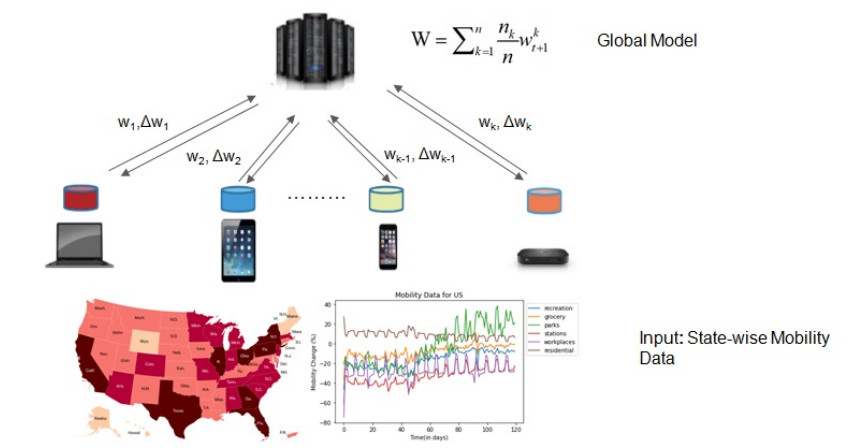
Aggregating a massive amount of disease-related data from heterogeneous devices, a distributed learning framework called Federated Learning(FL) is employed. But, FL suffers in distributing the global model, due to the heterogeneity of local data distributions. To overcome this issue, personalized models can be learned by using Federated multitask learning(FMTL). Due to the heterogeneous data from distributed environment, we propose a personalized model learned by federated multitask learning (FMTL) to predict the updated infection rate of COVID-19 in the USA using a mobility-based SEIR model. Furthermore, using a mobility-based SEIR model with an additional constraint we can analyze the availability of beds. We have used the real-time mobility data sets in various states of the USA during the years 2020 and 2021. We have chosen five states for the study and we observe that there exists a correlation among the number of COVID-19 infected cases even though the rate of spread in each case is different. We have considered each US state as a node in the federated learning environment and a linear regression model is built at each node. Our experimental results show that the root-mean-square percentage error for the actual and prediction of COVID-19 cases is low for Colorado state and high for Minnesota state. Using a mobility-based SEIR simulation model, we conclude that it will take at least 400 days to reach extinction when there is no proper vaccination or social distance.
Citation: M Kumaresan, M Senthil Kumar, Nehal Muthukumar. Analysis of mobility based COVID-19 epidemic model using Federated Multitask Learning[J]. Mathematical Biosciences and Engineering, 2022, 19(10): 9983-10005. doi: 10.3934/mbe.2022466
[1] | Songfeng Liu, Jinyan Wang, Wenliang Zhang . Federated personalized random forest for human activity recognition. Mathematical Biosciences and Engineering, 2022, 19(1): 953-971. doi: 10.3934/mbe.2022044 |
[2] | Hamdy M. Youssef, Najat A. Alghamdi, Magdy A. Ezzat, Alaa A. El-Bary, Ahmed M. Shawky . A new dynamical modeling SEIR with global analysis applied to the real data of spreading COVID-19 in Saudi Arabia. Mathematical Biosciences and Engineering, 2020, 17(6): 7018-7044. doi: 10.3934/mbe.2020362 |
[3] | Lin Feng, Ziren Chen, Harold A. Lay Jr., Khaled Furati, Abdul Khaliq . Data driven time-varying SEIR-LSTM/GRU algorithms to track the spread of COVID-19. Mathematical Biosciences and Engineering, 2022, 19(9): 8935-8962. doi: 10.3934/mbe.2022415 |
[4] | Kefeng Fan, Cun Xu, Xuguang Cao, Kaijie Jiao, Wei Mo . Tri-branch feature pyramid network based on federated particle swarm optimization for polyp segmentation. Mathematical Biosciences and Engineering, 2024, 21(1): 1610-1624. doi: 10.3934/mbe.2024070 |
[5] | Michael James Horry, Subrata Chakraborty, Biswajeet Pradhan, Maryam Fallahpoor, Hossein Chegeni, Manoranjan Paul . Factors determining generalization in deep learning models for scoring COVID-CT images. Mathematical Biosciences and Engineering, 2021, 18(6): 9264-9293. doi: 10.3934/mbe.2021456 |
[6] | Thyago Celso C. Nepomuceno, Thalles Vitelli Garcez, Lúcio Camara e Silva, Artur Paiva Coutinho . Measuring the mobility impact on the COVID-19 pandemic. Mathematical Biosciences and Engineering, 2022, 19(7): 7032-7054. doi: 10.3934/mbe.2022332 |
[7] | Fen-fen Zhang, Zhen Jin . Effect of travel restrictions, contact tracing and vaccination on control of emerging infectious diseases: transmission of COVID-19 as a case study. Mathematical Biosciences and Engineering, 2022, 19(3): 3177-3201. doi: 10.3934/mbe.2022147 |
[8] | Shubashini Velu . An efficient, lightweight MobileNetV2-based fine-tuned model for COVID-19 detection using chest X-ray images. Mathematical Biosciences and Engineering, 2023, 20(5): 8400-8427. doi: 10.3934/mbe.2023368 |
[9] | A. Q. Khan, M. Tasneem, M. B. Almatrafi . Discrete-time COVID-19 epidemic model with bifurcation and control. Mathematical Biosciences and Engineering, 2022, 19(2): 1944-1969. doi: 10.3934/mbe.2022092 |
[10] | Cheng-Cheng Zhu, Jiang Zhu . Spread trend of COVID-19 epidemic outbreak in China: using exponential attractor method in a spatial heterogeneous SEIQR model. Mathematical Biosciences and Engineering, 2020, 17(4): 3062-3087. doi: 10.3934/mbe.2020174 |
Aggregating a massive amount of disease-related data from heterogeneous devices, a distributed learning framework called Federated Learning(FL) is employed. But, FL suffers in distributing the global model, due to the heterogeneity of local data distributions. To overcome this issue, personalized models can be learned by using Federated multitask learning(FMTL). Due to the heterogeneous data from distributed environment, we propose a personalized model learned by federated multitask learning (FMTL) to predict the updated infection rate of COVID-19 in the USA using a mobility-based SEIR model. Furthermore, using a mobility-based SEIR model with an additional constraint we can analyze the availability of beds. We have used the real-time mobility data sets in various states of the USA during the years 2020 and 2021. We have chosen five states for the study and we observe that there exists a correlation among the number of COVID-19 infected cases even though the rate of spread in each case is different. We have considered each US state as a node in the federated learning environment and a linear regression model is built at each node. Our experimental results show that the root-mean-square percentage error for the actual and prediction of COVID-19 cases is low for Colorado state and high for Minnesota state. Using a mobility-based SEIR simulation model, we conclude that it will take at least 400 days to reach extinction when there is no proper vaccination or social distance.
COVID-19 is the most challenging infectious disease, which has been threatening human beings all over the globe ever since World Health Organization (WHO) [1] declared as a pandemic. Today, adequate awareness about the virus has been reached to a majority of people and all the countries around the world are imposing various precautionary measures to curb the spread of the virus. The COVID-19 pandemic accelerates itself into different phases of waves, where, many countries experienced the first two waves and now emerging into the third wave [2]. In the first wave, many people were affected with symptomatic and asymptomatic and the number of people hospitalized and death cases were at the peak. Due to various preventive measures, there was a decrease in the number of cases, however, after a certain period there was a substantial increase in the number of infected people and this is called the second wave. During the second wave, vaccinations are started and more understanding about the virus is known. Despite various measures, the early stages of the third wave have started in many countries.
The WHO recommendations to avoid the spreading of the virus include social spacing, wearing the mask, good aeration, sanitizing, restricted movement, and home quarantine. Many countries around the world have put strict restrictions on travel, closure of malls, theaters, and shops leading to severe impact on the economic impact on all sectors. In addition, the closure of schools and colleges leads to a severe impact on the learning process and the results are yet to wait and be seen. The major sectors affected by the restrictions are Agriculture, Petroleum and Oil, the Manufacturing industry, Education, Finance industry, Tourism, Real estate, and the Food industry. Severe social impacts like an increase in violence, mental stress, increased usage of video games and emotional issues are faced during this lockdown period [3]. A fine-tuned strategy is needed to relax the restrictions in such a way that both economic and social factors are taken care of. There is a mutual relationship between the number of COVID-19 cases and human mobility. The accurate prediction of the epidemic concerning mobility helps to take preventive measures [4].
A plethora of data is stored and transmitted from different mobile devices to humans due to mobility. The mobility data are heterogeneous including demographic information, diagnosis results, and clinical notes. A distributed machine learning approach known as Federated learning is used for training the model from heterogeneous mobile devices. This approach ensures data privacy by keeping the data in the local devices. The effective aggregation of locally trained models in the centralized server is playing a vital role in this approach [5,6].
Myriad research works in the literature are studied to analyse the spread of various epidemic diseases like COVID-19, SARS, Choleara and etc, . In [7], a positivity-preserving nonstandard implicit finite-difference scheme is developed and analysed to solve an advection- reaction nonlinear epidemic model. Efficient nonstandard computational implementations were studied for a nonlinear epidemic model like COVID-19 [8] and Hepatitis B model [9]. In [10], the authors identified 63 studies and summarized the three aspects of these studies: epidemiological parameters estimation, trend prediction, and control measure evaluation. By using the nonstandard finite difference method (NSFDS), the SEIR epidemic is studied by [11]. The Ising-model and percolation theory for COVID-19 epidemic is analysed in [12]. In [13], COVID-19 transmission dynamics are studied with various mathematical techniques. Furthermore, the fractional order epidemic models are analysed for SARS-CoV2 with the variant in [14]. In [15], the authors have analysed the COVID-19 model with fractional derivatives using real data from Pakistan. Stability analysis of an incommensurate fractional-order SIR model has been studied by [16]. The dynamic analysis of cholera disease by using two fractional numerical methods has been discussed in [17]. A fractional order pandemic model [18] is developed both to examine the spread of COVID-19 and its relationship with diabetes using real data from Turkey. A mathematical calcium model [19] is developed in the form of the Hilfer fractional reaction-diffusion equation to examine the calcium diffusion in the cells. A mathematical model of stem cells and chemotherapy for cancer treatment [20], in which the model is represented by fractional-order differential equations. The effect of the vaccination campaign of COVID-19 is analyzed in [21]. A modified SIR (susceptible-infected-recovered/removed) model [22] described the evolution in time of the infectious disease caused by Sars-Cov-2. The stochastic COVID-19 epidemic model with time delay is analysed in [23]. All the above epidemic models explore population-based epidemic behaviors without considering the movement of infectious entities.
Recently, various machine learning based predictive models developed to analyse the spread of COVID-19. A network inference-based prediction algorithm was discussed by Achterberg et al. [24]. They have shown that the network-based algorithm is superior to other algorithms. Machine learning techniques to predict different levels of hospital care for COVID-19 were presented by Elena Hernandez-Pereira et al. [25]. A robust model of predictive control feedback using social distancing was proposed by Kohler et al. [26]. Similar work was presented by Morato et al. [27] which emphasized various measures of social distancing. Variations of SIS and SEIR models were used by Yan and Zhou [28], Khouzani et al. [29]. Multitask learning [30] which aims to improve the performance of multiple related learning tasks and nonlinear optimal control of the COVID-19 outbreak using mobility data was proposed by Mikhail Hayhoe et al [31]. However, in our work, to preserve the data privacy, we have used a federated learning approach.
Federated learning is a machine learning(ML) method that enables ML models which experience different data sets located in different sites (e.g., local data centers, a central server) without sharing training data [32]. Federated learning [33,34] has been performed in the following manner:
● Local ML models are trained on local heterogeneous datasets. For example, as users use an ML application, they identify mistakes in the ML application's predictions and correct those mistakes. These create local training datasets in each user's device.
● The parameters of the models are traded between these local data centers periodically. In many models, these parameters are encrypted before exchanging. Local data samples are not shared. This improves data protection and data privacy.
● An aggregated global model is built.
● The predictability of the global model is shared with local data centers to integrate the global model into their ML local models.
In recent years, several federated learning approaches to analyse COVID-19 are available in the literature. Abdul salam et al. [35] used federated learning to predict COVID-19. They have shown that the federated learning approach has more accuracy compared to other machine learning models. AkhilVaid et al. [36] predict the mortality of COVID-19 patients based on electronic health records received from the hospital using a federated learning approach. A federated multitask learning to detect COVID-19 from chest radiography images was proposed by MahbubUlAlam et al. [37]. In our work, the federated multitask learning is applied over a mobility based epidemic model to predict the infection rate. To the best of our knowledge, there exists no prior research works predicting the infection rate using the FMTL approach.
Hence, we have considered various mobility data of states in the US and considered federated learning environments. We have also studied the availability of bed facilities in the hospital using our prediction of the proposed model. Our approach uses a federated environment to predict the COVID-19 spread based on mobility and our experimental results show the accuracy of our predictions compared with the actual cases. Data privacy is ensured in our approach.
In the FMTL model, we learn the model across m distributed nodes (states) and each node needs to perform multiple tasks. After finding parameters for each state, and aggregating all the parameters, we get a global model and repeat this process n number of times; finally, we get the best model without sharing the state data.
In this paper, we are using the FMTL model to predict infection rates by using mobility data. Mobility data is the data about the geographic location of a device passively produced through normal activity. We used mobility data to understand patterns of COVID-19's spread and the impact of disease control measures. This mobility dataset from Google measures visitor numbers to specific categories of location (e.g., grocery stores, workplace, etc.) every day and compares this change relative to baseline days before the pandemic outbreak. Baseline days represent a normal value for that day of the week and are given as the median daily visits to each place over the period and it is helpful because the people have different routine work for a week. The schematic representation of the proposed work is presented in Figure 1.
In Figure 1, we have the following notions briefly:
● Each device (state) gets a copy of the global ML model from the server. This model may be an initial version with just random weights or could be one that has been trained in the past.
● The device collects local data and trains its local copy of the model (w1,w2…) using this data.
● This device then sends its model changes (Δw1,Δw2…) to the server (e.g., updates to the model's weights). These Δ′s represent the dissimilarity between the initial model and the trained model, which means that the primary training data is never sent outside the device.
● The server combines the Δw1,Δw2… from the respective devices to update the global model. After the combined changes are consolidated into the global model W, the new and improved version of the global model W is ready for inference. This global update W can then be sent back to the devices and/or used for speculation in the server. Using the updated global model, the global parameters can be estimated.
Hence, our main contributions to this paper are listed as follows:
ⅰ) Using Multi-task learning under federated environments, the infection spreading rate β of COVID-19 is predicted under mobility constraints.
ⅱ) The availability of bed facilities in the hospital is determined using predictive models.
ⅲ) Various machine learning algorithms are compared with the FMTL approach.
We aim to model the mobility based COVID-19 scenario by considering the privacy of the data. The flow diagram of the proposed algorithm is shown in Figure 2. This experiment is conducted in two stages. In stage one, we try to predict the US state wise infection numbers in a fed Data privacy could also be an issue when dealing with medical data, including in the COVID-19 scenario. Therefore, a federated learning approach is employed to address this issue. The infection number is modeled as a function of the categories in the mobility data using the federated multi-task learning approach in [38]. Even though the cases in each state are observed independently, it is only logical that there will be some relatedness in the COVID-19 trend between the states. Therefore using multitask learning [39] would be a good fit.
In stage two of the experiment, we emulate the pandemic using the classic SEIR model to analyse the rise and extinction of the pandemic. The infection number obtained in stage-1 is used as the β(Probability of disease transmission per contact × the number of contacts per unit time) for the mobility based SEIR model. Also the susceptible, exposed, infected and recovered ratios of the states are compared. Finally, we try to put a threshold on the infection rate and discuss the time till which vaccination must be continued to prevent regions from running out of bed availability at medical care centers.
The proposed epidemic FMTL framework inherits the traditional FMTL [38]. Several epidemic prediction methods are estimating the parameter of their model, estimation is based on existing medical data. The idea of FMTL is to learn infection rates from mobility data. In FMTL the mobility data will include the effect of social distancing and lockdown restrictions, and the movement of people in a region visiting different places or locations. Federated learning activities of Ml(l=1,2,...,m) region in a network based on their data, and each location generates data with distinct distribution, so far each sub region gets separate models, W={w1,w2,...,wm}T for each local data set. Each device model has similarities between the models, using multitask learning [40] to improve the performance of the structure of model.
minW,Ωm∑t=11ntnt∑i=1lt(wTt,xit,yit)+R(W,Ω),such that trace(M−1)=1,M−1≥0, | (3.1) |
where lt is the loss function, R(W,Ω)=tr(WT(λ1Ω+λ2I)W), and M−1=λ1Ω+λ2I,λ1,λ2>0, Ω∈Rm×m is the matrix that shows the relationship of models among the tasks and it is clear that strongly convex concerning M−1.
Considering dual formulation of the (3.1) will give the global problem into local sub problem for federated computations:
minγ{D(γ):=m∑t=1nt∑i=1l∗t(−γit)+R∗(Xγ)}, | (3.2) |
where l∗t and R∗ are the conjugate dual function of lt and R.
We can define a local sub problem of the original dual optimization problem, which is formed by using a quadratic approximation of the dual problem to separate nodes for computational purposes.
minΔγtGσ′t=nt∑i=1l∗t(−γit−Δγit)+⟨wt(γ),XtΔγt⟩+σ′2‖XtΔγt‖2Mt+k(γ), | (3.3) |
where k(γ)=R∗(Xγ)m and Mt∈Rd×d is the tth diagonal block of the symmetric positive definite matrix.
In every sub problem, we are finding the update for the model and aggregating all sub region models. Finally we have an aggregated model for a given region. This new model also benefited from other sub region models through the multitask learning framework. It will provide the best parameter estimation to estimate the infection rate from the mobility data and overcome the issue of over fitting.
For mathematically modeling of infectious diseases, we used to apply epidemic models [41]. Usually, the population is assigned to compartments with labels - for example, S, E, I, R, (Susceptible, Exposed, Infected, Recovered, ..) etc., of the disease pattern. In recent years, individual based disease propagation models are analysed based on mean field approach. Here we consider mobility-based SEIR epidemic model.
Assume that Nl is the population at the location l∈{1,2,..m}. Let j∈{1,2,..m} represent a set of locations which are connected to location l. Hence, ∑jNj is the maximum number of possible individuals connected from location j to location l. Let Aj,l represents the mobility of individuals to location l from location j. Here the total population is subdivided into four compartments for location l as follows:
● sl(t) represents fraction of susceptible individual in location l at time t.
● il(t) represents fraction of infected individual in location l at time t.
● el(t) represents fraction of exposed individual in location l at time t.
● rl(t) represents fraction of recovered individual in location l at time t.
Medical interventions like vaccination are more effective in preventing disease propagation. Disease spread can be controlled through this vaccination campaign. So, we consider that u is the vaccination proportion of the population, α is the average incubation rate(that is α=1average incubation time), β is the spreading rate and η is the recovery rate. The mobility based SEIR epidemic model for COVID-19 is defined as follows
dsldt=−(1−u)βslm∑j=1Aj,lijdeldt=(1−u)βslm∑j=1Aj,lij−αeldildt=αel−ηildrldt=ηil | (3.4) |
Consider the infection free equilibrium(IFE) E0=(em,0,0,0) where em and 0 are unit and zero row vectors of order m.
By using next generation matrix approach, we can determine the reproduction number R0 as follows:
R0=λmax(A)((1−u)βη) | (3.5) |
where λmax(A) is the maximum eigenvalue of mobility matrix A.
The asymptotic stability of the IFE points is represented in terms of R0. Following [42] we can directly obtain that E0 is locally asymptotically stable if R0<1 and unstable if R0>1. Theorem 1 proves the global stability of the IFE, E0.
Theorem 1. If R0<1, then the IFE E0 is globally asymptotically stable in Ω1={(S,E,I,R):sl+el+il+rl≤1∀l∈{1,2,...,m}} where S=(s1,s2,...,sm),E=(e1,e2,...,em),I=(i1,i2,...,im), and R=(r1,r2,...,rm).
Proof. Let Ll(t)=el(t)+il(t). By taking the derivative of Ll(t) with respect to time, we have,
L′l(t)=(1−u)βslm∑j=1Ajlij−ηil | (3.6) |
At E0, Eq (3.6) can be expressed in the long-run as follows:
L′l≤(1−u)ηβm∑j=1(Ajl−ejj)ij | (3.7) |
where ejj is 1. Thus L′l≤0 when R0<1. As a result, for every location l, Ll is Lyapunov function on Ω1. Using Lasalle's invariant principle, the largest compact invariant set for model (3.4) is E0 which completes the proof.
Using the following FMTL algorithm, we can determine the updated spreading rate β. Here, "baseline" is the median daily visits to each place over the period comprising January 3 through February 6, 2020.
Algorithm 1 Infection Rate - FMTL Algorthm |
Require: Mobility data Ml where l∈ location l=1,2,...m distributed across n nodes with initial matrix Ω0,γ(0)=0∈Rm,v(0)=0∈Rp for Global Epochs g=0,1,2... do SET sub-problem parameter σ0 and the number of federated iterations F for each location l;l∈{1,2,...m} do GET Δγl of the local sub-problem from local servers UPDATE γl←γl+Δγl RETURN ΔVl=MlΔγl REDUCE Vl←Vl+ΔVl UPDATE Ω centrally based on w(γ) for latest γ COMPUTE wl=w(γ) based on the latest γ at the central node end for end for PREDICT infection number for day j, ζj=WTMj, for the next t days COMPUTE infection rate β=∑tj=1ζjt |
We use the mobility data [43] and the US state-wise COVID-19 data [44] to model phase 1 of the experiment. Data provided by Google [43], which is curated from March 2020 until August 2021 used for modeling represent mobility vectors using notation. The mobility vector contains the percentage change from a baseline in the volume of people visiting a particular category of public place(namely retail and recreation, grocery, and pharmacy, parks, transit stations, and residential). The mobility data of the US for the year 2020 can be seen in Figure 3.
The US state-wise case count maintained live by the New York Times is utilized. This data contains all the covid cases starting from 14-01-2020 till the present day. For phase 2, US state-wise population and bed availability is required. The former was taken from [45] and the latter was collected from [46].
Our objective for stage 1 is to find the function that map from mobility vector to infection number. Inherently, people's movement incurs the spread of the disease and we try to capture that correlation. The state-wise COVID-19 data are given as aggregated figures i.e., the data contains the total number of cases up today D, where D ranges from 2020-03-14 to 2021-08-21. However, the state-wise recorded number for a particular day is required for building the analytic model. Therefore, a rolling difference is taken between day D and D-1 for COVID-19 cases and this first-order difference is employed throughout the experiment.
The mobility data-set consists of the data for every country and sub-regions. However, for our experiment, we only took the US mobility data for the years 2020 and 2021 and appended it to a single source. We also only stick on analyzing the state-level data, so we further filter it based on sub-regions and remove other micro-level divisions.
Finally, both the mobility and infection data are joined by their corresponding states and date. This data contains all US states with their corresponding mobility and infection numbers. Nevertheless, the states show erratic trends and patterns in terms of both infection spread and movement of people. This could be the result of the state's population, foreign population lockdown policies, etc. The geographic distribution of COVID-19 cases across states can be visualized from the map plot in Figure 4. As a result, to obtain a better model, the scenario is to identify the states that show similar trends and proceed with the prediction for these chosen states. This is done to reduce the heterogeneity in the system. Firstly the mean and total case counts as shown in Table-1 are considered, followed by the similarity in a pattern followed during the two waves of COVID-19 spread as depicted in Figures 5 and 6. Five states were chosen for the experiment which include Alabama, Colorado, Kentucky, Minnesota, and Washington.
Records with less than 50 cases per day and more than 10,000 cases per day were removed, considering them as extreme values. Data ranging from 2020-05-01 to 2021-06-01 were trained and data validation was performed on the data ranging from 2021-06-01 and 2021-08-01.
Each state is considered as a task(node) in the federated setup. Even if the spread of cases in each state is different, there still exists a correlation between those states. Using multi-task learning, unravel the relatedness among the nodes which can be included while building the model.
Therefore, in each node, a linear regression model is built corresponding to a state, which is, then aggregated following the MOCHA framework as proposed in [38]. Initial parameters, hyperparameters, and settings are mimicked from [38] as well. Each node has a minimum of 395 points for Minnesota and a maximum of 420 points for Washington. This data is then used to learn the regression model in three ways: multitask, local and global models. 5-fold cross-validation is done so as to obtain the optimal regularization parameter from 1e−5,1e−4,1e−3,1e−2,0.1,1,10. The process is iterated 10 times and the average of errors for predictions across iterations is reported. The weight parameter from the training is given in Table 2.
State | Total cases | Average case count |
Alabama | 839947 | 1372.46 |
Colorado | 793899 | 1280.48 |
Kentucky | 768090 | 1240.86 |
Minnesota | 847010 | 1368.35 |
Washington | 756733 | 1184.25 |
Weights (W) | Public places |
-24.58 | retail and recreation percent change from baseline |
21.79 | grocery and pharmacy percent change from baseline |
-4.13 | parks percent change from baseline |
-4.44 | transit stations percent change from baseline |
-37.42 | workplaces percent change from baseline |
-60.55 | residential percent change from baseline |
Infection number XTW is obtained from the dot product of the mobility vector and weights learned from training where X: Mobility data, W: weight corresponding to mobility feature vector in Table 2.
The covid pandemic for 1000 days starting from 2021-06-01 using the SEIR model. The population of states is taken from [45] which is used as one of the initial parameters for the start of the simulation. β is determined by averaging the infection numbers predicted from phase 1 of the experiment. Incubation time was fixed at a standard 7 days and reproduction ratio was taken as 2.
We have included a vaccination parameter u in the model to indicate the percentage of the population who have been vaccinated. Here u=0 indicates no vaccination and u=1 indicates that everyone is vaccinated. The α,β and γ of states are as in Table 3.
State | α% | β% | γ% |
Alabama | 0.14285714285714285 | 0.011674899882130375 | 0.0025818812733749853 |
Colorado | 0.14285714285714285 | 0.00810977051954281 | 0.002442870349158038 |
Kentucky | 0.14285714285714285 | 0.008496661147563526 | 0.00245179535677162 |
Minnesota | 0.14285714285714285 | 0.0020389879314963886 | 0.0005762155029835365 |
Washington | 0.14285714285714285 | 0.00946 | 0.00034070090325599126 |
In Table 4, we defined five different model parameters' values. We have compared the error results of the five models using two metrics: root mean squared percentage error(RMSPE) and mean absolute percentage error(MAPE), as in Figure 7, and the values can be seen in Table 5. Federated learning has lesser error than all the other models, proving a better fit. Federated learning has the least MAPE of 4.4%, while the neural network has the highest MAPE of 15.12 %. Overall the statistical models perform better than the machine learning models, which the scarcity of data could reason.
Model | Parameters |
Neural Network | number of Hidden Layers = 3, neurons count at each layer = [128, 64, 1] |
activation = relu, solver = adams optimizer, α = 0.001 | |
learning rate = 0.5, number of iterations = 100 | |
Multiple Linear Regression | Optimization algorithm = Stochastic Gradient Descent |
Decision tree | CART Algorithm |
Random Forest | max depth =[1,2,3....,10] |
number of trees =[5,10,15,20] | |
FMTL | λ=[1e−5,1e−4,1e−3,1e−2,0.1,1,10], |
5-fold cross validation, trials =10 |
Models | RMSPE(%) | MAPE(%) |
FMTL | 2.10 | 4.4 |
Decision Tree | 3.23 | 15.11 |
Linear Regression | 4.22 | 9.46 |
Neural Net | 3.13 | 9.46 |
Random Forest | 2.68 | 9, 57 |
The predictions for the state-wise COVID-19 cases are tabulated in Table 6 and to check the confidence of the prediction model we have compared the cumulative case counts between the actual and prediction, which are visualised in Figure 8–12. In Figure 13, the RMSE and MAPE errors of cumulative cases between actual and predicted values are shown in bar chart.
State | MAPE(%) | RMSPE(%) |
Alabama | 3.23 | 2.41 |
Colorado | 2.10 | 1.83 |
Kentucky | 3.13 | 2.28 |
Minnesota | 4.22 | 12.28 |
Washington | 2.68 | 3.10 |
The fraction that is susceptible, infected, exposed, and recovered from simulations for the states(Stage 2) are compared in Figures 14–17. These simulations show that it will take at least another 400 days for the pandemic to reach near extinction given no proper vaccination or social distancing is followed.
One additional constraint has been introduced in the mobility based SEIR model to check the availability of beds in a particular state so that the situation can be managed effectively as in [46]. The simple constraint is that infection number < no of beds available. Given the bed availability constraint, our vaccination parameter u, will fine-tune and show the percentage of vaccination to be done to maintain the bed availability in hospitals.
We simulated a scenario for Colorado state where bed availability is 50% and the infection rate goes beyond that. So we fix the constraint and simulate it, which can be seen in Figure 18. This implies that vaccination drives should be done effectively for at least another 200 days to maintain the infection number to less than 50%. Similar insights can be derived for other states as well.
In this paper, we have proposed an FMTL to predict the number of state-wise COVID-19 infected people in the US based on mobility. Mobility is considered by using data privacy into consideration. Here, due to heterogeneous data, a personalized model is learned by federated multitask learning (FMTL) to predict the updated infection rate of COVID-19 in the USA using the mobility-based SEIR model. So, the infection rate obtained in the FMTL approach dynamically is used as the β for the SEIR model. In addition, the susceptible, exposed, infected and recovered ratios of the states are compared. Our experimental results show that the root-mean-square percentage error for the actual and prediction of COVID-19 cases is low for Colorado state and high for Minnesota state. Also, based on the threshold value of the infected rate, we conclude that it will take at least 400 days to reach extinction when there is no proper vaccination or social distance.
The authors declare there is no conflict of interest.
[1] |
D. Cucinotta, M.Vanelli, WHO declares COVID-19 a pandemic, Acta. Biomed., 91 (2020), 157–160. https://doi.org/10.23750/abm.v91i1.9397 doi: 10.23750/abm.v91i1.9397
![]() |
[2] |
T. Fisayo, S. Tsukagoshi, Three waves of the COVID-19 pandemic, Postgrad. Med. J., 97 (2021), 332. https://doi.org/10.1016/j.ijsu.2020.04.018 doi: 10.1016/j.ijsu.2020.04.018
![]() |
[3] |
M. Nicola, Z. Alsafi, C. Sohrabi, A. Kerwan, A. Al-Jabir, C. Iosifidis, et al., The socio-economic implications of the coronavirus pandemic (COVID-19): A review, Int. J. Surg., 78 (2020), 185–193. https://doi.org/10.1016/j.ijsu.2020.04.018 doi: 10.1016/j.ijsu.2020.04.018
![]() |
[4] |
Z. Zheng, Z. Xie, Y. Qin, Exploring the influence of human mobility factors and spread prediction on early COVID-19 in the USA, BMC Public Health, 21 (2021). https://doi.org/10.1186/s12889-021-10682-3 doi: 10.1186/s12889-021-10682-3
![]() |
[5] | J. Konecny, H. B. McMahan, F. X. Yu, P. Richtárik, A. T. Suresh, D. Bacon, Federated learning: Strategies for improving communication efficiency, in 29th Conference on Neural Information Processing Systems, 2016. |
[6] | K. Bonawitz, H. Eichner, W. Grieskamp, D. Huba, A. Ingerman, V. Ivanov, et al., Towards federated learning at scale: System design, Proc. Mach. Learn. Syst., 1 (2019), 374–388. |
[7] |
S. Azam, J. E. Macías-Díaz, N. Ahmed, I. Khan, M. S. Iqbal, Numerical modeling and theoretical analysis of a nonlinear advection-reaction epidemic system, Comput. Methods Programs Biomed., 193 (2020), 67–83. https://doi.org/10.1016/j.cmpb.2020.105429 doi: 10.1016/j.cmpb.2020.105429
![]() |
[8] |
M. Rafiq, J. E. Macías-Díaz, A. Raza, N. Ahmed, Design of a nonlinear model for the propagation of COVID-19 and its efficient nonstandard computational implementation, Appl. Mathl. Model., 89 (2021), 1835–1846. https://doi.org/10.1016/j.apm.2020.08.082 doi: 10.1016/j.apm.2020.08.082
![]() |
[9] |
J. E. Macías-Díaz, N. Ahmed, M. Rafiq, Analysis and nonstandard numerical design of a discrete three-dimensional hepatitis B epidemic model, Mathematics, 7 (2019). https://doi.org/10.3390/math7121157 doi: 10.3390/math7121157
![]() |
[10] |
J. Guan, Y. Wei, Y. Zhao, F. Chen, Modeling the transmission dynamics of COVID-19 epidemic: a systematic review, J. Biomed. Res., 34 (2020), 422–430. https://doi.org/10.7555/JBR.34.20200119 doi: 10.7555/JBR.34.20200119
![]() |
[11] |
R. ud Din, A. R. Seadawy, K. Shah, A. Ullah, D. Baleanu, Study of global dynamics of COVID-19 via a new mathematical model, Results Phys., 19 (2020). https://doi.org/10.1016/j.rinp.2020.103468 doi: 10.1016/j.rinp.2020.103468
![]() |
[12] |
I. F. Mello, L. Squillante, G. O. Gomes, A. C. Seridonio, M. de Souza, Epidemics, theIsing-model and percolation theory: A comprehensive review focused on Covid-19, Phys. A: Statist. Mech. Appl., 573 (2021). https://doi.org/10.1016/j.physa.2021.125963 doi: 10.1016/j.physa.2021.125963
![]() |
[13] |
H. A. Adekola, I. A. Adekunle, H. O. Egberongbe, S. A. Onitilo, I. N. Abdullahi, Mathematical modeling for infectious viral disease: The COVID-19 perspective, J. Public Aff., 20 (2020). https://doi.org/10.1002/pa.2306 doi: 10.1002/pa.2306
![]() |
[14] |
F. Özköse, M. Yavuz, M. T. Şenel, R. Habbireeh, Fractional order modelling of omicron SARS-CoV-2 variant containing heart attack effect using real data from the United Kingdom, Chaos, Solitons Fractals, 157 (2022), 111954. https://doi.org/10.1016/j.chaos.2022.111954 doi: 10.1016/j.chaos.2022.111954
![]() |
[15] |
P. A. Naik, M. Yavuz, S. Qureshi, Modeling and analysis of COVID-19 epidemics with treatment in fractional derivatives using real data from Pakistan, Eur. Phys. J. Plus, 135 (2020), 795. https://doi.org/10.1140/epjp/s13360-020-00819-5 doi: 10.1140/epjp/s13360-020-00819-5
![]() |
[16] |
B. Dasbasi, Stability analysis of an incommensurate fractional-order SIR model, Math. Modell. Numer. Simul. Appl., 1 (2021). https://doi.org/10.53391/mmnsa.2021.01.005 doi: 10.53391/mmnsa.2021.01.005
![]() |
[17] |
P. Kumar, V. S. Erturk, Dynamics of cholera disease by using two recent fractional numerical methods, Math. Modell. Numer. Simul. Appl., 1 (2021), 102–111. https://doi.org/10.53391/mmnsa.2021.01.010 doi: 10.53391/mmnsa.2021.01.010
![]() |
[18] |
F. Özköse, M. Yavuz, Investigation of interactions between COVID-19 and diabetes with hereditary traits using real data: A case study in Turkey, Comput. Biol. Med., 141 (2022). https://doi.org/10.1016/j.compbiomed.2021.105044 doi: 10.1016/j.compbiomed.2021.105044
![]() |
[19] |
H. Joshi, B. K. Jha, Chaos of calcium diffusion in Parkinson's infectious disease model and treatment mechanism via Hilfer fractional derivative, Math. Modell. Numer. Simul. Appl., 1 (2021), 84–94. https://doi.org/10.53391/mmnsa.2021.01.008 doi: 10.53391/mmnsa.2021.01.008
![]() |
[20] |
F. Özköse, M. T. Şenel, R. Habbireeh, Fractional-order mathematical modelling of cancer cells-cancer stem cells-immune system interaction with chemotherapy, Math. Modell. Numer. Simul. Appl., 1 (2021), 67–83. https://doi.org/10.53391/mmnsa.2021.01.007 doi: 10.53391/mmnsa.2021.01.007
![]() |
[21] |
M. Yavuz, F. Ö. Coşar, F. Günay, F. N. Özdemir, A new mathematical modeling of the COVID-19 pandemic including the vaccination campaign, Open J. Modell. Simul., 9 (2021), 299–321. https://doi.org/10.4236/ojmsi.2021.93020 doi: 10.4236/ojmsi.2021.93020
![]() |
[22] |
S. Allegretti, I. M. Bulai, R. Marino, M. A. Menandro, K. Parisi, Vaccination effect conjoint to fraction of avoided contacts for a Sars-Cov-2 mathematical model, Math. Modell. Numer. Simul. Appl., 1 (2021), 56–66. https://doi.org/10.53391/mmnsa.2021.01.006 doi: 10.53391/mmnsa.2021.01.006
![]() |
[23] |
R. Ikram, A. Khan, M. Zahri, A. Saeed, M. Yavuz, P. Kumam, Extinction and stationary distribution of a stochastic COVID-19 epidemic model with time-delay, Comput. Biol. Med., 141 (2022). https://doi.org/10.1016/j.compbiomed.2021.105115 doi: 10.1016/j.compbiomed.2021.105115
![]() |
[24] |
M. A. Achterberg, B. Prasse, L. Ma, S. Trajanovski, M. Kitsak, P. V. Mieghem, Comparing the accuracy of several network-based COVID-19 prediction algorithms, Int. J. Forecast., 38 (2022), 489–504. https://doi.org/10.1016/j.ijforecast.2020.10.001 doi: 10.1016/j.ijforecast.2020.10.001
![]() |
[25] |
E. Hernández-Pereira, O. Fontenla-Romero, V. Bolón-Canedo, Machine learning techniques to predict different levels of hospital care of CoVid-19, Appl. Intell., 52 (2022), 6413–6431. https://doi.org/10.1007/s10489-021-02743-2 doi: 10.1007/s10489-021-02743-2
![]() |
[26] |
J. Köhler, L. Schwenkel, A. Koch, J. Berberich, P. Pauli, F. Allgöwer, Robust and optimal predictive control of the COVID-19 outbreak, Ann. Rev. Control, 51 (2021), 525–539. https://doi.org/10.1016/j.arcontrol.2020.11.002 doi: 10.1016/j.arcontrol.2020.11.002
![]() |
[27] |
M. M. Morato, S. B. Bastos, D. O. Cajueiro, J. E. Normey-Rico, An optimal predictive control strategy for COVID-19 (SARS-CoV-2) social distancing policies in Brazil, Ann. Rev. Control, 50 (2020), 417–431. https://doi.org/10.1016/j.arcontrol.2020.07.001 doi: 10.1016/j.arcontrol.2020.07.001
![]() |
[28] |
X. Yan, Y. Zou, Optimal and sub-optimal quarantine and isolation control in SARS epidemics, Math. Comput. Model., 47 (2008), 235–245. https://doi.org/10.1016/j.mcm.2007.04.003 doi: 10.1016/j.mcm.2007.04.003
![]() |
[29] | M. Khouzani, S. S. Venkatesh, S. Sarkar, Market-based control of epidemics, in 2011 49th Annual Allerton Conference on Communication, Control, and Computing (Allerton), (2011), 314–320. https://doi.org/10.1109/Allerton.2011.6120184 |
[30] | A. Argyriou, T. Evgeniou, M. Pontil, Multi-task feature learning, In NIPS '06, 19 (2006). |
[31] |
M. Hayhoe, F. Barreras, V. M. Preciado, Multitask learning and nonlinear optimal control of the COVID-19 outbreak: A geometric programming approach, Ann. Rev. Control, 52 (2021), 495–507, https://doi.org/10.1016/j.arcontrol.2021.04.014 doi: 10.1016/j.arcontrol.2021.04.014
![]() |
[32] |
T. Li, A. K. Sahu, A. Talwalkar, V. Smith, Federated learning: Challenges, methods, and future directions, IEEE Sig. Process Mag., 37 (2020), 50–60. https://doi.org/10.1109/MSP.2020.2975749 doi: 10.1109/MSP.2020.2975749
![]() |
[33] | S. Caldas, S. M. K. Duddu, P. Wu, T. Li, J. Konečný, H. B. McMahan, et al., Leaf: A benchmark for federated settings, 2018. https://doi.org/10.48550/arXiv.1812.01097 |
[34] | T. Li, A. K. Sahu, M. Zaheer, M. Sanjabi, A. Talwalkar, Federated optimization in heterogeneous networks, Proc. Mach. Learn. Syst., 2 (2020), 429–450. |
[35] |
S. M. Abdul, S. Taha, M. Ramadan, COVID-19 detection using federated machine learning, PLoS One, 16 (2021). https://doi.org/10.1371/journal.pone.0252573 doi: 10.1371/journal.pone.0252573
![]() |
[36] |
A. Vaid, S. K. Jaladanki, J. Xu, S. Teng, Federated learning of electronic health records to improve mortality prediction in hospitalized patients with COVID-19: Machine learning approach, JMIR Med. Inf., 9 (2021). https://doi.org/10.2196/24207 doi: 10.2196/24207
![]() |
[37] |
M. U. Alam, R. Rahmani, Federated semi-supervised multi-task learning to detect COVID-19 and lungs segmentation marking using chest radiography images and raspberry pi devices: An internet of medical things application, Sensors, 21 (2021). https://doi.org/10.3390/s21155025 doi: 10.3390/s21155025
![]() |
[38] | V. Smith, C. K. Chiang, M. Sanjabi, A. Talwalkar, Federated multi-task learning, Adv. Neural Inf. Proc. Syst., 30 (2017), 4424–4434, |
[39] |
A. Argyriou, T. Evgeniou, M. Pontil, A. Argyriou, T. Evgeniou, M. Pontil, Convex multi-task feature learning, Mach. Learn., 73 (2008), 243–272. https://doi.org/10.1007/s10994-007-5040-8 doi: 10.1007/s10994-007-5040-8
![]() |
[40] | A. Argyriou, C. A. Micchelli, M. Pontil, Y. Ying, A spectral regularization framework for multi-task structure learning, in International Conference on Neural Information, 20 (2007). |
[41] |
W. O. Kermack, A. G. Mckendrick, Contribution to the mathematical theory of epidemics, Proc. R. Soc. London Ser A, 115 (1927), 700–721. https://doi.org/10.1098/rspa.1927.0118 doi: 10.1098/rspa.1927.0118
![]() |
[42] |
P. van den Driessche, Reproduction numbers of infectious disease models, Infect. Dis. Modell., 2 (2017), 288–303. https://doi.org/10.1016/j.idm.2017.06.002 doi: 10.1016/j.idm.2017.06.002
![]() |
[43] | See How Your Community is Moving Around Differently due to COVID-19, Google, 2021. Available from: https://www.google.com/covid19/mobility/index.htmlhl=en. |
[44] | COVID-19 Data in the United States, Github, 2021. Available from: https://github.com/nytimes/covid-19-data. |
[45] | National Population Totals: 2010–2020, US Census Bureau, 2020. Available from: https://www.census.gov/programs-surveys/popest/technical-documentation/research/evaluation-estimates/2020-evaluation-estimates/2010s-totals-national.html. |
[46] | COVID-19 Hospital Data, HealthData.gov, 2022. |
1. | Abdul Majeed, Xiaohan Zhang, On the Adoption of Modern Technologies to Fight the COVID-19 Pandemic: A Technical Synthesis of Latest Developments, 2023, 3, 2673-8112, 90, 10.3390/covid3010006 | |
2. | Abdul Majeed, Xiaohan Zhang, Seong Oun Hwang, Applications and Challenges of Federated Learning Paradigm in the Big Data Era with Special Emphasis on COVID-19, 2022, 6, 2504-2289, 127, 10.3390/bdcc6040127 | |
3. | Bowen Wang, Ziwen Cheng, Liu Yi, Zhu Cheng, A Novel Disease Prediction Model Based on Blockchain and Federated Learning, 2023, 2504, 1742-6588, 012049, 10.1088/1742-6596/2504/1/012049 | |
4. | Zhiqing Huang, Xiao Zhang, Yanxin Zhang, Yusen Zhang, FedSH: a federated learning framework for safety helmet wearing detection, 2024, 36, 0941-0643, 10699, 10.1007/s00521-024-09632-y | |
5. | Laura C. Zwiers, Diederick E. Grobbee, Alicia Uijl, David S. Y. Ong, Federated learning as a smart tool for research on infectious diseases, 2024, 24, 1471-2334, 10.1186/s12879-024-10230-5 | |
6. | Yang Ye, Abhishek Pandey, Carolyn Bawden, Dewan Md. Sumsuzzman, Rimpi Rajput, Affan Shoukat, Burton H. Singer, Seyed M. Moghadas, Alison P. Galvani, Integrating artificial intelligence with mechanistic epidemiological modeling: a scoping review of opportunities and challenges, 2025, 16, 2041-1723, 10.1038/s41467-024-55461-x |
Algorithm 1 Infection Rate - FMTL Algorthm |
Require: Mobility data Ml where l∈ location l=1,2,...m distributed across n nodes with initial matrix Ω0,γ(0)=0∈Rm,v(0)=0∈Rp for Global Epochs g=0,1,2... do SET sub-problem parameter σ0 and the number of federated iterations F for each location l;l∈{1,2,...m} do GET Δγl of the local sub-problem from local servers UPDATE γl←γl+Δγl RETURN ΔVl=MlΔγl REDUCE Vl←Vl+ΔVl UPDATE Ω centrally based on w(γ) for latest γ COMPUTE wl=w(γ) based on the latest γ at the central node end for end for PREDICT infection number for day j, ζj=WTMj, for the next t days COMPUTE infection rate β=∑tj=1ζjt |
State | Total cases | Average case count |
Alabama | 839947 | 1372.46 |
Colorado | 793899 | 1280.48 |
Kentucky | 768090 | 1240.86 |
Minnesota | 847010 | 1368.35 |
Washington | 756733 | 1184.25 |
Weights (W) | Public places |
-24.58 | retail and recreation percent change from baseline |
21.79 | grocery and pharmacy percent change from baseline |
-4.13 | parks percent change from baseline |
-4.44 | transit stations percent change from baseline |
-37.42 | workplaces percent change from baseline |
-60.55 | residential percent change from baseline |
State | α% | β% | γ% |
Alabama | 0.14285714285714285 | 0.011674899882130375 | 0.0025818812733749853 |
Colorado | 0.14285714285714285 | 0.00810977051954281 | 0.002442870349158038 |
Kentucky | 0.14285714285714285 | 0.008496661147563526 | 0.00245179535677162 |
Minnesota | 0.14285714285714285 | 0.0020389879314963886 | 0.0005762155029835365 |
Washington | 0.14285714285714285 | 0.00946 | 0.00034070090325599126 |
Model | Parameters |
Neural Network | number of Hidden Layers = 3, neurons count at each layer = [128, 64, 1] |
activation = relu, solver = adams optimizer, α = 0.001 | |
learning rate = 0.5, number of iterations = 100 | |
Multiple Linear Regression | Optimization algorithm = Stochastic Gradient Descent |
Decision tree | CART Algorithm |
Random Forest | max depth =[1,2,3....,10] |
number of trees =[5,10,15,20] | |
FMTL | λ=[1e−5,1e−4,1e−3,1e−2,0.1,1,10], |
5-fold cross validation, trials =10 |
Models | RMSPE(%) | MAPE(%) |
FMTL | 2.10 | 4.4 |
Decision Tree | 3.23 | 15.11 |
Linear Regression | 4.22 | 9.46 |
Neural Net | 3.13 | 9.46 |
Random Forest | 2.68 | 9, 57 |
State | MAPE(%) | RMSPE(%) |
Alabama | 3.23 | 2.41 |
Colorado | 2.10 | 1.83 |
Kentucky | 3.13 | 2.28 |
Minnesota | 4.22 | 12.28 |
Washington | 2.68 | 3.10 |
Algorithm 1 Infection Rate - FMTL Algorthm |
Require: Mobility data Ml where l∈ location l=1,2,...m distributed across n nodes with initial matrix Ω0,γ(0)=0∈Rm,v(0)=0∈Rp for Global Epochs g=0,1,2... do SET sub-problem parameter σ0 and the number of federated iterations F for each location l;l∈{1,2,...m} do GET Δγl of the local sub-problem from local servers UPDATE γl←γl+Δγl RETURN ΔVl=MlΔγl REDUCE Vl←Vl+ΔVl UPDATE Ω centrally based on w(γ) for latest γ COMPUTE wl=w(γ) based on the latest γ at the central node end for end for PREDICT infection number for day j, ζj=WTMj, for the next t days COMPUTE infection rate β=∑tj=1ζjt |
State | Total cases | Average case count |
Alabama | 839947 | 1372.46 |
Colorado | 793899 | 1280.48 |
Kentucky | 768090 | 1240.86 |
Minnesota | 847010 | 1368.35 |
Washington | 756733 | 1184.25 |
Weights (W) | Public places |
-24.58 | retail and recreation percent change from baseline |
21.79 | grocery and pharmacy percent change from baseline |
-4.13 | parks percent change from baseline |
-4.44 | transit stations percent change from baseline |
-37.42 | workplaces percent change from baseline |
-60.55 | residential percent change from baseline |
State | α% | β% | γ% |
Alabama | 0.14285714285714285 | 0.011674899882130375 | 0.0025818812733749853 |
Colorado | 0.14285714285714285 | 0.00810977051954281 | 0.002442870349158038 |
Kentucky | 0.14285714285714285 | 0.008496661147563526 | 0.00245179535677162 |
Minnesota | 0.14285714285714285 | 0.0020389879314963886 | 0.0005762155029835365 |
Washington | 0.14285714285714285 | 0.00946 | 0.00034070090325599126 |
Model | Parameters |
Neural Network | number of Hidden Layers = 3, neurons count at each layer = [128, 64, 1] |
activation = relu, solver = adams optimizer, α = 0.001 | |
learning rate = 0.5, number of iterations = 100 | |
Multiple Linear Regression | Optimization algorithm = Stochastic Gradient Descent |
Decision tree | CART Algorithm |
Random Forest | max depth =[1,2,3....,10] |
number of trees =[5,10,15,20] | |
FMTL | λ=[1e−5,1e−4,1e−3,1e−2,0.1,1,10], |
5-fold cross validation, trials =10 |
Models | RMSPE(%) | MAPE(%) |
FMTL | 2.10 | 4.4 |
Decision Tree | 3.23 | 15.11 |
Linear Regression | 4.22 | 9.46 |
Neural Net | 3.13 | 9.46 |
Random Forest | 2.68 | 9, 57 |
State | MAPE(%) | RMSPE(%) |
Alabama | 3.23 | 2.41 |
Colorado | 2.10 | 1.83 |
Kentucky | 3.13 | 2.28 |
Minnesota | 4.22 | 12.28 |
Washington | 2.68 | 3.10 |