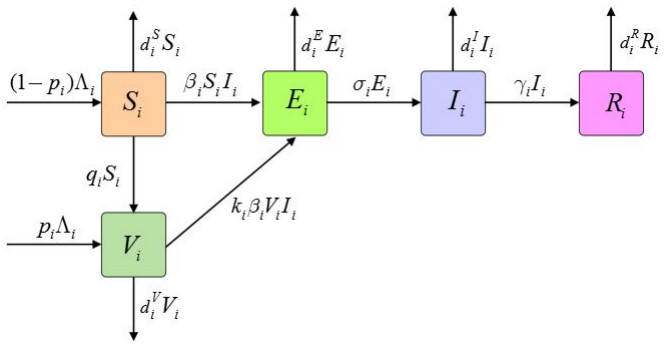
In this paper, we propose a multi-patch SVEIR epidemic model that incorporates vaccination of both newborns and susceptible populations. We determine the basic reproduction number R0 and prove that the disease-free equilibrium P0 is locally and globally asymptotically stable if R0<1, and it is unstable if R0>1. Moreover, we show that the disease is uniformly persistent in the population when R0>1. Numerical simulations indicate that vaccination strategies can effectively control disease spread in all patches while population migration can either intensify or prevent disease transmission within a patch.
Citation: Maoxing Liu, Yuhang Li. Dynamics analysis of an SVEIR epidemic model in a patchy environment[J]. Mathematical Biosciences and Engineering, 2023, 20(9): 16962-16977. doi: 10.3934/mbe.2023756
[1] | Qianqian Cui, Zhipeng Qiu, Ling Ding . An SIR epidemic model with vaccination in a patchy environment. Mathematical Biosciences and Engineering, 2017, 14(5&6): 1141-1157. doi: 10.3934/mbe.2017059 |
[2] | Chentong Li, Jinyan Wang, Jinhu Xu, Yao Rong . The Global dynamics of a SIR model considering competitions among multiple strains in patchy environments. Mathematical Biosciences and Engineering, 2022, 19(5): 4690-4702. doi: 10.3934/mbe.2022218 |
[3] | Jinliang Wang, Gang Huang, Yasuhiro Takeuchi, Shengqiang Liu . Sveir epidemiological model with varying infectivity and distributed delays. Mathematical Biosciences and Engineering, 2011, 8(3): 875-888. doi: 10.3934/mbe.2011.8.875 |
[4] | Ruixia Zhang, Shuping Li . Analysis of a two-patch SIS model with saturating contact rate and one- directing population dispersal. Mathematical Biosciences and Engineering, 2022, 19(11): 11217-11231. doi: 10.3934/mbe.2022523 |
[5] | Xi-Chao Duan, Xue-Zhi Li, Maia Martcheva . Dynamics of an age-structured heroin transmission model with vaccination and treatment. Mathematical Biosciences and Engineering, 2019, 16(1): 397-420. doi: 10.3934/mbe.2019019 |
[6] | Miled El Hajji, Amer Hassan Albargi . A mathematical investigation of an "SVEIR" epidemic model for the measles transmission. Mathematical Biosciences and Engineering, 2022, 19(3): 2853-2875. doi: 10.3934/mbe.2022131 |
[7] | Pengfei Liu, Yantao Luo, Zhidong Teng . Role of media coverage in a SVEIR-I epidemic model with nonlinear incidence and spatial heterogeneous environment. Mathematical Biosciences and Engineering, 2023, 20(9): 15641-15671. doi: 10.3934/mbe.2023698 |
[8] | Jinhu Xu, Yicang Zhou . Global stability of a multi-group model with vaccination age, distributed delay and random perturbation. Mathematical Biosciences and Engineering, 2015, 12(5): 1083-1106. doi: 10.3934/mbe.2015.12.1083 |
[9] | Chenwei Song, Rui Xu, Ning Bai, Xiaohong Tian, Jiazhe Lin . Global dynamics and optimal control of a cholera transmission model with vaccination strategy and multiple pathways. Mathematical Biosciences and Engineering, 2020, 17(4): 4210-4224. doi: 10.3934/mbe.2020233 |
[10] | Lei Shi, Hongyong Zhao, Daiyong Wu . Modelling and analysis of HFMD with the effects of vaccination, contaminated environments and quarantine in mainland China. Mathematical Biosciences and Engineering, 2019, 16(1): 474-500. doi: 10.3934/mbe.2019022 |
In this paper, we propose a multi-patch SVEIR epidemic model that incorporates vaccination of both newborns and susceptible populations. We determine the basic reproduction number R0 and prove that the disease-free equilibrium P0 is locally and globally asymptotically stable if R0<1, and it is unstable if R0>1. Moreover, we show that the disease is uniformly persistent in the population when R0>1. Numerical simulations indicate that vaccination strategies can effectively control disease spread in all patches while population migration can either intensify or prevent disease transmission within a patch.
Infectious diseases are a significant global public health threat that greatly impact people's lives and economies. Since the beginning of the 20-th century, convenient transportation has made traveling more frequent and common, leading to an increased risk of infectious disease transmission among regions. As a result, it is essential to investigate how population mobility affects disease transmission and whether it can increase disease persistence or not. In most classical deterministic epidemic models, the spatial structure is assumed to be homogeneous, but this does not take into account the spatial heterogeneity of population and disease spread [1,2]. To address this limitation, patch epidemic models have been recently proposed to investigate disease transmission in spatially heterogeneous populations [3,4,5,6,7,8,9,10,11].
Hethcote in [12] formulated an epidemic model with population migration among two patches. The patches can be towns, cities or relatively isolated regions. Ruan et al. [13] considered a multiregional model to analyze the effect of global travel on the geographical SARS. Wang and Zhao [14] developed an epidemic model of muti-patches and determined the diseases' threshold for persistence and extinction. Under the assumption that the migration rates of populations are the same, they proved that population migration does not alter the global attractivity of the disease-free equilibrium. Salmani et al. [15] introduced an SEIRS model with population migration among p patches. The authors determined the range of the basic reproduction number and proved that the disease-free equilibrium is globally asymptotically stable if R0<1. However, the global stability of the endemic equilibrium is not considered because of the high dimension of the model. Michael and Shuai [16] studied an SIR epidemic model in a patchy environment. Using a graph-theoretic approach to the method of Lyapunov functions, they discussed the uniqueness and global stability of the endemic equilibrium. Gao [17] presented a multi-patch epidemic model in order to consider travel frequency variations among residents, and he illustrated the influence of heterogeneity in human movement on the geographic spread of diseases. Wang et al. [18] proposed an n-patch SEIQR epidemic model to investigate the effect of entry-exit screening on the spread and control of diseases.
As is known to us, vaccination strategies prove to be the most effective control measure against many infectious diseases. Over the past few decades, many researchers have formulated various epidemic models with vaccination and analyzed their dynamical behavior, see [19,20,21,22,23,24]. However, most of these studies have only examined the impact of vaccination on control of disease spread in an isolated patch, ignoring spatial variation. Taking this into account, Cui et al. [25] established the following SIR epidemic model with vaccination in a patchy environment
{dSidt=(1−pi)μiNi−βiIiNiSi−μiSi+n∑j≠i(mijSj−mjiSi),dIidt=βiIiNiSi−(μi+γi)Ii,dRidt=piμiNi+γiIi−μiRi+n∑j≠i(mijRj−mjiRi),i=1,2,…,n. | (1.1) |
where Ni is the total population in the i-th patch; Si,Ii, and Ri denote the sizes of the susceptible, infected, and recovered individuals in patch i, respectively. They investigated the effect of the increasing mobility of populations on the vaccination strategy. Manuel et al. [26] considered an n-patch SIR epidemic model with constant and proportional vaccination controls. The authors illustrated the effect of vaccination control on disease eradication in a patchy environment.
In reality, many infectious diseases have an incubation period, such as influenza, tuberculosis, malaria and hepatitis B [27]. During the incubation period, the individuals may travel from one patch to another, which has a significant impact on disease spread. There are several that include both latency and mobility of individuals, see [28,29,30,31]. Zhang et al. [28] and Lou and Zhao [29] proposed a reaction-diffusion model with incubation periods. San and Wang [30] presented a two-group SIR epidemic model with incubation periods over a patchy environment. Li and Zou [31] synthesized latency, demographic structure and spatial heterogeneity into the SIR model and investigated the dynamics of the derived model. Motivated by the above works, we extend the model in paper [25] by including the vaccinated class and the exposed class, and formulate a multi-patch SVEIR model that incorporates vaccination for newborns and susceptible individuals. The model formulated in this paper can contribute to studying the impacts of migration and vaccination on disease transmission.
The paper is organized as follows. In Section 2, we introduce an n-patch epidemic model with vaccination. In Section 3, we derive the disease-free equilibrium and the basic reproduction number. In Section 4, we discuss the local and global stability of the disease-free equilibrium and establish uniform persistence of the disease. In Section 5, we perform some numerical simulations to confirm the theoretical results and investigate the impacts of migration and vaccination. Finally, the paper ends with a summary of our conclusions.
In this section, an n-patch SVEIR epidemic model that incorporates vaccination for newborns and susceptible individuals is formulated. Assume that the total population in patch i is divided into five compartments: susceptible, vaccinated, exposed (infected but non-infectious), infectious, and recovered. The number of individuals in each compartment at time t is denoted by Si(t),Vi(t),Ei(t),Ii(t) and Ri(t), respectively. The flow diagram of the disease transmission in each patch is depicted in Figure 1.
All individuals are assumed to be born susceptible, and a fraction pi of newborns in patch i are vaccinated, where pi∈(0,1]. Susceptible individuals in patch i receive vaccination at rate qi. The parameter Λi denotes the influx of newborns into patch i, and βi denotes the transmission coefficient in patch i. Since the vaccination fails to confer complete immunity to all vaccinated recipients, vaccinated individuals may become infected due to contacting with infected individuals. Thus, we use a scaling factor ki to reflect the vaccine efficacy, where ki∈[0,1]. For ki=0, the vaccine can provide complete protection against infection, that is, susceptible individuals will not be infected after vaccination. For ki=1, the vaccine will have no effect. The parameter σi is the rate that exposed individuals become infectious in patch i and γi is the recovery rate of the infectious individuals in patch i. The parameters dSi,dVi,dEi,dIi and dRi are mortality rates of the susceptible, vaccinated, exposed, infected and recovered individuals, respectively, in the i-th patch. The migration rates of susceptible, vaccinated, exposed, infected and recovered populations from patch j to patch i are given by aij,bij,cij,dij and eij, respectively. All the parameters are assumed to be non-negative.
Based on the above assumptions and Figure 1, an SVEIR epidemic model is presented as follows,
{dSidt=(1−pi)Λi−βiSiIi−(dSi+qi)Si+n∑j=1(aijSj−ajiSi),dVidt=piΛi−kiβiViIi−dViVi+qiSi+n∑j=1(bijVj−bjiVi),dEidt=βiSiIi+kiβiViIi−(dEi+σi)Ei+n∑j=1(cijEj−cjiEi),dIidt=σiEi−(dIi+γi)Ii+n∑j=1(dijIj−djiIi),dRidt=γiIi−dRiRi+n∑j=1(eijRj−ejiRi),i=1,2,…,n. | (2.1) |
Notice that the equations for Ri are independent of the first four equations of system (2.1) and hence, the dynamics is governed by the following system,
{dSidt=(1−pi)Λi−βiSiIi−(dSi+qi)Si+n∑j=1(aijSj−ajiSi),dVidt=piΛi−kiβiViIi−dViVi+qiSi+n∑j=1(bijVj−bjiVi),dEidt=βiSiIi+kiβiViIi−(dEi+σi)Ei+n∑j=1(cijEj−cjiEi),dIidt=σiEi−(dIi+γi)Ii+n∑j=1(dijIj−djiIi),i=1,2,…,n. | (2.2) |
In this section, we show that the system (2.2) has a unique disease-free equilibrium, and then, using the next generation matrix method, we derive the basic reproduction number R0.
In order to find the disease-free equilibrium with all Ii=0 of system (2.2), we consider the following linear system:
{(1−pi)Λi−(dSi+qi)Si+n∑j=1(aijSj−ajiSi)=0,piΛi−dViVi+qiSi+n∑j=1(bijVj−bjiVi)=0,−(dEi+σi)Ei+n∑j=1(cijEj−cjiEi)=0,σiEi=0,i=1,2,…,n. | (3.1) |
Then Ei=0(i=1,2,…,n) and
{(1−pi)Λi−(dSi+qi)Si+n∑j=1(aijSj−ajiSi)=0,piΛi−dViVi+qiSi+n∑j=1(bijVj−bjiVi)=0,i=1,2,⋯,n. | (3.2) |
Converted into the form of matrix system, we can get
{H1S=B,H2V=C, |
where
S=(S1,S2,…,Sn)T,B=((1−p1)Λ1,(1−p2)Λ2,…,(1−pn)Λn)T,V=(V1,V2,…,Vn)T,C=(p1Λ1+q1S1,p2Λ2+q2S2,…,pnΛn+qnSn)T, |
H1=[dS1+q1+n∑j≠1aj1−a12⋯−a1n−a21dS2+q2+n∑j≠2aj2⋯−a2n⋮⋮⋮−an1−an2⋯dSn+qn+n∑j≠najn],H2=[dV1+n∑j≠1bj1−b12⋯−b1n−b21dV2+n∑j≠2bj2⋯−b2n⋮⋮⋮−bn1−bn2⋯dVn+n∑j≠nbjn]. |
It is clear that all off-diagonal entries of H1 are negative, and the sum of the entries in each column is positive. Hence, it follows from [32] that H1 is a nonsingular M-matrix and H−11>0. Similarly, H2 is also a nonsingular matrix and H−12>0. Therefore, linear system (3.2) has a unique positive solution S0=(S01,S02,…,S0n)T=H−11B, V0=(V01,V02,…,V0n)T=H−12C, where S0i,V0i satisfies
0=dS0idt=(1−pi)Λi−(dSi+qi)S0i+n∑j=1(aijS0j−ajiS0i),0=dV0idt=piΛi−dViV0i+qiS0i+n∑j=1(bijV0j−bjiV0i). | (3.3) |
Thus, system (2.2) always has a unique disease-free equilibrium P0=(S01,V01,0,0,…,S0n,V0n,0,0).
Adding all the equations of (2.2), we have
dN(t)dt=_Λ−n∑j=1[dSiSi+dViVi+dEiEi+(dIi+γi)Ii]+n∑i=1[n∑j=1(aijSj−ajiSi)+n∑j=1(bijVj−bjiVi)+n∑j=1(cijEj−cjiEi)+n∑j=1(dijIj−djiIi)]=_Λ−n∑j=1[dSiSi+dViVi+dEiEi+(dIi+γi)Ii]≤_Λ−d∗N, | (3.4) |
where _Λ=n∑i=1Λi,d∗=min{dSi,dVi,dEi,dIi+γi} and N(t)=n∑i=1(Si+Vi+Ei+Ii). By the comparison principle, it is easy to see that limsupt→∞N(t)≤_Λd∗.
From the first equation of system (2.2), we obtain
dSidt≤(1−pi)Λi−(dSi+qi)Si+n∑j=1(aijSj−ajiSi)=(H1S0−H1S)i, | (3.5) |
where (H1S0−H1S)i is the i-th element of H1S0−H1S. Thus, dSidt≤0 if Si=S0i,Sj≤S0j,i,j=1,2,…,n, and j≠i. Similarly, dVidt≤0 if Vi=V0i,Vj≤V0j. From the aforementioned analysis, the compact feasible region
Γ={(S1,V1,E1,I1,…,Sn,Vn,En,In)∈R4n+:N(t)≤_Λd∗,0≤Si≤S0i,0≤Vi≤V0i,i=1,2,…,n} |
is positively invariant with respect to system (2.2).
The basic reproduction number R0 is known as the threshold of disease outbreak, which has important implications for disease control. In the following, the next-generation matrix method in van den Driessche and Watmough [33] is used to calculate the reproduction number of system (2.2).
Define
F=[0F100],V=[V110V21V22],whereF1=diag(β1S01+k1β1V01,β2S02+k2β2V02,…,βnS0n+knβnV0n), |
V11=[dE1+σ1+n∑j≠1cj1−c12⋯−c1n−c21dE2+σ2+n∑j≠2cj2⋯−c2n⋮⋮⋮−cn1−cn2⋯dEn+σn+n∑j≠ncjn].V21=diag(−σ1,−σ2,…,−σn),V22=[dI1+γ1+n∑j≠1dj1−d12⋯−d1n−d21dI2+γ2+n∑j≠2dj2⋯−d2n⋮⋮⋮−dn1−dn2⋯dIn+γn+n∑j≠ndjn]. |
It is clear that both V11 and V22 are nonsingular M-matrixes [32], which means that their inverses are nonnegative. Therefore, the matrix V is a nonsingular matrix. The next generation matrix is given by
FV−1=[−F1V−122V21V−111F1V−12200], |
Thus, the basic reproduction number R0 is defined as the spectral radius of the matrix FV−1, that is,
R0=ρ(FV−1)=ρ(−F1V−122V21V−111). |
In this section, the local and global stability of the disease-free equilibrium P0 are discussed, and the uniform persistence of system (2.2) is established. In order to discuss the stability of the disease-free equilibrium, we introduce the following lemma.
Lemma 4.1. If F is a non-negative matrix and V is a nonsingular matrix, then
s(F−V)<0⟺ρ(FV−1)<1,s(F−V)>0⟺ρ(FV−1)>1, | (4.1) |
where s(A):=max{Re(λ):λis an eigenvalue of matrixA}.
Theorem 4.1. The disease-free equilibrium P0 is locally asymptotically stable if R0<1 and it is unstable if R0>1.
Proof. From the third and the fourth equations of system (2.2), we obtain
.X=(F−V)X, | (4.2) |
where X=(E1,E2,…,En,I1,I2,…,In)T.
It follows from Lemma 4.1 that s(F−V)<0(>0) if and only if R0<1(>1). Thus, if R0<1, all the eigenvalues of the matrix F−V lie in the left half plane, and therefore, the disease-free equilibrium P0 is locally asymptotically stable. Similarly, if R0>1, at least one eigenvalue of matrix F−V lies in the right half plane, and the disease-free equilibrium P0 is unstable.
Theorem 4.2. Suppose that R0<1, then the disease-free equilibrium P0 is globally asymptotically stable.
Proof. Since V−1F=V−1FV−1V, according to the properties of similar matrixs, we have ρ(V−1F)=ρ(FV−1)=R0. Then from the Perron-Frobenius theorem [32], we can obtain that the non-negative irreducible matrix V−1F has a positive left eigenvector (w1,w2,…,w2n), which corresponds to the spectral radius ρ(V−1F), that is,
(w1,w2,…,w2n)V−1F=(w1,w2,…,w2n)ρ(V−1F). |
Let us consider a Lyapunov function
L=n∑i=1uiEi+n∑i=1viIi, | (4.3) |
where (u1,u2,…,un,v1,v2,…,vn)=(w1,w2,…,w2n)V−1.
Calculating the differentiation of L along the solutions of system (2.2), we obtain
dLdt=n∑i=1uidEidt+n∑i=1vidIidt=n∑i=1ui[βiSiIi+kiβiViIi−(dEi+σi)Ei+n∑j=1(cijEj−cjiEi)]+n∑i=1vi[σiEi−(dIi+γi)Ii+n∑j=1(dijIj−djiIi)]. |
Since Si≤S0i and Vi≤V0i, when R0<1, we have
dLdt≤n∑i=1ui[βiS0iIi+kiβiV0iIi−(dEi+σi)Ei+n∑j=1(cijEj−cjiEi)]+n∑i=1vi[σiEi−(dIi+γi)Ii+n∑j=1(dijIj−djiIi)]=(u1,u2,…,un)(F1I−V11E)−(v1,v2,…,vn)(V21E+V22I)=(u1,u2,…,un,v1,v2,…,vn)(F−V)X=(w1,w2,…,w2n)V−1(F−V)X=(w1,w2,…,w2n)(V−1F−E)X=(w1,w2,…,w2n)(R0−1)X≤0, | (4.4) |
where E=(E1,E2,…,En)T,I=(I1,I2,…,In)T.
Hence, dLdt=0 if and only if Si=S0i,Vi=V0i and Ei=0,Ii=0. When Si=S0i, from the first equation of system (2.2), we have
0=dS0idt=(1−pi)Λi−βiS0iIi−(dSi+qi)S0i+n∑j=1(aijS0j−ajiS0i). |
It can be obtained that Ii=0 for i=1,2,…,n. This implies that the largest compact invariant set in {(S1,V1,E1,I1,…,Sn,Vn,En,In)∈Γ:Si=S0i,Vi=V0i,Ei=Ii=0,i=1,2,…n} is the singleton {P0}. From LaSalle's Invariance Principle [34], we obtain that P0 is globally attractive. Therefore, P0 is globally asymptotically stable in Γ once R0<1.
Theorem 4.3. Suppose that cij>0, dij>0(i,j=1,2,…,n,j≠i). If R0>1, then system (2.2) is uniformly persistent, i.e., there exists a constant c>0 such that every solution φt(x0)≡(S1,V1,E1,I1,…,Sn,Vn,En,In) of system (2.2) satisfies
liminft→∞Ei≥c,liminft→∞Ii≥c,i=1,2,…,n, |
where x0=(S1(0),V1(0),E1(0),I1(0),…,Sn(0),Vn(0),En(0),In(0))∈Rn+×Rn+×(Rn+∖{0})×(Rn+∖{0}), and system (2.2) admits at least one endemic equilibrium.
Proof. According to the uniform persistence theorem formulated in Wang and Zhao [14,35], we prove our result as follows. For convenience, we denote the solution (S1,V1,E1,I1,…,Sn,Vn,En,In) of system (2.2) by (S,V,E,I). Define
X={(S,V,E,I):Si≥0,Vi≥0,Ei≥0,Ii≥0,i=1,2,…,n},X0={(S,V,E,I)∈X:Ei>0,Ii>0,i=1,2,…,n},∂X0=X∖X0. |
It suffices to prove that system (2.2) is uniformly persistent with respect to (X0,∂X0). By the form of (2.2), it is clear that both X and X0 are positively invariant.∂X0 is relatively closed in X. Moreover, system (2.2) is point dissipative in Section 3.
The set M∂={x0∈∂X0:φt(x0)∈∂X0,∀t>0} and M={(S,V,0,0):S≥0,V≥0}. We next claim that M∂=M. Clearly, M⊂M∂. it suffices to show that M∂∖M=∅. Assume that x0∈M∂∖M, then there is an i0, 1≤i0≤n and a t0≥0 such that Ei0(t0)>0,Ii0(t0)>0. The set {1,2,…,n} can be decomposed into two sets L1 and L2, where
Ei(t0)=Ii(t0)=0,∀i∈L1,Ei(t0)>0,Ii(t0)>0,∀i∈L2. |
Clearly, L1 and L2 are both nonempty. For any j∈L1,i0∈L2, we have
dEj(t0)dt≥n∑m=1cjmEm(t0)≥cji0Ei0(t0)>0,dIj(t0)dt≥n∑m=1djmIm(t0)≥dji0Ii0(t0)>0. | (4.5) |
Hence there exist an ϵ0 such that Ej(t)>0,Ij(t)>0,j∈L1 for t0<t<t0+ϵ0. Obviously, we can restrict ϵ0 small enough such that Ei(t)>0,Ii(t)>0,i∈L2 for t0<t<t0+ϵ0. Therefore, we know that φt(x0)∉∂X0 for t0<t<t0+ϵ0, which contradicts the assumption that x0∈M∂.
It is easy to verify that P0 is the unique equilibrium in M∂. Next, we will show that WS(P0)∩X0=∅, where WS(P0) is the stable manifold of P0. Choose δ small enough such that limsupt→∞|φt(x0)−P0|>δ for x0∈X0. Suppose that this does not hold. Then we have |φt(x0)−P0|≤δ for all t≥0. This implies that, for any sufficiently small positive constant ϵ, there exists a T>0 such that
S0i−ϵ≤Si(t),V0i−ϵ≤Vi(t),for∀t>T. |
From system (2.2), we obtain
dEidt≥βiIi(S0i−ε)+kiβiIi(V0i−ε)−(dEi+σi)+n∑j=1(cijEj−cjiEi),dIidt=σiEi−(dIi+γi)+n∑j=1(dijIj−djiIi),for∀t>T. | (4.6) |
Let
G=[−V11F1−εG1−V21−V22]=F−V−ε[0G100], |
where G1=diag{β1(k1+1),…,βn(kn+1)}. Then it follows from (4.2) and (4.6) that dXdt≥GX. Applying Lemma 4.1 yields that s(F−V)>0 if and only if R0>1. Hence there exists a small enough ε1 such that s(G)>0 for ε1∈[0,ε]. Therefore, matrix G has a positive eigenvalue s(G), which has a positive eigenvector. By the comparison principle, it is clear that (Ei,Ii)→(∞,∞) as t→∞,i=1,2,…,n. This contradicts the previous assumption. Consequently, limsupt→∞|φt(x0)−P0|>δ, that is, WS(P0)∩X0=∅.
Obviously, every orbit in M∂ converges to {P0} and {P0} is an isolated invariant set and acyclic. Thus, Theorem 4.6 of Thieme [36] implies that system (2.2) is uniformly persistent with respect to (X0,∂X0). The proof is completed. By Theorem 2.4 in Zhao [35], system (2.2) has an equilibrium E∗=(S∗1,V∗1,E∗1,I∗1,…,S∗n,V∗n,E∗n,I∗n). The equations governing Si and Vi in system (2.2) ensure that S∗i>0 and V∗i>0 for i=1,2,…,n. This indicates that E∗ is an endemic equilibrium of system (2.2).
In this section, we give some numerical simulations to show the feasibility of our theoretical results and illustrate the impacts of vaccination and migration on disease prevalence.
We consider a special case of system (2.2) with n=3, where the values of migration rates are given in Table 1 and the remaining parameter values are shown in Table 2. The initial condition is considered as (S1(0),V1(0),E1(0),I1(0),S2(0),V2(0),E2(0),I2(0),S3(0),V3(0),E3(0),I3(0))=(800,120,100,80,1000,400,248,250,1200,300,240,200). Based on these parameter values, we can calculate that P0=(317,259,0,0,258,265,0,0,244,304,0,0) and R0=0.5<1. Figure 2 shows that the trajectories of system (2.2) ultimately converge to P0, which is globally asymptotically stable. So the disease will eventually die out. Now, we assume that the transmission coefficients βi, are relatively high, e.g., β1=0.0012,β2=0.0015,β3=0.0025, and keep the remaining parameter values unchanged. Then we can calculate that R0=1.53>1. As seen in Figure 3, system (2.2) has a positive equilibrium, which confirms that the disease is uniformly persistent.
Para. | a12 | a13 | a21 | a23 | a31 | a32 | b12 | b13 | b21 | b23 | b31 | b32 |
Value | 0.025 | 0.027 | 0.022 | 0.039 | 0.034 | 0.032 | 0.06 | 0.1 | 0.08 | 0.15 | 0.12 | 0.16 |
Para. | c12 | c13 | c21 | c23 | c31 | c32 | d12 | d13 | d21 | d23 | d31 | d32 |
Value | 0.05 | 0.06 | 0.04 | 0.1 | 0.08 | 0.12 | 0.03 | 0.05 | 0.05 | 0.09 | 0.06 | 0.1 |
Para. | pi | Λi | βi | qi | ki | σi | γi | dSi | dVi | dEi | dIi |
i=1 | 0.6 | 30 | 0.004 | 0.01 | 0.03 | 0.14 | 0.15 | 0.013 | 0.06 | 0.02 | 0.12 |
i=2 | 0.7 | 20 | 0.005 | 0.018 | 0.04 | 0.2 | 0.18 | 0.012 | 0.1 | 0.01 | 0.15 |
i=3 | 0.8 | 40 | 0.008 | 0.04 | 0.05 | 0.28 | 0.2 | 0.005 | 0.13 | 0.03 | 0.1 |
Next, the effects of vaccination on infectious populations are shown in Figures 4 and 5. Assume p1=p2=p3 and keep the other parameters in Tables 1 and 2 unchanged. From Figure 4, it can be observed that the numbers of infectious population decrease as the vaccination rate increases. The results imply that vaccination strategy plays an important role in preventing disease spread. Then, assume q1=q2=q3. The impact of different vaccination strategies is shown in Figure 5. The blue solid represents that no vaccination strategy is implemented; the green solid represents vaccination of only susceptible populations; the red solid represents vaccination of only newborns; and the black solid represents that both vaccination strategies are implemented simultaneously. It can be seen from Figure 5 that the simultaneous execution of both vaccination strategies is the most effective way to reduce the number of infectious populations. Therefore, it should be suggested that both kinds of vaccination strategies should be implemented simultaneously.
The effects of migration on disease transmission are presented in Figure 6. Figure 6(a)–(c) show that as the migration rate increases, the numbers of infectious populations in patch 1 and patch 2 both decrease. However, with the increase in migration rate, the number of infectious populations in patch 3 increases. Hence, population migration can inhibit disease spread in patch 1 and patch 2, while spread in patch 3. Then, assume β1=0.0008,β2=0.001,β3=0.0015. It is shown that R0 increases with the increase of migration rate in Figure 6d. Thus, in order to prevent the outbreak of disease, we should limit or prohibit the migration of infectious populations from patch 1 to patch 3.
In this paper, we present an SVEIR epidemic model with vaccination in a patchy environment to investigate the impacts of vaccination strategy and population migration on disease dynamics. Based on the basic reproduction number R0, we prove that the disease-free equilibrium P0 is locally as well as globally asymptotically stable if R0<1. In the case of R0>1, we show that the system (2.2) is uniformly persistent. The numerical simulation results validate our stability analysis. In addition, numerical simulations indicate that vaccination is helpful for disease control in all patches and simultaneous execution of two vaccination strategies can be more effective in controlling the disease. However, population migration does not always have a positive impact on disease spread. An increase in migration rate can either promote or inhibit disease transmission within a patch.
The impacts of vaccination strategy and population migration have been investigated in our paper. It can be concluded that vaccination can effectively control the spread of diseases. However, in the early stage of some emerging infectious diseases, vaccines have not been developed, so it is necessary to consider more control measures to prevent the outbreaks of diseases. In future work, we will extend the model by taking into account other control measures such as treatment and isolation.
The authors declare they have not used Artificial Intelligence (AI) tools in the creation of this article.
This research was partly supported by the National Natural Science Foundation of China (Nos. 12071445 and 12271519) and the High-level Talent Introduction Support Project (No. GDRC20220802).
The authors declare there is no conflict of interest.
[1] |
A. L. Lloyd, R. M. May, Spatial heterogeneity in epidemic models, J. Theor. Biol., 179 (1996), 1–11. https://doi.org/10.1006/jtbi.1996.0042 doi: 10.1006/jtbi.1996.0042
![]() |
[2] |
D. J. Rodríguez, L. Torres-Sorando, Models of infectious diseases in spatially heterogeneous environments, Bull. Math. Biol., 63 (2001), 547–571. https://doi.org/10.1006/bulm.2001.0231 doi: 10.1006/bulm.2001.0231
![]() |
[3] |
J. Arino, J. R. Davis, D. Hartley, R. Jordan, J. M. Miller, P. Van Den Driessche, A multi-species epidemic model with spatial dynamics, Math. Med. Biol., 22 (2005), 129–142. https://doi.org/10.1093/imammb/dqi003 doi: 10.1093/imammb/dqi003
![]() |
[4] |
W. Wang, G. Mulone, Threshold of disease transmission in a patch environment, J. Math. Anal. Appl., 285 (2003), 321–335. https://doi.org/10.1016/S0022-247X(03)00428-1 doi: 10.1016/S0022-247X(03)00428-1
![]() |
[5] |
C. Wolf, M. Langlais, F. Sauvage, D. Pontier, A multi-patch epidemic model with periodic demography, direct and indirect transmission and variable maturation rate, Math. Popul. Stud., 13 (2006), 153–177. https://doi.org/10.1080/08898480600788584 doi: 10.1080/08898480600788584
![]() |
[6] |
D. Gao, S. Ruan, A multipatch malaria model with logistic growth populations, SIAM J. Appl. Math., 72 (2012), 819–841. https://doi.org/10.1137/110850761 doi: 10.1137/110850761
![]() |
[7] |
Q. Liu, D. Jiang, Global dynamical behavior of a multigroup SVIR epidemic model with Markovian switching, Int. J. Biomath., 15 (2022), 2150080. https://doi.org/10.1142/S1793524521500807 doi: 10.1142/S1793524521500807
![]() |
[8] |
Z. Qiu, Q. Kong, X. Li, M. Martcheva, The vector–host epidemic model with multiple strains in a patchy environment, J. Math. Anal. Appl., 405 (2013), 12–36. https://doi.org/10.1016/j.jmaa.2013.03.042 doi: 10.1016/j.jmaa.2013.03.042
![]() |
[9] | Y. Chen, M. Yan, Z. Xiang, Transmission dynamics of a two-city SIR epidemic model with transport-related infections, J. Appl. Math., 2014 (2014). https://doi.org/10.1155/2014/764278 |
[10] |
H. L. Li, L. Zhang, Z. Teng, Y. L. Jiang, A. Muhammadhaji, Global stability of an SI epidemic model with feedback controls in a patchy environment, Appl. Math. Comput., 321 (2018), 372–384. https://doi.org/10.1016/j.amc.2017.10.057 doi: 10.1016/j.amc.2017.10.057
![]() |
[11] |
V. P. Bajiya, J. P. Tripathi, V. Kakkar, Y. Kang, Modeling the impacts of awareness and limited medical resources on the epidemic size of a multi-group SIR epidemic model, Int. J. Biomath., 15 (2022), 2250045. https://doi.org/10.1142/S1793524522500450 doi: 10.1142/S1793524522500450
![]() |
[12] |
H. W. Hethcote, Qualitative analyses of communicable disease models, Math. Biosci., 28 (1976), 335–356. https://doi.org/10.1016/0025-5564(76)90132-2 doi: 10.1016/0025-5564(76)90132-2
![]() |
[13] |
S. Ruan, W. Wang, S. A. Levin, The effect of global travel on the spread of SARS, Math. Biosci. Eng., 3 (2006), 205. https://doi.org/10.3934/mbe.2006.3.205 doi: 10.3934/mbe.2006.3.205
![]() |
[14] |
W. Wang, X. Q. Zhao, An epidemic model in a patchy environment, Math. Biosci., 190 (2004), 97–112. https://doi.org/10.1016/j.mbs.2002.11.001 doi: 10.1016/j.mbs.2002.11.001
![]() |
[15] |
M. Salmani, P. van den Driessche, A model for disease transmission in a patchy environment, Discrete Contin. Dyn. Syst. Ser. B., 6 (2006), 185–202. https://doi.org/10.3934/dcdsb.2006.6.185 doi: 10.3934/dcdsb.2006.6.185
![]() |
[16] |
M. Y. Li, Z. Shuai, Global stability of an epidemic model in a patchy environment, Canad. Appl. Math. Q., 17 (2009), 175–187. https://doi.org/10.1016/j.amc.2017.10.057 doi: 10.1016/j.amc.2017.10.057
![]() |
[17] |
D. Gao, Travel frequency and infectious diseases, SIAM J. Appl. Math., 79 (2019), 1581–1606. https://doi.org/10.1137/18M1211957 doi: 10.1137/18M1211957
![]() |
[18] |
X. Wang, S. Liu, L. Wang, W. Zhang, An epidemic patchy model with entry–exit screening, Bull. Math. Biol., 77 (2015), 1237–1255. https://doi.org/10.1007/s11538-015-0084-6 doi: 10.1007/s11538-015-0084-6
![]() |
[19] |
M. El Hajji, A. H. Albargi, A mathematical investigation of an "SVEIR" epidemic model for the measles transmission, Math. Biosci. Eng., 19 (2022), 2853–2875. https://doi.org/10.3934/mbe.2022131 doi: 10.3934/mbe.2022131
![]() |
[20] |
Z. Wang, Q. Zhang, Optimal vaccination strategy for a mean-field stochastic susceptible-infected-vaccinated system, Int. J. Biomath., 16 (2023), 2250061. https://doi.org/10.1142/S1793524522500619 doi: 10.1142/S1793524522500619
![]() |
[21] |
X. Liu, Y. Takeuchi, S. Iwami, SVIR epidemic models with vaccination strategies, J. Theor. Biol., 253 (2008), 1–11. https://doi.org/10.1016/j.jtbi.2007.10.014 doi: 10.1016/j.jtbi.2007.10.014
![]() |
[22] |
J. Li, Y. Yang, SIR-SVS epidemic models with continuous and impulsive vaccination strategies, J. Theor. Biol., 280 (2011), 108–116. https://doi.org/10.1016/j.jtbi.2011.03.013 doi: 10.1016/j.jtbi.2011.03.013
![]() |
[23] |
X. Duan, S. Yuan, Z. Qiu, J. Ma, Global stability of an SVEIR epidemic model with ages of vaccination and latency, Comput. Math. Appl., 68 (2014), 288–308. https://doi.org/10.1016/j.camwa.2014.06.002 doi: 10.1016/j.camwa.2014.06.002
![]() |
[24] |
L. M. Cai, Z. Li, X. Song, Global analysis of an epidemic model with vaccination, J. Appl. Math. Comput., 57 (2018), 605–628. https://doi.org/10.1007/s12190-017-1124-1 doi: 10.1007/s12190-017-1124-1
![]() |
[25] |
Q. Cui, Z. Qiu, L. Ding, An SIR epidemic model with vaccination in a patchy environment, Math. Biosci. Eng., 14 (2017), 1141–1157. https://doi.org/10.3934/mbe.2017059 doi: 10.3934/mbe.2017059
![]() |
[26] |
M. De la Sen, A. Ibeas, S. Alonso-Quesada, R. Nistal, On a SIR model in a patchy environment under constant and feedback decentralized controls with asymmetric parameterizations, Symmetry, 11 (2019), 430. https://doi.org/10.3390/sym11030430 doi: 10.3390/sym11030430
![]() |
[27] | M. De la Sen, A. Ibeas, S. Alonso-Quesada, R. Nistal, Infectious diseases of humans: dynamics and control, Oxford University Press, 1991. https://doi.org/10.1016/0169-5347(91)90048-3 |
[28] |
L. Zhang, Z. C. Wang, X. Q. Zhao, Threshold dynamics of a time periodic reaction–diffusion epidemic model with latent period, J. Differ. Equ., 258 (2015), 3011–3036. https://doi.org/10.1016/j.jde.2014.12.032 doi: 10.1016/j.jde.2014.12.032
![]() |
[29] |
Y. Lou, X. Q. Zhao, A reaction–diffusion malaria model with incubation period in the vector population, J. Math. Biol., 62 (2011), 543–568. https://doi.org/10.1007/s00285-010-0346-8 doi: 10.1007/s00285-010-0346-8
![]() |
[30] |
X. F. San, Z. C. Wang, Traveling waves for a two-group epidemic model with latent period in a patchy environment, J. Math. Anal. Appl., 475 (2019), 1502–1531. https://doi.org/10.1016/j.jmaa.2019.03.029 doi: 10.1016/j.jmaa.2019.03.029
![]() |
[31] |
J. Li, X. Zou, Dynamics of an epidemic model with non-local infections for diseases with latency over a patchy environment, J. Math. Biol., 60 (2010), 645–686. https://doi.org/10.1007/s00285-009-0280-9 doi: 10.1007/s00285-009-0280-9
![]() |
[32] | A. Berman, R. J. Plemmons, Nonnegative Matrices in the Mathematical Sciences, Academic Press, New York, 1979. https://doi.org/10.1016/C2013-0-10361-3 |
[33] |
P. van den Driessche, J. Watmough, Reproduction numbers and sub-threshold endemic equilibria for compartmental models of disease transmission, Math. Biosci., 180 (2002), 29–48. https://doi.org/10.1016/S0025-5564(02)00108-6 doi: 10.1016/S0025-5564(02)00108-6
![]() |
[34] | J. P. LaSalle, The Stability of Dynamical Systems, SIAM, 1976. https://doi.org/10.1137/1.9781611970432 |
[35] | X. Q. Zhao, Uniform persistence and periodic coexistence states in infinite-dimensional periodic semiflows with applications, Canad. Appl. Math. Q., 3 (1995), 473–495. |
[36] |
H. R. Thieme, Persistence under relaxed point-dissipativity (with application to an endemic model), SIAM J. Math. Anal., 24 (1993), 407–435. https://doi.org/10.1137/0524026 doi: 10.1137/0524026
![]() |
1. | 鸿艳 王, Stability Analysis of Drug-Resistant Tuberculosis Model in a Two-Patch Environment, 2024, 13, 2324-7991, 3502, 10.12677/aam.2024.137335 | |
2. | Yibekal Walle, Joseph Y.T. Mugisha, Dawit Melese, Haileyesus Tessema, The impact of movement and vaccination on Peste des Petits Ruminants disease spread between two different agroecological zones, 2025, 27, 24682276, e02532, 10.1016/j.sciaf.2025.e02532 | |
3. | Wei Li, Yi Wang, Zhen Jin, Dynamic analysis of a two-strain tuberculosis model with imperfect treatment on complex networks, 2025, 0, 1531-3492, 0, 10.3934/dcdsb.2025036 |
Para. | a12 | a13 | a21 | a23 | a31 | a32 | b12 | b13 | b21 | b23 | b31 | b32 |
Value | 0.025 | 0.027 | 0.022 | 0.039 | 0.034 | 0.032 | 0.06 | 0.1 | 0.08 | 0.15 | 0.12 | 0.16 |
Para. | c12 | c13 | c21 | c23 | c31 | c32 | d12 | d13 | d21 | d23 | d31 | d32 |
Value | 0.05 | 0.06 | 0.04 | 0.1 | 0.08 | 0.12 | 0.03 | 0.05 | 0.05 | 0.09 | 0.06 | 0.1 |
Para. | pi | Λi | βi | qi | ki | σi | γi | dSi | dVi | dEi | dIi |
i=1 | 0.6 | 30 | 0.004 | 0.01 | 0.03 | 0.14 | 0.15 | 0.013 | 0.06 | 0.02 | 0.12 |
i=2 | 0.7 | 20 | 0.005 | 0.018 | 0.04 | 0.2 | 0.18 | 0.012 | 0.1 | 0.01 | 0.15 |
i=3 | 0.8 | 40 | 0.008 | 0.04 | 0.05 | 0.28 | 0.2 | 0.005 | 0.13 | 0.03 | 0.1 |
Para. | a12 | a13 | a21 | a23 | a31 | a32 | b12 | b13 | b21 | b23 | b31 | b32 |
Value | 0.025 | 0.027 | 0.022 | 0.039 | 0.034 | 0.032 | 0.06 | 0.1 | 0.08 | 0.15 | 0.12 | 0.16 |
Para. | c12 | c13 | c21 | c23 | c31 | c32 | d12 | d13 | d21 | d23 | d31 | d32 |
Value | 0.05 | 0.06 | 0.04 | 0.1 | 0.08 | 0.12 | 0.03 | 0.05 | 0.05 | 0.09 | 0.06 | 0.1 |
Para. | pi | Λi | βi | qi | ki | σi | γi | dSi | dVi | dEi | dIi |
i=1 | 0.6 | 30 | 0.004 | 0.01 | 0.03 | 0.14 | 0.15 | 0.013 | 0.06 | 0.02 | 0.12 |
i=2 | 0.7 | 20 | 0.005 | 0.018 | 0.04 | 0.2 | 0.18 | 0.012 | 0.1 | 0.01 | 0.15 |
i=3 | 0.8 | 40 | 0.008 | 0.04 | 0.05 | 0.28 | 0.2 | 0.005 | 0.13 | 0.03 | 0.1 |