthew J. Carpenter, K. Michael Cummings, Charlene Pope, Vanessa A. Diaz . A Qualitative Study to Assess Factors Supporting Tobacco Use in A Homeless Population. AIMS Medical Science, 2017, 4(1): 83-98. doi: 10.3934/medsci.2017.1.83 |
[3] | Timothy Hamerly, Margaret H. Butler, Steve T. Fisher, Jonathan K. Hilmer, Garth A. James, Brian Bothner . Mass Spectrometry Imaging of Chlorhexidine and Bacteria in a Model Wound. AIMS Medical Science, 2015, 2(3): 150-161. doi: 10.3934/medsci.2015.3.150 |
[4] | Margarida Pujol-López, Luis Ortega-Paz, Manel Garabito, Salvatore Brugaletta, Manel Sabaté, Ana Paula Dantas . miRNA Update: A Review Focus on Clinical Implications of miRNA in Vascular Remodeling. AIMS Medical Science, 2017, 4(1): 99-112. doi: 10.3934/medsci.2017.1.99 |
[5] | Sanjay Konakondla, Steven A. Toms . Cerebral Connectivity and High-grade Gliomas: Evolving Concepts of Eloquent Brain in Surgery for Glioma. AIMS Medical Science, 2017, 4(1): 52-70. doi: 10.3934/medsci.2017.1.52 |
[6] | Maureen C. Ashe, Khalil Merali, Nicola Edwards, Claire Schiller, Heather M. Hanson, Lena Fleig, Karim M. Khan, Wendy L. Cook, Heather A. McKay . Integrating research into clinical practice for hip fracture rehabilitation: Implementation of a pragmatic RCT. AIMS Medical Science, 2018, 5(2): 102-121. doi: 10.3934/medsci.2018.2.102 |
[7] | Nitin Chandra Mohan, Thomas W. Johnson . Role of intracoronary imaging in severely calcific disease. AIMS Medical Science, 2024, 11(4): 388-402. doi: 10.3934/medsci.2024027 |
[8] | Turki M. Bin Mahfoz, Ahmad K. Alnemare . Optic neuropathy related to Onodi cell mucocele: a systematic review and meta-analysis of randomized controlled trials. AIMS Medical Science, 2021, 8(3): 203-223. doi: 10.3934/medsci.2021018 |
[9] | Yonggang Cui, Giuseppe S. Camarda, Anwar Hossain, Ge Yang, Utpal N. Roy, Terry Lall, Ralph B. James . Modeling an Interwoven Collimator for A 3D Endocavity Gamma Camera. AIMS Medical Science, 2016, 3(1): 114-125. doi: 10.3934/medsci.2016.1.114 |
[10] | Lena Fleig, Maureen C. Ashe, Jan Keller, Sonia Lippke, Ralf Schwarzer . Putting psychology into telerehabilitation: Coping planning as an example for how to integrate behavior change techniques into clinical practice. AIMS Medical Science, 2019, 6(1): 13-32. doi: 10.3934/medsci.2019.1.13 |
Recently, medical interventions have moved from completely intrusive interventions, through the medical team's tactile skills and direct visual observations, to adjusted, image-guided, closed-loop robotic processes. These precise and minimally intrusive procedures primarily involve complex surgical procedures and drug delivery, which can be supervised by medical staff. In these two intervention categories, local position accuracy is crucial for strictly covering the affected area without affecting the surroundings. Thus, patient well-being (comfort and safety) and staff performance (accuracy and efficiency) shape a successful medical outcome. This advancement is not only beneficial for humans but follows the “One Health” concept regarding humans, animals, and ecosystems [1]–[3]. This concept was established to describe the awareness that human health is convolutedly associated with other animals and their environments. This is normally linked to vital threats but also to improvements.
In the image-assisted robotic process mentioned above (replacing human fingers and vision), image assistance could be achieved using predetermined digitized images of the relevant body area. A more effective and instantly controlled method is to directly use an imaging scanner. This solution, even more expensive, avoids doubts and possible interruptions of the procedure. In this case, the robotic process is assisted by an instantaneous image. In theory, all types of scanners could be used in these image-guided robotic interventions; different categories of scanners are normally used, each tailored to a specific target. An important selection factor is the usage interval: For treatments at long intervals, it is preferable to avoid scanners reflecting ionizing radiation. Thus, the relatively long exposure interval of image-guided interventions suggests the use of non-ionizing scanners, namely magnetic resonance imaging (MRI) and ultrasound scanners (see e.g., [4]–[7]). The first is more complex and expensive but works with all tissues of the body and is more precise. Caution is advised when using MRI due to its sensitivity to electromagnetic noise. The ultrasound scanner is almost portable and adequate for easy daily use but does not work in bones or air cavities. In general, image-assisted robotic interventions are minimally invasive and non-ionizing. Different dedicated scanners have been built to allow the introduction of different body parts inside the imaging scaffold. In addition, in the case of MRI, the robot and medical tools are made from materials not sensitive to electromagnetic fields, called MRI-compatible. Several investigations and clinical applications could be found in the literature: see e.g., [8]–[10].
The closed-loop, quasi-autonomous image-guided robotic procedure, in addition to having a beneficial impact on patient well-being, can enable medical staff to perform useful tasks in the absence of the patient. These tasks are primarily related to the exploration of new therapies and treatments as well as training exercises and testing. This could be done using “copies” of the patient's body: a physical phantom constructed from materials matching the biological properties of real body tissues. Regarding training, exercises performed by medical staff on physical phantoms help to reveal the most suitable treatment for the real patient. Another type of body copy is a digital replica matching real tissue properties; it would be a digital phantom. These physical and digital phantoms make it possible to investigate different scenarios without risk for the patient, but the construction of such physical and digital phantoms is not without problems. Physical phantoms have been used in conventional medical testing for a while and can be easily found on the market. Much research relating to digital constructs can be found in the literature (e.g., [11]–[15]).
The purpose of this commentary is to analyze and illustrate the possibilities of increasing the capabilities of medical personnel to manage training exercises for image-assisted robotic diligent interventions. The contribution will focus on the use of the physical-virtual digital twin concept to supervise image-assisted robotic interventions, thus reducing the complexity and uncertainties involved in such a procedure.
Section 2 illustrates the image-assisted robotic intervention procedure and analyzes the characteristics of physical and digital tissue phantoms. The representation of the dynamic behavior of tissues will be highlighted via the concept of complexity. Section 3 presents the characters of the physical-virtual pairs and their roles in reducing the complexity issue. Section 4 concerns the use of the physical-virtual digital twin concept in healthcare, focusing on its use in image-assisted robotic interventions and its extension to human-in-the-loop DT. Section 5 concerns the therapeutic tutoring of medical staff; disease monitoring and diagnosis, general training, and personalized planning training are also analyzed.
As mentioned previously, image-assisted robotic interventions enable minimally invasive and generally non-ionizing procedures, allowing for high-precision localization. Thus, these interventions are mainly used for complex surgical procedures and restricted drug delivery. Figure 1 illustrates an example of an autonomous, collaborative, autonomous, image-assisted restricted drug delivery system.
A fully image-assisted robotic intervention can be performed with a physical phantom. Additionally, a digital phantom could be used in a digitally simulated intervention. The main difficulty of these two options lies in the representation of the tissues. Accounting for biological assets allows the construction of these phantom representations with static behavior. Such a static picture could be appropriate for specific living tissues and applications. However, as mentioned earlier, physical and digital phantoms should approach real living tissues through realistic biological properties. In general, the deformation and movement (mechanical behavior) of living soft tissues, irrigated by the fluids that enable their functioning, correspond to a complex dynamic behavior model [16]–[20]. This complex behavior of living tissues requires real, estimated constitutive laws, adjusted computational methods, or both to solve this open research problem. We can only approach this type of model or representation in an approximate manner.
The notion of complexity is implicated in many natural and artificial phenomena [21] as well as societal concerns. Thus, complexity could be found in various areas, e.g., neuroscience [22], global climate [23], space shuttles [24], computer image processing [25], fair division economics [26], strategic interactions in game theory [27], politics and governments [28], and history sciences [29],[30]. These composite activities interact in complex ways, exhibiting nonlinear, codependent spatiotemporal behaviors.
A suitable solution to the complexity of dynamic tissue behavior is the use of physical representation and digital replication in a tailored procedure. Such a coupling process is well suited to uncertain (approximate) physical and numerical aspects. Matching iterations allow each side of the physical-digital pair to correct the other, thereby reducing physical and digital uncertainties [31]. This matching twin corresponds to the concept of digital twin (DT) introduced by Grieves in 2001 [32]. DTs are used in many applications (e.g., [33]–[35]) in different fields such as industrial manufacturing and production, mobility, aeronautics, space, or health. This strategy makes it possible to supervise complex dynamic procedures in real-time, reducing uncertainties and controlling all unforeseen events and undesirable and dangerous behaviors. Another particularity of DT is that the virtual model is supposed to be precise and very close to the real procedure. For a complex procedure, its model would also be complex, therefore with a high calculation time leading to slow and degraded matching. For fast matching, we need to reduce the computation time by using strategies to preserve the accuracy of key attributes. Thus, reduced order or surrogate models can be used for this purpose; see e.g., [36],[37].
Many applications in the health field use virtual replicas of physical entities, which go further than a static image, integrating the dynamic behavior of a real living character [38]–[44].
In the present study, the concept of DT could be used to monitor the entire image-assisted robotic intervention system [45], including a physical phantom of the concerned part of human tissue; this represents the true physical side of the twin. A virtual model involving the digital phantom replica of the real side will represent the virtual side of the twin. Between the two sides is a link involving the matching and processing of information (Figure 2).
In principle, the concept of DT can be used with real patient tissues, which can enable precise supervision of the intervention. In such a case, we can use a modified DT by including a human-in-the-loop. This corresponds to a human–robot interaction associated with DT, called HDT [46]–[48], which allows a predominantly human manipulation in the procedure, thus minimizing the risks for the patient. HDT requires access to numerous health data sources, including genetic and historical records, Internet of Things (IoT), and other appropriate providers. Data is processed through advanced analytics and artificial intelligence (AI) methods, including deep learning models that can improve the intelligence of HDT simulations, enabling predictive models and confirmation of decision-making. Indeed, the involvement of AI practices in image-assisted robotic intervention contributes to reducing the complexity of information gaining and post-processing in imaging through the practice of acceleration approach, with quicker analysis times and simpler image processing [49],[50]. AI can also be used to perform planned repeated training jobs in image-assisted robotics.
DT has the promise to transform patient care by delivering progressively tailored, data-driven medical treatment. Its application is a part of digital medicine and is generally used in personalized medical treatments, which can be the observation and diagnosis of diseases, tutoring, or interventions. DTs are often associated with AI and augmented reality (AR) tools, as well as virtual reality (VR) and chatbots. Increased interaction between the human and the robot increases the system's performance through AR-assisted robotic operations. Also, VR training improves the ability to mimic real-world exercise scenarios with the skill to correctly quantify performance, while chatbots allow training via the intelligent creation of tutoring paths. Tutoring primarily involves planning a personalized case or the exploration of new therapies through general training.
DTs allow medical staff to supervise the evolution of disorders and adjust care strategies, choosing the most appropriate therapy, thus enabling the development of personalized and early-diagnosis procedures and research of new medications or interventions [51],[52].
The elementary DT training of younger staff is related to the creation of virtual anatomy and bodily processes models to allow practicing, enhance dexterity, and refine their understanding of the human body tissues. DT general staff training includes acting out healthcare scenarios, getting vital marks, dispensing drugs, and facing urgent situations. This can be related to patient concerns, e.g., cardiac arrests, or environmental conditions, e.g., fires. In addition, DT allows training on the functioning and safeguarding of medical tools and infection-handling routines [38],[39].
Personal DT patient planning allows medical staff to predict the disruptions that patients may experience during procedures. This could be related to tools, drugs, or implementations. Furthermore, DT allows staff, in a safe and patient-free situation, to practice procedures, make judgments, and understand possible errors of interpretation [45],[53]–[56].
This commentary focused on the analysis of image-assisted robotics, complex surgery, and restricted drug delivery, which require high-precision positioning for minimally invasive and safe procedures. The uncertainty in the representation of the dynamic behavior of living tissues is reduced by a matched physical-virtual pair applied to interventional procedures. These developments enable effective training of staff on tissue copies in the case of general information as well as planning personalized interventions. The various subjects concerned were supported by a review of the literature. It should be noted that, although matched pairs could reduce the uncertainty of the tissue dynamics model, this model is still difficult to determine and corresponds to an unresolved open challenge.
Future prospects include improving robotic assistance by the scanner using AR and AI tools, achieving complete automation, ensuring patient safety, and utilizing DT in real-time. This allows for more precise, minimally intrusive restricted actions with the option of firm human control. One of the most challenging prospects involves the real-time virtual representation of tissues (dynamic model) within DT, as mentioned before. The main challenge is the nonlinear tissue behavior, which exhibits complex responses to deformation and displacement of elastic tissues.
The author declares he has not used Artificial Intelligence (AI) tools in the creation of this article.
[1] | World Health Organization, One Health—One Health Technical Advisory Group First meeting 31 Oct-1 Nov 2022, Concept note. World Health Organization, 2023. Available from: https://www.who.int/europe/initiatives/one-health. Accessed March 14, 2024. |
[2] | Centers for Disease Control and Prevention, One Health. Atlanta Centers for Disease Control and Prevention, 2023. Available from: https://www.cdc.gov/onehealth/index.html. Accessed March 14, 2024. |
[3] |
Pitt SJ, Gunn A (2024) The one health concept. Br J Biomed Sci 81: 12366. https://doi.org/10.3389/bjbs.2024.12366 ![]() |
[4] |
Huang S, Lou C, Zhou Y, et al. (2023) MRI-guided robot intervention—current state-of-the-art and new challenges. Med-X 1: 4. https://doi.org/10.1007/s44258-023-00003-1 ![]() |
[5] |
Faoro G, Maglio S, Pane S, et al. (2023) An artificial intelligence-aided robotic platform for ultrasound-guided transcarotid revascularization. In: Ozyar, E., Onal, C., Hackett, S.L., Editors,. IEEE Robot Autom Lett 8: 2349-2356. https://doi.org/10.1109/LRA.2023.3251844 ![]() |
[6] | Morris ED, O'Connell DP, Gao Y, et al. (2023) MR safety considerations for MRI-guided radiotherapy, In: Ozyar, E., Onal, C., Hackett, S.L., Editors,. Advances in Magnetic Resonance Technology and Applications. Cambridge: Academic Press, 8: 81-100. https://doi.org/10.1016/B978-0-323-91689-9.00005-4 |
[7] |
Su H, Kwok KW, Cleary K, et al. (2022) State of the art and future opportunities in MRI-guided robot-assisted surgery and interventions. Proc IEEE 110: 968-992. https://doi.org/10.1109/jproc.2022.3169146 ![]() |
[8] |
Farooq MU, Ko SY (2023) A decade of MRI compatible robots: systematic review. IEEE T Robot 39: 862-884. https://doi.org/10.1109/TRO.2022.3212626 ![]() |
[9] |
Farooq MU, Ko SY, Seung S, et al. (2023) An MRI-compatible endonasal surgical robotic system: Kinematic analysis and performance evaluation. Mechatronics 94: 103029. https://doi.org/10.1016/j.mechatronics.2023.103029 ![]() |
[10] |
Manjila S, Rosa B, Price K, et al. (2023) Robotic instruments inside the MRI bore: key concepts and evolving paradigms in imaging-enhanced cranial neurosurgery. World Neurosurg 176: 127-139. https://doi.org/10.1016/j.wneu.2023.01.025 ![]() |
[11] |
Gabriel S, Lau RW, Gabriel C (1996) The dielectric properties of biological tissues: II. measurements in the frequency range 10 Hz to 20 GHz. Phys Med Biol 41: 2251-2269. https://doi.org/10.1088/0031-9155/41/11/002 ![]() |
[12] |
Barchanski A, Steiner T, De Gersem H, et al. (2006) Local Grid refinement for low-frequency current computations in 3-D Human anatomy models. IEEE Trans Magn 42: 1371-1374. https://doi.org/10.1109/TMAG.2006.871449 ![]() |
[13] | Hasgall P, Di Gennaro F, Baumgartner C, et al. (2022) IT'IS database for thermal and electromagnetic parameters of biological tissues. Available from: https://itis.swiss/virtual-population/tissue-properties/overview/. Accessed November 1, 2023. |
[14] |
Makarov SN, Noetscher GM, Yanamadala J, et al. (2017) Virtual human models for electromagnetic studies and their applications. IEEE Rev Biomed Eng 10: 95-121. https://doi.org/10.1109/RBME.2017.2722420 ![]() |
[15] | Noetscher GM (2020) The CAD-compatible VHP-male computational phantom, In: Makarov, S.N., Noetscher, G.M., Nummenmaa, A., Editors,. Brain and Human Body Modeling 2020. Cham: Springer, 309-323. https://doi.org/10.1007/978-3-030-45623-8_19 |
[16] | Humphrey JD (2008) Biological Soft Tissues, In: Sharpe, W., Editor,. Springer Handbook of Experimental Solid Mechanics. Boston: Springer. https://doi.org/10.1007/978-0-387-30877-7_7 |
[17] | Kallin S (2019) Deformation of human soft tissues: experimental and numerical aspects. Jönköping University, Licentiate thesis. Available from: https://hj.diva-portal.org/smash/get/diva2:1344790/FULLTEXT01.pdf. Accessed March 17, 2024. |
[18] |
Al-Dirini RMA, Reed MP, Hu JW, et al. (2016) Development and validation of a high anatomical fidelity FE model for the buttock and thigh of a seated individual. Ann Biomed Eng 44: 2805-2816. https://doi.org/10.1007/s10439-016-1560-3 ![]() |
[19] | Fung YC (1993) Biomechanics: Mechanical Properties of Living Tissues. 2 Eds., New York: Springer. https://doi.org/10.1007/978-1-4757-2257-4 |
[20] |
Henninger HB, Reese SP, Anderson AE, et al. (2010) Validation of computational models in biomechanics. Proc Inst Mech Eng H 224: 801-812. https://doi.org/10.1243/09544119JEIM649 ![]() |
[21] |
Razek A (2024) Matching of an observed event and its virtual model in relation to smart theories, coupled models and supervision of complex procedures—a review. Comptes Rendus Physique 25: 1-16. https://doi.org/10.5802/crphys.184 ![]() |
[22] |
Monteiro J, Pedro A, Silva AJ (2022) A Gray Code model for the encoding of grid cells in the Entorhinal Cortex. Neural Comput Applic 34: 2287-2306. https://doi.org/10.1007/s00521-021-06482-w ![]() |
[23] |
Wang F, Tian D (2022) On deep learning-based bias correction and downscaling of multiple climate models simulations. Clim Dyn 59: 3451-3468. https://doi.org/10.1007/s00382-022-06277-2 ![]() |
[24] |
Pendergraft JG, Carter DR, Tseng S, et al. (2019) Learning from the past to advance the future: the adaptation and resilience of NASA's spaceflight multiteam systems across four eras of spaceflight. Front Psychol 10: 1633. https://doi.org/10.3389/fpsyg.2019.01633 ![]() |
[25] | Mittal V, Bhushan B (2020) Accelerated computer vision inference with AI on the edge. 2020 IEEE 9th Int Conference on Communication Systems and Network Technologies (CSNT), Gwalior, India, 2020: 55-60. https://doi.org/10.1109/CSNT48778.2020.9115770 |
[26] |
Amanatidis G, Aziz H, Birmpas G, et al. (2023) Fair division of indivisible goods: recent progress and open questions. Artif Intell 322: 103965. https://doi.org/10.1016/j.artint.2023.103965 ![]() |
[27] | Harris K, Anagnostides I, Farina G, et al. (2022) Meta-learning in games. arXiv preprint arXiv: 2209.14110 . https://doi.org/10.48550/arXiv.2209.14110 |
[28] |
Esposito G, Terlizzi A (2023) Governing wickedness in megaprojects: discursive and institutional perspectives. Policy Soc 42: 131-147. https://doi.org/10.1093/polsoc/puad002 ![]() |
[29] |
Zonneveld KAF, Harper K, Klügel A, et al. (2024) Climate change, society, and pandemic disease in Roman Italy between 200 BCE and 600 CE. Sci Adv 10: eadk1033. https://doi.org/10.1126/sciadv.adk1033 ![]() |
[30] | Harper K (2019) Comment l'empire romain s'est effondré. Le climat, les maladies et la chute de Rome (How the Roman Empire Collapsed. Climate, disease and the fall of Rome). Available from: https://www.editionsladecouverte.fr/comment_l_empire_romain_s_est_effondre-9782348037146 |
[31] | Razek A (2023) Strategies for managing models regarding environmental confidence and complexity involved in intelligent control of energy systems—a review. Adv Environ Energies 2. https://doi.org/10.58396/aee020104 |
[32] |
Grieves M, Vickers J (2017) Digital twin: mitigating unpredictable, undesirable emergent behavior in complex systems, In: Kahlen, J., Flumerfelt, S., Alves, A., Editors,. Transdisciplinary Perspectives on Complex Systems. Cham: Springer, 85-113. https://doi.org/10.1007/978-3-319-38756-7_4 ![]() |
[33] |
Tao F, Sui F, Liu A, et al. (2019) Digital twin-driven product design framework. Int J Prod Res 57: 3935-3953. https://doi.org/10.1080/00207543.2018.1443229 ![]() |
[34] |
He B, Bai KJ (2021) Digital twin-based sustainable intelligent manufacturing: a review. Adv Manuf 9: 1-21. https://doi.org/10.1007/s40436-020-00302-5 ![]() |
[35] |
Rassõlkin A, Rjabtšikov V, Kuts V, et al. (2022) Interface development for digital twin of an electric motor based on empirical performance model. IEEE Access 10: 15635-15643. https://doi.org/10.1109/ACCESS.2022.3148708 ![]() |
[36] | Guo Z, Yan S, Xu X, et al. (2022) Twin-model based on model order reduction for rotating motors. IEEE Trans Magn 58: 1-4. https://doi.org/10.1109/TMAG.2022.3187620 |
[37] |
Kudela J, Matousek R (2022) Recent advances and applications of surrogate models for finite element method computations: a review. Soft Comput 26: 13709-13733. https://doi.org/10.1007/s00500-022-07362-8 ![]() |
[38] | Sun T, He X, Li Z (2023) Digital twin in healthcare: recent updates and challenges. Digit Health 9: 20552076221149651. https://doi.org/10.1177/20552076221149651 |
[39] |
Sun T, He X, Song X, et al. (2022) The digital twin in medicine: a key to the future of healthcare. Front Med 9: 907066. https://doi.org/10.3389/fmed.2022.907066 ![]() |
[40] |
De Benedictis A, Mazzocca N, Somma A, et al. (2022) Digital twins in healthcare: an architectural proposal and its application in a social distancing case study. IEEE J Biomed Health Inform 27: 5143-5154. https://doi.org/10.1109/JBHI.2022.3205506 ![]() |
[41] |
Haleem A, Javaid M, Singh RP, et al. (2023) Exploring the revolution in healthcare systems through the applications of digital twin technology. Biomed Technol 4: 28-38. https://doi.org/10.1016/j.bmt.2023.02.001 ![]() |
[42] |
Ricci A, Croatti A, Montagna S (2022) Pervasive and connected digital twins—a vision for digital health. IEEE Internet Comput 26: 26-32. https://doi.org/10.1109/MIC.2021.3052039 ![]() |
[43] |
Das C, Mumu AA, Ali MF, et al. (2022) Toward IoRT collaborative digital twin technology enabled future surgical sector: technical innovations, opportunities and challenges. IEEE Access 10: 129079-129104. https://doi.org/10.1109/ACCESS.2022.3227644 ![]() |
[44] | Strobel G, Möller F, van der Valk H (2022) Healthcare in the era of digital twins: towards a domain-specific taxonomy. Proceedings of the 30th European Conference on Information Systems, Timişoara, Romania, 2022. Available from: https://www.researchgate.net/publication/360086393_HEALTHCARE_IN_THE_ERA_OF_DIGITAL_TWINS_TOWARDS_A_DOMAIN-SPECIFIC_TAXONOMY. Accessed November 1, 2023. |
[45] |
Razek A (2023) Image-guided surgical and pharmacotherapeutic routines as part of diligent medical treatment. Appl Sci 13: 13039. https://doi.org/10.3390/app132413039 ![]() |
[46] |
Song Y (2023) Human digital twin, the development and impact on design. J Comput Inf Sci Eng 23: 060819. https://doi.org/10.1115/1.4063132 ![]() |
[47] |
Hagmann K, Hellings-Kuß A, Klodmann J, et al. (2021) A digital twin approach for contextual assistance for surgeons during surgical robotics training. Front Robot AI 8: 735566. https://doi.org/10.3389/frobt.2021.735566 ![]() |
[48] | Okegbile SD, Cai J (2022) Edge-assisted human-to-virtual twin connectivity scheme for human digital twin frameworks. 2022 IEEE 95th Vehicular Technology Conference: (VTC2022-Spring), Helsinki, Finland, 2022: 1-6. https://doi.org/10.1109/VTC2022-Spring54318.2022.9860619 |
[49] | Moodley D, Seebregts C (2023) Re-imagining health and well-being in low resource African settings using an augmented AI system and a 3D digital twin. arXiv preprint arXiv:2306.01772 . https://doi.org/10.48550/arXiv.2306.01772 |
[50] |
Shimron E, Perlman O (2023) AI in MRI: computational frameworks for a faster, optimized, and automated imaging workflow. Bioengineering 10: 492. https://doi.org/10.3390/bioengineering10040492 ![]() |
[51] |
Kukushkin K, Ryabov Y, Borovkov A (2022) Digital twins: a systematic literature review based on data analysis and topic modeling. Data 7: 173. https://doi.org/10.3390/data7120173 ![]() |
[52] |
Armeni P, Polat I, De Rossi LM, et al. (2022) Digital twins in healthcare: is it the beginning of a new era of evidence-based medicine? A critical review. J Pers Med 12: 1255. https://doi.org/10.3390/jpm12081255 ![]() |
[53] |
Sears VA, Morris JM (2022) Establishing a point-of-care virtual planning and 3D printing program. Semin Plast Surg 36: 133-148. https://doi.org/10.1055/s-0042-1754351 ![]() |
[54] |
Elkefi S, Asan O (2022) Digital twins for managing health care systems: rapid literature review. J Med Internet Res 24: e37641. https://doi.org/10.2196/37641 ![]() |
[55] |
Björnsson B, Borrebaeck C, Elander N, et al. (2020) Digital twins to personalize medicine. Genome Med 12: 4. https://doi.org/10.1186/s13073-019-0701-3 ![]() |
[56] |
Cellina M, Cè M, Alì M, et al. (2023) Digital twins: the new frontier for personalized medicine?. Appl Sci 13: 7940. https://doi.org/10.3390/app13137940 ![]() |