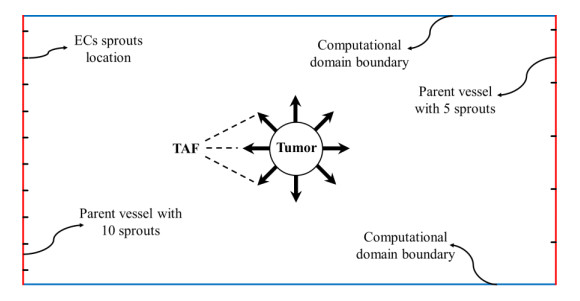
Anti-angiogenesis as a treatment strategy for normalizing the microvascular network of tumors is of great interest among researchers, especially in combination with chemotherapy or radiotherapy. According to the vital role that angiogenesis plays in tumor growth and in exposing the tumor to therapeutic agents, this work develops a mathematical framework to study the influence of angiostatin, a plasminogen fragment that shows the anti-angiogenic function, in the evolutionary behavior of tumor-induced angiogenesis. Angiostatin-induced microvascular network reformation is investigated in a two-dimensional space by considering two parent vessels around a circular tumor by a modified discrete angiogenesis model in different tumor sizes. The effects of imposing modifications on the existing model, i.e., the matrix-degrading enzyme effect, proliferation and death of endothelial cells, matrix density function, and a more realistic chemotactic function, are investigated in this study. Results show a decrease in microvascular density in response to the angiostatin. A functional relationship exists between angiostatin's ability to normalize the capillary network and tumor size or progression stage, such that capillary density decreases by 55%, 41%, 24%, and 13% in tumors with a non-dimensional radius of 0.4, 0.3, 0.2, and 0.1, respectively, after angiostatin administration.
Citation: Mahya Mohammadi, M. Soltani, Cyrus Aghanajafi, Mohammad Kohandel. Investigation of the evolution of tumor-induced microvascular network under the inhibitory effect of anti-angiogenic factor, angiostatin: A mathematical study[J]. Mathematical Biosciences and Engineering, 2023, 20(3): 5448-5480. doi: 10.3934/mbe.2023252
[1] | Austin Baird, Laura Oelsner, Charles Fisher, Matt Witte, My Huynh . A multiscale computational model of angiogenesis after traumatic brain injury, investigating the role location plays in volumetric recovery. Mathematical Biosciences and Engineering, 2021, 18(4): 3227-3257. doi: 10.3934/mbe.2021161 |
[2] | John D. Nagy, Dieter Armbruster . Evolution of uncontrolled proliferation and the angiogenic switch in cancer. Mathematical Biosciences and Engineering, 2012, 9(4): 843-876. doi: 10.3934/mbe.2012.9.843 |
[3] | Urszula Ledzewicz, Helmut Maurer, Heinz Schättler . Optimal and suboptimal protocols for a mathematical model for tumor anti-angiogenesis in combination with chemotherapy. Mathematical Biosciences and Engineering, 2011, 8(2): 307-323. doi: 10.3934/mbe.2011.8.307 |
[4] | Wencong Zhang, Yuxi Tao, Zhanyao Huang, Yue Li, Yingjia Chen, Tengfei Song, Xiangyuan Ma, Yaqin Zhang . Multi-phase features interaction transformer network for liver tumor segmentation and microvascular invasion assessment in contrast-enhanced CT. Mathematical Biosciences and Engineering, 2024, 21(4): 5735-5761. doi: 10.3934/mbe.2024253 |
[5] | Urszula Foryś, Yuri Kheifetz, Yuri Kogan . Critical-Point Analysis For Three-Variable Cancer Angiogenesis Models. Mathematical Biosciences and Engineering, 2005, 2(3): 511-525. doi: 10.3934/mbe.2005.2.511 |
[6] | Luis L. Bonilla, Vincenzo Capasso, Mariano Alvaro, Manuel Carretero, Filippo Terragni . On the mathematical modelling of tumor-induced angiogenesis. Mathematical Biosciences and Engineering, 2017, 14(1): 45-66. doi: 10.3934/mbe.2017004 |
[7] | Heinz Schättler, Urszula Ledzewicz, Benjamin Cardwell . Robustness of optimal controls for a class of mathematical models for tumor anti-angiogenesis. Mathematical Biosciences and Engineering, 2011, 8(2): 355-369. doi: 10.3934/mbe.2011.8.355 |
[8] | Cristian Morales-Rodrigo . A therapy inactivating the tumor angiogenic factors. Mathematical Biosciences and Engineering, 2013, 10(1): 185-198. doi: 10.3934/mbe.2013.10.185 |
[9] | Avner Friedman, Kang-Ling Liao . The role of the cytokines IL-27 and IL-35 in cancer. Mathematical Biosciences and Engineering, 2015, 12(6): 1203-1217. doi: 10.3934/mbe.2015.12.1203 |
[10] | Jucheng Zhang, Minwen Ma, Huajun Li, Zhaoxia Pu, Haipeng Liu, Tianhai Huang, Huan Cheng, Yinglan Gong, Yonghua Chu, Zhikang Wang, Jun Jiang, Ling Xia . Early diagnosis of coronary microvascular dysfunction by myocardial contrast stress echocardiography. Mathematical Biosciences and Engineering, 2023, 20(5): 7845-7858. doi: 10.3934/mbe.2023339 |
Anti-angiogenesis as a treatment strategy for normalizing the microvascular network of tumors is of great interest among researchers, especially in combination with chemotherapy or radiotherapy. According to the vital role that angiogenesis plays in tumor growth and in exposing the tumor to therapeutic agents, this work develops a mathematical framework to study the influence of angiostatin, a plasminogen fragment that shows the anti-angiogenic function, in the evolutionary behavior of tumor-induced angiogenesis. Angiostatin-induced microvascular network reformation is investigated in a two-dimensional space by considering two parent vessels around a circular tumor by a modified discrete angiogenesis model in different tumor sizes. The effects of imposing modifications on the existing model, i.e., the matrix-degrading enzyme effect, proliferation and death of endothelial cells, matrix density function, and a more realistic chemotactic function, are investigated in this study. Results show a decrease in microvascular density in response to the angiostatin. A functional relationship exists between angiostatin's ability to normalize the capillary network and tumor size or progression stage, such that capillary density decreases by 55%, 41%, 24%, and 13% in tumors with a non-dimensional radius of 0.4, 0.3, 0.2, and 0.1, respectively, after angiostatin administration.
World health authorities consider cancer to be one of the most serious public health problems [1]. Cancer is the world's second leading cause of death, accounting for an estimated 9.6 million deaths (1 out of every 6) in 2018, and 85% of cancers involve solid tumors [2,3]. Angiogenesis is the process of forming new capillaries from existing blood vessels during the growth phase of a vascular tumor. Folkman introduced the concept of anti-angiogenesis in cancer treatment in 1971, based on preventing vessel spread into the tumor [4]. Folkman observed that if new vessels do not form, the tumor cannot grow more than 2–3 mm. This phenomenon is related to angiogenesis and has become the foundation of anti-angiogenesis treatment. Excessive endothelial cells (ECs) and abnormal cells around the tumor microvasculature cause tortuous capillaries that are poorly organized and have high permeability [5]. This abnormal structure with high density increases the resistance to flow, which makes it difficult to supply blood. Furthermore, the capillaries' high permeability prevents the formation of a pressure gradient between the microvasculature and the interstitial space, resulting in excessive interstitial fluid pressure. The lack of a pressure gradient disrupts the fluid flow and macromolecule transfer. These characteristics of solid tumors complicate therapeutic agent delivery and efficacy [6,7].
Anti-angiogenesis research has been conducted in both pre-clinical and clinical settings. Fukumura and Jain [8] investigated anti-angiogenic therapy's ability to normalize the capillary network and its surroundings. They discovered that anti-angiogenic therapy could temporarily normalize tumor vessels and their environment, both pre-clinically and clinically. Different anti-angiogenesis drugs work in different ways. Few drugs, such as combretastatin, angiostatin, and endostatin, directly attack the ECs. Angiostatin and endostatin inhibit the migration or proliferation of ECs [9,10]. Oehler and Biknell [11] provided valuable information about angiostatin and endostatin. In the study of O'Reilly et al. [12], angiostatin was discovered as a plasminogen fragment secreted by the primary tumor, which showed an inhibitory effect in tumor metastases. The results of an in-vivo investigation [13] showed the inhibitory effect of tranexamic acid on endometrial cancer as a result of angiostatin secretion during the study. In a pre-clinical study on mice, Peng et al. [14] found that treatment with endostar, a novel recombinant human endostatin, reduced tumor vessel density.
Studies of tumor progression and endothelial cell behavior during angiogenesis and anti-angiogenesis in living tissue or with laboratory equipment have some difficulties [15]. Mathematical models have been used to simulate angiogenesis since the 1970s [16]. Angiogenesis mathematical models are classified into three types: continuous, discrete, and hybrid [17]. The continuous model is based on mass conservation and chemical kinetics and employs differential equations. Individual cells are not considered in this model because cell clusters are modeled. Although this model produces good qualitative results, it cannot describe network behavior in detail. The discrete angiogenesis model employs the same equations as the continuous model but considers different interpretations of the equations' coefficients. Individual cell growth and motility can be modeled using this method. This model shows capillary network details. Endothelial cells are described using the discrete method in the hybrid model, while other parameters are expressed using the continuous model's partial differential equations [18]. The most important advantage of this method is that it allows for studying individual cells while continuously modeling the behavior of angiogenic factors and extracellular matrix (ECM). Anderson and his colleague(s) [19,20] used discrete and continuous models to describe angiogenesis in a basic study that is the foundation of many papers on capillary network formation. They considered different mechanisms of random motility, chemotaxis, and haptotaxis affecting the migration of ECs and studied their effect on angiogenesis. The ECs' behavior was modeled in a continuous angiogenesis model by taking into account EC proliferation and death parameters [21], which are applied to the 2D discrete model of the current study. Some previous studies used the discrete model of angiogenesis of Anderson and his colleague(s) [19,20], then investigated different phenomena of cancer, like blood flow in the microvascular network [22] and intravascular and extravascular flow behavior [23,24,25,26].
Stéphanou et al. and Mcdougall et al. [27,28] modified the discrete angiogenesis model to include matrix-degrading enzyme (MDE), but they did not study the microvasculature by excluding the MDE effect to observe the MDE function in tumor capillary network formation. Moreover, unlike the present study, they modeled the production of MDE locally by imposing a Boolean value (1 or 0) as the production coefficient of the MDE equation, which represents the absence or presence of EC at specific grid points. In a recent study by our group [29], the MDE is imposed on a hybrid mathematical model of linear tumor-induced angiogenesis with one parent vessel. In recent years, there has been a need for the development of mathematical models for anti-angiogenesis [30]. Most numerical studies in this field are at intracellular scales or rely on differential equations that do not provide a detailed physical view of the structure of the capillary network. Orme and Chaplain's study [31] is one of the fundamental studies in which anti-angiogenic therapy was investigated by applying the cytotoxic targeting of ECs, inhibition of cell mitosis, anti-chemotactic function, and anti-haptotactic function on continuous equations of the angiogenesis. Stéphanou et al. [32] predicted the anti-angiogenic efficiency in a model that provided the flow behavior in a discrete model-based angiogenesis-induced microvascular network by random-based or flow-based vessel removal. Different methodologies for pruning the microvascular network to mimic anti-angiogenesis were applied in other studies [26,33]. There is previous [34] and recent [35,36,37,38] research that studied vascular normalization by considering the effect of anti-angiogenic therapy on the transport properties of the interstitial and intravascular flow. Some groups [39,40,41,42,43] investigated different phenomena of solid tumors, ECs' evolution, interstitial fluid flow, and intravascular fluid flow by using the anti-angiogenic effect of angiostatin and endostatin, with various assumptions that one or more of them considered in the aforementioned studies, including (1) Considering only one parent vessel. (2) Excluding the MDE factor. (3) Defining a chemotactic function, in which ECs' sensitivity to it decreases by approaching the tumor. (4) Excluding the Fibronectin from the mathematical model. (5) Not considering the proliferation and loss of ECs. Baish et al. [44] extracted two parameters related to the capillary network geometry by image data. They examined the effect of normalization induced by anti-angiogenesis by modifying these parameters.
The current numerical study is developed to consider two parent vessels sprouting toward a circular tumor to provide a more realistic model for predicting the anti-angiogenic effect of angiostatin in ECs' evolution during the tumor-induced angiogenesis procedure. The mathematical model of angiogenesis is modified here compared to previous research to include the proliferation and death of ECs and the MDE effect. Defining a chemotactic function that facilitates ECs migration into the tumor site and applying a function of matrix density are other contributions of the present research. Different tumor sizes as a factor of tumor progression stage are considered in investigating the angiostatin effect on the capillaries' density.
This section consists of the computational domain, a representation of the mechanism of action of angiostatin affecting angiogenesis procedure, angiogenesis and anti-angiogenesis mathematical model, simulation details, and parameters value.
Figure 1 shows the computational domain of the present study. The mathematical modeling is performed in 2D space, where two parent vessels are located around a circular tumor. Ten sprouts on the left side of the domain and five sprouts on the right side are considered. The position of the sprouts is demonstrated in Figure 1.
Figure 2 shows the schematic view of the angiogenesis process and angiostatin function. Tumor angiogenic factor (TAF), oozed from the hypoxic region of the tumor, causes ECs' activation. Activated ECs degrade the ECM by secreting MDE, then start to migrate and proliferate towards the primary tumor by the tip EC [45]. Angiostatin is produced by well-vascularized primary tumor cells and enters the main blood vessels. Angiostatin is delivered to the parent vessel(s) stimulated by the secondary tumor via the bloodstream. Accordingly, the angiogenesis of the secondary tumor is affected by the inhibitory influence of angiostatin [46].
The mathematical statements that describe tumor-induced angiogenesis and how it is affected by angiostatin's anti-angiogenic action will be discussed in this section.
In this study, the main mechanisms controlling the migration of ECs are discussed, i.e., random motility, chemotaxis, and haptotaxis, based on the work of Anderson and Chaplain [19]. The model of this research also includes the proliferation and death of ECs during tumor-induced angiogenesis [39]. Another principal factor in describing angiogenesis, which is included in the present study, is the MDE secreted by the ECs based on previous works [16,27,28]. The one-dimensional mathematical model of [46] is developed to the present model to describe the inhibitory effect of angiostatin in the angiogenesis process.
The following equations governing angiogenesis and anti-angiogenesis are discretized. Then a probabilistic model of reinforced random walk is developed based on the discretized non-dimensional governing equations to provide details on the evolutionary behavior of ECs in a discrete model. More details can be found in section 2.4.
After the secretion of hypoxia-induced factors (HIFs) by tumor cells, HIFs release angiogenic factors, the most common of which is vascular endothelial growth factor [47]. ECs are activated next, then activated ECs produce MDE to open a migration route for EC sprouts to migrate and proliferate [48,49].
Two physical mechanisms of EC movement are chemotaxis and haptotaxis [50]. The gradient of tumor angiogenic factor (TAF) in the ECM is responsible for the migration of ECs toward the tumor under the influence of chemotaxis. The ECs maintain their forward motion by mechanically contacting components of the ECM, such as fibronectin. The fibronectin gradient makes an adhesion gradient in the ECM that causes the directional movement of ECs. This movement is referred to as haptotaxis [16,19,51]. For modeling purposes, a non-physical movement mechanism is assumed to explain the random movement of ECs.
Angiostatin, a substance secreted by the primary tumor to inhibit angiogenesis in secondary tumors, is examined in this study. The contribution of angiostatin is included in the mathematical model of this study by considering its inhibitory effect on the migratory behavior of ECs. Therefore, the dose-dependent 1D model of Anderson et al. [46] is developed for the 2D model of the current work to simulate the behavior of ECs in response to the angiostatin gradient.
To develop the governing equation of angiogenesis, the conservation equation for the EC density (n) is used (Eq. (1)) [19], in which EC flux (Jn) is controlled by the gradients of chemical concentrations involved in the migratory behavior of ECs [46], i.e., fibronectin concentration (f), TAF concentration (c), and angiostatin concentration (a, when its effect is considered in the model). In addition to the physical components contributing to Jn, a diffusion flux to the EC concentration gradient is considered by adding a random motility term for facilitating ECs' movement [16]. In this study, Jn is multiplied by a function (R(ρ)) to apply the ECM density effect to the model. R(ρ) is explained in section 2.3.3 after non-dimensionalizing the governing equations.
∂n∂t+∇.Jn=0Jn=R(ρ)[Jc+Jf+Ja+Jrandom] | (1) |
Jc, Jf, Ja, and Jrandom are described based on previous studies [19,29,46] as follows;
Jc=χ(c)n∇cJf=ψn∇fJa=ζ(a)n∇aJrandom=−Dn∇n | (2) |
χ(c) shows the chemotactic function with a modified equation of χ(c)=τ0(1+κc) in this study. As mentioned earlier, one of the contributions of EC flux is because of the fibronectin gradient that is expressed by Jf in Eq. (2). The gradient of fibronectin, as a dominant component of the ECM, causes haptotaxis. In other words, haptotaxis refers to the directional movement of ECs up an adhesive gradient induced by the fibronectin gradient in the ECM.
It was observed that angiostatin inhibited angiogenesis in a dose-dependent manner [12], i.e., angiogenesis was inhibited more as angiostatin concentration increased [46]. Anderson et al. [46] defined the angiostatin function with a linear distribution in a one-dimensional continuous model of angiogenesis to simulate that the greater the angiostatin concentration, the more response from ECs occurs. ζ(a) of [46] with an equation of ζ(a)=α0a is applied in the two-dimensional discrete angiogenesis model of the present study.
The parameters of the EC flux are as follows. τ0: chemotactic coefficient. κ: a positive constant of the chemotactic function. ψ: haptotactic coefficient. α0: a positive constant of the angiostatin function. Dn: the coefficient of random motility of ECs.
It is worth mentioning that although the sign of ∇a and ∇c is the same in Eq. (1), they act in opposite directions according to their physical-based distribution in the ECM (Figures S1 and S5). In other words, in the evolutionary journey of the ECs from the parent vessels to the tumor, the gradient of angiostatin acts against the gradient of TAF (chemotaxis). This opposite function of the angiostatin gradient against the chemotaxis in ECs' evolution is interpreted as the inhibitory effect of angiostatin.
TAF secretion by the tumor cells and the establishment of its gradient in the ECM stimulate the surrounding vessels to spread toward the tumor [16,19]. To simulate TAF diffusion through the ECM at the beginning of the angiogenesis process, a diffusion equation (Eq. (3)) is used.
∂c∂t=Dc∇2c−ιc | (3) |
in which Dc shows the diffusion coefficient of TAF and ι is its decay rate [19]. Eq. (3) is considered in its steady state for detecting the condition of TAF distribution at the initial stage of angiogenesis [19]. The reason for considering Eq. (3) in steady-state during the solution is because of the difference in the time scale of this equation and that of angiogenesis. In other words, TAF diffusion in the ECM occurs more quickly than in the angiogenesis process [16].
By augmenting the logistic type of growth for ECs' proliferation (∂n∂t=αr(1−nn0)nG(c)) and a first-order function for ECs' death (∂n∂t=−αdn) [21,39] with Eqs. (1) and (2), Eq. (4) is derived.
∂n∂t=Dn∇2n−R(ρ)∇.[χ(c)n∇c+ψn∇f+ζ(a)n∇a]+αr(1−nn0)nG(c)−αdnG(c)={0ifc⩽c∗c−c∗c0ifc>c∗ | (4) |
where G(c) is a function of the TAF concentration threshold below which proliferation does not occur. αr, n0, c∗, c0, and αd are the parameters of the functions of proliferation and death of ECs. They show the ECs proliferation coefficient, reference value of the EC density, TAF concentration threshold in G(c), reference value of the TAF concentration, and ECs death coefficient, respectively.
Three other equations should be developed for describing c, f, and a during modeling angiogenesis and angiostatin-induced anti-angiogenesis.
TAF and angiostatin are uptaken by ECs as they migrate through the ECM [19,46]. The following equations with the uptake coefficients for TAF (λ) and angiostatin (η) describe the behavior of TAF and angiostatin.
∂c∂t=−λnc | (5) |
∂a∂t=−ηna | (6) |
ECs produce fibronectin and uptake the fibronectin of ECM by making contact with it [19,20]. The first form of Eq. (7) defines the fibronectin equation without considering the effect of MDE. A degradation of fibronectin in the ECM simulates the effect of MDE on the ECM [27,28]. The second form of Eq. (7) describes the fibronectin equation when MDE is applied to the model. Section 2.3.3 discusses the operation of MDE in the tumor-induced angiogenesis process.
ByexcludingtheMDEeffect:∂f∂t=ωn−μnfByincludingtheMDEeffect:∂f∂t=ωn−ϑmf | (7) |
where ω, μ, and ϑ show the production coefficient of fibronectin, the uptake coefficient due to the binding of fibronectin to the ECs, and the uptake coefficient of fibronectin due to the MDE, respectively.
The produced MDE by activated ECs is diffused through the ECM and degraded at a constant rate. The following equation shows the MDE concentration (m) equation [27,28].
∂m∂t=ϖn+Dm∇2m−ξm | (8) |
Parameters of Eq. (8) show the production coefficient of MDE (ϖ), the diffusion coefficient of MDE (Dm), and the decay rate of MDE (ξ).
To non-dimensionalize the governing equations, the variables are made non-dimensional as follows;
ˉn=nn0,ˉc=cc0,ˉf=ff0,ˉa=aa0,ˉm=mm0,ˉt=tτ,ˉx=xL,ˉy=yL |
τ is used for rescaling time with an equation of τ=L2Dc, in which L shows the distance between the parent vessel(s) and the tumor. n0, c0, f0, a0, and m0 are reference values that are obtained based on previous studies [16,19,29,46,52]. x and y are spatial variables in the 2D computational domain.
Non-dimensional governing equations are presented below in which the non-dimensionalizing mark of variables (-) is dropped.
∂n∂t=D∇2n−Z(ρ)∇.[χ0(1+δc)n∇c+ϕn∇f+αaan∇a]+βr(1−n)nM(c)−βdnM(c)={0ifc⩽c∙c−c∙ifc>c∙ | (9) |
∂c∂t=−νnc | (10) |
∂a∂t=−σna | (11) |
ByexcludingtheMDEeffect:∂f∂t=ςn−γnfByincludingtheMDEeffect:∂f∂t=ςn−εmf | (12) |
∂m∂t=φn+θ∇2m−om | (13) |
where the non-dimensional parameters are as follows;
D=DnDc,χ0=τ0c0Dc,δ=κc0,ϕ=ψf0Dc,αa=α0a20Dc,βr=αrL2Dc,βd=αdL2Dc,c∙=c∗c0,ν=λn0L2Dc,σ=ηn0L2Dc,ς=ωn0L2f0Dc,γ=μn0L2Dc,ε=ϑm0L2Dc,φ=ϖn0L2m0Dc,θ=DmDc,o=ξL2Dc |
Z(ρ) is the non-dimensional form of R(ρ) with a function of Z(ρ)=exp(−(ρ−ρ0)22υ2) with different values of ρ as the matrix density. ρ0 and υ are positive constants. More details about Z(ρ) can be found in section 2.3.3.
Eqs. (9)–(13) present the general form of the non-dimensional mathematical model of angiogenesis induced by the tumor and anti-angiogenesis induced by angiostatin. More details can be found in previous studies [16,19,27,28,39]. It should be noted that Eq. (11) and the fourth term on the right-hand side of Eq. (9) are excluded from the model when anti-angiogenesis is not considered.
The chemotactic function was defined in previous studies [19,20,27,28,39,40,42,46,50,51] so that as ECs approached the tumor, its effect was thought to diminish. This effect was applied to the model by introducing a function that decreases the TAF gradient stimulant impact as the ECs get closer to the tumor. In other words, the chemotactic function was defined so that its sensitivity was reversely proportional to the TAF concentration. In this study, the chemotactic function is modified to be more effective during the ECs' movement toward the tumor. The reason for this modification is to reflect the growth of capillaries more realistic because it was observed that as developing capillaries approach the tumor, they grow faster [53].
Another modification to the model of previous research [19,20,27,28,39,40,42,46,50,51] in this study is taking into account the ECM density effect on microvascular network formation. As a representative of medium density, matrix density could impact the establishment of ECs' connections to ECM components and, subsequently the speed of migration of ECs. The matrix density is defined in this study by a non-dimensional equation, and its effect is investigated by multiplying terms associated with ECs' migration by this function.
Another contribution of the present study in comparison to the previous research [19,20,27,28,39,40,42,46,50,51] is subsuming the MDE secreted by the ECs into the mathematical modeling of evolutionary behavior of ECs toward a circular tumor. The ECM is degraded by MDE to facilitate the migration of ECs. The present study simulates the MDE effect based on the degradation of fibronectin by MDE. So, the equation of fibronectin concentration is developed by proportionating the fibronectin decay to the MDE concentration.
It was assumed in the former research that birth and loss of ECs are not involved in the mathematical model of angiogenesis. In the present study, a logistic growth function is considered to describe the mitosis of ECs based on the work of Chaplain and Stuart [21]. ECs' loss is governed by a first-order process in this work.
The governing equations are discretized by Euler's finite difference approximation. Discretized form of Eqs. (9) – (13) are as follows;
ni,jq+1=P0nqi,j+P1nqi+1,j+P2nqi−1,j+P3nqi,j+1+P4nqi,j−1+Δtβr(1−nqi,j)nqi,jM(cqi,j)−Δtβdnqi,j | (14) |
cq+1i,j=cqi,j(1−νnqi,jΔt) | (15) |
aq+1i,j=aqi,j(1−σnqi,jΔt) | (16) |
ByexcludingtheMDEeffect:fq+1i,j=fqi,j(1−γnqi,jΔt)+ςnqi,jΔtByincludingtheMDEeffect:fq+1i,j=fqi,j(1−εmqi,jΔt)+ςnqi,jΔt | (17) |
mq+1i,j=mqi,j(1−4θΔth2−oΔt)+Δtθh2(mqi+1,j+mqi−1,j+mqi,j+1+mqi,j−1)+φΔtnqi,j | (18) |
where P0–P4 (P0,P1,P2,P3,P4) are coefficients of the discrete model of tumor-induced angiogenesis under the inhibitory effect of angiostatin. They are interpreted as EC migration probabilities, which are explained in the next section and expressed by Eqs. (S1) – (S5). The size of the grids of the computational domain in the x and y directions is considered to be equal to h. Δt shows the time step.
The discretized non-dimensional set of governing equations is solved numerically in an iterative procedure to find P0–P4 at each time step. EC density, TAF, fibronectin, and angiostatin concentrations are calculated at each time step starting from their initial values. These calculated values are used for the next time step, and the solution will continue until the specified time. Ten capillary sprouts on the left side of the domain and five capillary sprouts on the right side of the domain are considered initiated by ten and five ECs occupying specified grid positions on the parent vessels.
The answer to how ECs move in a time step is determined using the theory of reinforced (biased) random walk model of Stevens and Othmer [54] by generating a spectrum of probability distributions by a function summing P0–P4 (Eq. (19) and Figure 3). The discrete stochastic model of angiogenesis relies on the tip EC of a sprout controlling the sprout's path within the ECM. If an EC occupies the (i, j) position in the ECM, and its next movement is, for example, upward, it will also occupy the (i, j+1) grid.
P0–P4 are proportional to the probabilities of ECs being stationary (P0) or moving (P1–P4). P1 and P2 correspond to the probability of ECs migrating toward or away from the tumor based on the parent vessels from which they originated. P3 and P4 are related to the journey of ECs in up and down directions, respectively. The biased physics of the current study's model is due to the fact that each of the coefficients of P0–P4 involves four terms. One for random motility and three terms of local gradients of different chemicals concentration. So, if the model does not include TAF, fibronectin, and angiostatin, the probabilities of ECs' movement in four directions (P1–P4) would be the same, and there is no preference for any movement direction [19]. It is worth mentioning that in this study, the probability of backward migration of ECs is not considered.
The probabilistic model steps after finding P0–P4 in each time step consist of 1) generating a random number between zero and one and 2) determining the current tip EC migration direction to be stationary (R0), migrate toward or away from the tumor (R1andR2), move up (R3), and move down (R4) depending on which range of probabilities this random number belongs to [19]. There is a greater probability that the coefficient associated with a given range will be selected when it is larger [19].
The numerical modeling is carried out in a 2D computational field with non-dimensional values of [0,2]×[0,1]. The domain is gridded into 200×100 meshes. It should be noted that the anastomosis and branching are considered here based on the previous studies [19,20].
Thespectrumofprobabilitydistributionranges{Beingstationaryprobability:R0=0−P0MovementProbability:Rj=j−1∑i=0Pi−j∑i=0Pi,j=1−4(Movementtowardsorawayfromthetumor:R1andR2Moveup:R3andMovedown:R4) | (19) |
It is worth mentioning that not all vessels produced by the mathematical model are necessarily physically interpreted. Therefore, in this study, the vessels that do not make a loop in the network are pruned, and the results are reported after the pruning as well.
Boundary conditions (BCs) of the simulation are reported in Table 1. Periodic BC is applied on the top and bottom sides of the computational field. The no flux BC is considered for the left and right sides of the domain.
Zone | Equation |
Top and bottom sides | θi,j+1=θi,1,forj=Nj& i=2:Ni−1θi,j−1=θi,Nj,forj=1& i=2:Ni−1}θ:c,f,m,n,a |
Left and right sides | θi−1,j=θi+1,j,fori=1& j=1:Njθi+1,j=θi−1,j,fori=Ni& j=1:Njθi,j−1=θi,j+1,forj=1& i=1andNiθi,j+1=θi,j−1,forj=Nj& i=1andNi}θ:c,f,m,n,a |
Initial conditions (ICs) of the variables of the mathematical models in this study are determined based on their physical initial distributions according to the literature [16,19,42]. Figures S1 – S5 demonstrate the profile of ICs. The initial locations of ECs on the left-side parent vessel are at x=0 and y=0.05,0.15,0.25,0.35,0.45,0.55,0.65,0.75,0.85,0.95. The ECs initiate migration at x=2 and y=0.15,0.35,0.55,0.75,0.95 on the right-side parent vessel.
Figure 4 shows the flowchart of simulation details in this study. The numerical calculation of movement probability coefficients is the first step, which finds the evolution of ECs during tumor-induced angiogenesis. Modifications of this research may affect angiogenesis, so that is investigated next. Finally, under the influence of angiostatin, the density of capillaries (DoCs) is determined.
The parameters of the non-dimensional governing equations listed in Table 2 have physical concepts, and the relations that represent them are explained in detail in Section 2.3.2. The values of these parameters are derived from previous studies in which as much experimental data as possible was used. Among these experimental studies, the works of Gimbrone et al. [55] and Muthukkaruppan et al. [56] can be mentioned, in which a small solid tumor or a part of it was implanted in the cornea of an animal eye close to the limbal vessels that were lined with endothelial cells. In the previous studies cited in Table 2, other experimental works also were used to extract the value of the mathematical model parameters. Moreover, some parameters (θ and υ) were adapted within the limits of the physical conditions in previous work [29].
Parameter | Description | Value | Reference(s) |
D | Random motility coefficient | 0.00035 | [16,19,27] |
ϕ | Haptotaxis coefficient | 0.16 | [27,28] |
βr | EC proliferation coefficient | 7.67 | [39] |
βd | EC loss coefficient | 2.13×10−4 | [39] |
c∙ | TAF concentration threshold in M(c) | 0.2 | [39] |
ν | Uptake coefficient of TAF | 0.1 | [19,28,40] |
ς | Production coefficient of fibronectin | 0.05 | [19,27,32] |
ε | Uptake coefficient of fibronectin due to the MDE | 0.1 | [27,28] |
γ | Uptake coefficient of fibronectin due to the binding to the ECs | 0.1 | [16,19] |
φ | Production coefficient of MDE | 10×10−6 | [27,28] |
θ | Diffusion coefficient of MDE | 0.001 | [16] |
o | Decay coefficient of MDE | 3 | [27,28] |
χ0 | Chemotactic coefficient | 0.38 | [19,27,28] |
δ | Positive constant in chemotactic function | 0.6 | [19] |
υ | Positive constant in matrix density function | 0.2 | [16] |
ρ0 | Reference value of matrix density | 0.35 | [57] |
αa | Positive constant in angiostatin function | 0.38 | [46] |
σ | uptake coefficient of angiostatin | 0.1 | [42] |
The experimental validation of the present study was not possible due to the lack of laboratory equipment. So, the study of Tee and DiStefano Ⅲ [39] is duplicated with two parent vessels around a circular tumor in this research to compare the results. Tee and DiStefano Ⅲ [39] investigated the response of angiogenesis to endostatin, another anti-angiogenic factor secreted by the primary tumor, in a 2D computational domain with considering one parent vessel on one side of the domain and the tumor on the other side. They developed the model of Anderson and Chaplain [19] to include mitogen-stimulated proliferation, then applied a non-linear equation that represents the inhibition function of endostatin in plasma to the proliferation term. The anti-angiogenic effect of endostatin is assessed in this paper by introducing the function of Tee and DiStefano Ⅲ [39] into the present study's model, which simulates the dynamic response to exogenous endostatin therapy. The simulation is carried out by applying a continuous injection of endostatin with a dosage of 20 mg/kg/day. As illustrated in Figure 5, the capillary migration toward the TAF source is ceased at this dose of treatment, which is in accordance with the results of Tee and DiStefano Ⅲ [39]. They showed that continuous infusion of endostatin prevents the growth of blood vessels into the tumor, even at the lowest dose of 20 mg/kg/day considered in their simulation over various clearance rates of the drug. Additionally, the results of this study, which are discussed in the following sections, are qualitatively in agreement with the results of the in-vivo study of O'Reilly et al. [12]. After removing the primary tumor and subsequently the angiostatin secreted from it, O'Reilly et al. [12] found that neovascularization and metastases were intensified.
By solving the non-dimensional discrete model of angiogenesis and modifying it to include ECs' proliferation and death, MDE secreted by ECs, and matrix density, the present study examines the evolution of ECs during angiogenesis induced by a circular tumor around which two parent vessels are considered. Moreover, angiostatin, produced by the primary tumor, is applied to the model to examine the DoCs of the microvascular network in different tumor sizes under the inhibitory effect of angiostatin.
According to the nature of the randomness of the mathematical model, each simulation is taken ten times, and each time the DoCs is extracted. The DoCs is divided into its maximum value for non-dimensionalization. Then, the average of the non-dimensional DoCs is taken and reported.
The case, which includes all the proliferation and death of ECs, MDE secreted by ECS, and matrix density, is considered a criterion for examining the effects of the modifications of angiogenesis simulation (control case). To this aim, the modifications are excluded one by one from the model, and the results are compared to the control case. Figure 6 displays the snapshots of the samples of the microvascular networks generated in different cases after 7.5 days. ρ is considered to be 0.35, and the tumor non-dimensional radius (TNDR) is equal to 0.1 in this figure.
As illustrated in Figure 6 (a) and (b), excluding the ECs' proliferation from the model can cause a decrease in the DoCs in the ECM, especially in areas close to the tumor where the TAF concentration is higher. Because according to Eq. (9), the M(c) function operates in locations where the TAF concentration is higher than a threshold (c∙). The proliferation will therefore be more effective when approaching the tumor, which could be more pronounced in areas close to the tumor when not taken into account in the model. Excluding the death of ECs from the discrete model of angiogenesis results in an increase in the DoCs, as shown in Figure 6 (a) and (c).
By comparing the spatiotemporal distribution of ECs with and without the MDE effect (Figure 6 (a) and (d), it is seen that, by applying the MDE effect, the migration path of the ECs from the parent vessels to the tumor site is more converging towards the TAF source (circular tumor) than when the MDE effect is excluded. The reason for this will be discussed in section 5.
Figure 7 demonstrates the capillary network of the control case before and after pruning to manifest its operation in this study.
As a quantitative illustration of the effects of adding the proliferation and death of ECs to the discrete mathematical model of angiogenesis, the average and standard deviation of non-dimensional DoCs for ten simulations of Figure 6 (a), (b), and (c) are given in Figure 8.
Figure 8 confirms that the discussion made about the results of Figure 6 is also accurate after pruning. Moreover, as can be seen in Figure 8 (a) and discussed before, the effect of proliferation is more evident at 7.5 days, as the ECs are closer to the tumor.
Figure 9 (a) shows the area in which ECs migrate, converging from the sprouts of the parent vessels toward a circular tumor, named the convergence spatial range (CSR). For investigating the MDE effect, the average and standard deviation of the non-dimensional DoCs data are found in Figure 9 (b) outside the CSR with and without considering the MDE in the angiogenesis mathematical model with TNDR = 0.1 and ρ=0.35.
It is seen in Figure 9 (b) that the MDE acts as a booster for the converging migration of ECs by promoting the haptotaxis mechanism to direct the ECs toward a circular tumor.
In order to compare the migration speed of ECs, Figure 10 presents samples of the capillary network progression after five days for different matrix densities of ρ=0.15,0.25,0.35,0.45,0.55.
To investigate the effect of matrix density, which is a parameter that shows the density of ECM medium, the migration speed of sprouts is calculated for different ρs with considering all parameters of proliferation and death of ECs, MDE secreted by ECs, and matrix density, as shown in Figure 11. In this study, migration speed is defined as the average number of capillaries reaching coordinates of x = 0.4, 0.6, 0.8, 1, 1.2, 1.4, and 1.6 in the growth path towards the tumor in 5 days after ECs started moving from their parent vessels. This calculation is done for all ten runs. Figure 11 displays the average result of repeated simulations.
Figure 11 shows the performance of matrix density in ECs migration speed. As shown in Figure 11, increasing ρ from 0.15 to 0.35 causes ECs' migration faster, but the ECs' migration speed decreases by increasing ρ from 0.35 to 0.55. This behavior arises from the introduced function of Z(ρ) to the mathematical model. This behavior is caused by the fact that at low matrix densities, ECs cannot establish a connection with matrix fibers, which prevents them from migrating. In this case, the ECs show a kind of discontinuity and are unable to form capillaries, as observed experimentally [58]. The dense medium of the ECM, on the other hand, acts as a barrier and slows migration at high matrix density.
Figure 12 shows the sample of the evolution of the capillary network in different TNDRs with and without applying the angiostatin function in the mathematical model at t=7.5 days. Figure 13 illustrates the results of Figure 12 after pruning the non-physical capillaries, which don't fall in a loop. As shown in Figure 12 and Figure 13, the DoCs increases by approaching the tumor caused by increasing the branching probability, which is observed in the experiments [56].
As seen in Figure 12 and Figure 13, the morphology of tumor-induced angiogenesis is impacted by angiostatin. The microvascular density is decreased under the inhibitory effect of angiostatin. In the small tumor size (TNDR = 0.1), the migration speed of ECs decreases after applying the angiostatin function. Angiostatin administration reduces branching and anastomosis, which is more visible in small tumor sizes. In TNDR = 0.1, 0.2, in which chemotaxis stimulates ECs to migrate in a more convergent spatial range, the sprouts have a great tendency to converge towards the tumor under the influence of angiostatin. This convergence is caused by the high intensity of angiostatin function near the parent vessel(s) as opposed to the lateral movement that would cause ECs to migrate out of the CSR. Subsequently, the ECs' convergence halfway from the parent vessel to the circular tumor site in TNDR = 0.1, 0.2 is obvious in the second column of Figure 12 and Figure 13. The migration of sprouts of ECs toward the CSR starts to decrease by increasing the tumor size to TNDR = 0.3.
By pruning the capillaries that have only a mathematical sense and not a physical one, it is observed in Figure 13 that angiostatin may prevent blood vessels from reaching the tumor in TNDR = 0.1. This could result in preventing the therapeutic agent delivery to the tumor site. Therefore, aggressive normalization of the microvascular network could have the opposite effect on the quality of drug delivery. Because the capillary network is like a double-edged sword, which on the one hand, disrupts efficient drug delivery, and on the other hand, transfers therapeutic agents [36,59,60].
Vessel density in tumor-induced angiogenesis is known as a prognostic benchmark for a variety of cancers. Although modification in vessel density during the anti-angiogenic therapy is not suitable as a stand-alone method to assess the anti-angiogenic therapy effect, a decrease in microvascular density may demonstrate the activity of the anti-angiogenic agent [61]. Figure 14 shows the average and standard deviation of the DoCs data before and after applying the anti-angiogenic factor, angiostatin, in different time spans and TNDRs.
As seen in Figure 14, it is found that the DoCs is decreased by angiostatin administration. The percentage of DoCs decrease as a result of an anti-angiogenic factor in TNDR = 0.1, 0.2, 0.3, and 0.4 is approximately equal to 55%, 41%, 24%, and 13%, respectively, at t = 7.5 days before pruning the microvascular network. There exist experimental studies that show a decrease in tumor microvascular density in response to anti-angiogenic therapy. Soto-Pantoja et al. [62], in an in-vivo study, showed a 50% decrease in vessel density of human A549 lung tumor xenografted S.C. in mice after the injection of angiostatin. A decrease in the density of blood vessels of established ovarian cancer in mice after treatment with angiostatin was reported [63].
After 2.5 days of the start of the simulation, when capillary network evolution spends the initial progression, the decrease of DoCs in response to anti-angiogenic therapy reduces by increasing the tumor size or expanding the chemotaxis stimulant in a wider spatial range. Though the influence of angiostatin in decreasing the DoCs is more evident in lower tumor size, it cannot be interpreted as the better efficiency of an anti-angiogenic factor in the lower stage of the tumor, as discussed above. On the other hand, as can be seen in Figure 12 and Figure 13, the microvascular network reformation in TNDR = 0.4 by applying the angiostatin function to the model is not significant in comparison to other sizes. This phenomenon illustrates the dependency of angiostatin efficiency on the tumor's size in modifying the microvascular network that is mentioned in the literature [36,64], which studied vascular normalization induced by anti-angiogenic therapy from a different point of view.
The study of anti-angiogenesis is determining because angiogenesis causes tumor growth, which leads to the fatal vascular phase. In this study, a mathematical model of angiogenesis is modified to investigate the effect of an anti-angiogenic agent (angiostatin) on the angiogenesis process in different tumor sizes. This study takes into account various mechanisms that control the evolution of ECs during their migration journey towards the tumor, i.e., movement strategies of chemotaxis, haptotaxis, and random motility, as well as the proliferation and death of ECs. Moreover, matrix density as a characteristic of ECM and MDE as a parameter influencing the ECM are imposed on the model.
This study demonstrates different results induced by modifications applied to the discrete model of angiogenesis. In the present study, it is shown that capillaries reaching into the tumor is facilitated by the modification in chemotactic function, in contrast to previous research [19,39], where it was assumed that the ECs' sensitivity to TAF would decrease when approaching the tumor. The reason for this is that previous studies [19,39] introduced a chemotactic function that reduces the effect of TAF gradient stimulant when ECs approach the TAF source (tumor). Based on the results of this model, the effect of proliferation on increasing the DoCs becomes evident five days after the initiation of the angiogenesis process. In accordance with observed mitosis in ECs that occurred a few days after the start of the process [49], this is a result of the physics of the defined function for proliferation.
When a circular TAF source lies in the center of the computational domain, similar to the one in this study, ECs are controlled to move laterally (in up and down directions) by both chemotaxis and haptotaxis. On the other hand, it has been mentioned that the MDE operation is in accordance with the distribution of ECs. Therefore, since ECs move towards the circular tumor under the influence of chemotaxis, the MDE is also present in the CSR when the MDE is included in the model. As the MDE acts by degrading the fibronectin molecules, it enhances the fibronectin gradient in the CSR and can boost the converging migration of ECs toward the circular TAF source. Therefore it is shown that the average of non-dimensional DoCs is higher outside the CSR when MDE is not present in the mathematical model.
This study shows the matrix density effect in accelerating or decelerating the migration of ECs. In other words, ECs are unable to migrate at low matrix densities due to their inability to connect to matrix components. Besides, the speed of ECs migration decreases at high matrix density. So, as indicated in the present study, there is an extreme point for matrix density values in which ECs' migration does not slow.
Based on the results of this study, the function of angiostatin is dependent on tumor size. In small tumor sizes of 0.1 and 0.2, ECs migrate to the tumor in a more convergent direction under the influence of angiostatin. This behavior is due to the control of the gradient of lateral movement stimuli by the gradient of angiostatin, whose effect is more evident in small circular tumor sizes due to the greater concentration of the TAF source in the smaller area. After the early times and establishing the stability of sprouts, it is observed in the present study that angiostatin-induced capillary network suppression decreases by increasing the tumor size. It does not mean that the normalizing behavior of angiostatin is better in the small tumor size because of the dual role of the microvascular network, one is disrupting the drug delivery, and the other is acting as an interface for drug delivery.
The financial support from the Canadian Institutes of Health Research (CIHR) is gratefully acknowledged (MK).
The authors declare there is no conflict of interest.
The coefficients of the modified discrete model of angiogenesis considering the anti-angiogenesis effect induced by angiostatin administration are as follows;
P0=1−4ΔtDh2−Z(ρ)χ0(1+δcqi,j)Δth2(cqi+1,j+cqi−1,j−4cqi,j+cqi,j+1+cqi,j−1)−Z(ρ)χ0δΔt4h2[(cqi+1,j−cqi−1,j)2+(cqi,j+1−cqi,j−1)2]−Z(ρ)ϕΔth2(fqi+1,j+fqi−1,j−4fqi,j+fqi,j+1+fqi,j−1)−Δtαaaqi,jZ(ρ)h2(aqi+1,j+aqi−1,j−4aqi,j+aqi,j+1+aqi,j−1)−αaΔtZ(ρ)4h2[(aqi+1,j−aqi−1,j)2+(aqi,j+1−aqi,j−1)2] | (S1) |
P1=DΔth2−χ0Z(ρ)Δt4h2(1+δcqi+1,j)(cqi+1,j−cqi−1,j)−ϕZ(ρ)Δt4h2(fqi+1,j−fqi−1,j)−αaaqi+1,jΔtZ(ρ)4h2(aqi+1,j−aqi−1,j) | (S2) |
P2=DΔth2+χ0Z(ρ)Δt4h2(1+δcqi−1,j)(cqi+1,j−cqi−1,j)+ϕZ(ρ)Δt4h2(fqi+1,j−fqi−1,j)+αaaqi−1,jΔtZ(ρ)4h2(aqi+1,j−aqi−1,j) | (S3) |
P3=DΔth2−χ0Z(ρ)Δt4h2(1+δcqi,j+1)(cqi,j+1−cqi,j−1)−ϕZ(ρ)Δt4h2(fqi,j+1−fqi,j−1)−αaaqi,j+1ΔtZ(ρ)4h2(aqi,j+1−aqi,j−1) | (S4) |
P4=DΔth2+χ0Z(ρ)Δt4h2(1+δcqi,j−1)(cqi,j+1−cqi,j−1)+ϕZ(ρ)Δt4h2(fqi,j+1−fqi,j−1)+αaaqi,j−1ΔtZ(ρ)4h2(aqi,j+1−aqi,j−1) | (S5) |
Figures (S1)–(S5) show the initial conditions of the mathematical model.
[1] |
F. M. Kashkooli, M. Soltani, M. Rezaeian, E. Taatizadeh, M. H. Hamedi, Image-based spatio-temporal model of drug delivery in a heterogeneous vasculature of a solid tumor-computational approach, Microvasc. Res., 123 (2019), 111–124. https://doi.org/10.1016/j.mvr.2019.01.005 doi: 10.1016/j.mvr.2019.01.005
![]() |
[2] |
M. Soltani, M. Jabarifar, F. M. Kashkooli, A. Rahmim, Evaluation of inverse methods for estimation of mechanical parameters in solid tumors, Biomed. Phys. Eng. Express, 6 (2020), 035027. https://doi.org/10.1088/2057-1976/ab872b doi: 10.1088/2057-1976/ab872b
![]() |
[3] |
R. K. Jain, Normalization of tumor vasculature: An emerging concept in antiangiogenic therapy, Science, 307 (2005), 58–62. https://doi.org/10.1126/science.1104819 doi: 10.1126/science.1104819
![]() |
[4] |
J. Folkman, Tumor angiogenesis: Therapeutic implications, N. Engl. J. Med., 285 (1971), 1182–1186. https://doi.org/10.1056/NEJM197111182852108 doi: 10.1056/NEJM197111182852108
![]() |
[5] | J. W. Baish, R. K. Jain, Fractals and cancer, Cancer Res., 60 (2000), 3683–3688. Retrieved from https://aacrjournals.org/cancerres |
[6] |
F. M. Kashkooli, M. Soltani, Evaluation of solid tumor response to sequential treatment cycles via a new computational hybrid approach, Sci. Rep., 11 (2021), 21475. https://doi.org/10.1038/s41598-021-00989-x doi: 10.1038/s41598-021-00989-x
![]() |
[7] |
F. M. Kashkooli, M. Soltani, M. M. Momeni, A. Rahmim, Enhanced drug delivery to solid tumors via drug-loaded nanocarriers: An image-based computational framework, Front. Oncol., 11 (2021), 655781. https://doi.org/10.3389/fonc.2021.655781 doi: 10.3389/fonc.2021.655781
![]() |
[8] |
D. Fukumura, R. K. Jain, Tumor microvasculature and microenvironment: Targets for anti-angiogenesis and normalization, Microvasc. Res., 74 (2007), 72–84. https://doi.org/10.1016/j.mvr.2007.05.003 doi: 10.1016/j.mvr.2007.05.003
![]() |
[9] |
K. Eriksson, P. Magnusson, J. Dixelius, L. Claesson-Welsh, M. J. Cross, Angiostatin and endostatin inhibit endothelial cell migration in response to FGF and VEGF without interfering with specific intracellular signal transduction pathways, FEBS Lett., 536 (2003), 19–24. https://doi.org/10.1016/S0014-5793(03)00003-6 doi: 10.1016/S0014-5793(03)00003-6
![]() |
[10] |
C. O. Madu, S. Wang, C. O. Madu, Y. Lu, Angiogenesis in breast cancer progression, diagnosis, and treatment, J. Cancer, 11 (2020), 4474–4494. https://doi.org/10.7150/jca.44313 doi: 10.7150/jca.44313
![]() |
[11] |
M. K. Oehler, R. Bicknell, The promise of anti-angiogenic cancer therapy, Br. J. Cancer, 82 (2000), 749–752. https://doi.org/10.1054/bjoc.1999.0991 doi: 10.1054/bjoc.1999.0991
![]() |
[12] |
M. S. O'Reilly, L. Holmgren, Y. Shing, C. Chen, R. A. Rosenthal, M. Moses, et al., Angiostatin: A novel angiogenesis inhibitor that mediates the suppression of metastases by a Lewis lung carcinoma, Cell, 79 (1994), 315–328. https://doi.org/10.1016/0092-8674(94)90200-3 doi: 10.1016/0092-8674(94)90200-3
![]() |
[13] |
K. Hiramoto, Y. Yamate, Tranexamic acid reduces endometrial cancer effects through the production of angiostatin, J. Cancer, 13 (2022), 1603–1610. https://doi.org/10.7150/jca.68169 doi: 10.7150/jca.68169
![]() |
[14] |
F. Peng, Z. Xu, J. Wang, Y. Chen, Q. Li, Y. Zuo, et al., Recombinant human endostatin normalizes tumor vasculature and enhances radiation response in xenografted human nasopharyngeal carcinoma models, PLoS One., 7 (2012), e34646. https://doi.org/10.1371/journal.pone.0034646 doi: 10.1371/journal.pone.0034646
![]() |
[15] | T. H. Adair, J. -P. Montani, Angiogenesis, Morgan and Claypool Life Sciences, San Rafael, CA, 2010. https: //doi.org/10.4199/C00017ED1V01Y201009ISP010 |
[16] | M. Soltani, Numerical Modeling of Drug Delivery to Solid Tumor Microvasculature, Ph.D. thesis, University of Waterloo, 2013. Retrieved from https: //uwspace.uwaterloo.ca |
[17] | S. Laranjeira, R. Coy, R. J. Shipley, Mathematical Modeling for Nerve Repair Research, in: J. B. Phillips, D. Hercher, T. Hausner (Eds.) Peripheral Nerve Tissue Engineering and Regeneration Springer, Cham, (2022), 189–241. https: //doi.org/10.1007/978-3-030-21052-6_10 |
[18] |
H. A. Harrington, M. Maier, L. Naidoo, N. Whitaker, P. G. Kevrekidis, A hybrid model for tumor-induced angiogenesis in the cornea in the presence of inhibitors, Math. Comput. Model., 46 (2007), 513–524. https://doi.org/10.1016/j.mcm.2006.11.034 doi: 10.1016/j.mcm.2006.11.034
![]() |
[19] |
A. R. A. Anderson, M. A. J. Chaplain, Continuous and discrete mathematical models of tumor-induced angiogenesis, Bull. Math. Biol., 60 (1998), 857–899. https://doi.org/10.1006/bulm.1998.0042 doi: 10.1006/bulm.1998.0042
![]() |
[20] | A. R. A. Anderson, M. A. J. Chaplain, S. R. McDougall, A Hybrid Discrete-Continuum Model of Tumour Induced Angiogenesis, in: T. L. Jackson (Ed.) Modeling Tumor Vasculature, Springer, New York, NY, (2012), 105–133. https: //doi.org/10.1007/978-1-4614-0052-3_5 |
[21] |
M. A. J. Chaplain, A. M. Stuart, A model mechanism for the chemotactic response of endothelial cells to tumour angiogenesis factor, Math. Med. Biol., 10 (1993), 149–168. https://doi.org/10.1093/imammb/10.3.149 doi: 10.1093/imammb/10.3.149
![]() |
[22] |
S. R. McDougall, A. R. A. Anderson, M. A. J. Chaplain, J. A. Sherratt, Mathematical modelling of flow through vascular networks: implications for tumour-induced angiogenesis and chemotherapy strategies, Bull. Math. Biol., 64 (2002), 673–702. https://doi.org/10.1006/bulm.2002.0293 doi: 10.1006/bulm.2002.0293
![]() |
[23] |
M. Soltani, P. Chen, Numerical modeling of interstitial fluid flow coupled with blood flow through a remodeled solid tumor microvascular network, PLoS One, 8 (2013), e67025. https://doi.org/10.1371/journal.pone.0067025 doi: 10.1371/journal.pone.0067025
![]() |
[24] |
M. Sefidgar, M. Soltani, K. Raahemifar, M. Sadeghi, H. Bazmara, M. Bazargan, et al., Numerical modeling of drug delivery in a dynamic solid tumor microvasculature, Microvasc. Res., 99 (2015), 43–56. https://doi.org/10.1016/j.mvr.2015.02.007 doi: 10.1016/j.mvr.2015.02.007
![]() |
[25] |
J. Lyu, J. Cao, P. Zhang, Y. Liu, H. Cheng, Coupled hybrid continuum-discrete model of tumor angiogenesis and growth, PLoS One, 11 (2016), e0163173. https://doi.org/10.1371/journal.pone.0163173 doi: 10.1371/journal.pone.0163173
![]() |
[26] |
J. Wu, Q. Long, S. Xu, A. R. Padhani, Study of tumor blood perfusion and its variation due to vascular normalization by anti-angiogenic therapy based on 3D angiogenic microvasculature, J. Biomech., 42 (2009), 712–721. https://doi.org/10.1016/j.jbiomech.2009.01.009 doi: 10.1016/j.jbiomech.2009.01.009
![]() |
[27] |
A. Stéphanou, S. R. McDougall, A. R. A. Anderson, M. A. J. Chaplain, Mathematical modelling of the influence of blood rheological properties upon adaptative tumour-induced angiogenesis, Math. Comput. Model., 44 (2006), 96–123. https://doi.org/10.1016/j.mcm.2004.07.021 doi: 10.1016/j.mcm.2004.07.021
![]() |
[28] |
S. R. McDougall, A. R. A. Anderson, M. A. J. Chaplain, Mathematical modelling of dynamic adaptive tumour-induced angiogenesis: clinical implications and therapeutic targeting strategies, J. Theor. Biol., 241 (2006), 564–589. https://doi.org/10.1016/j.jtbi.2005.12.022 doi: 10.1016/j.jtbi.2005.12.022
![]() |
[29] |
M. Soltani, Capillary network formation and structure in a modified discrete mathematical model of angiogenesis, Biomed. Phys. Eng. Express, 8 (2022), 015023. https://doi.org/10.1088/2057-1976/ac4175 doi: 10.1088/2057-1976/ac4175
![]() |
[30] |
J. Ciccolini, S. Benzekry, B. Lacarelle, F. Barlési, Improving efficacy of the combination between antiangiogenic and chemotherapy: time for mathematical modeling support, Proc. Natl. Acad. Sci., 112 (2015), E3453. https://doi.org/10.1073/pnas.1506689112 doi: 10.1073/pnas.1506689112
![]() |
[31] |
M. E. Orme, M. A. J. Chaplain, Two-dimensional models of tumour angiogenesis and anti-angiogenesis strategies, Math. Med. Biol., 14 (1997), 189–205. https://doi.org/10.1093/imammb/14.3.189 doi: 10.1093/imammb/14.3.189
![]() |
[32] |
A. Stéphanou, S. R. McDougall, A. R. A. Anderson, M. A. J. Chaplain, Mathematical modelling of flow in 2D and 3D vascular networks: applications to anti-angiogenic and chemotherapeutic drug strategies, Math. Comput. Model., 41 (2005), 1137–1156. https://doi.org/10.1016/j.mcm.2005.05.008 doi: 10.1016/j.mcm.2005.05.008
![]() |
[33] |
A. Moath, Y. X. Xiao, The influence of tumour vasculature on fluid flow in solid tumours: a mathematical modelling study, Biophys. Rep., 7 (2021), 35–54. https://doi.org/10.52601/bpr.2021.200041 doi: 10.52601/bpr.2021.200041
![]() |
[34] |
R. K. Jain, R. T. Tong, L. L. Munn, Effect of vascular normalization by antiangiogenic therapy on interstitial hypertension, peritumor edema, and lymphatic metastasis: Insights from a mathematical model, Cancer Res., 67 (2007), 2729–2735. https://doi.org/10.1158/0008-5472.CAN-06-4102 doi: 10.1158/0008-5472.CAN-06-4102
![]() |
[35] | M. Mohammadi, C. Aghanajafi, M. Soltani, Numerical Modelling of Drug Delivery in an Isolated Solid Tumor under the Influence of Vascular Normalization. In: D. M. Kilgour, H. Kunze, R. Makarov, R. Melnik, X. Wang (eds) Recent Developments in Mathematical, Statistical, and Computational Sciences in: AMMCS 2019, Springer Proceedings in Mathematics & Statistics, vol 343. Springer, Cham, (2021), 565–577. https: //doi.org/10.1007/978-3-030-63591-6_52 |
[36] |
M. Mohammadi, C. Aghanajafi, M. Soltani, K. Raahemifar, Numerical investigation on the anti-angiogenic therapy-induced normalization in solid tumors, Pharmaceutics, 14 (2022), 363. https://doi.org/10.3390/pharmaceutics14020363 doi: 10.3390/pharmaceutics14020363
![]() |
[37] |
P. W. Sweeney, A. d'Esposito, S. Walker-Samuel, R. J. Shipley, Modelling the transport of fluid through heterogeneous, whole tumours in silico, PLoS Comput. Biol., 15 (2019), e1006751. https://doi.org/10.1371/journal.pcbi.1006751 doi: 10.1371/journal.pcbi.1006751
![]() |
[38] |
F. Moradi Kashkooli, M. Soltani, M. Rezaeian, C. Meaney, M. H. Hamedi, M. Kohandel, Effect of vascular normalization on drug delivery to different stages of tumor progression: In-silico analysis, J. Drug. Deliv. Sci. Technol., 60 (2020), 101989. https://doi.org/10.1016/j.jddst.2020.101989 doi: 10.1016/j.jddst.2020.101989
![]() |
[39] |
D. Tee, J. DiStefano Ⅲ, Simulation of tumor-induced angiogenesis and its response to anti-angiogenic drug treatment: Mode of drug delivery and clearance rate dependencies, J. Cancer Res. Clin. Oncol., 130 (2004), 15–24. https://doi.org/10.1007/s00432-003-0491-1 doi: 10.1007/s00432-003-0491-1
![]() |
[40] |
G. Zhao, W. Yan, E. Chen, X. Yu, W. Cai, Numerical simulation of the inhibitory effect of angiostatin on metastatic tumor angiogenesis and microenvironment, Bull. Math. Biol., 75 (2013), 274–287. https://doi.org/10.1007/s11538-012-9805-2 doi: 10.1007/s11538-012-9805-2
![]() |
[41] |
Y. Cai, J. Zhang, Z. Li, Multi-scale mathematical modelling of tumour growth and microenvironments in anti-angiogenic therapy, Biomed. Eng. Online, 15 (2016), 685–700. https://doi.org/10.1186/s12938-016-0275-x doi: 10.1186/s12938-016-0275-x
![]() |
[42] |
G. Zhao, E. Chen, X. Yu, H. Cui, J. LV, J. Wu, Three-dimensional model of metastatic tumor angiogenesis in response to anti-angiogenic factor angiostatin, J. Mech. Med. Biol., 17 (2017), 1750094. https://doi.org/10.1142/S0219519417500944 doi: 10.1142/S0219519417500944
![]() |
[43] |
J. Wu, Z. R. DING, Y. Cai, S. Xu, G. Zhao, Q. Long, Simulation of tumor microvasculature and microenvironment response to anti-angiogenic treatment by angiostatin and endostatin, Appl. Math. Mech. (English Edition), 32 (2011), 437–448. https://doi.org/10.1007/s10483-011-1428-7 doi: 10.1007/s10483-011-1428-7
![]() |
[44] |
J. W. Baish, T. Stylianopoulos, R. M. Lanning, R. K. Jain, Scaling rules for diffusive drug delivery in tumor and normal tissues, Proc. Natl. Acad. Sci., 108 (2011), 1799–1803. https://doi.org/10.1073/pnas.1018154108 doi: 10.1073/pnas.1018154108
![]() |
[45] |
A. L. Bauer, T. L. Jackson, Y. Jiang, A cell-based model exhibiting branching and anastomosis during tumor-induced angiogenesis, Biophys. J., 92 (2007), 3105–3121. https://doi.org/10.1529/biophysj.106.101501 doi: 10.1529/biophysj.106.101501
![]() |
[46] |
A. R. A. Anderson, M. A. J. Chaplain, C. García-Reimbert, C. A. Vargas, A gradient-driven mathematical model of antiangiogenesis, Math. Comput. Model., 32 (2000), 1141–1152. https://doi.org/10.1016/S0895-7177(00)00196-5 doi: 10.1016/S0895-7177(00)00196-5
![]() |
[47] |
D. Hanahan, J. Folkman, Patterns and emerging mechanisms of the angiogenic switch during tumorigenesis, Cell, 86 (1996) 353–364. https://doi.org/10.1016/S0092-8674(00)80108-7 doi: 10.1016/S0092-8674(00)80108-7
![]() |
[48] |
P. Carmeliet, R. K. Jain, Angiogenesis in cancer and other diseases, Nature, 407 (2000), 249–257. https://doi.org/10.1038/35025220 doi: 10.1038/35025220
![]() |
[49] |
N. Paweletz, M. Knierim, Tumor-related angiogenesis, Crit. Rev. Oncol. Hematol., 9 (1989), 197–242. https://doi.org/10.1016/S1040-8428(89)80002-2 doi: 10.1016/S1040-8428(89)80002-2
![]() |
[50] |
M. A. J. Chaplain, S. R. McDougall, A. R. A. Anderson, Mathematical modeling of tumor-induced angiogenesis, Annu. Rev. Biomed. Eng., 8 (2006) 233–257. https://doi.org/10.1146/annurev.bioeng.8.061505.095807 doi: 10.1146/annurev.bioeng.8.061505.095807
![]() |
[51] | M. A. J. Chaplain, S. R. McDougall, A. R. A. Anderson, Blood Flow and Tumour-Induced Angiogenesis: Dynamically Adapting Vascular Networks, in: T.L. Jackson (Ed.) Modeling Tumor Vasculature, Springer, New York, NY, (2012), 167–212. https: //doi.org/10.1007/978-1-4614-0052-3_8 |
[52] |
M. A. Ghazani, M. Soltani, P. Jalali, R. Hassannejad, A novel numerical and artificial intelligence based approach to study anti-angiogenic drugs: endostatin, Appl. Math. Model., 105 (2022), 258–283. https://doi.org/10.1016/j.apm.2021.12.033 doi: 10.1016/j.apm.2021.12.033
![]() |
[53] |
J. Folkman, The vascularization of tumors, Sci. Am., 234 (1976), 58–73. http://dx.doi.org/10.1038/scientificamerican0576-58 doi: 10.1038/scientificamerican0576-58
![]() |
[54] |
A. Stevens, H. G. Othmer, Aggregation, blowup, and collapse: The ABC's of taxis in reinforced random walks, SIAM J. Appl. Math., 57 (1997), 1044–1081. https://doi.org/10.1137/S0036139995288976 doi: 10.1137/S0036139995288976
![]() |
[55] |
M. A. Gimbrone, R. S. Cotran, S. B. Leapman, J. Folkman, Tumor growth and neovascularization: an experimental model using the rabbit cornea, J. Natl. Cancer Inst., 52 (1974), 413–427. https://doi.org/10.1093/jnci/52.2.413 doi: 10.1093/jnci/52.2.413
![]() |
[56] |
V. R. Muthukkaruppan, L. Kubai, R. Auerbach, Tumor-induced neovascularization in the mouse eye, J. Natl. Cancer Inst., 69 (1982), 699–708. https://doi.org/10.1093/jnci/69.3.699 doi: 10.1093/jnci/69.3.699
![]() |
[57] |
A. L. Bauer, T. L. Jackson, Y. Jiang, Topography of extracellular matrix mediates vascular morphogenesis and migration speeds in angiogenesis, PLoS Comput. Biol., 5 (2009), e1000445. https://doi.org/10.1371/journal.pcbi.1000445 doi: 10.1371/journal.pcbi.1000445
![]() |
[58] |
A. Shamloo, S. C. Heilshorn, Matrix density mediates polarization and lumen formation of endothelial sprouts in VEGF gradients, Lab. Chip., 10 (2010), 3061–3068. https://doi.org/10.1039/C005069E doi: 10.1039/C005069E
![]() |
[59] | T. Browder, C. E. Butterfield, B. M. Kräling, B. Shi, B. Marshall, M. S. O'Reilly, et al., Antiangiogenic scheduling of chemotherapy improves efficacy against experimental drug-resistant cancer, Cancer Res., 60 (2000), 1878–1886. Retrieved from https://aacrjournals.org/cancerres |
[60] |
B. A. Teicher, A systems approach to cancer therapy, Cancer Metastasis Rev., 15 (1996), 247–272. https://doi.org/10.1007/BF00437479 doi: 10.1007/BF00437479
![]() |
[61] |
L. Hlatky, P. Hahnfeldt, J. Folkman, Clinical application of antiangiogenic therapy: Microvessel density, what it does and doesn't tell us, J. Natl. Cancer Inst., 94 (2002), 883–893. https://doi.org/10.1093/jnci/94.12.883 doi: 10.1093/jnci/94.12.883
![]() |
[62] |
D. R. Soto-Pantoja, J. Menon, P. E. Gallagher, E. A. Tallant, Angiotensin-(1-7) inhibits tumor angiogenesis in human lung cancer xenografts with a reduction in vascular endothelial growth factor, Mol. Cancer Ther., 8 (2009), 1676–1683. https://doi.org/10.1158/1535-7163.MCT-09-0161 doi: 10.1158/1535-7163.MCT-09-0161
![]() |
[63] | Y. Yokoyama, M. Dhanabal, A. W. Griffioen, V. P. Sukhatme, S. Ramakrishnan, Synergy between angiostatin and endostatin: inhibition of ovarian cancer growth, Cancer Res., 60 (2000) 2190–2196. Retrieved from https: //aacrjournals.org/cancerres |
[64] |
D. Ozturk, S. Yonucu, D. Yilmaz, M. B. Unlu, Influence of vascular normalization on interstitial flow and delivery of liposomes in tumors, Phys. Med. Biol., 60 (2015), 1477–1496. https://doi.org/10.1088/0031-9155/60/4/1477 doi: 10.1088/0031-9155/60/4/1477
![]() |
1. | Mahya Mohammadi, Mostafa Sefidgar, Cyrus Aghanajafi, Mohammad Kohandel, M. Soltani, Computational Multi-Scale Modeling of Drug Delivery into an Anti-Angiogenic Therapy-Treated Tumor, 2023, 15, 2072-6694, 5464, 10.3390/cancers15225464 | |
2. | Farshad Moradi Kashkooli, Michael C. Kolios, Multi-Scale and Multi-Physics Models of the Transport of Therapeutic/Diagnostic Cancer Agents, 2023, 15, 2072-6694, 5850, 10.3390/cancers15245850 | |
3. | Mohammad Mahmoodi, Ahmadreza Pishevar, Farzaneh Azargoshasbi, Numerical investigation of the pharmacokinetics and pharmacodynamics of the chemotherapeutic drug in avascular and vascular stages of a brain tumor, 2023, 575, 00225193, 111633, 10.1016/j.jtbi.2023.111633 |
Zone | Equation |
Top and bottom sides | θi,j+1=θi,1,forj=Nj& i=2:Ni−1θi,j−1=θi,Nj,forj=1& i=2:Ni−1}θ:c,f,m,n,a |
Left and right sides | θi−1,j=θi+1,j,fori=1& j=1:Njθi+1,j=θi−1,j,fori=Ni& j=1:Njθi,j−1=θi,j+1,forj=1& i=1andNiθi,j+1=θi,j−1,forj=Nj& i=1andNi}θ:c,f,m,n,a |
Parameter | Description | Value | Reference(s) |
D | Random motility coefficient | 0.00035 | [16,19,27] |
ϕ | Haptotaxis coefficient | 0.16 | [27,28] |
βr | EC proliferation coefficient | 7.67 | [39] |
βd | EC loss coefficient | 2.13×10−4 | [39] |
c∙ | TAF concentration threshold in M(c) | 0.2 | [39] |
ν | Uptake coefficient of TAF | 0.1 | [19,28,40] |
ς | Production coefficient of fibronectin | 0.05 | [19,27,32] |
ε | Uptake coefficient of fibronectin due to the MDE | 0.1 | [27,28] |
γ | Uptake coefficient of fibronectin due to the binding to the ECs | 0.1 | [16,19] |
φ | Production coefficient of MDE | 10×10−6 | [27,28] |
θ | Diffusion coefficient of MDE | 0.001 | [16] |
o | Decay coefficient of MDE | 3 | [27,28] |
χ0 | Chemotactic coefficient | 0.38 | [19,27,28] |
δ | Positive constant in chemotactic function | 0.6 | [19] |
υ | Positive constant in matrix density function | 0.2 | [16] |
ρ0 | Reference value of matrix density | 0.35 | [57] |
αa | Positive constant in angiostatin function | 0.38 | [46] |
σ | uptake coefficient of angiostatin | 0.1 | [42] |
Zone | Equation |
Top and bottom sides | θi,j+1=θi,1,forj=Nj& i=2:Ni−1θi,j−1=θi,Nj,forj=1& i=2:Ni−1}θ:c,f,m,n,a |
Left and right sides | θi−1,j=θi+1,j,fori=1& j=1:Njθi+1,j=θi−1,j,fori=Ni& j=1:Njθi,j−1=θi,j+1,forj=1& i=1andNiθi,j+1=θi,j−1,forj=Nj& i=1andNi}θ:c,f,m,n,a |
Parameter | Description | Value | Reference(s) |
D | Random motility coefficient | 0.00035 | [16,19,27] |
ϕ | Haptotaxis coefficient | 0.16 | [27,28] |
βr | EC proliferation coefficient | 7.67 | [39] |
βd | EC loss coefficient | 2.13×10−4 | [39] |
c∙ | TAF concentration threshold in M(c) | 0.2 | [39] |
ν | Uptake coefficient of TAF | 0.1 | [19,28,40] |
ς | Production coefficient of fibronectin | 0.05 | [19,27,32] |
ε | Uptake coefficient of fibronectin due to the MDE | 0.1 | [27,28] |
γ | Uptake coefficient of fibronectin due to the binding to the ECs | 0.1 | [16,19] |
φ | Production coefficient of MDE | 10×10−6 | [27,28] |
θ | Diffusion coefficient of MDE | 0.001 | [16] |
o | Decay coefficient of MDE | 3 | [27,28] |
χ0 | Chemotactic coefficient | 0.38 | [19,27,28] |
δ | Positive constant in chemotactic function | 0.6 | [19] |
υ | Positive constant in matrix density function | 0.2 | [16] |
ρ0 | Reference value of matrix density | 0.35 | [57] |
αa | Positive constant in angiostatin function | 0.38 | [46] |
σ | uptake coefficient of angiostatin | 0.1 | [42] |