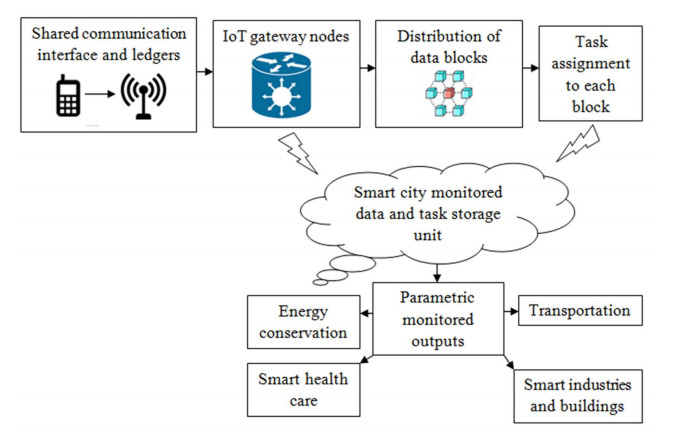
The security of the Internet of Things (IoT) is crucial in various application platforms, such as the smart city monitoring system, which encompasses comprehensive monitoring of various conditions. Therefore, this study conducts an analysis on the utilization of blockchain technology for the purpose of monitoring Internet of Things (IoT) systems. The analysis is carried out by employing parametric objective functions. In the context of the Internet of Things (IoT), it is imperative to establish well-defined intervals for job execution, ensuring that the completion status of each action is promptly monitored and assessed. The major significance of proposed method is to integrate a blockchain technique with neuro-fuzzy algorithm thereby improving the security of data processing units in all smart city applications. As the entire process is carried out with IoT the security of data in both processing and storage units are not secured therefore confidence level of monitoring units are maximized at each state. Due to the integration process the proposed system model is implemented with minimum energy conservation where 93% of tasks are completed with improved security for about 90%.
Citation: Shitharth Selvarajan, Hariprasath Manoharan, Celestine Iwendi, Taher Al-Shehari, Muna Al-Razgan, Taha Alfakih. SCBC: Smart city monitoring with blockchain using Internet of Things for and neuro fuzzy procedures[J]. Mathematical Biosciences and Engineering, 2023, 20(12): 20828-20851. doi: 10.3934/mbe.2023922
[1] | Roman Gumzej, Bojan Rosi . Open interoperability model for Society 5.0's infrastructure and services. Mathematical Biosciences and Engineering, 2023, 20(9): 17096-17115. doi: 10.3934/mbe.2023762 |
[2] | Shiyou Chen, Baohui Li, Lanlan Rui, Jiaxing Wang, Xingyu Chen . A blockchain-based creditable and distributed incentive mechanism for participant mobile crowdsensing in edge computing. Mathematical Biosciences and Engineering, 2022, 19(4): 3285-3312. doi: 10.3934/mbe.2022152 |
[3] | Yanmei Jiang, Mingsheng Liu, Jianhua Li, Jingyi Zhang . Reinforced MCTS for non-intrusive online load identification based on cognitive green computing in smart grid. Mathematical Biosciences and Engineering, 2022, 19(11): 11595-11627. doi: 10.3934/mbe.2022540 |
[4] | Yanxu Zhu, Hong Wen, Jinsong Wu, Runhui Zhao . Online data poisoning attack against edge AI paradigm for IoT-enabled smart city. Mathematical Biosciences and Engineering, 2023, 20(10): 17726-17746. doi: 10.3934/mbe.2023788 |
[5] | Jiahe Xu, Yuan Tian, Tinghuai Ma, Najla Al-Nabhan . Intelligent manufacturing security model based on improved blockchain. Mathematical Biosciences and Engineering, 2020, 17(5): 5633-5650. doi: 10.3934/mbe.2020303 |
[6] | Ridha Ouni, Kashif Saleem . Secure smart home architecture for ambient-assisted living using a multimedia Internet of Things based system in smart cities. Mathematical Biosciences and Engineering, 2024, 21(3): 3473-3497. doi: 10.3934/mbe.2024153 |
[7] | Bin Wang, Fagui Liu . Task arrival based energy efficient optimization in smart-IoT data center. Mathematical Biosciences and Engineering, 2021, 18(3): 2713-2732. doi: 10.3934/mbe.2021138 |
[8] | Yongjun Ren, Yan Leng, Yaping Cheng, Jin Wang . Secure data storage based on blockchain and coding in edge computing. Mathematical Biosciences and Engineering, 2019, 16(4): 1874-1892. doi: 10.3934/mbe.2019091 |
[9] | Gaurav Tripathi, Kuldeep Singh, Dinesh Kumar Vishwakarma . Applied convolutional neural network framework for tagging healthcare systems in crowd protest environment. Mathematical Biosciences and Engineering, 2021, 18(6): 8727-8757. doi: 10.3934/mbe.2021431 |
[10] | Dawei Li, Enzhun Zhang, Ming Lei, Chunxiao Song . Zero trust in edge computing environment: a blockchain based practical scheme. Mathematical Biosciences and Engineering, 2022, 19(4): 4196-4216. doi: 10.3934/mbe.2022194 |
The security of the Internet of Things (IoT) is crucial in various application platforms, such as the smart city monitoring system, which encompasses comprehensive monitoring of various conditions. Therefore, this study conducts an analysis on the utilization of blockchain technology for the purpose of monitoring Internet of Things (IoT) systems. The analysis is carried out by employing parametric objective functions. In the context of the Internet of Things (IoT), it is imperative to establish well-defined intervals for job execution, ensuring that the completion status of each action is promptly monitored and assessed. The major significance of proposed method is to integrate a blockchain technique with neuro-fuzzy algorithm thereby improving the security of data processing units in all smart city applications. As the entire process is carried out with IoT the security of data in both processing and storage units are not secured therefore confidence level of monitoring units are maximized at each state. Due to the integration process the proposed system model is implemented with minimum energy conservation where 93% of tasks are completed with improved security for about 90%.
The Internet of Things (IoT) has facilitated the implementation of intelligent operational nodes and connectivity interfaces for all remote units through the utilization of suitable wireless storage devices. Therefore, it is feasible for all individuals to oversee smart cities, and the results obtained from monitoring systems may be reproduced instantaneously. To facilitate the transformation of cities into smart cities, it is important to monitor several state metrics like hospitality, transportation, energy rates, and other relevant factors. In order to incorporate the aforementioned metrics, it is imperative to build a design model that effectively allocates the requisite activities. Additionally, a wireless examination unit should be integrated to enhance the overall efficiency of the operation. Additionally, it is imperative to ensure that the assigned work is allotted to a specific node, as each unit is processed in order to carry out sensing operations. Furthermore, it is imperative that all assigned jobs are executed with utmost dependability. Consequently, each activity can be structured into distinct operational entities through the use of blockchain methodology.
One of the primary justifications for integrating Internet of Things (IoT) networks with blockchain technology is the ability to facilitate universal access to vital system activities. By sharing information across entities, a distinct safety state may be established and sustained. The aforementioned functionality is of great utility to smart city applications, as it facilitates the collection of operational data from diverse smart cities by leveraging existing data sources. Furthermore, it is imperative that all the integrated comments are implemented through a systematic approach utilizing blockchain technology. This ensures that every piece of data is gathered, exchanged, and processed, hence enhancing the trustworthiness of smart cities in a more efficient manner. An additional significant consideration in smart city applications pertains to the evaluation of the energy conservation levels at different locations throughout varying time periods. This assessment is crucial in order to accomplish all autonomous operations at the lowest possible energy consumption rate. Figure 1 is the block diagram that outlines the process of monitoring a smart city using Internet of Things (IoT) technology. Figure 1 illustrates the interconnected communication interface between various ledgers and mobile devices, enabling the development of smart city apps with adequate resources. From Figure 1 the shared communication interface with all possible ledgers is connected with mobile devices thereby the smart city applications can be built with proper resources. All the interfaces are then connected with the help of gateways thus enabling communication process to take place with open cluster functions. Due to the presence of clusters an individual block function is established and individual tasks are assigned and the response of every task is observed. Since all the above mentioned process happens with the help of IoT, a cloud monitoring and storage unit can be integrated to store all defined tasks. Thereafter the output representations are made for each blocks where smart city monitoring units are separated as energy conservation, health care, transportation and industries.
The smart city monitoring system involves several background tasks that are closely associated with multiple parametric functions. Hence, it is imperative to analyze the functions and methods of integrating distinctive attributes of the Internet of Things (IoT) inside smart urban environments. Despite the existence of numerous methods for monitoring smart cities, one of the primary objectives of implementing the Internet of Things (IoT) and its successful outcomes is emphasized by several academics. Currently, the majority of the smart city monitoring procedures have been developed and continue to serve as a significant benefit in offering a remote monitoring system. In this study, a scalable Internet of Things (IoT) framework is proposed to enhance the coverage of smart cities, ensuring that all connected states maintain cohesive representations [1]. In smart cities, the monitoring of logistics is often conducted in many sectors to ensure accurate perception and minimize misinterpretation. This is mostly achieved through individual bonding and collaboration. However, a significant limitation in these bonding scenarios is the inability to share network access or assigned resources inside the Internet of Things (IoT) medium, leading to operational failures. Furthermore, this study examines the validation process of Internet of Things (IoT) for various applications by employing constructive methods. This approach aims to facilitate suitable modifications to the connected processes of smart cities [2]. In order to develop an effective solution, a smart infrastructure system is designed with interoperability requirements that adequately address diverse features across various Internet of Things (IoT) supported settings. However, in real-time scenarios, if all properties are thoroughly analyzed, it is possible for the Internet of Things (IoT) process to become significantly vulnerable to environmental variables that are beyond modification at any given moment. Furthermore, the anomaly detections found in IoT smart management systems are characterized by a collection of variable functions that are assessed by Gaussian analysis [3].
The inference distribution in the constructed Gaussian model is shown to be in accordance with local conditions, as it incorporates a recurrent unit to effectively minimize energy consumption. However, the establishment of a recurrent unit can address the issue of energy sources by providing a solution for the lack of flexibility in operations and the higher cost of implementation. It is imperative to emphasize the importance of incorporating blockchain technology into the security system of smart city monitoring in order to promote energy conservation [4]. The implementation of smart contracts enables the potential for comprehensive monitoring of users with significant energy consumption. However, effective control over transactions can only be achieved through the utilization of external conditional operations. By incorporating external conditional actions, it is possible to develop a trading platform in the near future that can effectively address excessive utilization conditions. Researchers have explored the possibility of implementing a cloud operating system for the Internet of Things (IoT) instead of relying on a conditional operation. This system would enable the storage of all monitored data in a smart city, allowing access to this data at any given time [5]. In order to substantiate the aforementioned process, a genetic algorithm is incorporated into cloud-based Internet of Things (IoT) systems. This integration enables the monitoring of energy mutants at a reduced rate, while also facilitating the timely detection of task demands. In the aforementioned monitoring systems, it has been found that the delay and sensitivity of established cloud systems are significantly higher. As a result, their suitability for smart city monitoring applications remains only partial. In keeping with previous discussions on the integration of blockchain technology, it is feasible to implement a linear network coding approach to mitigate energy usage [6]. In the linear design model, the objective is to maximize the dependability of monitoring sources and achieve optimized energy states. However, the convenience of IoT and other data storage systems is hindered by the introduction of alternative linear circumstances. It is widely acknowledged that the optimization of energy consumption can only be achieved when the interconnected devices are maintained in an active mode of operation [7]. If the various interconnected devices within a smart city application are constructed using advanced approaches, it becomes feasible to efficiently disseminate and retrieve data across faraway places without encountering external faults.
However, it is imperative that cutting-edge technologies remain in a supported state for all energy monitoring devices. In the event that any equipment remains in an unavoidable "on" status, the utilization of the Internet of Things (IoT) as an extended supporting platform becomes significantly challenging. On the other hand, as a potential alternative to existing smart IoT platforms, it is possible to offer a job prediction model that effectively balances the trade-off between energy supply and energy storage [8]. In order to generate trade-off conditions, one can employ a scheduling algorithm to determine the arrival time period while making priority adjustments. One significant limitation of the arrival duration is the requirement to develop a robust energy-efficient model with a comprehensive depiction of connections, which would enable the avoidance of various external disturbances. Therefore, it is possible to provide a practical application model that can be used across many applications in order to conserve energy in Internet of Things (IoT) networks. This model would involve the utilization of automated sensing operations to enable early prediction of energy usage scenarios [9]. Since sensors are passive components, it is necessary to reduce the absorption of energy through the use of applied power sources. This allows for the normalization of various energy characteristics in smart cities, regardless of any changes in dimensions. One of the most effective alternative choices for advancing daily living is the integration of sensors in smart cities. This approach involves comparing various approaches and their associated specified objective functions in smart city applications, as outlined in Table 1.
References | Main characteristics | Objectives | |||
A | B | C | D | ||
[10] | Cognitive actions based smart city applications | ✓ | ✓ | ||
[11] | Sustainable smart city applications with constrained application protocol | ✓ | ✓ | ||
[12] | Machine learning for smart environment detection | ✓ | ✓ | ||
[13] | Data routing in smart city infrastructure services | ✓ | ✓ | ||
[14] | Secured recommendation systems using IoT | ✓ | ✓ | ||
[15] | Application mapping procedure for building smart infrastructures | ✓ | ✓ | ||
[16] | Decision making for prediction of task success using fuzzy algorithm | ✓ | ✓ | ||
[17] | Renewable energy unit monitoring with fuzzy systems | ✓ | ✓ | ||
[18] | Analysis of various threats in neuro fuzzy implementation | ✓ | |||
Proposed | Blockchain and Neuro fuzzy based IoT for smart city applications | ✓ | ✓ | ✓ | ✓ |
A: Quantified task and task promptness; B: Transfer and confidence rate; C: Energy consumption; D: Privacy and reliability |
The current technologies offer several benefits in the implementation of different features for smart city applications and effective means of monitoring essential parameters through the integration of algorithmic structures and patterns. However, the current approaches have the capability to address some objective patterns that are somewhat related, but they are constrained in their own respective ways. As a result, the following queries have emerged as significant gaps, serving as a motivation for the creation of the proposed method.
RG1: Whether the IoT systems can able to provide complete the task at high speed and transfer rate?
RG2: Can the implemented IoT model supports low energy consumption with maximized confidence rate?
RG3: Is the designed system model for IoT in smart cities highly reliable with establishment of blockchain protocols?
RG4: Whether the IoT cloud platform support confidence rate for storing various data according to the user needs with minimized allocation rates?
The major contributions of the proposed method is to solve all the queries that are present in existing gaps therefore it is possible to monitor all smart cities appropriately by following a set of secured procedures. Hence the major contributions are listed as follows.
● To design a system model that provides complete support to all the allocated tasks to complete it in short period of time.
● To implement blockchain technique thereby enhancing the security of smart city monitoring operations at high confidence rate.
● To integrate neuro fuzzy algorithm with blockchain protocol thus increasing reliability of smart city monitoring at low allocation rates.
The mathematical model utilized in the context of the Internet of Things (IoT) facilitates the analysis of smart cities, enabling the extraction of valuable insights pertaining to the architectural configuration in a remarkably adaptable manner. To ensure optimal decision-making in the context of smart city operations, it is imperative to consider the dynamic nature of the environment and make appropriate adjustments accordingly. Hence, it is imperative to establish a comprehensive system model in order to effectively address the assigned task within the system, while ensuring that no external factors influence its execution.
Given that smart city applications are designed to perform duties depending on specific time periods, it is imperative to build a robust connection with the cloud, as it is responsible for processing all the data involved. Let us denote t1,t2…tn as distinct time intervals assigned to specific jobs, where the problem at hand can be efficiently handled by utilizing Eq (1). Since the smart city applications are used for executing tasks based on time periods it is necessary to have proper establishment with cloud as it process every data. Let us consider as separate time period that is allocated to individual tasks where the optimized problem can be solved using Eq (1) as follows.
taski=max∑ni=1t1+..+ti→0,1 | (1) |
Equation (1) represents the allocation of individual time periods for the completion of a greater number of activities, resulting in the successful execution of each activity and enabling the Internet of Things (IoT) to effectively facilitate the smart city process.
In order to adhere to the designated time periods for each individual activity, it is imperative that the pace at which every Internet of Things (IoT) task is executed surpasses that of typical operational conditions. However, it is vital to verify the quantity of data that is incorporated into the provided assignment for smart city applications. Smart cities encompass a multitude of task linkages, including but not limited to public security and transportation. The speeds for advanced IoT techniques are denoted as s1,s2..sn, which must be greater as described by Eq (2).
RRi=max∑ni=1Ns(i)PS | (2) |
Equation (2) delineates the necessity for congruence between the total quantity of data and the processing speed in order to effectively execute designated duties within smart city frameworks. This congruence is crucial for assessing the timeliness of the system.
Another significant factor that influences the performance of activities in smart cities is the transfer rate, which can be computed using Eq (3). This is in addition to the task completion speed, which is determined based on the data size.
TRi=max∑ni=1(D1×t1)+..+(Di×ti)Dt(t) | (3) |
Equation (3) delineates that in order to enhance the speed of IoT data transmission systems, it is imperative to optimize the transfer rate ratio between individual data and the total accessible data.
Energy consumption is a critical characteristic that necessitates monitoring in all smart city applications based on the Internet of Things (IoT), since it significantly impacts the data transfer process. Therefore, Eq (4) is derived to calculate the energy rate as follows.
ERi=min∑ni=1Ci+Te(i) | (4) |
According to Eq (4), it is necessary to allocate an individual energy rate for each activity. In cases where additional energy is required, it can be supplied as transmitted energy.
The preservation of privacy in connected networks or clusters is imperative due to the utilization of extensive deployment data ways in the smart city data processing. Therefore, it is imperative to appoint a network leader who can facilitate data exchanges, as depicted in Eq (5).
BPi=max∑ni=1D1+..+DiVt(i) | (5) |
Equation (5) represents the concept that increasing the number of blocks assigned to each data element can enhance the security of data transactions. However, it is important to remember that when a new block is created, the configuration of the current block must be adjusted accordingly.
The reliability of data connection and establishments must be evaluated by utilizing the lifetime, as represented by Eq (6), due to the fact that the blockchain technique processes all data as a block.
reli=max∑ni=11−ρ1+..+ρiLTi | (6) |
In the context of smart city monitoring systems, it is imperative to ensure a high allocation rate for each node. This is achieved by the implementation of a scheduling mechanism, which enables the processing of each monitoring task in an individualized manner. Therefore, the allocation rate can be analyzed by utilizing Eq (7) in the following manner.
ARi=min∑ni=1Insiγi | (7) |
The development of sophisticated smart cities using Internet of Things (IoT) technology necessitates the resolution of a multifaceted platform through the application of confidence rates. Hence, it is imperative that the sensing sequence in the Internet of Things (IoT) is accurately and reliably represented, as denoted by the high confidence rate indicated in Eq (8).
CRi=max∑ni=1αin+ORin | (8) |
The proposed objective functions for the smart city regulating process with secured data transfer process are determined based on the established parametric relationships in the field of Internet of Things (IoT), as indicated in Eqs (9) and (10).
obj1=min∑ni=1ERi,ARi | (9) |
obj2=max∑ni=1taski,RRi,TRi,BPi,reli,CRi | (10) |
The objective functions are formulated as multi-objective functions that exhibit a min-max parametric relationship with the functions representing the components of a smart city. Consequently, the objective functions are incorporated with appropriate design principles through the utilization of blockchain protocols and neuro-fuzzy algorithms.
The preservation of privacy and security in the context of the Internet of Things (IoT) assumes a critical role in the implementation of smart city monitoring apps. This is due to the requirement of transmitting all data in the form of blocks. During this procedure, it is seen that a significant portion of the data is transferred without any identifiable form, hence facilitating easier access to the data for registered users. During the process of block generation, the majority of the data contained within the blocks serves as a supportive element for all peers involved, ensuring that the transmission time is appropriately maintained under all situations. Furthermore, the primary rationale for including blockchain technology in the monitoring process of smart cities is the inherent immutability of data. This means that even when data transactions are executed using shared resources, the integrity of the data stays intact and cannot be tampered with. Furthermore, due to the interconnectedness of the smart city process with the broader society, it is imperative to uphold transparency in order to proactively avert instances of system failure. Furthermore, it is not vital to continuously monitor identical situational circumstances over an extended duration. Consequently, the sharing time period is implemented inside the smart city monitoring procedure [19,20,21]. Nevertheless, the aforementioned sharing mechanism is accompanied by a predetermined set of function and design principles within the network. The fundamental principle governing the blockchain process necessitates the decentralized monitoring of all network data through the utilization of encrypted keys. Moreover, the blockchain technology is intricately linked to the proposed system model, as it necessitates the tracing of every energy transaction. This enables the potential for significant energy savings, as information pertaining to diverse energy sources is exchanged throughout a verified network. On the other hand, the demand for transmitting diverse data to end users is growing in light of everyday life situations. Consequently, all electronic transmission systems utilizing the Internet of Things (IoT) need to be restructured to align with stringent resilience requirements. The suggested method presents a mathematical formulation for the representation of blockchain.
To ensure the accuracy of incoming data, it is important to arrange it in a sorted manner, hence facilitating the creation of monitored values for all levels of the Internet of Things (IoT). Let us analyze the sum of hash values, denoted as h1+..+hi, which represents the hash values of smart city data that is connected with the subsequent layer of each node. Hence, the block values might be presented in the following manner
vi=∑ni=1E1(h1)+..+Ei(hi) | (11) |
In order to provide effective encryption and restrict access to monitored data, a distinct key is generated for each element, hence allowing only authenticated users to access and share the data. The aforementioned technique for key construction serves the dual purpose of data protection and prevention of transmission failures in IoT. Therefore, the process of key establishment is formulated utilizing Eq (12) in the following manner.
Ki=∑ni=1βin(Ei,ℵi) | (12) |
Each block of data must be associated with a unique header point that varies according to the time period. Therefore, the block transaction ensures a consistent time interval, resulting in an increased execution rate for each block. The related formulation is presented as follows.
BHi=∑ni=1ϑnmT | (13) |
Equation (13) establishes a correlation between the block header ratio and the number of generated sources, indicating that each authorized data is systematically extracted. Figure 2 depicts the block representations of the blockchain protocol, while the associated flow graph showcases the step implementation process.
Protocol Blockchain |
Begin PROCEDURE BC |
Given |
h1, hi: Hash values for monitored elements |
E1, Ei: Total number of encryptions in each block |
for i = 1:ndo |
1. βin forproviding cryptographic functions after key establishment |
2. ℵi for allocating total number of key elements in monitored data |
end |
else |
for all i = 1:n do |
3. BHi for identifying block headers after removing various data points |
end |
end PROCEDURE |
If bee colony optimization is used in smart city application process then it is possible to complete the task and convergence for task completion can be achieved but the major drawback of bee colony optimization is that if unstructured data is provided then the achieved convergence will be premature thereby trained data does not meet the optimized values. Moreover for all smart city applications the implementation codes must be executed fast therefore every regulatory is followed thereby crossing iteration periods but in case of simulated annealing the execution process is much slower thereby making every task to be completed after crossing certain time interval. In addition to the above mentioned algorithms back propagation algorithm can be implemented to handle more amount of data but if the testing data contains any irregularities then it is much difficult to complete the task hence the promptness of every task is reduced. Hence a neuro fuzzy optimization of preferred for data training and testing in case of smart city application monitoring. In order to effectively implement monitoring applications in smart cities, it is imperative to integrate human interaction systems with neuro modeling. This integration allows for the establishment of suitable relationships with fuzzy functions and components. The neural fuzzy interface system functions by utilizing localized information, hence facilitating the establishment of a sustainable network operation that enables the conversion of entire cities into green Internet of Things (IoT) representations. The ability to forecast traffic congestion in different places and then mitigate network delay is facilitated by the existence of three-layer formation systems. The primary focus of the proposed method lies in the energy management process, wherein a balanced strategy can be produced through the utilization of a neuro-fuzzy algorithm. The utilization of fuzzy integration in digital design allows for enhanced support in neural operations. Furthermore, the utilization of neuro fuzzy algorithms enables the efficient management of energy consumption across diverse renewable sources. This approach also allows for the customization of visual sensing units to cater to certain dimensional units. The integration of artificial neural networks in the algorithm of smart city monitoring systems has the potential to enhance their reliability. In comparison to other neural network methods, fuzzy logic facilitates the rapid determination of responsive actions in the system's monitored states prior to any form of detachment. Furthermore, the implementation of a neural fuzzy system in the decision-making process enhances the security features, resulting in more efficient resource management. On the other hand, the process of combining can efficiently facilitate the processing, storage, and retrieval of data units, so enabling more accurate handling of uncertainties within the system. The formulation of the mathematical model for the neuro-fuzzy algorithm in the suggested method is as follows.
In the suggested method, the observation of incoming signals in the smart city is facilitated through the utilization of a distinct layer. This approach effectively circumvents the need for individual data measurements during the process. Therefore, the data that is combined in neural fuzzy representations is formulated using Eq (14) in the following manner.
fa(i)=∑ni=1l4(i)×pl3(i) | (14) |
Equation (14) stipulates that the aggregation of all capabilities with preceding layers is imperative in order to facilitate the estimation of the membership function through the use of the requisite incoming signal.
The estimation of a defined membership function can be achieved within a neuro-fuzzy system, but only if the appropriate clusters are produced. For each cluster, it is possible to establish a Gaussian relationship by utilizing Eq (15) in the following manner.
MBFi=∑ni=1e(yi−clusteri2) | (15) |
Equation (10) delineates that when the quantity of class functions, such as smart energy management and transportation systems, increases, the establishment of membership functions can be achieved by taking the average between two class individuals.
Since the membership functions are established by using individual functions error measurements are defined in the logical representation process. As the process combines more smart monitoring units errors will occur during the learning procedure as indicated in Eq (16).
errori=min∑ni=1OMi(1−IMi) | (16) |
where,
OMi, IMi denotes output and input membership functions
Algorithm Neuro fuzzy |
Begin PROCEDURE NF |
Given |
l1+..+l4: Total number of layer representations |
pl3: Third layer control blocks |
for i=1:ndo |
1. fa(i) foraggregating the data functions in smart cities |
2. MBFi for computing the defined membership functions |
end |
else |
for all i=1:ndo |
3. errori for identifying total number of errors in established membership functions |
end |
end PROCEDURE |
The block representations of blockchain protocol are illustrated in Figure 2 and corresponding flow graph is provided with step implementation process and implementation codes are also provided.
close all;
QT = single(zeros(SS, 2));
QT1 = single(zeros(SS/41, 2));
n = size(test, 2);
[n, QT1] = size(input);
Settings
σ = 3 × 10−2;
Δ = 2 × 10−3;
ℵ(success) = 1;
ℵ(failure) = 0;
failure = Δ;
TP = 1;
for bestepoch = 1:100
if (ℵ(success) = = 1)
σ = σ /normalized;
TP = σ + σ *ℵ(success);
z = (reshape((*ℵ(success):end, 1), TP))';
[z_temp] = grad_anfis_(ID, city, country, environment, sustainability);
δ = z+ z_temp;
end
function [out] = output_anfis (ID, city, country, environment, sustainability)
if n > 100
target = 0;
end
n = size (Task, 1);
memory = single (zeros(TP, 1));
for i = 1:100
output = input-ones(TP, 1)/(ones(TP, 1)*n((TP-1));
end
Since the proposed method is based on multiple application monitoring which is used as smart city the neuro fuzzy approach can able to monitor all applications that are related to IoT where different patterns can be recognized with appropriate testing and training. Even if collected IoT data is incomplete the assigned task will be completed in every smart city monitoring process as neuro fuzzy can able to store both structured and unstructured data. Moreover the rules of neuro fuzzy that is provided for smart city application monitoring process is flexible at input and output units thereby achieving aggregate data at every layer as from previous layers the inputs are combined. Additionally membership functions of smart city applications are obtained only if the data is divided into various clusters and by using this type of conditions the fuzzy model divides various cities according to sustainability and environmental conditions. Once the data is processed then amount of errors will be reduced in neuro fuzzy procedure as every task is executed with secured data transmission technique after generating hash values.
Variables | Implication |
0,1 | Successful and failure of task events |
Ns | Number of data in each time period |
PS | Speed of processing each data |
D1, Di | Individual data |
Dt | Total data for smart city representations |
Ci | Energy of each smart city task |
Te | Total transmission tasks |
Vt | Maximum threshold values of each data blocks |
ρ1+..+ρi | Hash value of each block |
LTi | Life time of data blocks |
Insi | Number of execution task |
γi | Current node representations |
αin, ORin | Total trust and overall data return rates |
E1(h1), Ei(hi) | Values of each monitored elements in smart city |
βin | Cryptographic functions |
ℵi | Individual key functions |
ϑn | Data exertions |
mT | Removal of data points |
l4 | Fourth layer control |
pl3 | Inputs from third layer control |
yi | Member class |
clusteri | Total number of clusters |
OMi, IMi | Output and input membership functions |
Real-time experimentation analysis is conducted in order to enhance smart city applications by integrating a larger number of sensors under varied parametric situations. Based on predefined stimuli, multiple actions are executed. Real-time verification involves four distinct phases: cluster formation, sequence layout, symbol conversion, and stimulus decision-making. The aforementioned steps are established for each task that must be executed in alignment with specified IoT systems. Furthermore, the utilization of blockchain technology enables the concealment of all unprocessed data through the representation of symbols. As a result, the neuro-fuzzy system integrates a secure Internet of Things (IoT) module. During the initial phase, the hardware connections are established by taking into account eight distinct modules, which encompass not only energy conservation processes but also hospitality and transportation. Collecting data becomes more challenging when all eight possible activities are present, thus necessitating the representation of a greater number of gateway points in the proposed system. Some judgments are made based on the promptness rate, which involves transmitting data in a sequential manner using different tokens. The utilization of this particular sequence enables the acquisition of IoT smart city data from all cluster units, allowing for the characterization of the defined energies at this stage. Furthermore, within the context of logic implementation, each individual key is established as an integral component of pre-defined layers. This process effectively establishes a membership function for each header character. The hardware components are interconnected in a flexible manner, which enhances the reliability of data monitoring systems. In the event that any smart city sensing units are misplaced, the arrival rate of each component will be assessed and errors will be eliminated. A study was conducted to examine the results of four parametric scenarios for smart city Internet of Things (IoT). The significance of each scenario is depicted in Table 3.
Scenarios | Importance |
Processing of allocated tasks | To complete task outputs at high rates each cluster units |
Rate of data processing | To define confidence rate of each task units |
Energy conservation | To reduce total energy during data processing and task performance |
Privacy and security | To secure the data with blockchain technique and preventing it from external factors |
Scenario 1: Processing of allocated tasks
Scenario 2: Rate of data processing
Scenario 3: Energy conservation
Scenario 4: Privacy and security
The data that is used for training in the proposed method for smart city is collected by using IoT where names of different cities that includes application process with unique identity is provided. Since it is much difficult to monitor same conditions such as health care, transportation at same city the proposed system model is implemented in a such a way to gather entire data from different cities. Therefore the country and city names are provided for unique ID. Further from the collected data set evaluations are made with respect to mobility where the analysis that is carried out for corresponding applications ensembles the measurement process. The above mentioned data set indications provides information on separated task allocation and for such separations speed of processing is determines in order to train the data at much faster speed. The foremost importance in data set training is provided to type of environments where every smart city application monitoring changes and if any impact is found then training for various tasks are designed accordingly. Such type of changes with environmental conditions denotes the possibility to achieve maximum transfer rate by allocating minimum energy rates. The aforementioned data with unique ID, number of individuals, name of city, name of country, mobility and environments will be considered as training values and the same will be tested with security factors to ensure maximum accurate operations. All of the aforementioned scenarios have been devised with task considerations in mind. Therefore, if the assigned tasks alter, the parametric conditions can be defined accordingly. The hardware representations are transformed into tool analyses through the utilization of the MATLAB IoT toolbox. Therefore, all the corresponding elements, such as nodes, gateways, essential data points, and the number of peers, are interconnected in a mutually dependent manner. Table 4 presents a comprehensive overview of the essential environmental variables and software requirements that are required.
Bounds | Requirement |
Operating systems | Windows 8 and above |
Platform | MATLAB and Network IoT toolbox |
Version (MATLAB) | 2015 and above |
Version (Network simulator) | 2015 v7 |
Applications | Network connections with gateways at all INSPEC points |
Data sets | Unique function representation with previously defined values |
In MATLAB, the process of converting equivalent descriptions is accomplished solely through the utilization of three-dimensional plots. This approach ensures that output representations may be generated with precision, devoid of any errors. The dimensional areas, however, exhibit variations in accordance with the specific design situations. Consequently, simulated outputs are accompanied with consistent block drawings. In order to determine the simulation, a comprehensive data collection is gathered from a pre-existing established set of values. Subsequently, a neuro-fuzzy choice can be made by constructing monitoring outputs. The comprehensive depiction of each scenario is provided below.
In this particular situation, the assigned tasks will be categorized according to predetermined types, and it will be feasible to assess the level of success and failure for each task within the established frameworks. Furthermore, each task is characterized by specific attributes, which in turn define the speed at which it may be completed throughout different time intervals. In the context of smart city applications utilizing the Internet of Things (IoT), the digital representations employed consist of binary code, namely 0's and 1's. These binary digits serve to indicate the respective rates of success and failure pertaining to the monitoring of each data point. After monitoring the data, certain tasks are established to mitigate the risk of failure or prevent the occurrence of empty transaction blocks. If there are empty transaction blocks present, it is necessary to delete them from the system as they contribute to increased space usage in the work progress and have a direct impact on the time period. Additionally, the velocity at which each activity is performed is influenced by the amount of the data being processed, which is further categorized based on the categories of data processing that must be maintained at consistent operational levels. Figure 4 depicts the comparative results of the provided work and its related speed.
According to the data presented in Figure 1, it can be observed that the suggested technique consistently achieves efficient completion of the monitoring process for all assigned jobs. This can be attributed to the utilization of modern chip devices, which enable rapid execution. Furthermore, all jobs are executed at a favorable rate, hence mitigating the occurrence of system failures. In order to validate the results of the real-time simulation using an Internet of Things (IoT) tool, a set of data points consisting of 10,000, 13,000, 15,000, 18,000, and 20,000 correspondingly is taken into consideration. It is feasible to create total task functions for all data types, specifically 47, 58, 65, 69, and 71. The task promptness for the existing method is significantly lower compared to the suggested technique. The primary factor contributing to the improvement in job promptness is not just attributed to a reduction in data size, but rather to the utilization of a data processing system incorporated into the projected model. Furthermore, the proposed approach consistently maintains a success rate of 93% for all tasks, whereas the previous method only achieves a success rate of 74%.
In every Internet of Things (IoT) processing system, it is imperative to optimize the data rate of each monitoring system in order to facilitate the processing of data to its fullest capacity. On the contrary, in each Internet of Things (IoT) monitoring procedure, when data rates are increased, there is a corresponding increase in the ability to collect data with a higher level of confidence, so indicating the accuracy of the data. In order to provide efficient data processing inside an IoT monitoring system, it is imperative to maintain a minimum transfer rate. This is necessary to facilitate the execution of operations without any delays in the determination process. If the assigned transfer rate is lower, it becomes impossible to transmit the data to the targeted destination, resulting in an increased failure rate with a low confidence factor. In this particular scenario, all data is subjected to analysis by end user management systems, which are distinct from the overall count of data units. If the separation ratio is not properly aligned, data processing to the intended destination is hindered, resulting in a decrease in trust rate and an increase in the return rate of each data to its fullest extent. Figure 5 illustrates the data processing and confidence rates of both the proposed and existing approaches.
According to the findings presented in Figure 5, it can be inferred that in the context of an Internet of Things (IoT) smart city application involving diverse data, the processing rate is optimized. Consequently, this optimization instills a high level of confidence in users regarding the reliability and integrity of the connected gateways and nodes at each data point. Furthermore, the utilization of blockchain technology ensures the preservation of data privacy. This enables management systems to exclusively process monitored data with a high level of trust, hence eliminating any return data values within the interconnected system through gateways. In order to validate the operational perspective of Internet of Things (IoT) data processing systems, the complete quantity of data is taken into account, maintaining identical values as indicated in scenario 1. In this scenario, the percentage of trust, attributed to the presence of blockchain technology, is seen to be 87, 91, 93, 97, and 99, respectively. By including the aforementioned block and data trust values, the confidence level of each user is optimized in both the existing and suggested methods. However, the projected model has a maximizing rate of over 75%, whereas the previous approach only maintains a confidence level of 61%.
In order to optimize performance, it is important to monitor the energy allocation for each assigned task, taking into consideration factors such as data rate and confidence level. There exist two distinct methods for ensuring the successful transmission of data to the intended recipient. In the first scenario, if a sufficient amount of energy is allocated, it is possible to expedite the completion of all data-related tasks. In the second form of energy minimization, the confidence level and transfer rate can be enhanced, resulting in the conservation of energy. The proposed methodology introduces a second form of energy-preserving model that aims to conserve a greater quantity of energy resources, resulting in an optimized data transmission rate. In order to calculate the energy consumption in the suggested method, the entire transmission tasks are combined with distinct data units. This approach aims to transfer more data to end users while minimizing energy usage. Additionally, the information is stored in IoT cloud units at a reduced rate. Figure 6 illustrates the energy representations of both the existing approach and the suggested approach.
Based on the findings presented in Figure 6, it can be inferred that the suggested method exhibits a pragmatic approach towards energy preservation. This can be attributed to the utilization of aggregated data, which allows for the transmission of each data point using a basic sequence. Given that the data is aggregated solely at the fourth level, there exists the potential to further optimize resources at each cluster point where individual member classes are established. The primary rationale for implementing individual membership functions is to enhance the understanding of each allotted energy source. This approach allows for the exclusion of data with similar characteristics, leading to greater energy savings. In order to validate the principle of energy conservation, a real-time analysis was conducted on a set of transmission tasks, namely tasks numbered 24, 29, 38, 45, and 52. The energy percentages for both existing and proposed approaches were found to be 57 and 20% respectively, throughout all the tasks under consideration. Furthermore, if the number of transmission tasks is reduced, the existing method still allocates a maximum of 47% of energy, whereas the proposed method only allocates 31% of energy while maximizing data transfers.
Ensuring the preservation of individual privacy and maintaining the security of all data within the interconnected Internet of Things (IoT) network is crucial for the successful implementation of smart city applications. In the realm of security, it is crucial to prioritize the maintenance of robust hash values through the utilization of authenticated keys. Furthermore, it is vital to effectively oversee each allotted key for every set of data. In the event that any violation is detected, it signifies a potential compromise to the confidentiality of the data included within each respective block. Therefore, in this scenario, there is a heightened emphasis on ensuring the security of all data blocks, with a focus on maximizing the lifespan of each block. As previously stated, the enhancement of hash values incorporates the inclusion of the lifespan of each block, which is subtracted from the original remaining value of 1. To mitigate concerns regarding privacy and security, a data header is implemented to effectively disallow the inclusion of any exertion values within the system. Figure 7 presents the simulation results for the proposed and existing approach under conditions of maximum security.
The analysis of Figure 7 reveals that the suggested system model offers enhanced security for all IoT monitored data in smart city applications, in comparison to the present technique. In order to ascertain the level of security, an examination was conducted on a series of hash values, specifically 2, 4, 6, 8, and 10. These hash values correspond to defined blocks with lifetimes of 5, 6, 9, 10, and 11 periodic times. The analysis revealed that the proposed approach yielded a security percentage exceeding 90% in terms of hash values. However, the current approach is limited in its ability to maximize security in IoT smart cities. This is because it relies on using the same hash values in each block, resulting in only 75% of the data being effectively secured. The remaining data either remains idle or is occupied by other users. Due to the optimization of security measures, it becomes feasible to sustain appropriate gateway connections despite the inclusion of supplementary monitoring units inside the system. The suggested system maintains its security inside each block, even in situations where additional monitoring is required. This is achieved through the utilization of aggregated data functions.
The considered four scenarios provides improved performance in case of projected model due to the involvement of training and testing set where appropriate data and tasks are considered for training. In addition only the considered data is tested with minimized energy allocation thereby more amount of data processing speed is provided in such cases. Since a separate key is established for every smart city application and data units are introduced with proper encryption it is observed that confidence level of transmission is increased but limited to 75% due to the major challenge on removing all data points from previous member class values with fuzzy optimization approach.
Since the proposed method is carried out with neuro fuzzy algorithm the robustness characteristics of smart city that includes variations with reference values are analyzed and discussed. The robustness characteristics of an algorithm defines the maximum possible way to achieve secured solutions thereafter indicating that constant values are denoted without any changes. In robustness analysis every data unit will be analyzed for all allocated task and if there are maximum changes in any iteration point then it will be compared with reference values. Further the robustness of an algorithm determines the functionality design and its operation with conventional units hence the system can be analyzed in a better way with maximum number of iterations. As every smart city applications are linked with environmental units the system must be susceptible to low robust conditions and in case if the system is robust to changing environments then precise solution (indications that are related to completion of tasks) can never be achieved. Figure 8 indicates the robustness characteristics of proposed and existing approach.
From Figure 8 it is obvious that proposed method is not robust to changing environments as every allocated tasks are completed within the allocated time periods. To analyze the robustness characteristics number of iterations is considered from 10 to 100 and it is observed that set of values with 10 step variations provides only minor changes for negligible amount. Hence only best epoch values with 20, 40, 60, 80 and 100 is considered with low energy point representations for completing all allotted tasks with high confidence level. During the above mentioned variations in iteration values the proposed method provides robustness for about 20% in case of all allocated tasks whereas existing approach [9] completes all allocated tasks with robustness of 32%. This can be observed for maximum iteration conditions that indicates best epoch value of 100 however at low iteration values the robustness is increased to 24 and 51% in case of proposed and existing approaches respectively.
The discussion is around the implementation of a monitoring technique that yields real-time outcome observations in response to the conversion of numerous areas that are equipped with comprehensive facilities, as part of the development of smart cities. The primary advancement of the suggested methodology is rooted in a comprehensive system model that formulates all necessary parameters for monitoring smart cities, taking into account a range of technological elements. A significant emphasis is placed on the awareness of task assignment and accomplishment, as a substantial portion of data transmission relies on the delineation of essential tasks. The scope of the IoT monitoring system is extended to encompass energy usage, allowing for the identification and implementation of several strategies aimed at conserving energy. In the presence of a wireless monitoring system utilizing the Internet of Things (IoT), it is imperative to uphold data privacy. To address this concern, a blockchain protocol is incorporated into the system model, ensuring the establishment of a unique key. Furthermore, it is also feasible to include a block header, which allows for the identification of suitable data types and the separation of data and allocated tasks into distinct clusters. By adhering to the aforementioned premise, the projected model demonstrates a high level of reliability in its output, which can be compared to the present approach. In addition, it is necessary to have human interactions with system-defined functions in order to facilitate a conversion operation. This conversion technique employs a neuro-fuzzy approach, which allows for the aggregation of all data while minimizing error functions.
The outcomes of the proposed system model is compared with existing approach in terms of task allocation and processing, energy conservation and security as for every smart city monitoring individual applications are carried out with maximum data allocations. Hence the integrated model with neuro fuzzy provides success rate of greater than 90% whereas existing approach completes only 74% of assigned tasks. Further all the completed tasks are processed with a confidence level of 75% in case of proposed approach whereas the confidence level of completed task in existing approach is limited to 61%. In addition with low energy utilization of less than 30% the proposed method can able to achieve 90% security as compared to existing approach with high energy utilization for 47% where the security is limited below 70%. In future the proposed method on smart city application can be controlled with optimization algorithm that includes more amount of data in training set which is processed by using automatic processing with artificial intelligence procedures.
The authors declare they have not used Artificial Intelligence (AI) tools in the creation of this article.
This research was supported by the Researchers Supporting Project number (RSP2023R206), King Saud University, Riyadh, Saudi Arabia.
The authors declare there is no conflict of interest.
[1] |
A. W. Abbas, S. N. K. Marwat, Scalable emulated framework for IoT devices in smart logistics based cyber-physical systems: Bonded coverage and connectivity analysis, IEEE Access, 8 (2020), 138350–138372. https://doi.org/10.1109/ACCESS.2020.3012458 doi: 10.1109/ACCESS.2020.3012458
![]() |
[2] |
S. Pandiaraj, R. Krishnamoorthy, S. Ushasukhanya, J. V. N. Ramesh, R. A. Alsowail, S. Selvarajan, Optimization of IoT circuit for flexible optical network system with high speed utilization, Opt. Quant. Electron., 55 (2023), 1206. https://doi.org/10.1007/s11082-023-05452-x doi: 10.1007/s11082-023-05452-x
![]() |
[3] |
M. Padmaja, S. Shitharth, K. Prasuna, A. Chaturvedi, P. R. Kshirsagar, A. Vani, Growth of artificial intelligence to challenge security in IoT application, Wireless Personal Commun., 127 (2022), 1829–1845. https://doi.org/10.1007/s11277-021-08725-4 doi: 10.1007/s11277-021-08725-4
![]() |
[4] |
R. Aluvalu, V. N. S. Kumaran, M. Thirumalaisamy, S. Basheer, E. Ali aldhahri, S. Selvarajan, Efficient data transmission on wireless communication through a privacy-enhanced blockchain process, Peer J. Comput. Sci., 9 (2023), e1308. https://doi.org/10.7717/peerj-cs.1308 doi: 10.7717/peerj-cs.1308
![]() |
[5] |
Z. E. Ahmed, M. K. Hasan, RA Saeed, R Hassan, S Islam, R. A. Mokhtar, et al., Optimizing energy consumption for cloud Internet of Things, Front. Phys., 8 (2020), 1–10. https://doi.org/10.3389/fphy.2020.00358 doi: 10.3389/fphy.2020.00358
![]() |
[6] |
S. Selvarajan, G. Srivastava, A. O. Khadidos, A. O. Khadidos, M. Baza, A. Alsheri, et al., An artificial intelligence lightweight blockchain security model for security and privacy in IIoT systems, J. Cloud Comp., 12 (2023), 38. https://doi.org/10.1186/s13677-023-00412-y doi: 10.1186/s13677-023-00412-y
![]() |
[7] |
M. Humayun, M. S. Alsaqer, N. Jhanjhi, Energy optimization for smart cities using IoT, Appl. Artif. Intell., 36 (2022), 2037255. https://doi.org/10.1080/08839514.2022.2037255 doi: 10.1080/08839514.2022.2037255
![]() |
[8] | B. Wang, F. Liu, Task arrival based energy efficient optimization in smart-IoT data center, Math. Biosci. Eng., 18 (2021), 2713–2732. http://aimspress.com/article/doi/10.3934/mbe.2021138 |
[9] |
P. K. R. Maddikunta, G. Srivastava, T. R. Gadekallu, N. Deepa, P. Boopathy, Predictive model for battery life in IoT networks, IET Intell. Transp. Syst., 14 (2020), 1388–1395. https://doi.org/10.1049/iet-its.2020.0009 doi: 10.1049/iet-its.2020.0009
![]() |
[10] |
S. Rani, H. Babbar, S. H. A. Shah, A. Singh, Improvement of energy conservation using blockchain-enabled cognitive wireless networks for smart cities, Sci. Rep., 12 (2022), 13013. https://doi.org/10.1038/s41598-022-16916-7 doi: 10.1038/s41598-022-16916-7
![]() |
[11] |
A. Aldribi, A. Singh, Blockchain empowered smart home: A scalable architecture for sustainable smart cities, Mathematics, 10 (2022), 2378. https://doi.org/10.3390/math10142378 doi: 10.3390/math10142378
![]() |
[12] |
A. Ullah, S. M. Anwar, J. Li, L. Nadeem, T. Mahmood, A. Rehman, et al., Smart cities: the role of Internet of Things and machine learning in realizing a data-centric smart environment. Complex Intell. Syst., 2023 (2023), 1–23. https://doi.org/10.1007/s40747-023-01175-4 doi: 10.1007/s40747-023-01175-4
![]() |
[13] |
S. M. Bommu, M. Aravind, K. Babburu, L. N. Thalluri, V. Ganesh, et al., Smart city IoT system network level routing analysis and blockchain security based implementation, J. Electr. Eng. Technol., 18 (2023), 1351–1368. https://doi.org/10.1007/s42835-022-01239-4 doi: 10.1007/s42835-022-01239-4
![]() |
[14] |
V. Mohammadi, A. M. Rahmani, A. M. Darwesh, A. Sahafi, Trust-based recommendation systems in Internet of Things: a systematic literature review, Human Centric Comput. Inf. Sci., 9 (2019), 21. https://doi.org/10.1186/s13673-019-0183-8 doi: 10.1186/s13673-019-0183-8
![]() |
[15] |
S. Shitharth, A. M. Alshareef, A. O. Khadidos, K. H. Alyoubi, A. O. Khadidos, M. Uddin, A conjugate self-organizing migration (CSOM) and reconciliate multi-agent Markov learning (RMML) based cyborg intelligence mechanism for smart city security, Sci. Rep., 13 (2023), 15681. https://doi.org/10.1038/s41598-023-42257-0 doi: 10.1038/s41598-023-42257-0
![]() |
[16] |
D. Pamučar, D. Bozanic, A. Puška, D. Marinković, Application of neuro-fuzzy system for predicting the success of a company in public procurement, Decis. Making Appl. Manage. Eng., 5 (2022), 135–153. https://doi.org/10.31181/dmame0304042022p doi: 10.31181/dmame0304042022p
![]() |
[17] |
J. Simon, R. Sánta, Energy efficient smart home heating system using renewable energy source with fuzzy control design, Decis. Making Appl. Manage. Eng., 6 (2023), 948–948. https://doi.org/10.31181/dmame622023825 doi: 10.31181/dmame622023825
![]() |
[18] |
D. Bozanic, D. Tešić, A. Puška, A. Štilić, Y. R. Muhsen, Ranking challenges, risks and threats using fuzzy inference system, Decis. Making Appl. Manage. Eng., 6 (2023), 933–947. https://doi.org/10.31181/dmame622023926 doi: 10.31181/dmame622023926
![]() |
[19] | A. K. Al-Ani, S. Ul Arfeen Laghari, H. Manoharan, S. Selvarajan, M. Uddin, Improved transportation model with Internet of Things using artificial intelligence algorithm, Comput. Mater. Contin., 76 (2023). |
[20] |
S. Selvarajan, H. Manoharan, C. Iwendi, R. A. Alsowail, S. Pandiaraj, A comparative recognition research on excretory organism in medical applications using artificial neural networks, Front. Bioeng. Biotechnol., 11 (2023), 1211143. https://doi.org/10.3389/fbioe.2023.1211143 doi: 10.3389/fbioe.2023.1211143
![]() |
[21] |
J. Chen, W. Gan, M. Hu, C. M. Chen, On the construction of a post-quantum blockchain for smart city, J. Inf. Secur. Appl., 58 (2021), 102780. https://doi.org/10.1016/j.jisa.2021.102780 doi: 10.1016/j.jisa.2021.102780
![]() |
1. | Niusha Mesgaribarzi, Youcef Djenouri, Ahmed Nabil Belbachir, Tomasz Michalak, Gautam Srivastava, Intelligent explainable optical sensing on Internet of nanorobots for disease detection, 2024, 13, 2191-9097, 10.1515/ntrev-2024-0019 | |
2. | Shitharth Selvarajan, Hariprasath Manoharan, Sonali Goel, Chiranjeevi Prakash Akili, S. Murugesan, Vaishali Joshi, SCMC: Smart city measurement and control process for data security with data mining algorithms, 2024, 31, 26659174, 100980, 10.1016/j.measen.2023.100980 | |
3. | Shitharth Selvarajan, Hariprasath Manoharan, Alaa O. Khadidos, Achyut Shankar, Adil O. Khadidos, Wattana Viriyasitavat, Li Da Xu, SSCM: a secured approach to supply chain management with control management using blowfish optimization, 2024, 18, 1751-7575, 10.1080/17517575.2024.2351871 | |
4. | Salman Ali Syed, Selvakumar Manickam, Mueen Uddin, Hamed Alsufyani, Mohammad Shorfuzzaman, Shitharth Selvarajan, Gouse Baig Mohammed, Dickson polynomial-based secure group authentication scheme for Internet of Things, 2024, 14, 2045-2322, 10.1038/s41598-024-55044-2 | |
5. | Giuseppe Tricomi, Maurizio Giacobbe, Ilenia Ficili, Nicola Peditto, Antonio Puliafito, Smart City as Cooperating Smart Areas: On the Way of Symbiotic Cyber–Physical Systems Environment, 2024, 24, 1424-8220, 3108, 10.3390/s24103108 | |
6. | Osama Bassam J. Rabie, Shitharth Selvarajan, Tawfiq Hasanin, Abdulrhman M. Alshareef, C. K. Yogesh, Mueen Uddin, A novel IoT intrusion detection framework using Decisive Red Fox optimization and descriptive back propagated radial basis function models, 2024, 14, 2045-2322, 10.1038/s41598-024-51154-z | |
7. | Shitharth Selvarajan, Achyut Shankar, Mueen Uddin, Abdullah Saleh Alqahtani, Taher Al‐Shehari, Wattana Viriyasitavat, A smart decentralized identifiable distributed ledger technology‐based blockchain (DIDLT‐BC) model for cloud‐IoT security, 2024, 0266-4720, 10.1111/exsy.13544 | |
8. | Shitharth Selvarajan, Hariprasath Manoharan, Achyut Shankar, Alaa O. Khadidos, Adil O. Khadidos, Antonino galletta, PUDT: Plummeting uncertainties in digital twins for aerospace applications using deep learning algorithms, 2024, 153, 0167739X, 575, 10.1016/j.future.2023.11.034 | |
9. | Darwin Nesakumar A, Deepak P, Sanjay P, Aishwarya B, Aswini R, Pavithra K N, 2024, IoT-Powered Precision Farming and Home Gardening for Seamless Monitoring, Automation and Security, 979-8-3503-5084-5, 1, 10.1109/WiSPNET61464.2024.10532927 | |
10. | Punitha S., Preetha K. S., Shonak Bansal, Enhanced Light‐Gradient Boosting Machine (GBM)‐Based Artificial Intelligence‐Blockchain‐Based Telesurgery in Sixth Generation Communication Using Optimization Concept, 2024, 2024, 2090-0147, 10.1155/2024/3214572 | |
11. | Vimala Josphine C, Theodore Kingslin M, Fatima Vincy R, Mohana M, S. Babitha, Efficient quantum inspired blockchain-based cyber security framework in IoT using deep learning and huristic algorithms, 2024, 18, 18724981, 1203, 10.3233/IDT-230579 | |
12. | Shitharth Selvarajan, Hariprasath Manoharan, Adil O. Khadidos, Alaa O. Khadidos, Abdulrhman M. Alshareef, Aisha Y. Alsobhi, Secured 6G Communication for Consumer Electronics With Advanced Artificial Intelligence Algorithms, 2024, 70, 0098-3063, 5711, 10.1109/TCE.2024.3382779 | |
13. | Arunkumar Muniswamy, R. Rathi, Trust-Based Consensus and ABAC for Blockchain Using Deep Learning to Secure Internet of Things, 2025, 39, 0883-9514, 10.1080/08839514.2025.2459461 | |
14. | Wahida Mansouri, Manal Abdullah Alohali, Hamed Alqahtani, Nuha Alruwais, Menwa Alshammeri, Ahmed Mahmud, Deep convolutional neural network-based enhanced crowd density monitoring for intelligent urban planning on smart cities, 2025, 15, 2045-2322, 10.1038/s41598-025-90430-4 |
References | Main characteristics | Objectives | |||
A | B | C | D | ||
[10] | Cognitive actions based smart city applications | ✓ | ✓ | ||
[11] | Sustainable smart city applications with constrained application protocol | ✓ | ✓ | ||
[12] | Machine learning for smart environment detection | ✓ | ✓ | ||
[13] | Data routing in smart city infrastructure services | ✓ | ✓ | ||
[14] | Secured recommendation systems using IoT | ✓ | ✓ | ||
[15] | Application mapping procedure for building smart infrastructures | ✓ | ✓ | ||
[16] | Decision making for prediction of task success using fuzzy algorithm | ✓ | ✓ | ||
[17] | Renewable energy unit monitoring with fuzzy systems | ✓ | ✓ | ||
[18] | Analysis of various threats in neuro fuzzy implementation | ✓ | |||
Proposed | Blockchain and Neuro fuzzy based IoT for smart city applications | ✓ | ✓ | ✓ | ✓ |
A: Quantified task and task promptness; B: Transfer and confidence rate; C: Energy consumption; D: Privacy and reliability |
Protocol Blockchain |
Begin PROCEDURE BC |
Given |
h1, hi: Hash values for monitored elements |
E1, Ei: Total number of encryptions in each block |
for i = 1:ndo |
1. βin forproviding cryptographic functions after key establishment |
2. ℵi for allocating total number of key elements in monitored data |
end |
else |
for all i = 1:n do |
3. BHi for identifying block headers after removing various data points |
end |
end PROCEDURE |
Algorithm Neuro fuzzy |
Begin PROCEDURE NF |
Given |
l1+..+l4: Total number of layer representations |
pl3: Third layer control blocks |
for i=1:ndo |
1. fa(i) foraggregating the data functions in smart cities |
2. MBFi for computing the defined membership functions |
end |
else |
for all i=1:ndo |
3. errori for identifying total number of errors in established membership functions |
end |
end PROCEDURE |
Variables | Implication |
0,1 | Successful and failure of task events |
Ns | Number of data in each time period |
PS | Speed of processing each data |
D1, Di | Individual data |
Dt | Total data for smart city representations |
Ci | Energy of each smart city task |
Te | Total transmission tasks |
Vt | Maximum threshold values of each data blocks |
ρ1+..+ρi | Hash value of each block |
LTi | Life time of data blocks |
Insi | Number of execution task |
γi | Current node representations |
αin, ORin | Total trust and overall data return rates |
E1(h1), Ei(hi) | Values of each monitored elements in smart city |
βin | Cryptographic functions |
ℵi | Individual key functions |
ϑn | Data exertions |
mT | Removal of data points |
l4 | Fourth layer control |
pl3 | Inputs from third layer control |
yi | Member class |
clusteri | Total number of clusters |
OMi, IMi | Output and input membership functions |
Scenarios | Importance |
Processing of allocated tasks | To complete task outputs at high rates each cluster units |
Rate of data processing | To define confidence rate of each task units |
Energy conservation | To reduce total energy during data processing and task performance |
Privacy and security | To secure the data with blockchain technique and preventing it from external factors |
Bounds | Requirement |
Operating systems | Windows 8 and above |
Platform | MATLAB and Network IoT toolbox |
Version (MATLAB) | 2015 and above |
Version (Network simulator) | 2015 v7 |
Applications | Network connections with gateways at all INSPEC points |
Data sets | Unique function representation with previously defined values |
References | Main characteristics | Objectives | |||
A | B | C | D | ||
[10] | Cognitive actions based smart city applications | ✓ | ✓ | ||
[11] | Sustainable smart city applications with constrained application protocol | ✓ | ✓ | ||
[12] | Machine learning for smart environment detection | ✓ | ✓ | ||
[13] | Data routing in smart city infrastructure services | ✓ | ✓ | ||
[14] | Secured recommendation systems using IoT | ✓ | ✓ | ||
[15] | Application mapping procedure for building smart infrastructures | ✓ | ✓ | ||
[16] | Decision making for prediction of task success using fuzzy algorithm | ✓ | ✓ | ||
[17] | Renewable energy unit monitoring with fuzzy systems | ✓ | ✓ | ||
[18] | Analysis of various threats in neuro fuzzy implementation | ✓ | |||
Proposed | Blockchain and Neuro fuzzy based IoT for smart city applications | ✓ | ✓ | ✓ | ✓ |
A: Quantified task and task promptness; B: Transfer and confidence rate; C: Energy consumption; D: Privacy and reliability |
Protocol Blockchain |
Begin PROCEDURE BC |
Given |
h1, hi: Hash values for monitored elements |
E1, Ei: Total number of encryptions in each block |
for i = 1:ndo |
1. βin forproviding cryptographic functions after key establishment |
2. ℵi for allocating total number of key elements in monitored data |
end |
else |
for all i = 1:n do |
3. BHi for identifying block headers after removing various data points |
end |
end PROCEDURE |
Algorithm Neuro fuzzy |
Begin PROCEDURE NF |
Given |
l1+..+l4: Total number of layer representations |
pl3: Third layer control blocks |
for i=1:ndo |
1. fa(i) foraggregating the data functions in smart cities |
2. MBFi for computing the defined membership functions |
end |
else |
for all i=1:ndo |
3. errori for identifying total number of errors in established membership functions |
end |
end PROCEDURE |
Variables | Implication |
0,1 | Successful and failure of task events |
Ns | Number of data in each time period |
PS | Speed of processing each data |
D1, Di | Individual data |
Dt | Total data for smart city representations |
Ci | Energy of each smart city task |
Te | Total transmission tasks |
Vt | Maximum threshold values of each data blocks |
ρ1+..+ρi | Hash value of each block |
LTi | Life time of data blocks |
Insi | Number of execution task |
γi | Current node representations |
αin, ORin | Total trust and overall data return rates |
E1(h1), Ei(hi) | Values of each monitored elements in smart city |
βin | Cryptographic functions |
ℵi | Individual key functions |
ϑn | Data exertions |
mT | Removal of data points |
l4 | Fourth layer control |
pl3 | Inputs from third layer control |
yi | Member class |
clusteri | Total number of clusters |
OMi, IMi | Output and input membership functions |
Scenarios | Importance |
Processing of allocated tasks | To complete task outputs at high rates each cluster units |
Rate of data processing | To define confidence rate of each task units |
Energy conservation | To reduce total energy during data processing and task performance |
Privacy and security | To secure the data with blockchain technique and preventing it from external factors |
Bounds | Requirement |
Operating systems | Windows 8 and above |
Platform | MATLAB and Network IoT toolbox |
Version (MATLAB) | 2015 and above |
Version (Network simulator) | 2015 v7 |
Applications | Network connections with gateways at all INSPEC points |
Data sets | Unique function representation with previously defined values |