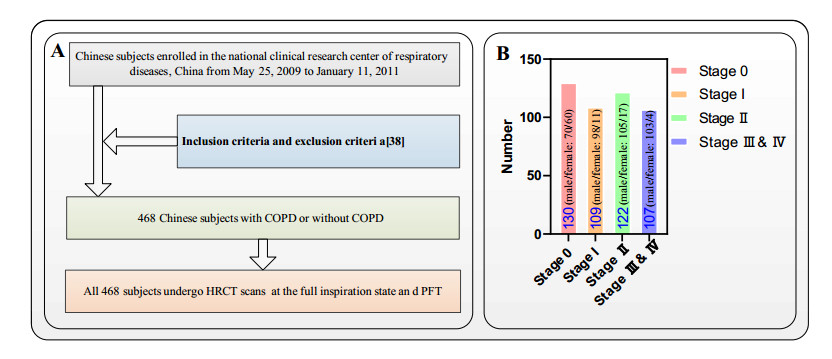
Computed tomography (CT) has been the most effective modality for characterizing and quantifying chronic obstructive pulmonary disease (COPD). Radiomics features extracted from the region of interest in chest CT images have been widely used for lung diseases, but they have not yet been extensively investigated for COPD. Therefore, it is necessary to understand COPD from the lung radiomics features and apply them for COPD diagnostic applications, such as COPD stage classification. Lung radiomics features are used for characterizing and classifying the COPD stage in this paper. First, 19 lung radiomics features are selected from 1316 lung radiomics features per subject by using Lasso. Second, the best performance classifier (multi-layer perceptron classifier, MLP classifier) is determined. Third, two lung radiomics combination features, Radiomics-FIRST and Radiomics-ALL, are constructed based on 19 selected lung radiomics features by using the proposed lung radiomics combination strategy for characterizing the COPD stage. Lastly, the 19 selected lung radiomics features with Radiomics-FIRST/Radiomics-ALL are used to classify the COPD stage based on the best performance classifier. The results show that the classification ability of lung radiomics features based on machine learning (ML) methods is better than that of the chest high-resolution CT (HRCT) images based on classic convolutional neural networks (CNNs). In addition, the classifier performance of the 19 lung radiomics features selected by Lasso is better than that of the 1316 lung radiomics features. The accuracy, precision, recall, F1-score and AUC of the MLP classifier with the 19 selected lung radiomics features and Radiomics-ALL were 0.83, 0.83, 0.83, 0.82 and 0.95, respectively. It is concluded that, for the chest HRCT images, compared to the classic CNN, the ML methods based on lung radiomics features are more suitable and interpretable for COPD classification. In addition, the proposed lung radiomics combination strategy for characterizing the COPD stage effectively improves the classifier performance by 12% overall (accuracy: 3%, precision: 3%, recall: 3%, F1-score: 2% and AUC: 1%).
Citation: Yingjian Yang, Wei Li, Yingwei Guo, Nanrong Zeng, Shicong Wang, Ziran Chen, Yang Liu, Huai Chen, Wenxin Duan, Xian Li, Wei Zhao, Rongchang Chen, Yan Kang. Lung radiomics features for characterizing and classifying COPD stage based on feature combination strategy and multi-layer perceptron classifier[J]. Mathematical Biosciences and Engineering, 2022, 19(8): 7826-7855. doi: 10.3934/mbe.2022366
[1] | Yingjian Yang, Wei Li, Yan Kang, Yingwei Guo, Kai Yang, Qiang Li, Yang Liu, Chaoran Yang, Rongchang Chen, Huai Chen, Xian Li, Lei Cheng . A novel lung radiomics feature for characterizing resting heart rate and COPD stage evolution based on radiomics feature combination strategy. Mathematical Biosciences and Engineering, 2022, 19(4): 4145-4165. doi: 10.3934/mbe.2022191 |
[2] | Qian Shao, Rongrong Xuan, Yutao Wang, Jian Xu, Menglin Ouyang, Caoqian Yin, Wei Jin . Deep learning and radiomics analysis for prediction of placenta invasion based on T2WI. Mathematical Biosciences and Engineering, 2021, 18(5): 6198-6215. doi: 10.3934/mbe.2021310 |
[3] | Tao Zhang, Hao Zhang, Ran Wang, Yunda Wu . A new JPEG image steganalysis technique combining rich model features and convolutional neural networks. Mathematical Biosciences and Engineering, 2019, 16(5): 4069-4081. doi: 10.3934/mbe.2019201 |
[4] | Yutao Wang, Qian Shao, Shuying Luo, Randi Fu . Development of a nomograph integrating radiomics and deep features based on MRI to predict the prognosis of high grade Gliomas. Mathematical Biosciences and Engineering, 2021, 18(6): 8084-8095. doi: 10.3934/mbe.2021401 |
[5] | Binju Saju, Neethu Tressa, Rajesh Kumar Dhanaraj, Sumegh Tharewal, Jincy Chundamannil Mathew, Danilo Pelusi . Effective multi-class lungdisease classification using the hybridfeature engineering mechanism. Mathematical Biosciences and Engineering, 2023, 20(11): 20245-20273. doi: 10.3934/mbe.2023896 |
[6] | Zhigao Zeng, Cheng Huang, Wenqiu Zhu, Zhiqiang Wen, Xinpan Yuan . Flower image classification based on an improved lightweight neural network with multi-scale feature fusion and attention mechanism. Mathematical Biosciences and Engineering, 2023, 20(8): 13900-13920. doi: 10.3934/mbe.2023619 |
[7] | Chunmei He, Hongyu Kang, Tong Yao, Xiaorui Li . An effective classifier based on convolutional neural network and regularized extreme learning machine. Mathematical Biosciences and Engineering, 2019, 16(6): 8309-8321. doi: 10.3934/mbe.2019420 |
[8] | Peng An, Xiumei Li, Ping Qin, YingJian Ye, Junyan Zhang, Hongyan Guo, Peng Duan, Zhibing He, Ping Song, Mingqun Li, Jinsong Wang, Yan Hu, Guoyan Feng, Yong Lin . Predicting model of mild and severe types of COVID-19 patients using Thymus CT radiomics model: A preliminary study. Mathematical Biosciences and Engineering, 2023, 20(4): 6612-6629. doi: 10.3934/mbe.2023284 |
[9] | Xiaochen Liu, Weidong He, Yinghui Zhang, Shixuan Yao, Ze Cui . Effect of dual-convolutional neural network model fusion for Aluminum profile surface defects classification and recognition. Mathematical Biosciences and Engineering, 2022, 19(1): 997-1025. doi: 10.3934/mbe.2022046 |
[10] | Jingren Niu, Qing Tan, Xiufen Zou, Suoqin Jin . Accurate prediction of glioma grades from radiomics using a multi-filter and multi-objective-based method. Mathematical Biosciences and Engineering, 2023, 20(2): 2890-2907. doi: 10.3934/mbe.2023136 |
Computed tomography (CT) has been the most effective modality for characterizing and quantifying chronic obstructive pulmonary disease (COPD). Radiomics features extracted from the region of interest in chest CT images have been widely used for lung diseases, but they have not yet been extensively investigated for COPD. Therefore, it is necessary to understand COPD from the lung radiomics features and apply them for COPD diagnostic applications, such as COPD stage classification. Lung radiomics features are used for characterizing and classifying the COPD stage in this paper. First, 19 lung radiomics features are selected from 1316 lung radiomics features per subject by using Lasso. Second, the best performance classifier (multi-layer perceptron classifier, MLP classifier) is determined. Third, two lung radiomics combination features, Radiomics-FIRST and Radiomics-ALL, are constructed based on 19 selected lung radiomics features by using the proposed lung radiomics combination strategy for characterizing the COPD stage. Lastly, the 19 selected lung radiomics features with Radiomics-FIRST/Radiomics-ALL are used to classify the COPD stage based on the best performance classifier. The results show that the classification ability of lung radiomics features based on machine learning (ML) methods is better than that of the chest high-resolution CT (HRCT) images based on classic convolutional neural networks (CNNs). In addition, the classifier performance of the 19 lung radiomics features selected by Lasso is better than that of the 1316 lung radiomics features. The accuracy, precision, recall, F1-score and AUC of the MLP classifier with the 19 selected lung radiomics features and Radiomics-ALL were 0.83, 0.83, 0.83, 0.82 and 0.95, respectively. It is concluded that, for the chest HRCT images, compared to the classic CNN, the ML methods based on lung radiomics features are more suitable and interpretable for COPD classification. In addition, the proposed lung radiomics combination strategy for characterizing the COPD stage effectively improves the classifier performance by 12% overall (accuracy: 3%, precision: 3%, recall: 3%, F1-score: 2% and AUC: 1%).
Chronic obstructive pulmonary disease (COPD) is a heterogeneous inflammatory disease [1] characterized by persistent airflow limitations [2]. Due to this characteristic, the gold standard for diagnosing and evaluating COPD is the pulmonary function test (PFT) [2], which yields the forced expiratory volume in 1 second per forced vital capacity (FEV1/FVC) and FEV1 percentage of predicted (FEV1 % predicted). COPD's primary anatomical and pathophysiological manifestations are small airway lesions and emphysema [1,3]. Although PFTs can explain the impact on the symptoms and quality of life of COPD patients [4,5], it cannot reflect the change of the lung tissue in COPD patients according to the COPD stage evolution. PFT changes only occur when lung tissue is destroyed to a certain extent. Therefore, it is also difficult for the PFT to identify the etiology of COPD.
Compared with PFTs, computed tomography (CT) has been regarded as the most effective modality for characterizing and quantifying COPD [6]. For example, chest CT images can indicate that the patients have suffered from mild lobular central emphysema and reveal decreased exercise tolerance in smokers without airflow limitations in their PFT results [7]. In addition, the chest CT images have also been used to quantitatively analyze the bronchial [8,9], airway disease [10,11,12,13,14,15], emphysema [16] and vascular [17,18] problems in COPD patients by measuring the parameters of the bronchi and vasculature, or by using the analysis methods for airway disease and emphysema. Furthermore, since radiomics was proposed to mine more information from medical images by using advanced feature analysis in 2007 [19], it has been widely used for the analysis of lung disease images [20,21,22,23,24] and other diseases [25,26]. Unlike normal lungs, the lung texture and density of COPD patients are influenced by the increased air abundance [20], leading to changes in chest CT images. The radiomics features, which reflect lung texture and density changes, can also predict severe COPD exacerbations [27]. They have also been applied to the spirometric assessment of emphysema presence and COPD severity [28]. However, radiomics has not been extensively investigated in COPD yet. Currently, there are potential applications of radiomics features in COPD, particularly for the diagnosis, treatment and follow-up of COPD and future directions of radiomics features in COPD [29]. Due to the characteristics of COPD, an important reason limiting the development of radiomics in COPD is its diffuse distribution in the lungs. Therefore, it is challenging to segment the COPD regions. Especially, because of the limitations of CT resolution, small airways (diameter < 2 mm) and their associated vessels cannot be segmented from chest CT images. However, COPD results from the joint action of the lung parenchyma. Therefore, the lung radiomics features calculated from the lung parenchyma images are considered in this paper.
Most scholars are committed to improving the classifiers to get better classification results [30,31]. However, they ignore the effect of the input features on classification. Therefore, it is necessary to construct the lung radiomics combination features that characterize the COPD stage to improve the classification performance of the existing classifier. COPD and its heart complications (such as a higher resting heart rate) have been studied by using the lung radiomics features [32,33,34,35,36,37], but the lung radiomics features have not been applied for COPD stage classification.
Our contributions in this paper are briefly described as follows: 1) Lung radiomics features are applied to COPD stage classification. The best accuracy, precision, recall, F1-score and area under the curve (AUC) of the multi-layer perceptron (MLP) classifier with the 19 lung radiomics features selected by Lasso were 0.80, 0.80, 0.80, 0.80 and 0.94, respectively. 2) Two lung radiomics combination features, Radiomics-FIRST and Radiomics-ALL, were constructed to characterize COPD stage evolution. Radiomics-FIRST or Radiomics-ALL improves the performance of the MLP classifier. The accuracy, precision, recall, F1-score and AUC of the MLP classifier with the 19 selected lung radiomics features and Radiomics-ALL improved by 3, 3, 3, 2 and 1%, respectively. 3) Compared to the classic convolutional neural network (CNN) application to chest high-resolution CT (HRCT) images, the machine learning (ML) methods based on lung radiomics features are more suitable and interpretable for the COPD classification.
The subjects were Chinese people aged 40 to 79 who were enrolled in the National Clinical Research Center of Respiratory Diseases in China from May 25, 2009 to January 11, 2011. The enrolled subjects rigorously followed this study's inclusion and exclusion criteria [38]. The 468 subjects underwent chest HRCT scans at the full inspiration state and PFTs. The COPD stage was diagnosed from Stage 0 to Ⅳ by using the PFT, and according to the Global Initiative for Chronic Obstructive Lung Disease (GOLD) 2008. COPD Stage 0 is diagnosed without COPD according to GOLD, but it may involve some symptoms of respiratory diseases. Please refer to our previous study [36] for a more detailed description of the materials.
The ethics committee of the National Clinical Research Center of Respiratory Diseases at Guangzhou Medical University in China has approved this study. All 468 subjects submitted written informed consent to the First Affiliated Hospital of Guangzhou Medical University before the chest HRCT scans and PFTs were performed.
Our proposed method classifies the COPD stage by applying lung radiomics features selected by Lasso and lung radiomics combination features (characterizing the COPD stage evolution) based on best-performance ML methods. Figure 2 shows the overall block diagram of this study. We further describe our methods in the following sections, i.e., Section 2.2.1 (ROI segmentation), Section 2.2.2 (Lung radiomics feature calculation) and Section 2.2.3 (COPD stage classification).
Like the previous radiomics feature analysis methods, we also need to segment the region of interest (ROI) in the chest HRCT images and calculate the lung radiomics features based on the ROI. We finally use the lung radiomics features to characterize and classify the COPD stage. Due to the COPD characteristics of diffuse distribution in the lungs, it is challenging to segment the COPD regions. In addition, CT resolution also limits the segmentation of small airways (diameter < 2 mm) and their associated vessels. However, COPD results from the joint action of the lung parenchyma. Therefore, lung parenchyma images segmented from chest HRCT images, such as the ROI, were used to calculate the lung radiomics features in this paper.
A trained ResU-Net [39] was used to automatically segment the lung region from the chest HRCT images. The lung region includes both the right and left lungs in this study. The architecture of the ResU-Net has been described in detail in our previous paper [40]. In addition, three experienced radiologists have checked and modified all of the lung region segmentation images to ensure the accuracy of the segmentation images. Please refer to our previous study [36] for a more detailed description of the ROI segmentation process.
The 468 sets of original lung parenchyma images were extracted from the chest HRCT images using our previous method [41]. Figure 3 shows the typical parenchyma images with the Hounsfield unit (HU) value in the transverse plane. PyRadiomics [42] with the predefined class of radiomics features was implemented to calculate the lung radiomics features based on the original and derived lung parenchyma images. Finally, 1316 lung radiomics features per subject were obtained. Please also refer to our previous study [36] for a more detailed description of the lung radiomics feature calculation.
Lung radiomics features are the input features of the ML methods to classify the COPD stage. Before the COPD stage classification, the least absolute shrinkage and selection operator (Lasso) [43,44] selects the lung radiomics features by establishing the relationship between the lung radiomics features and COPD stages. Then, the selected lung radiomics features are used to pick up the best classifier from the different preset ML methods shown in Figure 3(C). This paper also introduces a radiomics combination strategy to construct the lung radiomics combination features for characterizing COPD stage evolution. Finally, the lung radiomics combination feature characterizing the COPD stage evolution is used to improve the performance of the best classifier.
First, 19 lung radiomics features per subject were selected by Lasso from 1316 lung radiomics features. COPD Stages Ⅲ and Ⅳ were considered as one COPD stage to balance each COPD stage. The mathematical expression (Eq (1)) of the Lasso model [37] is
argmin{n∑i=1(y∗i−β0−p∑j=1βjx∗ij)2+λp∑j=0|βj|} | (1) |
where x∗ij is the value of the independent variable (1316 lung radiomics features) after a normalization operation, y∗i is the value of the dependent variable (COPD Stages 0, Ⅰ, Ⅱ and Ⅲ & Ⅳ), λ is the penalty parameter (λ ≥0), βj is the regression coefficient, i∈[1, n] and j∈[0, p].
The lung radiomics features of the four COPD stages are normalized by Eq (2).
x∗ij=(xij−¯xj)/(xjmax−xjmin) | (2) |
where i = 1~468 (468 subjects), j = 1~1316 (1316 kinds of lung radiomics features for each subject), Xij is the ith row and jth column of the 468 × 1316 lung radiomics features and ¯xj, xjmax and Xjmin are the mean, maximum and minimum of each kind of lung radiomics feature xj, respectively.
Second, the best-performance classifier, i.e., the MLP classifier, is determined from ML classifiers, such as Random Forest (RF) [45], Adaboost (Ada) [46], Gradient boosting (GB) [47], Multi-layer perceptron (MLP) [48], Linear discriminant analysis (LDA) [49], and Support vector machine (SVM) [50] classifiers, as shown in Figure 2(C). The 468 subjects with the selected lung radiomics features were divided into 70 and 30%. The data for 70% of subjects trained the ML classifiers. Then, the data for 30% of the subjects were used to validate or test the trained ML classifiers. Of course, the labels for the 468 subjects were COPD stages (i.e., 0, 1, 2 and 3).
The evaluation metrics for the classifiers were set as the accuracy, precision, recall, F1-score and AUC (a performance measurement for classification). The AUC evaluation metric was calculated based on the receiver operating characteristic (ROC) curve. The ROC curve, accuracy, precision, recall and F1-score can be calculated and drawn by the confusion matrix, which shows the distribution of the predicted and true labels (the COPD stages). A standard Python package "classification_report" was used to calculate the accuracy, precision, recall and F1-score. The AUC is usually the evaluation metric of binary classification.
Figure 4 shows the confusion matrix and a schematic diagram of the ROC curve drawing for multi-classification. Figure 4(A) shows the confusion matrix of the binary classification. The true positive (TP) and false positive (FP) respectively represent the positive and negative samples predicted to be positive by the classifier. The false negative (FN) and true negative (TN) respectively represent the positive and negative samples predicted to be negative by the classifier. Like Figure 4(A), 4(B) shows that T00–T33 on the diagonal represents the correct classification results, and F represents the wrong classification results. Figure 4(C) shows a schematic diagram of the ROC curve drawing for multi-classification. The test set's COPD stages (the true and predicted label) are encoded by 0's and 1's. The position of 1 represents its classification. For example, the COPD stages 0, Ⅰ(1), Ⅱ(2) and Ⅲ & Ⅳ(3) are encoded as 1000, 0100, 0010 and 0001, respectively. Suppose the predicted label (classification result) is correct. In that case, the position value corresponding to 1 in the probability matrix P generated from the classifier is greater than the probability value of the position corresponding to 0. The coded COPD stages and their probability matrix P were used to draw the ROC curve according to the binary classification method.
Third, a radiomics combination strategy was proposed to construct the lung radiomics combination features used to characterize the COPD stage evolution. The lung radiomics combination features can be constructed using the class of the selected radiomics features. Eq (3) is the mathematical form of the lung radiomics combination strategy:
Radiomics−X=N∑i=1β2x2=β1x1+β2x2+⋯+βNxN | (3) |
where N is the number of the selected lung radiomics features in each class, and βi is the coefficient of the selected lung radiomics features xi generated by Lasso.
The lung radiomics combination features are named Radiomics-X. The symbol "X" in Radiomics-X is the class name of the selected lung radiomics features, such as FIRST, SHAPE, GLCM, GLRLM, GLSZM, NGTDM and GLDM in Figure 2(B). In particular, Radiomics-ALL is constructed by using all selected lung radiomics features and their coefficients generated by Lasso. Finally, Radiomics-FIRST and Radiomics-ALL were picked up from the lung radiomics combination features (P-value < 0.05 between any two COPD stages) to characterize the COPD stage.
Lastly, the 19 selected lung radiomics features with Radiomics-FIRST/Radiomics-ALL were used to train and validate the MLP classifier to improve the performance of the MLP classifier.
Figure 5 presents the experimental design of this study to show the lung radiomics features' classification ability, highlight Lasso's role in classification and prove the proposed lung radiomics combination strategy's effectiveness in improving the performance of the MLP classifier.
First, to compare the classification abilities of CNNs derived from chest HRCT image results and ML methods based on the lung radiomics features, we adopted two classic CNNs, DenseNet and GoogleNet, which achieved the best classification performance in our previous study [51]. The input images of DenseNet (2D/3D) and GoogleNet (2D/3D) were the original chest HRCT images or original parenchyma images, respectively. To obtain good classification performance based on the chest HRCT image results, before inputting the two classic CNNs, we also applied the following processes to the original chest HRCT images: 1) deleting the non-lung-region images (Fine selection); 2) deleting 1/6 images at the beginning and the end of all the slicers, respectively (Rough selection) and 3) applying multiple-instance learning [52]. After Rough selection, only the middle 4/6 slicers of the original chest HRCT images are used for COPD classification. In addition, multiple-instance learning was also applied to the original parenchyma images before inputting to the two classic CNNs. Table 1 shows the chest HRCT image data set division for the two classic CNNs. Tables 2 and 3 show the detailed parameters that were set for DenseNet and GoogleNet training. For the 2D CNN, the classification results were decided by the mean probability of all slices of the chest HRCT images. However, for the 3D CNN, the classification results were determined by the probability of the selected slices from the chest HRCT images. The numbers of selected slices were 20 slices and 16 slices, as shown in Tables 2 and 3.
Data set (6:1:3) | Stage 0 | Stage Ⅰ | Stage Ⅱ | Stage Ⅲ & Ⅳ | Total |
Training set | 76 subjects | 65 subjects | 75 subjects | 64 subjects | 280 subjects |
43,694 images | 40,550 images | 43,510 images | 40,944 images | 168,698 images | |
Validation set | 13 subjects | 11 subjects | 12 subjects | 11 subjects | 47 subjects |
7705 images | 6981 images | 7672 images | 6924 images | 29,282 images | |
Test set | 41 subjects | 33 subjects | 35 subjects | 32 subjects | 141 subjects |
23,940 images | 21,141 images | 22,283 images | 20,478 images | 87,842 images |
DenseNet: Input images | Batch size (2D/3D) | Input size (2D/3D) | Epoch (2D/3D) | Drop rate (2D/3D) |
Original chest HRCT images | 20/2 | 512 × 512/512 × 512 × 20* | 50/50 | 0.5/0.2 |
Fine selection (HRCT images) | ||||
Rough selection (HRCT images) | ||||
Rough selection (HRCT images) | ||||
Rough selection (HRCT images) | ||||
Multiple instance (HRCT images) | 16/2 | 512 × 512**/512 × 512 × 16*** | 50/50 | 0.5/0.2 |
Multiple instance (parenchyma) | ||||
Note:* Each case (a set of chest HRCT images) was equally divided into 20 segments, with one slice taken equidistantly to obtain 20 slices in each case. ** After rough selection, each case was equally divided into 10 bags, with one slice taken randomly to obtain 10 slices in each case. *** After rough selection, each case was equally divided into 16 bags, with one slice taken equidistantly to obtain 16 slices in each case. |
DenseNet: Input images | Batch size (2D/3D) | Input size (2D/3D) | Epoch (2D/3D) | Drop rate (2D/3D) |
Original chest HRCT images | 16/2 | 512 × 512/512 × 512 × 20* | 50/50 | 0.2/0.2 |
Fine selection (HRCT images) | ||||
Rough selection (HRCT images) | ||||
Rough selection (HRCT images) | ||||
Rough selection (HRCT images) | ||||
Multiple instance (HRCT images) | 16/2 | 512 × 512**/512 × 512 × 16*** | 50/50 | 0.2/0.2 |
Multiple instance (parenchyma) | ||||
Note:* Each case (a set of chest HRCT images) was equally divided into 20 segments, with one slice taken equidistantly to obtain 20 slices in each case. ** After rough selection, each case was equally divided into 10 bags, with one slice taken randomly to obtain 10 slices in each case. *** After rough selection, each case was equally divided into 16 bags, with one slice taken equidistantly to obtain 16 slices in each case. |
Second, the lung radiomics features and their selected lung radiomics features were respectively used to train and test the different ML classifier, as shown in Figure 5, to highlight Lasso's role in classification. The selected lung radiomics features were determined by Lasso from the lung radiomics features directly calculated by PyRadiomics. Then, the ML classifier with the best classification performance was determined.
Finally, the lung radiomics combination features that characterized the COPD stage were used to improve the performance of the best classifier.
This section shows the results of Lasso, the radiomics combination strategy and the experiments.
Table 4 presents the lung radiomics features selected by Lasso in detail, including the name, class and regression coefficient. To conveniently describe the selected lung radiomics features, we define the selected lung radiomics features as Radiomics1–19. Figure 6 further shows more detailed information on Radiomics1–19. Specifically, Figure 6(A) shows that Radiomics18 was the dominant feature in Radiomics1–19. Figure 6(B) shows that the FIRST class had seven selected lung radiomics features, i.e., the maximum number in all classes. Figure 6(C) also shows that the FIRST class is the most important of all classes.
Definition | Name of the 19 selected lung radiomics features | Class | Coefficient |
Radiomics1 | original_shape_Elongation | Shape | 0.0056 |
Radiomics2 | original_shape_Maximum2DDiameterSlice | Shape | -0.0789 |
Radiomics3 | original_shape_Sphericity | Shape | 0.0624 |
Radiomics4 | log.sigma.1.0.mm.3D_firstorder_Maximum | First Order | 0.0665 |
Radiomics5 | log.sigma.1.0.mm.3D_glcm_ClusterProminence | GLCM 1 | -0.0425 |
Radiomics6 | log.sigma.1.0.mm.3D_glszm_ZoneEntropy | GLSZM 2 | 0.0394 |
Radiomics7 | log.sigma.2.0.mm.3D_firstorder_Maximum | First Order | 0.0129 |
Radiomics8 | log.sigma.2.0.mm.3D_ngtdm_Contrast | NGTDM 3 | -0.0318 |
Radiomics9 | log.sigma.2.0.mm.3D_gldm_DependenceVariance | GLDM 4 | -0.0136 |
Radiomics10 | log.sigma.4.0.mm.3D_firstorder_10Percentile | First Order | -0.0760 |
Radiomics11 | log.sigma.5.0.mm.3D_firstorder_10Percentile | First Order | -0.1669 |
Radiomics12 | wavelet.LLH_firstorder_RootMeanSquared | First Order | -0.0252 |
Radiomics13 | wavelet.HLH_firstorder_Mean | First Order | 0.0599 |
Radiomics14 | wavelet.HLH_glcm_Idmn | GLCM 1 | -0.0022 |
Radiomics15 | wavelet.HLH_ngtdm_Busyness | NGTDM 3 | 0.0444 |
Radiomics16 | wavelet.HHL_gldm_SmallDependenceLowGrayLevelEmphasis | GLDM 4 | -0.0168 |
Radiomics17 | wavelet.HHH_glszm_GrayLevelNonUniformityNormalized | GLSZM 2 | -0.0043 |
Radiomics18 | wavelet.LLL_firstorder_10Percentile | First Order | -0.5314 |
Radiomics19 | wavelet.LLL_glcm_Imc2 | GLCM 1 | 0.1383 |
Note: 1 Gray level co-occurrence matrix. 2 Gray level size zone matrix. 3 Neighboring gray tone difference matrix. 4 Gray level dependence matrix. |
The P-values and significant differences for Radiomics1–19 according to COPD stage evolution were further investigated. A Bonferroni-Dunn multiple comparisons test was applied to calculate the P-values among Radiomics1–19 according to COPD stage. Figure 7(N) and Table 5 show no significant differences for Radiomics14, regardless of COPD stage. Figure 7(A)–(C), (E), (H), (I), (L), (M), (O), (R) and (S) and Table 5 show that only Radiomics1–3, 9, 13, 15 and 19 significantly increased, and that Radiomics5, 8, 12 and 18 significantly decreased with COPD stage evolution from COPD Stage 0 to COPD Stage Ⅰ, respectively. Figure 7(A), (C), (D), (F), (H), (J)–(L), (O), (P), (R) and (S) and Table 5 show that only Radiomics1, 3, 4, 6, 13, 15 and 19 significantly increased, and that Radiomics8, 10–12, 16 and 18 significantly decreased with COPD stage evolution from COPD Stage 0 to COPD Stage Ⅱ, respectively. Figure 7(A), (C)–(E), (F)–(H), (J)–(M) and (O)–(S) and Table 5 show that only Radiomics1, 3, 4, 6, 7, 13, 15 and 19 significantly increased and Radiomics5, 8, 10–12 and 16–18 significantly decreased with COPD stage evolution from COPD Stage 0 to COPD Stages Ⅲ & Ⅳ, respectively. Figure 7(A)–(D), (F)–(K), (M), (R) and (S) and Table 5 show that only Radiomics1, 3, 4, 6, 7, 13 and 19 significantly increased, and that Radiomics2, 8–11 and 18 significantly decreased with COPD stage evolution from COPD Stage Ⅰ to COPD Stages Ⅲ & Ⅳ, respectively. Figure 7(A)–(C), (J)–(L), (O), (R) and (S) and Table 5 show that only Radiomics1–3, 15 and 19 significantly increased, and that Radiomics10–12 and 18 significantly decreased with COPD stage evolution from COPD Stage Ⅱ to COPD Stages Ⅲ & Ⅳ, respectively. Unfortunately, there were no significant differences between at least two COPD stages for the 19 selected lung radiomics features.
Features | 0 vs. Ⅰ | 0 vs. Ⅱ | 0 vs. Ⅲ & Ⅳ | Ⅰ vs. Ⅱ | Ⅰ vs. Ⅲ & Ⅳ | Ⅱ vs. Ⅲ & Ⅳ |
Radiomics1 | < 0.0001 | < 0.0001 | < 0.0001 | 0.9999 (ns1) | < 0.0001 | < 0.0001 |
Radiomics2 | 0.0039 | 0.4406 (ns) | > 0.9999 (ns) | 0.5975 (ns) | < 0.0001 | 0.0164 |
Radiomics3 | 0.0004 | < 0.0001 | < 0.0001 | > 0.9999 (ns) | < 0.0001 | 0.0026 |
Radiomics4 | > 0.9999 (ns) | 0.0244 | 0.0016 | 0.0066 | 0.0004 | > 0.9999 (ns) |
Radiomics5 | 0.0009 | 0.4707 (ns) | 0.0243 | 0.2483 (ns) | > 0.9999 (ns) | > 0.9999 (ns) |
Radiomics6 | 0.8609 (ns) | < 0.0001 | < 0.0001 | 0.0016 | < 0.0001 | 0.7552 (ns) |
Radiomics7 | > 0.9999 (ns) | 0.1892 (ns) | 0.0005 | 0.2978 (ns) | 0.0013 | 0.3961 (ns) |
Radiomics8 | < 0.0001 | < 0.0001 | < 0.0001 | > 0.9999 (ns) | 0.0229 | 0.5546 (ns) |
Radiomics9 | 0.0021 | > 0.9999 (ns) | 0.6507 (ns) | 0.0026 | < 0.0001 | 0.6705 (ns) |
Radiomics10 | > 0.9999 (ns) | 0.0001 | < 0.0001 | < 0.0001 | < 0.0001 | 0.0045 |
Radiomics11 | 0.0626 (ns) | < 0.0001 | < 0.0001 | 0.0001 | < 0.0001 | 0.0055 |
Radiomics12 | < 0.0001 | 0.0006 | < 0.0001 | 0.4505 (ns) | 0.2677 (ns) | 0.0006 |
Radiomics13 | < 0.0001 | < 0.0001 | < 0.0001 | 0.1717 (ns) | < 0.0001 | 0.0800 (ns) |
Radiomics14 | > 0.9999 (ns) | > 0.9999 (ns) | > 0.9999 (ns) | > 0.9999 (ns) | 0.1873 (ns) | 0.6492 (ns) |
Radiomics15 | < 0.0001 | 0.0011 | < 0.0001 | > 0.9999 (ns) | 0.0878 (ns) | 0.0019 |
Radiomics16 | 0.7928 (ns) | 0.0077 | 0.0005 | 0.6650 (ns) | 0.1161 (ns) | 0.8141 (ns) |
Radiomics17 | > 0.9999 (ns) | 0.1001 (ns) | 0.0011 | > 0.9999 (ns) | 0.1153 (ns) | 0.9721 (ns) |
Radiomics18 | < 0.0001 | < 0.0001 | < 0.0001 | 0.1691 (ns) | < 0.0001 | < 0.0001 |
Radiomics19 | < 0.0001 | < 0.0001 | < 0.0001 | > 0.9999 (ns) | < 0.0001 | < 0.0001 |
Note: 1 ns: no significance. |
Features | 0 vs. Ⅰ | 0 vs. Ⅱ | 0 vs. Ⅲ & Ⅳ | Ⅰ vs. Ⅱ | Ⅰ vs. Ⅲ & Ⅳ | Ⅱ vs. Ⅲ & Ⅳ |
Radiomics-SHAPE | > 0.999 (ns1) | 0.4005 (ns) | < 0.0001 | 0.2587 (ns) | < 0.0001 | 0.0003 |
Radiomics-FIRST | < 0.0001 | < 0.0001 | < 0.0001 | 0.0003 | < 0.0001 | < 0.0001 |
Radiomics-GLCM | < 0.0001 | < 0.0001 | < 0.0001 | > 0.999 (ns) | < 0.0001 | < 0.0001 |
Radiomics-GLSZM | 0.9780 (ns) | < 0.0001 | < 0.0001 | 0.0010 | < 0.0001 | 0.7294 (ns) |
Radiomics-NGTDM | < 0.0001 | < 0.0001 | < 0.0001 | > 0.999 (ns) | < 0.0051 | 0.0211 |
Radiomics-GLDM | > 0.999 (ns) | 0.0111 | < 0.0001 | 0.0057 | < 0.0001 | 0.3038 (ns) |
Radiomics-ALL | < 0.0001 | < 0.0001 | < 0.0001 | 0.0006 | < 0.0001 | < 0.0001 |
Note:1 ns: no significance. |
The P-values and significant differences among different COPD stages are respectively shown in Figure 8 and Table 3 for the seven lung radiomics combination features. The Bonferroni-Dunn multiple comparisons test was also applied to calculate the P-values for the seven lung radiomics combination features according to COPD stage.
Figure 8(B), (C), (E) and (G) and Table 3 show that only Radiomics-FIRST, Radiomics-GLCM, Radiomics-NGTDM and Radiomics-ALL significantly increased from COPD Stage 0 to Ⅰ, respectively. Figure 8(B)–(G) and Table 3 show that only Radiomics-SHAPE, Radiomics-GLCM, Radiomics-GLSZM, Radiomics-NGTDM, Radiomics-GLDM and Radiomics-ALL significantly increased from COPD Stage 0 to Ⅱ, respectively. Figure 8(A)–(G) and Table 3 show that all seven of the lung radiomics combination features significantly increased from COPD Stage 0 to Stages Ⅲ & Ⅳ, and from COPD Stage Ⅰ to Stages Ⅲ & Ⅳ. Figure 8(B), (E), (F) and (G) and Table 3 show that only Radiomics-FIRST, Radiomics-GLSZM, Radiomics-GLDM and Radiomics-ALL significantly increased from COPD Stage Ⅰ to Ⅱ. Figure 8(A)–(C), (E) and (G) and Table 3 show that only Radiomics-SHAPE, Radiomics-FIRST, Radiomics-GLCM, Radiomics-NGTDM and Radiomics-ALL significantly increased from COPD Stage Ⅱ to Stages Ⅲ & Ⅳ. Therefore, only Radiomics-FIRST and Radiomics-ALL significantly increased with COPD stage evolution (P-value < 0.05).
This section shows the classification results for the CNN classifier, ML classifier and our proposed method.
Figures 9–11 show the classification results for the DenseNet and GoogleNet. The other evaluation metrics in Tables 7 and 8 were calculated from Figures 10 and 11, respectively. In Figures 10 and 11, the confusion matrices visually show the classification effect of each COPD stage.
DenseNet: Input images | Accuracy (2D/3D) | Precision (2D/3D) | Recall (2D/3D) | F1-score (2D/3D) |
Original chest HRCT images | 0.39/0.54 | 0.22/0.56 | 0.39/0.54 | 0.28/0.51 |
Fine selection (HRCT images) | 0.41/0.54 | 0.38/0.58 | 0.41/0.54 | 0.33/0.52 |
Rough selection (HRCT images) | 0.34/0.57 | 0.45/0.65 | 0.34/0.57 | 0.24/0.54 |
Multiple instance (HRCT images) | 0.40/0.59 | 0.32/0.62 | 0.40/0.59 | 0.32/0.57 |
Original parenchyma images | 0.47/0.58 | 0.47/0.61 | 0.47/0.58 | 0.43/0.58 |
Multiple instance (parenchyma) | 0.49/0.50 | 0.48/0.59 | 0.49/0.50 | 0.44/0.44 |
GoogleNet: Input images | Accuracy (2D/3D) | Precision (2D/3D) | Recall (2D/3D) | F1-score (2D/3D) |
Original chest HRCT images | 0.55/0.40 | 0.67/0.49 | 0.55/0.40 | 0.50/0.37 |
Fine selection (HRCT images) | 0.39/0.48 | 0.40/0.56 | 0.39/0.48 | 0.37/0.44 |
Rough selection (HRCT images) | 0.37/0.36 | 0.31/0.37 | 0.37/0.36 | 0.33/0.32 |
Multiple instance (HRCT images) | 0.39/0.38 | 0.37/0.36 | 0.39/0.38 | 0.28/0.33 |
Original parenchyma images | 0.55/0.39 | 0.56/0.47 | 0.55/0.39 | 0.55/0.34 |
Multiple instance (parenchyma) | 0.41/0.49 | 0.54/0.46 | 0.41/0.49 | 0.33/0.43 |
Figure 9(A) and Table 7 show that the DenseNet with 3D images (3D DenseNet) had consistently better classification performance (based on the evaluation metrics) than the DenseNet with 2D images (2D DenseNet). Figure 10 intuitively shows the classification results for the 2D and 3D GoogleNet. The classification performance of the 2D and 3D DenseNet with original parenchyma images was better than that with the original chest HRCT images. Compared with the chest HRCT images after the fine selection, the classification performance of the 2D DenseNet with the chest HRCT images after the rough selection was lower for the test set, except for precision. However, the classification ability of the 3D DenseNet with the chest HRCT images after the rough selection was higher than that with the chest HRCT images after the fine selection. In particular, Figure 9(A) shows that the best AUC value (0.82) for the DenseNet was achieved by applying multiple-instance learning to 3D chest HRCT images. Table 7 shows that the other evaluation metrics based on applying multiple-instance learning to the 3D chest HRCT images processed with DenseNet were 0.59 (accuracy), 0.62 (precision), 0.59 (recall) and 0.57 (F1-score). However, it was lower than the rough selection result for the 3D chest HRCT images (0.65) in terms of precision.
Figure 9(B) and Table 8 show that the best performance of GoogleNet was based on the 2D original parenchyma images. Finally, Figure 11 intuitively shows the classification results for the 2D and 3D GoogleNet. Specifically, the classification performance of the 2D GoogleNet with the original chest HRCT images/the original parenchyma images was better than that of the 3D GoogleNet. Furthermore, the classification performance of the 2D GoogleNet with the rough selection of the original chest HRCT images was also better than that of the 3D GoogleNet, except for precision. The classification performance of the 2D GoogleNet with the multiple-instance learning of the original chest HRCT images was also better than that of the 3D GoogleNet, except for the F1-score. However, the classification performance of the 2D GoogleNet with the fine selection of the original chest HRCT images was worse than that of the 3D GoogleNet. Furthermore, the classification performance of the 2D GoogleNet with the multiple-instance learning of the original parenchyma images was also worse than that of the 3D GoogleNet, except for precision. In particular, Figure 9(A) shows that the best AUC value (0.81) for the GoogleNet was achieved with the 2D original parenchyma images. Table 8 further shows that the other evaluation metrics of the 2D original parenchyma images process using GoogleNet were 0.55 (accuracy), 0.56 (precision), 0.55 (recall) and 0.55 (F1-score). It was lower than the 2D original chest HRCT images (0.67) in terms of precision.
For all of the six kinds of input images in Tables 7 and 8, the results show that the classification performance of the 3D DenseNet was better than that of the 3D GoogleNet. However, the classification performance of the 2D GoogleNet with the original chest HRCT images/the original parenchyma images was also better than that of the 2D DenseNet.
The classification performances of different ML classifiers were evaluated by using 1316 lung radiomics features and 19 selected lung radiomics features. In addition, the best-performance classifier (MLP classifier) was also determined, as described in this section.
Figure 13 intuitively shows the classification results for different ML classifiers with 1316 lung radiomics features and 19 selected lung radiomics features. Table 9 reports the classification performance of the classifier with 1316 lung radiomics features. Compared with the classification performance of the CNNs (DenseNet and GoogleNet), that of the ML classifiers with 1316 lung radiomics features had an overwhelming effect on COPD stage classification. The accuracy, precision, recall, F1-score and AUC of the ML classifiers improved significantly. Overall, the classification performance of the ML classifiers was better than the DenseNet with the multiple-instance learning of the 3D chest HRCT images (best performance in DenseNet), even for the worst LDA classifier with 1316 lung radiomics features (except for precision). Compared with the classification performance of the ML classifiers with 1316 lung radiomics features, that of the ML classifiers with 19 selected lung radiomics features was further improved.
Classifier | Accuracy | Precision | Recall | F1-score | AUC |
RF classifier | 0.72 | 0.72 | 0.72 | 0.72 | 0.90 |
Ada classifier | 0.70 | 0.69 | 0.70 | 0.69 | 0.90 |
GB classifier | 0.72 | 0.73 | 0.72 | 0.72 | 0.92 |
MLP classifier | 0.78 | 0.78 | 0.78 | 0.78 | 0.92 |
LDA classifier | 0.60 | 0.60 | 0.60 | 0.59 | 0.85 |
SVM classifier | 0.62 | 0.62 | 0.63 | 0.61 | 0.87 |
RF classifier | 0.72 | 0.72 | 0.72 | 0.72 | 0.90 |
Table 9 shows that the accuracy, precision, recall, F1-score and AUC of the MLP classifier with the 1316 lung radiomics features were 0.78, 0.78, 0.78, 0.78 and 0.92, respectively. Therefore, the MLP classifier is regarded as the best-performance classifier for the 1316 lung radiomics features. In addition, Table 10 shows that all of the evaluation metrics improved, and that the MLP classifier was also the best-performance classifier for the 19 selected lung radiomics features. The accuracy, precision, recall, F1-score and AUC of the MLP classifier with the 19 selected lung radiomics features were 0.80, 0.80, 0.80, 0.80 and 0.94, respectively.
Classifier | Accuracy | Precision | Recall | F1-score | AUC |
RF classifier | 0.76 | 0.76 | 0.76 | 0.76 | 0.93 |
Ada classifier | 0.77 | 0.76 | 0.77 | 0.76 | 0.93 |
GB classifier | 0.73 | 0.74 | 0.73 | 0.73 | 0.92 |
MLP classifier | 0.80 | 0.80 | 0.80 | 0.80 | 0.94 |
LDA classifier | 0.65 | 0.65 | 0.65 | 0.65 | 0.89 |
SVM classifier | 0.71 | 0.71 | 0.71 | 0.71 | 0.92 |
RF classifier | 0.76 | 0.76 | 0.76 | 0.76 | 0.93 |
The 19 selected lung radiomics features with Radiomics-FIRST/Radiomics-ALL were used to further evaluate the MLP classifier's performance.
Figure 14(A) and Table 11 show the evaluation metrics for the MLP classifier with one lung radiomics combination feature (Radiomics-X). Figure 14(A) shows that the AUC of the MLP classifier with Radiomics-FIRST/Radiomics-ALL was 0.87/0.85, which is better than that of the other lung radiomics combination features. Figure 15(A) intuitively shows the classification results for the MLP classifier with seven lung radiomics combination features. The MLP classifier with Radiomics-GLSZM/ Radiomics-GLSZM/ Radiomics-GLDM could not distinguish COPD Stage Ⅰ from the other COPD stages ("0" at COPD stage, predicted label). Radiomics-FIRST and Radiomics-ALL, which characterized the COPD stage, showed better classification performance than the other lung radiomics combination features. However, Radiomics-ALL showed the best classification performance for all lung radiomics combination features. The accuracy, precision, recall, F1-score and AUC of the MLP classifier with Radiomics-ALL were 0.60, 0.58, 0.60, 0.59 and 0.87, respectively.
Radiomics-X | Accuracy | Precision | Recall | F1-score | AUC |
Radiomics-FIRST | 0.56 | 0.56 | 0.56 | 0.56 | 0.85 |
Radiomics-SHAPE | 0.39 | 0.40 | 0.39 | 0.36 | 0.64 |
Radiomics-GLCM | 0.49 | 0.51 | 0.49 | 0.47 | 0.72 |
Radiomics-GLSZM | 0.36 | 0.27 | 0.36 | 0.31 | 0.62 |
Radiomics-NGTDM | 0.42 | 0.32 | 0.42 | 0.36 | 0.66 |
Radiomics-GLDM | 0.28 | 0.21 | 0.28 | 0.24 | 0.58 |
Radiomics-ALL | 0.60 | 0.58 | 0.60 | 0.59 | 0.87 |
Figure 14(B) and Table 12 show the evaluation metrics for the MLP classifier with 19 selected lung radiomics features and Radiomics-FIRST/Radiomics-ALL. Figure 15(B) intuitively shows the classification results for the MLP classifier with the 19 selected lung radiomics features and Radiomics-FIRST/Radiomics-ALL. Compared with the MLP classifier with the 19 selected lung radiomics features, all of the evaluation metrics for the MLP classifier with the 19 selected lung radiomics features and Radiomics-FIRST/Radiomics-ALL improved. The accuracy, precision, recall, F1-score and AUC of the MLP classifier with the 19 selected lung radiomics features and Radiomics- FIRST were 0.81, 0.82, 0.81, 0.81 and 0.94, respectively. The accuracy, precision, recall, F1-score and AUC of the MLP classifier with the 19 selected lung radiomics features and Radiomics-ALL were 0.83, 0.83, 0.83, 0.82 and 0.95, respectively.
MLP Classifier: Input features | Accuracy | Precision | Recall | F1-score | AUC |
19 radiomics features + Radiomics-FIRST | 0.81 | 0.82 | 0.81 | 0.81 | 0.94 |
19 radiomics features + Radiomics-ALL | 0.83 | 0.83 | 0. 83 | 0.82 | 0.95 |
Four topics will be discussed, including 1) the classification ability of the classic CNN based on the images and that of the ML classifiers based on the lung radiomics features; 2) the role of feature selection and 3) the reason why the constructed lung radiomics combination features characterizing the COPD stage can improve the lung radiomics combination features.
The classification ability of the classic CNN based on the images was worse than that of the ML classifiers based on the lung radiomics features. The following discussion focuses on the characteristics of COPD and the classic CNN.
First, COPD diffusely distributes in the lung. Therefore, there may be no lesions on some slices of chest HRCT images from a patient with COPD (Stage Ⅰ to Ⅳ). In addition, even if some participants were diagnosed without COPD (no airflow restriction), primary or mild lesions may already exist on their HRCT images. Alternatively, some slices of the chest HRCT images may not have lesions. Although we have made many attempts, including fine selection, rough selection and multiple-instance learning [52], to eliminate the above problems, the classification ability of the classic CNN based on the images still makes us disappointed.
Second, the 3D DenseNet achieved better classification results than the 2D DenseNet. The reason is that, compared with the 2D DenseNet, the 3D DenseNet can extract interlayer information. Compared with the original chest HRCT images, the lung parenchyma image removes the non-lung region containing redundant information. Therefore, when inputting the lung parenchyma images, DenseNet (2D and 3D) can focus more on extracting the features of the lung region to achieve better classification results. Compared with the chest HRCT images after the fine selection (deleting the non-lung-region images), the classification ability of the 2D DenseNet with the chest HRCT images after the rough selection (deleting 1/6 images at the beginning and the end, respectively) was lower, except for precision. The reason is that, although the rough selection of the chest HRCT images deletes no redundant information interference (non-lung images), there is also a lack of effective information on the 2/6 deleted images for COPD classification. However, compared with the chest HRCT images after the fine selection, the classification ability of the 3D DenseNet with the chest HRCT images after the rough selection was better. The reason is that the spacing of the 20 slices (Table 2) after the rough selection was less than that after the fine selection.
Third, similar to DenseNet, GoogleNet also cannot achieve the ideal classification effect with a small amount of training data. When the network dimension was transformed from 2D to 3D, the classification performance of DenseNet was greatly improved. However, the classification performance of GoogleNet improved only slightly, or even decreased. The 2D GoogleNet was proposed based on the classification of natural images (RGB images). Its structure does not have a targeted design for the details of these natural images. Inception network structure of 2D GoogleNet with many 1 × 1 convolution kernels strengthens the channel connection of RGB images, which cannot be reflected in the chest HRCT images. The classification ability of the 2D GoogleNet with the chest HRCT images after rough/fine selection was worse than that of the 2D GoogleNet with the original chest HRCT images. The reason is that non-lung slicers (fine selection) or few lung slicers (rough selection) are removed, but they are still effective information for COPD classification. The accuracy of the 2D GoogleNet with the chest HRCT images after the fine selection was higher than that resulting from rough selection, which confirms the above discussion. The accuracy of the 2D GoogleNet with the original chest HRCT images and the multiple-instance learning was the same. This also shows the role of multiple-instance learning in dealing with COPD classification, which aligns with its original intention. The AUC resulting from the 2D GoogleNet with the original parenchyma images was the maximum, showing that the ROI improves the AUC. In addition, the classification performance of the 2D GoogleNet with the multiple-instance learning of the original parenchyma images was better than that based on the original chest HRCT images. This further illustrates that the ROI improves the classification performance under the conditions of multiple-instance learning. The research on 3D GoogleNets is minimal [53,54,55,56]. As the number of 3D GoogleNet parameters increases, the problem of the limited number of training data will become more obvious. The Inception module structure is specially designed for 2D images. Different convolution kernels extract features from the 2D images, and then feature concatenation is implemented. The stitching is aligned and spliced according to the two dimensions of the tensor. Therefore, compared to the 2D GoogleNet, the 3D GoogleNet has more dimension tensors, which weakens the effect of the receptive fields.
The classification ability of the ML classifiers based on the lung radiomics features is better than that of the classic CNN based on the images. Compared with the classic CNN based on the images, the ML classifiers with the lung radiomics features calculated by preset formulas are more interpretable for the COPD classification. The lung radiomics features were calculated based on information from all of the slicers of the parenchyma images. Therefore, the lung radiomics features cannot be affected by the location of lesions in the chest HRCT images.
Compared to the classification performance of the ML classifiers with the 1316 lung radiomics features, that of the ML classifiers with the 19 selected lung radiomics features was better. Lasso is often used with survival analysis models to determine variables and eliminate the collinearity problem between variables [43,44]. In this study, Lasso was applied to select the classification features, and the classification performance improved. Lasso selects the classification features by establishing the relationship between the independent and dependent variables (lung radiomics features and the COPD stages). This operation selects the lung radiomics features related to COPD stages to reduce the complexity of the ML classifiers and avoid overfitting. While reducing the complexity of the ML classifiers, the ML classifiers can focus on the 19 selected lung radiomics features and improve the classification performance. At the same time, it also endows the lung radiomics features used for classification with strong explanatory power. Radiomics18 of the 19 selected lung radiomics features was the dominant feature with the maximum coefficient.
T-tests have been widely used to select significant variables in survival analysis models, generalized linear models and regression models [57]. Therefore, we were inspired to construct features that can characterize the COPD stage to improve classification performance. The two lung radiomics combination features, Radiomics-FIRST and Radiomics-ALL, were constructed to characterize the COPD stage (P-value < 0.05 for all COPD stages). The features with P-value < 0.05 for all COPD stages showed improved classification performance. The reason can be explained from the perspective of statistics. Generally, a P-value of two groups that is < 0.05 means significant correlation between these two groups. Therefore, using them (P-value < 0.05) to classify the two groups can improve the classification performance.
There are some limitations of this study. First, regarding the materials used in this study, there were not enough cases at the COPD Stage Ⅳ. Second, regarding the methods used in this study, many attempts were made to eliminate the problems of lesions in the HRCT images mentioned in Section 4.1, but the classification performance of the classic CNN remained unsatisfactory. The MLP classifiers with the 19 selected lung radiomics features and Radiomics-ALL achieved good classification performance, but the fixed calculation equations limit further development of the lung radiomics features. However, the CNN based on the chest HRCT images was not subject to the above restrictions. Fully combining a CNN classifier with the limited number of 3D medical images is an urgent problem to be solved. Transfer learning [58] in CNNs has become the first choice to solve the problem of a limited number of 3D medical images. Similarly, the method of data augmentation should be further tried. Inspired by lung radiomics features, which derive many features from each set of chest HRCT images, the 3D chest HRCT images of each subject can be resized into small-sized 3D images. For example, 3D chest HRCT images with 512 × 512 × N can be resized into other sizes, such as 256 × 256 × 300 and 64 × 64 × 50. Finally, the chest HRCT images used in this study were collected from 2009 to 2011, but they are still a rare and standard study cohort. We will also try our best to collect the updated study cohort in the future.
The lung radiomics features were used to characterize and classify the COPD stage in this study. Compared with classic CNN classifiers based on the chest HRCT images, the ML method based on the use of lung radiomics features is more suitable and interpretable for COPD classification. Lasso was applied to select the lung radiomics features for enhancing the ML method's classification performance. The best-performance classifier, i.e., the MLP classifier, was determined. Two lung radiomics combination features, Radiomics-FIRST and Radiomics-ALL, were constructed based on 19 selected lung radiomics features by using the proposed lung radiomics combination strategy for characterizing COPD stage evolution. Radiomics-FIRST/Radiomics-ALL was used further to improve the classification performance of the MLP classifier. As a result, the accuracy, precision, recall, F1-score and AUC of the MLP classifier with the 19 selected lung radiomics features and Radiomics-ALL were 0.83, 0.83, 0.83, 0.82 and 0.95, respectively.
Thanks to the Department of Radiology of the First Affiliated Hospital of Guangzhou Medical University for providing the data set, and to the National Natural Science Foundation of China (62071311), Natural Science Foundation of Guangdong Province, China (2019A1515011382), Stable Support Plan for Colleges and Universities in Shenzhen, China (SZWD2021010), Scientific Research Fund of Liaoning Province, China (JL201919) and the Special Program for Key Fields of Colleges and Universities in Guangdong Province (biomedicine and health) of China (2021ZDZX2008) for the funding support.
The authors declare no conflict of interest.
[1] |
A. G. Mathioudakis, G. A. Mathioudakis, The phenotypes of chronic obstructive pulmonary disease, Arch. Hellenic Med., 31 (2014), 558-569. https://doi.org/10.1080/15412550701629663 doi: 10.1080/15412550701629663
![]() |
[2] | GOLD 2022: Global initiative for chronic obstructive lung disease, 2022. |
[3] |
D. A. Suffredini, R. M. Reed, At the twisted heart of nicotine addiction, BMJ Case Rep., 2012. https://doi.org/10.1136/bcr-2012-006240 doi: 10.1136/bcr-2012-006240
![]() |
[4] |
P. W. Jones, Health status measurement in chronic obstructive pulmonary disease, Thorax, 56 (2001). https://doi.org/10.1201/9780203913406-14 doi: 10.1201/9780203913406-14
![]() |
[5] |
C. D. Brown, J. O. Benditt, F. C. Sciurba, S. M. Lee, G. J. Criner, Z. Mosenifar, et al., Exercise testing in severe emphysema: association with quality of life and lung function, COPD J. Chron. Obstruct. Pulm. Dis., 5 (2008), 117-124. https://doi.org/10.1080/15412550801941265 doi: 10.1080/15412550801941265
![]() |
[6] |
D. A. Lynch, Progress in Imaging COPD, 2004-2014, Chron. Obstruct. Pulm. Dis.: J. COPD Found., 1 (2014), 73-82. https://doi.org/10.15326/jcopdf.1.1.2014.0125 doi: 10.15326/jcopdf.1.1.2014.0125
![]() |
[7] |
P. J. Castaldi, R. S. J. Estépar, C. S. Mendoza, C. P. Hersh, N. Laird, J. D. Crapo, et al., Distinct quantitative computed tomography emphysema patterns are associated with physiology and function in smokers, Am. J. Respir. Crit. Care Med., 188 (2013), 1083-1090. https://doi.org/10.1164/rccm.201305-0873oc doi: 10.1164/rccm.201305-0873oc
![]() |
[8] |
T. B. Grydeland, A. Dirksen, H. O. Coxson, T. M. Eagan, E. Thorsen, S. G. Pillai, et al., Quantitative computed tomography measures of emphysema and airway wall thickness are related to respiratory symptoms, Am. J. Respir. Crit. Care Med., 181 (2010), 353-359. https://doi.org/10.1164/rccm.200907-1008oc doi: 10.1164/rccm.200907-1008oc
![]() |
[9] |
V. Kim, A. Davey, A. P. Comellas, M. K. Han, G. Washko, C. H. Martinez, et al., Clinical and computed tomographic predictors of chronic bronchitis in COPD: a cross Sectional analysis of the COPDGene study, Respir. Res., 15 (2014), 1-9. https://doi.org/10.1186/1465-9921-15-52 doi: 10.1186/1465-9921-15-52
![]() |
[10] |
S. P. Bhatt, N. L. Terry, H. Nath, J. A. Zach, J. Tschirren, M. S. Bolding, et al., Association between expiratory central airway collapse and respiratory outcomes among smokers, Jama, 315 (2016), 498-505. https://doi.org/10.1164/rccm.202008-3122le doi: 10.1164/rccm.202008-3122le
![]() |
[11] |
C. P. Hersh, G. R. Washko, R. S. J. Estépar, S. Lutz, P. J. Friedman, M. K. Han, et al., Paired inspiratory-expiratory chest CT scans to assess for small airways disease in COPD, Respir. Res., 14 (2013), 1-11. https://doi.org/10.1164/ajrccm-conference.2012.185.1_meetingabstracts.a6539 doi: 10.1164/ajrccm-conference.2012.185.1_meetingabstracts.a6539
![]() |
[12] |
S. Bodduluri, J. M. Reinhardt, E. A. Hoffman, J. D. Newell Jr, H. Nath, M. T. Dransfield, et al., Signs of gas trapping in normal lung density regions in smokers, Am. J. Respir. Crit. Care Med., 196 (2017), 1404-1410. https://doi.org/10.1164/rccm.201705-0855oc doi: 10.1164/rccm.201705-0855oc
![]() |
[13] |
C. J. Galbán, M. K. Han, J. L. Boes, K. A. Chughtai, C. R. Meyer, T. D. Johnson, et al. Computed tomography-based biomarker provides unique signature for diagnosis of COPD phenotypes and disease progression, Nat. Med., 18 (2012), 1711-1715. https://doi.org/10.1038/nm.2971 doi: 10.1038/nm.2971
![]() |
[14] |
S. Bodduluri, S. P. Bhatt, E. A. Hoffman, J. D. Newell, C. H. Martinez, M. T. Dransfield, et al., Biomechanical CT metrics are associated with patient outcomes in COPD, Thorax, 72 (2017), 409-414. https://doi.org/10.1136/thoraxjnl-2016-209544 doi: 10.1136/thoraxjnl-2016-209544
![]() |
[15] |
S. P. Bhatt, S. Bodduluri, E. A. Hoffman, J. D. Newell Jr, J. C. Sieren, M. T. Dransfield, et al., Computed tomography measure of lung at risk and lung function decline in chronic obstructive pulmonary disease, Am. J. Respir. Crit. Care Med., 196 (2017), 569-576. https://doi.org/10.1164/rccm.201701-0050oc doi: 10.1164/rccm.201701-0050oc
![]() |
[16] |
G. R. Washko, G. L. Kinney, J. C. Ross, R. S. J. Estépar, M. K. Han, M. T. Dransfield, et al., Lung Mass in Smokers, Acad. Radiol., 24 (2016), 386-392. https://doi.org/10.1016/j.acra.2016.10.011 doi: 10.1016/j.acra.2016.10.011
![]() |
[17] |
J. M. Wells, G. R. Washko, M. K. Han, N. Abbas, H. Nath, A. J. Mamary, et al., Pulmonary arterial enlargement and acute exacerbations of COPD, N. Engl. J. Med., 367 (2012), 913-921. https://doi.org/10.1136/thoraxjnl-2013-203397 doi: 10.1136/thoraxjnl-2013-203397
![]() |
[18] |
R. S. J. Estépar, G. L. Kinney, J. L. Black-Shinn, R. P. Bowler, G. L. Kindlmann, J. C. Ross, et al., Computed tomographic measures of pulmonary vascular morphology in smokers and their clinical implications, Am. J. Respir. Crit. Care Med., 188 (2013), 231-239. https://doi.org/10.1164/rccm.201301-0162oc doi: 10.1164/rccm.201301-0162oc
![]() |
[19] |
P. Lambin, E. Rios-Velazquez, R. Leijenaar, S. Carvalho, R. G. Van Stiphout, P. Granton, et al., Radiomics: Extracting more information from medical images using advanced feature analysis, Eur. J. Cancer, 43 (2007), 441-446. https://doi.org/10.1016/j.ejca.2011.11.036 doi: 10.1016/j.ejca.2011.11.036
![]() |
[20] |
A. N. Frix, F. Cousin, T. Refaee, F. Bottari, A. Vaidyanathan, C. Desir, et al., Radiomics in lung diseases imaging: State-of-the-art for clinicians, J. Pers. Med., 11 (2021), 1-20. https://doi.org/10.3390/jpm11070602 doi: 10.3390/jpm11070602
![]() |
[21] |
S. M. Rezaeijo, R. Abedi-Firouzjah, M. Ghorvei, S. Sarnameh, Screening of COVID-19 based on the extracted radiomics features from chest CT images, J. X-Ray Sci. Technol., 29 (2021), 1-15. https://doi.org/10.3233/xst-200831 doi: 10.3233/xst-200831
![]() |
[22] |
F. Xiao, R. Sun, W. Sun, D. Xu, L. Lan, H. Li, et al., Radiomics analysis of chest CT to predict the overall survival for the severe patients of COVID-19 pneumonia, Phys. Med. Biol., 66 (2021), 1-11. https://doi.org/10.1088/1361-6560/abf717 doi: 10.1088/1361-6560/abf717
![]() |
[23] |
F. Xiong, Y. Wang, T. You, H. Li, T. Fu, H. Tan, et al., The clinical classification of patients with COVID-19 pneumonia was predicted by Radiomics using chest CT, Medicine, 100 (2021), 1-8. https://doi.org/10.1097/md.0000000000025307 doi: 10.1097/md.0000000000025307
![]() |
[24] |
M. Tamal, M. Alshammari, M. Alabdullah, R. Hourani, H. A. Alola, T. M. Hegazi, An integrated framework with machine learning and radiomics for accurate and rapid early diagnosis of COVID-19 from chest x-ray, Expert Syst. Appl., 180 (2021), 1-8. https://doi.org/10.1101/2020.10.01.20205146 doi: 10.1101/2020.10.01.20205146
![]() |
[25] |
Y. Tang, T. Zhang, X. Zhou, Y. Zhao, H. Xu, Y. Liu, et al., The preoperative prognostic value of the radiomics nomogram based on CT combined with machine learning in patients with intrahepatic cholangiocarcinoma, World J. Surg. Oncol., 19 (2021), 1-13. https://doi.org/10.1186/s12957-021-02162-0 doi: 10.1186/s12957-021-02162-0
![]() |
[26] |
X. Han, J. Yang, J. Luo, P. Chen, Z. Zhang, A. Alu, et al., Application of CT-based radiomics in discriminating pancreatic cystadenomas from pancreatic neuroendocrine tumors using machine learning methods, Front. Oncol., 11 (2021), 1-13. https://doi.org/10.3389/fonc.2021.606677 doi: 10.3389/fonc.2021.606677
![]() |
[27] | M. F. A. Chaudhary, E. A. Hoffman, A. P. Comellas, J. Guo, S. Fortis, S. Bodduluri, et al., CT texture features predict severe COPD exacerbations in spiromics, in American Thoracic Society 2021 International Conference, (2021), 1122-1122. https://doi.org/10.1164/ajrccm-conference.2021.203.1_meetingabstracts.a1122 |
[28] |
M. Occhipinti, M. Paoletti, B. J. Bartholmai, S. Rajagopalan, R. A. Karwoski, C. Nardi, et al., Spirometric assessment of emphysema presence and severity as measured by quantitative CT and CT-based radiomics in COPD, Respir. Res., 20 (2019), 1-11. https://doi.org/10.1186/s12931-019-1049-3 doi: 10.1186/s12931-019-1049-3
![]() |
[29] |
G. Wu, A. Ibrahim, I. Halilaj, R. T. Leijenaar, W. Rogers, H. A. Gietema, et al., The emerging role of radiomics in COPD and lung cancer, Respiration, 99 (2020), 99-107. https://doi.org/10.1159/000505429 doi: 10.1159/000505429
![]() |
[30] |
G. Maragatham, S. Rajendran, Improving the classifier accuracy with an integrated approach using medical data-a study, Int. J. Med. Eng. Inf., 12 (2020), 313-321. https://doi.org/10.1504/ijmei.2020.10029317 doi: 10.1504/ijmei.2020.10029317
![]() |
[31] |
D. Lu, Q. Weng, A survey of image classification methods and techniques for improving classification performance, Int. J. Remote Sens., 28 (2007), 823-870. https://doi.org/10.1080/01431160600746456 doi: 10.1080/01431160600746456
![]() |
[32] |
R. C. Au, W. C. Tan, J. Bourbeau, J. C. Hogg, M. Kirby, Impact of image pre-processing methods on computed tomography radiomics features in chronic obstructive pulmonary disease, Phys. Med. Biol., 66 (2021). https://doi.org/10.2139/ssrn.3349696 doi: 10.2139/ssrn.3349696
![]() |
[33] |
J. Yun, Y. H. Cho, S. M. Lee, J. Hwang, J. S. Lee, Y. M. Oh, et al., Deep radiomics-based survival prediction in patients with chronic obstructive pulmonary disease, Sci. Rep., 11 (2021), 1-9. https://doi.org/10.1038/s41598-021-94535-4 doi: 10.1038/s41598-021-94535-4
![]() |
[34] | R. C. Au, W. C. Tan, J. Bourbeau, J. C. Hogg, M. Kirby, Radiomics Analysis to Predict Presence of Chronic Obstructive Pulmonary Disease and Symptoms Using Machine Learning, in TP121 COPD: FROM CELLS TO THE CLINIC, American Thoracic Society, 2021. https://doi.org/10.1164/ajrccm-conference.2021.203.1_meetingabstracts.a4568 |
[35] | C. Liang, J. Xu, F. Wang, H. Chen, J. Tang, D. Chen, et al., Development of a radiomics model for predicting COPD exacerbations based on complementary visual information, in TP041 DIAGNOSIS AND RISK ASSESSMENT IN COPD, American Thoracic Society, 2021. https://doi.org/10.1164/ajrccm-conference.2021.203.1_meetingabstracts.a2296 |
[36] |
Y. Yang, W. Li, Y. Guo, Y. Liu, Q. Li, K. Yang, et al., Early COPD risk decision for adults aged from 40 to 79 years based on lung radiomics features, Front. Med., 9 (2022), 1-15. https://doi.org/10.3389/fmed.2022.845286 doi: 10.3389/fmed.2022.845286
![]() |
[37] |
Y. Yang, W. Li, Y. Kang, Y. Guo, K. Yang, Q. Li, et al., A novel lung radiomics feature for characterizing resting heart rate and COPD stage evolution based on radiomics feature combination strategy, Math. Biosci. Eng., 19 (2022), 4145-4165. https://doi.org/10.3934/mbe.2022191 doi: 10.3934/mbe.2022191
![]() |
[38] |
Y. Zhou, P. L. Bruijnzeel, C. McCrae, J. Zheng, U. Nihlen, R. Zhou, et al., Study on risk factors and phenotypes of acute exacerbations of chronic obstructive pulmonary disease in Guangzhou, China-design and baseline characteristics, J. Thorac. Dis., 7 (2015), 720-733. https://doi:10.3978/j.issn.2072-1439.2015.04.14 doi: 10.3978/j.issn.2072-1439.2015.04.14
![]() |
[39] |
J. Hofmanninger, F. Prayer, J. Pan, S. Rohrich, H. Prosch, G. Langs, Automatic lung segmentation in routine imaging is a data diversity problem, not a methodology problem, Eur. Radiol. Exp., 4 (2020), 1-13. https://doi.org/10.1186/s41747-020-00173-2 doi: 10.1186/s41747-020-00173-2
![]() |
[40] |
Y. Yang, Q. Li, Y. Guo, Y. Liu, X. Li, J. Guo, et al., Lung parenchyma parameters measure of rats from pulmonary window computed tomography images based on ResU-Net model for medical respiratory researches, Math. Biosci. Eng., 18 (2021), 4193-4211. https://doi.org/10.3934/mbe.2021210 doi: 10.3934/mbe.2021210
![]() |
[41] | Y. Yang, Y. Guo, J. Guo, Y. Gao, Y. Kang, A method of abstracting single pulmonary lobe from computed tomography pulmonary images for locating COPD, in Proceedings of the Fourth International Conference on Biological Information and Biomedical Engineering, (2020), 1-6. https://doi.org/10.1145/3403782.3403805 |
[42] |
J. J. M. van Griethuysen, A. Fedorov, C. Parmar, A. Hosny, N. Aucoin, V. Narayan, et al., Computational radiomics system to decode the radiographic phenotype, Cancer Res., 77 (2017), 104-107. https://doi.org/10.1158/0008-5472.can-17-0339 doi: 10.1158/0008-5472.can-17-0339
![]() |
[43] |
R. Tibshirani, Regression shrinkage and selection via the Lasso, J. R. Stat. Soc. B, 58 (2007), 267-288. https://doi.org/10.1111/j.2517-6161.1996.tb02080.x doi: 10.1111/j.2517-6161.1996.tb02080.x
![]() |
[44] |
N. Simon, J. Friedman, T. Hastie, R. Tibshirani, Regularization paths for Cox's proportional hazards model via coordinate descent, J. Stat. Software, 39 (2011), 1-13. https://doi.org/10.18637/jss.v039.i05 doi: 10.18637/jss.v039.i05
![]() |
[45] | Y. Qi, Random forest for bioinformatics, in Ensemble machine learning: methods and applications, Springer, Boston, MA, (2012), 307-323. https://doi.org/10.1007/978-1-4419-9326-7_11 |
[46] | T. H. Kim, D. C. Park, D. M. Woo, T. Jeong, S. Y. Min, Multi-class classifier-based adaboost algorithm, in International conference on intelligent science and intelligent data engineering, Springer, Berlin, Heidelberg, (2011), 122-127. https://doi.org/10.1007/978-3-642-31919-8_16 |
[47] | V. K. Ayyadevara, Gradient boosting machine, in Pro machine learning algorithms, Apress, Berkeley, CA, (2018), 117-134. https://doi.org/10.1007/978-1-4842-3564-5_6 |
[48] |
M. Taki, A. Rohani, F. Soheili-Fard, A. Abdeshahi, Assessment of energy consumption and modeling of output energy for wheat production by neural network (MLP and RBF) and Gaussian process regression (GPR) models, J. Cleaner Prod. 172 (2018), 3028-3041. https://doi.org/10.1016/j.jclepro.2017.11.107 doi: 10.1016/j.jclepro.2017.11.107
![]() |
[49] |
W. Hu, W. Hu, S. Maybank, Adaboost-based algorithm for network intrusion detection, IEEE Trans. Syst. Man Cybern. Part B Cybern., 38 (2008), 577-583. https://doi.org/10.1109/tsmcb.2007.914695 doi: 10.1109/tsmcb.2007.914695
![]() |
[50] | S. Suthaharan, Support vector machine, in Machine learning models and algorithms for big data classification, Springer, Boston, MA, (2016), 207-235. https://doi.org/10.1007/978-1-4899-7641-3_9 |
[51] |
Q. Li, Y. Yang, Y. Guo, W. Li, Y. Liu, H. Liu, et al., Performance evaluation of deep learning classification network for image features, IEEE Access, 9 (2021), 9318-9333. https://doi.org/10.1109/access.2020.3048956 doi: 10.1109/access.2020.3048956
![]() |
[52] |
M. A. Carbonneau, V. Cheplygina, E. Granger, G. Gagnon, Multiple instance learning: A survey of problem characteristics and applications, Pattern Recognit., 77 (2018), 329-353. https://doi.org/10.1016/j.patcog.2017.10.009 doi: 10.1016/j.patcog.2017.10.009
![]() |
[53] |
H. Polat, H. D. Mehr, Classification of pulmonary CT images by using hybrid 3D-deep convolutional neural network architecture, Appl. Sci., 9 (2019), 1-15. https://doi.org/10.3390/app9050940 doi: 10.3390/app9050940
![]() |
[54] |
A. Chon, N. Balachandar, P. Lu, Deep convolutional neural networks for lung cancer detection, Standford Univ., (2017), 1-9. https://doi.org/10.1109/uemcon47517.2019.8993023 doi: 10.1109/uemcon47517.2019.8993023
![]() |
[55] |
S. P. Singh, L. Wang, S. Gupta, H. Goli, P. Padmanabhan, B. Gulyás, 3D deep learning on medical images: a review, Sensors, 20 (2020), 1-24. https://doi.org/10.3390/s20185097 doi: 10.3390/s20185097
![]() |
[56] |
B. H. Lee, D. H. Oh, T. Y. Kim, 3D Virtual reality game with deep learning-based hand gesture recognition, J. Korea Comput. Graphics Soc., 24 (2018), 41-48. https://doi.org/10.15701/kcgs.2018.24.5.41 doi: 10.15701/kcgs.2018.24.5.41
![]() |
[57] |
B. George, S. Seals, I. Aban, Survival analysis and regression models, J. Nucl. Cardiol., 21 (2014), 686-694. https://doi.org/10.1007/s12350-014-9908-2 doi: 10.1007/s12350-014-9908-2
![]() |
[58] | L. Torrey, J. Shavlik, Transfer learning, in Handbook of research on machine learning applications and trends: algorithms, methods, and techniques, IGI global, (2010), 242-264. https://doi.org/10.4018/978-1-60566-766-9.ch011 |
1. | Yingjian Yang, Shicong Wang, Nanrong Zeng, Wenxin Duan, Ziran Chen, Yang Liu, Wei Li, Yingwei Guo, Huai Chen, Xian Li, Rongchang Chen, Yan Kang, Lung Radiomics Features Selection for COPD Stage Classification Based on Auto-Metric Graph Neural Network, 2022, 12, 2075-4418, 2274, 10.3390/diagnostics12102274 | |
2. | Yingjian Yang, Ziran Chen, Wei Li, Nanrong Zeng, Yingwei Guo, Shicong Wang, Wenxin Duan, Yang Liu, Huai Chen, Xian Li, Rongchang Chen, Yan Kang, Multi-modal data combination strategy based on chest HRCT images and PFT parameters for intelligent dyspnea identification in COPD, 2022, 9, 2296-858X, 10.3389/fmed.2022.980950 | |
3. | Yanan Wu, Shuyue Xia, Zhenyu Liang, Rongchang Chen, Shouliang Qi, Artificial intelligence in COPD CT images: identification, staging, and quantitation, 2024, 25, 1465-993X, 10.1186/s12931-024-02913-z | |
4. | Meng Zhao, Yanan Wu, Yifu Li, Xiaoyu Zhang, Shuyue Xia, Jiaxuan Xu, Rongchang Chen, Zhenyu Liang, Shouliang Qi, Learning and depicting lobe-based radiomics feature for COPD Severity staging in low-dose CT images, 2024, 24, 1471-2466, 10.1186/s12890-024-03109-3 | |
5. | Xingguang Deng, Wei Li, Yingjian Yang, Shicong Wang, Nanrong Zeng, Jiaxuan Xu, Haseeb Hassan, Ziran Chen, Yang Liu, Xiaoqiang Miao, Yingwei Guo, Rongchang Chen, Yan Kang, COPD stage detection: leveraging the auto-metric graph neural network with inspiratory and expiratory chest CT images, 2024, 62, 0140-0118, 1733, 10.1007/s11517-024-03016-z | |
6. | TaoHu Zhou, Yu Guan, XiaoQing Lin, XiuXiu Zhou, Liang Mao, YanQing Ma, Bing Fan, Jie Li, ShiYuan Liu, Li Fan, CT-based whole lung radiomics nomogram for identification of PRISm from non-COPD subjects, 2024, 25, 1465-993X, 10.1186/s12931-024-02964-2 | |
7. | TaoHu Zhou, Yu Guan, XiaoQing Lin, XiuXiu Zhou, Liang Mao, YanQing Ma, Bing Fan, Jie Li, WenTing Tu, ShiYuan Liu, Li Fan, A clinical-radiomics nomogram based on automated segmentation of chest CT to discriminate PRISm and COPD patients, 2024, 13, 23520477, 100580, 10.1016/j.ejro.2024.100580 | |
8. | Fei Shan, Minwen Zheng, 2024, Chapter 9, 978-981-99-8440-4, 153, 10.1007/978-981-99-8441-1_9 | |
9. | Peng An, Junjie Liu, Mengxing Yu, Jinsong Wang, Zhongqiu Wang, Predicting mixed venous oxygen saturation (SvO2) impairment in COPD patients using clinical-CT radiomics data: A preliminary study, 2024, 32, 09287329, 1569, 10.3233/THC-230619 | |
10. | Zecheng Zhu, Shunjin Zhao, Jiahui Li, Yuting Wang, Luopiao Xu, Yubing Jia, Zihan Li, Wenyuan Li, Gang Chen, Xifeng Wu, Development and application of a deep learning-based comprehensive early diagnostic model for chronic obstructive pulmonary disease, 2024, 25, 1465-993X, 10.1186/s12931-024-02793-3 | |
11. | Yingjian Yang, Nanrong Zeng, Ziran Chen, Wei Li, Yingwei Guo, Shicong Wang, Wenxin Duan, Yang Liu, Rongchang Chen, Yan Kang, Weihua Yang, Multi‐Layer Perceptron Classifier with the Proposed Combined Feature Vector of 3D CNN Features and Lung Radiomics Features for COPD Stage Classification, 2023, 2023, 2040-2295, 10.1155/2023/3715603 | |
12. | Tao-Hu Zhou, Xiu-Xiu Zhou, Jiong Ni, Yan-Qing Ma, Fang-Yi Xu, Bing Fan, Yu Guan, Xin-Ang Jiang, Xiao-Qing Lin, Jie Li, Yi Xia, Xiang Wang, Yun Wang, Wen-Jun Huang, Wen-Ting Tu, Peng Dong, Zhao-Bin Li, Shi-Yuan Liu, Li Fan, CT whole lung radiomic nomogram: a potential biomarker for lung function evaluation and identification of COPD, 2024, 11, 2054-9369, 10.1186/s40779-024-00516-9 | |
13. | Taohu Zhou, Xiuxiu Zhou, Jiong Ni, Yu Guan, Xin’ang Jiang, Xiaoqing Lin, Jie Li, Yi Xia, Xiang Wang, Yun Wang, Wenjun Huang, Wenting Tu, Peng Dong, Zhaobin Li, Shiyuan Liu, Li Fan, A CT-Based Lung Radiomics Nomogram for Classifying the Severity of Chronic Obstructive Pulmonary Disease, 2024, Volume 19, 1178-2005, 2705, 10.2147/COPD.S483007 | |
14. | Yingjian Yang, Jie Zheng, Peng Guo, Qi Gao, Yingwei Guo, Ziran Chen, Chengcheng Liu, Tianqi Wu, Zhanglei Ouyang, Huai Chen, Yan Kang, Three-stage registration pipeline for dynamic lung field of chest X-ray images based on convolutional neural networks, 2025, 8, 2624-8212, 10.3389/frai.2025.1466643 | |
15. | Farzat Farha, Sageer Abass, Saba Khan, Javed Ali, Bushra Parveen, Sayeed Ahmad, Rabea Parveen, Transforming pulmonary healthcare: the role of artificial intelligence in diagnosis and treatment, 2025, 1747-6348, 10.1080/17476348.2025.2491723 |
Data set (6:1:3) | Stage 0 | Stage Ⅰ | Stage Ⅱ | Stage Ⅲ & Ⅳ | Total |
Training set | 76 subjects | 65 subjects | 75 subjects | 64 subjects | 280 subjects |
43,694 images | 40,550 images | 43,510 images | 40,944 images | 168,698 images | |
Validation set | 13 subjects | 11 subjects | 12 subjects | 11 subjects | 47 subjects |
7705 images | 6981 images | 7672 images | 6924 images | 29,282 images | |
Test set | 41 subjects | 33 subjects | 35 subjects | 32 subjects | 141 subjects |
23,940 images | 21,141 images | 22,283 images | 20,478 images | 87,842 images |
DenseNet: Input images | Batch size (2D/3D) | Input size (2D/3D) | Epoch (2D/3D) | Drop rate (2D/3D) |
Original chest HRCT images | 20/2 | 512 × 512/512 × 512 × 20* | 50/50 | 0.5/0.2 |
Fine selection (HRCT images) | ||||
Rough selection (HRCT images) | ||||
Rough selection (HRCT images) | ||||
Rough selection (HRCT images) | ||||
Multiple instance (HRCT images) | 16/2 | 512 × 512**/512 × 512 × 16*** | 50/50 | 0.5/0.2 |
Multiple instance (parenchyma) | ||||
Note:* Each case (a set of chest HRCT images) was equally divided into 20 segments, with one slice taken equidistantly to obtain 20 slices in each case. ** After rough selection, each case was equally divided into 10 bags, with one slice taken randomly to obtain 10 slices in each case. *** After rough selection, each case was equally divided into 16 bags, with one slice taken equidistantly to obtain 16 slices in each case. |
DenseNet: Input images | Batch size (2D/3D) | Input size (2D/3D) | Epoch (2D/3D) | Drop rate (2D/3D) |
Original chest HRCT images | 16/2 | 512 × 512/512 × 512 × 20* | 50/50 | 0.2/0.2 |
Fine selection (HRCT images) | ||||
Rough selection (HRCT images) | ||||
Rough selection (HRCT images) | ||||
Rough selection (HRCT images) | ||||
Multiple instance (HRCT images) | 16/2 | 512 × 512**/512 × 512 × 16*** | 50/50 | 0.2/0.2 |
Multiple instance (parenchyma) | ||||
Note:* Each case (a set of chest HRCT images) was equally divided into 20 segments, with one slice taken equidistantly to obtain 20 slices in each case. ** After rough selection, each case was equally divided into 10 bags, with one slice taken randomly to obtain 10 slices in each case. *** After rough selection, each case was equally divided into 16 bags, with one slice taken equidistantly to obtain 16 slices in each case. |
Definition | Name of the 19 selected lung radiomics features | Class | Coefficient |
Radiomics1 | original_shape_Elongation | Shape | 0.0056 |
Radiomics2 | original_shape_Maximum2DDiameterSlice | Shape | -0.0789 |
Radiomics3 | original_shape_Sphericity | Shape | 0.0624 |
Radiomics4 | log.sigma.1.0.mm.3D_firstorder_Maximum | First Order | 0.0665 |
Radiomics5 | log.sigma.1.0.mm.3D_glcm_ClusterProminence | GLCM 1 | -0.0425 |
Radiomics6 | log.sigma.1.0.mm.3D_glszm_ZoneEntropy | GLSZM 2 | 0.0394 |
Radiomics7 | log.sigma.2.0.mm.3D_firstorder_Maximum | First Order | 0.0129 |
Radiomics8 | log.sigma.2.0.mm.3D_ngtdm_Contrast | NGTDM 3 | -0.0318 |
Radiomics9 | log.sigma.2.0.mm.3D_gldm_DependenceVariance | GLDM 4 | -0.0136 |
Radiomics10 | log.sigma.4.0.mm.3D_firstorder_10Percentile | First Order | -0.0760 |
Radiomics11 | log.sigma.5.0.mm.3D_firstorder_10Percentile | First Order | -0.1669 |
Radiomics12 | wavelet.LLH_firstorder_RootMeanSquared | First Order | -0.0252 |
Radiomics13 | wavelet.HLH_firstorder_Mean | First Order | 0.0599 |
Radiomics14 | wavelet.HLH_glcm_Idmn | GLCM 1 | -0.0022 |
Radiomics15 | wavelet.HLH_ngtdm_Busyness | NGTDM 3 | 0.0444 |
Radiomics16 | wavelet.HHL_gldm_SmallDependenceLowGrayLevelEmphasis | GLDM 4 | -0.0168 |
Radiomics17 | wavelet.HHH_glszm_GrayLevelNonUniformityNormalized | GLSZM 2 | -0.0043 |
Radiomics18 | wavelet.LLL_firstorder_10Percentile | First Order | -0.5314 |
Radiomics19 | wavelet.LLL_glcm_Imc2 | GLCM 1 | 0.1383 |
Note: 1 Gray level co-occurrence matrix. 2 Gray level size zone matrix. 3 Neighboring gray tone difference matrix. 4 Gray level dependence matrix. |
Features | 0 vs. Ⅰ | 0 vs. Ⅱ | 0 vs. Ⅲ & Ⅳ | Ⅰ vs. Ⅱ | Ⅰ vs. Ⅲ & Ⅳ | Ⅱ vs. Ⅲ & Ⅳ |
Radiomics1 | < 0.0001 | < 0.0001 | < 0.0001 | 0.9999 (ns1) | < 0.0001 | < 0.0001 |
Radiomics2 | 0.0039 | 0.4406 (ns) | > 0.9999 (ns) | 0.5975 (ns) | < 0.0001 | 0.0164 |
Radiomics3 | 0.0004 | < 0.0001 | < 0.0001 | > 0.9999 (ns) | < 0.0001 | 0.0026 |
Radiomics4 | > 0.9999 (ns) | 0.0244 | 0.0016 | 0.0066 | 0.0004 | > 0.9999 (ns) |
Radiomics5 | 0.0009 | 0.4707 (ns) | 0.0243 | 0.2483 (ns) | > 0.9999 (ns) | > 0.9999 (ns) |
Radiomics6 | 0.8609 (ns) | < 0.0001 | < 0.0001 | 0.0016 | < 0.0001 | 0.7552 (ns) |
Radiomics7 | > 0.9999 (ns) | 0.1892 (ns) | 0.0005 | 0.2978 (ns) | 0.0013 | 0.3961 (ns) |
Radiomics8 | < 0.0001 | < 0.0001 | < 0.0001 | > 0.9999 (ns) | 0.0229 | 0.5546 (ns) |
Radiomics9 | 0.0021 | > 0.9999 (ns) | 0.6507 (ns) | 0.0026 | < 0.0001 | 0.6705 (ns) |
Radiomics10 | > 0.9999 (ns) | 0.0001 | < 0.0001 | < 0.0001 | < 0.0001 | 0.0045 |
Radiomics11 | 0.0626 (ns) | < 0.0001 | < 0.0001 | 0.0001 | < 0.0001 | 0.0055 |
Radiomics12 | < 0.0001 | 0.0006 | < 0.0001 | 0.4505 (ns) | 0.2677 (ns) | 0.0006 |
Radiomics13 | < 0.0001 | < 0.0001 | < 0.0001 | 0.1717 (ns) | < 0.0001 | 0.0800 (ns) |
Radiomics14 | > 0.9999 (ns) | > 0.9999 (ns) | > 0.9999 (ns) | > 0.9999 (ns) | 0.1873 (ns) | 0.6492 (ns) |
Radiomics15 | < 0.0001 | 0.0011 | < 0.0001 | > 0.9999 (ns) | 0.0878 (ns) | 0.0019 |
Radiomics16 | 0.7928 (ns) | 0.0077 | 0.0005 | 0.6650 (ns) | 0.1161 (ns) | 0.8141 (ns) |
Radiomics17 | > 0.9999 (ns) | 0.1001 (ns) | 0.0011 | > 0.9999 (ns) | 0.1153 (ns) | 0.9721 (ns) |
Radiomics18 | < 0.0001 | < 0.0001 | < 0.0001 | 0.1691 (ns) | < 0.0001 | < 0.0001 |
Radiomics19 | < 0.0001 | < 0.0001 | < 0.0001 | > 0.9999 (ns) | < 0.0001 | < 0.0001 |
Note: 1 ns: no significance. |
Features | 0 vs. Ⅰ | 0 vs. Ⅱ | 0 vs. Ⅲ & Ⅳ | Ⅰ vs. Ⅱ | Ⅰ vs. Ⅲ & Ⅳ | Ⅱ vs. Ⅲ & Ⅳ |
Radiomics-SHAPE | > 0.999 (ns1) | 0.4005 (ns) | < 0.0001 | 0.2587 (ns) | < 0.0001 | 0.0003 |
Radiomics-FIRST | < 0.0001 | < 0.0001 | < 0.0001 | 0.0003 | < 0.0001 | < 0.0001 |
Radiomics-GLCM | < 0.0001 | < 0.0001 | < 0.0001 | > 0.999 (ns) | < 0.0001 | < 0.0001 |
Radiomics-GLSZM | 0.9780 (ns) | < 0.0001 | < 0.0001 | 0.0010 | < 0.0001 | 0.7294 (ns) |
Radiomics-NGTDM | < 0.0001 | < 0.0001 | < 0.0001 | > 0.999 (ns) | < 0.0051 | 0.0211 |
Radiomics-GLDM | > 0.999 (ns) | 0.0111 | < 0.0001 | 0.0057 | < 0.0001 | 0.3038 (ns) |
Radiomics-ALL | < 0.0001 | < 0.0001 | < 0.0001 | 0.0006 | < 0.0001 | < 0.0001 |
Note:1 ns: no significance. |
DenseNet: Input images | Accuracy (2D/3D) | Precision (2D/3D) | Recall (2D/3D) | F1-score (2D/3D) |
Original chest HRCT images | 0.39/0.54 | 0.22/0.56 | 0.39/0.54 | 0.28/0.51 |
Fine selection (HRCT images) | 0.41/0.54 | 0.38/0.58 | 0.41/0.54 | 0.33/0.52 |
Rough selection (HRCT images) | 0.34/0.57 | 0.45/0.65 | 0.34/0.57 | 0.24/0.54 |
Multiple instance (HRCT images) | 0.40/0.59 | 0.32/0.62 | 0.40/0.59 | 0.32/0.57 |
Original parenchyma images | 0.47/0.58 | 0.47/0.61 | 0.47/0.58 | 0.43/0.58 |
Multiple instance (parenchyma) | 0.49/0.50 | 0.48/0.59 | 0.49/0.50 | 0.44/0.44 |
GoogleNet: Input images | Accuracy (2D/3D) | Precision (2D/3D) | Recall (2D/3D) | F1-score (2D/3D) |
Original chest HRCT images | 0.55/0.40 | 0.67/0.49 | 0.55/0.40 | 0.50/0.37 |
Fine selection (HRCT images) | 0.39/0.48 | 0.40/0.56 | 0.39/0.48 | 0.37/0.44 |
Rough selection (HRCT images) | 0.37/0.36 | 0.31/0.37 | 0.37/0.36 | 0.33/0.32 |
Multiple instance (HRCT images) | 0.39/0.38 | 0.37/0.36 | 0.39/0.38 | 0.28/0.33 |
Original parenchyma images | 0.55/0.39 | 0.56/0.47 | 0.55/0.39 | 0.55/0.34 |
Multiple instance (parenchyma) | 0.41/0.49 | 0.54/0.46 | 0.41/0.49 | 0.33/0.43 |
Classifier | Accuracy | Precision | Recall | F1-score | AUC |
RF classifier | 0.72 | 0.72 | 0.72 | 0.72 | 0.90 |
Ada classifier | 0.70 | 0.69 | 0.70 | 0.69 | 0.90 |
GB classifier | 0.72 | 0.73 | 0.72 | 0.72 | 0.92 |
MLP classifier | 0.78 | 0.78 | 0.78 | 0.78 | 0.92 |
LDA classifier | 0.60 | 0.60 | 0.60 | 0.59 | 0.85 |
SVM classifier | 0.62 | 0.62 | 0.63 | 0.61 | 0.87 |
RF classifier | 0.72 | 0.72 | 0.72 | 0.72 | 0.90 |
Classifier | Accuracy | Precision | Recall | F1-score | AUC |
RF classifier | 0.76 | 0.76 | 0.76 | 0.76 | 0.93 |
Ada classifier | 0.77 | 0.76 | 0.77 | 0.76 | 0.93 |
GB classifier | 0.73 | 0.74 | 0.73 | 0.73 | 0.92 |
MLP classifier | 0.80 | 0.80 | 0.80 | 0.80 | 0.94 |
LDA classifier | 0.65 | 0.65 | 0.65 | 0.65 | 0.89 |
SVM classifier | 0.71 | 0.71 | 0.71 | 0.71 | 0.92 |
RF classifier | 0.76 | 0.76 | 0.76 | 0.76 | 0.93 |
Radiomics-X | Accuracy | Precision | Recall | F1-score | AUC |
Radiomics-FIRST | 0.56 | 0.56 | 0.56 | 0.56 | 0.85 |
Radiomics-SHAPE | 0.39 | 0.40 | 0.39 | 0.36 | 0.64 |
Radiomics-GLCM | 0.49 | 0.51 | 0.49 | 0.47 | 0.72 |
Radiomics-GLSZM | 0.36 | 0.27 | 0.36 | 0.31 | 0.62 |
Radiomics-NGTDM | 0.42 | 0.32 | 0.42 | 0.36 | 0.66 |
Radiomics-GLDM | 0.28 | 0.21 | 0.28 | 0.24 | 0.58 |
Radiomics-ALL | 0.60 | 0.58 | 0.60 | 0.59 | 0.87 |
MLP Classifier: Input features | Accuracy | Precision | Recall | F1-score | AUC |
19 radiomics features + Radiomics-FIRST | 0.81 | 0.82 | 0.81 | 0.81 | 0.94 |
19 radiomics features + Radiomics-ALL | 0.83 | 0.83 | 0. 83 | 0.82 | 0.95 |
Data set (6:1:3) | Stage 0 | Stage Ⅰ | Stage Ⅱ | Stage Ⅲ & Ⅳ | Total |
Training set | 76 subjects | 65 subjects | 75 subjects | 64 subjects | 280 subjects |
43,694 images | 40,550 images | 43,510 images | 40,944 images | 168,698 images | |
Validation set | 13 subjects | 11 subjects | 12 subjects | 11 subjects | 47 subjects |
7705 images | 6981 images | 7672 images | 6924 images | 29,282 images | |
Test set | 41 subjects | 33 subjects | 35 subjects | 32 subjects | 141 subjects |
23,940 images | 21,141 images | 22,283 images | 20,478 images | 87,842 images |
DenseNet: Input images | Batch size (2D/3D) | Input size (2D/3D) | Epoch (2D/3D) | Drop rate (2D/3D) |
Original chest HRCT images | 20/2 | 512 × 512/512 × 512 × 20* | 50/50 | 0.5/0.2 |
Fine selection (HRCT images) | ||||
Rough selection (HRCT images) | ||||
Rough selection (HRCT images) | ||||
Rough selection (HRCT images) | ||||
Multiple instance (HRCT images) | 16/2 | 512 × 512**/512 × 512 × 16*** | 50/50 | 0.5/0.2 |
Multiple instance (parenchyma) | ||||
Note:* Each case (a set of chest HRCT images) was equally divided into 20 segments, with one slice taken equidistantly to obtain 20 slices in each case. ** After rough selection, each case was equally divided into 10 bags, with one slice taken randomly to obtain 10 slices in each case. *** After rough selection, each case was equally divided into 16 bags, with one slice taken equidistantly to obtain 16 slices in each case. |
DenseNet: Input images | Batch size (2D/3D) | Input size (2D/3D) | Epoch (2D/3D) | Drop rate (2D/3D) |
Original chest HRCT images | 16/2 | 512 × 512/512 × 512 × 20* | 50/50 | 0.2/0.2 |
Fine selection (HRCT images) | ||||
Rough selection (HRCT images) | ||||
Rough selection (HRCT images) | ||||
Rough selection (HRCT images) | ||||
Multiple instance (HRCT images) | 16/2 | 512 × 512**/512 × 512 × 16*** | 50/50 | 0.2/0.2 |
Multiple instance (parenchyma) | ||||
Note:* Each case (a set of chest HRCT images) was equally divided into 20 segments, with one slice taken equidistantly to obtain 20 slices in each case. ** After rough selection, each case was equally divided into 10 bags, with one slice taken randomly to obtain 10 slices in each case. *** After rough selection, each case was equally divided into 16 bags, with one slice taken equidistantly to obtain 16 slices in each case. |
Definition | Name of the 19 selected lung radiomics features | Class | Coefficient |
Radiomics1 | original_shape_Elongation | Shape | 0.0056 |
Radiomics2 | original_shape_Maximum2DDiameterSlice | Shape | -0.0789 |
Radiomics3 | original_shape_Sphericity | Shape | 0.0624 |
Radiomics4 | log.sigma.1.0.mm.3D_firstorder_Maximum | First Order | 0.0665 |
Radiomics5 | log.sigma.1.0.mm.3D_glcm_ClusterProminence | GLCM 1 | -0.0425 |
Radiomics6 | log.sigma.1.0.mm.3D_glszm_ZoneEntropy | GLSZM 2 | 0.0394 |
Radiomics7 | log.sigma.2.0.mm.3D_firstorder_Maximum | First Order | 0.0129 |
Radiomics8 | log.sigma.2.0.mm.3D_ngtdm_Contrast | NGTDM 3 | -0.0318 |
Radiomics9 | log.sigma.2.0.mm.3D_gldm_DependenceVariance | GLDM 4 | -0.0136 |
Radiomics10 | log.sigma.4.0.mm.3D_firstorder_10Percentile | First Order | -0.0760 |
Radiomics11 | log.sigma.5.0.mm.3D_firstorder_10Percentile | First Order | -0.1669 |
Radiomics12 | wavelet.LLH_firstorder_RootMeanSquared | First Order | -0.0252 |
Radiomics13 | wavelet.HLH_firstorder_Mean | First Order | 0.0599 |
Radiomics14 | wavelet.HLH_glcm_Idmn | GLCM 1 | -0.0022 |
Radiomics15 | wavelet.HLH_ngtdm_Busyness | NGTDM 3 | 0.0444 |
Radiomics16 | wavelet.HHL_gldm_SmallDependenceLowGrayLevelEmphasis | GLDM 4 | -0.0168 |
Radiomics17 | wavelet.HHH_glszm_GrayLevelNonUniformityNormalized | GLSZM 2 | -0.0043 |
Radiomics18 | wavelet.LLL_firstorder_10Percentile | First Order | -0.5314 |
Radiomics19 | wavelet.LLL_glcm_Imc2 | GLCM 1 | 0.1383 |
Note: 1 Gray level co-occurrence matrix. 2 Gray level size zone matrix. 3 Neighboring gray tone difference matrix. 4 Gray level dependence matrix. |
Features | 0 vs. Ⅰ | 0 vs. Ⅱ | 0 vs. Ⅲ & Ⅳ | Ⅰ vs. Ⅱ | Ⅰ vs. Ⅲ & Ⅳ | Ⅱ vs. Ⅲ & Ⅳ |
Radiomics1 | < 0.0001 | < 0.0001 | < 0.0001 | 0.9999 (ns1) | < 0.0001 | < 0.0001 |
Radiomics2 | 0.0039 | 0.4406 (ns) | > 0.9999 (ns) | 0.5975 (ns) | < 0.0001 | 0.0164 |
Radiomics3 | 0.0004 | < 0.0001 | < 0.0001 | > 0.9999 (ns) | < 0.0001 | 0.0026 |
Radiomics4 | > 0.9999 (ns) | 0.0244 | 0.0016 | 0.0066 | 0.0004 | > 0.9999 (ns) |
Radiomics5 | 0.0009 | 0.4707 (ns) | 0.0243 | 0.2483 (ns) | > 0.9999 (ns) | > 0.9999 (ns) |
Radiomics6 | 0.8609 (ns) | < 0.0001 | < 0.0001 | 0.0016 | < 0.0001 | 0.7552 (ns) |
Radiomics7 | > 0.9999 (ns) | 0.1892 (ns) | 0.0005 | 0.2978 (ns) | 0.0013 | 0.3961 (ns) |
Radiomics8 | < 0.0001 | < 0.0001 | < 0.0001 | > 0.9999 (ns) | 0.0229 | 0.5546 (ns) |
Radiomics9 | 0.0021 | > 0.9999 (ns) | 0.6507 (ns) | 0.0026 | < 0.0001 | 0.6705 (ns) |
Radiomics10 | > 0.9999 (ns) | 0.0001 | < 0.0001 | < 0.0001 | < 0.0001 | 0.0045 |
Radiomics11 | 0.0626 (ns) | < 0.0001 | < 0.0001 | 0.0001 | < 0.0001 | 0.0055 |
Radiomics12 | < 0.0001 | 0.0006 | < 0.0001 | 0.4505 (ns) | 0.2677 (ns) | 0.0006 |
Radiomics13 | < 0.0001 | < 0.0001 | < 0.0001 | 0.1717 (ns) | < 0.0001 | 0.0800 (ns) |
Radiomics14 | > 0.9999 (ns) | > 0.9999 (ns) | > 0.9999 (ns) | > 0.9999 (ns) | 0.1873 (ns) | 0.6492 (ns) |
Radiomics15 | < 0.0001 | 0.0011 | < 0.0001 | > 0.9999 (ns) | 0.0878 (ns) | 0.0019 |
Radiomics16 | 0.7928 (ns) | 0.0077 | 0.0005 | 0.6650 (ns) | 0.1161 (ns) | 0.8141 (ns) |
Radiomics17 | > 0.9999 (ns) | 0.1001 (ns) | 0.0011 | > 0.9999 (ns) | 0.1153 (ns) | 0.9721 (ns) |
Radiomics18 | < 0.0001 | < 0.0001 | < 0.0001 | 0.1691 (ns) | < 0.0001 | < 0.0001 |
Radiomics19 | < 0.0001 | < 0.0001 | < 0.0001 | > 0.9999 (ns) | < 0.0001 | < 0.0001 |
Note: 1 ns: no significance. |
Features | 0 vs. Ⅰ | 0 vs. Ⅱ | 0 vs. Ⅲ & Ⅳ | Ⅰ vs. Ⅱ | Ⅰ vs. Ⅲ & Ⅳ | Ⅱ vs. Ⅲ & Ⅳ |
Radiomics-SHAPE | > 0.999 (ns1) | 0.4005 (ns) | < 0.0001 | 0.2587 (ns) | < 0.0001 | 0.0003 |
Radiomics-FIRST | < 0.0001 | < 0.0001 | < 0.0001 | 0.0003 | < 0.0001 | < 0.0001 |
Radiomics-GLCM | < 0.0001 | < 0.0001 | < 0.0001 | > 0.999 (ns) | < 0.0001 | < 0.0001 |
Radiomics-GLSZM | 0.9780 (ns) | < 0.0001 | < 0.0001 | 0.0010 | < 0.0001 | 0.7294 (ns) |
Radiomics-NGTDM | < 0.0001 | < 0.0001 | < 0.0001 | > 0.999 (ns) | < 0.0051 | 0.0211 |
Radiomics-GLDM | > 0.999 (ns) | 0.0111 | < 0.0001 | 0.0057 | < 0.0001 | 0.3038 (ns) |
Radiomics-ALL | < 0.0001 | < 0.0001 | < 0.0001 | 0.0006 | < 0.0001 | < 0.0001 |
Note:1 ns: no significance. |
DenseNet: Input images | Accuracy (2D/3D) | Precision (2D/3D) | Recall (2D/3D) | F1-score (2D/3D) |
Original chest HRCT images | 0.39/0.54 | 0.22/0.56 | 0.39/0.54 | 0.28/0.51 |
Fine selection (HRCT images) | 0.41/0.54 | 0.38/0.58 | 0.41/0.54 | 0.33/0.52 |
Rough selection (HRCT images) | 0.34/0.57 | 0.45/0.65 | 0.34/0.57 | 0.24/0.54 |
Multiple instance (HRCT images) | 0.40/0.59 | 0.32/0.62 | 0.40/0.59 | 0.32/0.57 |
Original parenchyma images | 0.47/0.58 | 0.47/0.61 | 0.47/0.58 | 0.43/0.58 |
Multiple instance (parenchyma) | 0.49/0.50 | 0.48/0.59 | 0.49/0.50 | 0.44/0.44 |
GoogleNet: Input images | Accuracy (2D/3D) | Precision (2D/3D) | Recall (2D/3D) | F1-score (2D/3D) |
Original chest HRCT images | 0.55/0.40 | 0.67/0.49 | 0.55/0.40 | 0.50/0.37 |
Fine selection (HRCT images) | 0.39/0.48 | 0.40/0.56 | 0.39/0.48 | 0.37/0.44 |
Rough selection (HRCT images) | 0.37/0.36 | 0.31/0.37 | 0.37/0.36 | 0.33/0.32 |
Multiple instance (HRCT images) | 0.39/0.38 | 0.37/0.36 | 0.39/0.38 | 0.28/0.33 |
Original parenchyma images | 0.55/0.39 | 0.56/0.47 | 0.55/0.39 | 0.55/0.34 |
Multiple instance (parenchyma) | 0.41/0.49 | 0.54/0.46 | 0.41/0.49 | 0.33/0.43 |
Classifier | Accuracy | Precision | Recall | F1-score | AUC |
RF classifier | 0.72 | 0.72 | 0.72 | 0.72 | 0.90 |
Ada classifier | 0.70 | 0.69 | 0.70 | 0.69 | 0.90 |
GB classifier | 0.72 | 0.73 | 0.72 | 0.72 | 0.92 |
MLP classifier | 0.78 | 0.78 | 0.78 | 0.78 | 0.92 |
LDA classifier | 0.60 | 0.60 | 0.60 | 0.59 | 0.85 |
SVM classifier | 0.62 | 0.62 | 0.63 | 0.61 | 0.87 |
RF classifier | 0.72 | 0.72 | 0.72 | 0.72 | 0.90 |
Classifier | Accuracy | Precision | Recall | F1-score | AUC |
RF classifier | 0.76 | 0.76 | 0.76 | 0.76 | 0.93 |
Ada classifier | 0.77 | 0.76 | 0.77 | 0.76 | 0.93 |
GB classifier | 0.73 | 0.74 | 0.73 | 0.73 | 0.92 |
MLP classifier | 0.80 | 0.80 | 0.80 | 0.80 | 0.94 |
LDA classifier | 0.65 | 0.65 | 0.65 | 0.65 | 0.89 |
SVM classifier | 0.71 | 0.71 | 0.71 | 0.71 | 0.92 |
RF classifier | 0.76 | 0.76 | 0.76 | 0.76 | 0.93 |
Radiomics-X | Accuracy | Precision | Recall | F1-score | AUC |
Radiomics-FIRST | 0.56 | 0.56 | 0.56 | 0.56 | 0.85 |
Radiomics-SHAPE | 0.39 | 0.40 | 0.39 | 0.36 | 0.64 |
Radiomics-GLCM | 0.49 | 0.51 | 0.49 | 0.47 | 0.72 |
Radiomics-GLSZM | 0.36 | 0.27 | 0.36 | 0.31 | 0.62 |
Radiomics-NGTDM | 0.42 | 0.32 | 0.42 | 0.36 | 0.66 |
Radiomics-GLDM | 0.28 | 0.21 | 0.28 | 0.24 | 0.58 |
Radiomics-ALL | 0.60 | 0.58 | 0.60 | 0.59 | 0.87 |
MLP Classifier: Input features | Accuracy | Precision | Recall | F1-score | AUC |
19 radiomics features + Radiomics-FIRST | 0.81 | 0.82 | 0.81 | 0.81 | 0.94 |
19 radiomics features + Radiomics-ALL | 0.83 | 0.83 | 0. 83 | 0.82 | 0.95 |