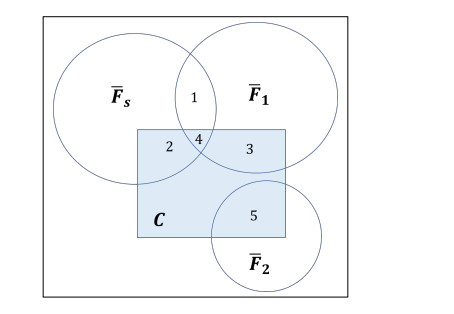
This paper presents some recent views on the aspects of vitamin D levels in relation to the COVID-19 infections and analyzes the relationship between the prevalence rates of vitamin D deficiency and COVID-19 death rates per million of various countries in Europe and Asia using the data from the PubMed database. The paper also discusses a new mathematical model of time-delay interactions between the body's immune healthy cells, infected cells, and virus particles with the effect of vitamin D levels. The model can be used to monitor the timely progression of healthy immune cells with the effects of the levels of vitamin D and probiotics supplement. It also can help to predict when the infected cells and virus particles free state can ever be reached as time progresses. The consideration of the time delay in the modeling due to effects of the infected cells or virus particles and the growth of healthy cells is also an important factor that can significantly change the outcomes of the body's immune cells as well as the infections.
Citation: Hoang Pham. Analyzing the relationship between the vitamin D deficiency and COVID-19 mortality rate and modeling the time-delay interactions between body's immune healthy cells, infected cells, and virus particles with the effect of vitamin D levels[J]. Mathematical Biosciences and Engineering, 2022, 19(9): 8975-9004. doi: 10.3934/mbe.2022417
[1] | Ming-Zhen Xin, Bin-Guo Wang, Yashi Wang . Stationary distribution and extinction of a stochastic influenza virus model with disease resistance. Mathematical Biosciences and Engineering, 2022, 19(9): 9125-9146. doi: 10.3934/mbe.2022424 |
[2] | Tingting Xue, Xiaolin Fan, Zhiguo Chang . Dynamics of a stochastic SIRS epidemic model with standard incidence and vaccination. Mathematical Biosciences and Engineering, 2022, 19(10): 10618-10636. doi: 10.3934/mbe.2022496 |
[3] | Fathalla A. Rihan, Hebatallah J. Alsakaji . Analysis of a stochastic HBV infection model with delayed immune response. Mathematical Biosciences and Engineering, 2021, 18(5): 5194-5220. doi: 10.3934/mbe.2021264 |
[4] | Kangbo Bao, Qimin Zhang, Xining Li . A model of HBV infection with intervention strategies: dynamics analysis and numerical simulations. Mathematical Biosciences and Engineering, 2019, 16(4): 2562-2586. doi: 10.3934/mbe.2019129 |
[5] | Yang Chen, Wencai Zhao . Dynamical analysis of a stochastic SIRS epidemic model with saturating contact rate. Mathematical Biosciences and Engineering, 2020, 17(5): 5925-5943. doi: 10.3934/mbe.2020316 |
[6] | Helong Liu, Xinyu Song . Stationary distribution and extinction of a stochastic HIV/AIDS model with nonlinear incidence rate. Mathematical Biosciences and Engineering, 2024, 21(1): 1650-1671. doi: 10.3934/mbe.2024072 |
[7] | H. J. Alsakaji, F. A. Rihan, K. Udhayakumar, F. El Ktaibi . Stochastic tumor-immune interaction model with external treatments and time delays: An optimal control problem. Mathematical Biosciences and Engineering, 2023, 20(11): 19270-19299. doi: 10.3934/mbe.2023852 |
[8] | Tianfang Hou, Guijie Lan, Sanling Yuan, Tonghua Zhang . Threshold dynamics of a stochastic SIHR epidemic model of COVID-19 with general population-size dependent contact rate. Mathematical Biosciences and Engineering, 2022, 19(4): 4217-4236. doi: 10.3934/mbe.2022195 |
[9] | Divine Wanduku . The stochastic extinction and stability conditions for nonlinear malaria epidemics. Mathematical Biosciences and Engineering, 2019, 16(5): 3771-3806. doi: 10.3934/mbe.2019187 |
[10] | Xiaomeng Wang, Xue Wang, Xinzhu Guan, Yun Xu, Kangwei Xu, Qiang Gao, Rong Cai, Yongli Cai . The impact of ambient air pollution on an influenza model with partial immunity and vaccination. Mathematical Biosciences and Engineering, 2023, 20(6): 10284-10303. doi: 10.3934/mbe.2023451 |
This paper presents some recent views on the aspects of vitamin D levels in relation to the COVID-19 infections and analyzes the relationship between the prevalence rates of vitamin D deficiency and COVID-19 death rates per million of various countries in Europe and Asia using the data from the PubMed database. The paper also discusses a new mathematical model of time-delay interactions between the body's immune healthy cells, infected cells, and virus particles with the effect of vitamin D levels. The model can be used to monitor the timely progression of healthy immune cells with the effects of the levels of vitamin D and probiotics supplement. It also can help to predict when the infected cells and virus particles free state can ever be reached as time progresses. The consideration of the time delay in the modeling due to effects of the infected cells or virus particles and the growth of healthy cells is also an important factor that can significantly change the outcomes of the body's immune cells as well as the infections.
Recently, classification systems have a wide range in many fields such as text classification, intrusion detection, bio-informatics, and image retrieval [1]. Unfortunately, a huge amount of data which may include irrelevant or redundant features is one of the main challenges of these systems. The negative effect of these undesirable features reduces the classification performance [2]. For this reason, reducing the number of features by finding an effective subset of features is an important task in classification systems [2]. Feature reduction has two techniques: feature selection and feature extraction [3]. Both reduce a high-dimensional dataset into a representative feature subset of low-dimensional. Feature extraction is effective when the original features fail to discriminate the classes [4], but it requires extra computation. Moreover, it changes the true meaning of the original features. In contrast, the feature selection preserves the true meaning of the selected features, which is important for some classification systems [5]. Furthermore, the result of FS is more understandable for domain experts [6].
FS tries to find the best feature subset which represents the dataset well and improves the performance of classification systems [7]. It can be classified into three approaches [6]: wrapper, embedded, and filter. According to an evaluation strategy, wrapper and embedded are called classifier-dependent approaches, while filter is called classifier-independent approach [8]. In this paper, we use the filter approach according to its advantages over wrapper or embedded approaches in terms of efficiency, simplicity, scalability, practicality, and classifier-independently [6,9]. Filter approach is a pre-processing task which finds the highly ranked features to be the input of classification systems [7,10]. There are two criteria to rank features: feature relevance, and feature redundancy [11]. Feature relevance is related to how features discriminate different classes, while feature redundancy is related to how features share the same information of each other [12]. To define these criteria, filter approach uses many weighting functions which rank features based on their significance [10] such as correlation [13], mutual information (MI) [14]. MI overcomes the weakness of correlation, whereas, correlation is suitable only for linear relationship and numerical features [1]. MI is suitable for any kind of relationship such as linear and non-linear. Moreover, MI deals with both numerical and categorical features [1].
Although MI has been widely used in many methods to find the best feature subset that maximizes the relevancy between the candidate feature and class label, and minimizes the redundancy between the candidate feature and pre-selected features [15]. The main limitations of these methods are: (1) difficult to indicate the best candidate features with the same new classification information [16], (2) difficult to deal with continuous features without information loss [17], and (3) consider the inner-class information only [18]. In this paper, we integrate fuzzy concept with mutual information to propose a new FS method called Fuzzy Joint Mutual Information Maximization (FJMIM). The fuzzy concept helps the proposed method to exploit all possible information of data where it can deal with any numerical data and extract the inner and outer-class information. Moreover, the objective function of FJMIM can overcome the feature overestimation problem which happens when the candidate feature be completely correlated with some of pre-selected features and does not depend on the majority of the subset at the same time [8].
The rest of this paper is organized as follows: Section 2 presents the basic measures of fuzzy information theory. Then, we present the proposed method in section 3. After that, the experiment design was presented in section 4, followed by the results and discussion in section 5. Finally, section 6 concludes the paper.
For the purpose of measuring the significance of features, information theory introduced many information measures such as entropy, and mutual information. To enhance these measures, fuzzy concept is used to estimate new extensions of information measures based on fuzzy equivalence relations such as fuzzy entropy, and fuzzy mutual information [19,20]. Fuzzy entropy measures the average amount of uncertainty of fuzzy relation in order to estimate its discriminative power, while fuzzy mutual information measures the shared amount of information between two fuzzy relations. In the following, we present the basic measures of fuzzy information theory:
Given a dataset D=F∪C, where F is a set of n features, and C is the class label. Let ˉF={a1,a2,…..,am} be a feature of m samples, where ˉF∈F. Let S is the feature subset with d of selected features, and the remaining set is {F−S}, where ˉFf∈F−S and ˉFs∈S. Based on the fuzzy equivalence relation RˉF on ˉF, the feature ˉF can be represented by the relation matrix M(RˉF).
M(RˉF)=(r11r12…r1mr21……r2m…………rm1rm2…rmm) | (2.1) |
where rij=RˉF(ai,aj) is the fuzzy equivalence relation between two samples ai and aj.
In this paper, the used fuzzy equivalence relation between two elements ai and aj is defined as [21]:
RˉF(ai,aj)=exp−‖ai−aj‖ | (2.2) |
Fuzzy equivalence class of sample ai on RˉF can be defined as:
[ai]RˉF=[ri1]a1+[ri2]a2+⋯+[rim]am | (2.3) |
Fuzzy entropy of feature ¯F1 based on fuzzy equivalence relation is defined as:
H(ˉF)=1mm∑i=1logm|[ai]RˉF| | (2.4) |
where |[ai]RˉF|=∑mi=1rij.
Let ¯F1 and ¯F2 be two features of F, fuzzy joint entropy of ¯F1 and ¯F2 is defined as:
H(¯F1,¯F2)=H(R¯F1,R¯F2)=1mm∑i=1logm|[ai]R¯F1∩[ai]R¯F2| | (2.5) |
Fuzzy conditional entropy of ¯F1 given ¯F2 is defined as
H(¯F1|¯F2)=H(R¯F1|R¯F2)=1mm∑i=1log|[ai]R¯F2||[ai]R¯F1∩[ai]R¯F2| | (2.6) |
Fuzzy Mutual information between two features ¯F1 and ¯F2 is defined as:
I(¯F1;¯F2)=I(R¯F1;R¯F2)=1mm∑i=1logm|[ai]R¯F1∩[ai]R¯F2||[ai]R¯F1.[ai]R¯F2| | (2.7) |
Fuzzy conditional mutual information between feature ¯F1 and ¯F2 given class C is defined as:
I(¯F1;¯F2|C)=H(¯F1|C)+H(¯F2|C)−H(¯F1,¯F2|C) | (2.8) |
Fuzzy joint mutual information between two features ¯F1, ¯F2 and class C is defined as:
I(¯F1,¯F2;C)=I(¯F1;C)+I(¯F2;C|¯F1) | (2.9) |
Fuzzy interaction information between among ¯F1, ¯F2 and C is defined as:
I(¯F1;¯F2;C)=I(¯F1;C)+I(¯F2;C)−I(¯F1,¯F2;C) | (2.10) |
In this section, we presented the general theoretical frameworks of different feature selection methods based on mutual information. Then, we studied the limitation of previous work. Finally, we introduced the proposed method.
Brown et al. [22] studied the exist feature selection methods based on MI and analyzed the different criteria to propose the following theoretical framework of these methods.
J(ˉFf)=I(ˉFf;C)−β∑ˉFs∈SI(ˉFf;ˉFs)+γ∑ˉFs∈SI(ˉFf;ˉFs|C) | (3.1) |
This framework is a linear combination of three terms: relevance, redundancy, and conditional that measures the individual predictive power of the feature, the unconditional relation, and the class-conditional relation, respectively. The criteria of different feature selection based on MI depends on the value of β and γ. MIM (β=γ=0) [23] is the simplest FS method based on MI. It considers only the relevance relation only. However, It may suffer from the redundant features. MIFS (γ=0) [24] introduced two criteria to estimate the feature relevance and redundancy. An extension of MIFS, called MIFS-U [24] is proposed to improve the redundancy term of MIFS by considering the uniform distribution of the information. However, Both MIFS and MIFS-U still require an input parameter β. To avoid this limitation, MRMR (β=1|S|, γ=0) [25] introduced the mean of the redundancy term as automatic value to the input parameter (β). JMI (β=γ=1|S|) [26] extended MRMR to extract the benefit of conditional term. In addition, Brown et al. [22] introduced also a similar non-linear framework to represent some methods as CMIM method [27]. According to [22], CMIM can be written as:
Jcmim=minˉFs∈S[I(ˉFf;C|ˉFs)]=I(ˉFf;C)−maxˉFs∈S[I(ˉFf;ˉFs)−I(ˉFf;ˉFs|C)] | (3.2) |
The reason of the non-linear relation on CMIM returns to the using of max operation. Similar to CMIM, JMIM [8] introduces a non-linear relation as follows:
Jjmim=minˉFs∈S[I(ˉFs;C)+I(ˉFf;C|ˉFs)]=I(ˉFf;C)−maxˉFs∈S[I(ˉFf;ˉFs)−I(ˉFf;ˉFs|C)−I(ˉFs;C)] | (3.3) |
Although MI has been widely used in many feature selection methods such as MIFS [24], JMI [26], mRMR [25], DISR [28], IGFS [29], NMIFS [30] and MIFS-ND [31]. These methods suffer from the overestimation of the feature significance problem [8]. For this reason, Bennasar et al. [8] proposed JMIM method to address the overestimation of the feature significance problem. However, it may fail to select the best candidate features if they have the same new classification information. To illustrate this problem, Figure 1 shows the FS scenario, where ¯F1 and ¯F2 are two candidate features, ¯Fs is the pre-selected feature subset, and C is the class label. ¯F1 is partially redundant with ¯Fs, while ¯F2 is independent to ¯Fs. Suppose that ¯F1 and ¯F2 have the same new classification information I(¯F1;C|¯Fs) = (area 3) and I(¯F2;C|¯Fs) = (area 5) respectively. In this case, JMIM may fail to indicate the best feature where I(¯F1,¯Fs;C) and I(¯F1,¯Fs;C) are equal.
Unfortunately, JMIM also shares some limitations with the previous methods. Firstly, it can not directly estimate MI between continuous features [17]. To address this limitation, there are two methods were introduced. One is to estimate MI based on Parzen window [18], but it is inefficient in high-dimensional feature spaces with spare samples [17]. Moreover, its performance depends on the used window function which requires a window width parameter [32]. The other one is to discretize continuous features before estimating MI [33], but it may cause information loss [34]. Secondly, JMIM depends only on inner-class information without considering outer-class information [18].
Motivated by the previous limitation of JMIM, we proposed a new FS method, called Fuzzy Joint Mutual Information Maximization (FJMIM). Both of FJMIM and JMIM depends on "maximum of the minimum" approach. The main difference is that JMIM maximize the joint mutual information of the candidate feature and pre-selected feature subset with class, whereas FJMIM maximizes the joint mutual information of the candidate feature and pre-selected feature subset with class without considering the class-relevant redundancy. To illustrate the difference in Figure 1, JMIM depends on the union of areas 2, 4 and 3, while FJMIM depends on the union of areas 2 and 3. The proposed method discarded the class-relevant redundancy (area 4) because it can reduce the predictive ability of the feature subset when a candidate feature is selected [15]. On the other hand, integrating fuzzy concept with MI has many benefits. Firstly, using a fuzzy concept helps to deal directly with continuous features. Furthermore, it enables MI to take the advantages of inner and outer-class information [35]. Moreover, FS methods based on fuzzy concept are more robust toward any change of the data than methods based on probability concept [36].
According to FJMIM, the candidate feature ˉFf must satisfy the following condition
ˉFf=argmaxˉFf∈F−S(minˉFs∈S(I(ˉFf,ˉFs;C)−I(ˉFf;ˉFs;C))) | (3.4) |
FJMIM also can be written according to the non-linear framework as follows:
ˉFf=2∗I(ˉFf;C)−maxˉFs∈S[I(ˉFf;ˉFs)−I(C;ˉFs)−I(ˉFf;ˉFs|C)−I(ˉFf;C|ˉFs)] | (3.5) |
The proposed method can be summarized to find the best feature subset of size d as follows:
Input: F is a set of n features, C is the class label, and d is the number of selected features.
Step 1: Initialize the empty selected feature subset S.
Step 2: Update the selected feature set S and the feature set F.
Step 2.1: Compute I(ˉF;C) for all ˉF in the feature set F.
Step 2.2: Add the feature ˉF that maximizes I(ˉF;C) to the selected feature set S.
Step 2.3: Remove the feature ˉF from the feature set F.
Step 3: Repeat until |S|=d
Step 3.1: Add the feature ˉF that satisfies
argmaxˉFf∈F−S(minˉFs∈S(I(ˉFf,ˉFs;C)−I(ˉFf;ˉFs;C)))
to the selected feature set S.
Step 3.2: Remove the feature ˉF from the feature set F.
Output: Return the selected feature set S.
Success of FS methods depends on different criteria such as classification performance, and stability [11]. Consequently, we design the experiment based on these criteria (Figure 2). To clarify our improvement, we compared our proposed method FJMIM, with four conventional methods (CMIM [27], JMI [26], QPFS [37], Relief [38]) and five state-of-the-art methods (CMIM3 [39], JMI3 [39], JMIM [8], MIGM [40], and WRFS [41]). The compared methods can be divided into two groups: FS based on fuzzy concept and FS based on probability concept. For the methods which depend on probability concept, data discretization is required as a pre-processing step prior to the FS process. So, the continuous features are transformed into ten bins using EqualWidth discretization [42]. Then, we selected feature subset from all methods based on threshold which is defined as the median position of the ranked features (or the nearest integer position when the number of ranked features is even).
FS plays an important role to improve the classification performance. There are many measures to evaluate classification performance such as classification accuracy, precision, F-measure, area-under-ROC (AUC), and area-under-PRC (AUCPR) [43]. To clarify our improvement, popular classifiers were used in this study such as Naive Bayes (NB), Support Vector Machine (SVM), and 3-Nearest Neighbors (KNN) [44]. The average classification performance measures were computed by 10-fold cross-validation approach [45].
Another important evaluation criterion for FS is stability. FS stability measures the impact of any change in the input data on FS result [46]. In this study, we measure the impact of noise on the selected feature subset. Firstly, we produce the noise using standard deviation and the normal distribution of each feature [47]. Then, we injected 10% of the data by adding noise. After that, we repeated this step ten times. Each time produces a different sequence of selected features. Finally, we computed the stability of each method using Kuncheva stability index [48].
Our experiment was conducted using 13 datasets from UCI machine learning repository [49]. Table 1 presents a brief description about these datasets.
No. | Datasets | Instances | Features | Classes |
1 | Acute Inflammations | 120 | 6 | 2 |
2 | Arrhythmia | 452 | 279 | 13 |
3 | Blogger | 100 | 6 | 2 |
4 | Diabetic Retinopathy Debrecen (DRD) | 1151 | 20 | 2 |
5 | Hayes-Roth | 160 | 5 | 3 |
6 | Indian Liver Patient Dataset (ILPD) | 583 | 10 | 2 |
7 | Lenses | 24 | 4 | 3 |
8 | Lymphography | 148 | 18 | 4 |
9 | Congressional Voting Records (CVR) | 435 | 16 | 2 |
10 | Sonar | 208 | 60 | 2 |
11 | Thoracic Surgery | 470 | 17 | 2 |
12 | Wilt | 4889 | 6 | 2 |
13 | Zoo | 101 | 17 | 7 |
1) Accuracy: A paired two-tailed t-test is employed between FJMIM and other compared methods. The notations ([=], [+], and [-]) indicate the statistically significant (5%) that the proposed method (equals, wins, and losses) other methods. According to NB classifier, FJMIM achieved the maximum average accuracy with score 78.02%, while Relief achieved the minimum average accuracy with score 75.34% (Table 2). The proposed method outperformed compared methods in the range from 0.06 to 2.68%. In SVM classifier, FJMIM outperformed other methods with score 80.03%, while Relief achieved the minimum average accuracy with score 77.33% (Table 3). The proposed method outperformed compared methods in the range from 0.69 to 2.7%. Similarly with KNN classifier, FJMIM kept the maximum average accuracy by 81.45%, while Relief achieved the minimum average accuracy with score 77.98% (Table 4). The proposed method outperformed compared methods in the range from 0.57 to 3.47%. Across all datasets, Figure 3(a) shows the distribution of the average accuracy values of all used classifiers. In a detailed box-plot, the box represents upper and lower quartiles, while the black circle represents the median. The box-plot confirms that FJMIM is more consistent and outperformed other compared methods. Figure 3(b) shows the average accuracy of the three used classifiers. FJMIM achieved the best accuracy, followed by QPFS, JMI3, both of JMI and CMIM, both of CMIM3 and JMIM, MIGM, WRFS, and Relief respectively. The proposed method outperformed compared methods in the range from 0.6 to 2.9%.
Dataset | CMIM | CMIM3 | JMI | JMI3 | JMIM | MIGM | QPFS | Relief | WRFS | FJMIM |
Acute Inflammations | 100±0[=] | 100±0[=] | 100±0[=] | 100±0[=] | 100±0[=] | 99.75±2.5[=] | 99.75±2.5[=] | 100±0[=] | 90.83±8.5[+] | 100±0 |
Arrhythmia | 60.64±7.01[=] | 59.22±6.17[=] | 59.42±6.49[=] | 59.58±6.58[=] | 60.62±6.93[=] | 58.98±6.39[=] | 63.7±6.57[-] | 64.94±6.33[-] | 59.31±6.3[=] | 59.74±6.43 |
Blogger | 61.9±10.7[=] | 61.9±10.7[=] | 61.9±10.7[=] | 64.9±10.2[=] | 61.9±10.7[=] | 61.9±10.7[=] | 61.9±10.7[=] | 65.4±7.84[=] | 66.1±9.09[=] | 65.9±10.26 |
DRD | 57.59±4.95[=] | 60.66±4.69[-] | 60.3±5.04[-] | 60.6±4.58[-] | 57.59±4.95[=] | 60.3±5.04[-] | 61.19±4.49[-] | 60.61±5.06[-] | 57.47±4.98[=] | 57.56±4.83 |
Hayes-Roth | 45.81±10.77[=] | 45.81±10.77[=] | 45.81±10.77[=] | 45.81±10.77[=] | 45.81±10.77[=] | 45.81±10.77[=] | 49.37±10.53[=] | 46.31±11.48[=] | 45.81±10.77[=] | 49.37±10.53 |
ILPD | 68.99±5.68[=] | 67.33±5.87[=] | 68.99±5.68[=] | 67.33±5.87[=] | 68.99±5.68[=] | 68.99±5.68[=] | 68.73±5.64[=] | 64.96±6.45[+] | 70.11±6.74[=] | 69.54±5.75 |
Lenses | 86.83±21.88[=] | 86.83±21.88[=] | 86.83±21.88[=] | 86.83±21.88[=] | 86.83±21.88[=] | 86.83±21.88[=] | 86.83±21.88[=] | 54.83±30.73[+] | 86.83±21.88[=] | 86.83±21.88 |
Lymphography | 79.02±10.57[=] | 82.05±9.59[=] | 79.02±10.57[=] | 77.93±10.87[=] | 78.27±10.98[=] | 77.25±11.38[=] | 79.02±10.57[=] | 82.61±9.77[=] | 76.91±11.64[+] | 81.5±10.29 |
CVR | 94.3±3.15[=] | 94.32±3.12[=] | 93.75±3.57[=] | 93.75±3.57[=] | 93.75±3.57[=] | 93.75±3.57[=] | 94.32±3.26[=] | 93.82±3.28[=] | 93.52±3.18[=] | 93.75±3.57 |
Sonar | 75.05±8.33[=] | 75.42±9.08[=] | 75.75±8.85[=] | 76.23±9.99[=] | 72.2±9.93[=] | 72.14±9.54[+] | 76.42±8.69[=] | 74.25±8.33[=] | 77.91±8.74[=] | 77±8.98 |
Thoracic Surgery | 83.83±3.1[=] | 83.38±3.09[=] | 83.38±3.09[=] | 83.85±2.24[=] | 83.38±3.09[=] | 84.64±1.83[=] | 83.55±3.08[=] | 83.06±2.77[=] | 83.85±2.74[=] | 83.38±3.09 |
Wilt | 94.61±0.06[=] | 94.61±0.06[=] | 94.61±0.06[=] | 94.61±0.06[=] | 94.61±0.06[=] | 94.61±0.06[=] | 94.61±0.06[=] | 94.61±0.06[=] | 94.61±0.06[=] | 94.61±0.06 |
Zoo | 95.15±5.74[=] | 96.05±5.6[=] | 96.03±5.66[=] | 96.03±5.66[=] | 96.03±5.66[=] | 96.93±5.42[=] | 94.08±6.6[=] | 94.08±6.6[=] | 92.96±7.05[=] | 95.05±5.87 |
Average | 77.21 | 77.51 | 77.37 | 77.50 | 76.92 | 77.07 | 77.96 | 75.34 | 76.63 | 78.02 |
Dataset | CMIM | CMIM3 | JMI | JMI3 | JMIM | MIGM | QPFS | Relief | WRFS | FJMIM |
Acute Inflammations | 98.33±3.35[=] | 99.67±1.64[=] | 98.33±3.35[=] | 98.33±3.35[=] | 98.33±3.35[=] | 99.42±2.44[=] | 99.42±2.44[=] | 100±0[+] | 89.92±9.5[+] | 99.42±2.44 |
Arrhythmia | 66.09±6.81[=] | 64.08±6.11[=] | 65.01±5.96[=] | 65.7±6.23[=] | 66.14±6.61[=] | 65.23±6.39[=] | 66.82±6.2[=] | 67.22±6.05[=] | 65.23±6.15[=] | 67.11±6.7 |
Blogger | 68±4.02[=] | 67.7±4.89[=] | 68±4.02[=] | 67.4±5.62[=] | 68±4.02[=] | 68±4.02[=] | 67.7±4.89[=] | 67.7±4.89[=] | 68±4.02[=] | 67.7±4.89 |
DRD | 68.03±3.92[=] | 67.55±4.07[=] | 67.15±3.85[=] | 67.03±3.95[=] | 68.03±3.92[=] | 67.19±3.8[=] | 65.1±4.33[+] | 61.91±4.51[+] | 67.7±4[=] | 67.96±4.23 |
Hayes-Roth | 49.94±12.83[=] | 49.94±12.83[=] | 49.94±12.83[=] | 49.94±12.83[=] | 49.94±12.83[=] | 49.94±12.83[=] | 52±11.61[=] | 48.75±13.21[=] | 49.94±12.83[=] | 52±11.61 |
ILPD | 71.36±0.73[=] | 71.36±0.73[=] | 71.36±0.73[=] | 71.36±0.73[=] | 71.36±0.73[=] | 71.36±0.73[=] | 71.36±0.73[=] | 71.36±0.73[=] | 71.36±0.73[=] | 71.36±0.73 |
Lenses | 76.17±22.13[=] | 76.17±22.13[=] | 76.17±22.13[=] | 76.17±22.13[=] | 76.17±22.13[=] | 76.17±22.13[=] | 76.17±22.13[=] | 54.5±30.42[+] | 76.17±22.13[=] | 76.17±22.13 |
Lymphography | 81.57±10.06[=] | 80.56±9.09[=] | 81.42±10.15[=] | 84.55±8.84[=] | 82.02±10.48[=] | 79.06±10.44[+] | 81.57±10.11[=] | 80.3±9[=] | 74.25±11.45[+] | 85.5±8.3 |
CVR | 94.09±3.33[=] | 94.46±3.37[=] | 94.3±3.34[=] | 94.32±3.32[=] | 94.3±3.34[=] | 94.3±3.34[=] | 94±3.44[=] | 94.43±3.41[=] | 94.37±3.41[=] | 94.3±3.34 |
Sonar | 79.34±8.37[=] | 80.48±8.05[=] | 80.18±8.33[=] | 78.46±8.44[=] | 80.54±8.43[=] | 76.93±8.71[=] | 79.91±7.73[=] | 82.1±8.07[=] | 77.41±8.41[=] | 80.67±7.88 |
Thoracic Surgery | 85.11±0[=] | 85.11±0[=] | 85.11±0[=] | 85.11±0[=] | 85.11±0[=] | 85.11±0[=] | 85.11±0[=] | 85.11±0[=] | 85.11±0[=] | 85.11±0 |
Wilt | 97.62±0.57[+] | 97.97±0.49[=] | 97.97±0.49[=] | 97.97±0.49[=] | 97.62±0.57[+] | 97.97±0.49[=] | 94.61±0.06[+] | 97.97±0.49[=] | 97.62±0.57[+] | 97.98±0.49 |
Zoo | 95.75±5.12[=] | 93.88±6.72[=] | 89.39±5.72[+] | 89.49±5.64[+] | 89.49±5.64[+] | 94.06±6.9[=] | 95.27±5.85[=] | 93.89±6.96[=] | 90.49±7.96[+] | 95.06±6.2 |
Average | 79.34 | 79.15 | 78.79 | 78.91 | 79.00 | 78.83 | 79.16 | 77.33 | 77.51 | 80.03 |
Dataset | CMIM | CMIM3 | JMI | JMI3 | JMIM | MIGM | QPFS | Relief | WRFS | FJMIM |
Acute Inflammations | 99.5±1.99[=] | 100±0[=] | 99.5±1.99[=] | 99.5±1.99[=] | 99.5±1.99[=] | 100±0[=] | 100±0[=] | 100±0[=] | 99.67±1.64[=] | 100±0 |
Arrhythmia | 58.27±5.17[+] | 57.63±5.17[+] | 57.79±5.34[+] | 58.32±5.08[+] | 58.94±5.13[=] | 57.67±5.21[+] | 60.73±4.64[=] | 65.13±4.43[-] | 58.39±5.22[+] | 61.11±5.23 |
Blogger | 72.1±11.31[=] | 72.1±11.31[=] | 72.1±11.31[=] | 78.8±10.18[=] | 72.1±11.31[=] | 72.1±11.31[=] | 72.1±11.31[=] | 70.3±11.41[=] | 76.1±12.05[=] | 78.6±11.37 |
DRD | 64.3±4.19[=] | 62.62±5.29[=] | 63.69±4.58[=] | 61.96±4.68[=] | 64.3±4.19[=] | 63.58±4.56[=] | 60.47±4.64[+] | 63.51±4.63[=] | 64.06±4.4[=] | 64.7±4.23 |
Hayes-Roth | 59.19±10.41[=] | 59.19±10.41[=] | 59.19±10.41[=] | 59.19±10.41[=] | 59.19±10.41[=] | 59.19±10.41[=] | 63.12±11.32[=] | 57.56±10.82[=] | 59.19±10.41[=] | 63.12±11.32 |
ILPD | 67.16±5.41[=] | 68.22±5.06[=] | 67.54±5.44[=] | 68.22±5.06[=] | 67.16±5.41[=] | 67.3±5.42[=] | 68.74±5.01[=] | 67.84±5.56[=] | 66.66±5.25[=] | 69.15±5.62 |
Lenses | 86.83±21.88[=] | 86.83±21.88[=] | 86.83±21.88[=] | 86.83±21.88[=] | 86.83±21.88[=] | 86.83±21.88[=] | 86.83±21.88[=] | 54.17±30.46[+] | 86.83±21.88[=] | 86.83±21.88 |
Lymphography | 84.61±7.58[=] | 72.61±9.14[+] | 84.61±7.58[=] | 84.16±9.46[=] | 80.99±9.49[=] | 76.62±8.87[=] | 84.61±7.58[=] | 81.44±9.42[=] | 79.74±9.36[=] | 78.87±8.35 |
CVR | 93.63±3.43[=] | 94.09±3.33[=] | 95.26±3.01[=] | 95.26±3.01[=] | 95.26±3.01[=] | 95.26±3.01[=] | 94.14±3.37[=] | 93.67±3.5[=] | 94.09±2.95[=] | 95.26±3.01 |
Sonar | 85.09±7.43[=] | 83.51±7.68[=] | 87.74±7.51[=] | 85.53±8.83[=] | 82.7±9.06[=] | 82.4±7.72[+] | 86.15±7.24[=] | 87.95±6.78[=] | 84.17±7.73[=] | 87.65±7.27 |
Thoracic Surgery | 80.96±3.45[=] | 81.38±3.53[=] | 81.38±3.53[=] | 83.45±3.05[=] | 81.38±3.53[=] | 82.64±3.28[=] | 83.89±2.95[=] | 82.68±3.08[=] | 84.85±0.97[-] | 81.38±3.53 |
Wilt | 97.69±0.57[+] | 98.12±0.55[=] | 98.12±0.55[=] | 98.12±0.55[=] | 97.69±0.57[+] | 98.12±0.55[=] | 94.44±0.52[+] | 98.12±0.55[=] | 97.69±0.57[+] | 98.12±0.55 |
Zoo | 91.9±7.21[=] | 92.06±7.35[=] | 92.05±7.24[=] | 92.05±7.24[=] | 92.05±7.24[=] | 91.16±7.48[=] | 91.42±6.94[=] | 91.42±6.94[=] | 90.29±7.45[+] | 94.08±6.6 |
Average | 80.09 | 79.10 | 80.45 | 80.88 | 79.85 | 79.45 | 80.51 | 77.98 | 80.13 | 81.45 |
2) Precision: Figure 4 shows the precision results of NB, SVM, KNN, and their average. FJMIM achieved the highest precision, while Relief achieved the lowest precision. The proposed method outperformed other methods in the range from 0.6 to 12.7% based on NB, from 0.3 to 3.3% based on SVM, and from 2 to 8.2% based on KNN. According to the average of all classifiers, FJMIM achieved the highest precision by 71.2%, while Relief kept the lowest precision by 63.1%. The second-best method achieved by CMIM3, followed by JMI3, QPFS, JMI, JMIM, MIGM, CMIM and WRFS. The results of precision distribution are inconsistent as shown in Figure 5. Both FJMIM and QPFS achieved the best distribution. FJMIM achieved the highest upper quartile and median, while QPFS achieved the highest lower quartile and median. In addition, FJMIM shares the highest upper quartile with JMI, JMIM and MIGM.
3) F-measure: Figure 6 shows the F-measure results of the three used classifiers and their average. FJMIM achieved the highest F-measure by 79.8, 71.5 and 84% on NB, SVM and KNN respectively. Relief achieved the lowest F-measure on NB and SVM, while WRFS achieved the lowest score on SVM. The proposed method outperformed other methods in the range from 0.3 to 16.6% based on NB, from 0.1 to 1.4% based on SVM, and from 0.6 to 15.2% based on KNN. According to the average of all classifiers, FJMIM achieved the highest precision by 78.4% and outperformed other methods in the range from 2.5 to 10.8%. The second-best method achieved by JMI3, followed by QPFS, CMIM3, JMI, JMIM, CMIM, MIGM, WRFS and Relief. Figure 7 shows the distribution of F-measure across all datasets. The box-plot confirms the outperformance of FJMIM compared to other methods.
4) AUC: Figure 8 shows the AUC results of the used classifiers and their average. FJMIM achieved the highest AUC on NB and KNN by 83.9 and 85.2%, while it achieved the second-best score on SVM by 74.8%. On the other hand, Relief achieved the lowest AUC on all classifiers. Although MIGM achieved the best AUC on SVM, FJMIM outperformed on the average of all classifiers. The proposed method outperformed other methods in the range from 0.4 to 4.2%. As shown in the box-plot (Figure 9), the proposed method achieved the highest lower quartile and median values. On the other hand, JMI achieved also the highest lower quartile, while CMIM achieved the highest upper quartile.
5) AUCPR: The highest AUCPR was achieved by both FJMIM and CMIM3 using NB, MIGM using SVM, and FJMIM using KNN (Figure 10). On the other hand, Relief achieved the lowest AUCPR using all classifiers. According to the average of all classifiers, FJMIM achieved the best AUCPR by 81.6%, while Relief kept the lowest AUCPR by 77.2%. The proposed method outperformed other methods in the range from 0.3 to 4.4%. The second-best AUCPR was achieved by CMIM3, followed by MIGM, both QPFS and JMIM, both CMIM and JMI, and both JMI3 and WRFS. Figure 11 shows the distribution of AUCPR across all datasets. It's obvious that CMIM achieved the highest median, lower, and upper quartiles, while FJMIM achieved the highest median, upper quartile.
Figure 12(a) shows the stability of the used FS methods on all datasets. It's obvious that FJMIM is more consistent and stable compared to all other methods. Figure 12(b) confirms the stability of the compared method. FJMIM achieved the highest average stability by 87.8%. The proposed method outperformed other methods in the range from 6.6 to 43%. JMI achieved the second-best position by 81.2%, while Relief achieved the lowest stability by 44.3%.
More detailed results are presented in the appendix section. According to previous results, It is obvious that FJMIM achieves the best results in most measures. This is expected because our proposed method addresses the feature overestimation problem and handle the candidate feature problem well. Moreover, it avoids the discretization step. Another reason is the advantages of both inner and outer class information which FJMIM depends on it. This information helps the proposed method to be more robust toward the noise. On the other hand, the other compared methods are close to FJMIM than Relief that achieved the lowest result. This is because all compared method except Relief depends on mutual information as the proposed method to estimate the significant of features.
In this paper, we propose a new FS method, called, Fuzzy Joint Mutual Information Maximization (FJMIM). The proposed method depends on integrating an improved JMIM objective function with fuzzy concept. The benefits of our proposed method include: 1) The ability to deal directly with discrete and continuous features. 2) The suitability to handle any kind of relations between features such as linear and non-linear relation. 3) The ability to take the advantages of inner and outer class information. 4) The robustness toward the noise. 5) The ability to select the most significant feature subset and avoiding the undesirable features.
To confirm the effectiveness of FJMIM, 13 benchmark datasets have been used to evaluate the proposed method in the term of classification performance (accuracy, precision, F-measure, AUC, and AUCPR) and feature selection stability. According to nine conventional and state-of-the-art feature selection methods, the proposed method achieved promising improvement on feature selection in the terms of classification performance and stability.
In future work, we plan to extend the proposed method to cover multi-label classification problem. Moreover, we plan to study the effectiveness of imbalanced data on the proposed method.
This research has been supported by the National Natural Science Foundation (61572368).
The authors declare no conflict of interest.
Tables A1–A6 show some numerical results of Figures 3(b), 4, 6, 8, 10 and 12(b), while Figures A1–A4 show the statistical results (mean ± standard deviation) of some datasets (DRD, Sonar, Wilt and Zoo) by precision, F-measure, AUC and AUCPR, respectively.
FS method | Average |
CMIM | 78.9 |
CMIM3 | 78.6 |
JMI | 78.9 |
JMI3 | 79.1 |
JMIM | 78.6 |
MIGM | 78.4 |
QPFS | 79.2 |
Relief | 76.9 |
WRFS | 78.1 |
FJMIM | 79.8 |
FS method | NB | SVM | KNN | Average |
CMIM | 0.702 | 0.641 | 0.704 | 0.682 |
CMIM3 | 0.742 | 0.641 | 0.705 | 0.696 |
JMI | 0.705 | 0.640 | 0.713 | 0.686 |
JMI3 | 0.712 | 0.636 | 0.723 | 0.690 |
JMIM | 0.701 | 0.642 | 0.707 | 0.683 |
MIGM | 0.701 | 0.637 | 0.708 | 0.682 |
QPFS | 0.712 | 0.636 | 0.711 | 0.686 |
Relief | 0.621 | 0.612 | 0.661 | 0.631 |
WRFS | 0.698 | 0.632 | 0.708 | 0.679 |
FJMIM | 0.748 | 0.645 | 0.743 | 0.712 |
FS method | NB | SVM | KNN | Average |
CMIM | 0.722 | 0.712 | 0.818 | 0.750 |
CMIM3 | 0.795 | 0.712 | 0.752 | 0.753 |
JMI | 0.724 | 0.712 | 0.823 | 0.753 |
JMI3 | 0.729 | 0.712 | 0.834 | 0.759 |
JMIM | 0.719 | 0.714 | 0.821 | 0.751 |
MIGM | 0.722 | 0.710 | 0.818 | 0.750 |
QPFS | 0.732 | 0.712 | 0.825 | 0.756 |
Relief | 0.632 | 0.708 | 0.688 | 0.676 |
WRFS | 0.716 | 0.701 | 0.826 | 0.748 |
FJMIM | 0.798 | 0.715 | 0.840 | 0.784 |
FS method | NB | SVM | KNN | Average |
CMIM | 0.835 | 0.733 | 0.812 | 0.793 |
CMIM3 | 0.836 | 0.748 | 0.844 | 0.809 |
JMI | 0.837 | 0.734 | 0.815 | 0.795 |
JMI3 | 0.831 | 0.729 | 0.805 | 0.788 |
JMIM | 0.836 | 0.742 | 0.812 | 0.796 |
MIGM | 0.832 | 0.754 | 0.807 | 0.798 |
QPFS | 0.838 | 0.710 | 0.807 | 0.785 |
Relief | 0.804 | 0.707 | 0.801 | 0.771 |
WRFS | 0.831 | 0.732 | 0.806 | 0.789 |
FJMIM | 0.839 | 0.748 | 0.852 | 0.813 |
FS method | NB | SVM | KNN | Average |
CMIM | 0.848 | 0.708 | 0.798 | 0.785 |
CMIM3 | 0.862 | 0.715 | 0.862 | 0.813 |
JMI | 0.850 | 0.708 | 0.797 | 0.785 |
JMI3 | 0.837 | 0.705 | 0.800 | 0.781 |
JMIM | 0.852 | 0.713 | 0.797 | 0.787 |
MIGM | 0.857 | 0.724 | 0.827 | 0.803 |
QPFS | 0.857 | 0.708 | 0.797 | 0.787 |
Relief | 0.842 | 0.685 | 0.791 | 0.772 |
WRFS | 0.850 | 0.697 | 0.795 | 0.781 |
FJMIM | 0.862 | 0.718 | 0.869 | 0.816 |
FS method | Stability |
CMIM | 72.6 |
CMIM3 | 67.3 |
JMI | 81.2 |
JMI3 | 75.2 |
JMIM | 75.9 |
MIGM | 66.2 |
QPFS | 71.7 |
Relief | 44.3 |
WRFS | 66.6 |
FJMIM | 87.8 |
[1] | Available online: https://patch.com/new-jersey/oceancity/nj-coronavirus-update-gov-murphy-considers-curfew-31-new-cases (accessed on16 March 2020). |
[2] | Available online: https://www.osha.gov/SLTC/covid-19/medicalinformation.html (accessed on.17 March 2020). |
[3] | Centers for Disease Control and Prevention, https://www.cdc.gov/coronavirus/2019-ncov/prevent-getting-sick/social-distancing.html (accessed on 8 April 2020) |
[4] |
H. Pham, On estimating the number of deaths related to Covid-19, Mathematics, 8 (2020), 655. https://doi.org/10.3390/math8050655 doi: 10.3390/math8050655
![]() |
[5] | Worldometers, 2022 (accessed on April 1, 2022). |
[6] | https://www.worldometers.info/coronavirus/?utm_campaign = homeAdvegas1?#countries |
[7] | World Health Organization, https://covid19.who.int/ (accessed on April 1, 2022). |
[8] |
H. Pham, Estimating the COVID-19 death toll by considering the time-dependent effects of various pandemic restrictions, Mathematics, 8 (2020), 1628. https://doi.org/10.3390/math8091628 doi: 10.3390/math8091628
![]() |
[9] |
A. W. H. Chin, J. T. S. Chu, M. R. A. Perera, K. P. Y. Hui, H. L. Yen, M. C. W. Chan, et al., Stability of SARS-CoV-2 in different environmental conditions, Lancet Microbe, 1 (2020), 1. https://doi.org/10.1016/S2666-5247(20)30003-3 doi: 10.1016/S2666-5247(20)30003-3
![]() |
[10] |
E. Dong, H. Du, L. Gardner, An interactive web-based dashboard to track COVID-19 in real time, Lancet Infect. Dis. 20 (2020), 533-534. https://doi.org/10.1016/S1473-3099(20)30120-1 doi: 10.1016/S1473-3099(20)30120-1
![]() |
[11] |
K. Prem, Y. Liu, T. W. Russell, A. J. Kucharski, R. M. Eggo, N. Davies, et al., The effect of control strategies to reduce social mixing on outcomes of the COVID-19 epidemic in Wuhan, China: A modelling study, Lancet Public Health, 20 (2020), e261-e270. https://doi.org/10.1016/S2468-2667(20)30073-6 doi: 10.1016/S2468-2667(20)30073-6
![]() |
[12] |
G. Sebastiani, M. Massa, E. Riboli, Covid-19 epidemic in Italy: Evolution, projections and impact of government measures, Eur. J. Epidemiol., 35 (2020), 341-345. https://doi.org/10.1007/s10654-020-00631-6 doi: 10.1007/s10654-020-00631-6
![]() |
[13] |
G. Onder, G. Rezza, S. Brusaferro, Case-Fatality rate and characteristics of patients dying in relation to COVID-19 in Italy, JAMA, 20 (2020), 1775-1776. https://doi.org/10.1001/jama.2020.4683 doi: 10.1001/jama.2020.4683
![]() |
[14] |
D. D. Rajgor, M. H. Lee, S. Archuleta, N. Bagdasarian, S. C. Quek, The many estimates of the COVID-19 case fatality rate, Lancet Infect. Dis., 20 (2020), 776-777. https://doi.org/10.1016/S1473-3099(20)30244-9 doi: 10.1016/S1473-3099(20)30244-9
![]() |
[15] |
A. J. Kucharski, T. W. Russell, C. Diamond, Y. Liu, J. Edmunds, S. Funk, et al., Early dynamics of transmission and control of COVID-19: A mathematical modelling study, Lancet Infect. Dis., 20 (2020), 553-558. https://doi.org/10.1016/S1473-3099(20)30144-4 doi: 10.1016/S1473-3099(20)30144-4
![]() |
[16] |
X. Wang, Y. Zhang, F. Fang, Role of vitamin D in COVID-19 infections and deaths, J. Evid. Based Med., 14 (2021), 5-6. https://doi.org/10.1111/jebm.12421 doi: 10.1111/jebm.12421
![]() |
[17] |
E. D. Gorham, C. F. Garland, F. C. Garland, W. B. Grant, S. B. Mohr, M. Lipkin, et al., Optimal vitamin D status for colorectal cancer prevention: A quantitative meta analysis, Am. J. Prev. Med., 32 (2007), 210-216. https://doi.org/10.1016/j.amepre.2006.11.004 doi: 10.1016/j.amepre.2006.11.004
![]() |
[18] |
A. G. Pittas, S. S. Harris, P. C. Stark, H. B. Dawson, The effects of calcium and vitamin D supplementation on blood glucose and markers of inflammation in nondiabetic adults, Diabetes Care, 30 (2007), 980-986. https://doi.org/10.2337/dc06-1994 doi: 10.2337/dc06-1994
![]() |
[19] |
M. Urashima, T. Segawa, M. Okazaki, M. Kurihara, Y. Wada, H. Ida, Randomized trial of vitamin D supplementation to prevent seasonal influenza A in schoolchildren, Am. J. Clin. Nutr., 91 (2010), 1255-1260. https://doi.org/10.3945/ajcn.2009.29094 doi: 10.3945/ajcn.2009.29094
![]() |
[20] |
H. Pham, D. H. Pham, A novel generalized logistic dependent model to predict the presence of breast cancer based on biomarkers, Concurr. Comput. Pract. Exper., 32 (2020), 1. https://doi.org/10.1002/cpe.5467 doi: 10.1002/cpe.5467
![]() |
[21] |
V. Verhoeven, K. Vanpuyenbroeck, M. Lopez-Hartmann, J. Wens, R. Remmen, Walk on the sunny side of life—epidemiology of hypovitaminosis D and mental health in elderly nursing home residents, J. Nutr. Health Aging, 16 (2012), 417-420. https://doi.org/10.1007/s12603-011-0361-5 doi: 10.1007/s12603-011-0361-5
![]() |
[22] |
D. Boersma, O. Demontiero, A. Z. Mohtasham, S. Hassan, H. Suarez, D. Geisinger, et al., Vitamin D status in relation to postural stability in the elderly, J. Nutr. Health Aging, 16 (2012), 270-275. https://doi.org/10.1007/s12603-011-0345-5 doi: 10.1007/s12603-011-0345-5
![]() |
[23] |
N. Charoenngam, M. F. Holick, Immunologic effects of vitamin D on human health and disease, Nutrients, 12 (2020), 2097. https://doi.org/10.3390/nu12072097 doi: 10.3390/nu12072097
![]() |
[24] |
A. R. Martineau, D. A. Jolliffe, L. Greenberg, J. F. Aloia, P. Bergman, G. Dubnov-Raz, Vitamin D supplementation to prevent acute respiratory infections: Individual participant data meta-analysis, Health Technol. Assess., 23 (2019), 23020. https://doi.org/10.3310/hta23020 doi: 10.3310/hta23020
![]() |
[25] | C. E. Hayes, J. M. Ntambi, Multiple sclerosis: Lipids, Lymphocytes, and vitamin D, Immunometabolism, 10 (2020), 19. |
[26] |
K. de Haan, A. B. J. Groeneveld, H. R. H. de Geus, M. Egal, A. Struijs, Vitamin D deficiency as a risk factor for infection, sepsisand mortality in the critically ill: Systematic review and meta-analysis, Crit. Care, 66 (2014), 6604. https://doi.org/10.1186/s13054-014-0660-4 doi: 10.1186/s13054-014-0660-4
![]() |
[27] |
A. Braun, D. Chang, K. Mahadevappa, F. K. Gibbons, Y. Liu, E. Giovannucci, Association of low serum 25-hydroxyvitamin D levels and mortality in the critically ill, Crit. Care Med., 39 (2011), 671. https://doi.org/10.1097/CCM.0b013e318206ccdf doi: 10.1097/CCM.0b013e318206ccdf
![]() |
[28] |
P. Autier, M. Boniol, C. Pizot, P. Mullie, Vitamin D status and ill health: A systematic review, Lancet Diabetes Endocrinol., 2 (2014) 76. https://doi.org/10.1016/S2213-8587(13)70165-7 doi: 10.1016/S2213-8587(13)70165-7
![]() |
[29] |
Y. F. Zhou, B. A. Luo, L. L. Qin, The association between vitamin D deficiency and community-acquired pneumonia, Medicine, 98 (2019), e17252. https://doi.org/10.1097/MD.0000000000017252 doi: 10.1097/MD.0000000000017252
![]() |
[30] |
E. C. Goodall, A. C. Granados, K. Luinstra, E. Pullenayegum, B. L. Coleman, M. Loeb, Vitamin D3 and gargling for the prevention of upper respiratory tract infections: A randomized controlled trial, BMC Infect. Dis., 11 (2014), 14273. https://doi.org/10.1186/1471-2334-14-273 doi: 10.1186/1471-2334-14-273
![]() |
[31] |
A. S. Vanherwegen, C. Gysemans, C. Mathieu, Regulation of immune function by vitamin D and its use in diseases of immunity, Endocrinol. Metab. Clin. N. Am., 46 (2017), 1061. https://doi.org/10.1016/j.ecl.2017.07.010 doi: 10.1016/j.ecl.2017.07.010
![]() |
[32] |
C. Greiller, A. Martineau, Modulation of the immune response to respiratory viruses by vitamin D, Nutrients, 7 (2015), 4240-4270. https://doi.org/10.3390/nu7064240 doi: 10.3390/nu7064240
![]() |
[33] |
M. T. Zdrenghea, H. Makrinioti, C. Bagacean, A. Bush, S. L. Johnston, L.A. Stanciu, Vitamin D modulation of innate immune responses to respiratory viral infections, Rev. Med. Virol., 27 (2016), e1909. https://doi.org/10.1002/rmv.1909 doi: 10.1002/rmv.1909
![]() |
[34] |
J. R. Sabetta, P. DePetrillo, R. J. Cipriani, J. Smardin, L. A. Burns, M. L. Landry, Serum 25-hydroxyvitamin d and the incidence of acute viral respiratory tract infections in healthy adults, PLoS One, 5 (2010), e11088. https://doi.org/10.1371/journal.pone.0011088 doi: 10.1371/journal.pone.0011088
![]() |
[35] | T. R. Ingham, B. Jones, C. A. Camargo, J. Kirman, A. C. Dowell, J. Crane, Association of vitamin D deficiency with severity of acute respiratory infection: A case-control study in New Zealand children, Eur. Respir. J. Eur. Respir. Soc., 44 (2014), 124. |
[36] |
C. FGunville, P. MMourani, A. AGinde, The role of vitamin D in prevention and treatment of infection, Inflamm. Allergy-DrugTargets, 12 (2013), 239-245. https://doi.org/10.2174/18715281113129990046 doi: 10.2174/18715281113129990046
![]() |
[37] |
A. L. Khoo, L. Chai, H. Koenen, I. Joosten, M. Netea, A. van der Ven, Translating the role of vitamin D3 in infectious diseases, Crit. Rev. Microbiol., 38 (2012), 122. https://doi.org/10.3109/1040841X.2011.622716 doi: 10.3109/1040841X.2011.622716
![]() |
[38] |
R. Taha, S. Abureesh, S. Alghamdi, R. Y. Hassan, M. M. Cheikh, R. A. Bagabir, The relationship between vitamin D and infections including COVID-19: Any hopes? Int. J. Gen. Med., 14 (2021), 3849. https://doi.org/10.2147/IJGM.S317421 doi: 10.2147/IJGM.S317421
![]() |
[39] |
A. R. Martineau, D. A. Jolliffe, R. L. Hooper, L. Greenberg, J. F. Aloia, P. Bergman, Vitamin D supplementation to prevent acute respiratory tract infections: Systematic review and meta-analysis of individual participant data, BMJ, 65 (2017), 6583. https://doi.org/10.1136/bmj.i6583 doi: 10.1136/bmj.i6583
![]() |
[40] |
R. C. A. Dancer, D. Parekh, S. Lax, V. D'Souza, S. Zheng, C. R. Bassford, Vitamin D deficiency contributes directly to the acute respiratory distress syndrome (ARDS), Thorax BMJ, 70 (2015), 617. https://doi.org/10.1136/thoraxjnl-2014-206680 doi: 10.1136/thoraxjnl-2014-206680
![]() |
[41] |
D. R. Thickett, T. Moromizato, A. A. Litonjua, K. Amrein, S. A. Quraishi, K. A. Lee-Sarwar, Association between prehospital vitamin D status and incident acute respiratory failure in critically ill patients: A retrospective cohort study, BMJ Open Respir. Res., 2 (2015), e74. https://doi.org/10.1136/bmjresp-2014-000074 doi: 10.1136/bmjresp-2014-000074
![]() |
[42] | M. T. Cantorna, B. D. Mahon, D-hormone and the immune system, J. Rheumatol. Suppl., 76 (2005), 11-20. |
[43] |
A. Antico, M. Tampoia, R. Tozzoli, N. Bizzaro, Can supplementation with vitamin D reduce the risk or modify the course of autoimmune diseases? A systematic review of the literature, Autoimmun. Rev., 12 (2012), 127. https://doi.org/10.1016/j.autrev.2012.07.007 doi: 10.1016/j.autrev.2012.07.007
![]() |
[44] | T. Michigami, O. Rickets, Consensus on vitamin D deficiency and insufficiency in children, Clin. Calcium., 28 (2018), 1307-1311. |
[45] |
W. Grant, H. Lahore, S. McDonnell, C. Baggerly, C. French, J. Aliano, Evidence that vitamin D supplementation could reduce risk of influenza and COVID-19 infections and deaths, Nutrients, 12 (2020), 988. https://doi.org/10.3390/nu12040988 doi: 10.3390/nu12040988
![]() |
[46] |
P. A. Danai, S. Sinha, M. Moss, M. J. Haber, G. S. Martin, Seasonal variation in the epidemiology of sepsis, Crit. Care Med., 35 (2007), 410-415. https://doi.org/10.1097/01.CCM.0000253405.17038.43 doi: 10.1097/01.CCM.0000253405.17038.43
![]() |
[47] |
W. B. Grant, Variations in vitamin D production could possibly explain the seasonality of childhood respiratory infections in Hawaii, Pediatr. Infect. Dis. J., 27 (2008), 853. https://doi.org/10.1097/INF.0b013e3181817bc1 doi: 10.1097/INF.0b013e3181817bc1
![]() |
[48] |
J. J. Cannell, R. Vieth, J. Umhau, M. Holick, W. Grant, S. Madronich, et al., Epidemic influenza and vitamin D, Epidemiol. Infect., 134 (2006), 1129-1140. https://doi.org/10.1017/S0950268806007175 doi: 10.1017/S0950268806007175
![]() |
[49] |
V. Dimitrov, C. Barbier, A. Ismailova, Y. Wang, K. Dmowski, R. Salehi-Tabar, et al., Vitamin D-regulated gene expression profiles: species-specificity and cell-specific effects on metabolism and immunity, Endocrinology, 162 (2021), 218. https://doi.org/10.1210/endocr/bqaa218 doi: 10.1210/endocr/bqaa218
![]() |
[50] |
M. Olliver, L. Spelmink, J. Hiew, U. Meyer-Hoffert, B. Henriques-Normark, P. Bergman, Immunomodulatory effects of vitamin D on innate and adaptive immune responses to Streptococcus pneumoniae, J. Infect. Dis., 208 (2013), 1474-1481. https://doi.org/10.1093/infdis/jit355 doi: 10.1093/infdis/jit355
![]() |
[51] |
S. Maggini, E. S. Wintergerst, S. Beveridge, D. H. Hornig, Selected vitamins and trace elements support immune function by strengthening epithelial barriers and cellular and humoral immune responses, Br. J. Nut., 98 (2007), S29-S35. https://doi.org/10.1017/S0007114507832971 doi: 10.1017/S0007114507832971
![]() |
[52] |
W. B. Grant, H. Lahore, S. L. McDonnell, C. A. Baggerly, C. B. French, J. L. Aliano, et al., Evidence that vitamin D supplementation could reduce risk of influenza and COVID-19 infections and deaths, Nutrients, 12 (2020), 988. https://doi.org/10.3390/nu12040988 doi: 10.3390/nu12040988
![]() |
[53] |
S. Piumika, T. J. Dhanushya, K. Neil, J. Ranil, Impact of vitamin D deficiency on COVID-19, Clin. Nutr. ESPEN, 44 (2021), 372-378. https://doi.org/10.1016/j.clnesp.2021.05.011 doi: 10.1016/j.clnesp.2021.05.011
![]() |
[54] | E. Laird, R. A. Kenny, Vitamin D deficiency in Ireland-implications for COVID-19. Results from the Irish Longitudinal Study on Ageing (TILDA), April 2020. https://doi.org/10.38018/TildaRe.2020-05 |
[55] |
D. P. Richardson, J. A. Lovegrove, Nutritional status of micronutrients as a possible and modifiable risk factor for COVID-19: A UK perspective, Br. J. Nutr., 125 (2021), 678-684. https://doi.org/10.1017/S000711452000330X doi: 10.1017/S000711452000330X
![]() |
[56] |
M. Ebadi, A. J. Montano-Loza, Perspective: Improving vitamin D status in the management of COVID-19, Eur. J. Clin. Nutr., 74 (2020), 856-859. https://doi.org/10.1038/s41430-020-0661-0 doi: 10.1038/s41430-020-0661-0
![]() |
[57] |
W. B. Grant, H. Lahore, S. L. McDonnell, C. A. Baggerly, C. B. French, J. L. Aliano, et al., Evidence that vitamin D supplementation could reduce risk of influenza and COVID-19 infections and deaths, Nutrients, 12 (2020), 988. https://doi.org/10.3390/nu12040988 doi: 10.3390/nu12040988
![]() |
[58] | D. M. McCartney, D. G. Byrne, Optimisation of vitamin D status for enhanced Immuno-protection against Covid-19, Ir. Med. J., 113 (2020), 58. |
[59] |
M. Di Rosa, M. Malaguarnera, F. Nicoletti, L. Malaguarnera, Vitamin D3: A helpful immuno-modulator. Immunology, 134 (2020), 123-139. https://doi.org/10.1111/j.1365-2567.2011.03482.x doi: 10.1111/j.1365-2567.2011.03482.x
![]() |
[60] |
J. Zhong, J. Tang, C. Ye, L. Dong, The immunology of COVID-19: Is immune modulation an option for treatment? Lancet Rheumatol., 2 (2020), e428-e436. https://doi.org/10.1016/S2665-9913(20)30120-X doi: 10.1016/S2665-9913(20)30120-X
![]() |
[61] |
A. Panarese, E. Shahini, Covid-19, and vitamin D, Aliment. Pharmacol. Ther., 51 (2020), 993. https://doi.org/10.1111/apt.15752 doi: 10.1111/apt.15752
![]() |
[62] |
G. Grasselli, T. Tonetti, A. Protti, T. Langer, M. Girardis, G. Bellani, et al., Pathophysiology of COVID-19-associated acute respiratory distress syndrome: A multicentre prospective observational study, Lancet Respir. Med., 8 (2020), 1201-1208. https://doi.org/10.1016/S2213-2600(20)30370-2 doi: 10.1016/S2213-2600(20)30370-2
![]() |
[63] |
A. A. Ginde, J. M. Mansbach, C. A. Camargo, Association between serum 25-hydroxyvitamin D level and upper respiratory tract infection in the Third National Health and Nutrition Examination Survey, Arch. Int. Med., 169 (2009), 384-390. https://doi.org/10.1001/archinternmed.2008.560 doi: 10.1001/archinternmed.2008.560
![]() |
[64] |
D. A. Jolliffe, C. J. Griffiths, A. R. Martineau, Vitamin D in the prevention of acute respiratory infection: Systematic review of clinical studies, J. Steroid Biochem. Mol. Biol., 136 (2020), 321-329. https://doi.org/10.1016/j.jsbmb.2012.11.017 doi: 10.1016/j.jsbmb.2012.11.017
![]() |
[65] |
D. Prantas, S. Nandeeta, O. A. Bright, E. H. John, P. Prince, M. Aliu, et al., Effect of vitamin D deficiency on COVID-19 status: A systematic review, COVID, 1 (2021), 97-104. https://doi.org/10.3390/covid1010008 doi: 10.3390/covid1010008
![]() |
[66] |
A. Radujkovic, T. Hippchen, S. Tiwari-Heckler, S. Dreher, M. Boxberger, U. Merle, Vitamin D deficiency and outcome of COVID-19 patients, Nutrients, 12 (2020), 2757. https://doi.org/10.3390/nu12092757 doi: 10.3390/nu12092757
![]() |
[67] |
D. O. Meltzer, T. J. Best, H. Zhang, T. Vokes, V. Arora, J. Solway, Association of vitamin D status and other clinical characteristics with COVID-19 test results, JAMA Netw. Open, 3 (2020), e2019722. https://doi.org/10.1001/jamanetworkopen.2020.19722 doi: 10.1001/jamanetworkopen.2020.19722
![]() |
[68] |
C. E. Hastie, D. F. Mackay, F. Ho, C. A. Celis-Morales, S. V. Katikireddi, C. L. Niedzwiedz, et al., Vitamin D concentrations and COVID-19 infection in UK Biobank, Diabetes Metab. Syndr. Clin. Res. Rev., 14 (2020), 561-565. https://doi.org/10.1016/j.dsx.2020.04.050 doi: 10.1016/j.dsx.2020.04.050
![]() |
[69] |
A. Abrishami, N. Dalili, P. M. Torbati, R. Asgari, M. Arab-Ahmadi, B. Behnam, et al., Possible association of vitamin D status with lung involvement and outcome in patients with COVID-19: A retrospective study, Eur. J. Nutr., 60 (2020), 2249-2257. https://doi.org/10.1007/s00394-020-02411-0 doi: 10.1007/s00394-020-02411-0
![]() |
[70] |
V. Baktash, T. Hosack, N. Patel, S. Shah, P. Kandiah, K. Van den Abbeele, et al., Vitamin D status and outcomes for hospitalised older patients with COVID-19, Postgrad. Med. J., 2 (2020), 1-6. https://doi.org/10.1136/postgradmedj-2020-138712 doi: 10.1136/postgradmedj-2020-138712
![]() |
[71] |
Z. Maghbooli, M. A. Sahraian, M. Ebrahimi, M. Pazoki, M. Kafan, S. Tabriz, et al., Vitamin D sufficiency, a serum 25-hydroxyvitamin D at least 30 ng/mL reduced risk for adverse clinical outcomes in patients with COVID-19 infection, PLoS One, 15 (2020), e0239799. https://doi.org/10.1371/journal.pone.0239799 doi: 10.1371/journal.pone.0239799
![]() |
[72] |
K. Ye, F. Tang, X. Liao, B. A. Shaw, M. Deng, G. Huang, et al., Does serum vitamin D level affect COVID-19 infection and its severity? A case-control study, J. Am. Coll. Nutr., (2020), 1-8. https://doi.org/10.1080/07315724.2020.1826005 doi: 10.1080/07315724.2020.1826005
![]() |
[73] |
P. Bergman, A. C. Norlin, S. Hansen, R. S. Rekha, B. Agerberth, L. Björkhem-Bergman, et al., Vitamin D3 supplementation in patients with frequent respiratory tract infections: A randomised and double-blind intervention study, BMJ Open, 2 (2012), e001663. https://doi.org/10.1136/bmjopen-2012-001663 doi: 10.1136/bmjopen-2012-001663
![]() |
[74] |
A. R. Martineau, N. G. Forouhi, Vitamin D for COVID-19: A case to answer? Lancet Diabetes Endocrinol, 8 (2020), 735-736. https://doi.org/10.1016/S2213-8587(20)30268-0 doi: 10.1016/S2213-8587(20)30268-0
![]() |
[75] |
N. Ali, Role of vitamin D in preventing of COVID-19 infection, progression and severity, J. Infect. Public Health, 13 (2020), 1373-1380. https://doi.org/10.1016/j.jiph.2020.06.021 doi: 10.1016/j.jiph.2020.06.021
![]() |
[76] |
P. C. Ilie, S. Stefanescu, L. Smith, The role of vitamin D in the prevention of coronavirus disease 2019 infection and mortality, Aging Clin. Exp. Res., 32 (2020), 1195-1198. https://doi.org/10.1007/s40520-020-01570-8 doi: 10.1007/s40520-020-01570-8
![]() |
[77] |
S. Walrand, Autumn COVID-19 surge dates in Europe correlated to latitudes, not to temperature-humidity, pointing to vitamin D as contributing factor, Sci. Rep., 11 (2021), 1981. https://doi.org/10.1038/s41598-021-81419-w doi: 10.1038/s41598-021-81419-w
![]() |
[78] |
E. Klingberg, G. Oleröd, J. Konar, M. Petzold, O. Hammarsten, Seasonal variations in serum 25-hydroxy vitamin D levels in a Swedish cohort, Endocrine, 49 (2015), 800-808. https://doi.org/10.1007/s12020-015-0548-3 doi: 10.1007/s12020-015-0548-3
![]() |
[79] | Institute of Medicine. Dietary reference intakes for calcium and vitamin D. Washington, DC: The National Academies Press, 2011. |
[80] |
M. F. Holick N. C. Binkley, H. A. Bischoff-Ferrari, C. M. Gordon, D. A. Hanley, R. P. Heaney, et al., Evaluation, treatment, and prevention of vitamin D deficiency: An Endocrine Society Clinical Practice Guideline, J. Clin. Endocrinol. Metab., 96 (2011), 1911-1130. https://doi.org/10.1210/jc.2011-0385 doi: 10.1210/jc.2011-0385
![]() |
[81] |
D. E. Roth, P. Martz, R. Yeo, C. Prosser, M. Bell, A. B. Jones, Are national vitamin D guidelines sufficient to maintain adequate blood levels in children? Can. J. Public Health, 96 (2005), 443-449. https://doi.org/10.1007/BF03405185 doi: 10.1007/BF03405185
![]() |
[82] | D. Rucker, J. A. Allan, G. H. Fick, D. A. Hanley, Vitamin D insufficiency in a population of healthy western Canadians, CMAJ, 166 (2002), 1517-1524. |
[83] |
R. Vieth, D. E. Cole, G. A. Hawker, H. M. Trang, L. A. Rubin, Winter time vitamin D insufficiency is common in young Canadian women, and their vitamin D intake does not prevent it, Eur. J. Clin. Nutr., 55 (2001), 1091-1097. https://doi.org/10.1038/sj.ejcn. 1601275 doi: 10.1038/sj.ejcn.1601275
![]() |
[84] |
R. P. Heaney, K. M. Davies, T. C. Chen, M. F. Holick, M. J. Barger-Lux, Human serum 25-hydroxycholecalciferol response to extended oral dosing with cholecalciferol, Am. J. Clin. Nutr., 77 (2003), 204-210. https://doi.org/10.1093/ajcn/77.1.204 doi: 10.1093/ajcn/77.1.204
![]() |
[85] |
J. N. Hathcock, A. Shao, R. Vieth, R. Heaney, Risk assessment for vitamin D, Am. J. Clin. Nutr., 85 (2007), 6-18. https://doi.org/10. 1093/ajcn/85.1.6 doi: 10.1093/ajcn/85.1.6
![]() |
[86] |
M. Pfeifer, B. Begerow, H. W. Minne, C. Abrams, D. Nachtigall, C. Hansen, Effects of a short-term vitamin D and calcium supplementation on body sway and secondary hyperparathyroidism in elderly women, J. Bone Miner Res., 15 (2000), 1113-1118. https://doi.org/10.1359/jbmr.2000.15.6.1113 doi: 10.1359/jbmr.2000.15.6.1113
![]() |
[87] | Medicine Io, Dietary reference intakes for calcium, phosphorus, magnesium, vitamin D, and fluoride. The Washington, DC: National Academies Press, 1997. |
[88] |
J. F. Aloia, M. Patel, R. DiMaano, M. Li-Ng, S. A. Talwar, M. Mikhail, et al., Vitamin D intake to attain a desired serum 25- hydroxyvitamin D concentration, Am. J. Clin. Nutr., 87 (2008), 1952-1958. https://doi.org/10.1093/ajcn/87.6.1952 doi: 10.1093/ajcn/87.6.1952
![]() |
[89] |
A. C. Ross, J. E. Manson, S. A. Abrams, J. F. Aloia, P. M. Brannon, S. K. Clinton, et al., The 2011 report on dietary reference intakes for calcium and vitamin D from the institute of medicine: what clinicians need to know, J. Clin. Endocrinol. Metab., 96 (2011), 53-58. https://doi.org/10.1016/j.jada.2011.01.004 doi: 10.1016/j.jada.2011.01.004
![]() |
[90] | EFSA Panel on Dietetic Products N, Allergies. Scientific opinion on the tolerable upper intake level of vitamin D, EFSA J., 10 (2012), 2813. https://doi.org/10.2903/j.efsa.2012.2813 |
[91] |
D. A. Hanley, A. Cranney, G. Jones, S. J. Whiting, W. D. Leslie, D. E. Cole, et al., Vitamin D in adult health and disease: A review and guideline statement from Osteoporosis Canada, CMAJ, 182 (2010), E610-E618. https://doi.org/10.1503/cmaj.080663 doi: 10.1503/cmaj.080663
![]() |
[92] |
S. M. Pietras, B. K. Obayan, M. H. Cai, M. F. Holick, Vitamin D2 treatment for vitamin D deficiency and insufficiency for up to 6 Years, JAMA Intern. Med., 169 (2009), 1806-1818. https://doi.org/10. 1001/archinternmed.2009.361 doi: 10.1001/archinternmed.2009.361
![]() |
[93] |
S. L. McDonnell, C. A. Baggerly, C. B. French, L. L. Baggerly, C. F. Garland, E. D. Gorham, et al., Breast cancer risk markedly lower with serum 25-hydroxyvitamin D concentrations ≥60 vs < 20 ng/ml (150 vs 50 nmol/L): Pooled analysis of two randomized trials and a prospective cohort, PLoS One, 13 (2018), e0199265. https://doi.org/10.1371/journal.pone.0199265 doi: 10.1371/journal.pone.0199265
![]() |
[94] |
J. M. Madden, L. Murphy, L. Zgaga, K. K. Bennett, De novo vitamin D supplement use post-diagnosis is associated with breast cancer survival, Breast Cancer Res. Treat., 172 (2018), 179-90. https://doi.org/10.1007/s10549-018-4896-6 doi: 10.1007/s10549-018-4896-6
![]() |
[95] |
N. Mirhosseini, H. Vatanparast, S. M. Kimball, The association between Serum 25(OH)D status and blood pressure in participants of a community-based program taking vitamin D supplements, Nutrients, 9 (2017), 1244. https://doi.org/10.3390/nu9111244 doi: 10.3390/nu9111244
![]() |
[96] |
A. Rusińska, P. Płudowski, M. Walczak, M. K. Borszewska-Kornacka, A. Bossowski, D. Chlebna-Sokół, et al., Vitamin D supplementation guidelines for general population and groups at risk of vitamin D deficiency in poland—recommendations of the polish society of pediatric endocrinology and diabetes and the expert panel with participation of national specialist consultants and representatives of scientific societies, Front. Endocrinol., 9 (2018). https://doi.org/10.3389/fendo.2018.00246 doi: 10.3389/fendo.2018.00246
![]() |
[97] |
K. Amrein, M. Scherkl, M. Hoffmann, S. Neuwersch-Sommeregger, M. Köstenberger, A. Tmava Berisha, et al., Vitamin D deficiency 2.0: An update on the current status worldwide, European J. Clin. Nutr., 74 (2020), 1498-1513. https://doi.org/10.1038/s41430-020-0558-y doi: 10.1038/s41430-020-0558-y
![]() |
[98] |
N. Charoenngam, M. F. Holick, Immunologic effects of vitamin d on human health and disease, Nutrients, 12 (2020), 1-28. https://doi.org/10.3390/nu12072097 doi: 10.3390/nu12072097
![]() |
[99] |
M. Chakhtoura, M. Rahme, N. Chamoun, G. El-Hajj Fuleihan, Vitamin D in the Middle East and North Africa, Bone Rep., 8 (2018), 135-146. https://doi.org/10.1016/j.bonr.2018.03.004 doi: 10.1016/j.bonr.2018.03.004
![]() |
[100] |
A. Batieha, Y. Khader, H. Jaddou, D. Hyassat, Z. Batieha, M. Khateeb, et al., Vitamin D status in Jordan: dress style and gender discrepancies, Ann. Nutr. Metab., 58 (2011), 10-18. https://doi.org/10.1159/000323097 doi: 10.1159/000323097
![]() |
[101] |
P. Bergman, A. U. Lindh, L. Björkhem-Bergman, J. D. Lindh, Vitamin D and respiratory tract infections: A systematic review and meta-analysis of randomized controlled trials, PLoS One, 8 (2013), e65835. https://doi.org/10.1371/journal.pone.0065835 doi: 10.1371/journal.pone.0065835
![]() |
[102] |
D. A. Jolliffe, C. A. Camargo, J. D. Sluyter, M. Aglipay, J. F. Aloia, D. Ganmaa, et al., Vitamin D supplementation to prevent acute respiratory infections: A systematic review and meta-analysis of aggregate data from randomised controlled trials, Lancet Diab. Endocrinol., 9 (2021), 276-292. https://doi.org/10.1136/thorax-2020-BTSabstracts.105 doi: 10.1136/thorax-2020-BTSabstracts.105
![]() |
[103] |
J. M. Rhodes, S. Subramanian, E. Laird, G. Griffig, R. A. Kenny, Perspective: Vitamin D deficiency and COVID-19 severity—plausibly linked by latitude, ethnicity, impacts on cytokines, ACE2 and thrombosis, J. Intern. Med., 289 (2021), 97-115. https://doi.org/10.1111/joim.13149 doi: 10.1111/joim.13149
![]() |
[104] |
D. O. Meltzer, T. J. Best, H. Zhang, T. Vokes, V. Arora, J. Solway, Association of vitamin D status and other clinical characteristics with COVID-19 test results, JAMA Netw. Open., 3 (2020), e2019722. https://doi.org/10.1001/jamanetworkopen.2020.19722 doi: 10.1001/jamanetworkopen.2020.19722
![]() |
[105] |
E. Merzon, D. Tworowski, A. Gorohovski, S. Vinker, A. G. Cohen, I. Green, et al., Low plasma 25(OH) vitamin D level is associated with increased risk of COVID-19 infection: An Israeli population-based study, FEBS J., 287 (2020), 3693-3702. https://doi.org/10.1111/febs.15495 doi: 10.1111/febs.15495
![]() |
[106] |
A. Jain, R. Chaurasia, N. S. Sengar, M. Singh, S. Mahor, S, Narain, Analysis of vitamin D level among asymptomatic and critically ill COVID-19 patients and its correlation with inflammatory markers, Sci. Rep., 10 (2020), 20191. https://doi.org/10.1038/s41598-020-77093-z doi: 10.1038/s41598-020-77093-z
![]() |
[107] | D. De Smet, K. De Smet, P. Herroelen, S. Gryspeerdt, G. A. Martens, Vitamin D deficiency as risk factor for severe COVID-19: A convergence of two pandemics, medRxiv, (2020). https://doi.org/10.1101/2020.05.01.20079376 |
[108] |
R. L. Schleicher, M. R. Sternberg, A. C. Looker, E. A. Yetley, D. A. Lacher, C. T. Sempos, et al., National estimates of serum total 25-Hydroxyvitamin D and metabolite concentrations measured by liquid chromatography-Tandem mass spectrometry in the US population during 2007-2010, J. Nutr., 146 (2016), 1051-1061.https://doi.org/10.3945/jn.115.227728 doi: 10.3945/jn.115.227728
![]() |
[109] |
K. Sarafin, R. Durazo-Arvizu, L. Tian, K. W. Phinney, S. Tai, J. E. Camara, et al., Standardizing 25-hydroxyvitamin D values from the Canadian health measures survey, Am. J. Clin. Nutr., 102 (2015), 1044-1050. https://doi.org/10.3945/ajcn.114.103689 doi: 10.3945/ajcn.114.103689
![]() |
[110] |
K. D. Cashman, K. G. Dowling, Z. Škrabáková, M. Gonzalez-Gross, J. Valtueña, S. De Henauw, et al., Vitamin D deficiency in Europe: pandemic? Am. J. Clin. Nutr., 103 (2016), 1033-1044. https://doi.org/ 10.3945/ajcn.115.120873 doi: 10.3945/ajcn.115.120873
![]() |
[111] |
K. D. Cashman, Vitamin D deficiency: Defining, prevalence, causes, and strategies of addressing, Calcif. Tissue Int., (2019). https://doi.org/10.1007/s00223-019-00559-4 doi: 10.1007/s00223-019-00559-4
![]() |
[112] |
H. Pham, Modeling U.S. mortality and risk-cost optimization on life expectancy, IEEE Trans. Reliabil., 60 (2011), 125-133. https://doi.org/10.1109/TR.2010.2103990 doi: 10.1109/TR.2010.2103990
![]() |
[113] |
A. A. Dror, N. Morozov, A. Daoud, Y. Namir, O. Yakir, Y. Shachar, et al., Pre-infection 25-hydroxyvitamin D3 levels and association with severity of COVID-19 illness, PLoS One, 17 (2022), e0263069. https://doi.org/10.1371/journal.pone.0263069 doi: 10.1371/journal.pone.0263069
![]() |
[114] |
J. Ng, Y. R. Stovezky, D. J. Brenner, S. C. Formenti, I. Shuryak, Development of a model to estimate the association between delay in cancer treatment and local tumor control and risk of metastates, JAMA Netw. Open, 4 (2021), 1-10. https://doi.org/10.1001/jamanetworkopen.2020.34065 doi: 10.1001/jamanetworkopen.2020.34065
![]() |
[115] |
A. Talkington, R. Durrett, Estimating tumor growth rates in vivo, Bull. Math. Biol., 77 (2015), 1934-1954. https://doi.org/10.1007/s11538-015-0110-8 doi: 10.1007/s11538-015-0110-8
![]() |
[116] |
C. Vaghi, A. Rodallec, R. Fanciullino, J. Ciccolini, J. P. Mochel, M. Mastri, Population modeling of tumor growth curves and the reduced Gompertz model improve prediction of the age of experimental tumors, PLoS Comput. Biol., 16 (2020), e1007178. https://doi.org/10.1371/journal.pcbi.1007178 doi: 10.1371/journal.pcbi.1007178
![]() |
[117] |
D. J. Yin, A. R. Moes, J. G. C. van Hasselt, J. J. Swen, H. J. Guchelaar, A review of mathematical models for tumor dynamics and treatment resistance evolution of solid tumors, CPTPharmacomet. Syst. Pharmacol., 8 (2019), 720-737. https://doi.org/10.1002/psp4.12450 doi: 10.1002/psp4.12450
![]() |
[118] |
T. L. Jackson, H. M. A. Byrne, A mathematical model to study the effects of drug resistance and vasculature on the response of solid tumors to chemotherapy, Math. Biosci., 104 (2000), 17-38. https://doi.org/10.1016/S0025-5564(99)00062-0 doi: 10.1016/S0025-5564(99)00062-0
![]() |
[119] |
C. E. Meacham, S. J. Morrison, Tumour heterogeneity and cancer cell plasticity, Nature, 501 (2013), 328-337. https://doi.org/10.1038/nature12624 doi: 10.1038/nature12624
![]() |
[120] |
X. Sun, J. Bao, Y. Z. Shao, Mathematical modeling of therapy-induced cancer drug resistance: Connecting cancer mechanisms to population survival rates, Sci. Rep., 6 (2016) 22498. https://doi.org/10.1038/srep22498 doi: 10.1038/srep22498
![]() |
[121] |
K. Taniguchi, J. Okami, K. Kodama, M. Higashiyama, K. Kato, Intratumor heterogeneity of epidermal growth factor receptor mutations in lung cancer and its correlation to the response to gefitinib, Cancer Sci., 99 (2008), 929-935. https://doi.org/10.1111/j.1349-7006.2008.00782.x doi: 10.1111/j.1349-7006.2008.00782.x
![]() |
[122] |
E. R. Lestari, H. Arifah, Dynamics of a mathematical model of cancer cells with chemotherapy, J. Phys. Conf. Ser., 1320 (2019), 1-8. https://doi.org/10.1088/1742-6596/1320/1/012026 doi: 10.1088/1742-6596/1320/1/012026
![]() |
[123] |
H. Pham, A dynamic model of multiple time-delay interactions between the virus-infected cells and body's immune system with autoimmune diseases, Axioms, 10 (2021), 216. https://doi.org/10.3390/axioms10030216 doi: 10.3390/axioms10030216
![]() |
[124] |
H. Pham, Mathematical modeling the time-delay interactions between tumor viruses and the immune system with the effects of chemotherapy and autoimmune Diseases, Mathematics, 10 (2022), 756. https://doi.org/10.3390/math10050756 doi: 10.3390/math10050756
![]() |
1. | Lin Sun, Shanshan Si, Jing Zhao, Jiucheng Xu, Yaojin Lin, Zhiying Lv, Feature selection using binary monarch butterfly optimization, 2023, 53, 0924-669X, 706, 10.1007/s10489-022-03554-9 | |
2. | Amjad Qtaish, Malik Braik, Dheeb Albashish, Mohammad T. Alshammari, Abdulrahman Alreshidi, Eissa Jaber Alreshidi, Enhanced coati optimization algorithm using elite opposition-based learning and adaptive search mechanism for feature selection, 2024, 1868-8071, 10.1007/s13042-024-02222-3 | |
3. | Xi-Ao Ma, Hao Xu, Yi Liu, Justin Zuopeng Zhang, Class-specific feature selection using fuzzy information-theoretic metrics, 2024, 136, 09521976, 109035, 10.1016/j.engappai.2024.109035 | |
4. | Lei Xiao, 2024, Evaluation Model Construction Based on 'Three-dimensional' Data Fusion in Cross-cultural Blended Course, 9798400710360, 11, 10.1145/3686424.3686426 | |
5. | Pedro J. Gutiérrez-Diez, Jorge Alves-Antunes, Stock market uncertainty determination with news headlines: A digital twin approach, 2023, 9, 2473-6988, 1683, 10.3934/math.2024083 | |
6. | Ah. E. Hegazy, B. Hafiz, M. A. Makhlouf, Omar A. M. Salem, Optimizing medical data classification: integrating hybrid fuzzy joint mutual information with binary Cheetah optimizer algorithm, 2025, 28, 1386-7857, 10.1007/s10586-025-05102-9 |
No. | Datasets | Instances | Features | Classes |
1 | Acute Inflammations | 120 | 6 | 2 |
2 | Arrhythmia | 452 | 279 | 13 |
3 | Blogger | 100 | 6 | 2 |
4 | Diabetic Retinopathy Debrecen (DRD) | 1151 | 20 | 2 |
5 | Hayes-Roth | 160 | 5 | 3 |
6 | Indian Liver Patient Dataset (ILPD) | 583 | 10 | 2 |
7 | Lenses | 24 | 4 | 3 |
8 | Lymphography | 148 | 18 | 4 |
9 | Congressional Voting Records (CVR) | 435 | 16 | 2 |
10 | Sonar | 208 | 60 | 2 |
11 | Thoracic Surgery | 470 | 17 | 2 |
12 | Wilt | 4889 | 6 | 2 |
13 | Zoo | 101 | 17 | 7 |
Dataset | CMIM | CMIM3 | JMI | JMI3 | JMIM | MIGM | QPFS | Relief | WRFS | FJMIM |
Acute Inflammations | 100±0[=] | 100±0[=] | 100±0[=] | 100±0[=] | 100±0[=] | 99.75±2.5[=] | 99.75±2.5[=] | 100±0[=] | 90.83±8.5[+] | 100±0 |
Arrhythmia | 60.64±7.01[=] | 59.22±6.17[=] | 59.42±6.49[=] | 59.58±6.58[=] | 60.62±6.93[=] | 58.98±6.39[=] | 63.7±6.57[-] | 64.94±6.33[-] | 59.31±6.3[=] | 59.74±6.43 |
Blogger | 61.9±10.7[=] | 61.9±10.7[=] | 61.9±10.7[=] | 64.9±10.2[=] | 61.9±10.7[=] | 61.9±10.7[=] | 61.9±10.7[=] | 65.4±7.84[=] | 66.1±9.09[=] | 65.9±10.26 |
DRD | 57.59±4.95[=] | 60.66±4.69[-] | 60.3±5.04[-] | 60.6±4.58[-] | 57.59±4.95[=] | 60.3±5.04[-] | 61.19±4.49[-] | 60.61±5.06[-] | 57.47±4.98[=] | 57.56±4.83 |
Hayes-Roth | 45.81±10.77[=] | 45.81±10.77[=] | 45.81±10.77[=] | 45.81±10.77[=] | 45.81±10.77[=] | 45.81±10.77[=] | 49.37±10.53[=] | 46.31±11.48[=] | 45.81±10.77[=] | 49.37±10.53 |
ILPD | 68.99±5.68[=] | 67.33±5.87[=] | 68.99±5.68[=] | 67.33±5.87[=] | 68.99±5.68[=] | 68.99±5.68[=] | 68.73±5.64[=] | 64.96±6.45[+] | 70.11±6.74[=] | 69.54±5.75 |
Lenses | 86.83±21.88[=] | 86.83±21.88[=] | 86.83±21.88[=] | 86.83±21.88[=] | 86.83±21.88[=] | 86.83±21.88[=] | 86.83±21.88[=] | 54.83±30.73[+] | 86.83±21.88[=] | 86.83±21.88 |
Lymphography | 79.02±10.57[=] | 82.05±9.59[=] | 79.02±10.57[=] | 77.93±10.87[=] | 78.27±10.98[=] | 77.25±11.38[=] | 79.02±10.57[=] | 82.61±9.77[=] | 76.91±11.64[+] | 81.5±10.29 |
CVR | 94.3±3.15[=] | 94.32±3.12[=] | 93.75±3.57[=] | 93.75±3.57[=] | 93.75±3.57[=] | 93.75±3.57[=] | 94.32±3.26[=] | 93.82±3.28[=] | 93.52±3.18[=] | 93.75±3.57 |
Sonar | 75.05±8.33[=] | 75.42±9.08[=] | 75.75±8.85[=] | 76.23±9.99[=] | 72.2±9.93[=] | 72.14±9.54[+] | 76.42±8.69[=] | 74.25±8.33[=] | 77.91±8.74[=] | 77±8.98 |
Thoracic Surgery | 83.83±3.1[=] | 83.38±3.09[=] | 83.38±3.09[=] | 83.85±2.24[=] | 83.38±3.09[=] | 84.64±1.83[=] | 83.55±3.08[=] | 83.06±2.77[=] | 83.85±2.74[=] | 83.38±3.09 |
Wilt | 94.61±0.06[=] | 94.61±0.06[=] | 94.61±0.06[=] | 94.61±0.06[=] | 94.61±0.06[=] | 94.61±0.06[=] | 94.61±0.06[=] | 94.61±0.06[=] | 94.61±0.06[=] | 94.61±0.06 |
Zoo | 95.15±5.74[=] | 96.05±5.6[=] | 96.03±5.66[=] | 96.03±5.66[=] | 96.03±5.66[=] | 96.93±5.42[=] | 94.08±6.6[=] | 94.08±6.6[=] | 92.96±7.05[=] | 95.05±5.87 |
Average | 77.21 | 77.51 | 77.37 | 77.50 | 76.92 | 77.07 | 77.96 | 75.34 | 76.63 | 78.02 |
Dataset | CMIM | CMIM3 | JMI | JMI3 | JMIM | MIGM | QPFS | Relief | WRFS | FJMIM |
Acute Inflammations | 98.33±3.35[=] | 99.67±1.64[=] | 98.33±3.35[=] | 98.33±3.35[=] | 98.33±3.35[=] | 99.42±2.44[=] | 99.42±2.44[=] | 100±0[+] | 89.92±9.5[+] | 99.42±2.44 |
Arrhythmia | 66.09±6.81[=] | 64.08±6.11[=] | 65.01±5.96[=] | 65.7±6.23[=] | 66.14±6.61[=] | 65.23±6.39[=] | 66.82±6.2[=] | 67.22±6.05[=] | 65.23±6.15[=] | 67.11±6.7 |
Blogger | 68±4.02[=] | 67.7±4.89[=] | 68±4.02[=] | 67.4±5.62[=] | 68±4.02[=] | 68±4.02[=] | 67.7±4.89[=] | 67.7±4.89[=] | 68±4.02[=] | 67.7±4.89 |
DRD | 68.03±3.92[=] | 67.55±4.07[=] | 67.15±3.85[=] | 67.03±3.95[=] | 68.03±3.92[=] | 67.19±3.8[=] | 65.1±4.33[+] | 61.91±4.51[+] | 67.7±4[=] | 67.96±4.23 |
Hayes-Roth | 49.94±12.83[=] | 49.94±12.83[=] | 49.94±12.83[=] | 49.94±12.83[=] | 49.94±12.83[=] | 49.94±12.83[=] | 52±11.61[=] | 48.75±13.21[=] | 49.94±12.83[=] | 52±11.61 |
ILPD | 71.36±0.73[=] | 71.36±0.73[=] | 71.36±0.73[=] | 71.36±0.73[=] | 71.36±0.73[=] | 71.36±0.73[=] | 71.36±0.73[=] | 71.36±0.73[=] | 71.36±0.73[=] | 71.36±0.73 |
Lenses | 76.17±22.13[=] | 76.17±22.13[=] | 76.17±22.13[=] | 76.17±22.13[=] | 76.17±22.13[=] | 76.17±22.13[=] | 76.17±22.13[=] | 54.5±30.42[+] | 76.17±22.13[=] | 76.17±22.13 |
Lymphography | 81.57±10.06[=] | 80.56±9.09[=] | 81.42±10.15[=] | 84.55±8.84[=] | 82.02±10.48[=] | 79.06±10.44[+] | 81.57±10.11[=] | 80.3±9[=] | 74.25±11.45[+] | 85.5±8.3 |
CVR | 94.09±3.33[=] | 94.46±3.37[=] | 94.3±3.34[=] | 94.32±3.32[=] | 94.3±3.34[=] | 94.3±3.34[=] | 94±3.44[=] | 94.43±3.41[=] | 94.37±3.41[=] | 94.3±3.34 |
Sonar | 79.34±8.37[=] | 80.48±8.05[=] | 80.18±8.33[=] | 78.46±8.44[=] | 80.54±8.43[=] | 76.93±8.71[=] | 79.91±7.73[=] | 82.1±8.07[=] | 77.41±8.41[=] | 80.67±7.88 |
Thoracic Surgery | 85.11±0[=] | 85.11±0[=] | 85.11±0[=] | 85.11±0[=] | 85.11±0[=] | 85.11±0[=] | 85.11±0[=] | 85.11±0[=] | 85.11±0[=] | 85.11±0 |
Wilt | 97.62±0.57[+] | 97.97±0.49[=] | 97.97±0.49[=] | 97.97±0.49[=] | 97.62±0.57[+] | 97.97±0.49[=] | 94.61±0.06[+] | 97.97±0.49[=] | 97.62±0.57[+] | 97.98±0.49 |
Zoo | 95.75±5.12[=] | 93.88±6.72[=] | 89.39±5.72[+] | 89.49±5.64[+] | 89.49±5.64[+] | 94.06±6.9[=] | 95.27±5.85[=] | 93.89±6.96[=] | 90.49±7.96[+] | 95.06±6.2 |
Average | 79.34 | 79.15 | 78.79 | 78.91 | 79.00 | 78.83 | 79.16 | 77.33 | 77.51 | 80.03 |
Dataset | CMIM | CMIM3 | JMI | JMI3 | JMIM | MIGM | QPFS | Relief | WRFS | FJMIM |
Acute Inflammations | 99.5±1.99[=] | 100±0[=] | 99.5±1.99[=] | 99.5±1.99[=] | 99.5±1.99[=] | 100±0[=] | 100±0[=] | 100±0[=] | 99.67±1.64[=] | 100±0 |
Arrhythmia | 58.27±5.17[+] | 57.63±5.17[+] | 57.79±5.34[+] | 58.32±5.08[+] | 58.94±5.13[=] | 57.67±5.21[+] | 60.73±4.64[=] | 65.13±4.43[-] | 58.39±5.22[+] | 61.11±5.23 |
Blogger | 72.1±11.31[=] | 72.1±11.31[=] | 72.1±11.31[=] | 78.8±10.18[=] | 72.1±11.31[=] | 72.1±11.31[=] | 72.1±11.31[=] | 70.3±11.41[=] | 76.1±12.05[=] | 78.6±11.37 |
DRD | 64.3±4.19[=] | 62.62±5.29[=] | 63.69±4.58[=] | 61.96±4.68[=] | 64.3±4.19[=] | 63.58±4.56[=] | 60.47±4.64[+] | 63.51±4.63[=] | 64.06±4.4[=] | 64.7±4.23 |
Hayes-Roth | 59.19±10.41[=] | 59.19±10.41[=] | 59.19±10.41[=] | 59.19±10.41[=] | 59.19±10.41[=] | 59.19±10.41[=] | 63.12±11.32[=] | 57.56±10.82[=] | 59.19±10.41[=] | 63.12±11.32 |
ILPD | 67.16±5.41[=] | 68.22±5.06[=] | 67.54±5.44[=] | 68.22±5.06[=] | 67.16±5.41[=] | 67.3±5.42[=] | 68.74±5.01[=] | 67.84±5.56[=] | 66.66±5.25[=] | 69.15±5.62 |
Lenses | 86.83±21.88[=] | 86.83±21.88[=] | 86.83±21.88[=] | 86.83±21.88[=] | 86.83±21.88[=] | 86.83±21.88[=] | 86.83±21.88[=] | 54.17±30.46[+] | 86.83±21.88[=] | 86.83±21.88 |
Lymphography | 84.61±7.58[=] | 72.61±9.14[+] | 84.61±7.58[=] | 84.16±9.46[=] | 80.99±9.49[=] | 76.62±8.87[=] | 84.61±7.58[=] | 81.44±9.42[=] | 79.74±9.36[=] | 78.87±8.35 |
CVR | 93.63±3.43[=] | 94.09±3.33[=] | 95.26±3.01[=] | 95.26±3.01[=] | 95.26±3.01[=] | 95.26±3.01[=] | 94.14±3.37[=] | 93.67±3.5[=] | 94.09±2.95[=] | 95.26±3.01 |
Sonar | 85.09±7.43[=] | 83.51±7.68[=] | 87.74±7.51[=] | 85.53±8.83[=] | 82.7±9.06[=] | 82.4±7.72[+] | 86.15±7.24[=] | 87.95±6.78[=] | 84.17±7.73[=] | 87.65±7.27 |
Thoracic Surgery | 80.96±3.45[=] | 81.38±3.53[=] | 81.38±3.53[=] | 83.45±3.05[=] | 81.38±3.53[=] | 82.64±3.28[=] | 83.89±2.95[=] | 82.68±3.08[=] | 84.85±0.97[-] | 81.38±3.53 |
Wilt | 97.69±0.57[+] | 98.12±0.55[=] | 98.12±0.55[=] | 98.12±0.55[=] | 97.69±0.57[+] | 98.12±0.55[=] | 94.44±0.52[+] | 98.12±0.55[=] | 97.69±0.57[+] | 98.12±0.55 |
Zoo | 91.9±7.21[=] | 92.06±7.35[=] | 92.05±7.24[=] | 92.05±7.24[=] | 92.05±7.24[=] | 91.16±7.48[=] | 91.42±6.94[=] | 91.42±6.94[=] | 90.29±7.45[+] | 94.08±6.6 |
Average | 80.09 | 79.10 | 80.45 | 80.88 | 79.85 | 79.45 | 80.51 | 77.98 | 80.13 | 81.45 |
FS method | Average |
CMIM | 78.9 |
CMIM3 | 78.6 |
JMI | 78.9 |
JMI3 | 79.1 |
JMIM | 78.6 |
MIGM | 78.4 |
QPFS | 79.2 |
Relief | 76.9 |
WRFS | 78.1 |
FJMIM | 79.8 |
FS method | NB | SVM | KNN | Average |
CMIM | 0.702 | 0.641 | 0.704 | 0.682 |
CMIM3 | 0.742 | 0.641 | 0.705 | 0.696 |
JMI | 0.705 | 0.640 | 0.713 | 0.686 |
JMI3 | 0.712 | 0.636 | 0.723 | 0.690 |
JMIM | 0.701 | 0.642 | 0.707 | 0.683 |
MIGM | 0.701 | 0.637 | 0.708 | 0.682 |
QPFS | 0.712 | 0.636 | 0.711 | 0.686 |
Relief | 0.621 | 0.612 | 0.661 | 0.631 |
WRFS | 0.698 | 0.632 | 0.708 | 0.679 |
FJMIM | 0.748 | 0.645 | 0.743 | 0.712 |
FS method | NB | SVM | KNN | Average |
CMIM | 0.722 | 0.712 | 0.818 | 0.750 |
CMIM3 | 0.795 | 0.712 | 0.752 | 0.753 |
JMI | 0.724 | 0.712 | 0.823 | 0.753 |
JMI3 | 0.729 | 0.712 | 0.834 | 0.759 |
JMIM | 0.719 | 0.714 | 0.821 | 0.751 |
MIGM | 0.722 | 0.710 | 0.818 | 0.750 |
QPFS | 0.732 | 0.712 | 0.825 | 0.756 |
Relief | 0.632 | 0.708 | 0.688 | 0.676 |
WRFS | 0.716 | 0.701 | 0.826 | 0.748 |
FJMIM | 0.798 | 0.715 | 0.840 | 0.784 |
FS method | NB | SVM | KNN | Average |
CMIM | 0.835 | 0.733 | 0.812 | 0.793 |
CMIM3 | 0.836 | 0.748 | 0.844 | 0.809 |
JMI | 0.837 | 0.734 | 0.815 | 0.795 |
JMI3 | 0.831 | 0.729 | 0.805 | 0.788 |
JMIM | 0.836 | 0.742 | 0.812 | 0.796 |
MIGM | 0.832 | 0.754 | 0.807 | 0.798 |
QPFS | 0.838 | 0.710 | 0.807 | 0.785 |
Relief | 0.804 | 0.707 | 0.801 | 0.771 |
WRFS | 0.831 | 0.732 | 0.806 | 0.789 |
FJMIM | 0.839 | 0.748 | 0.852 | 0.813 |
FS method | NB | SVM | KNN | Average |
CMIM | 0.848 | 0.708 | 0.798 | 0.785 |
CMIM3 | 0.862 | 0.715 | 0.862 | 0.813 |
JMI | 0.850 | 0.708 | 0.797 | 0.785 |
JMI3 | 0.837 | 0.705 | 0.800 | 0.781 |
JMIM | 0.852 | 0.713 | 0.797 | 0.787 |
MIGM | 0.857 | 0.724 | 0.827 | 0.803 |
QPFS | 0.857 | 0.708 | 0.797 | 0.787 |
Relief | 0.842 | 0.685 | 0.791 | 0.772 |
WRFS | 0.850 | 0.697 | 0.795 | 0.781 |
FJMIM | 0.862 | 0.718 | 0.869 | 0.816 |
FS method | Stability |
CMIM | 72.6 |
CMIM3 | 67.3 |
JMI | 81.2 |
JMI3 | 75.2 |
JMIM | 75.9 |
MIGM | 66.2 |
QPFS | 71.7 |
Relief | 44.3 |
WRFS | 66.6 |
FJMIM | 87.8 |
No. | Datasets | Instances | Features | Classes |
1 | Acute Inflammations | 120 | 6 | 2 |
2 | Arrhythmia | 452 | 279 | 13 |
3 | Blogger | 100 | 6 | 2 |
4 | Diabetic Retinopathy Debrecen (DRD) | 1151 | 20 | 2 |
5 | Hayes-Roth | 160 | 5 | 3 |
6 | Indian Liver Patient Dataset (ILPD) | 583 | 10 | 2 |
7 | Lenses | 24 | 4 | 3 |
8 | Lymphography | 148 | 18 | 4 |
9 | Congressional Voting Records (CVR) | 435 | 16 | 2 |
10 | Sonar | 208 | 60 | 2 |
11 | Thoracic Surgery | 470 | 17 | 2 |
12 | Wilt | 4889 | 6 | 2 |
13 | Zoo | 101 | 17 | 7 |
Dataset | CMIM | CMIM3 | JMI | JMI3 | JMIM | MIGM | QPFS | Relief | WRFS | FJMIM |
Acute Inflammations | 100±0[=] | 100±0[=] | 100±0[=] | 100±0[=] | 100±0[=] | 99.75±2.5[=] | 99.75±2.5[=] | 100±0[=] | 90.83±8.5[+] | 100±0 |
Arrhythmia | 60.64±7.01[=] | 59.22±6.17[=] | 59.42±6.49[=] | 59.58±6.58[=] | 60.62±6.93[=] | 58.98±6.39[=] | 63.7±6.57[-] | 64.94±6.33[-] | 59.31±6.3[=] | 59.74±6.43 |
Blogger | 61.9±10.7[=] | 61.9±10.7[=] | 61.9±10.7[=] | 64.9±10.2[=] | 61.9±10.7[=] | 61.9±10.7[=] | 61.9±10.7[=] | 65.4±7.84[=] | 66.1±9.09[=] | 65.9±10.26 |
DRD | 57.59±4.95[=] | 60.66±4.69[-] | 60.3±5.04[-] | 60.6±4.58[-] | 57.59±4.95[=] | 60.3±5.04[-] | 61.19±4.49[-] | 60.61±5.06[-] | 57.47±4.98[=] | 57.56±4.83 |
Hayes-Roth | 45.81±10.77[=] | 45.81±10.77[=] | 45.81±10.77[=] | 45.81±10.77[=] | 45.81±10.77[=] | 45.81±10.77[=] | 49.37±10.53[=] | 46.31±11.48[=] | 45.81±10.77[=] | 49.37±10.53 |
ILPD | 68.99±5.68[=] | 67.33±5.87[=] | 68.99±5.68[=] | 67.33±5.87[=] | 68.99±5.68[=] | 68.99±5.68[=] | 68.73±5.64[=] | 64.96±6.45[+] | 70.11±6.74[=] | 69.54±5.75 |
Lenses | 86.83±21.88[=] | 86.83±21.88[=] | 86.83±21.88[=] | 86.83±21.88[=] | 86.83±21.88[=] | 86.83±21.88[=] | 86.83±21.88[=] | 54.83±30.73[+] | 86.83±21.88[=] | 86.83±21.88 |
Lymphography | 79.02±10.57[=] | 82.05±9.59[=] | 79.02±10.57[=] | 77.93±10.87[=] | 78.27±10.98[=] | 77.25±11.38[=] | 79.02±10.57[=] | 82.61±9.77[=] | 76.91±11.64[+] | 81.5±10.29 |
CVR | 94.3±3.15[=] | 94.32±3.12[=] | 93.75±3.57[=] | 93.75±3.57[=] | 93.75±3.57[=] | 93.75±3.57[=] | 94.32±3.26[=] | 93.82±3.28[=] | 93.52±3.18[=] | 93.75±3.57 |
Sonar | 75.05±8.33[=] | 75.42±9.08[=] | 75.75±8.85[=] | 76.23±9.99[=] | 72.2±9.93[=] | 72.14±9.54[+] | 76.42±8.69[=] | 74.25±8.33[=] | 77.91±8.74[=] | 77±8.98 |
Thoracic Surgery | 83.83±3.1[=] | 83.38±3.09[=] | 83.38±3.09[=] | 83.85±2.24[=] | 83.38±3.09[=] | 84.64±1.83[=] | 83.55±3.08[=] | 83.06±2.77[=] | 83.85±2.74[=] | 83.38±3.09 |
Wilt | 94.61±0.06[=] | 94.61±0.06[=] | 94.61±0.06[=] | 94.61±0.06[=] | 94.61±0.06[=] | 94.61±0.06[=] | 94.61±0.06[=] | 94.61±0.06[=] | 94.61±0.06[=] | 94.61±0.06 |
Zoo | 95.15±5.74[=] | 96.05±5.6[=] | 96.03±5.66[=] | 96.03±5.66[=] | 96.03±5.66[=] | 96.93±5.42[=] | 94.08±6.6[=] | 94.08±6.6[=] | 92.96±7.05[=] | 95.05±5.87 |
Average | 77.21 | 77.51 | 77.37 | 77.50 | 76.92 | 77.07 | 77.96 | 75.34 | 76.63 | 78.02 |
Dataset | CMIM | CMIM3 | JMI | JMI3 | JMIM | MIGM | QPFS | Relief | WRFS | FJMIM |
Acute Inflammations | 98.33±3.35[=] | 99.67±1.64[=] | 98.33±3.35[=] | 98.33±3.35[=] | 98.33±3.35[=] | 99.42±2.44[=] | 99.42±2.44[=] | 100±0[+] | 89.92±9.5[+] | 99.42±2.44 |
Arrhythmia | 66.09±6.81[=] | 64.08±6.11[=] | 65.01±5.96[=] | 65.7±6.23[=] | 66.14±6.61[=] | 65.23±6.39[=] | 66.82±6.2[=] | 67.22±6.05[=] | 65.23±6.15[=] | 67.11±6.7 |
Blogger | 68±4.02[=] | 67.7±4.89[=] | 68±4.02[=] | 67.4±5.62[=] | 68±4.02[=] | 68±4.02[=] | 67.7±4.89[=] | 67.7±4.89[=] | 68±4.02[=] | 67.7±4.89 |
DRD | 68.03±3.92[=] | 67.55±4.07[=] | 67.15±3.85[=] | 67.03±3.95[=] | 68.03±3.92[=] | 67.19±3.8[=] | 65.1±4.33[+] | 61.91±4.51[+] | 67.7±4[=] | 67.96±4.23 |
Hayes-Roth | 49.94±12.83[=] | 49.94±12.83[=] | 49.94±12.83[=] | 49.94±12.83[=] | 49.94±12.83[=] | 49.94±12.83[=] | 52±11.61[=] | 48.75±13.21[=] | 49.94±12.83[=] | 52±11.61 |
ILPD | 71.36±0.73[=] | 71.36±0.73[=] | 71.36±0.73[=] | 71.36±0.73[=] | 71.36±0.73[=] | 71.36±0.73[=] | 71.36±0.73[=] | 71.36±0.73[=] | 71.36±0.73[=] | 71.36±0.73 |
Lenses | 76.17±22.13[=] | 76.17±22.13[=] | 76.17±22.13[=] | 76.17±22.13[=] | 76.17±22.13[=] | 76.17±22.13[=] | 76.17±22.13[=] | 54.5±30.42[+] | 76.17±22.13[=] | 76.17±22.13 |
Lymphography | 81.57±10.06[=] | 80.56±9.09[=] | 81.42±10.15[=] | 84.55±8.84[=] | 82.02±10.48[=] | 79.06±10.44[+] | 81.57±10.11[=] | 80.3±9[=] | 74.25±11.45[+] | 85.5±8.3 |
CVR | 94.09±3.33[=] | 94.46±3.37[=] | 94.3±3.34[=] | 94.32±3.32[=] | 94.3±3.34[=] | 94.3±3.34[=] | 94±3.44[=] | 94.43±3.41[=] | 94.37±3.41[=] | 94.3±3.34 |
Sonar | 79.34±8.37[=] | 80.48±8.05[=] | 80.18±8.33[=] | 78.46±8.44[=] | 80.54±8.43[=] | 76.93±8.71[=] | 79.91±7.73[=] | 82.1±8.07[=] | 77.41±8.41[=] | 80.67±7.88 |
Thoracic Surgery | 85.11±0[=] | 85.11±0[=] | 85.11±0[=] | 85.11±0[=] | 85.11±0[=] | 85.11±0[=] | 85.11±0[=] | 85.11±0[=] | 85.11±0[=] | 85.11±0 |
Wilt | 97.62±0.57[+] | 97.97±0.49[=] | 97.97±0.49[=] | 97.97±0.49[=] | 97.62±0.57[+] | 97.97±0.49[=] | 94.61±0.06[+] | 97.97±0.49[=] | 97.62±0.57[+] | 97.98±0.49 |
Zoo | 95.75±5.12[=] | 93.88±6.72[=] | 89.39±5.72[+] | 89.49±5.64[+] | 89.49±5.64[+] | 94.06±6.9[=] | 95.27±5.85[=] | 93.89±6.96[=] | 90.49±7.96[+] | 95.06±6.2 |
Average | 79.34 | 79.15 | 78.79 | 78.91 | 79.00 | 78.83 | 79.16 | 77.33 | 77.51 | 80.03 |
Dataset | CMIM | CMIM3 | JMI | JMI3 | JMIM | MIGM | QPFS | Relief | WRFS | FJMIM |
Acute Inflammations | 99.5±1.99[=] | 100±0[=] | 99.5±1.99[=] | 99.5±1.99[=] | 99.5±1.99[=] | 100±0[=] | 100±0[=] | 100±0[=] | 99.67±1.64[=] | 100±0 |
Arrhythmia | 58.27±5.17[+] | 57.63±5.17[+] | 57.79±5.34[+] | 58.32±5.08[+] | 58.94±5.13[=] | 57.67±5.21[+] | 60.73±4.64[=] | 65.13±4.43[-] | 58.39±5.22[+] | 61.11±5.23 |
Blogger | 72.1±11.31[=] | 72.1±11.31[=] | 72.1±11.31[=] | 78.8±10.18[=] | 72.1±11.31[=] | 72.1±11.31[=] | 72.1±11.31[=] | 70.3±11.41[=] | 76.1±12.05[=] | 78.6±11.37 |
DRD | 64.3±4.19[=] | 62.62±5.29[=] | 63.69±4.58[=] | 61.96±4.68[=] | 64.3±4.19[=] | 63.58±4.56[=] | 60.47±4.64[+] | 63.51±4.63[=] | 64.06±4.4[=] | 64.7±4.23 |
Hayes-Roth | 59.19±10.41[=] | 59.19±10.41[=] | 59.19±10.41[=] | 59.19±10.41[=] | 59.19±10.41[=] | 59.19±10.41[=] | 63.12±11.32[=] | 57.56±10.82[=] | 59.19±10.41[=] | 63.12±11.32 |
ILPD | 67.16±5.41[=] | 68.22±5.06[=] | 67.54±5.44[=] | 68.22±5.06[=] | 67.16±5.41[=] | 67.3±5.42[=] | 68.74±5.01[=] | 67.84±5.56[=] | 66.66±5.25[=] | 69.15±5.62 |
Lenses | 86.83±21.88[=] | 86.83±21.88[=] | 86.83±21.88[=] | 86.83±21.88[=] | 86.83±21.88[=] | 86.83±21.88[=] | 86.83±21.88[=] | 54.17±30.46[+] | 86.83±21.88[=] | 86.83±21.88 |
Lymphography | 84.61±7.58[=] | 72.61±9.14[+] | 84.61±7.58[=] | 84.16±9.46[=] | 80.99±9.49[=] | 76.62±8.87[=] | 84.61±7.58[=] | 81.44±9.42[=] | 79.74±9.36[=] | 78.87±8.35 |
CVR | 93.63±3.43[=] | 94.09±3.33[=] | 95.26±3.01[=] | 95.26±3.01[=] | 95.26±3.01[=] | 95.26±3.01[=] | 94.14±3.37[=] | 93.67±3.5[=] | 94.09±2.95[=] | 95.26±3.01 |
Sonar | 85.09±7.43[=] | 83.51±7.68[=] | 87.74±7.51[=] | 85.53±8.83[=] | 82.7±9.06[=] | 82.4±7.72[+] | 86.15±7.24[=] | 87.95±6.78[=] | 84.17±7.73[=] | 87.65±7.27 |
Thoracic Surgery | 80.96±3.45[=] | 81.38±3.53[=] | 81.38±3.53[=] | 83.45±3.05[=] | 81.38±3.53[=] | 82.64±3.28[=] | 83.89±2.95[=] | 82.68±3.08[=] | 84.85±0.97[-] | 81.38±3.53 |
Wilt | 97.69±0.57[+] | 98.12±0.55[=] | 98.12±0.55[=] | 98.12±0.55[=] | 97.69±0.57[+] | 98.12±0.55[=] | 94.44±0.52[+] | 98.12±0.55[=] | 97.69±0.57[+] | 98.12±0.55 |
Zoo | 91.9±7.21[=] | 92.06±7.35[=] | 92.05±7.24[=] | 92.05±7.24[=] | 92.05±7.24[=] | 91.16±7.48[=] | 91.42±6.94[=] | 91.42±6.94[=] | 90.29±7.45[+] | 94.08±6.6 |
Average | 80.09 | 79.10 | 80.45 | 80.88 | 79.85 | 79.45 | 80.51 | 77.98 | 80.13 | 81.45 |
FS method | Average |
CMIM | 78.9 |
CMIM3 | 78.6 |
JMI | 78.9 |
JMI3 | 79.1 |
JMIM | 78.6 |
MIGM | 78.4 |
QPFS | 79.2 |
Relief | 76.9 |
WRFS | 78.1 |
FJMIM | 79.8 |
FS method | NB | SVM | KNN | Average |
CMIM | 0.702 | 0.641 | 0.704 | 0.682 |
CMIM3 | 0.742 | 0.641 | 0.705 | 0.696 |
JMI | 0.705 | 0.640 | 0.713 | 0.686 |
JMI3 | 0.712 | 0.636 | 0.723 | 0.690 |
JMIM | 0.701 | 0.642 | 0.707 | 0.683 |
MIGM | 0.701 | 0.637 | 0.708 | 0.682 |
QPFS | 0.712 | 0.636 | 0.711 | 0.686 |
Relief | 0.621 | 0.612 | 0.661 | 0.631 |
WRFS | 0.698 | 0.632 | 0.708 | 0.679 |
FJMIM | 0.748 | 0.645 | 0.743 | 0.712 |
FS method | NB | SVM | KNN | Average |
CMIM | 0.722 | 0.712 | 0.818 | 0.750 |
CMIM3 | 0.795 | 0.712 | 0.752 | 0.753 |
JMI | 0.724 | 0.712 | 0.823 | 0.753 |
JMI3 | 0.729 | 0.712 | 0.834 | 0.759 |
JMIM | 0.719 | 0.714 | 0.821 | 0.751 |
MIGM | 0.722 | 0.710 | 0.818 | 0.750 |
QPFS | 0.732 | 0.712 | 0.825 | 0.756 |
Relief | 0.632 | 0.708 | 0.688 | 0.676 |
WRFS | 0.716 | 0.701 | 0.826 | 0.748 |
FJMIM | 0.798 | 0.715 | 0.840 | 0.784 |
FS method | NB | SVM | KNN | Average |
CMIM | 0.835 | 0.733 | 0.812 | 0.793 |
CMIM3 | 0.836 | 0.748 | 0.844 | 0.809 |
JMI | 0.837 | 0.734 | 0.815 | 0.795 |
JMI3 | 0.831 | 0.729 | 0.805 | 0.788 |
JMIM | 0.836 | 0.742 | 0.812 | 0.796 |
MIGM | 0.832 | 0.754 | 0.807 | 0.798 |
QPFS | 0.838 | 0.710 | 0.807 | 0.785 |
Relief | 0.804 | 0.707 | 0.801 | 0.771 |
WRFS | 0.831 | 0.732 | 0.806 | 0.789 |
FJMIM | 0.839 | 0.748 | 0.852 | 0.813 |
FS method | NB | SVM | KNN | Average |
CMIM | 0.848 | 0.708 | 0.798 | 0.785 |
CMIM3 | 0.862 | 0.715 | 0.862 | 0.813 |
JMI | 0.850 | 0.708 | 0.797 | 0.785 |
JMI3 | 0.837 | 0.705 | 0.800 | 0.781 |
JMIM | 0.852 | 0.713 | 0.797 | 0.787 |
MIGM | 0.857 | 0.724 | 0.827 | 0.803 |
QPFS | 0.857 | 0.708 | 0.797 | 0.787 |
Relief | 0.842 | 0.685 | 0.791 | 0.772 |
WRFS | 0.850 | 0.697 | 0.795 | 0.781 |
FJMIM | 0.862 | 0.718 | 0.869 | 0.816 |
FS method | Stability |
CMIM | 72.6 |
CMIM3 | 67.3 |
JMI | 81.2 |
JMI3 | 75.2 |
JMIM | 75.9 |
MIGM | 66.2 |
QPFS | 71.7 |
Relief | 44.3 |
WRFS | 66.6 |
FJMIM | 87.8 |