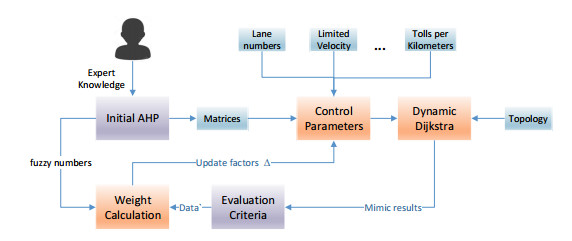
Concerning decisions for modern public transportation project, the lack of consensus between stakeholders and foreseeability of future transportation requirements might cause poor sustainability of the project. Unfortunately, many decision models give decision opinions without the test of the sustainability. Therefore, a dynamical Dijkstra simulation model is proposed to simulate the real traffic flows. In the model, the cost of the road connections is dynamically updated according to the change of the passenger flows. Then a combined decision support model using fuzzy AHP and dynamical Dijkstra simulation tests is designed. The combined model is capable of analyzing and creating consensus among different stakeholder participants in a transport development problem. The application of FAHP and dynamical Dijkstra ensures that the consensus creation is not only based on the FAHP decision making process but also on the response of the simulated execution of the decisions by dynamical Dijkstra. Thus, the decision makers by FAHP can firstly make their initial preferences in transportation planning, given the pairwise comparison matrices and generate the related weight for the traffic control parameters. And the dynamical Dijkstra simulations test the plan's setting and gives a response to iteratively adjust the FAHP matrices and parameters. The combined model is tested in different scenarios. And the results show that by the application of the proposed model, decision-makers can be more aware of the conflicts of interests among the involved groups, and they can pay more attention to possible violations causing by the change of traffic environment, including the citizen numbers, the construction cost, the roll cost, and etc., to get a more sustainable plan.
Citation: Xinlei Ma, Wen Chen, Zhan Gao, Tao Yang. Adaptive decision support model for sustainable transport system using fuzzy AHP and dynamical Dijkstra simulations[J]. Mathematical Biosciences and Engineering, 2022, 19(10): 9895-9914. doi: 10.3934/mbe.2022461
[1] | Zhongxue Yang, Yiqin Bao, Yuan Liu, Qiang Zhao, Hao Zheng, Wenbin Xu . Lightweight blockchain fuzzy decision scheme through MQTT and Fibonacci for sustainable transport. Mathematical Biosciences and Engineering, 2022, 19(12): 11935-11956. doi: 10.3934/mbe.2022556 |
[2] | Muhammad Akram, Maheen Sultan, José Carlos R. Alcantud, Mohammed M. Ali Al-Shamiri . Extended fuzzy N-Soft PROMETHEE method and its application in robot butler selection. Mathematical Biosciences and Engineering, 2023, 20(2): 1774-1800. doi: 10.3934/mbe.2023081 |
[3] | Yongfeng Yin, Routing Zhang, Qingran Su . Threat assessment of aerial targets based on improved GRA-TOPSIS method and three-way decisions. Mathematical Biosciences and Engineering, 2023, 20(7): 13250-13266. doi: 10.3934/mbe.2023591 |
[4] | Colella Ylenia, De Lauri Chiara, Improta Giovanni, Rossano Lucia, Vecchione Donatella, Spinosa Tiziana, Giordano Vincenzo, Verdoliva Ciro, Santini Stefania . A Clinical Decision Support System based on fuzzy rules and classification algorithms for monitoring the physiological parameters of type-2 diabetic patients. Mathematical Biosciences and Engineering, 2021, 18(3): 2654-2674. doi: 10.3934/mbe.2021135 |
[5] | Haiping Ren, Laijun Luo . A novel distance of intuitionistic trapezoidal fuzzy numbers and its-based prospect theory algorithm in multi-attribute decision making model. Mathematical Biosciences and Engineering, 2020, 17(4): 2905-2922. doi: 10.3934/mbe.2020163 |
[6] | Kun Zhang, Hanping Hou, Zhiqiang Dong, Ziheng Liu . Research on integrated inventory transportation optimization of inbound logistics via a VMI-TPL model of an existing enterprise. Mathematical Biosciences and Engineering, 2023, 20(9): 16212-16235. doi: 10.3934/mbe.2023724 |
[7] | Chaoyue Wang, Zhiyao Ma, Shaocheng Tong . Adaptive fuzzy output-feedback event-triggered control for fractional-order nonlinear system. Mathematical Biosciences and Engineering, 2022, 19(12): 12334-12352. doi: 10.3934/mbe.2022575 |
[8] | Wei Liu, Yi Huang, Yue Sun, Changlong Yu . Research on design elements of household medical products for rhinitis based on AHP. Mathematical Biosciences and Engineering, 2023, 20(5): 9003-9017. doi: 10.3934/mbe.2023395 |
[9] | Nian Zhang, Yifan Zhou, Qiang Pan, Guiwu Wei . Multi-attribute decision-making method with triangular fuzzy numbers based on regret theory and the catastrophe progression method. Mathematical Biosciences and Engineering, 2022, 19(12): 12013-12030. doi: 10.3934/mbe.2022559 |
[10] | Yefu Zheng, Jun Xu, Hongzhang Chen . TOPSIS-based entropy measure for intuitionistic trapezoidal fuzzy sets and application to multi-attribute decision making. Mathematical Biosciences and Engineering, 2020, 17(5): 5604-5617. doi: 10.3934/mbe.2020301 |
Concerning decisions for modern public transportation project, the lack of consensus between stakeholders and foreseeability of future transportation requirements might cause poor sustainability of the project. Unfortunately, many decision models give decision opinions without the test of the sustainability. Therefore, a dynamical Dijkstra simulation model is proposed to simulate the real traffic flows. In the model, the cost of the road connections is dynamically updated according to the change of the passenger flows. Then a combined decision support model using fuzzy AHP and dynamical Dijkstra simulation tests is designed. The combined model is capable of analyzing and creating consensus among different stakeholder participants in a transport development problem. The application of FAHP and dynamical Dijkstra ensures that the consensus creation is not only based on the FAHP decision making process but also on the response of the simulated execution of the decisions by dynamical Dijkstra. Thus, the decision makers by FAHP can firstly make their initial preferences in transportation planning, given the pairwise comparison matrices and generate the related weight for the traffic control parameters. And the dynamical Dijkstra simulations test the plan's setting and gives a response to iteratively adjust the FAHP matrices and parameters. The combined model is tested in different scenarios. And the results show that by the application of the proposed model, decision-makers can be more aware of the conflicts of interests among the involved groups, and they can pay more attention to possible violations causing by the change of traffic environment, including the citizen numbers, the construction cost, the roll cost, and etc., to get a more sustainable plan.
With the rapid growth of population and urbanization, each year thousands of new roads, subways and many other types of transportation lines are built in the world. How to ensure the new transportation projects could satisfy our requirements in a long term is a worldwide concerned problem. Currently, many countries have reached a consensus that sustainable transportation is the right solution.
Sustainable transportation can be referred to a type of transportation that allows the movement of goods and people by modalities that are sustainable from social, economic, and environmental perspectives [1]. Therefore, many factors should be taken in to considerations when a new transportation project is proposed. For example, its impact on the environments, the possible number of passengers, the cost and etc. And all these factors affecting the transportation sustainability should be considered when we make decisions for the project.
Multi-criteria decision making (MCDM) is a widely utilized approach for making rational decisions based on multiple factors and alternatives. It allows for comparison of alternatives to select the one with the highest overall utility. Before the construction of public traffic projects, MCDM is usually applied to select appropriate plans with contradictory objectives and factors of sustainability [2]. For example, Zhou [3] proposed a coupling evaluation index model of urban spatial structure and public transport system to determine the weight of the index. Madhushree [4] applied an improved genetic algorithm to ship routing and scheduling problems, aiming at minimizing the risk and cost to achieve sustainable ship routing in an uncertain environment. Cam and Sezen [5] proposed a linear programming model for vehicle routing problem using a customized objective function to minimize the idle time of passenger station vehicles. Aslan and Yazici [6] improved the classical A-star algorithm to enable it to simultaneously plan routes for multiple start and end nodes. Surnin [7] conducted real-time evaluation of urban traffic conditions using big data analysis based on neural networks. In the route planning platform proposed by Ismael [8], the long and short-term memory model was employed to predict the traffic situation, which was in turn put into a route planner to construct an optimal green route. In addition to the above methods, analytic hierarchy process (AHP) is a well-known robust MCDM method to make decision for complex problems [9,10]. AHP incorporates the expert knowledge through a structured framework for organizing and analyzing complex and crucial decision problems. It carries out a pairwise comparative analysis between the alternatives and some criteria to achieve a specified goal, and selects the most suitable alternative by ranking the alternatives with respect to each of the criteria. The AHP approach has been successfully applied in many areas, including transport engineering, construction engineering, and etc. [11,12,13].
Public participation in decision making is a crucial issue, as the implementations will only be practical if they meet the requirement of citizens' travels. The subjective judgments based on the preference of decision-participants have a significant influence on the results. Therefore, avoiding the uncertainty and vagueness involved in the public evaluators' judgments becomes a crucial objective of decision makers. In fact, most of the decisions that address transportation are made under vague and uncertain situations.
However, the traditional AHP method does not take into account the uncertainty associated with the mapping of human judgment to a number by natural language [14]. The ranking of the AHP is somewhat imprecise. Moreover, the dependencies between variables of the AHP usually result in some inconsistencies in the weighting of criteria [15]. Thus it is considered to be inadequate when addressing uncertainty and impreciseness.
To solve the decision making problems under uncertainty and improve the decision quality of AHP, it is often integrated with other models [16,17], which are capable of dealing with ambiguous issues. For example, the sensitivity and uncertainty analyses are statistically evaluated and integrated with the AHP by using the Monte Carlo simulation (MCS) [18]. Frequency Ratio (FR) assessment model and spatial multi-criteria evaluation (SMCE) model are combined with knowledge-driven AHP models to make decisions for a sustainable planning of mitigations [19]. To augment the AHP model, both the MCS and FR methods require an inventory dataset, which is usually not available for all decision problems. Duleba [10] examined Pareto-optimality on real data pairwise comparison matrices gained from a public transport AHP survey. They proposed that conducting Pareto optimality test on real pairwise comparison data can be successful in a sense that non-Pareto optimal weight vector could be detected in the AHP structure.
Obviously, the fuzzy theory is superior in most of the MCDM applications as it is capable of representing vague measures within mathematical operators to make decisions in the fuzzy domain. Thus, currently, most of the researchers are inclined to integrate fuzzy theories with the hierarchical decision models [20,21,22,23], such as FAHP, fuzzy-TOPSIS to overcome the uncertain problems in decision making process. Buran [24] applied Fuzzy Analytic Hierarchy Process (F-AHP) to evaluate the business model, and extended the model to incorporate intuitive fuzzy sets (IFS) and spherical fuzzy sets. Wątrobski [25] used PROMETHEE Ⅱ and fuzzy TOPSIS to evaluate electric vans for city logistics applications. Ha et al. [26] used the AHP method integrated with fuzzy-TOPSIS. Yavuz [27] developed a Hierarchical Hesitant Fuzzy Linguistic model to evaluate alternative fuel heavy duty vehicles. Tuzkaya [28] developed an approach based on the concept of fuzzy AHP and the method of PROMETHEE Ⅰ and Ⅱ to evaluate the most environmental friendly way of transportation in the Marmara region, Turkey while considering all the concerned stakeholders. Awasthi [29] introduced a model based on affinity diagram, AHP and TOPSIS for sustainable city logistics planning under fuzzy uncertainty.
Despite all the efforts to model the uncertainty of a sustainable transportation evaluation problem in AHP methods, most of them were still hard to test applicability of the final decisions. Especially when we take the variable factors into consideration, such as the population size, the variation of transportation cost. Unfortunately, many decision models give decision opinions without evaluation with these variable factors and the test of the project's sustainability. Thus with regard to the sustainable evaluation of new constructed transportation lines, we cannot ensure the decisions made by fuzzy MCDM are compatible with the real situations in the near future.
In this study we concerned about the optimization of decisions for the transportation projects. Obviously, the Dijkstra simulation is suitable to simulate the passengers' transport behaviors and make a response to the decision makers based on the simulation results. In order to support consensual decision making concerning sustainable transport development, a fuzzy AHP decision model is proposed to working with a dynamical Dijkstra simulation model, which is designed to mimic the dynamical behavior of the transportation lines built based on the decision results and test whether the decision is sustainable through the mimic results. The fuzzy AHP and dynamical Dijkstra models are built from the aspect of different stakeholder groups: passengers and the local governance. It is believed that only the requirement of both the users (passengers) and providers (local governance) are simultaneously satisfied, the transportation can be sustainable. Once the result of dynamical Dijkstra demonstrated that the transportation is not sustainable, and then the pairwise comparison matrix of fuzzy AHP along with the important factors(control parameters) of the transportation systems will be automatically adjusted based on the responded results, and then begin a new round of dynamical Dijkstra simulation until the result is sustainable or clearly demonstrated that the sustainable transportation with current plans is impractical even with optimized parameters, and the plans should be changed fundamentally. To the best of our knowledge, the initial work here is the first to apply dynamical Dijkstra algorithm to working with the transportation plans based on fuzzy AHP; consequently, the test result can provide effective means for the validation of the decisions for sustainable transportation.
The main contributions of this paper include:
1) A dynamical Dijkstra simulation model is proposed to simulate the real traffic flows, and test the sustainability of the transportation systems. In the model, the cost (including physical distance, money cost, time cost, mental comfortable, and etc.) of the road connections is dynamically updated according to the change of the passenger flows. Thus, our model is more similar with the real situations than the traditional static Dijkstra algorithm which just concerns static physical distances.
2) The dynamic Dijkstra model is combined with Fuzzy Analytic Hierarchy Process for the first time. The combination ensures that the consensus creation is not only based on the FAHP decision making process but also on the response from the simulated execution of the decisions by dynamical Dijkstra. Such that, the decisions of the FAHP is tested by the dynamical Dijkstra to make a response, and then decision can be fine-tuned until consensus are created among different stakeholder participants.
3) Empirical analysis of the proposed model is carried out, the results demonstrated that the our model not only considers different criteria to realize the integrated decision of traffic planning, but also continuously optimizes the planning to make traffic more sustainable
The rest of this paper is organized as follows: Section 2 introduces the overall methodology. Sections 3 and 4 respectively discusses the fuzzy AHP and Dynamic Dijkstra simulations applied to sustainable transportation. In Section 5, the proposed methodology is empirically analyzed. Finally, conclusions and future scope are presented in Section 6.
In our model, there are two directly involved groups including: regular passengers (users) and constructors (providers). The two groups' requirements must be simultaneously satisfied; otherwise the transport project definitely cannot be sustainable. For example, if a newly built road is too congestion, or charge too much tolls, then nearly no passengers will travel on the road; on the contrary, if the revenues of the road cannot afford the cost of the constructions and maintaining, then the project will be also unstainable. To simplify the discussion, we just take passengers and constructors into our model, but more roles can be incorporated to make a comprehensive discussion, such as the environment protester, non-passengers, and even the laws and competition lines.
We incorporated the fuzzy AHP as a central part of MCDA and used this approach along with dynamical Dijkstra simulations. As shown in Figure 1 these two parts constitute a response-and-control system. The overall methodology of this study is as follows: firstly, FAHP is built based on the linguistic variables of experts and obtaining the final fuzzy weight scores for all groups, and then the weight of different criteria of FAHP is obtained based on the pairwise comparison matrices. The road control parameters of transportations are estimated based on the weights. After that, the control parameters are input into a dynamical Dijkstra model, which simulated the planned transportation system, to mimic the running of the system. Finally, after the simulations, the simulated results are evaluated by a group of criteria and the FAHP matrix along with the control parameters are adjusted according to the evaluation results. The adjustable parameters include lane numbers, limited velocity, tolls and etc. After the updating, a new round of response-and-control is issued until the requirements of each group are satisfied.
This study aims to design a new decision model for sustainable transport supply project based on the response-and-control between FAHP's decisions and dynamical Dijkstra's simulations. For this, a case study in Figure 2 is chosen. For easy of discussion, a virtual city with 10 zones are used throughout this paper. In the figure, Zi represents the i-th zone of the city and the zones can be connected by three different types of lines: subway, normal road and fast road. Noted that, there can be multiple lines connecting any pair of zones or can be no lines at all. The service quality and the revenues are two important criteria to test the sustainable of the transportation project. And the criteria is affected by many factors, some of which are adjustable including high speed limit, number of lanes, road quality. In Section 4, these factors are input into a dynamical Dijkstra model as adjustable parameters to generate a response for the updating of themselves and the FAHP's pairwise comparison matrices.
Fuzzy AHP (FAHP) is a combination of the AHP method with the Fuzzy concept approach. It is a synthetic model that is centered on fuzzy logic and introduce the multi-criteria decision making (MCDM) method to assess the quality of the alternatives. On the one hand, analytic hierarchy process (AHP) is widely used in MCDM as it can solve the unstructured problems and sort out the stakeholders' preferences into structured hierarchies [30]. On the other hand, the possibility of uncertainty between factors can be evaluated using fuzzy logic, which deals with uncertainty and imprecise problems more sophisticatedly. Integrating fuzzy theory with AHP provides a synthetic method to eliminate the ambiguity between the border of two criteria and the inconsistency of linguistic and numerable criteria and support decision-makers to give interval judgements [31]. Currently, most of the geographic phenomena tend to be described by vague terms, and the fuzzy theory is widely integrated with different MCDA for solving the spatial problems [32].
The expert's assessment greatly influences the calculation of the Analytic Hierarchy Process (AHP) method to obtain a priority scale. The assessments are the primary source to weigh the criteria based on their importance regarding the spatial problem [21,25]. Originally, the assessments are qualitatively expressed by imprecise linguistic variables. In the paper, Fuzzy-AHP is used to overcome the uncertainty of linguistic data involved in determining preferences for the assessment of transportation criteria. As shown in Table 1, a fuzzy set comprised triangular fuzzy numbers is designed to quantify the linguistic assessment using respective membership function shown in Figure 3.
Linguistic variables | Triangular fuzzy numbers | Reciprocal triangular fuzzy numbers |
Extremely strong | (9, 9, 9) | (1/9, 1/9, 1/9) |
Very strong | (6, 7, 8) | (1/8, 1/7, 1/6) |
Strong | (4, 5, 6) | (1/6, 1/5, 1/4) |
Moderately strong | (2, 3, 4) | (1/4, 1/3, 1/2) |
Equally strong | (1, 1, 1) | (1, 1, 1) |
Intermediates | (7, 8, 9), (5, 6, 7) | (1/9, 1/8, 1/7), (1/7, 1/6, 1/5) |
(3, 4, 5), (1, 2, 3) | (1/5, 1/4, 1/3), (1/3, 1/2, 1) |
To get a more flexible pairwise comparison value in AHP, the triangular fuzzy number approach is used in FAHP, which firstly creates a hierarchical structure of the alternatives of transportation plans, and then determines the pairwise comparisons matrix of the stakeholders (passengers and constructors) with a triangular fuzzy number scale. As shown in Figure 4, the hierarchical structural model has been integrated and employed for elaborating the public transport situation.
After that, the pairwise comparison matrices of the AHP are reproduced by the fuzzy numbers in Table 1 to deal with the vague linguistic terms of experts. Accordingly, the experts' assessments and pairwise comparisons with regard to the criteria in the hierarchical tree in Figure 4 are expressed by triangular fuzzy numbers. Take the target 'Sustainable Transportation' in level 1 as an example, there are three sub-criteria: 'Time cost', 'Construction Cost' and 'Oil Cost', the experts compare the relative importance of them with regard to the target. Compared with 'Construction Cost', if the 'Time Cost' is supposed to be very important, and it is as same import as 'Passenger Cost', the first line of the comparison matrix will be [(111), (6, 7, 8), (1, 1, 1)], according to the symmetrical characteristic of matrix, the second and third lines of the matrix are [(1/8, 1/7, 1/6), (111), (1/8, 1/7, 1/6)], [(111), (6, 7, 8), (111)]. In the same way, we can get the pairwise comparison matrices of each level with the fuzzy numbers as defined in Eq (1).
A=[(1,1,1)(l12,m12,u12)…(l1n,m1n,u1n)…(1,1,1)…(l2n,m2n,u12n)…(ln1,mn1,un1)…(ln2,mn2,un2)………(1,1,1)] | (1) |
where the element in the matrix satisfy the inverse rule that if aij = (lij, mij, uij), then aij-1 = (1/lij, 1/mij, 1/ uij) for i, j = 1, 2…n and i ≠ j, lij, mij and uij are the first to third components in the fuzzy number aij.
After all elements are defined by the triangular fuzzy numbers, the initial weight of the three criteria 'Time cost', 'Construction Cost' and 'Oil Cost' are calculated by the following Eq (2).
Si=∑nj=1aij⊗[∑ni=1∑nj=1aij]−1 | (2) |
where n is the number of criteria and ⊗ is extended operations of two fuzzy triangular numbers: (l1, m1, u1)⊗ (l2, m2, u2) = (l1 l2, m1 m2, u1 u2). aij = (lij, mij, uij), is the triangular fuzzy numbers in the pairwise matrices. The summations in Eq (2) are calculated by Eqs (3) and (4).
∑nj=1aij=(∑nj=1lij,∑nj=1mij,∑nj=1uij) | (3) |
∑ni=1∑nj=1aij=∑ni=1(∑nj=1lij,∑nj=1mij,∑nj=1uij) | (4) |
Let S1 = (l1, m1, u1), S2 = (l2, m2, u2), S3 = (l3, m3, u3) represent the initial fuzzy weight of the three criteria, to get the final weight of the criteria by defuzzification, then the 'degree of possibility' Si in comparison with Sj was defined as in Eq (5).
P(S1≥S2)={1l2−u1(m1−u1)−(m2−l2)0m1≥m2m1≤m2,u1≥l2otherwise | (5) |
Then the probability that a triangular number Si is bigger than the other fuzzy number S1, S2, …, Sn is defined in Eq (6).
P(Si≥S1,S2,…,Sn)=MinP(Si≥Sj),j=1,2,…,n | (6) |
Equation (7) is utilized to calculate the final weight of criteria in the paired comparative matrices,
W(Si)=MinP(Si≥Sj),j=1,2…,n,andi≠j | (7) |
Accordingly, the normalized vector is defined in following
W0=(W(S1),W(S2),…,W(Sn))i=1,2,…,n | (8) |
And the final weight vector of the criteria in level 2 is calculated through the normalization of Eq (8), as defined in Eq (9)
W=(W(Si)/Wsum,W(S2)/Wsum…,W(Sn)/Wsum)i=1,2,…,n | (9) |
where Wsum = W (S1) + W (S2) +… W (Sn). In the same way, we can calculate the weight of criteria in level 3 with regard to each criteria in the higher level.
Usually, the matrix is tested to be consistent through an extended CR test using interval pairwise comparison matrices. However, even the matrix is consistent and reliable based on the consensus of experts. We cannot afford to ignore the trues that the transport is sustainable only if the construction is economical and can satisfy the passengers' requirements, which permanently changes as time goes by. For example, the number of passengers gradually increases or decreases along with the population size in a city. Therefore, even the matrix is consistent and the weight in Eq (9) is suitable for current situation, but in the near future, the transportation system construction based on the weighting results may be inconsistent with the social economic and populations.
The consensus among the stakeholders in different interest groups (users and constructors) is much important for the sustainability of transport systems. However, even consensus is reached between stakeholders at the current time, how it would be possible to obtain more knowledge on the violations or considerations of different interests of the involved groups in the future. Sustainability aspect requires this knowledge in order to avoid the opposition of one involved group or another [21]. A variety of methods have been successfully integrated with knowledge-based models for optimization of the model for transportation improvement projects. However, all the decision methods give the final decision based on current input of factors, which may be totally different as the social context changed and the transportation system built based on the decisions is outdated and unsustainable.
In this section, we proposed to combine the FAHP with dynamic the Dijkstra simulation for supporting consensual decision making concerning sustainability in urban transport development. To ensure the Dijkstra simulations can dynamically reflect the passengers' travel situations, we periodically recount the number of passengers and update the distance matrix of Dijkstra according to the newest traffic situations, and finally the time cost, transportation expenses, and revenues are periodically counted to judge the sustainability of the transportation system. It should be noted that the distance is not only evaluated based on the original physical distances, but also based on the time cost, tolls, road quality to reflect the passengers routing selection preference.
First of all, we give the basic notations related to dynamical Dijkstra. Let G = (V, E, C) be a simple undirected graph, where V and E are the sets of vertices and edges, respectively, Let e = (u, v) e ∈ E in the graph is assigned with a cost C(u, v), and C is a function from E to the set of non-negative real numbers, it gives the cost of the corresponding edges. Each vertex v represents a city zone in the 'virtual city' in Figure 2 and it contains some auxiliary information, including the predicted shortest distance from v to a given source vertex s and the number of passengers in zone v.
Obviously, the changes of some factors affect the sustainability of transportations, including user number, charge, time cost and etc. The changes increase the uncertainty around the decisions influencing the shift towards sustainable transport due to financial, social, and technical uncertainties. Therefore, taken these variable factors into consideration and further, to examine the plans from the development decision point of view, the decision results from FAHP may be improper for the situations in the further.
As defined in Eq (10), the dynamical updating of the cost matrix Cos is realized by recounting the number of traveler nij between zone i and j, the velocity vij between the zones, and the line quality QoSij.
Cosij=ϖ1⋅Lijvij+ϖ2⋅Lij⋅Ucost+ϖ3⋅LijUcomf | (10) |
where Lij is the original physical distance between zonei and zonej, Ucost and Ucomf represent the cost and mental comfortable of the passengers per kilometer, which can be estimated according the experience, and ϖi is the corresponding weight, vij is the velocity between zonei and zonej, which is estimated by Eq (11)
vij=HsL⋅e−(nij/Nij)2⋅QoSij | (11) |
where HsL is the high speed limit, QoSij∈(0, 10] is the level of construction quality, nij is the number of passengers between zonei and zonej, Nij is the maximum allowed number of passengers on the line connected zonei and zonej, and it is estimated by Eq (12)
Nij=LijIoV⋅Cov | (12) |
where IoV is the interval between vehicles, Cov is the capacity of each vehicle.
After that, the shortest path for each passenger's routing is selected, and accordingly nij is updating, i, j ∈{1, 2, …, m}, m is the number of zones and then begin a new round of simulations.
The proposed algorithm is a modification of the standard Dijkstra's algorithm, such that the distances in the graph are changeable and any distance variation can immediately affect the following passengers' nearest path selection. The algorithm accommodates the distance change and the number of passengers in each zone to find the shortest path for each passenger.
Dynamic Dijkstra Simulations: DyDijstra |
Input: n: the number of passengers between each couple of zones; s: the start zone; e: the target zone |
Step 1: read the following parameters from a composition file L original physical distance matrix QoS: line quality level matrix Ucost: passenger's cost per kilometer m: number of zones Ucomf: the comfort level per kilometer Step 2: Initial set vis(i) = 0, i = 1, 2…m, i ≠ s, vis(s) = 1 set Disi = Lsj, midpathi = -1, i, j = 1, 2, …, m, n' = n Step 3: Update cost matrix Cos using Eqs (10)–(12), reserve vij in matrix v Step 4: Try to find zonek, such that k=argi min Disi∧vis(i)=0 If found k ∧ Disk + Coski < Disi, set Disi = Disk + Coski, midpathi = k i = 1, 2…m else return NULL, n', NULL Step5: If k! = e goes to Step4 //construct the shortest path to from s to e else PATH0 = e, i = e, j = 0 while (midpathi! = -1): PATHj = midpathi, nij++ i = midpathe, j++ PATHj = s return PATH, n, v |
For the dynamic shortest path problem, we consider that the travel cost Cos changes according to the distance and number of travelers on each edge, so does the velocity vij and time cost Lij/vij. Therefore, before the searching process of the shortest path in steps 4 and 5, the cost of each edge is firstly updated. Then, the nearest unvisited zone k to the starting point s is found, accordingly the distance matrix Dis is updated by comparisons between Disi and Disk + Coski in step 4. As we aim to found the shortest path from s to e, the visited zone on the path should be conserved, so the matrix midpath is utilized to rebuild the path in step5. It should be noted that, sometimes, we may fail to found the path in step 4, that's because the edges connecting the zones from s to e may be congested, which simulated the scenarios that there are too many passengers on the lines resulting the velocity tends to zero.
After all the simulated passengers have reached the destinations, the transportation system's sustainability is judged under a group of criteria, and then feedback the results to FAHP to update its comparison matrices as well as the control factors.
Sustainability Test |
Input: M: number of simulated passengers, α: probability of travers to a neighbor zone Threshold: λm, λt, λf, λr |
Step 1: Initial set nij = 0, i, j = 1, 2, …, m, TotalMonCost = 0, TotalTimeCost = 0, FailedNum = 0 Step 2: for i = 1 to M Step 2.1 Randomly choice s, e with probability α that s and e are neighbor zones, otherwise s and e are not directly connected Step 2.2 path, n, v = DyDijstra (n, s, e) Step 2.3 if path! = Null time cost tcost = ∑Lijvij, mcost = ∑Lij⋅FpK, Lij∈path else FailedNum++ Step 2.4 TotalMonCost = TotalMonCost + mcost, TotalTimeCost = TotalTimeCost + tcost Step 3: avgMoncost = TotalMonCost/M, avgTimecost = TotalTimeCost/M Step 4: Response: Step4.1 if avgMoncost > λm adjust the pairwise comparison matrix by Eq (13) Step4.2 if avgTimecost > λt adjust the pairwise comparison matrix by Eq (14) Step4.4 if TotalMonCost > λr adjust the pairwise comparison matrix by Eq (15) Step4.3 if FailedNum > λf the transportation is unsustainable. Step4.5 if avgMoncost > λm and TotalMonCost > λr the transportation is unsustainable. |
Let λm and λt represent the expected higher bound of average money and time cost of passengers per kilometer. λf and λr denote the allowed failed time of searing shortest path by dynamic Dijkstra and revenue of the transportation system per M travelers.
If avgMoncost - λm = Δm > 0, it means the money cost is too high for the travelers, then the weight of the passenger cost in the matrix of level defined by Eq (1) should be increased, accordingly the other two criteria construction cost and time cost should be decrease to keep consistence of the matrix. The change of the matrix in Eq (1) is defined in Eq (13).
{A[3][i].l3i=A[3][i].l3i+ΔA[3][i].m3i=A[3][i].m3i+ΔA[3][i].u3i=A[3][i].u3i+Δ,{A[i][3].li3=1/(A[3][i].u3i+Δ)A[i][3].mi3=1/(A[3][i].m3i+Δ)A[i][3].ui3=1/(A[3][i].l3i+Δ),i=1,2 | (13) |
where A[3][i]=(l3i,m3i,u3i) represents the fuzzy comparison important of passenger cost to the other two criteria: time cost and construction cost, and A[i][3] is the reverse comparison important
In the same way, if avgTimecost > λt or TotalMonCost < λr the matrix should be adjust by Eqs (14) and (15) respectively.
{A[1][i].l1i=A[1][i].l1i+ΔA[1][i].m1i=A[1][i].m1i+ΔA[1][i].u1i=A[1][i].u1i+Δ,{A[i][1].li1=1/(A[1][i].u1i+Δ)A[i][1].mi1=1/(A[1][i].m1i+Δ)A[i][1].ui1=1/(A[1][i].l1i+Δ), i=2,3 | (14) |
{A[2][i].l2i=A[2][i].l2i+ΔA[2][i].m2i=A[2][i].m2i+ΔA[2][i].u2i=A[2][i].u2i+Δ,{A[i][2].li2=1/(A[2][i].u2i+Δ)A[i][2].mi2=1/(A[2][i].m2i+Δ)A[i][2].ui2=1/(A[2][i].l2i+Δ), i=1,3 | (15) |
Accordingly, the matrices of level 2 for the criteria: high speed limit, number of lanes, line quality, rolls and oil cost in Figure 4 are also adjusted in the same manner.
The key control parameters affecting the transportation simulation result of the dynamical Ddijkstra should also be updated along with the adjusting of the matrices, including high speed limit, number of lanes, line quality, roll and oil cost.
Suppose:
1) The weight of time cost, construction cost and passenger cost for 'sustainable transportation' in level 1 of the FAHP in Figure 4 is ω1, ω2, ω3, respectively.
2) The weight of high speed limit, number of lanes, and line quality for time cost in level 2 of the FAHP in Figure 4 is ω11, ω21, ω31, respectively.
3) The weight of number of lanes, line quality for construction cost in level 2 of the FAHP in Figure 4 is ω12, ω22, respectively.
4) The weight of passenger cost, roll cost oil cost for passenger cost in level 2 of the FAHP in Figure 4 is ω13, ω23, respectively.
When avgMoncost-λm = Δm > 0, avgTimecost-λt = Δt > 0 or TotalMonCost-λr = Δr < 0, which means the money cost, time cost or revenues cannot satisfy the requirement of passengers or constructors, thus the transportation cannot be sustainable, thus not only the comparison matrix, but also the control parameters related to the criteria in level 2 should be updated. For example, when Δt > 0, the parameters: high speed limit P1, number of lanes P2, line quality P3 for construction cost in level 2 is adjusted as shown in Eq (16)
Pi=Pi−ωi1⋅ω1⋅Δt⋅η, i=1,2,3 | (16) |
η represents the learning rate. Similarly, when Δr < 0, the parameters: number of lanes P1, line quality P2 for construction cost in leve2 is adjusted as shown in Eq (17)
Pi=Pi+ωi2⋅ω2⋅Δr⋅η, i=1,2 | (17) |
When Δm > 0, it means the parameters: roll cost P1 and oil cost P2 affecting 'passenger cost' is adjusted as shown in Eq (18).
Pi=Pi−ωi3⋅ω3⋅Δm⋅η, i=1,2 | (18) |
In this section, the proposed algorithm is empirically analyzed. The transportation system connecting the 10 zones shown in Figure 2, and the alternative lines include: normal road, fast road and subway. The parameters affecting the transportation service quality and revenues are shown in Table 2.
Name | Initial value | Description |
Cov | 5 for a car, 2000 for a train | capacity of each vehicle |
HsL | 80 for subway, 120 for fast road, 50 for normal road | High speed limit |
Cpk | [1,100] | Cost of passengers per kilometer |
Iov | 25m for cars on normal road, 50m for cars on fast road, 500m for trains | Security Interval between neighbor vehicles |
QoS | (0, 10) | Quality of the line |
FpK | 1 for subway, 4 for normal road, 8 for fast road | Fare ware (toll) per kilometer |
Concost | 500 million for subway per kilometer, 30 million for fast road per kilometer, 10 million for normal road per kilometer |
Construction cost of the lines |
ExpectTime | 25 years | Expected duration of the revenues after constructions |
Firstly, the empirical fuzzy AHP matrices are given. ST is the matrix for level 1 'sustainable transportation', and the matrices for 'time cost', 'construction cost' and 'passenger cost', TC, CC and PC are shown in the following.
ST=[(1,1,1)(0.33,0.5,1)(1,1,1)(1,2,3)(1,1,1)(1,2,3)(1,1,1)(0.33,0.5,1)(1,1,1)], CC=[(1,1,1)(1,2,3)(0.33,0.5,1)(1,1,1)]TC=[(1,1,1)(1,1,1)(1,2,3)(1,1,1)(1,1,1)(1,2,3)(0.33,0.5,1)(0.33,0.5,1)(1,1,1)], PC=[(1,1,1)(0.33,0.5,1)(1,2,3)(1,1,1)] | (19) |
To simulate a scenario that from the beginning of a day, usually 7 o'clock, the number of passengers slowly increases among different zones. Suppose there are M∈[1000,100000] travelers uniformly distributed in the 10 zones in Figure 2, and 80% of them travel to the directly connected neighbor zones, and the others travel to the targets crossing multiple zones, which is similar to the real situation that most of the time, people working and living in a small area, and do not need to travel across the city. As the subway has more capacity of passengers, in the simulations, the number of passengers on subway is 10 times than that on the other two lines. In the first round of simulations, the traffic parameters are constant, which means the factors: lane numbers, high speed limit and toll fees are all the same during the experiment.
The passengers' average time cost, average fare toll cost and failed number to select a reachable line to the target zones are shown in Figures 5–7. From the figures we can see that, for the three different lines, the travelers' average time cost and failed number basically increases with the number of passengers, and the average money cost firstly fluctuates as the increasing of the passengers, but it increases in a whole. That's because when there are too many passengers, some lines become overwhelmed, and the new arrivers have to select longer path to their destinations, which cost more time and money. As for the average money cost of passengers on the subway, it decreased at the end of the simulations. As shown in Figure 5(c), there are too many users failed to select a reachable routing line to the target when the passenger number surpassed 80,000, and at this moment their money cost is not counted, thus the average cost become less. It can be seen that for fast road and normal road, near half of the passengers failed to reach their destinations when the number of passengers surpassed 4000. Therefore, the lines should incorporate more lanes to improve traffic capability. From the results we can see, if the control factors of traffics are not adjusted according to the real transportations, the system cannot be sustainable, since it failed to provide users fast and relatively cheap transportations. On the other hand, if the toll fees can not compensate the construction cost or maintain fees of the transportation system, it will also fail to be sustainable.
In the second round of simulations, every 10 percent of the progress, the pairwise comparison matrix of the FAHP and the control parameters are iteratively adjusted according to the simulation result of the dynamical Dijkstra. And the results are shown in Figures 8–10.
From the results we can see that, the time cost, money cost and failed time periodically decreased as a result of the updating of the control parameters. The results demonstrated the effectiveness of the combined model of FAHP and dynamical Dijkstra. By the Comparison of the failed number in Figures 5(c) to 10(c), we can see that, obviously in Figures 5(c), 6(c) and 7(c) the failed number of arriving the destinations continually increases with the passengers' number, as the pairwise comparison matrices and control parameters are fixed, and when the population increased, the original optimized transportation system becomes unsuitable for the requirements of travelers and constructors, thus it is not sustainable. On the contrary, from Figures 8–10, we can see that, the cost of passengers and the failed numbers firstly increase at the beginning of a short period, and then they all decreased after the adjustment of the FAHP matrices and parameters. However, we also noted that, although the matrices and parameters are periodically adjusted, as the continually increase of passenger number, the cost and failed times still increase on the whole, that's because the traffic capacity must match with the passenger numbers. Once the transportation burden exceeds its maximum capacity, the system will definitely failed to provide transportation service for passengers.
Furthermore, if all of the project revenue (user cost) cannot compensate the project cost, the transportation still unsustainable. As shown in Figure 11(a), the revenues of the subway are always lower than the construction cost. In this situation, the constructor as well as the maintainers has to abandon the transportation system. From the figures we can see that, the revenue of normal roads is easier to surpass the cost than the others, that's because in our simulation scenarios, its construction cost is much lower than the others. As for the subway, although the capacity is higher which means it is capable of providing transportation services for more passengers, however, it is built with enormous cost while the subway toll is the cheapest one. Furthermore, the real number of users is not far more than the other two types of lines: fast road and normal road, resulting in its revenues cannot compensate the construction cost, and failed to be sustainable for a long time.
To avoid the failures, we need to build cheap lanes, increase the burden (toll, tax, oil cost, etc.) of passengers or raise the bound of the high speed, which may not be applicable, as we also must take the passengers' affordability and security factors into considerations. Therefore, we believe that our research proposed a new way to test the transportation plans whether it is sustainable, especially suitable for the scenarios that the traveler number continuously change. Furthermore, to ensure the sustainable, we need to carefully taken all the factors, including both the current and future number of citizens, roll and time cost of passengers, revenues of constructors, environment protections and etc., into considerations to make sure both the users and constructions can get consensus for a long period.
In modern transportation plan decision, it is important to satisfy the involved groups' requirement to ensure a sustainable result. In case this criterion is violated due to the increase of population size, oil cost or other impact factors, the development and sustainable will be less than expected due to the resistance of one group or another. In this paper, we proposed a combined decision make model for the transportation project plan, on the one hand, it takes the experts' knowledge into the decision process through a fuzzy Multi-Criteria Decision-Making model FAHP, which is more suitable for the anthropological mode of human thinking directly based on the linguistic variables using fuzzy numbers. On the other hand, the decision matrices along with the transportation control parameters are put into a dynamical Dijkstra simulation model to test the plans and response to FAHP to realize iteratively adjustment of the plans. Different scenarios are designed to test the proposed model, and the results demonstrated that our model not only take different criteria into consideration to realize a fusion decision for the transportation plan, but it also continually optimize the plan to make the transportation more sustainable. To the best of our known, our model is the first one to take the variation of impacting factors into the MCDM decision process to ensure a sustainable plan for the transportation lines.
Overall, with the development of new urbanization, green, intelligent and sharing are becoming the focus and breakthrough of sustainable transportation development. In the future, more criteria of FAHP, which impacting the environment, road constructions, operations, matins will be taken into considerations, and some more sophisticated methodology can be applied to iteratively test the decision plans.
This work was supported by the National Key Research and Development Program of China (020YFB1805405, 2019QY0800), the Natural Science Foundation of China (61872255, U19A2068), Sichuan Science and Technology Program under Grant 2022YFG0171, Fundamental Research Funds for the Central Universities 2021SCU12136.
The authors declare there is no conflict of interest.
[1] |
A. A. Rassafi, M. Vaziri, Sustainable transport indicators: definition and integration, Int. J. Environ. Sci. Technol., 2 (2005), 83–96. https://doi.org/10.1007/BF03325861 doi: 10.1007/BF03325861
![]() |
[2] |
T. A. Shiau, Evaluating transport infrastructure decisions under uncertainty, Transp. Plann. Technol., 37 (2014), 525–538. http://dx.doi.org/10.1080/03081060.2014.921405 doi: 10.1080/03081060.2014.921405
![]() |
[3] | K. Zhou, X. Peng, Z. Guo, Evaluation method of coupling between urban spatial structure and public transport system, in 2019 5th International Conference on Transportation Information and Safety (ICTIS), (2019), 723–729. http://dx.doi.org/10.1109/ICTIS.2019.8883745 |
[4] |
M. Das, A. Roy, S. Maity, S. Kar, S. Sengupta, Solving fuzzy dynamic ship routing and scheduling problem through new genetic algorithm, Decis. Making: Appl. Manage. Eng., 2021. https://doi.org/10.31181/dmame181221030d doi: 10.31181/dmame181221030d
![]() |
[5] |
Ö. N. Çam, H. K. Sezen, The formulation of a linear programming model for the vehicle routing problem in order to minimize idle time, Decis. Making: Appl. Manage. Eng., 3 (2020), 22–29. https://doi.org/10.31181/dmame2003132h doi: 10.31181/dmame2003132h
![]() |
[6] | O. Aslan, A. Yazici, Conflict-free route planning for autonomous transport vehicle, in 2020 28th Signal Processing and Communications Applications Conference (SIU), (2020), 1–4. https://doi.org/10.1109/SIU49456.2020.9302504 |
[7] | O. Surnin, A. Ivaschenko, P. Sitnikov, A. Suprun, A. Stolbova, O. Golovnin, Urban public transport digital planning based on an intelligent transportation system, in 2019 25th Conference of Open Innovations Association (FRUCT), (2019), 292–298. https://doi.org/10.23919/FRUCT48121.2019.8981507 |
[8] | I. Estalayo, E. Osaba, I. Laña, J. D. Ser, Deep recurrent neural networks and optimization meta-heuristics for green urban route planning with dynamic traffic estimates, in 2019 IEEE Intelligent Transportation Systems Conference (ITSC), (2019), 1336–1342. https://doi.org/10.1109/ITSC.2019.8916957 |
[9] |
S. Duleba, S. Moslem, Sustainable Urban transport development with stakeholder participation, an AHP-Kendall model: A case study for Mersin, Sustainability, 10 (2018), 36–47. http://doi.org/10.3390/su10103647 doi: 10.3390/su10103647
![]() |
[10] |
S. Duleba, S. Moslem, Examining Pareto optimality in analytic hierarchy process on real data: An application in public transport service development, Expert Syst., 116 (2019), 21–30. https://doi.org/10.1016/j.eswa.2018.08.049 doi: 10.1016/j.eswa.2018.08.049
![]() |
[11] |
M. Balaji, S. Santhanakrishnan, S. N. Dinesh, An application of analytic hierarchy process in vehicle routing problem, Period. Polytech. Transp. Eng., 47 (2019), 196–205. http://doi.org/10.3311/PPtr.10701 doi: 10.3311/PPtr.10701
![]() |
[12] |
S. Duleba, An AHP-ISM approach for considering public preferences in a public transport development decision, Transport, 6 (2019), 1–10. http://doi.org/10.3846/transport.2019.9080 doi: 10.3846/transport.2019.9080
![]() |
[13] |
H. Upadhyay, S. Juneja, A. Juneja, G. Dhiman, S. Kautish, Evaluation of ergonomics-related disorders in online education using fuzzy AHP, Comput. Intell. Neurosci., 2021 (2021), 1–11. https://doi.org/10.1155/2021/2214971 doi: 10.1155/2021/2214971
![]() |
[14] | O. Ghorbanzadeh, B. Feizizadeh, T. Blaschke, R. Khosravi, Spatially explicit sensitivity and uncertainty analysis for the landslide risk assessment of the gas pipeline networks, in Proceedings of the 21st AGILE conference on Geo-information science, (2018), 12–15. |
[15] |
M. M. De Brito, M. Evers, A. D. S. Almoradie, Participatory flood vulnerability assessment: A multi-criteria approach, Hydrol. Earth Syst. Sci., 22 (2018), 373–390. http://doi.org/10.5194/hess-22-373-2018 doi: 10.5194/hess-22-373-2018
![]() |
[16] |
H. Jin, M. Zhang, Y. Yuan, Analytic network process-based multi-criteria decision approach and sensitivity analysis for temporary facility layout planning in construction projects, Appl. Sci., 8 (2018), 24–34. https://doi.org/10.3390/app8122434 doi: 10.3390/app8122434
![]() |
[17] |
M. Hervás-Peralta, S. Poveda-Reyes, G. D. Molero, F. E. Santarremigia, J. P. Pastor-Ferrando, Improving the performance of dry and maritime ports by increasing knowledge about the most relevant functionalities of the terminal operating system (TOS), Sustainability, 11 (2019), 1–23. https://doi.org/10.3390/su11061648 doi: 10.3390/su11061648
![]() |
[18] |
O. Ghorbanzadeh, B. Feizizadeh, T. Blaschke, Multi-criteria risk evaluation by integrating an analytical network process approach into GIS-based sensitivity and uncertainty analyses, Geomat. Nat. Hazards Risk, 9 (2018), 127–151. https://doi.org/10.1080/19475705.2017.1413012 doi: 10.1080/19475705.2017.1413012
![]() |
[19] |
S. R. Meena, O. Ghorbanzadeh, T. Blaschke, A comparative study of statistics-based landslide susceptibility models: A case study of the region affected by the Gorkha earthquake in Nepal, ISPRS Int. J. Geo-Inf., 8 (2019). https://doi.org/10.3390/ijgi8020094 doi: 10.3390/ijgi8020094
![]() |
[20] |
A. Hawbani, E. Torbosh, X. Wang, P. Sincak, L. Zhao, A. Al-Dubai, Fuzzy-based distributed protocol for vehicle-to-vehicle communication, IEEE Trans. Fuzzy Syst., 29 (2021), 612–626. https://doi.org/10.1109/TFUZZ.2019.2957254 doi: 10.1109/TFUZZ.2019.2957254
![]() |
[21] |
S. Moslem, O. Ghorbanzadeh, T. Blaschke, S. Duleba, Analysing stakeholder consensus for a sustainable transport development decision by the fuzzy AHP and interval AHP, Sustainability, 11 (2019), 1–22. http://doi.org/10.3390/su11123271 doi: 10.3390/su11123271
![]() |
[22] |
V. Mohagheghi, S. M. Mousavi, M. Aghamohagheghi, B. Vahdani, A new approach of multi-criteria analysis for the evaluation and selection of sustainable transport investment projects under uncertainty: A case study, Int. J. Comput. Intell. Syst., 10 (2017), 605–626. https://doi.org/10.2991/ijcis.2017.10.1.41 doi: 10.2991/ijcis.2017.10.1.41
![]() |
[23] |
P. Ziemba, Selection of electric vehicles for the needs of sustainable transport under conditions of uncertainty—a comparative study on fuzzy MCDA Methods, Energies, 14 (2021), 7786. https://doi.org/10.3390/en14227786 doi: 10.3390/en14227786
![]() |
[24] |
B. Buran, M. Erçek, Public transportation business model evaluation with Spherical and Intuitionistic Fuzzy AHP and sensitivity analysis, Expert Syst. Appl., 204 (2022), 117519. https://doi.org/10.1016/j.eswa.2022.117519 doi: 10.1016/j.eswa.2022.117519
![]() |
[25] |
J. Wątróbski, K. Małecki, K. Kijewska, S. Iwan, A. Karczmarczyk, R. G. Thompson, Multi-criteria analysis of electric vans for city logistics, Sustainability, 9 (2017), 1453. https://doi.org/10.3390/su9081453 doi: 10.3390/su9081453
![]() |
[26] |
M. H. Ha, Z. Yang, M. W. Heo, A new hybrid decision making framework for prioritising port performance improvement strategies, Asian J. Shipp. Logist., 33 (2017), 105–116. https://doi.org/10.1016/j.ajsl.2017.09.001 doi: 10.1016/j.ajsl.2017.09.001
![]() |
[27] |
M. Yavuz, B. Oztaysi, S. C. Onar, C. Kahraman, Multi-criteria evaluation of alternative fuel vehicles via a hierarchical hesitant fuzzy linguistic model, Expert Syst., 42 (2015), 2835–2848. https://doi.org/10.1016/j.eswa.2014.11.010 doi: 10.1016/j.eswa.2014.11.010
![]() |
[28] |
U. R. Tuzkaya, Evaluating the environmental effects of transportation modes using an integrated methodology and an application, Int. J. Environ. Sci. Tech., 6 (2009), 277–290. http://doi.org/10.1007/BF03327632 doi: 10.1007/BF03327632
![]() |
[29] |
A. Awasthi, S. SChauhan, A hybrid approach integrating Affinity Diagram, AHP and fuzzy TOPSIS for sustainable city logistics planning, Appl. Math. Modell., 36 (2012), 573–584. https://doi.org/10.1016/j.apm.2011.07.033 doi: 10.1016/j.apm.2011.07.033
![]() |
[30] |
W. Pedrycz, Analytic hierarchy process (AHP) in group decision making and its optimization with an allocation of information granularity, IEEE Trans. Fuzzy Syst., 19 (2011), 527–539. https://doi.org/10.1109/TFUZZ.2011.2116029 doi: 10.1109/TFUZZ.2011.2116029
![]() |
[31] |
T. T. Nguyen, L. Gordon-Brown, Constrained fuzzy hierarchical analysis for portfolio selection under higher moments, IEEE Trans. Fuzzy Syst., 20 (2012), 666–682. https://doi.org/10.1109/TFUZZ.2011.2181520 doi: 10.1109/TFUZZ.2011.2181520
![]() |
[32] |
K. T. T. Bui, D. T. Bui, J. Zou, C. Van Doan, I. Revhaug, A novel hybrid artificial intelligent approach based on neural fuzzy inference model and particle swarm optimization for horizontal displacement modeling of hydropower dam, Neural Comput., 29 (2018), 1495–1506. https://doi.org/10.1007/s00521-016-2666-0 doi: 10.1007/s00521-016-2666-0
![]() |
1. | Juan C. Orobio , Daniel E. Guzmán , Jackeline Murillo Hoyos, Ciro Jaramillo Molina, Toma de decisiones en la gestión sostenible del transporte: revisión bibliométrica de la literatura, 2025, 27, 2027-8284, 10.25100/iyc.v27i1.14497 |
Linguistic variables | Triangular fuzzy numbers | Reciprocal triangular fuzzy numbers |
Extremely strong | (9, 9, 9) | (1/9, 1/9, 1/9) |
Very strong | (6, 7, 8) | (1/8, 1/7, 1/6) |
Strong | (4, 5, 6) | (1/6, 1/5, 1/4) |
Moderately strong | (2, 3, 4) | (1/4, 1/3, 1/2) |
Equally strong | (1, 1, 1) | (1, 1, 1) |
Intermediates | (7, 8, 9), (5, 6, 7) | (1/9, 1/8, 1/7), (1/7, 1/6, 1/5) |
(3, 4, 5), (1, 2, 3) | (1/5, 1/4, 1/3), (1/3, 1/2, 1) |
Name | Initial value | Description |
Cov | 5 for a car, 2000 for a train | capacity of each vehicle |
HsL | 80 for subway, 120 for fast road, 50 for normal road | High speed limit |
Cpk | [1,100] | Cost of passengers per kilometer |
Iov | 25m for cars on normal road, 50m for cars on fast road, 500m for trains | Security Interval between neighbor vehicles |
QoS | (0, 10) | Quality of the line |
FpK | 1 for subway, 4 for normal road, 8 for fast road | Fare ware (toll) per kilometer |
Concost | 500 million for subway per kilometer, 30 million for fast road per kilometer, 10 million for normal road per kilometer |
Construction cost of the lines |
ExpectTime | 25 years | Expected duration of the revenues after constructions |
Linguistic variables | Triangular fuzzy numbers | Reciprocal triangular fuzzy numbers |
Extremely strong | (9, 9, 9) | (1/9, 1/9, 1/9) |
Very strong | (6, 7, 8) | (1/8, 1/7, 1/6) |
Strong | (4, 5, 6) | (1/6, 1/5, 1/4) |
Moderately strong | (2, 3, 4) | (1/4, 1/3, 1/2) |
Equally strong | (1, 1, 1) | (1, 1, 1) |
Intermediates | (7, 8, 9), (5, 6, 7) | (1/9, 1/8, 1/7), (1/7, 1/6, 1/5) |
(3, 4, 5), (1, 2, 3) | (1/5, 1/4, 1/3), (1/3, 1/2, 1) |
Name | Initial value | Description |
Cov | 5 for a car, 2000 for a train | capacity of each vehicle |
HsL | 80 for subway, 120 for fast road, 50 for normal road | High speed limit |
Cpk | [1,100] | Cost of passengers per kilometer |
Iov | 25m for cars on normal road, 50m for cars on fast road, 500m for trains | Security Interval between neighbor vehicles |
QoS | (0, 10) | Quality of the line |
FpK | 1 for subway, 4 for normal road, 8 for fast road | Fare ware (toll) per kilometer |
Concost | 500 million for subway per kilometer, 30 million for fast road per kilometer, 10 million for normal road per kilometer |
Construction cost of the lines |
ExpectTime | 25 years | Expected duration of the revenues after constructions |