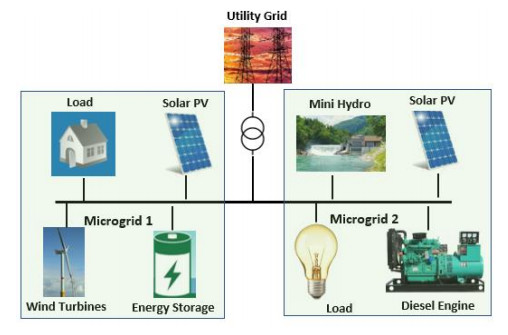
This paper makes a survey about the recent development of optimal control based on adaptive dynamic programming (ADP). First of all, based on DP algorithm and reinforcement learning (RL) algorithm, the origin and development of the optimization idea and its application in the control field are introduced. The second part introduces achievements in the optimal control direction, then we classify and summarize the research results of optimization method, constraint problem, structure design in control algorithm and practical engineering process based on optimal control. Finally, the possible future research topics are discussed. Through a comprehensive and complete investigation of its application in many existing fields, this survey fully demonstrates that the optimal control algorithms via ADP with critic-actor neural network (NN) structure, which also have a broad application prospect, and some developed optimal control design algorithms have been applied to practical engineering fields.
Citation: Xiaoxuan Pei, Kewen Li, Yongming Li. A survey of adaptive optimal control theory[J]. Mathematical Biosciences and Engineering, 2022, 19(12): 12058-12072. doi: 10.3934/mbe.2022561
[1] | W. E. P. Sampath Ediriweera, N. W. A. Lidula, R. Samarasinghe . Ring connected microgrid clusters for improved resiliency in distribution systems with high solar PV penetration. AIMS Energy, 2024, 12(4): 872-904. doi: 10.3934/energy.2024041 |
[2] | T S S Senarathna, K T M Udayanga Hemapala . Review of adaptive protection methods for microgrids. AIMS Energy, 2019, 7(5): 557-578. doi: 10.3934/energy.2019.5.557 |
[3] | Mohamed G Moh Almihat . An overview of AC and DC microgrid energy management systems. AIMS Energy, 2023, 11(6): 1031-1069. doi: 10.3934/energy.2023049 |
[4] | Pooja Khandare, Sanjay Deokar, Arati Dixit . Relay Coordination and Optimization techniques using DWT-Differentiation Algorithms for Fault Detection in Microgrid. AIMS Energy, 2020, 8(4): 563-579. doi: 10.3934/energy.2020.4.563 |
[5] | Ahsan Iqbal, Ayesha Ayoub, Asad Waqar, Azhar Ul-Haq, Muhammad Zahid, Syed Haider . Voltage stability enhancement in grid-connected microgrid using enhanced dynamic voltage restorer (EDVR). AIMS Energy, 2021, 9(1): 150-177. doi: 10.3934/energy.2021009 |
[6] | Aaron St. Leger . Demand response impacts on off-grid hybrid photovoltaic-diesel generator microgrids. AIMS Energy, 2015, 3(3): 360-376. doi: 10.3934/energy.2015.3.360 |
[7] | Mohamed G Moh Almihat, MTE Kahn . Centralized control system for islanded minigrid. AIMS Energy, 2023, 11(4): 663-682. doi: 10.3934/energy.2023033 |
[8] | Konstantinos Christidis, Michael Devetsikiotis . Adaptive multi-tiered resource allocation policy for microgrids. AIMS Energy, 2016, 4(2): 300-312. doi: 10.3934/energy.2016.2.300 |
[9] | H. H. H. De Silva, D. K. J. S. Jayamaha, N. W. A. Lidula . Review on design and control of solid state transformer based microgrids. AIMS Energy, 2019, 7(6): 901-923. doi: 10.3934/energy.2019.6.901 |
[10] | Paul K. Olulope, Oyinlolu A. Odetoye, Matthew O. Olanrewaju . A review of emerging design concepts in applied microgrid technology. AIMS Energy, 2022, 10(4): 776-800. doi: 10.3934/energy.2022035 |
This paper makes a survey about the recent development of optimal control based on adaptive dynamic programming (ADP). First of all, based on DP algorithm and reinforcement learning (RL) algorithm, the origin and development of the optimization idea and its application in the control field are introduced. The second part introduces achievements in the optimal control direction, then we classify and summarize the research results of optimization method, constraint problem, structure design in control algorithm and practical engineering process based on optimal control. Finally, the possible future research topics are discussed. Through a comprehensive and complete investigation of its application in many existing fields, this survey fully demonstrates that the optimal control algorithms via ADP with critic-actor neural network (NN) structure, which also have a broad application prospect, and some developed optimal control design algorithms have been applied to practical engineering fields.
Electrical demand has been continually increasing while fossil fuels have been depleting, which leads to fluctuating electricity rates. At the same time, with the concerns on greenhouse gas emissions and awareness of sustainable energy growth, attention has been paid to renewable power generation to satisfy future energy demands [1]. Renewable power sources such as solar photovoltaic (PV) arrays, micro wind turbines, biomass power plants and fuel cells are being used for power generation. The use of these renewable power sources, which are usually connected to the distribution network, is called distributed generation (DG) [2,3]. To facilitate the higher-order integration of renewable power generation into the distribution system, it is necessary to have smart and intelligent distribution networks with proper controllability to achieve the required level of system reliability. Thus, the concept of microgrids has been emerging as a solution [4,5].
A microgrid can be defined as a small-scale power system containing DG sources and energy storage elements designed to supply the power to a local area within an identified boundary. The microgrid can be connected to the main grid as a controllable load/generator, offering islanded and grid-connected operation [6,7,8]. By now, a lot of microgrid projects have been started to promote rural electrification and energy sustainability.
With the increasing penetration of distributed energy resources (DERs) and high uncertainties, it becomes complex and infeasible to coordinate a large number of distributed generators in a single microgrid [9]. Complexity in managing the increasing penetration of renewable power generation and the quest for power quality have continued to direct more attention onto smart grids [10,11]. The existing distribution network can be transferred to a smart grid having a more reliable, efficient, sustainable and customer-interactive status by adding metering, protection and communication infrastructure. Among the smart features of smart grids, self-healing is an important feature in improving the system reliability, as the smart grid can continuously detect and respond to faults and restore feeders with minimum human invention.
A microgrid cluster, on the other hand, is a self-healing reconfiguring technique in which the distribution network is divided into smaller, controllable grids [12,13]. It incorporates several geographically close microgrids into a single network of interconnected microgrids. Microgrid clusters offer numerous economic benefits to both the utility grid and the microgrids. Each microgrid will have the benefits of increased reliability, stability and reduced cost [14,15,16]. During a failure of the main grid, the individual microgrids may fail to meet the demand continuously in the microgrid due to intermittency of renewable-energy-based distributed generation. Having interconnections among each other, the microgrids in the cluster overcome this barrier by mutually supporting each microgrid during islanded operation. Figure 1 illustrates an example of a microgrid cluster consisting of two microgrids. However, interconnecting several microgrids into a cluster requires a control and energy management architecture to allow safe and reliable operation of the entire cluster during the grid-connected and islanded modes of operation.
The main objective of this paper is to present a comprehensive and analytical review of the microgrid clustering architecture and protection techniques. Furthermore, an in-depth investigation has been made to identify the currently available control concepts and test systems on microgrid clusters. In addition, existing grid synchronization methods are presented to identify their suitabilities for interconnected microgrids. Furthermore, the advantages and challenges of networked operation are discussed.
The study presented in [17] was focused on the optimization of the energy management of networked microgrids, along with control methods for regulating voltage, frequency and power under different operation scenarios.
Possible multi-microgrid layouts to form a grid of microgrids, along with a comparison among them considering different aspects, were explored in [18]. Furthermore, new business models to promote the installation of distributed energy resources were discussed. However, microgrid layouts were categorized mainly by considering the modes of operation: grid-connected operation and islanded operation. Therefore, it lacked an in-depth analysis of the recent research on possible microgrid layouts. In [19], common interconnected microgrid layouts were briefly summarized, and energy management systems relevant to the most used optimization objectives, the operation timescales and the scheduling optimization structures were analyzed. However, control and challenges associated with different layouts of microgrids were not included in this review. Energy trading among multiple microgrids and energy-market designs to facilitate energy trading among the participating producers and customers were discussed in [16]. Even though an in-depth review of the strengths and drawbacks of the existing protection techniques were not given, [16] briefly discussed the existing protection techniques for interconnected microgrids. The review presented in [20] explored major issues such as stability, protection coordination and the privacy of private and community microgrids, along with failure of the communication system. However, it did not highlight the design perspective of interconnected microgrids. The study in [21] provided a comprehensive review of distributed control and communication strategies in networked microgrids. Communication reliability issues, including data timeliness, availability and accuracy, which would affect the performance of control strategies in network microgrids, were also discussed in [21].
Compared with previous review studies in [17,18,19], this paper presents a detailed evaluation of the robustness of the existing main types of microgrid cluster architectures. Also, compared to the previous review in [16], protection techniques utilized in microgrid clusters are evaluated through their advantages and limitations to identify the techniques that are effective and feasible for ensuring fast and accurate isolation of faults.
The paper is organized as follows: In Section 2, reported test systems on microgrid clusters and their objectives and clustering techniques are discussed. Section 3 introduces possible and existing architectures for clustering microgrids. Line technologies and interconnection technologies used in interconnecting microgrids are reviewed in Section 4 and Section 5, respectively. Section 6 presents an analysis of the existing architectures, considering four aspects: 1) scalability, 2) stability, 3) reliability and 4) protection. Line technologies and interconnection technologies in the view of implementing the architectures are discussed under this section. Control hierarchy in implementing the objectives of the interconnected microgrids is discussed in Section 7, and controlling concepts used to implement control objectives of microgrid clusters are discussed in Section 8 along with their advantages and disadvantages. Section 9 presents the possible grid synchronization methods. Existing protection techniques for interconnected microgrids, along with associated advantages and disadvantages, are analyzed in Section 10. The paper concludes with suggestions for possible future research directions.
The concept of a microgrid cluster is emerging as one of the promising alternatives to increase the reliability of power systems with increasing penetration of DERs. Voltage and frequency stability issues with high penetration of renewable power generation, weather-related events and other day-to-day incidents (equipment failures, control issues, etc.) can result in partial failure or total failure of the network, leading to blackouts [22] and huge financial losses. Microgrid clusters can provide electricity supply with improved power quality and reliability [23] at a reduced cost of generation while providing ancillary services. During emergencies, islanded microgrid clusters can supply power to the critical loads for a longer period of time through the interconnection among microgrids; also, it can provide black start support to the power station to restore the power in the main grid.
Microgrid clustering is being identified as a trending solution for modern reliable power grids, and a significant amount of research has been conducted, with different test systems identifiable in the literature [24,25]. Based on the method of creating the network, the test systems can be divided into two main categories: 1) test systems from dividing an existing test system into interconnected microgrids [26,27,28,29,30] and 2) test systems from adding microgrids to an existing test system [31,32,33,34,35].
In the first category, the topology of the cluster is the same as the original system, as shown in Figure 2, and the capacity of the existing DERs has not been changed. However, the locations of them have been changed, while adding new resources to optimize the objectives.
In the second category, new microgrids have been introduced to the existing test system at different locations, as shown in Figure 3. In this method, there may be resources that do not belong to any of the connected microgrids. Individual microgrids have been introduced to optimize the entire network, subjected to different objectives.
In both of the test systems, complete sets of network data are not reported. Therefore, adopting them into new studies and research becomes difficult. Objectives and the methods used in developing the test systems are illustrated in Tables 1 and 2, respectively. Test systems are used to perform various simulation studies, such as power flow, control, stability, protection and energy management, for microgrid clusters. Microgrid clusters help individual microgrids to operate more reliably during islanded operations while providing numerous economic benefits to both the utility grid and the microgrids in the cluster. However, reported studies on test systems have considered IEEE test bus systems in deriving test systems of networked microgrids. Still, there is no research reported on developing microgrid clusters based on an actual distribution feeder integrating DERs as required. Dynamics of the distributed generation and unbalanced nature of loads can be expected in actual systems.
Reference | Objective | Method |
[20] | To maximize the generation under the constraints of distribution system and microgrids | Mixed-integer linear program subjected to different operating conditions of microgrids and distribution network |
[21] | Voltage control and power management | A two-level control structure Primary layer coordinates the load sharing among DERs Second layer controls the power exchange among microgrids and restores voltage to reference values Analysis of eigenvalues to select the control parameters of the entire distribution network |
[22] | Optimal dynamic planning of interconnected microgrids | Probabilistic approach for different cases with the locations of DERs and number of microgrids |
[23] | To minimize the total load shedding of a distribution network under natural disasters | Linear programming to find the optimal locations of DERs |
[24] | To study the dynamics of the system with switching transients of network microgrids | No mechanism for clustering Grid-friendly appliance controllers to mitigate the problems with transients |
[30] | Robust microgrid clustering in a distribution system with inverter-based DERs | Concept of interrelationships between ecological species translates into the dynamic behavior of DERs Analysis of eigenvalues to find the optimal locations of DERs |
Reference | Objective | Method |
[25] | To study the operation of networked microgrids | No mechanism for clustering Different operational scenarios are simulated for different modes of operation |
[26] | To reduce the total operational cost of microgrids To smooth the load curves of the distribution network |
A two-stage optimization model subjected to the changes of generation cost |
[27] | To assess the stability of networked microgrids with uncertainties of DERs | Eigenvalue analysis for different penetrations of six microgrids |
[28] | To introduce an energy management system for microgrid clusters to increase profit | A stochastic bi-level optimization problem |
[29] | To introduce an energy management system for microgrid clusters | Stochastic decentralized bi-level optimization method for islanded and grid-connected modes of operation |
Therefore, to make the concept of microgrid clusters a widespread reality, it is necessary to continue the discussion and research on developing robust microgrid clusters based on actual distribution feeders in operation.
Microgrids form a physical network to achieve local and global objectives through the interactions between the microgrid and the main grid. The layout among the microgrids depends on the requirements and agreement among microgrids. Microgrid clusters can be classified into three types based on the network formation: (1) parallel connected, (2) ring connected and (3) mesh connected.
In the parallel-connected microgrid cluster architecture, all the microgrids are connected in parallel to the main grid, thus making a radial or star topology.
In the star-connected topology, several microgrids are attached to the main grid through a common bus [37,38]. A typical example of a star-connected architecture is shown in Figure 4. If any microgrid has surplus power, it is transmitted to the adjacent microgrids in the cluster or to the main grid. On the other hand, if a microgrid experiences a deficit in generation, power is acquired through neighboring microgrids or the main grid. In the islanded mode of operation, the same common bus bar can be used for power sharing.
In the radially connected topology, large microgrids can be connected directly to the main grid through separate electrical connections, with the separate point of common coupling as in Figure 5, and power sharing among the microgrids can only occur through the main grid. However, in islanded operation, all the microgrids should be self-sufficient, as there are no interconnections among microgrids [18].
If there are small microgrids in the cluster, they are connected to a large microgrid through a separate common bus bar, as shown in Figure 6, and they will communicate with the large microgrid for power sharing. This can be categorized under the radially connected microgrid cluster. In the cluster, a large microgrid among the group is considered the dominant microgrid, which is responsible for maintaining the power flow supporting other microgrids during power deficiencies in the islanded mode of operation. However, a single-point failure in the dominant microgrid can lead to the failure of operation of the entire microgrid cluster [17].
The ring architecture considers a concept where each microgrid in the cluster is connected to two adjacent microgrids in the shape of a ring, and energy and information can be shared between them [39,40,41,42,43,44]. Figure 7 presents the basic layout of the ring architecture.
Several microgrids can be connected to the main grid, with separate points of common coupling for each, where power exchanges directly with the main grid [45,46]. Neighboring microgrids can communicate with each other to exchange power between them. The ring network has improved redundancy and stability compared to the radial structure. With secure fault isolation and improved reliability, the ring architecture becomes a suitable option for microgrid clustering. However, the network is comparatively complex, and the power exchange can occur between two adjacent microgrids and directly with the main grid. Therefore, controlling and protection of the system become complex [46].
In the mesh structure, microgrids are interconnected with each other, making a complex network [46,47,48,49]. Therefore, each microgrid is connected to all neighboring microgrids through a power transmission and communication network, as shown in Figure 8.
Therefore, each microgrid can exchange power with the main grid and the neighboring microgrids. Having redundant connections, this configuration inherits improved operational performance with improved stability and reliability. However, control and protection of this type of complex network are challenging tasks [30]. Dispatching and scheduling of distributed generation of each microgrid are affected by all the connected microgrids and its local demand and supply [46,50]. As each microgrid is connected to others, power-sharing management is difficult. In grid-connected operation, the cluster can provide ancillary services to the main grid [20].
Microgrids in the cluster can be connected, and power transmission can be in the form of AC and/or DC. Efficiency, power transmission capacity, voltage drops, stability, power quality, protection systems and the overall cost depend on the line technology (AC and/or DC) being adopted [51,52,53,54].
Table 3 lists a summary of the advantages and disadvantages of line technologies applied in microgrid clusters. A detailed analysis of line technologies used in networked microgrids, along with their advantages and disadvantages, is available in [55].
Advantages | Disadvantages | |
AC | Transformers can be used for changing voltage levels and for isolation Well established protection techniques can be used |
Requires power and frequency control Transient and dynamic instabilities High operational cost due to losses, skin effect and dielectric losses Need for synchronism and large power oscillations Load-dependent voltage control Applicable for short distances (<50 km) |
DC | Increased efficiency Losses are minimized with constant currents and fewer conversion stages Low variety of faults No electromagnetic interference Low operational cost and low cost associated with conductors and insulators Better transient and dynamic stability No reactive power controls Long-distance bulk power transmission |
High costs are associated with the interfaces Fault isolation is difficult due to the unavailability of a zero-crossing point |
Microgrids in the cluster can be AC, DC or hybrid. For the AC systems, three-phase transmission is more efficient than single-phase transmission. Due to the reduced losses and the absence of reactive power flows, DC microgrid clusters have gained greater attention. In [56], a power electronic device called a power router was used to connect microgrids to the DC grid, as shown in Figure 9.
Power transformers and power electronics converters are usually used to interconnect different line technologies [18]. The dual H-bridge converter can facilitate bidirectional power flow among DC/DC microgrids. It uses two H-bridges and a high-frequency transformer. A phase shift has been introduced between the two bridges to control the active power [57,58]. The dual half-bridge topology can also be used for the DC/DC conversion. Even though its current ratings are twice the rating of switches on dual H-bridges, it reduces the voltage stress [59,60].
In [61], a bidirectional converter with an H–bridge at the low voltage side and a push-pull topology at the high voltage side was proposed. Voltage source converters can be used to realize AC-DC interconnection. Table 4 summarizes the advantages and disadvantages of interconnection technologies. Transformers can be used to interconnect AC systems at different voltage levels while providing electrical isolation at a low cost. However, they offer less controllability in changing the voltage levels. Conversely, power electronics converters can be used to connect both AC and DC line technologies with high controllability. However, the cost is considerably high. Electrical isolation and controllability both can be achieved by combining a converter and a transformer.
Advantages | Disadvantages | |
Transformer | Reliable and cost-effective method to change AC/AC voltage levels | Low controllability Low operational performance |
Converter | Better voltage regulation High voltage controllability Can be applied with the interconnection of any line technology (AC/AC, AC/DC or DC/DC) |
Protection requirements Dependency on communication Expensive solution |
Microgrid cluster architectures can be compared in terms of scalability, protection, reliability and stability to evaluate their performances.
The term scalability considers the capability of a microgrid cluster to accept new DERs and microgrids. It accounts for the possibility of the growth of existing microgrids and connection of new microgrids to the cluster. With the growth of microgrids, power flow, as well as power generation and consumption, in each microgrid would change. This affects the power import and export requirements. Power exchange among the microgrids is limited by the capacity of the interconnection and line technology, which could be improved with an additional cost to meet the augmentation requirements.
However, if an individual microgrid in the cluster becomes more self-sufficient, the power exchanges among the microgrids and from/to the main grid would be reduced, and the scaling capacity of the system could be increased without any infrastructure augmentation. When the growth of the entire microgrid cluster is considered, if the cluster can be operated even in islanded operation without affecting the local generation-demand balance, then the scalability is better. When the growth of an individual microgrid is considered, it is more favorable if the microgrid is connected to other microgrids in the cluster at several points, as then it can facilitate more energy exchange. Hence, mesh architecture has better acceptance in terms of the growth of the microgrids in the cluster (or scalability).
When new microgrids are connected to the existing microgrid cluster, the number of interconnections and the complexity of the system will increase. The parallel structure is the simplest one in this regard. However, in some parallel configurations, power exchange through the main grid or the dominant microgrid can make them saturate. Therefore, possible saturation can limit the advantage of simplicity in scalability. This drawback is avoided in the ring structure because each microgrid is connected to two adjacent microgrids, and its complexity is lower than that of the mesh structure. Table 5 compares the scalabilities for the different layout architectures.
Layout architecture | Microgrid cluster growing capacity | Individual microgrid growing capacity | Overall scaling capacity | Complexity |
Parallel | Low | Medium | Low | Low |
Ring | High | Medium | Medium | Medium |
Mesh | High | High | High | High |
With the growth of the microgrids, the power flow will increase through interconnections, and this will lead to unacceptable voltage drops in AC lines, reducing the power quality. Compared to the AC transmission, DC transmission offers a higher transmission capacity, with minimum voltage drops [53,62]. Therefore, from an economic point of view, DC transmission lines with high capacity can be recommended.
In an event of the interconnecting of a new microgrid to the system, even though the power transformers can be overloaded, power converters should be replaced with new, expensive converters according to the increased power exchange requirements. Therefore, economic factors limit the growth of microgrids if the power electronics interfaces, which offer higher controllability, are being used.
Stability refers to the ability of the microgrid cluster to return to the steady state after a disturbance, and it refers to voltage stability, frequency stability and rotor angle stability [46]. The stability of the microgrid cluster mainly depends on the external main grid, and it is supported by the interconnections among the microgrids in the cluster.
The external grid may have synchronous generators, storage systems and other equipment to ensure the balance between demand and generation. The microgrid cluster, while connected to the external grid, provides enhanced network stability to the microgrids. However, when the microgrid cluster is islanded, the stability of a microgrid is supported by other microgrids in the cluster.
In islanded operation, the stability of the cluster mainly depends on the power reserves, synchronous inertia, reactive power sources, etc. Therefore, unavailability of a single microgrid can be critical for the stability of the cluster, because it can disconnect the critical power flow balancing sources in the cluster. In the parallel architecture, a fault in the interconnection can completely isolate a microgrid, breaking the unique access to the rest of the microgrid and making the cluster less stable. Table 6 compares the levels of stability of the different architectures of microgrid clusters.
Layout architecture | Stability |
Parallel | Medium |
Ring | High |
Mesh | High |
The stability of AC microgrids is ensured if the voltage and the frequency are within acceptable limits. The voltage stability is usually associated with the reactive power flow, and the frequency stability is associated mainly with the active power flow. The absence of reactive power flow favors the DC line technology from the stability point of view.
The quantity and sizes of connected synchronous generators can affect the magnitude and the dynamics of the system frequency [63]. With the integration of renewable power generation sources such as solar PV arrays via power electronics devices into microgrids, the synchronous inertia of the system decreases depending on the controls being used. Thus, the integration of the power electronics converters into the system can reduce network stability. However, control algorithms integrated with converters can mimic the behavior of the conventional synchronous generators. Thus, it becomes possible for a microgrid to increase or decrease the power exchange in response to a frequency deviation. Also, they can effectively control the reactive power, regulating the voltage levels.
The number of elements inside microgrids, how they are controlled and how the microgrids are connected can decide the reliability of the microgrid cluster. Once a contingency occurs, the affected area will be disconnected by the protection scheme, and a part of the power consumed or generated will be no longer associated with the microgrid cluster. Therefore, the reliability of the cluster mainly depends on the connection parts and the redundancy of the system.
An architecture with many interconnections to the main grid will have the advantage in a contingency. When the main grid becomes stronger, it decreases the impact on stability of a grid-connected microgrid cluster [65]. Connections with the main grid and other microgrids become a drawback in terms of reliability if the protection scheme cannot ensure fast fault detection and isolation. Assuming the microgrids are properly connected and coordinated to ensure fast fault isolation, a qualitative evaluation of the reliability depending on the microgrid architecture is summarized in Table 7.
Layout architecture | Reliability |
Parallel | Medium |
Ring | High |
Mesh | High |
Studies have shown that the failure rate is similar with both AC and DC technologies [66]. Therefore, it is not possible to identify the most reliable technology out of AC and DC. On the other hand, as transformers can be overloaded, their failure rate is significantly lower compared to power electronic converters [18].
The protection system should protect the microgrid cluster against electrical failures, isolating the fault area without affecting the rest of the system. Also, it should minimize the damage that can happen to the assets and facilities in the affected area [67,68].
In a cluster of microgrids, each microgrid will import and export power to/from other microgrids and the main grid, leading to a bidirectional power flow. Therefore, protection and coordination should be designed accordingly [69,70,71,72,73]. In the parallel connected architecture, there may be a unique path to the main grid or to another neighboring microgrid. In the event of a failure at the interconnection point, the protection system can effect the complete isolation of the microgrid, reducing the reliability. However, protection coordination is very simple, guaranteeing good selectivity, discrimination, accurate sensitivity and fast protection actuation. In ring and mesh architectures, the microgrids are operated in closed-loop operation, having more interconnections. Therefore, a robust protection system and a good communication system are required. Due to the high complexity, the cost will also increase [74].
Table 8 compares the fulfillment of protection requirements for the different layout architectures. The protection scheme should detect the direction of the power flow and the fault current level, which depend on the network and available generation. When a fault occurs within a microgrid, the fault current is supplied by local distributed generation and other microgrids in the cluster. Under DC line technology, power electronics converters are required, and they cannot withstand high fault current as power transformers do. Therefore, fast fault detection and isolation systems are required to minimize the damage that can occur to the infrastructure. More sensitive relays and breakers should be employed for fast fault detection and isolation. Also, with DC technology, it is difficult to interrupt high fault current, as it makes a large arc during a fault, and contactors within the breakers have to move far away if fault current limiting technology is not incorporated. However, in AC, fault isolation at zero crossing reduces the intensity of the arc [75].
Layout architecture | Selectivity applicability | Coordination requirements | Overall protection difficulty |
Radial | High | Low | Low |
Ring | Medium | Medium | Medium |
Mesh | Low | High | High |
Power transformers can withstand a fault for about two to five seconds, and it depends on the rated power of the transformer. Fuses are being used to protect small transformers, while differential, earth fault, overvoltage, current, thermal, overload, etc. protections are being used to protect large transformers. However, power converters can withstand overvoltage and overcurrent for a lower time duration than transformers, and they demand more accurate protection, making the cost of protecting power converters higher [54,55].
Optimal power sharing and regulation of the voltage and frequency in the microgrid cluster are challenging tasks due to different operating scenarios and system architectures [76]. There are two major control structures being used: namely, (1) hierarchical structure and (2) distributed structure.
In the hierarchical control structure, control objectives are divided into three control layers [20]. Figure 10 illustrates a hierarchical control structure used in microgrid clusters.
In the primary control layer, local control operations are implemented. Voltage and frequency regulation, active and reactive power control, islanding detection and local protection are achieved in the primary control layer [77]. The secondary control layer ensures voltage and frequency regulation due to the deviations done by the control actions in the primary level. Other than that, grid synchronization, optimal operation of DERs and real-time energy management are performed by the secondary control layer [78]. The distributed network operator in collaboration with the third control layer performs the market operations, such as economic dispatch and unit commitment.
The distributed control structure considers a two-level structure [20]. Figure 11 illustrates a typical distributed control strategy adopted in microgrid cluster control. In the distributed primary control layer, voltage, current and frequency regulation, islanding detection, grid synchronization and load power management are at the microgrid level in a fully distributed manner [27]. In the secondary control level, each microgrid shares the information with other microgrids and the distributed network operator to perform the market operations and optimum power flow.
A comparison of some of the features of hierarchical and distributed control strategies is given in Table 9. A detailed analysis of various control strategies used in networked microgrids, along with their advantages and disadvantages, is available in [20]. Even if the hierarchical control strategy can provide the best possible supervision for a well-defined network, it does not support the growth of the microgrid cluster. However, with the distributed control strategy, more flexibility can be achieved to expand the existing network with "plug-and-play" functionality. The hierarchical control strategy can guarantee better economic operation of the microgrid cluster compared to distributed control.
Feature | Hierarchical control | Distributed control |
Reliability | Moderate | High |
Plug and play | Low | High |
Flexibility/Expandability | Low | High |
Communication bandwidth | Low | High |
Time/Space complexity | High | Low |
Design complexity | Complex | Simple |
However, flexibility and scalability are the most important aspects of a robust microgrid cluster. Therefore, research has been focused mostly on the distributed control strategy, which is more robust in the operational aspect compared to the hierarchical control strategy.
Control layers of distributed and hierarchical control structures have different time scales. Normally, the operating time of the primary layer is in the range of the microsecond scale, and it increases and scales up with the number of layers. So, the control signal may be lost or get delayed during the transmission due to communication delay caused by the scaling property of the control structures. Furthermore, the high sampling rate and control strategy adopted can worsen the situation, leading to instability of the microgrid cluster [79].
Information and command signals to implement the control objectives of the microgrids are transmitted as data packets via the communication network. If the data is encoded with the IEC 61850 protocol, then the size of the data packets is confined to between 32 and 200 bytes. Signals associated with protection and control are critical for the safety and stability of microgrid clusters, and they do not entertain latency and data loss. However, control objectives on the secondary and tertiary layers of control structures do not expect accurate data, as for those in the first control layer. Therefore, it can accommodate considerable latency [80,81,82].
Table 10 lists a summary of maximum latency requirements for different control objectives of microgrids according to two different protocols.
Task | IEC 61850 | ETSI open SG protocol |
Protection | 4 ms | 1–10 ms |
Control | 16–100 ms | 100 ms |
Messages requiring immediate action | 1A: 3 or 10 ms 1B: 20 or 100 ms |
Not specified |
Time synchronization | Accuracy | Not specified |
Monitoring | 1 s | 1 s |
Operation and maintenance | 1 s | Not specified |
Any delay or data corruption degrades the efficient operation of the microgrid cluster, reducing stability and safety. Cyberattacks on microgrids can be classified into three major attacks: (1) data availability, (2) data integrity and (3) data confidentiality.
Cyberattacks can create economic and technical/physical issues in microgrid clusters. Therefore, cybersecurity is an important aspect to be considered in the operation of networked microgrids. Different cybersecurity mechanisms can be implemented to improve the resilience of microgrid clusters by incorporating simple cyber hygiene principles [83]. More details on the cybersecurity of microgrid clusters can be found in [84].
During the discussion of main control structures, it was identified that in each control layer, there are control objectives being used to coordinate the operation of the microgrid cluster. Each control objective can be implemented using four main controlling concepts: namely, (1) centralized control, (2) decentralized control, (3) hybrid control and (4) distributed control.
In the centralized control concept, all the micro-source controllers and load controllers are handled by a central controller using a bidirectional communication system, as shown in Figure 12(a). The central controller receives the local information, such as current and voltage measurements and load and demand data, from each microgrid in the cluster and then processes the data within it, executing the algorithms considering the objectives and constraints [16].
Then, control actions are sent back to the microgrids, and they are executed by the local controllers. Therefore, the central controller manages the power flow among the microgrids and the load and demand balance in the system. Also, it participates in economic aspects while keeping stability in the system. Therefore, the overall benefit of the system is maximized. However, the central controller has a high computation burden, and with the increasing number of microgrids, with high renewable penetration, the central controller can fail in some situations. Failure in the central controller can lead to the total failure of the entire cluster [85,86,87]. For this reason, the centralized control concept is not very popular in the implementation of microgrid clusters.
The decentralized control concept considers a concept where each microgrid in the cluster determines its control actions independently. The local controllers of each microgrid process the data provided by microcontrollers and load controllers, with a reduced burden compared to the central controller in the centralized approach, and send the control actions to local controllers. Figure 12(b) shows the control structure of the decentralized approach. A microgrid can operate autonomously, and control actions can be generated for the optimal operation of each microgrid without relying on any communication among individual microgrids. Therefore, each local controller has the responsibility of collecting necessary information in order to optimize the microgrid operation. Therefore, decentralized control increases the stability of each microgrid in the cluster with a reduction in communication failures. Also, due to the autonomous operation capability, the cluster can scale in size. A failure in a microgrid does not affect the operation of the entire microgrid cluster [88]. The droop control, as an effective decentralized method, could accomplish power sharing among different sources in AC and DC microgrid clusters without relying on the communication network [89,90]. However, the control of the renewable energy resource in droop control mode rather than maximum power point tracking (MPPT) mode leads to poor utilization of renewable energy sources. A decentralized control strategy is proposed in [89] for a hybrid AC/DC microgrid cluster to realize autonomous power exchanges between sub-grids in a single microgrid and among microgrids. Considering the restriction of the energy storage, an adaptive droop control method was proposed to coordinate the power sharing via a DC bus in the hybrid cluster
However, when it comes to the entire cluster, with the decentralized control, the optimum operation cannot be guaranteed, due to the absence of communication among microgrids [91,92]. Each microgrid tries to work on the individual objectives without knowing the information of other neighboring microgrids, which increases the competitiveness across the cluster. Therefore, this structure is more suitable for a microgrid cluster with different owners for individual microgrids.
The hybrid control concept has the features of both the centralized and decentralized concepts, resulting in a central controller at the microgrid cluster level and local controllers at the microgrid level [93]. The control structure is similar to the centralized control structure shown in Figure 12(a). Local controllers of each microgrid perform energy management while optimizing the operation of a particular microgrid. The central controller is informed in case of a surplus or deficit of energy. Therefore, the central controller comes into action and negotiates with microgrids to ensure the optimal state of the cluster and the generation-demand balance [41,42]. This structure has become popular due to its flexibility and low operational costs.
The distributed control concept considers a two-level operation to optimize the microgrid cluster. Central controllers, called central agents, and micro-controllers and local controllers of microgrids take part in the control concept via two-way communication, as shown in Figure 12(c). Each agent is connected to the neighboring agents, making it possible to share the information of each microgrid with other agents. Therefore, the operation of each microgrid in the cluster is controlled and coordinated by multiple dispersed central agents. This structure eliminates the competitiveness that exists in the decentralized structure, and the optimal operation can be achieved [94].
However, since the data of each microgrid are being shared among the agents, security is compromised [94,95].
A microgrid cluster is expected to operate in both islanded and grid-connected modes, and the system should allow a seamless transition between the two operating modes. The voltage phasors on both sides of the point of common coupling should be synchronized with each other before an islanded microgrid cluster is reconnected to the main grid. The mismatch can create an excessive transient current in the cluster, which depends on the cluster topology and the level of mismatch [96]. The grid voltage phasor is uncontrollable. Therefore, the microgrid cluster side phasor is controlled to match the voltage phasor, which has three components: magnitude, frequency and phase shift. Previous works related to synchronizing the microgrid clusters are scarce. However, several algorithms have been used in previous studies to synchronize a single microgrid with the main grid. These include methods involving the discrete Fourier transform (DFT), phase-locked loops, nonlinear least squares and artificial intelligence, as discussed below.
The discrete Fourier transform (DFT) can be used to transform a time domain waveform into a frequency domain with time information [97,98]. A recursive DFT technique was proposed to filter incoming grid voltage. Even if this technique offers a high degree of resistance against noise, a phase shift can occur between the filtered voltage and the grid voltage [99]. Therefore, an improved phase detection method, named sliding DFT, was proposed to get a robust performance with fast transient response under grid contingencies [100]. This method is based on a simple operation and requires less time to extract a single frequency component.
A phase-locked loop (PLL) is the simplest effective and robust synchronization mechanism to track voltage, phase angle and frequency [101]. It has been used in a wide range of applications, such as control systems, communications and instrumentation [102,103]. As shown in Figure 13, a PLL has a non-linear closed-loop feedback control system, and it consists of three main blocks: 1) a phase detector (PD), 2) a loop filter (LF) and 3) a voltage controlled oscillator (VCO). The phase detector compares the two input signals, and the error signal is filtered by the loop filter. It is fed to the voltage controlled oscillator to generate the output phase. This feedback control system continues until the phase error becomes minimal, and then the output phase is locked. However, the performance of the system decreases when the power electronics converters are integrated with weaker grids. This situation can be worsened with the presence of voltage imbalances and harmonic distortion. Therefore, numerous improvements were introduced to mitigate these issues [104,105].
The nonlinear least squares method aims to minimize the square error between the modeled and measured signal. However, the performance of the algorithm can be affected by the choice of sampling frequency [41], and the estimation of the harmonic content of the periodic signal can make the algorithm complex. A tuned resonator-based filter bank was proposed to solve for periodic signal estimation in [106].
Artificial neural networks can be used in conjunction with the least-squares technique to identify phase, amplitude, frequency and harmonic deviations in power systems [101]. Results have shown that the method proposed in [107] has a very fast response and a high convergence rate.
The electrical protection system of a microgrid cluster should be properly designed for reliable operation in both grid-connected and islanded operations. With microgrid clusters, bidirectional power flow can be seen among neighboring microgrids and between the main grid and microgrids.
Use of protection devices and coordination should be done properly to avoid protection problems such as false tripping and blinding protection [108]. Also, conventional protection schemes implemented on a single microgrid cannot be used to solve the protection problems of microgrid clusters with more interconnections among microgrids. In the event of a fault, the protection system should quickly isolate the fault section with the operation of the remaining healthy system. Even though the literature on electrical protection of microgrid clusters is scarce, a few studies can be found discussing the electrical protection of parallel connected microgrid clusters. However, most of them have focused only on either the islanded operation or grid-connected operation of the microgrid cluster, as discussed in the following subsections.
The magnitude and phase differences of the admittances of different feeders before and after a fault were used to develop a protection scheme in [109] for detecting internal faults in microgrid clusters. Feeders are classified as double-terminal feeders, single-terminal feeders (DG feeders) or load feeders, depending on their location. Three criteria were proposed for determining the type of feeder on which the fault occurs. At three different locations of a parallel connected cluster architecture in grid-connected operation, phase-phase-to-ground faults are simulated, taking into account the interconnections and layout of the DG sources and microgrids in the cluster. The magnitude and angle of the admittance associated with each feeder are calculated using the current and voltage of each feeder. The location of the fault is determined by comparing the admittances of the feeders to that of a healthy feeder on a continuous basis.
The superiority of the proposed scheme and accuracy were demonstrated through theoretical analysis and simulations. With the added redundancy in the protection criteria, faults at different feeders can be effectively identified. Since high impedance faults do not create significant impacts on current and voltage waveforms, the algorithm does not perform as expected during such faults.
High fault current levels are the basis for the overcurrent protection scheme. The fault current will be high enough for the overcurrent relays to function once a fault occurs within the microgrid [110,111,112]. Even if an instantaneous overcurrent relay provides high-speed protection once a high current level is detected, inverse time-delay overcurrent relays can be coordinated so that the relay closest to the fault is activated before the neighboring relays [9]. The characteristic curves of the relays can be used to change their time delays, and they can be used to provide backup protection to the adjacent feeder.
Based on the overcurrent technique incorporating insulated-gate bipolar transistor-based solid-state circuit breakers (SSCBs), a method for detecting and isolating the faulty sections of islanded DC microgrid clusters was proposed in [113]. The controllers associated with each breaker send the interruption command when the flowing current exceeds a threshold value, as shown in Figure 14. The proposed system's ability to detect short circuit current and maintain DC cluster safety has been proven. Simulation results show that the proposed short-circuit interruption strategy is fast enough to maintain DC microgrid safety along with stabilization and operational performance improvement. However, due to the limited current contribution from inverter-connected DG sources, the fault current is significantly lower when the microgrid cluster is in islanded operation compared to grid-connected operation to activate the overcurrent relays. As a result, it may cause relays to malfunction, resulting in protection issues.
A differential protection scheme can be used to protect a specific zone or piece of equipment, where it uses the differences between the inputs and outputs to identify the faults. Differential protection schemes easily detect internal faults without being affected by the different fault current levels or the presence of DG sources. Therefore, it is reported as a good protection scheme for both AC and DC microgrids [114,115].
A protection scheme for fault detection in the DC link of two interconnected microgrids was presented based on differential current as the secondary protection scheme in [116]. A fuzzy logic-based algorithm was used to detect the fault condition, depending on the differential current. The flow chart presenting the proposed protection method of [116] is shown in Figure 15. This scheme minimizes the false tripping associated with low thresholds set to detect high impedance faults in conventional differential protection schemes. A laboratory-scale prototype implementation of the proposed protection scheme had proven that faults are detected within a few milliseconds.
A flexible protection scheme was introduced for islanded microgrid clusters using microprocessors that implement protection strategies such as differential protection, high impedance fault (HIF) detection and directional protection in [117]. The flow chart presenting the proposed protection method of [117] is illustrated in Figure 16. The protection scheme considers different fault current levels that have arisen from different fault scenarios for a successful protection scheme. Primary protection is facilitated by the combination of three protection methods.
Differential protection sends trip signals if the current difference across a feeder exceeds the threshold values. The high impedance protection module extracts fault-imposed component from the three-phase fault current and converts it into sequence components. The system imbalance ratio is defined as the division of the sum of the negative- and zero-sequence components by the positive-sequence component. The fault ratio given by the division of the system imbalance ratio and the measured current is compared with the threshold value to detect the fault condition. The directional protection module measures the phase angles of the sequence components of the three-phase currents and compares them with the phase angles of the sequence components for the three-phase voltages to send the trip signal if the threshold is exceeded. If the primary protection fails to operate, backup protection based on differential current sends the trip signal to the adjacent breaker.
Adaptive protection architecture is an online protection scheme that can be applied for protection of various system topologies under all short-circuit fault operating conditions. A variety of techniques are adopted in adaptive protection of microgrids. However, the simple technique presented in [118] includes having two sets of relay threshold values for islanded and grid-connected modes. Relay settings are updated with changes in microgrid status while different trip delays are used to deal with changes in fault current levels.
An adaptive protection method was introduced for microgrid clusters in [108]. It changes the relay characteristics according to the layout of the microgrid cluster and the time delay of the trip signal depending on the different fault current levels. Figure 17 shows the flow chart representing the proposed protection technique in [108]. The controllers are expected to identify the layout of the microgrid cluster, considering the significant changes in generation and load. Depending on the cluster configuration, the operating parameter of the inverse-time overcurrent characteristic curve of each relay is determined. The protection scheme is controlled using a hierarchical control structure, which requires a microgrid cluster central controller, microgrid controllers, measurement devices, relays, circuit breakers (CBs) and communication links. Once a fault occurs, due to contributions from each connected DG source to the fault current, there will be bidirectional power flow. Therefore, the central controller determines the threshold values for each relay considering fault current contributions by individual DG sources. Also, considering the direction of the power flow, the DG sources that are contributing to the total fault in each feeder section can be determined. Therefore, the protection system continuously monitors the current and compares it with the threshold values. If the values exceed the threshold values, then the corresponding operating time is determined based on the characteristic curve defined for each relay. Once the time duration of the overcurrent condition exceeds the delay time, the relay sends the trip signal to the breaker. The effectiveness of the protection strategy was evaluated for a cluster of two microgrids.
In this section, a comparative analysis of the performances of each fault detection technique was presented. To summarize the above analysis, the applicabilities, strengths and drawbacks of the fault detection techniques of microgrid clusters are provided in Table 11.
Scheme | Applicability | Strength | Drawbacks |
Overcurrent protection | ● DC microgrids ● Islanded mode ● Parallel connected architecture |
● Relatively fast short-circuit interruption ● Stabilization and operational performance improvement ● Longer life span of the breakers |
● Expensive option with semiconductors ● False tripping due to different levels of fault current with dynamic changes of the cluster |
Hybrid protection scheme | ● AC microgrids ● Islanded mode ● Parallel connected architecture |
● Allows the system to isolate small sections where the faults exist ● Responds to different levels of fault currents with more than one microgrid |
● Requires communication links to operate ● Possibility of failure in operation with different microgrid structures |
Feeder admittance based protection | ● AC microgrids ● Grid-connected and islanded modes ● Parallel connected architecture |
● Simple and flexible fault detection ● High sensitivity and reliability ● The burden on the communication requirement is reduced |
● Malfunction with high impedance faults |
Differential current based fuzzy protection | ● DC microgrids ● Microgrid interconnection system ● Grid-connected and islanded modes ● Parallel connected architecture |
● Enables fast fault detection ● Allows equipment with lower-rated insulation capacity ● High impedance faults can be detected |
● Requires high-speed communication and synchronization ● Current limiting power electronics devices can make fault detection difficult |
Adaptive protection | ● AC microgrid cluster ● Grid-connected and islanded modes ● Parallel connected architecture |
● Allows the system to isolate small faulty sections and change the relay settings according to the cluster configuration ● Protection problems such as blinding of protection, false tripping and failed reclosing can be clearly addressed |
● Requires high-speed communication and microprocessor online operation ● Failure of the central controller or the communication network can cause the protection to fail |
A reliable protection system is required to ensure fast fault isolation and to maintain safety for the rest of the microgrid cluster. Also, during the fault clearance, electric arcing should be suppressed and decreased to increase the lifetime of the circuit breakers. For AC faults, this is possible with an interruption at the moment of the zero-crossing point of the waveform.
However, for DC faults, interruptions are required to have an interrupting capability of fault currents with high speed to avoid huge short-circuit currents and destruction of the components. The fault protection becomes a critical factor in DC microgrid clusters, as a fault is fed by more than one microgrid with more interconnections, and slow-moving mechanical breakers cannot ensure fast fault isolation.
The overcurrent protection system proposed in [114] for the DC microgrid cluster uses high-speed semiconductor breakers offering high speed and a longer lifespan with the absence of moving mechanical parts. However, dynamic changes in the microgrid cluster operation can cause the protection scheme to malfunction. During islanded operation, fault current contributions from inverter-based DG sources are limited. Therefore, the overcurrent protection cannot provide successful fault detection in both grid-connected and islanded modes with the same relay settings. Therefore, overcurrent protection should be improved to change the relay settings accordingly for the successful detection of faults under varying fault levels associated with the different operating conditions of the microgrid cluster.
The current and the voltage characteristics of different feeders can significantly affect the measured admittances associated with them. Based on the changes of the phase differences and amplitudes of measured bus admittances before the fault and after the fault, in [109] a criterion was used to detect the fault location and isolate the fault section in an AC microgrid cluster. As the protection techniques consider the pre-fault and post-fault conditions, dynamic changes of the clusters do not impact the accuracy of fault detection. Even if the measurement requires only the current and voltage distribution, leading to a reduced burden on the communication requirement, high-speed communication is required to inform the controllers about the dynamic changes of the system structure and to acquire the real-time data. However, as in the overcurrent protection, the high impedance faults cannot be detected by the comparison of the admittances.
Differential protection can protect a piece of equipment or a transmission line in the event of a fault in an AC or DC microgrid cluster. However, high impedance faults cannot be detected with high current threshold values. On the other hand, with low threshold values, the main controller makes wrong decisions and trips the system due to power swings, even if no fault has occurred. In [116], A fuzzy logic-based criterion considering the rates of change of the current at the input and output was used to detect the fault condition. Even if the protection technique is able to detect almost all kinds of faults, the power electronic devices limit the rate of change of current, causing the protection scheme to malfunction.
The interconnections among microgrids and bidirectional power flow can lead to protection problems in a microgrid cluster. Therefore, a combination of several protection techniques can overcome the limitations associated with an individual protection method. The hybrid protection proposed in [117] provides accurate and fast fault isolation. However, research can be conducted to optimize protection techniques to respond to different fault current levels associated with different dynamic changes of the microgrid cluster. In contrast to the conventional overcurrent protection, adaptive protection changes the relay characteristics with the dynamic changes of the microgrid cluster, addressing protection problems such as blinding protection, false protection and failure to reclose. The adaptive protection scheme proposed for AC microgrid clusters in [104] considers the variations of generation and demand, and relay thresholds and tripping time delays are adjusted according to fault current levels to achieve accurate fault isolation. Inverse time characteristics of the overcurrent relay ensure faster tripping at severe fault conditions. The protection system effectively isolates the faulted sections and enables continuous operation of the healthy sections for several configurations of two interconnected microgrids. However, the protection scheme is a communication-based protection technique. It requires a high-speed communication system to carry the information of the system changes to the central controller. Also, a large amount of data is processed within the central controller to determine the characteristics of each relay. This process will become very complex as the numbers of interconnections and protection devices increase.
The reported protection techniques for microgrid clusters are validated using only two interconnected microgrids. Also, assumptions such as balanced static loads and DG sources with fixed power output are considered. However, due to the intermittency of renewable energy resources, the same power flow cannot be expected in practice. To partly address this issue, in [108], connected microgrids were operated as net loads or net generators. To achieve realistic results, use of unbalanced loads and dynamic modeling of the distributed generators need to be considered.
A microgrid is capable of operating in both islanded and grid-connected modes. In a microgrid cluster, microgrid islanding can occur when the grid power is interrupted or when neighboring interconnected microgrids are disconnected. The transition between two operating modes is done by the circuit breaker at the point of common coupling (PCC) and the circuit breakers at the interconnections among individual microgrids. The change in microgrid operation mode can lead to the change of fault current level during a fault and cause protection problems. In the islanded mode of operation, fault current is supplied only by the sources in the microgrid, and hence the fault current magnitude is greatly reduced due to current-limiting power electronics inverters [118]. Therefore, detecting the islanding condition effectively is necessary to avoid protection problems. Also, islanding detection within the shortest period is a necessary requirement to save a microgrid or entire microgrid cluster from collapsing. More details on existing islanding detection methods in a single microgrid can be found in [119,120]. However, islanding detection in microgrid clusters having several interconnection points among two or more microgrids requires further investigation.
Communication links between different relays and controllers used in [108,116,117] lead to time delays with more microgrids even if high-speed communication is required. Also, these strategies have not considered the possibility of communication link failures. Alternative coordination strategies can be developed to detect and isolate faults when the communication links fail. This would make the protection coordination strategies more robust. However, decentralized protection systems without depending on the communication link are an under-researched topic that requires further investigation.
This paper analyzed microgrid cluster architectures and explored the current status of protection techniques and their limitations. Furthermore, the existing test systems adopted in microgrid clusters were compared. Also, the controlling concepts used in implementing control objectives and grid synchronization methods were discussed briefly. Microgrid clusters are still at the research stage, but the concept of microgrid clusters will continue to grow with high renewable energy penetration into the distribution system. Implementation of ring-connected and mesh-connected microgrid clusters would be challenging. To make microgrid clusters into a widespread reality, it is necessary to continue the discussion to overcome the challenges associated with microgrid clusters.
The following are the specific conclusions and recommendations:
● A significant amount of research has been done on developing test systems for microgrid clusters considering two methods: 1) dividing the existing test system into interconnected microgrids and 2) connecting microgrids to an existing distribution network at different locations. The first technique considers optimizing the controlling objectives by varying the locations of exiting DERs, while the second method focuses on optimizing the operation of the microgrid network with added microgrids. However, those systems cannot be identified as benchmark systems in new studies with the little information provided in the literature.
● Reported interconnected microgrids are categorized into three architectures: (1) parallel connected, (2) ring connected and (3) mesh connected, considering the layout. Their robustness was analyzed considering four aspects: (1) scalability, (2) stability, (3) reliability and (4) protection. The mesh microgrid cluster architecture has comparatively higher robustness vs. the other architectures, due to the higher number of interconnections associated with it. However, the protection coordination in the interconnected complex network requires further investigation to have accurate and fast fault isolation before the practical implementation of the mesh-type architecture.
● Four main types of controlling concepts—(1) centralized control, (2) decentralized control, (3) hybrid control and (4) distributed control—used in achieving control objectives were analyzed. Each controlling method has its own advantages and limitations associated with it. Therefore, it is not possible to distinguish a particular controlling method as the best one. Thus, careful selection of the controlling concepts at different levels of control structures according to the requirements would enable the optimal operation of the entire network, increasing the reliability.
● Bidirectional power flow among interconnected microgrids and between an individual microgrid and the main grid makes fault detection and localization challenging compared to the protection of individual microgrids. Protection problems such as false tripping, blinding protection, etc. make the protection coordination complex, and overall protection becomes difficult with the number of interconnections among interconnected microgrids. Conventional protection systems, such as overcurrent, distance, impedance and differential protection, require modification to suit the fault characteristics associated with dynamic changes of the microgrid cluster layout. Adaptive protection based on overcurrent relays can be identified as a solution for the protection of the interconnected microgrids, with its ability to change the relay characteristics according to the system changes.
● Synchronizing methods were analyzed, even if they are proposed on individual microgrids with a single grid in-feed. However, their applicability to interconnected microgrids with several grid in-feeds is an under-researched topic that requires further investigation.
● Although different techniques have been used to develop test systems for interconnected microgrids, the stability of the test systems under islanded mode operation is still a topic that requires further investigation. For example, stability of the microgrid cluster under different operating conditions, subjected to intermittencies of the non-dispatchable distributed generators, is an important consideration during the clustering, and more research needs to be carried out.
● Most of the reported studies on microgrid clusters have been focused on the control and protection of parallel connected microgrid clusters. It is necessary to continue the discussion and research to overcome the protection and controlling challenges associated with the other types of more robust microgrid cluster architectures to make them a widespread reality.
The authors gratefully acknowledge the support provided by the Senate Research Committee, University of Moratuwa (Grant number SRC/LT/2020/33).
The authors declare that there is no conflict of interest in this paper.
[1] |
R. E. Kalman, When is a linear control system optimal, J. Basic Eng., 86 (1964), 51–60. https://doi.org/10.1115/1.3653115 doi: 10.1115/1.3653115
![]() |
[2] | R. A. Freeman, P. V. Kokotovic, Inverse optimality in robust stabiliztion, SIAM J. Control Optim., 34 (1998). https://doi.org/10.1137/S0363012993258732 |
[3] | R. Bellman, Dynamic programming, Science, 153 (1966), 34–37. https://doi.org/10.1126/science.153.3731.34 |
[4] | P. J. Werbos, New Tools for Prediction and Analysis in the Behavioral Sciences, Ph.D thesis, Harvard University, 1974. |
[5] |
P. J. Werbos, Advanced forecasting methods for global crisis warning and models of intelligence, Gen. Syst., 1977 (1977), 25–38. https://doi.org/10.1086/292050 doi: 10.1086/292050
![]() |
[6] | P. J. Werbos, Optimization methods for brain-like intelligent control, in Proceedings of 1995 34th IEEE Conference on Decision and Control, 1 (1977), 579–584. https://doi.org/10.1109/CDC.1995.478957 |
[7] |
G. A. Rovithakis, M. A. Christodoulou, Adaptive control of unknown plants using dynamical neural networks, IEEE Trans. Syst. Man Cybern., 24 (1994), 400–412. https://doi.org/10.1109/21.278990 doi: 10.1109/21.278990
![]() |
[8] | J. J. Murray, C. J. Cox, G. G. Lendaris, R. Saeks, Adaptive dynamic programming, IEEE Trans. Syst. Man Cybern. Part C Appl. Rev., 32 (2002), 140–153. https://doi.org/10.1109/TSMCC.2002.801727 |
[9] |
M. Abu-Khalaf, F. L. Lewis, Nearly optimal control laws for nonlinear systems with saturating actuators using a neural network HJB approach, Automatica, 41 (2010), 779–791. https://doi.org/10.1016/j.automatica.2004.11.034 doi: 10.1016/j.automatica.2004.11.034
![]() |
[10] |
K. G. Vamvoudakis, F. L. Lewis, Online actor–critic algorithm to solve the continuous-time infinite horizon optimal control problem, Automatica, 46 (2010), 878–888. https://doi.org/10.1016/j.automatica.2010.02.018 doi: 10.1016/j.automatica.2010.02.018
![]() |
[11] |
X. D. Li, D. X. Peng, J. D. Cao, Lyapunov stability for impulsive systems via event-triggered impulsive control, IEEE Trans. Autom. Control, 65 (2020), 4908–4913. https://doi.org/10.1109/TAC.2020.2964558 doi: 10.1109/TAC.2020.2964558
![]() |
[12] |
X. D. Li, S. J. Song, J. H. Wu, Exponential stability of nonlinear systems with delayed impulses and applications, IEEE Trans. Autom. Control, 64 (2019), 4024–4034. https://doi.org/10.1109/TAC.2019.2905271 doi: 10.1109/TAC.2019.2905271
![]() |
[13] |
D. Wang, D. R. Liu, H. L. Li, Policy iteration algorithm for online design of robust control for a class of continuous-time nonlinear systems, IEEE Trans. Autom. Sci. Eng., 11 (2014), 627–632. https://doi.org/10.1109/TASE.2013.2296206 doi: 10.1109/TASE.2013.2296206
![]() |
[14] |
H. G. Zhang, L. L. Cui, X. Zhang, Y. H. Luo, Data-driven robust approximate optimal tracking control for unknown general nonlinear systems using adaptive dynamic programming method, IEEE Trans. Neural Networks, 22 (2011), 2226–2236. https://doi.org/10.1109/TNN.2011.2168538 doi: 10.1109/TNN.2011.2168538
![]() |
[15] |
D. R. Liu, D. Wang, F. Y. Wang, H. L. Li, X. Yang, Neural-network-based online HJB solution for optimal robust guaranteed cost control of continuous-time uncertain nonlinear systems, IEEE Trans. Cybern., 44 (2014), 2834–2847. https://doi.org/10.1109/TCYB.2014.2357896 doi: 10.1109/TCYB.2014.2357896
![]() |
[16] |
D. R. Liu, X. Yang, H. L. Li, Adaptive optimal control for a class of continuous-time affine nonlinear systems with unknown internal dynamics, Neural Comput. Appl., 23 (2013), 1843–1850. https://doi.org/10.1007/s00521-012-1249-y doi: 10.1007/s00521-012-1249-y
![]() |
[17] |
G. X. Wen, C. L. Philip Chen, S. Z. Sam Ge, H. L. Yang, X. G. Liu, Optimized adaptive nonlinear tracking control using actor-critic reinforcement learning strategy, IEEE Trans. Ind. Inf., 15 (2019), 4969–4977. https://doi.org/10.1109/TII.2019.2894282 doi: 10.1109/TII.2019.2894282
![]() |
[18] |
X. Yang, D. R. Liu, Y. Z. Huang, Neural-network-based online optimal control for uncertain non-linear continuous-time systems with control constraints, IET Control Theory Appl., 7 (2013), 2037–2047. https://doi.org/10.1049/iet-cta.2013.0472 doi: 10.1049/iet-cta.2013.0472
![]() |
[19] |
D. R. Liu, X. Yang, D. Wang, Q. L. Wei, Reinforcement-learning-based robust controller design for continuous-time uncertain nonlinear systems subject to input constraints, IEEE Trans. Cybern., 45 (2015), 1372–1385. https://doi.org/10.1109/TCYB.2015.2417170 doi: 10.1109/TCYB.2015.2417170
![]() |
[20] |
X. Yang, D. R. Liu, Q. L. Wei, Online approximate optimal control for affine non-linear systems with unknown internal dynamics using adaptive dynamic programming, IET Control Theory Appl., 8 (2014), 1676–1688. https://doi.org/10.1049/iet-cta.2014.0186 doi: 10.1049/iet-cta.2014.0186
![]() |
[21] |
S. Bhasin, R. Kamalapurkar, M. Johnson, K. G. Vamvoudakis, F. L. Lewis, W. E. Dixon, A novel actor-critic-identifier architecture for approximate optimal control of uncertain nonlinear systems, Automatica, 49 (2013), 82–92. https://doi.org/10.1016/j.automatica.2012.09.019 doi: 10.1016/j.automatica.2012.09.019
![]() |
[22] | M. Krstic, P. V. Kokotovic, I. Kanellakopoulos, Nonlinear and Adaptive Control Design, John Wiley & Sons, 1995. |
[23] |
G. X. Wen, S. Z. Sam Ge, F. W. Tu, Optimized backstepping for tracking control of strict-feedback systems, IEEE Trans. Neural Networks Learn. Syst., 29 (2018), 3850–3862. https://doi.org/10.1109/TNNLS.2018.2803726 doi: 10.1109/TNNLS.2018.2803726
![]() |
[24] |
S. C. Tong, K. K. Sun, S. Sui, Observer-based adaptive fuzzy decentralized optimal control design for strict-feedback nonlinear large-scale systems, IEEE Trans. Fuzzy Syst., 26 (2017), 569–584. https://doi.org/10.1109/TFUZZ.2017.2686373 doi: 10.1109/TFUZZ.2017.2686373
![]() |
[25] |
Y. M. Li, T. C. Wang, W. Liu, S. C. Tong, Neural network adaptive output-feedback optimal control for active suspension systems, IEEE Trans. Syst. Man Cybern.: Syst., 52 (2021), 4021–4032. https://doi.org/10.1109/TSMC.2021.3089768 doi: 10.1109/TSMC.2021.3089768
![]() |
[26] |
G. X. Wen, C. L. Philip Chen, W. N. Li, Simplified optimized control using reinforcement learning algorithm for a class of stochastic nonlinear systems, Inf. Sci., 517 (2020), 230–243. https://doi.org/10.1016/j.ins.2019.12.039 doi: 10.1016/j.ins.2019.12.039
![]() |
[27] | X. X. Pei, Y. M. Li, S. D. Yi, Adaptive neural network optimal control of hybrid electric vehicle power battery, J. Jilin Univ. (Eng. Technol. Edition), 2021 (2021). https://doi.org/10.13229/j.cnki.jdxbgxb20211422 |
[28] |
G. X. Wen, C. L. Philip Chen, S. Z. Sam Ge, Simplified optimized backstepping control for a class of nonlinear strict-feedback systems with unknown dynamic functions, IEEE Trans. Cybern., 51 (2020), 4567–4580. https://doi.org/10.1109/TCYB.2020.3002108 doi: 10.1109/TCYB.2020.3002108
![]() |
[29] |
J. Lan, Y. J. Liu, D. X. Yu, G. X. Wen, S. C. Tong, L. Liu, Time-varying optimal formation control for second-order multiagent systems based on neural network observer and reinforcement learning, IEEE Trans. Neural Networks Learn. Syst., 2022 (2022), 1–12. https://doi.org/10.1109/TNNLS.2022.3158085 doi: 10.1109/TNNLS.2022.3158085
![]() |
[30] |
W. B. Xiao, Q. Zhou, Y. liu, H. Y. Li, R. Q. Lu, Distributed reinforcement learning containment control for multiple nonholonomic mobile robots, IEEE Trans. Circuits Syst. I Regul. Pap., 69 (2021), 896–907. https://doi.org/10.1109/TCSI.2021.3121809 doi: 10.1109/TCSI.2021.3121809
![]() |
[31] |
B. X. Jiang, Y. J. Lou, J. Q. Lu, Input-to-state stability of delayed systems with bounded-delay impulses, Math. Modell. Control, 2 (2022), 44–54. https://doi.org/10.3934/mmc.2022006 doi: 10.3934/mmc.2022006
![]() |
[32] |
V. Djordjevic, L. Dubonjic, M. M. Morato, D. Prsic, V. Stojanovic, Sensor fault estimation for hydraulic servo actuator based on sliding mode observer, Math. Modell. Control, 2 (2022), 34–43. https://doi.org/10.3934/mmc.2022005 doi: 10.3934/mmc.2022005
![]() |
[33] |
Y. M. Li, Y. J. Liu, S. C. Tong, Observer-based neuro-adaptive optimized control of strict-feedback nonlinear systems with state constraints, IEEE Trans. Neural Networks Learn. Syst., 33 (2022), 3131–3145. https://doi.org/10.1109/TNNLS.2021.3051030 doi: 10.1109/TNNLS.2021.3051030
![]() |
[34] |
Y. M. Li, Y. L. Fan, K. W. Li, W. Liu, S. C. Tong, Adaptive optimized backstepping control-based RL algorithm for stochastic nonlinear systems with state constraints and its application, IEEE Trans. Cybern., 2021 (2021), 1–14. https://doi.org/10.1109/TCYB.2021.3069587 doi: 10.1109/TCYB.2021.3069587
![]() |
[35] |
Y. M. Li, J. X. Zhang, W. Liu, S. C. Tong, Observer-based adaptive optimized control for stochastic nonlinear systems with input and state constraints, IEEE Trans. Neural Networks Learn. Syst., 2021 (2021), 1–15. https://doi.org/10.1109/TNNLS.2021.3087796 doi: 10.1109/TNNLS.2021.3087796
![]() |
[36] |
Y. Wu, X. J. Xie, Robust adaptive control for state-constrained nonlinear systems with input saturation and unknown control direction, IEEE Trans. Syst. Man Cybern.: Syst., 51 (2019), 1192–1202. https://doi.org/10.1109/TSMC.2019.2895048 doi: 10.1109/TSMC.2019.2895048
![]() |
[37] |
Y. M. Li, J. X. Zhang, S. C. Tong, Fuzzy adaptive optimized leader-following formation control for second-order stochastic multi-agent systems, IEEE Trans. Ind. Inf., 18 (2021), 6026–6037. https://doi.org/10.1109/TII.2021.3133927 doi: 10.1109/TII.2021.3133927
![]() |
[38] |
K. Ezal, Z. G. Pan, P. Kokotovic, Locally optimal and robust backstepping design, IEEE Trans. Autom. Control, 45 (2000), 260–271. https://doi.org/10.1109/9.839948 doi: 10.1109/9.839948
![]() |
[39] |
Y. M. Li, X. Min, S. C. Tong, Adaptive fuzzy inverse optimal control for uncertain strict-feedback nonlinear systems, IEEE Trans. Fuzzy Syst., 28 (2019), 2363–2374. https://doi.org/10.1109/TFUZZ.2019.2935693 doi: 10.1109/TFUZZ.2019.2935693
![]() |
[40] |
Y. M. Li, X. Min, S. C. Tong, Observer-based fuzzy adaptive inverse optimal output feedback control for uncertain nonlinear systems, IEEE Trans. Fuzzy Syst., 29 (2020), 1484–1495. https://doi.org/10.1109/TFUZZ.2020.2979389 doi: 10.1109/TFUZZ.2020.2979389
![]() |
[41] |
K. X. Lu, Z. Liu, C. L. Philip Chen, Y. N. Wang, Y. Zhang, Inverse optimal design of direct adaptive fuzzy controllers for uncertain nonlinear systems, IEEE Trans. Fuzzy Syst., 30 (2022), 1669–1682. https://doi.org/10.1109/TFUZZ.2021.3064678 doi: 10.1109/TFUZZ.2021.3064678
![]() |
[42] |
X. Min, Y. M. Li, S. C. Tong, Adaptive fuzzy output feedback inverse optimal control for vehicle active suspension systems, Neurocomputing, 403 (2020), 257–267. https://doi.org/10.1016/j.neucom.2020.04.096 doi: 10.1016/j.neucom.2020.04.096
![]() |
[43] |
H. H. Long, J. K. Zhao, J. Q. Lai, inverse optimal adaptive fault-tolerant attitude control for flexible spacecraft with input saturation, J. Shanghai Jiaotong Univ. (Sci.), 20 (2015), 513–527. 10.1007/s12204-015-1659-y doi: 10.1007/s12204-015-1659-y
![]() |
[44] |
X. D. Li, D. W. C. Ho, J. D. Cao, Finite-time stability and settling-time estimation of nonlinear impulsive systems, Automatica, 99 (2019), 361–368. https://doi.org/10.1016/j.automatica.2018.10.024 doi: 10.1016/j.automatica.2018.10.024
![]() |
[45] |
X. D. Li, X. Y. Yang, S. J. Song, Lyapunov conditions for finite-time stability of time-varying time-delay systems, Automatica, 103 (2019), 135–140. https://doi.org/10.1016/j.automatica.2019.01.031 doi: 10.1016/j.automatica.2019.01.031
![]() |
[46] |
Y. M. Li, T. T. Yang, S. C. Tong, Adaptive neural networks finite-time optimal control for a class of nonlinear systems, IEEE Trans. Neural Networks Learn. Syst., 31 (2019), 4451–4460. https://doi.org/10.1109/TNNLS.2019.2955438 doi: 10.1109/TNNLS.2019.2955438
![]() |
[47] |
Y. M. Li, T. T. Yang, L. Liu, G. Feng, S. C. Tong, Finite-time optimal control for interconnected nonlinear systems, Int. J. Robust Nonlinear Control, 30 (2020), 3451–3470. https://doi.org/10.1002/rnc.4944 doi: 10.1002/rnc.4944
![]() |
[48] |
K. X. Lu, Z. Liu, H. Y. Yu, C. L. Philip Chen, Y. Zhang, Adaptive fuzzy inverse optimal fixed-time opntrol of uncertain nonlinear systems, IEEE Trans. Fuzzy Syst., 45 (2000), 260–271. https://doi.org/10.1109/TFUZZ.2021.3132151 doi: 10.1109/TFUZZ.2021.3132151
![]() |
[49] |
S. J. Cao, L. Sun, J. J. Jiang, Z. Y. Zuo, Reinforcement learning-based fixed-time trajectory tracking control for uncertain robotic manipulators with input saturation, IEEE Trans. Neural Networks Learn. Syst., 2021 (2021), 1–12. https://doi.org/10.1109/TNNLS.2021.3116713 doi: 10.1109/TNNLS.2021.3116713
![]() |
[50] |
J. T. Hu, G. X. Sui, X. X. Lv, X. D. Li, Fixed-time control of delayed neural networks with impulsive perturbations, IEEE Trans. Neural Networks Learn. Syst., 23 (2018), 904–920. https://doi.org/10.15388/NA.2018.6.6 doi: 10.15388/NA.2018.6.6
![]() |
1. | Guolong Ma, Jianing Li, Xiao-Ping Zhang, A Review on Optimal Energy Management of Multimicrogrid System Considering Uncertainties, 2022, 10, 2169-3536, 77081, 10.1109/ACCESS.2022.3192638 | |
2. | Santi Agatino Rizzo, Editorial to the 'Special Issue—Distribution network reliability in Smart Grids and Microgrids' of AIMS Energy, 2022, 10, 2333-8334, 533, 10.3934/energy.2022026 | |
3. | W. E. P. Sampath Ediriweera, N. W. A. Lidula, 2024, Hybrid Energy Storages for Islanded Microgrids with High Solar PV Penetration, 979-8-3315-2904-8, 694, 10.1109/MERCon63886.2024.10688488 | |
4. | W. E. P. Sampath Ediriweera, N. W. A. Lidula, 2023, Adaptive Load Shedding Technique for Energy Management in Networked Microgrids, 979-8-3503-2362-7, 465, 10.1109/ICIIS58898.2023.10253509 | |
5. | W. E. P. Sampath Ediriweera, N. W. A. Lidula, R. Samarasinghe, Ring connected microgrid clusters for improved resiliency in distribution systems with high solar PV penetration, 2024, 12, 2333-8334, 872, 10.3934/energy.2024041 | |
6. | Miłosz Krysik, Krzysztof Piotrowski, 2023, Crest Factor Oriented Finite Set Model Predictive Control of the Mesh Microgrid, 979-8-3503-2190-6, 174, 10.1109/PREE57903.2023.10370442 | |
7. | Marriam Liaqat, Turki Alsuwian, Arslan Ahmed Amin, Muhammad Adnan, Adil Zulfiqar, Transient stability enhancement in renewable energy integrated multi-microgrids: A comprehensive and critical analysis, 2024, 57, 0020-2940, 187, 10.1177/00202940231196193 | |
8. | Dessalegn Bitew Aeggegn, George Nyauma Nyakoe, Cyrus Wekesa, A state of the art review on energy management techniques and optimal sizing of DERs in grid-connected multi-microgrids, 2024, 11, 2331-1916, 10.1080/23311916.2024.2340306 | |
9. | Jorge Armando De La Cruz Saavedra, Sen Tan, Diptish Saha, Najmeh Bazmohammadi, Juan C. Vasquez, Josep M. Guerrero, Brain Modeling for Microgrid Control and Protection: State of the Art, Challenges, and Future Trends, 2024, 18, 1932-4529, 63, 10.1109/MIE.2024.3374234 |
Reference | Objective | Method |
[20] | To maximize the generation under the constraints of distribution system and microgrids | Mixed-integer linear program subjected to different operating conditions of microgrids and distribution network |
[21] | Voltage control and power management | A two-level control structure Primary layer coordinates the load sharing among DERs Second layer controls the power exchange among microgrids and restores voltage to reference values Analysis of eigenvalues to select the control parameters of the entire distribution network |
[22] | Optimal dynamic planning of interconnected microgrids | Probabilistic approach for different cases with the locations of DERs and number of microgrids |
[23] | To minimize the total load shedding of a distribution network under natural disasters | Linear programming to find the optimal locations of DERs |
[24] | To study the dynamics of the system with switching transients of network microgrids | No mechanism for clustering Grid-friendly appliance controllers to mitigate the problems with transients |
[30] | Robust microgrid clustering in a distribution system with inverter-based DERs | Concept of interrelationships between ecological species translates into the dynamic behavior of DERs Analysis of eigenvalues to find the optimal locations of DERs |
Reference | Objective | Method |
[25] | To study the operation of networked microgrids | No mechanism for clustering Different operational scenarios are simulated for different modes of operation |
[26] | To reduce the total operational cost of microgrids To smooth the load curves of the distribution network |
A two-stage optimization model subjected to the changes of generation cost |
[27] | To assess the stability of networked microgrids with uncertainties of DERs | Eigenvalue analysis for different penetrations of six microgrids |
[28] | To introduce an energy management system for microgrid clusters to increase profit | A stochastic bi-level optimization problem |
[29] | To introduce an energy management system for microgrid clusters | Stochastic decentralized bi-level optimization method for islanded and grid-connected modes of operation |
Advantages | Disadvantages | |
AC | Transformers can be used for changing voltage levels and for isolation Well established protection techniques can be used |
Requires power and frequency control Transient and dynamic instabilities High operational cost due to losses, skin effect and dielectric losses Need for synchronism and large power oscillations Load-dependent voltage control Applicable for short distances (<50 km) |
DC | Increased efficiency Losses are minimized with constant currents and fewer conversion stages Low variety of faults No electromagnetic interference Low operational cost and low cost associated with conductors and insulators Better transient and dynamic stability No reactive power controls Long-distance bulk power transmission |
High costs are associated with the interfaces Fault isolation is difficult due to the unavailability of a zero-crossing point |
Advantages | Disadvantages | |
Transformer | Reliable and cost-effective method to change AC/AC voltage levels | Low controllability Low operational performance |
Converter | Better voltage regulation High voltage controllability Can be applied with the interconnection of any line technology (AC/AC, AC/DC or DC/DC) |
Protection requirements Dependency on communication Expensive solution |
Layout architecture | Microgrid cluster growing capacity | Individual microgrid growing capacity | Overall scaling capacity | Complexity |
Parallel | Low | Medium | Low | Low |
Ring | High | Medium | Medium | Medium |
Mesh | High | High | High | High |
Layout architecture | Stability |
Parallel | Medium |
Ring | High |
Mesh | High |
Layout architecture | Reliability |
Parallel | Medium |
Ring | High |
Mesh | High |
Layout architecture | Selectivity applicability | Coordination requirements | Overall protection difficulty |
Radial | High | Low | Low |
Ring | Medium | Medium | Medium |
Mesh | Low | High | High |
Feature | Hierarchical control | Distributed control |
Reliability | Moderate | High |
Plug and play | Low | High |
Flexibility/Expandability | Low | High |
Communication bandwidth | Low | High |
Time/Space complexity | High | Low |
Design complexity | Complex | Simple |
Task | IEC 61850 | ETSI open SG protocol |
Protection | 4 ms | 1–10 ms |
Control | 16–100 ms | 100 ms |
Messages requiring immediate action | 1A: 3 or 10 ms 1B: 20 or 100 ms |
Not specified |
Time synchronization | Accuracy | Not specified |
Monitoring | 1 s | 1 s |
Operation and maintenance | 1 s | Not specified |
Scheme | Applicability | Strength | Drawbacks |
Overcurrent protection | ● DC microgrids ● Islanded mode ● Parallel connected architecture |
● Relatively fast short-circuit interruption ● Stabilization and operational performance improvement ● Longer life span of the breakers |
● Expensive option with semiconductors ● False tripping due to different levels of fault current with dynamic changes of the cluster |
Hybrid protection scheme | ● AC microgrids ● Islanded mode ● Parallel connected architecture |
● Allows the system to isolate small sections where the faults exist ● Responds to different levels of fault currents with more than one microgrid |
● Requires communication links to operate ● Possibility of failure in operation with different microgrid structures |
Feeder admittance based protection | ● AC microgrids ● Grid-connected and islanded modes ● Parallel connected architecture |
● Simple and flexible fault detection ● High sensitivity and reliability ● The burden on the communication requirement is reduced |
● Malfunction with high impedance faults |
Differential current based fuzzy protection | ● DC microgrids ● Microgrid interconnection system ● Grid-connected and islanded modes ● Parallel connected architecture |
● Enables fast fault detection ● Allows equipment with lower-rated insulation capacity ● High impedance faults can be detected |
● Requires high-speed communication and synchronization ● Current limiting power electronics devices can make fault detection difficult |
Adaptive protection | ● AC microgrid cluster ● Grid-connected and islanded modes ● Parallel connected architecture |
● Allows the system to isolate small faulty sections and change the relay settings according to the cluster configuration ● Protection problems such as blinding of protection, false tripping and failed reclosing can be clearly addressed |
● Requires high-speed communication and microprocessor online operation ● Failure of the central controller or the communication network can cause the protection to fail |
Reference | Objective | Method |
[20] | To maximize the generation under the constraints of distribution system and microgrids | Mixed-integer linear program subjected to different operating conditions of microgrids and distribution network |
[21] | Voltage control and power management | A two-level control structure Primary layer coordinates the load sharing among DERs Second layer controls the power exchange among microgrids and restores voltage to reference values Analysis of eigenvalues to select the control parameters of the entire distribution network |
[22] | Optimal dynamic planning of interconnected microgrids | Probabilistic approach for different cases with the locations of DERs and number of microgrids |
[23] | To minimize the total load shedding of a distribution network under natural disasters | Linear programming to find the optimal locations of DERs |
[24] | To study the dynamics of the system with switching transients of network microgrids | No mechanism for clustering Grid-friendly appliance controllers to mitigate the problems with transients |
[30] | Robust microgrid clustering in a distribution system with inverter-based DERs | Concept of interrelationships between ecological species translates into the dynamic behavior of DERs Analysis of eigenvalues to find the optimal locations of DERs |
Reference | Objective | Method |
[25] | To study the operation of networked microgrids | No mechanism for clustering Different operational scenarios are simulated for different modes of operation |
[26] | To reduce the total operational cost of microgrids To smooth the load curves of the distribution network |
A two-stage optimization model subjected to the changes of generation cost |
[27] | To assess the stability of networked microgrids with uncertainties of DERs | Eigenvalue analysis for different penetrations of six microgrids |
[28] | To introduce an energy management system for microgrid clusters to increase profit | A stochastic bi-level optimization problem |
[29] | To introduce an energy management system for microgrid clusters | Stochastic decentralized bi-level optimization method for islanded and grid-connected modes of operation |
Advantages | Disadvantages | |
AC | Transformers can be used for changing voltage levels and for isolation Well established protection techniques can be used |
Requires power and frequency control Transient and dynamic instabilities High operational cost due to losses, skin effect and dielectric losses Need for synchronism and large power oscillations Load-dependent voltage control Applicable for short distances (<50 km) |
DC | Increased efficiency Losses are minimized with constant currents and fewer conversion stages Low variety of faults No electromagnetic interference Low operational cost and low cost associated with conductors and insulators Better transient and dynamic stability No reactive power controls Long-distance bulk power transmission |
High costs are associated with the interfaces Fault isolation is difficult due to the unavailability of a zero-crossing point |
Advantages | Disadvantages | |
Transformer | Reliable and cost-effective method to change AC/AC voltage levels | Low controllability Low operational performance |
Converter | Better voltage regulation High voltage controllability Can be applied with the interconnection of any line technology (AC/AC, AC/DC or DC/DC) |
Protection requirements Dependency on communication Expensive solution |
Layout architecture | Microgrid cluster growing capacity | Individual microgrid growing capacity | Overall scaling capacity | Complexity |
Parallel | Low | Medium | Low | Low |
Ring | High | Medium | Medium | Medium |
Mesh | High | High | High | High |
Layout architecture | Stability |
Parallel | Medium |
Ring | High |
Mesh | High |
Layout architecture | Reliability |
Parallel | Medium |
Ring | High |
Mesh | High |
Layout architecture | Selectivity applicability | Coordination requirements | Overall protection difficulty |
Radial | High | Low | Low |
Ring | Medium | Medium | Medium |
Mesh | Low | High | High |
Feature | Hierarchical control | Distributed control |
Reliability | Moderate | High |
Plug and play | Low | High |
Flexibility/Expandability | Low | High |
Communication bandwidth | Low | High |
Time/Space complexity | High | Low |
Design complexity | Complex | Simple |
Task | IEC 61850 | ETSI open SG protocol |
Protection | 4 ms | 1–10 ms |
Control | 16–100 ms | 100 ms |
Messages requiring immediate action | 1A: 3 or 10 ms 1B: 20 or 100 ms |
Not specified |
Time synchronization | Accuracy | Not specified |
Monitoring | 1 s | 1 s |
Operation and maintenance | 1 s | Not specified |
Scheme | Applicability | Strength | Drawbacks |
Overcurrent protection | ● DC microgrids ● Islanded mode ● Parallel connected architecture |
● Relatively fast short-circuit interruption ● Stabilization and operational performance improvement ● Longer life span of the breakers |
● Expensive option with semiconductors ● False tripping due to different levels of fault current with dynamic changes of the cluster |
Hybrid protection scheme | ● AC microgrids ● Islanded mode ● Parallel connected architecture |
● Allows the system to isolate small sections where the faults exist ● Responds to different levels of fault currents with more than one microgrid |
● Requires communication links to operate ● Possibility of failure in operation with different microgrid structures |
Feeder admittance based protection | ● AC microgrids ● Grid-connected and islanded modes ● Parallel connected architecture |
● Simple and flexible fault detection ● High sensitivity and reliability ● The burden on the communication requirement is reduced |
● Malfunction with high impedance faults |
Differential current based fuzzy protection | ● DC microgrids ● Microgrid interconnection system ● Grid-connected and islanded modes ● Parallel connected architecture |
● Enables fast fault detection ● Allows equipment with lower-rated insulation capacity ● High impedance faults can be detected |
● Requires high-speed communication and synchronization ● Current limiting power electronics devices can make fault detection difficult |
Adaptive protection | ● AC microgrid cluster ● Grid-connected and islanded modes ● Parallel connected architecture |
● Allows the system to isolate small faulty sections and change the relay settings according to the cluster configuration ● Protection problems such as blinding of protection, false tripping and failed reclosing can be clearly addressed |
● Requires high-speed communication and microprocessor online operation ● Failure of the central controller or the communication network can cause the protection to fail |