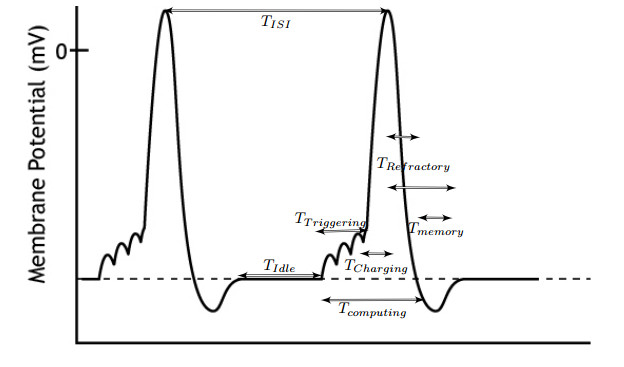
Neural information theory represents a fundamental method to model dynamic relations in biological systems. However, the notion of information, its representation, its content and how it is processed are the subject of fierce debates. Since the limiting capacity of neuronal links strongly depends on how neurons are hypothesized to work, their operating modes are revisited by analyzing the differences between the results of the communication models published during the past seven decades and those of the recently developed generalization of the classical information theory. It is pointed out that the operating mode of neurons is in resemblance with an appropriate combination of the formerly hypothesized analog and digital working modes; furthermore that not only the notion of neural information and its processing must be reinterpreted. Given that the transmission channel is passive in Shannon's model, the active role of the transfer channels (the axons) may introduce further transmission limits in addition to the limits concluded from the information theory. The time-aware operating model enables us to explain why (depending on the researcher's point of view) the operation can be considered either purely analog or purely digital.
Citation: János Végh, Ádám József Berki. Revisiting neural information, computing and linking capacity[J]. Mathematical Biosciences and Engineering, 2023, 20(7): 12380-12403. doi: 10.3934/mbe.2023551
[1] | Lubomir Kostal, Shigeru Shinomoto . Efficient information transfer by Poisson neurons. Mathematical Biosciences and Engineering, 2016, 13(3): 509-520. doi: 10.3934/mbe.2016004 |
[2] | Li Yang, Kai Zou, Kai Gao, Zhiyi Jiang . A fuzzy DRBFNN-based information security risk assessment method in improving the efficiency of urban development. Mathematical Biosciences and Engineering, 2022, 19(12): 14232-14250. doi: 10.3934/mbe.2022662 |
[3] | Karim El Laithy, Martin Bogdan . Synaptic energy drives the information processing mechanisms in spiking neural networks. Mathematical Biosciences and Engineering, 2014, 11(2): 233-256. doi: 10.3934/mbe.2014.11.233 |
[4] | Meiyuan Du, Chi Zhang, Sheng Xie, Fang Pu, Da Zhang, Deyu Li . Investigation on aortic hemodynamics based on physics-informed neural network. Mathematical Biosciences and Engineering, 2023, 20(7): 11545-11567. doi: 10.3934/mbe.2023512 |
[5] | Jiao Wu, Shi Qiu, Ming Liu, Huayi Li, Yuan Liu . Finite-time velocity-free relative position coordinated control of spacecraft formation with dynamic event triggered transmission. Mathematical Biosciences and Engineering, 2022, 19(7): 6883-6906. doi: 10.3934/mbe.2022324 |
[6] | Jue Wu, Lei Yang, Fujun Yang, Peihong Zhang, Keqiang Bai . Hybrid recommendation algorithm based on real-valued RBM and CNN. Mathematical Biosciences and Engineering, 2022, 19(10): 10673-10686. doi: 10.3934/mbe.2022499 |
[7] | Hongji Xu, Shi Li, Shidi Fan, Min Chen . A new inconsistent context fusion algorithm based on BP neural network and modified DST. Mathematical Biosciences and Engineering, 2021, 18(2): 968-982. doi: 10.3934/mbe.2021051 |
[8] | Liguo Zhang, Liangyu Zhao, Yongtao Yan . A hybrid neural network-based intelligent body posture estimation system in sports scenes. Mathematical Biosciences and Engineering, 2024, 21(1): 1017-1037. doi: 10.3934/mbe.2024042 |
[9] | Yang Liu, Tianran Tao, Xuemei Liu, Jiayun Tian, Zehong Ren, Yize Wang, Xingzhi Wang, Ying Gao . Knowledge graph completion method for hydraulic engineering coupled with spatial transformation and an attention mechanism. Mathematical Biosciences and Engineering, 2024, 21(1): 1394-1412. doi: 10.3934/mbe.2024060 |
[10] | Xiaomeng Feng, Taiping Wang, Xiaohang Yang, Minfei Zhang, Wanpeng Guo, Weina Wang . ConvWin-UNet: UNet-like hierarchical vision Transformer combined with convolution for medical image segmentation. Mathematical Biosciences and Engineering, 2023, 20(1): 128-144. doi: 10.3934/mbe.2023007 |
Neural information theory represents a fundamental method to model dynamic relations in biological systems. However, the notion of information, its representation, its content and how it is processed are the subject of fierce debates. Since the limiting capacity of neuronal links strongly depends on how neurons are hypothesized to work, their operating modes are revisited by analyzing the differences between the results of the communication models published during the past seven decades and those of the recently developed generalization of the classical information theory. It is pointed out that the operating mode of neurons is in resemblance with an appropriate combination of the formerly hypothesized analog and digital working modes; furthermore that not only the notion of neural information and its processing must be reinterpreted. Given that the transmission channel is passive in Shannon's model, the active role of the transfer channels (the axons) may introduce further transmission limits in addition to the limits concluded from the information theory. The time-aware operating model enables us to explain why (depending on the researcher's point of view) the operation can be considered either purely analog or purely digital.
Neuroscience applied information theory to its field immediately after that the theory became available [1,2]. Shannon [3] developed his mathematical theory with the needs of electric signal transmission in mind (implied approximations valid for that domain). The theory did not discuss the approximations needed to apply it to any non-mathematical domain. Hence, to apply it to a domain, approximations different from the ones used implicitly for electric communication might be needed, see [4]. Any new domain, including neuroscience, wanting to use Shannon's mathematical formalism must use his model or communicational system in the same sense and develop its useful approximations. However, in neuroscience, "the interpretational gamut implies hundreds, perhaps thousands, of different possible neuronal versions of Shannon's general communication system" [5].
Although the theory uses examples mainly from technical communication, it is general enough to be applied to different domains, provided that the conditions of applicability are satisfied. Information theory has taken neuroscience by storm, despite Shannon's early warning that "the hard core of information theory is, essentially, a branch of mathematics" and it "is not a trivial matter of translating words to a new domain. … The use of a few exciting words like information, entropy, redundancy, do not solve all our problems. It is certainly no panacea for the communication engineer or, a fortiori, for anyone else" [6]. The basic difficulty with using information theory is that "Communication engineers want to design systems; neuroscientists want to analyze an existing one they little understand" [7].
The appropriate interpretation of the fundamental concept is also vital in light of the experienced convergence of information theory and neuroscience. "Simply applying formulae from information theory without understanding the assumptions behind their definitions can lead to erroneous results and conclusions" [8]. The "great migration" [9], to convert information content (more precisely: the measured signals) to knowledge, is not possible if the information content of neural communication is not interpreted appropriately. The following sections of our paper attempt to contribute to the task.
Mathematics aims to describe nature, but it is also a science in its own right, with its methods and rules. The infinitely complex phenomena seen in nature must be approximated (some features neglected) to use a theory defined for abstract mathematical virtues while keeping the rules of mathematics. Mathematics cannot genuinely describe nature (taking a reasonable amount of effort) in its full complexity, and oversimplifying the features results in the rough (unrealistic) descriptions. Determining which features can be omitted is the task of the discipline wanting to apply a mathematical theory. The omissions also depend on the requested accuracy of description and the actual conditions and goals.
A common point of classic theories referred to below is how they handle time. Classic science fields still use Newtonian physics, where the objects on the scene of the theater (i.e., space) interact instantly, assuming that the interaction speed is infinitely large. The philosophical aspects of introducing time-aware interactions are discussed in [10], its relevance to computing science [11], information theory [4], biology [12] and cognitive sciences [13] are discussed separately. Despite the appearance of the so-called "modern science" some hundred years ago, the Newtonian equations work reasonably well for most practical cases. However, one must consider that the interaction speed is finite when approaching the extremely high speed of light. In biology, the interaction speed (the conduction velocity) is millions of times lower, so the effect of the finite interaction speed must be used everywhere where the speed of processes is near that speed. Fortunately, the required mathematical tools are available [12,14,15].
One should be cautious with using the basic notions of physics to draw parallels between biological and electric circuits. In an electric circuit, thought to be in parallel with the operation of a biological circuit, a battery is also present which applies a potential difference to the electric components to make them work; physical laws describe this phenomenon. In contrast, chemical forces move electrically charged particles in biological circuits, and the delivered charge produces the measurable potential. The phenomena due to similar significant differences led to the introduction of the virtue of the "non-ohmic" feature for the electric behavior of biology. "These results show non-ohmic properties of the extracellular medium around neurons, and suggest that source estimation methods, as well as the cable properties of neurons, which all assume ohmic extracellular medium, may need to be reevaluated" [16]. [17] demonstrated that other cellular components, including synapses and membrane, also show non-ohmic properties.
In addition, the several million times slower charge propagation speed needs to formulate physical laws differently. For example, Kirchoff's law essentially expresses the conservation of charge in a particular form. In the approximation of the "instant interaction" that classical physics uses, that law is perfect. In biology, the input current flows into the biological cell several msec earlier than the output current flows out; furthermore, due to the gated operating mode of the synapses and membrane, plus leaking, part of it flows to the extracellular space. Because of these effects, Kirchoff's law can be valid only when integrating for the total time domain, and the extracellular space is included in the model.
In physics, interactions have a speed, so for example, Coulomb's Law should be written as
(2.1) |
where the term takes into account that charges and at distance from each other –due to the finite propagation speed of the electric interaction– experience the force at time according to their distance was that time ago; k is the constant describing the electric interaction. This term has no role if the two charges do not change their position. If the distance changes, its effect is so small ( for a displacement) that the term can be omitted. So, our college knowledge can serve as a good approximation. However, given that the interaction speed is the same for gravitation, Earth experiences the force according to its distance to the Sun before; so we cannot neglect the effect anymore.
Similarly, we must not neglect the difference in interaction speeds of very different interactions. The usual discussion of the Nernst potential (see for example [18], Eq (11.28)) is
(2.2) |
with , the spatial variable across the membrane and the interaction speed-dependent term is omitted. ( is the gas constant, Faraday's constant, the valence of the axial current, the potential and is the concentration.) The interaction speeds on the left and right sides differ millions of times, but the description taken from classical physics handles them as if they were equal. In that case, other factors must compensate for the mistake, seriously affecting the description of the process.
In the theoretical description given by [18] "we choose a so-called point representation of a neuron. Here, the spatial dependency of the neuron is reduced to a single point or compartment". However, "dendritic trees can be quite large, containing up to 98% of the entire neuronal surface area. We, therefore, need to understand the behavior of these extended systems having cable-like structure". The attractive parallel with electronics covers that a cable is a passive electronic component while the membrane and the neuronal axon are active elements. In addition, biological networks of neurons comprise neurons with sub-millisecond operating time, connected by cables causing several millisecond delays. In contrast, the simulated networks usually do not have temporal behavior. The cooperation of neurons and their network is vital: "this interplay between the synaptic and neuronal dynamics, when the network is near a critical point, both recurrent spontaneous and stimulated phase transitions enable the phase-dependent processes to replace each other" [19]. Neuronal models using instant interaction describe biology-imitating artificial neural networks rather than biological neural networks. Using such models hinders understanding that the temporal interplay of neurons and their network defines brain operation.
The biological "computing process" inspired von Neumann [20] to invent his computing model. The model correctly described a computing process, but von Neumann introduced approximations valid only for the case of his target: the vacuum-tube-based electronic computer. He explicitly described that it would be unsound (sic) to apply his famous simplified paradigm to biological computing because, in that domain, the used approximations are not valid. [11] and [12] demonstrated that in another approximation (not omitting data transfer time), the same computing model on the one side could explain some issues of technical computing and on the other side, it can also describe biological computing. Notice that transferring data is just another word for communication. Although in neuroscience, it is commonly accepted that "The brain computes!" [18], in the generalization of the computing paradigm [11] (using a different approximation for the timing relations), it was pointed out that notions of biological computing differ very much from those of technical computing.
In the classic simplified computing paradigm, it is assumed that all of the limited numbers of non-replaceable digital arguments must reach the input section of the computing unit before operating, and the purpose-built logic gates can perform different operations with a mathematically well-defined result. Those elementary computing units alone can have relatively complex functionality. In biological computing, the first arriving "hole opening" [21] signal opens for the computing process a time window that is closed when the membrane reaches its threshold potential. Within that time window, the synaptic inputs "can replace each other" [19] (depending on their arrival time relative to opening the window [12]). [22] stated that "logical operations are all performed in the analog mode at the synapses". The gated neuronal condenser model operates with analog temporal arguments (the arrival of the postsynaptic spikes almost entirely determines the input arguments). Furthermore the result of the operation can only be issuing a spike (with some probability [23]). The result of the biological computation is the width of its time window (which is affected by short and long time learning [12]), and only an assembly of computing units (a neuron with its network) can perform a complex operation.
In light of the statements above, it is at least surprising to hypothesize very close analogies between biological and technical computing. However, – depending on the approximation – some resemblance can surely be found. When attempting to compare the brain to the computer, the first problem we face is to assess the computing power of the brain. We can "define an elementary operation of the brain as a single synaptic event" [24], and we know that both the operands and result are analog timing values; furthermore, "in a digital computer an elementary operation constitutes … a few instructions, such as load, add and multiply" [24] using digital signals. It is at least hard to compare these two elementary operations, either their computing power or computing speed. The second is how to map these operations to each other given that thousands of machine instructions are needed to emulate an analog integration (diffusion) and thousands of neurons are needed to perform a simple digital addition, not to mention the thousands of machine instructions needed to connect to the external world [25,26]. The third problem we face is the "non-deterministic", non-perfect way of operation of the brain as opposed to the deterministic, perfect operation of the computer. We can only admire von Neumann's genial prediction that "the language of the brain not the language of mathematics" [27], given that most of those cited experimental evidence was not available at his age. We also agree with von Neumann and Sejnowski that "whatever the system [of the brain] is, it cannot fail to differ from what we consciously and explicitly consider mathematics." However, we add that maybe that the appropriate mathematical methods are not yet invented. Furthermore, "it is also possible that non-biological hardware and computational paradigms may permit yet other varieties of machine intelligence we have not yet conceived" [28,29]. As a consequence, we can learn "that biological brains are more efficient learners than modern ML algorithms due to extra 'prior structure'" [28]. The false parallels with electric circuits (i.e., neglecting the fundamental differences between the digital and neuro-logical operating modes), moreover the preconception that nature-made biological computing must follow notions and conceptions of manufactured computing systems hinders understanding genuine biological computing.
Shannon [3] defined the logical components of his classic communication model. "An information source which produces a message or sequence of messages to be communicated to the receiving terminal". Correspondingly, "the destination is the person (or thing) for whom the message is intended." Notice that the information was handled as an abstract mathematical entity in these two components so that no approximation was needed.
"A transmitter which operates on the message in some way to produce a signal suitable for transmission over the channel" and "the receiver ordinarily performs the inverse operation of that done by the transmitter, reconstructing the message from the signal". Notice that the conversion between the abstract notion of "information" and its physical representation "signal" is performed by these units, and the receiver must be able to restore the abstract "information". Also, notice that converting and restoring information are just another word for computing, so computing and communication are inseparable. As pointed out by [30], "in information theory, entropy is associated with the probability distribution…: if there is no information lost or new information generated, then informational entropy is conserved." So, to apply information theory directly to neural communication, one should prove that in neural information representation no loss and generation happen.
The information can be coded (and will be converted to signal after coding), and if so, it must be correspondingly decoded and converted to information. Shannon [6] also warned that this coding/decoding must not be confused with that "the human being acts in some situations like an ideal decoder, this is an experimental and not a mathematical fact". Notice that a human observer sees a signal, instead of the coded information. Up to this point, we can assume that a presynaptic neuron produces information, and the system transfers it to the postsynaptic neuron using an axon. Notice also that Shannon requires to cut a neuron logically to a receiver (the synapses and the membrane) and a transmitter (the hillock) units, in line with as von Neumann introduced input and output sections.
In Shannon's model, the link connecting the communicating entities, "the channel is merely the medium used to transmit the signal from transmitter to receiver". Calculating its capacity was the main motivation for developing the theory. It could be calculated using physical entities [3]:
"Definition: The capacity C of a discrete channel is given by
(2.3) |
where is the number of allowed signals of duration T."
As explained in [4], the temporal length of a signal is the basic (from the point of view of the theory, external) entity. It should be defined per scientific field differently for electronic and biological communication. The theory implied that the digital amplitude carries the information (0/1) and that electromagnetic waves convey the signal, so the signal frequency defines .
When the theory of communication was applied to neuroscience, the role of the communication line, the "neuronal link", was in a similarly vital role. Notice that although anatomically, the axon is considered part of the neuron, it is an independent component from the point of view of communication functionality. The question of how information is represented remains out of scope: the theory confines its validity using strong mathematical theorems and definitions. However, the merit function must fulfill all the requirements of the strict mathematical frame and the model it is based upon.
The heavily cited and influencing review paper on "Information theory and neural coding" by [31] declares that "information theory quantifies how much information a neural response carries about the stimulus", and the authors "review information-theory basics before demonstrating its use in neural coding". Notice that for the elements of the neural communication system, 'neural response' corresponds to the abstract term 'information' while for the observers, 'neural response' corresponds to the physical appearance 'signal', the meaning of which is out of the scope of the theory. However, whether the elementary requirements of applicability of information theory for neural transmissions are satisfied, what is the abstract information discussed, how the abstract information is derived from the observed signals, what is the relation between the coding as Shannon used that notion and the observed one; furthermore if coding is a relevant metaphor [32] for the operation of the brain, are missing from this review. "Even more telling is the conclusion that examining a signal's entropy does not reveal whether the signal bears information or not" [7]. Another point is to scrutinize whether the assumed relation between stimulus and response is correct.
Given that Shannon's theory requires the transmitted information to be reproducible on the receiver side, if the encoded information is the state of the transmitter neuron, then the receiver neuron (in addition to its state) should be able to reproduce the state of the transmitter neuron. It would be a rather demanding task even in the case of a single transmitter neuron, and the receiver neuron may have the task of reproducing the state of up to thousands of transmitter neurons. The external observer may see some code in the patterns appearing on the neuronal links, but the neuron cannot decode them in the sense Shannon requires. The presence of multiple transmitters and the lack of reproducibility alone (see more lacking conditions in [4]) excludes directly applying Shannon's theory to neural transfer. In addition, as [32] pointed out, "the neural code depends on experimental details that are not carried by the coding variable" and "spikes are timed actions that mediate coupling in a distributed dynamical system". We can also conclude that the assumed "neural coding variable" is false. We must distinguish "coding" at the perception level and "coding" at the neural communication level; we discuss only the latter. The idea should be known in biology but still needs to be remembered: "Information that has been coded [at perception level] must at some point be decoded also. One suspects, then, that somewhere within the nervous system there is another interface, or boundary, but not necessarily a geometrical surface, where 'code' becomes 'image'" [22].
[31] asked the relevant questions "First, what is being encoded? Second, how is it being encoded? Third, with what precision?". In Shannon's terminology, the questions should be "First, what information is sent in a message? Second, how is it encoded and transmitted? Third, what do we measure and with what precision?". (Notice that the authors did not presuppose that a state is encoded.) The authors claimed that "using different stimulus ensembles and different response measures, one can begin to answer questions one and two", but they abandoned answering their question and make two methodical mistakes.
The first one is that they do not define what the measured quantity is: what they think to be the carrier of information; in Shannon's terminology, the signal. Instead, they attempt to define its measurement method. One can guess that they used the number of spikes as the merit function for information quantity: "the maximum information that could be transmitted as a function of firing rate has been estimated". Shannon [3] required that "two punched cards should have twice the capacity of one for information storage", one more requirement of the theory's applicability. However, in neural communication, "two spikes close together in time carry far more than twice the information carried by a single spike" [33]. Using firing rate as a merit function does not fulfill the linearity condition of adding information.
Furthermore, as pointed out from several theoretical points of view, spike count (and its derivates, such as firing rate) does not carry information [4,34]. Neural information science used not to quote the claim that "if a source can produce only one particular message, its entropy is zero" [3]. For biological communication, it means that identical spikes [34] alone (similarly, just counting the spikes in a time unit: an average or a rate) cannot be considered as a message. Therefore, the classic information theory applied to statistical entities derived from those measured firing rate data means applying the theory outside of its strictly cut range of validity.
The second mistake is that, in theory, the delivered information is about selecting one of a neuron's possible states. At the same time, the used stimulus is a temporal process instead (or at least a set of interrelated states). Given that the neuron has "a form of intracellular memory" [35] with time-dependent content which reflects the history of its environment (its "world"), it means that the same input can produce different outputs. However, information theory is about memory-less systems [36]. The static point of view of biology looks for "up and down" states [37] or "active states and ground state" [38], instead of considering the dynamic temporal nature of processes. Introducing hidden parameters [39] only circumvents the problem: a vector representation for the multiple input and memory states leads to a dimensional explosion [31], which blocks the practical usability of the method.
The importance of understanding the true nature of neural information and its processing is growing. There are very nice and wide-scan measurements on the energy costs of neural operation [40], on the role of compressive coding, the importance of redundancy and economy in neuron numbers [41] but their exact numeric figures are slightly doubtful because of the wrong interpretation of neural information (they measure firing rates and interpret coding from the signal patterns). One must be careful because "classic information theory is silent on using information theoretic measures to assess actual system performance" [7]. Energy efficiency is getting more crucial in modeling, and the importance of timing in achieving energy-efficient neural computing and communication is recognized [42].
Given the enormous efforts for simulating (at least large portions of) the brain, it would be vital and timely to endorse that the abstracted operation of the low-level brain functionality is correct, mainly because the operation of both individual neurons and their networks are mostly modeled using wrong parallels with their assumed electronic analogs. Also their information-processing features in the experimental research are evaluated along wrong principles. In this section, we attempt to oppose the opinion that "The obvious lack of a definitive, credible interpretation [of neural information] makes neuroscience calculations of channel capacity and information transmitted meaningless" [5].
An external observer can measure 'signals', so where the models mention 'information', we should read 'signal' instead. "The 'all-or-none' character [of neural signals] led naturally first to a model of the central nervous system in which information was represented in terms of binary digits … on the other hand, there has been a steady accumulation of other evidence adduced in favor of models employing frequency modulation of trains of impulses to represent the information transmitted" [43]. The model features were supported on both sides by strong experimental evidence. MacKay and McCulloch believed that "it is not our purpose to reopen the 'analogical versus digital' question, which we believe an unphysiological antithesis". Instead, they introduced an inclusive definition for signal, event and information: "A signal is a physical event that, to the receiver, was not bound to happen at the time or in the way it did. … A neuronal impulse carries information in the sense that it indicates something of the states of affairs at its point of origin. … For our purpose, it will be sufficient to consider the observable time-relations which obtain between incoming and outgoing impulses in cases in which these are temporarily coherent." It was found early that "precise discriminations could, at least in principle, be based on the occurrence of individual spikes or spike pairs at definite times" in [44], Epiloge. Later research by [31,45,46,47] completed it that precise timing of spikes matters and that the information transferred in neural communication is primarily temporal. Experimental and theoretical research agree that "the timing of spikes is important with a precision roughly two orders of magnitude greater than the temporal dynamics of the stimulus" [48]) and "it makes sense to report about changes in this rate over intervals as short as a few tens of microseconds because … some neurons … can and often do produce spikes at an average time separation of only a few microseconds" [42].
The role of timing relations persisted in the history of interpreting neural signals, although the notion of neural information, evidently due to the successes and easy-to-handle nature of the classic information theory changed towards a probabilistic interpretation: "Information is the reduction of uncertainty about some situation X associated with observing any variable Y that is causally correlated with X. … To convey information, a neuron must represent the state of its input as a distinct output. … It follows that the capacity of neuron is limited by the number of distinctly different outputs that it can generate. … This depends on two factors ( spikes per second) and the precision of spike timing ()" [46] (p 51). That is, the description contains only states and no transition processes between them but in addition to causality, also temporality is a significant issue. The discussion in [4] emphasized that the information is shared between a neuron and its environment. Furthermore, its storage and processing cannot be separated from its transmission, and in the transmitted signals, the timing matters.
[43] also found that "the amplitude of a nerve impulse is relatively slowly variable, and the total amount of information conveyable by such variation would be relatively small." Although this claim is valid in general, [49] provided experimental evidence that the shape of the spike can also carry additional information (whether the neuron shall be re-synchronized). Given that the time of information processing in neurons is in the order of , while the duration of a spike is in the order of ; if the shape of spikes conveys additional information, it may be located in the front part of the spike, per what can be concluded from our time-aware model.
For their further discussion, MacKay and McCulloch assumed that the amplitude of the signal can be either zero or one: "thus each impulse, simply by its presence or absence during the short period over which it was expected, could provide one unit or 'bit' of selective information" [43]. They did not state, however, that the neuronal impulse can be expected, i.e., that the presence or absence of an impulse can be interpreted as information. The fallacy that measuring the conveyed information by simply counting the spikes might take its roots in the sentence above (or that "the most readily recordable signs of nerve activity are action potentials of nerve fibers" [22] or, most probably stated by [50] that "The Information Capacity of Nerve Cells Using a Frequency Code". However, that paper mentioned explicitly that their result is valid only if "assuming the mean frequency of nerve impulses measures the intensity of a maintained stimulus", without stating that it was the case). Biology never provided proof that firing rate is proportional to the information produced and transmitted (in Shannon's sense: it was a good merit function). The time-aware model [4] identified this "presence or absence" part of neuronal communication as "non-payload information" that neurons use for synchronizing their communication. This theoretical conclusion endorses the former result that "information is carried only in the spike arrival times" [51].
With the assumption that signal is represented by an amplitude (such as voltage or light intensity, "relatively slowly variable") in the transfer medium, the number of allowed signals can be calculated from the condition that the transmission medium cannot have two different amplitudes at the same time. Physics, instead of mathematics, defines channel capacity. The statement that "information theory has fundamental theorems on the maximum information transferrable in a particular communication channel" [31] is true. However, as discussed in connection with Eq (2.3), channel capacity is an externally provided parameter and it depends on the speed of the signal and spatial/temporal length of a single signal. The number of allowed signals per unit of time can be defined by putting signals one after the other until we reach the distance the signal carrier propagates in a unit time. In electric communication, the signal propagation speed is fixed, but the signal frequency can vary. The number of allowed signals is defined by the speed of light divided by the signal's wavelength. A higher frequency means shorter "time duration" , so higher capacity. In neural communication, conduction velocity and spike length are fixed (although the conduction velocity can be different for different communicating pairs and can be fine-tuned during learning). If we use neural signals, the number of allowed signals can be defined as the conduction velocity divided by the spatial length of a spike. Notice that this definition implies that individual signals do not carry further information (in addition to their coded content), their lengths are identical, and the channel does not provide any more possibility to transmit additional information. i.e., we can directly apply Shannon's theory only for signals (including neural signals) satisfying these requirements.
With a perfect sense, MacKay and McCulloch [43] introduced the hypothesis: "In particular, we shall examine the suggestion that an all-or-none binary code using pulses separated by minimal quantized time intervals would be more efficient than a system using the same elements in which the varying time of occurrence of impulses represented the information to be conveyed. "
Given the lack of "detailed theoretization" at their time, furthermore having targeted only to provide limits for the information capacity of neuronal link, MacKay and McCulloch [43] have been very cautious about the operation mode of the brain: "It is considered equally unlikely that its actual mode of operation [of the nervous system] closely resembles either form" (the binary or pulse-interval modulation). In this implicit way, they called attention to the limitations of drawing parallels between biological and electronic operations: the nature-constructed brain should not necessarily use one of the operating modes of the man-constructed systems (it would be a 'technomorf biology'). Although their main result was to provide a rough estimation that the pulse-interval modulation mode transfer can deliver much more information than the binary transfer, for purely efficiency reasons, "it is concluded, not that interval modulation rather than binary modulation 'ought' to be the mode of action of the central nervous system, but that the contrary assumption is unsupported by considerations of efficiency." That is, from the point of view of efficiency of information transmission, they rather supported the idea of the pulse-modulated information transfer. Notice that since spikes can be grouped to form spike packets, the packet parameters offer further possibilities to transmit information.
This opinion became much more established and explicit with the passing years: "The nervous system represents time-dependent signals in sequences of discrete action potentials, or spikes are identical so that information is carried only in the spike arrival times" [34], per Shannon's conclusion. Furthermore, it was pointed out by [52] that in the case of electronic communication "computation that is most efficient in its use of resources is neither analog computation nor digital computation but, rather, a mixture of the two forms". The authors hypothesized that "it is likely that the brain computes in a hybrid fashion and that an underappreciated and important reason for the efficiency of the human brain". Although one cannot directly extrapolate their results, mainly their "cost functions", to neurobiology, the idea deserves attention. One must take care when drawing a parallel with the electronic case because of the differences between the different communication modes and carriers, see [4]; furthermore that the neuron does not "restore" [52] the signal: it issues a new one, based on the result of its computation. Maybe we can consider, from the point of view of efficiency of energy consumption [53], the neural communication as a hybrid communication regime, where the presence of spikes represents a digital channel and the time distribution of Inter-Spike Interval (ISI) represents an analogous channel, without explicitly sharing the information between the two channels? Or, maybe that the digital channel delivers synchronization signals and the analog channel the genuine temporal information?
The charged particles delivered by the mixed electrical/chemical charge transfer mechanism towards the membrane cannot enter because the input conductance is zero. However, the stalled ions create an increasing potential that opens the voltage-controlled ion channels. This phenomenon is formulated as "Synaptic inputs effectively open 'holes' in the membrane'' [21], then the charge in the spike can contribute (through transmitters) to the potential of the membrane. Similarly, when the membrane reaches its threshold potential, synaptic inputs of the neuron get closed, and the waiting charge must be dismissed. These (essential for a biological neuron) gating processes are not modeled electrically. In light of the time-aware model [4,11,12], the operation of a neuron is neither analog nor digital; furthermore, it is also not simply a mixture of two electric modes: it is neuro-logical. The false parallel with electronic circuits is misleading here, too. Our observation is in line with that by [22]: "neurons are sites where information is handled in analog rather than digital form … The digital pulse code of presynaptic fibers is thus converted into an analog process at the junction, only to be reconverted into a digital pulse code again in the axon of the postsynaptic cell. The logical operations are all performed in the analog mode at the synapses." Our model is essentially the one described, for example, in Chapter 3 of [17], except that the gating of charging process of the membrane at the beginning by the synapses and at the end by the membrane are explicitly considered. Furthermore, the operation is cut to an analog "input section" and a digital "output section" as [3,20,22] required, with an internal trigger signal [54] between them. Notice the important addition of our time-aware model that the timing precision of spikes matters, both in receiving and sending spikes. In this discussion, we use the model that a membrane is a simple (although voltage-gated) condenser. It is known, however, that the membrane is not isopotential: the charge delivery process on the surface of the membrane has finite speed and it is vital for the process of "neural computing". Moreover, it is essential contribution to the "neuronal processing time". The finite conduction speed's effect is visible in the form of "transients" [55,56] in the locale voltage gradient of the membrane.
We can calculate the "neuronal processing time" (see also Figure 1) as
(3.1) |
where is the time which neuron needs to collect its potential contributions from its synapses to reach the threshold, is the time (according to [54], it is about 200 s) needed to charge the membrane to its maximum potential, (a fraction of about the same size) is needed to reset the computing unit to be able to process its inputs again, and needed to restore its resting potential. After the period of , the neuron is ready to start a new computation. However, until it reaches its resting potential, in period , it "remembers" its previous spike, so the content of this (time-dependent) "memory" is added to its inputs. After preparing for a new computation, the neuron must wait for the first input's time.
We can identify the biophysically defined sum as the theoretical lower limit for [43] if there is no gap between the adjacent spikes and the membrane reaches its threshold potential instantly. In practice, as published in [43], about 250…300 counts/sec are experienced, showing that needs time. Both and affect and , and they change dynamically as the neuron changes its state (actually, it is a good idea to assume that ). This pinpointing does not significantly influence the calculated rough limiting information rates but explains why the observed computing time distribution needs parameters skewness and kurtosis [35]. It results as the superposition of two distributions [12], and nature requires back its two missing degrees of freedom, so they are misinterpreted as those additional parameters of the forced one distribution.
One more direct experimental evidence underpins that the inter-spike time gap comprises two components: and the charging-related sum of the rest of all other time contributions in Eq (3.1). As the right bottom inset of Figure 1 in [48] displays, when measuring the firing sequence after an external triggering, the standard deviations of the second and third spikes significantly reduce if their difference to the time of the first spike is used as a time reference instead of their absolute time. Using an absolute time base means that the neuron starts its first cycle in a random phase, relative to the time of issuing its own spike, and the second and third spikes relate their time scale to the time of issuing the previous spike. This difference leads to a time "jitter from trial to trial by ~1 ms" in random phase mode. The jitter time originates from the external trigger signal, independent of the synchrony of neural operation. From the point of view of neuronal operation, the measurement begins at a random phase of the neuronal cycle. Subtracting the first spike's time from the second and third spikes' times removes this contribution (the "jitter", presumably a random time having normal distribution) from the corresponding time values and also reduces those time values' standard deviation (performs a "software phase locking"). It is true that "this Self-Information Code is completely intrinsic to neurons themselves, with no need for outside observers to set any reference point such as time zeros of stimulation"[35]. However, the observer can set (unintentionally) that reference point and see its consequences, as we discussed the experiment by [48] in our paper [4].
The abstract neuronal condenser-based time-aware model of neuronal operation is based on the time-aware description of biological computing [4,12], finally introducing the true interpretation of the spatiotemporal behavior of the neural network, which has been missing for so long. We hope that our model "could inspire new ideas about how biological brains work" [57]. All former descriptions of the spatiotemporal behavior missed the vital point that the relevant interaction speed interconnects the spatial and temporal coordinates; although the required mathematical method (the so-called Minkowski-space coordinates) have been made available by [14] and are in use in science (in the theory of special relativity). This new view enables us to explain why research provided contradictory results (depending on the measuring and data evaluation conditions, the theoretical assumptions and hypotheses) and underpin/refute them theoretically.
Below, we slightly reformulate the hypotheses of the classic paper [43] in terms of our model-based notions [4]. Furthermore, we point out that the information carrier matters. The two works together point much behind the original goal of providing rough limits for neuronal links' information transfer capacity. They also suggest a strong hypothesis (underpinned by neurophysiology, physics, the economy of information transfer, energy consumption, communication and information theory) for setting up a model that can be validated experimentally and enables an understanding of its abstract neurophysiological details theoretically.
Information is a mathematical abstraction and it cannot be measured directly. The claim that "actual information transfer has been measured directly, without any assumptions about which stimulus parameters are encoded" [31] suggests the fallacy that information should not have a physical carrier (such as measurable action potential or integrated charge, or inter-spike interval), furthermore that the abstract information could be measured independently from its hypothesized carrier. Attempts originating in this idea, such as constructing "a model-free measure of effective connectivity" [58] or tracking causality [59], can mislead brain research and culminate, among others, in the "implosion of a billion-euro brain model" [60]. It was noted: "too little was known about the different types of neuron in the brain and how they were wired". We can add: too little was known about how much the temporal operation matters. In reality, if we measure time, voltage or current (etc.), we must hypothesize how that measurement data relates to the information it conveys.
To produce information, Shannon's theory required to derive a merit function that takes measured data (such as time difference, element of a histogram, voltage level) as input argument(s) and delivers information as its result. Assuming that the length of the inter-spike intervals (analog information) delivers the neural information, an order of magnitude higher information transmission rate can be achieved, than assuming the digital form of information transfer (in other words, counting the spikes or measuring firing rate). Later research by [52] hypothesized that in the case of electronic implementation, the mixed analog/digital computation is most efficient. In the case of biological implementation, the digital portion only carries non-payload information and the payload information (and its limiting bandwidth) shall be calculated from the analog portion of the transmitted message [4]. Scrutinizing the details of the operation of the abstract neuron revealed that the information carrier in neural information transfer is the length of the interval between the spikes (or groups of spikes), in contrast with electronic communication, where the signal amplitude carries the information. This difference alone makes using the classic information theory in biology irrelevant, at least directly.
Shannon [3] liberally enabled coding various pieces of information into the messages: "An information source produces a message or sequence of messages … The message may be of various types: … (c) A function of time and other variables". The transmitter neuron performs coding of information, and in the communication channel only the signal is accessible. As discussed in [4], transferred messages in all communications have multiple components, including unintended time and intended information content. Although, in principle, the observer's tools can emulate the functionality of a receiver (receive signals and decode them into information), the multiple inputs of neuron force the observer to combine signals from different transfer channels. It is impossible to "perform the inverse operation of that done by the transmitter" [3] without knowing the details of the operation, including which physical characteristics of the signal carry the information. Even the best possible quantization (see, for example, [61]) cannot circumvent the problem of defining what physical characteristics are thought to convey information. The decoding process can deliver information with high precision, the question is its relation to the information that the transmitter coded into the signal.
Using the notions of von Neumann [20], the time-aware model divides an abstract neuron into an "input section" and an "output section". Similarly, Shannon's theory requires a "receiver" and a "transmitter". The "input section" operation ends when the neuron's membrane reaches its threshold potential. That portion closely resembles the operation of a (voltage-gated) analog circuit. Reaching that potential triggers the operation of the "output section". The spike parameters do not depend on the signal received from the "input section" ("the spikes are identical" [34]): it shows a close resemblance with a digital circuit. Notice that the operations of the "input section" and the "output section" overlap (the two elementary neuronal operations can run in parallel): the spike preparation in the "output section" can begin independently while the "input section" is still in the (absolute) refractory period. Given that their ion channels are closed, the synaptic inputs cannot contribute anymore to the result of the neuronal computation. However, the non-equilibrium membrane potential enables a "memory effect" (see [35]) when at the end of the absolute refractory period the synaptic inputs get enabled again.
The classic analysis also noticed that "there is, however, another variable parameter of our neuronal signal. The time interval between successive impulses can vary, so that any given interval represents a choice out of a certain range of possible pulse positions on the time axis."
In analogy with Shannon's case, the classic paper assumed one input only. Our model considers that having more than one synaptic input, the membrane reaches its threshold potential at an earlier time so that the computing time is also variable, although the spike length is fixed. The synaptic calculation takes place in a time window ( in Figure 1), which is opened upon the arrival of the first spike and closed upon reaching the threshold potential. Furthermore, the time-aware model assumes a "time window" for the idle activity ( in Figure 1), too. This time window is opened upon the end of the refractory period and closed upon the arrival of the first spike. Per definitionem, these two windows do not overlap, and there is no gap between them: they are sequential. The computing unit is busy in period . For computation details, see [12].
Given that the operation of the synapse is voltage-gated, and the lead potential of the next spike is much higher than the trail potential of the previous spike; the idle time between spikes is not necessarily higher than zero, even for one single synaptic input. To approach zero inter-spike time, the next spike must arrive from the network immediately after the neuron has finished its refractory time. The too-early spikes cannot open the closed voltage-gated ion channels; the later ones may be delayed concerning the earliest reasonable arrival time.
There is a third time window (), opened by the end of the charge-up period and closed at the time when the membrane would be restored if no input spike is received (while it is open, the memory is active). This window overlaps with the first window.
Our approach suggests that a conveyed message comprises both a digital component (presence; used for synchronization) and an analog component (the inter-spike timings), which explains that under different conditions, including the point of view of the experimenter (what feature of the signal is hypothesized to be the merit function of the conveyed information), one of the two components may dominate. The false parallel with electronic communication might mislead researchers again.
One must be careful when extrapolating results derived for a single "neuronal link" to a set of neurons. If we consider that the number of input spikes carries the information, it is typical that several spikes arrive at a neuron, and only one spike is produced: "A single neuron may receive inputs from up to 15,000–20,000 neurons and may transmit a signal to 40,000–60,000 other neurons" [62]. "In the terminology of communication theory and information theory, [a neuron] is a multiaccess, partially degraded broadcast channel that performs computations on data received at thousands of input terminals and transmits information to thousands of output terminals by means of a time-continuous version of pulse position. Moreover, [a neuron] engages in an extreme form of network coding; it does not store or forward the information it receives but rather fastidiously computes a certain functional of the union of all its input spike trains which it then conveys to a multiplicity of select recipients" [42]. In this way, some "information loss" surely takes place. If we consider that the ISI carries information and a neuron takes into consideration in its "computing" only the spikes which arrive within an appropriate time window, some information is lost again. Even the result of the computation, a single output spike, may be issued before either of the input spikes was entirely delivered. One more reason why the notion of information should be revisited.
According to Levy's measurements, "communication consumes 35 times more energy than computation in the human cortex" [63]. Their result also suggests that the role of transferring data must not be neglected in neural systems; neither from the point of view of the needed power nor from the point of view of the time needed to carry out the operation. An interesting parallel with electronic computing is that in the latter systems [64], the same ratio is about 5, mainly because of the increased power consumption of processors due to the increased dispersion [11] and the data delivery within the processor.
It is well known in technical computing that the limit of operating performance of composite systems depends on the limits of operating characteristics of its components [66]. Furthermore, the lowest performance limit of the components also limits the composite system's performance. An example is (see Figure 2) that the technical development can cause a step-like change the limiting performance of the individual components of supercomputers. Consequently, another component will take over the role of the performance-limiting component.
We can consider the communication channel of neurons, the axon, similarly, as such a composite system where multiple mechanisms define the capacity of information transfer. As discussed above, the assumed operation limits the maximum information transmission speed: the digital and the analog operation assumed in the model provide different information transfer limits. We can find a parallel with the technical implementation's limiting "roofline" effect.
In the model used by Shannon, "the channel is merely the medium used to transmit the signal from transmitter to receiver" [3], i.e., a passive entity. This notion is also quite different in biology: the axon actively participates in transferring information. The axon transfers messages in the form of "transversal current" (ions move perpendicularly to the direction of the message), actively pumping ions through its membrane. Most of the energy consumption of neural signal processing is needed for this activity, and that energy needs to be produced locally by its distributed local resource (ATP) [63], providing a "power bandwidth" roofline. Under normal conditions and a healthy neural network, the local density of energy production is presumably slightly above the maximum the transmitted information might need, i.e., only the information bandwidth limits the information transmission. If an artificial excitation produces information density above the maximum level the axon can transmit (for a detailed discussion see [67] and Section 5.3 in [4]); or some illness decreases the density of energy production, the alternative "roofline" (power bandwidth) can also be observed in biology: either the information bandwidth or the power bandwidth dominates, depending on the actual conditions.
We succeeded to demonstrate that neuronal communication applies very special communication and operation modes, where (in terms of technical computing) mixed analog/digital signals deliver the intended information. Our abstract model is in line with the theoretical statement that neuronal network "engages in an extreme form of network coding" [42], at the single spike level; furthermore, that single spikes cannot convey information. At the next level, "it is widely appreciated that neuronal networks exhibit patterns of bursting and synchrony that are not captured by simple measures such as average spike rate. These patterns can encode information. However, methods for quantifying bursting and synchrony are not agreed upon and can be confounded with spike rate measures."[68] Our time-aware model demonstrated that more synaptic inputs enable more patterns in the transmitted signals, including spike groups and trains of groups, all with individual timing relations that enable extra information coding. We found that spikes form packets, which arrive in trains (and trains begin with a burst to maintain synchrony), furthermore different upstream neurons may fire at different times. All these effects introduce further potential timing information (much more than the technology-borrowed idea [43] of a simple time distribution suggests); a hypothesis that should be tested experimentally using carefully designed dedicated experiments and evaluation procedures. One must consider all their timing relations.
As [69] called attention to, "Warren Weaver in his prologue to the book version of Shannon's paper reminds us that Shannon's work was entitled 'A Mathematical Theory of Communication'. It's powerful results concern the limits of effective communication of signals and said little about what was being communicated. For example, as we have discovered, trying to discern the information carried by a signal is not addressed by what has become 'information theory.' Weaver said that future research must be concerned with semantics: determining what the information is and how best to determine the intended meaning". We did the first steps: reinterpreted the notion of neural information, its transfer, storage and processing; furthermore the biological meaning of Shannon's communication model. Our forthcoming paper will describe a practical automated dynamic analysis (as opposed to the commonly used static one, based on firing rates) of neurons' temporal behavior, its algorithms, first results and conclusions.
The classic analysis [43] revealed that the assumed operating mode of neurons strongly affects the limiting information capacity of their transfer lines. The time-aware model revealed that the information is shared between the "digital" channel (a piece of presence information, which is assumed to be non-payload synchronization information only), and the "analog" channel, which is timing information. That is, measuring the payload information transferred in a neuronal operation by counting the transferred spikes leads to a loss of information: the actual information content is dismissed and replaced by a false one. However, if the signal in the analog channel delivers only a low number of bits (comparable to the single bit in the "digital" channel), mismatching those different channels can remain hidden (obscured) because measuring the information in either channel (the single presence bit and the intended communication) delivers approximately the same bit rates. In other cases using the neural information theory demonstratively fails because the information measuring method was wrong, and the information conveyed is much more than the measured one synchrony bit per spike.
The experimenter's point of view (i.e., whether the information was delivered on the analog or digital channel) defines their measurement method. In Shannon's picture, losing information during regular operation between the transmitter and the receiver automatically concludes that either the transmission model or the information merit function on either side was wrong. On what the experimenter's point of view means, an excellent example is given in paper by [70]. On the one side, the claims, "To provide information about dynamic sensory stimuli, the pattern of action potentials in spiking neurons must be variable. To ensure reliability, these variations must be related, reproducibly, to the stimulus" are clear and true. However, on the other side, "each impulse, simply by its presence or absence … could provide one 'bit' of selective information" is wrong, see above.
The authors see that "constant-velocity motion produces irregular spike firing patterns, and spike counts typically have a variance comparable to the mean". In statistics, a large variance means that the numbers in a set are far from the mean and each other. In other words, in the actual case of a constant velocity, there is a very weak (if any) dependence between the number of spikes and the motion, clearly showing that some other parameter of the transferred signal delivers the information. The experimenters noticed this but wanted to think that the number of spikes delivered the information. Because of this reason, they looked for some other "more natural" case: "But more natural, time-dependent input signals yield patterns of spikes that are much more reproducible". (Notice that "pattern", i.e., the temporal distance of spikes and spike packets may change with the stimulation.) They decided what they wanted to hypothesize and found the experimental data that underpins their hypothesis in a "much more reproducible" way. Presumably, in the "much more reproducible" scenario, the number of bits per spike in the analog component is much slighter than in the "less natural" case, so using a wrong merit function for the information meets experimenters' (false) expectation much better. Nature must behave "more naturally" to produce spike trains to demonstrate the researcher's hypothesis. Despite all of this, we can agree with the authors' conclusion: "These observations on the encoding of naturalistic stimuli cannot be understood by extrapolation from quasi-static experiments, nor do such experiments provide any hint of the timing and counting accuracy that the brain can achieve." [70]
The fallacy of selecting a wrong merit function leads directly to the conclusion [71] that "energy efficiency [of neural transfer] falls by well over 90%" (many other similar examples could be cited, but this one shows the mistake explicitly). By defining their measuring method, "We calculated the firing rate of the spiking neuron model", and measured "spikes/s" [51,70] (assuming that the neuron is a repeater and the spike carries on/off information) they draw a false parallel with electronic circuits.
Experimental data as "The highest information rate attained by the spiking neuron model was 235 bits/s, whereas the graded neuron model attained information rates of 2240 bits/s" seem to support their published result. (Recall that both values match the ranges estimated by MacKay and McCulloch when using "digital" and "frequency modulation", respectively, hypothesis.) Their conclusion that "action potentials can encode less information per unit energy, proving metabolically inefficient [transmission]" can hardly be interpreted in terms of the overall metabolic efficiency of the biology of the evolution. The misinterpretation of neural information slightly degrades the value of otherwise crucial measured data on the "metabolic cost of neural information" [53].
The limitation of information transmission speed is closely related to how the information is represented during the operation of the nervous system. MacKay and McCulloch set the correct information limits for the capacity of a neuronal link early. However, –in the absence of a proper neuronal model– they did so conditionally, i.e., deriving two limits for cases if the operation of the brain is digital and if it is analog. Although they implicitly suggested the latter operating mode, i.e., that the timing of spikes carries the information on neural operation, only later research made clear that counting the spikes (a.k.a. firing rate) is senseless and misleading. The correct hints that the inter-spike timing rather than the spiking frequency could be the primary source of information in neural communication has been underpinned from several points of view. Our paper pointed out that the neural information transfer is neither digital nor analog and that the neuro-logical (resembled mixed analog/digital) mode can be more efficient, so it can probably provide higher limiting bandwidth. However, its value can be estimated only after understanding how neurons code information to transmitted signals.
Although it is not yet entirely understood how timing represents neural information, neuroscience should stop thinking that the brain is a complex electronic circuit implemented in chemical solutions and it is sending digital messages. Continuing to do so leads to conclusions such as "observations on the encoding of naturalistic stimuli cannot be understood by extrapolation from quasi-static experiments" [70] at the level of understanding elementary notions and operations, "energy efficiency [of neural transfer] falls by well over 90%" [71] at the level of processing neural information, and "studies on processes like plasticity, learning and development … are outside our reach" [72,73] at the highest level of neuronal operation. Although –using an appropriate interpretation given by [12] of the virtues– the brain is computing and communicating, the careless parallels with electronics block understanding of neural information processing and making neuroscience quantitative; furthermore, the incompletely understood abstract biological notions hinder developing technical fields such as biology-mimicking artificial networks [74] (including brain simulation [75]) and biomorph architectures [76,77].
Declarations of interest: none.
[1] |
W. S. McCulloch, W. Pitts, A logical calculus of the ideas immanent in nervous activity, J. Bull. Math. Biophys, 5 (1943), 115–133. https://doi.org/10.1007/BF02478259 doi: 10.1007/BF02478259
![]() |
[2] |
W. Pitts, W. S. McCulloch, How we know universals the perception of auditory and visual forms, J. Bull. Math. Biophys, 9 (1947), 127–147. https://doi.org/10.1007/BF02478291 doi: 10.1007/BF02478291
![]() |
[3] |
C. E. Shannon, A mathematical theory of communication, Bell System Techn. J., 27 (1948), 379–423. https://doi.org/10.1002/j.1538-7305.1948.tb01338.x doi: 10.1002/j.1538-7305.1948.tb01338.x
![]() |
[4] |
J. Végh, Á. J. Berki, Towards generalizing the information theory for neural communication, Entropy, 24 (2022), 1086. https://doi.org/10.3390/e24081086 doi: 10.3390/e24081086
![]() |
[5] | L. Nizami, Information theory is abused in neuroscience, Cybern. Human Knowing, 26 (2019), 47–97. |
[6] | C. E. Shannon, The Bandwagon, IRE Trans. Inf. Theory, 2 (1956), 3. |
[7] | D. H. Johnson, Information theory and neuroscience: Why is the intersection so small?, in 2008 IEEE Information Theory Workshop, (2008), 104–108. https://doi.org/10.1109/ITW.2008.4578631 |
[8] |
M. D. McDonnell, S. Ikeda, J. H. Manton, An introductory review of information theory in the context of computational neuroscience, Biol. Cybern., 105 (2011). https://doi.org/10.1007/s00422-011-0451-9 doi: 10.1007/s00422-011-0451-9
![]() |
[9] | J. N. Carbone, J. A. Crowder, The great migration: Information content to knowledge using cognition based frameworks, in Biomedical Engineering, Springer, New York, (2011), 17–46. https://doi.org/10.1007/978-1-4614-0116-2_2 |
[10] |
J. Végh, Why do we need to Introduce Temporal Behavior in both Modern Science and Modern Computing, Global J. Comput. Sci. Technol., 20 (2020), 13–29. https://doi.org/10.34257/GJCSTAVOL20IS1PG13 doi: 10.34257/GJCSTAVOL20IS1PG13
![]() |
[11] |
J. Végh, Revising the classic computing paradigm and its technological implementations, Informatics, 8 (2021). https://doi.org/10.3390/informatics8040071 doi: 10.3390/informatics8040071
![]() |
[12] |
J. Végh, Á. J. Berki, On the role of speed in technological and biological information transfer for computations, Acta Biotheor., 70 (2022), 26. https://doi.org/10.1007/s10441-022-09450-6 doi: 10.1007/s10441-022-09450-6
![]() |
[13] | G. Buzsáki, J. Végh, Space, Time and Memory, 1st edition, Oxford University Press, in print, 2023. |
[14] | H. Minkowski, Die Grundgleichungen für die electromagnetischen Vorgänge in bewegten Körpern, Nachr. Königl., Ges. der Wissenschaften zu Göttingen (in German), (1908), 53–111. |
[15] |
L. Pyenson, Hermann Minkowski and Einstein's special theory of relativity, Arch. Hist. Exact Sci., 17 (1977), 71–95. https://doi.org/10.1007/BF00348403 doi: 10.1007/BF00348403
![]() |
[16] |
J. M. Gomes, C. Bédard, S. Valtcheva, M. Nelson, V. Khokhlova, P. Pouget, et al., Intracellular impedance measurements reveal non-ohmic properties of the extracellular medium around neurons, Biophys. J., 110 (2016), 234–246. https://doi.org/10.1016/j.bpj.2015.11.019 doi: 10.1016/j.bpj.2015.11.019
![]() |
[17] | D. Johnston, S. M. S. Wu, Foundations of Cellular Neurophysiology, Massachusetts Institute of Technology, 1995. |
[18] | C. Koch, Biophysics of Computation, Oxford University Press, 1999. |
[19] |
B. Podobnik, M. Jusup, Z. Tiganj, W. X. Wang, J. M. Buld, H. E. Stanley, Biological conservation law as an emerging functionality in dynamical neuronal networks, PNAS, 45 (2017), 11826–11831. https://doi.org/10.1073/pnas.1705704114 doi: 10.1073/pnas.1705704114
![]() |
[20] |
J. von Neumann, First draft of a report on the EDVAC, IEEE Ann. Hist. Comput., 15 (1993), 27–75. https://doi.org/10.1109/85.238389 doi: 10.1109/85.238389
![]() |
[21] | C. Koch, T. A. Poggio, A theoretical analysis of electrical properties of spines, Proc. R. Soc. Ser. B Biol. Sci., 218 (1983), 455–477. |
[22] | G. Somjen, Sensory Coding in the Mammalian Nervous System, New York: Meredith Corporation, 1972. |
[23] |
C. Fiorillo, J. Kim, S. Hong, The meaning of spikes from the neuron's point of view: predictive homeostasis generates the appearance of randomness, Front. Comput. Neurosci., 8 (2014). https://doi.org/10.3389/fncom.2014.00049 doi: 10.3389/fncom.2014.00049
![]() |
[24] |
T. J. Sejnowski, The computer and the brain revisited, IEEE Ann. History of Computing, 11 (1989), 197–201. https://doi.org/10.1109/MAHC.1989.10028 doi: 10.1109/MAHC.1989.10028
![]() |
[25] | D. Tsafrir, The context-switch overhead inflicted by hardware interrupts (and the enigma of do-nothing loops), in Proceedings of the 2007 workshop on Experimental computer science, ACM, New York, USA, (2007), 4–es. |
[26] | F. M. David, J. C. Carlyle, R. H. Campbell, Context switch overheads for Linux on ARM platforms, in Proceedings of the 2007 workshop on Experimental computer science, ACM, New York, USA, (2007), 3–es. http://doi.acm.org/10.1145/1281700.1281703 |
[27] | J. von Neumann, The Computer and the Brain (The Silliman Memorial Lectures Series), New Haven, Yale University Press, 2012. |
[28] |
P. Mitra, Fitting elephants in modern machine learning by statistically consistent interpolation, Nat. Mach. Intell., 3 (2021), 378–386. https://doi.org/10.1038/s42256-021-00345-8 doi: 10.1038/s42256-021-00345-8
![]() |
[29] | R. P. Feynman, Feynman Lectures on Computation, CRC Press, 2018. |
[30] |
Y. A. Cengel, On entropy, information, and conservation of information, Entropy, 23 (2021), 779. https://doi.org/10.3390/e23060779 doi: 10.3390/e23060779
![]() |
[31] |
A. Borst, F. E. Theunissen, Information theory and neural coding, Nat. Neurosci., 2 (1999), 947–957. https://doi.org/10.1038/14731 doi: 10.1038/14731
![]() |
[32] |
R. Brette, Is coding a relevant metaphor for the brain, Behav. Brain Sci., 42 (2018), e215. https://doi.org/10.1017/S0140525X19000049 doi: 10.1017/S0140525X19000049
![]() |
[33] |
N. Brenner, S. P. Strong, R. Koberle, W. Bialek, R. R. de Ruyter van Steveninck, Synergy in a neural code, Neural Comput., 12 (2000), 1531–1552. https://doi.org/10.1162/089976600300015259 doi: 10.1162/089976600300015259
![]() |
[34] | S. P. Strong, R. R. de Ruyter van Steveninck, W. Bialek, R. Koberle, On the application of information theory to neural spike trains, Neural Comput., 1998 (1998), 621–632. |
[35] |
M. Li, J. Z. Tsien, Neural code—neural self-information theory on how cell-assembly code rises from spike time and neuronal variability, Front. Cell. Neurosci., 11 (2017). https://doi.org/10.3389/fncel.2017.00236 doi: 10.3389/fncel.2017.00236
![]() |
[36] | I. Csiszár, J. Körner, Information Theory: Coding Theorems for Discrete Memoryless Systems, Cambridge Universiy Press, 2011. |
[37] |
C. Wilson, Up and down states, Scholarpedia J., 6 (2008), 1410. https://doi.org/10.4249/scholarpedia.1410 doi: 10.4249/scholarpedia.1410
![]() |
[38] | D. Levenstein, G. Girardeau, J. Gornet, A. Grosmark, R. Huszár, A. Peyrache, et al., Distinct ground state and activated state modes of spiking in forebrain neurons, bioRxiv, 2021. https://doi.org/10.1101/2021.09.20.461152 |
[39] |
S. Eddy, What is a hidden markov model, Nat. Biotechnol., 22 (2004), 1315–1316. https://doi.org/10.1038/nbt1004-1315 doi: 10.1038/nbt1004-1315
![]() |
[40] |
S. B. Laughlin, Energy as a constraint on the coding and processing of sensory information, Curr. Opin. Neurobiol., 11 (2001), 475–480. https://doi.org/10.1016/S0959-4388(00)00237-3 doi: 10.1016/S0959-4388(00)00237-3
![]() |
[41] |
H. Barlow, Redundancy reduction revisited, Network: Comput. Neural Syst., 12 (2001), 241. https://doi.org/10.1088/0954-898X/12/3/301 doi: 10.1088/0954-898X/12/3/301
![]() |
[42] |
T. Berger, W. B. Levy, A mathematical theory of energy efficient neural computation and communication, IEEE Trans. Inf. Theory, 56 (2010), 852–874. https://doi.org/10.1109/TIT.2009.2037089 doi: 10.1109/TIT.2009.2037089
![]() |
[43] |
D. M. MacKay, W. S. McCulloch, The limiting information capacity of a neuronal link, Bull. Math. Biophys., 14 (1952), 127–135. https://doi.org/10.1007/BF02477711 doi: 10.1007/BF02477711
![]() |
[44] | F. Rieke, D. Warland, W. Bialek, Spikes: Exploring the Neural Code, 2nd edition, The MIT Press, 1997. |
[45] | J. V. Stone, Principles of Neural Information Theory, Sebtel Press, Sheffield, UK, 2018. |
[46] | P. Sterling, S. Laughlin, Principles of Neural Design, 1st edition, The MIT Press, 2017. |
[47] | P. M. DiLorenzo, J. D. Victor, Spike Timing: Mechanisms and Function, 1st edition, CRC Press, 2013. |
[48] |
I. Nemenman, G. D. Lewen, W. Bialek, R. R. de Ruyter van Steveninck, Neural coding of natural stimuli: Information at sub-millisecond resolution, PLoS Comput. Biol., 4 (2008), 1–12. https://doi.org/10.1371/journal.pcbi.1000025 doi: 10.1371/journal.pcbi.1000025
![]() |
[49] |
A. Losonczy, J. Magee, Integrative properties of radial oblique dendrites in hippocampal CA1 pyramidal neurons, Neuron, 50 (2006), 291–307. https://doi.org/10.1016/j.neuron.2006.03.016 doi: 10.1016/j.neuron.2006.03.016
![]() |
[50] |
R. B. Stein, The information capacity of nerve cells using a frequency code, Biophys. J., 6 (1967), 797–826. https://doi.org/10.1016/S0006-3495(67)86623-2 doi: 10.1016/S0006-3495(67)86623-2
![]() |
[51] |
S. P. Strong, R. Koberle, R. R. de Ruyter van Steveninck, W. Bialek, Entropy and information in neural spike trains, Phys. Rev. Lett., 80 (1998), 197–200. https://doi.org/10.1103/PhysRevLett.80.197 doi: 10.1103/PhysRevLett.80.197
![]() |
[52] |
R. Sarpeshkar, Analog versus digital: Extrapolating from electronics to neurobiology, Neural Comput., 10 (1998), 1601–1638. https://doi.org/10.1162/089976698300017052 doi: 10.1162/089976698300017052
![]() |
[53] |
S. B. Laughlin, R. R. de Ruyter van Steveninck, J. C. Anderson, The metabolic cost of neural information, Nat. Neurosci., 1 (1998), 36–41. https://doi.org/10.1038/236 doi: 10.1038/236
![]() |
[54] |
P. Singh, P. Sahoo, K. Saxena, J. S. Manna, K. Ray, S. Kanad, et al., Cytoskeletal filaments deep inside a neuron are not silent: They regulate the precise timing of nerve spikes using a pair of vortices, Symmetry, 13 (2021). https://doi.org/10.3390/sym13050821 doi: 10.3390/sym13050821
![]() |
[55] |
M. Stemmler, C. Koch, How voltage-dependent conductances can adapt to maximize the information encoded by neuronal firing rate, Nat. Neurosci., 2 (1999), 521–527. https://doi.org/10.1038/9173 doi: 10.1038/9173
![]() |
[56] |
P. Khorsand, F. Chance, Transient responses to rapid changes in mean and variance in spiking models, PLoS ONE, 3 (208), e3786. doi.org/10.1371/journal.pone.0003786 doi: 10.1371/journal.pone.0003786
![]() |
[57] |
K. Kar, S. Kornblith, E. Fedorenko, Interpretability of artificial neural network models in artificial intelligence versus neuroscience, Nature Mach. Intell., 4 (2022), 1065–1067. https://doi.org/10.1038/s42256-022-00592-3 doi: 10.1038/s42256-022-00592-3
![]() |
[58] |
R. Vicente, M. Wibral, M. Lindner, G. Pipa, Transfer entropy—a model-free measure of effective connectivity for the neurosciences, J. Comput. Neurosci., 30 (2011), 45–67. https://doi.org/10.1007/s10827-010-0262-3 doi: 10.1007/s10827-010-0262-3
![]() |
[59] |
K. Hlaváčková-Schindler, M. Paluš, M. Vejmelka, J. Bhattacharya, Causality detection based on information-theoretic approaches in time series analysis, Phys. Rep., 441 (2007), 1–46. https://doi.org/10.1016/j.physrep.2006.12.004 doi: 10.1016/j.physrep.2006.12.004
![]() |
[60] |
A. Abbott, Documentary follows implosion of billion-euro brain project, Nature, 588 (2020), 215–216. https://doi.org/10.1038/d41586-020-03462-3 doi: 10.1038/d41586-020-03462-3
![]() |
[61] |
A. G. Dimitrov, J. P. Miller, Neural coding and decoding: communication channels and quantization, Network: Comput. Neural Syst., 12 (2001), 441. https://doi.org/10.1088/0954-898X/12/4/303 doi: 10.1088/0954-898X/12/4/303
![]() |
[62] | G. M. Shepherd, The Synaptic Organization of the Brain, 5 edition, Oxford Academic, New York, 2006. |
[63] |
W. B. Levy, V. G. Calvert, Communication consumes 35 times more energy than computation in the human cortex, but both costs are needed to predict synapse number, Proc. Nat. Acad. Sci., 118 (2021), e2008173118. https://doi.org/10.1073/pnas.2008173118 doi: 10.1073/pnas.2008173118
![]() |
[64] | H. Simon, Why we need Exascale and why we won't get there by 2020, in Conference: AASCTS2: Exascale Radioastronomy Meeting, 2014. Accesse date: Oct. 23, 2021. Available from: https://www.researchgate.net/publication/261879110_Why_we_need_Exascale_and_why_we_won't_get_there_by_2020. |
[65] |
J. Végh, Finally, how many efficiencies the supercomputers have, J. Supercomput., 76 (2020), 9430–9455. https://doi.org/10.1007/s11227-020-03210-4 doi: 10.1007/s11227-020-03210-4
![]() |
[66] |
S. Williams, A. Waterman, D. Patterson, Roofline: An insightful visual performance model for multicore architectures, Commun. ACM, 52 (2009), 65–76. https://doi.org/10.1145/1498765.1498785 doi: 10.1145/1498765.1498785
![]() |
[67] |
F. Zeldenrust, S. de Knecht, W. J. Wadman, S. Denève, B. Gutkin, Estimating the information extracted by a single spiking neuron from a continuous input time series, Front. Comput. Neurosci., 11 (2017), 49. https://doi.org/10.3389/fncom.2017.00049 doi: 10.3389/fncom.2017.00049
![]() |
[68] |
L. Eisenman, C. Emnett, J. Mohan, C. Zorumski, S. Mennerick, Quantification of bursting and synchrony in cultured hippocampal neurons, J. Neurophysiol., 114 (2015). https://doi.org/10.1152/jn.00079.2015 doi: 10.1152/jn.00079.2015
![]() |
[69] | D. H. Johnson, Dialogue Concerning Neural Coding and Information Theory, 2003. Available from: http://www.ece.rice.edu/dhj/dialog.pdf. |
[70] |
R. R. de Ruyter van Steveninck, G. D. Lewen, S. P. Strong, R. Koberle, W. Bialek, Reproducibility and variability in neural spike trains, Science, 275 (1997), 1805–1808. https://doi.org/10.1126/science.275.5307.1805 doi: 10.1126/science.275.5307.1805
![]() |
[71] |
B. Sengupta, S. Laughlin, J. Niven, Consequences of converting graded to action potentials upon neural information coding and energy efficiency, PLoS Comput. Biol., 1 (2014). https://doi.org/10.1371/journal.pcbi.1003439 doi: 10.1371/journal.pcbi.1003439
![]() |
[72] |
S. J. van Albada, A. G. Rowley, J. Senk, M. Hopkins, M. Schmidt, A. B. Stokes, et al., Performance comparison of the digital neuromorphic hardware spiNNaker and the neural network simulation software NEST for a full-scale cortical microcircuit model, Front. Neurosci., 12 (2018), 291. https://doi.org/10.3389/fnins.2018.00291 doi: 10.3389/fnins.2018.00291
![]() |
[73] |
J. Végh, How Amdahl's Law limits performance of large artificial neural networks, Brain Inf., 6 (2019), 1–11. https://doi.org/10.1186/s40708-019-0097-2 doi: 10.1186/s40708-019-0097-2
![]() |
[74] |
J. Végh, Which scaling rule applies to Artificial Neural Networks, Neural Comput. Appl., 33 (2021), 16847–16864. https://doi.org/10.1007/s00521-021-06456-y doi: 10.1007/s00521-021-06456-y
![]() |
[75] | Human Brain Project, E. Human Brain Project, 2018. Available from: https://www.humanbrainproject.eu/en/. |
[76] |
A. Mehonic, A. Kenyon, Brain-inspired computing needs a master plan, Nature, 604 (2022), 255–260. https://doi.org/10.1038/s41586-021-04362-w doi: 10.1038/s41586-021-04362-w
![]() |
[77] |
D. Markovic, A. Mizrahi, D. Querlioz, J. Grollier, Physics for neuromorphic computing, Nat. Rev. Phys., 2 (2020), 499–510. https://doi.org/10.1038/s42254-020-0208-2 doi: 10.1038/s42254-020-0208-2
![]() |