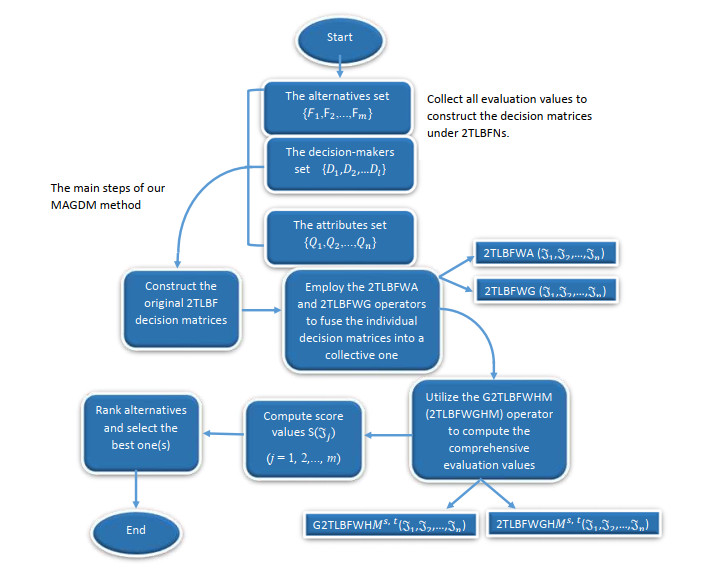
In this article, we introduce the 2-tuple linguistic bipolar fuzzy set (2TLBFS), a new strategy for dealing with uncertainty that incorporates a 2-tuple linguistic term into bipolar fuzzy set. The 2TLBFS is a better way to deal with uncertain and imprecise information in the decision-making environment. We elaborate the operational rules, based on which, the 2-tuple linguistic bipolar fuzzy weighted averaging (2TLBFWA) operator and the 2-tuple linguistic bipolar fuzzy weighted geometric (2TLBFWG) operator are presented to fuse the 2TLBF numbers (2TLBFNs). The Heronian mean (HM) operator, which can reflect the internal correlation between attributes and their influence on decision results, is integrated into the 2TLBF environment to analyze the effect of the correlation between decision factors on decision results. Initially, the generalized 2-tuple linguistic bipolar fuzzy Heronian mean (G2TLBFHM) operator and generalized 2-tuple linguistic bipolar fuzzy weighted Heronian mean (G2TLBFWHM) operator are proposed and properties are explained. Further, 2-tuple linguistic bipolar fuzzy geometric Heronian mean (2TLBFGHM) operator and 2-tuple linguistic bipolar weighted geometric Heronian mean (2TLBFWGHM) operator are proposed along with some of their desirable properties. Then, an approach to multi-attribute group decision-making (MAGDM) based on the proposed aggregation operators under the 2TLBF framework is developed. At last, a numerical illustration is provided for the selection of the best photovoltaic cell to demonstrate the use of the generated technique and exhibit its adequacy.
Citation: Sumera Naz, Muhammad Akram, Mohammed M. Ali Al-Shamiri, Mohammed M. Khalaf, Gohar Yousaf. A new MAGDM method with 2-tuple linguistic bipolar fuzzy Heronian mean operators[J]. Mathematical Biosciences and Engineering, 2022, 19(4): 3843-3878. doi: 10.3934/mbe.2022177
[1] | Muhammad Akram, Ayesha Khan, Uzma Ahmad, José Carlos R. Alcantud, Mohammed M. Ali Al-Shamiri . A new group decision-making framework based on 2-tuple linguistic complex $ q $-rung picture fuzzy sets. Mathematical Biosciences and Engineering, 2022, 19(11): 11281-11323. doi: 10.3934/mbe.2022526 |
[2] | Muhammad Akram, Usman Ali, Gustavo Santos-García, Zohra Niaz . 2-tuple linguistic Fermatean fuzzy MAGDM based on the WASPAS method for selection of solid waste disposal location. Mathematical Biosciences and Engineering, 2023, 20(2): 3811-3837. doi: 10.3934/mbe.2023179 |
[3] | Ayesha Khan, Muhammad Akram, Uzma Ahmad, Mohammed M. Ali Al-Shamiri . A new multi-objective optimization ratio analysis plus full multiplication form method for the selection of an appropriate mining method based on 2-tuple spherical fuzzy linguistic sets. Mathematical Biosciences and Engineering, 2023, 20(1): 456-488. doi: 10.3934/mbe.2023021 |
[4] | Han Wu, Junwu Wang, Sen Liu, Tingyou Yang . Research on decision-making of emergency plan for waterlogging disaster in subway station project based on linguistic intuitionistic fuzzy set and TOPSIS. Mathematical Biosciences and Engineering, 2020, 17(5): 4825-4851. doi: 10.3934/mbe.2020263 |
[5] | Ghous Ali, Adeel Farooq, Mohammed M. Ali Al-Shamiri . Novel multiple criteria decision-making analysis under $ m $-polar fuzzy aggregation operators with application. Mathematical Biosciences and Engineering, 2023, 20(2): 3566-3593. doi: 10.3934/mbe.2023166 |
[6] | Bo Sun, Ming Wei, Wei Wu, Binbin Jing . A novel group decision making method for airport operational risk management. Mathematical Biosciences and Engineering, 2020, 17(3): 2402-2417. doi: 10.3934/mbe.2020130 |
[7] | Tahir Mahmood, Zeeshan Ali, Kifayat Ullah, Qaisar Khan, Hussain AlSalman, Abdu Gumaei, Sk. Md. Mizanur Rahman . Complex pythagorean fuzzy aggregation operators based on confidence levels and their applications. Mathematical Biosciences and Engineering, 2022, 19(1): 1078-1107. doi: 10.3934/mbe.2022050 |
[8] | Muhammad Akram, Adeel Farooq, Maria Shabir, Mohammed M. Ali Al-Shamiri, Mohammed M. Khalaf . Group decision-making analysis with complex spherical fuzzy $ N $-soft sets. Mathematical Biosciences and Engineering, 2022, 19(5): 4991-5030. doi: 10.3934/mbe.2022234 |
[9] | Muhammad Akram, Tayyaba Ihsan, Tofigh Allahviranloo, Mohammed M. Ali Al-Shamiri . Analysis on determining the solution of fourth-order fuzzy initial value problem with Laplace operator. Mathematical Biosciences and Engineering, 2022, 19(12): 11868-11902. doi: 10.3934/mbe.2022554 |
[10] | Kaiyu Li, Xinxin Cai, Shuang Huang, Yuanbao Chen, Jinyang Li, Wenlin Wang . Research on the evaluation method of steam power system operation status based on the theory of deterioration degree and health value. Mathematical Biosciences and Engineering, 2023, 20(3): 4940-4969. doi: 10.3934/mbe.2023229 |
In this article, we introduce the 2-tuple linguistic bipolar fuzzy set (2TLBFS), a new strategy for dealing with uncertainty that incorporates a 2-tuple linguistic term into bipolar fuzzy set. The 2TLBFS is a better way to deal with uncertain and imprecise information in the decision-making environment. We elaborate the operational rules, based on which, the 2-tuple linguistic bipolar fuzzy weighted averaging (2TLBFWA) operator and the 2-tuple linguistic bipolar fuzzy weighted geometric (2TLBFWG) operator are presented to fuse the 2TLBF numbers (2TLBFNs). The Heronian mean (HM) operator, which can reflect the internal correlation between attributes and their influence on decision results, is integrated into the 2TLBF environment to analyze the effect of the correlation between decision factors on decision results. Initially, the generalized 2-tuple linguistic bipolar fuzzy Heronian mean (G2TLBFHM) operator and generalized 2-tuple linguistic bipolar fuzzy weighted Heronian mean (G2TLBFWHM) operator are proposed and properties are explained. Further, 2-tuple linguistic bipolar fuzzy geometric Heronian mean (2TLBFGHM) operator and 2-tuple linguistic bipolar weighted geometric Heronian mean (2TLBFWGHM) operator are proposed along with some of their desirable properties. Then, an approach to multi-attribute group decision-making (MAGDM) based on the proposed aggregation operators under the 2TLBF framework is developed. At last, a numerical illustration is provided for the selection of the best photovoltaic cell to demonstrate the use of the generated technique and exhibit its adequacy.
With the steady depletion of non-renewable resources like crude oil, natural gas, coal, and rising pollution levels, the development of solar photovoltaic is expanding, especially after the COVID-19 pandemic. Photovoltaic cells are commonly used as an economical and reliable energy source in different fields all across the world. Solar photovoltaic has become a sustainable and cost-effective energy alternative for company owners and has a significant reduction in the cost of purchasing and installing solar systems. Renewable energy has been associated with continuous development and a significant effort to select the alternative based on the supplier demands, since its beginnings. Depending on the manufacturing process, different types of photovoltaic cells are currently available. It is necessary to assess photovoltaic technologies on the market, one step in this direction was to build a MAGDM tool to assist decision-makers (DMs) in selecting the most appropriate photovoltaic technology.
Decision-making is an activity that people perform regularly in their daily lives [1,2]. The goal of decision-making is to classify the various possibilities according to the level of reliability for DMs. The situation in which individuals collectively choose the best alternative is known as group decision-making or MAGDM. The decision is then no longer attributable to any single group member, this is because the decision is influenced by all people and social group processes. Individual decisions are mostly different from group decisions. The MAGDM is a decision-making strategy that is treated as a logical and reasonable human activity. Due to the increased complexity of the environment in decision analysis and the problem itself, DMs are periodically provided with numerical data to demonstrate decision-making information. The fuzzy set (FS) theory introduced by Zadeh [3] is the modified version of the crisp sets. In fact, FS expresses the importance of the fuzzy value and this value lies between $ [0, 1] $. FS tells the membership degree (MD) of an object. Intuitionistic fuzzy set (IFS) was introduced by Atanassov [4] which is generalization of FS. IFS has two functions, MD and non-membership degree (NMD) functions, and both functions map on a closed unit interval from a non-empty set. The IFS has a condition that the sum of both degrees must belong to $ [0, 1] $. Zhang [5] proposed a bipolar fuzzy set (BFS) as an extension of FS, which is represented by two components: a degree of positive membership function that belongs to $ [0, 1] $ and a degree of negative membership function that belongs to $ [-1, 0] $.
BFS has recently gained popularity as a useful approach for resolving uncertainty in decision-making. Akram et al. [6] developed the BFNS-TOPSIS methods for the solution of multi-attribute decision-making and MAGDM problems defined by BF N-soft information. Zhao et al. [7] established a BF interactive multi-attribute decision-making strategy known as TODIM method depending on the cumulative prospect theory TODIM model for dealing with the MAGDM issues. Certain bipolar fuzzy graphs with applications are discussed in [8]. Poulik et al. [9] proposed the Wiener index for a BF graph, and the Wiener absolute index is dependent on the total accurate connectivity between all pairs of vertices and in the entire bipolar fuzzy graph. Akram et al. [10] proposed a method for solving the LR-bipolar fuzzy linear system, the LR-complex BF linear system with complex coefficients, and the LR-complex BF linear system with real coefficients. Further, Akram et al. [11,12,13] developed many decision-making methods utilizing BFSs and its extensions. Naz and Akram [14,15] developed a new DM approach to deal with the MADM problems based on graph theory. Further, Akram et al. [16,17,18,19,20] introduced several DM methods under generalized fuzzy scenario.
People like to express language such as "excellent", "good", "medium", "poor", and "very poor" to evaluate some attributes of the evaluation object in MAGDM consequently, the research of information aggregation operators using language as attribute value is significantly essential. The 2-tuple linguistic (2TL) term is an effective tool, which can avoid the loss of information and get more accurate evaluation results. The 2TL representation model, firstly introduced by Herrera and Martinez [21,22], is one of the most crucial approaches to deal with linguistic decision-making issues. Later on, several 2TL aggregation operators and decision-making approaches have been developed. Under the 2TL Pythagorean fuzzy sets, Zhang et al. [23] enhanced the standard cumulative prospect theory and TODIM method based on past study of researches. In the context of intuitionistic 2TL data, Faizi et al. [24] proposed two strategies, namely Linear best-worst method (BWM) and Euclidean BWM to the BWM, to produce superior attribute importance weights for MAGDM circumstances. To estimate correctness and generate explainable outputs, Labella et al. [25] used 2-tuple BWM to mitigate the amount of paired comparisons in MAGDM circumstances and quantify the uncertainties connected with them. For addressing MAGDM issues, Zhao et al. [26] developed the enhanced TODIM approach, which is dependent on 2TL neutrosophic sets and cumulative prospect theory. He et al. [27] introduced a combination procedure QUALIFLEX method and the Pythagorean 2TL fuzzy set to assess the effectiveness of management employees in infrastructure tasks, employing the traditional QUALIFLEX decision-making strategy and Pythagorean 2TL fuzzy numbers to convey DMs assessment on every strategy.
The Heronian mean (HM) is a powerful aggregation operator that demonstrates the aggregated parameter's interrelationships. Initially, Beliakov [28] demonstrated that the HM is an aggregation operator. Ayub et al. [29] developed a new family of cubic fuzzy HM Dombi (HMD) operators, including cubic fuzzy HMD, cubic fuzzy weighted HMD, cubic fuzzy geometric HMD, and cubic fuzzy weighted geometric HMD aggregation operators. Lin et al. [30] introduced the partitioned geometric HM operator and partitioned HM (PHM) operator, some picture fuzzy interactional PHM (PFIPHM), geometric PFIPHM operators, and its weighted forms. Deveci et al. [31] proposed a novel extension of the combined compromise solution methodology that incorporates the power Heronian function and logarithmic method. Pamucar et al. [32] utilized the weighted power Heronian and weighted geometric power Heronian functions to enhance the traditional weighted aggregated sum product assessment technique. Garg et al. [33] developed complex intuitionistic uncertain linguistic (CIUL) arithmetic HM, CIUL weighted arithmetic HM, CIUL geometric HM, and CIUL weighted geometric HM by combining the HM and the CIUL concepts. Liu et al. [34] introduced and investigated the neutrosophic cubic power Heronian and neutrosophic cubic power weighted Heronian aggregation operators.
BFS has a significant advantage when it comes to explaining decision information in MAGDM situations for DMs. Many real-world events are dependent on opposite affect, such as positive affect and negative affect, plausibility and preference consistency, neutrality and indifference, and so on. BFS plays a vital role in handling these types of situations when two opposite directional concepts are involved. When there is uncertainty due to fuzziness and vagueness, the use of fuzzy linguistic information to assess alternatives in unipolar scales might help to express DM's preferences for alternatives. However, in some situations, DMs must represent negative and positive perspectives that cannot be represented by unipolar scales. The goal of this study is to build an adaptive linguistic MAGDM model for bipolar linguistic scales in which both alternatives and attributes can change over time. People frequently use language to evaluate object attributes in actual MAGDM, and there are sometimes relationships between the attributes. As a result, the study of MAGDM with language as attributes and relationships between attributes are theoretically significant and practically beneficial. In MAGDM situations, aggregation operators are frequently used. Average and geometric aggregation operators are the two types of aggregation operators. These aggregation operators have in common that they emphasize the relevance of each attribute, but they are unable to express the interrelationships between the individual data. The HM operator is a significant operator that takes into account attribute interrelationships. This study intends to introduce the notion of the 2TLBFHM operator, because there is no published article on the 2TLBFS based on HM operator.
The following are some of the aspects of this research article that are unique:
1) We present the 2TLBFS as a novel innovation in FS theory for addressing data complexity. The 2TLBFS combines the benefits of both 2TL terms and BFS, increasing the adaptability of the BFS.
2) To cope with group decision-making situations in which the attributes have interrelationships, we propose a family of HM aggregation operators for 2TLBFS, such as G2TLBFHM, G2TLBFWHM, 2TLBFGHM, and 2TLBFWGHM operators.
3) Certain formal definitions, theorems, and properties of the suggested information aggregation operators are derived under the current conditions.
4) To rank the alternatives, a novel MAGDM approach is presented, which is based on the G2TLBFWHM and 2TLBFWGHM operators to integrate DM's evaluation preferences.
5) To demonstrate the applicability and robustness of the proposed method, an illustrative example for selecting a cost-effective solution of photovoltaic cells is presented.
To achieve this goal, the structure of this paper is arranged as follows: Section 2 introduces some initial concepts related to 2TL terms, BFSs, and HM aggregation operators. In Section 3, a new information representation form, i.e., 2TLBFS is defined, along with its basic theories, such as some basic operational rules, score function, and accuracy function of 2TLBFNs. Further, the 2TLBF weighted averaging and weighted geometric operators are developed. In Section 4, we propose a family of HM aggregation operators, including the G2TLBFHM, G2TLBFWHM, 2TLBFGHM, and 2TLBFWGHM operators along with some of its essential properties. Section 5 presents MAGDM model based on the proposed G2TLBFWHM and 2TLBFWGHM operators. Section 6 provides a numerical illustration of the approach presented in this article for selecting the optimal photovoltaic cell using 2TLBFNs. Finally, in Section 7, we summarize the paper.
Definition 2.1. [5] Let $ L $ be a fixed set. A bipolar fuzzy set (BFS) $ \mathfrak{B} $ in $ L $ is given as
$ \begin{equation} \mathfrak{B} = \{\langle\ell, (\varpi^{+}_{\mathfrak{B}}(\ell), \eta^{-}_{\mathfrak{B}}(\ell))\rangle|\ell\in L\} \end{equation} $ | (2.1) |
where the positive MD function $ \varpi^{+}_{\mathfrak{B}}(\ell):L\rightarrow [0, 1] $ represents the satisfaction degree of an element $ \ell $ to the property and negative MD function $ \eta^{-}_{\mathfrak{B}}(\ell):L\rightarrow [-1, 0] $ represents the satisfaction degree of an element $ \ell $ to some implicit counter property corresponding to a BFS $ \mathfrak{B} $, respectively, and, for every $ \ell\in L $. Let $ {\text{ ȷ }} = (\varpi^{+}, \eta^{-}) $ be a BF number (BFN).
Definition 2.2. Let $ {\text{ ȷ }} = (\varpi^{+}, \eta^{-}), \; {\text{ ȷ }}_{1} = (\varpi^{+}_{1}, \eta^{-}_{1}) $ and $ {\text{ ȷ }}_{2} = (\varpi^{+}_{2}, \eta^{-}_{2}) $ be three BFNs, then the basic operations are as follows:
(1) $ {\text{ ȷ }}_{1}\oplus{\text{ ȷ }}_{2} = (\varpi^{+}_{1}+\varpi^{+}_{2}-\varpi^{+}_{1}\varpi^{+}_{2}, -|\eta^{-}_{1}||\eta^{-}_{2}|) $;
(2) $ {\text{ ȷ }}_{1}\otimes{\text{ ȷ }}_{2} = (\varpi^{+}_{1}\varpi^{+}_{2}, -(|\eta^{-}_{1}|+|\eta^{-}_{2}|)+|\eta^{-}_{1}||\eta^{-}_{2}|) $;
(3) $ \lambda{\text{ ȷ }} = (1-(1-\varpi^{+})^{\lambda}, -|\eta^{-}|^{\lambda}), {\lambda} > 0 $;
(4) $ ({\text{ ȷ }})^{\lambda} = ((\varpi^{+})^{\lambda}, -1+|1+\eta^{-}|^{\lambda}), {\lambda} > 0 $;
(5) $ {\text{ ȷ }}^{c} = (1-\varpi^{+}, |\eta^{-}|-1) $;
(6) $ {\text{ ȷ }}_{1}\subseteq{\text{ ȷ }}_{2} $ if and only if $ \varpi^{+}_{1}\leq\varpi^{+}_{2} $ and $ \eta^{-}_{1}\geq\eta^{-}_{2} $;
(7) $ {\text{ ȷ }}_{1}\cup{\text{ ȷ }}_{2} = (\max\{\varpi^{+}_{1}, \varpi^{+}_{2}\}, \min\{\eta^{-}_{1}, \eta^{-}_{2}\}) $;
(8) $ {\text{ ȷ }}_{1}\cap{\text{ ȷ }}_{2} = (\min\{\varpi^{+}_{1}, \varpi^{+}_{2}\}, \max\{\eta^{-}_{1}, \eta^{-}_{2}\}) $.
Definition 2.3. Let $ S = \{\flat_{\jmath}|\jmath = 1, \ldots, \sigma\} $ be a linguistic term set (LTS) and $ \varphi \in [1, \sigma] $ be a number value representing the aggregation result of linguistic symbolic[21]. Then the function $ \Delta $ used to obtain the 2TL information equivalent to $ \varphi $ is defined as:
$ \begin{eqnarray*} \Delta:[1, \sigma]\rightarrow S\times [-\frac{1}{2}, \frac{1}{2}), \end{eqnarray*} $ |
$ \begin{eqnarray} \Delta(\varphi) = \begin{cases} \flat_{\jmath}, \jmath = \text{round}(\varphi)\\ \upsilon = \varphi-\jmath, \; \upsilon \in [-\frac{1}{2}, \frac{1}{2}). \end{cases} \end{eqnarray} $ | (2.2) |
Definition 2.4. Let $ S = \{\flat_{\jmath}|\jmath = 1, \ldots, \sigma\} $ be a LTS and $ (\flat_{\jmath}, \upsilon_{\jmath}) $ be a 2-tuple[21], there exists a function $ \Delta^{-1} $ that restore the 2-tuple to its equivalent numerical value $ \varphi \in [1, \sigma]\subset R, $ where
$ \begin{eqnarray} \Delta^{-1}: S\times[-\frac{1}{2}, \frac{1}{2})\rightarrow [1, \sigma], \\ \Delta^{-1}(\flat_{\jmath}, \upsilon) = \jmath+\upsilon = \varphi. \end{eqnarray} $ | (2.3) |
Definition 2.5. Let $ a_{{{k}}}({{k}} = 1, 2, \ldots, \mathfrak{n}) $ be a group of non-negative numbers, if[28]
$ \begin{equation} \text{HM}{(a_{1}, a_{2}, \ldots, a_{n})} = \frac{2}{n(n+1)}\sum\limits_{{{j}} = 1}^{n}\sum\limits_{{{k}} = {{j}}}^{n}\sqrt{a_{{{j}}}a_{{{k}}}} \end{equation} $ | (2.4) |
then HM is known as Heronian mean (HM) operator.
Based on Heronian mean, Yu [36] introduced the generalized Heronian mean (GHM) as follows:
Definition 2.6. Let $ s, t > 0 $ and $ a_{{{k}}} ({{k}} = 1, 2, \ldots, \mathfrak{n}) $ be a group of non-negative numbers[36]. If
$ \begin{equation} \text{GHM}^{s, t}{(a_{1}, a_{2}, \ldots, a_{n})} = \left(\frac{2}{n(n+1)}\sum\limits_{{{j}} = 1}^{n}\sum\limits_{{{k}} = {{j}}}^{n}{a^{s}_{{{j}}}a^{t}_{{{k}}}}\right)^{\frac{1}{s+t}} \end{equation} $ | (2.5) |
then $ GHM^{s, t} $ is called GHM operator. The GHM operator decreases the HM operator when $ s = t = \frac{1}{2} $. Yu [36] then introduced the geometric HM operator, which is as follows:
Definition 2.7. Let $ s, t > 0 $ and $ a_{{{k}}}({{k}} = 1, 2, \ldots, \mathfrak{n}) $ be a group of non-negative real numbers[36], then
$ \begin{equation} \text{GHM}^{s, t}{(a_{1}, a_{2}, \ldots, a_{n})} = \frac{1}{s+t}\left(\prod\limits_{{{j}} = 1, {{k}} = {{j}}}^{n}(s a_{{{j}}}+t a_{{{k}}})\right)^{\frac{2}{n(n+1)}} \end{equation} $ | (2.6) |
then $ GHM^{s, t} $ is called the geometric HM (GHM) operator.
In this section, by integrating BFS and 2TL terms, we introduce the new generalization of BFS, called 2TLBFS. Further, we develop the 2TLBFWA and 2TLBFWG operators and discuss their desirable properties.
Definition 3.1. Let $ S = \{\flat_{0}, \flat_{1}, \flat_{2}, \ldots \flat_{\sigma}\} $ be a 2TL term set with odd cardinality $ \sigma+1 $. If $ ((\flat_l, £), (\flat_n, \aleph)) $ is defined for $ \flat_{l}, \flat_{n} \in S $ and $ £, \aleph\in[-\frac{1}{2}, \frac{1}{2}) $ where $ (\flat_{l}, £) $ and $ (\flat_{n}, \aleph) $ express independently the MD and NMD by 2TLSs, then 2TLBFS is defined as follows:
$ \mathfrak{F}_{{{k}}} = ((\flat_{l_{{{k}}}}, £_{{{k}}}), (\flat_{n_{{{k}}}}, \aleph_{{{k}}})), $ |
where $ 0\leq\Delta^{-1}(\flat_{l_{{{k}}}}, £_{{{k}}})\leq \sigma, 0\leq\Delta^{-1}(\flat_{n_{{{k}}}}, \aleph_{{{k}}})\leq \sigma $.
Definition 3.2. Let $ \mathfrak{F} = ((\flat_l, £), (\flat_n, \aleph)) $ be a 2TLBFN. Then the score and accuracy functions of $ \mathfrak{F} $ are defined as:
$ \begin{equation} \mathcal{S}(\mathfrak{F}) = \Delta\left(\frac{\sigma}{2}\left(1+\left(\frac{\Delta^{-1}(\flat_{l}, £)}{\sigma}\right)-\left(\frac{\Delta^{-1}(\flat_{n}, \aleph)}{\sigma}\right)\right)\right), \; \; \; \mathcal{S}(\mathfrak{F})\in [0, \sigma] \end{equation} $ | (3.1) |
$ \begin{equation} \beth(\mathfrak{F}) = \Delta\left(\sigma\left(\left(\frac{\Delta^{-1}(\flat_{l}, £)}{\sigma}\right)+\left(\frac{\Delta^{-1}(\flat_{n}, \aleph)}{\sigma}\right)\right)\right), \; \; \; \beth(\mathfrak{F})\in [0, \sigma] \end{equation} $ | (3.2) |
Definition 3.3. Let $ \mathfrak{F}_1 = ((\flat_{l_{1}}, £_{1}), (\flat_{n_{1}}, \aleph_{1})) $ and $ \mathfrak{F}_2 = ((\flat_{l_{2}}, £_{2}), (\flat_{n_{2}}, \aleph_{2})) $ be two 2TLBFNs, then we have
(1) $ \mathcal{S}(\mathfrak{F}_{1}) < \mathcal{S}(\mathfrak{F}_{2}) $, then $ \mathfrak{F}_{1} < \mathfrak{F}_{2} $;
(2) $ \mathcal{S}(\mathfrak{F}_{1}) > \mathcal{S}(\mathfrak{F}_{2}) $, then $ \mathfrak{F}_{1} > \mathfrak{F}_{2} $;
(3) $ \mathcal{S}(\mathfrak{F}_{1}) = \mathcal{S}(\mathfrak{F}_{2}) $, $ \beth(\mathfrak{F}_{1}) < \beth(\mathfrak{F}_{2}) $, then $ \mathfrak{F}_{1} < \mathfrak{F}_{2} $;
(4) $ \mathcal{S}(\mathfrak{F}_{1}) = \mathcal{S}(\mathfrak{F}_{2}) $, $ \beth(\mathfrak{F}_{1}) > \beth(\mathfrak{F}_{2}) $, then $ \mathfrak{F}_{1} > \mathfrak{F}_{2} $;
(5) $ \mathcal{S}(\mathfrak{F}_{1}) = \mathcal{S}(\mathfrak{F}_{2}) $, $ \beth(\mathfrak{F}_{1}) = \beth(\mathfrak{F}_{2}) $, then $ \mathfrak{F}_{1} = \mathfrak{F}_{2} $.
Definition 3.4. Let $ \mathfrak{F}_{1} = ((\flat_{l_{1}}, £_{1}), (\flat_{n_{1}}, \aleph_{1})) $ and $ \mathfrak{F}_{2} = ((\flat_{l_{2}}, £_{2}), (\flat_{n_{2}}, \aleph_{2})) $ be two 2TLBFNs, then
(1) $ \mathfrak{F}_{1}\oplus \mathfrak{F}_{2} = { \left(\begin{array}{l} \Delta\left(\sigma\left(1-\left(1-\left(\frac{\Delta^{-1}(\flat_{l_{1}}, £_{1})}{\sigma}\right)\right) \left(1-\left(\frac{\Delta^{-1}(\flat_{l_{2}}, £_{2})}{\sigma}\right)\right)\right) \right), \; \Delta\left(\sigma\left(\frac{\Delta^{-1}(\flat_{n_{1}}, \aleph_{1})}{\sigma}\right)\left(\frac{\Delta^{-1}(\flat_{n_{2}}, \aleph_{2})}{\sigma}\right)\right) \end{array}\right)} $;
(2) $ \mathfrak{F}_{1}\otimes \mathfrak{F}_{2} = { \left(\begin{array}{l} \Delta\left(\sigma\left(\frac{\Delta^{-1}(\flat_{l_{1}}, £_{1})}{\sigma}\right)\left(\frac{\Delta^{-1}(\flat_{l_{2}}, £_{2})}{\sigma}\right)\right), \; \Delta\left(\sigma\left(1-\left(1-\left(\frac{\Delta^{-1}(\flat_{n_{1}}, \aleph_{1})}{\sigma}\right)\right) \left(1-\left(\frac{\Delta^{-1}(\flat_{n_{2}}, \aleph_{2})}{\sigma}\right)\right)\right)\right) \end{array}\right)} $;
(3) $ \lambda \mathfrak{F}_1 = { \left(\begin{array}{l} { \Delta\left(\sigma\left(1-\left(1-\left(\frac{\Delta^{-1}(\flat_{l_1}, £_1)}{\sigma}\right)\right)^{\lambda}\right)\right)}, \Delta\left(\sigma\left(\frac{\Delta^{-1}(\flat_{n_1}, \aleph_1)}{\sigma}\right)^{\lambda}\right) \end{array}\right)}, \; \lambda > 0 $;
(4) $ \mathfrak{F}_1^{\lambda} = { \left(\begin{array}{l} \Delta\left(\sigma\left(\frac{\Delta^{-1}(\flat_{l_1}, £_1)}{\sigma}\right)^{\lambda}\right), { \Delta\left(\sigma\left(1-\left(1-\left(\frac{\Delta^{-1}(\flat_{n_1}, \aleph_1)}{\sigma}\right)\right)^{\lambda}\right)\right)} \end{array}\right)}, \; \lambda > 0 $.
In this subsection, the two types of weighted aggregation operators are introduced: the 2TLBFWA operator and the 2TLBFWG operator.
Definition 3.5. Let $ \mathfrak{F}_{{{k}}} = ((\flat_{l_{{{k}}}}, £_{{{k}}}), (\flat_{n_{{{k}}}}, \aleph_{{{k}}})) ({{k}} = 1, 2, \ldots, \mathfrak{n}) $ be a collection of 2TLBFNs. The 2TLBFWA operator is a mapping $ \mathcal{P}^{n}\rightarrow \mathcal{P} $ such that
$ \begin{eqnarray*} \text{2TLBFWA}(\mathfrak{F}_{1}, \mathfrak{F}_{2}, \ldots, \mathfrak{F}_{n}) = \oplus_{{{k}} = 1}^{n}\varkappa^{\star}_{{{k}}}\mathfrak{F}_{{{k}}} \end{eqnarray*} $ |
where $ \varkappa^{\star} = (\varkappa^{\star}_{1}, \varkappa^{\star}_{2}, \ldots, \varkappa^{\star}_{n})^{T} $ is the weight vector of $ \mathfrak{F}_{{{k}}} ({{k}} = 1, 2, \ldots, \mathfrak{n}) $, such that $ \varkappa^{\star}_{{{k}}}\in[0, 1] $ and $ \sum\limits_{{{k}} = 1}^{n}\varkappa^{\star}_{{{k}}} = 1. $
Theorem 3.1. Let $ \mathfrak{F}_{{{k}}} = ((\flat_{l_{{{k}}}}, £_{{k}}), (\flat_{n_{{{k}}}}, \aleph_{{k}}))({{k}} = 1, 2, \ldots, \mathfrak{n}) $ be a collection of 2TLBFNs with weight vector $ \varkappa^{\star} = (\varkappa^{\star}_{1}, \varkappa^{\star}_{2}, \ldots, \varkappa^{\star}_{n})^{T} $, thereby satisfying $ \varkappa^{\star}_{{k}} \in [0, 1] $ and $ \sum\limits_{{{k}} = 1}^{n}\varkappa^{\star}_{{k}} = 1 $ $ ({{k}} = 1, 2, \ldots, \mathfrak{n}) $. Then, their aggregation value by the 2TLBFWA operator is still a 2TLBFN, and
$ \begin{eqnarray} 2TLBFWA (\mathfrak{F}_{1}, \mathfrak{F}_{2}, \ldots, \mathfrak{F}_{n}) = \left( \Delta\left(\sigma\left(1-\prod\limits_{{{k}} = 1}^{n}\left(1-\left(\frac{\Delta^{-1}(\flat_{l_{{{k}}}}, £_{{k}})}{\sigma}\right)\right)^{\varkappa^{\star}_{{{k}}}}\right)\right), \Delta\left({\sigma}\prod\limits_{{{k}} = 1}^{n}\left(\frac{\Delta^{-1}(\flat_{n_{{{k}}}}, \aleph_{{k}})}{\sigma}\right)^{\varkappa^{\star}_{{{k}}}}\right) \right). \end{eqnarray} $ | (3.3) |
Proof. we prove that the Eq (3.3) holds by using mathematical induction method for positive integer $ n $.
$ \bf(a) $ when $ n = 1 $, we have
$ \varkappa^{\star}_{1}\mathfrak{F}_{{1}} = \left(\begin{array}{l}\Delta\left(\sigma\left(1-\left(1-\left(\frac{\Delta^{-1}(\flat_{l_{1}}, £_{1})}{\sigma}\right)\right)^{\varkappa^{\star}_{1}}\right)\right), \Delta\left(\sigma\left(\frac{\Delta^{-1}(\flat_{n_{1}}, \aleph_{1})}{\sigma}\right)^{\varkappa^{\star}_{1}}\right) \end{array}\right). $
Thus, Eq (3.3) holds for $ n = 1 $.
$\bf (b) $ Suppose that Eq (3.3) holds for $ n = m $,
$ \begin{eqnarray*} \text{2TLBFWA}(\mathfrak{F}_{1}, \mathfrak{F}_{2}, \ldots, \mathfrak{F}_{m}) = \left(\begin{array}{l}\Delta\left({\sigma} \left( 1-\prod\limits_{{{k}} = 1}^{m}\left(1-\left(\frac{\Delta^{-1}(\flat_{l_{{{k}}}}, £_{{k}})}{\sigma}\right)\right)^{\varkappa^{\star}_{{{k}}}}\right)\right), \Delta\left({\sigma}\prod\limits_{{{k}} = 1}^{m}\left(\frac{\Delta^{-1}(\flat_{n_{{{k}}}}, \aleph_{{k}})}{\sigma}\right)^{\varkappa^{\star}_{{{k}}}}\right) \end{array}\right). \end{eqnarray*} $ |
Then, when $ n = m+1 $, by inductive assumption, we have
$ \begin{eqnarray*} \text{2TLBFWA}(\mathfrak{F}_{1}, \mathfrak{F}_{2}, \ldots, \mathfrak{F}_{m}, \mathfrak{F}_{m+1}) = \text{2TLBFWA}(\mathfrak{F}_{1}, \mathfrak{F}_{2}, \ldots, \mathfrak{F}_{m})\oplus \varkappa^{\star}_{m+1}\mathfrak{F}_{m+1}\\ = \left(\begin{array}{l}\Delta\left(\sigma\left(1-\prod\limits_{{{k}} = 1}^{m}\left(1-\left(\frac{\Delta^{-1}(\flat_{l_{{{k}}}}, £_{{k}})}{\sigma}\right)\right)^{\varkappa^{\star}_{{{k}}}}\right)\right), \Delta\left({\sigma}\prod\limits_{{{k}} = 1}^{m}\left(\frac{\Delta^{-1}(\flat_{n_{{{k}}}}, \aleph_{{k}})}{\sigma}\right)^{\varkappa^{\star}_{{{k}}}}\right) \end{array}\right)\\ \oplus\left(\begin{array}{l}\Delta\left(\sigma\left(1-\left(1-\left(\frac{\Delta^{-1}(\flat_{l_{m+1}}, £_{m+1})}{\sigma}\right)\right)^{\varkappa^{\star}_{m+1}}\right)\right), \Delta\left(\sigma\left(\frac{\Delta^{-1}(\flat_{n_{m+1}}, \aleph_{m+1})}{\sigma}\right)^{\varkappa^{\star}_{m+1}}\right) \end{array}\right).\\ = \left(\begin{array}{l} \Delta\left(\sigma\left(1-\prod\limits_{{{k}} = 1}^{m+1}\left(1-\left(\frac{\Delta^{-1}(\flat_{l_{{{k}}}}, £_{{k}})}{\sigma}\right)\right)^{\varkappa^{\star}_{{{k}}}}\right)\right), \Delta\left({\sigma}\prod\limits_{{{k}} = 1}^{m+1}\left(\frac{\Delta^{-1}(\flat_{n_{{{k}}}}, \aleph_{{k}})}{\sigma}\right)^{\varkappa^{\star}_{{{k}}}}\right) \end{array}\right). \end{eqnarray*} $ |
Therefore, Eq (3.3) holds for positive integer $ n = m+1 $. Thus, by mathematical induction method, we know that Eq (3.3) holds for any $ n\geq1 $.
Theorem 3.2. Let $ \mathfrak{F}_{{k}} = ((\flat_{l_{{{k}}}}, £_{{k}}), (\flat_{n_{{{k}}}}, \aleph_{{k}})), \; \mathfrak{F}'_{{k}} = ((\flat'_{l_{{{k}}}}, £'_{{k}}), (\flat'_{n_{{{k}}}}, \aleph'_{{k}})) ({{k}} = 1, 2, \ldots, \mathfrak{n}) $ be two sets of 2TLBFNs, then the 2TLBFWA operator has the following properties:
(1) (Idempotency) If all $ \mathfrak{F}_{{k}} = ((\flat_{l_{{{k}}}}, £_{{k}}), (\flat_{n_{{{k}}}}, \aleph_{{k}}))({{k}} = 1, 2, \ldots, \mathfrak{n}) $ are equal, for all $ {{k}} = 1, 2, \ldots, \mathfrak{n} $, then
$ \begin{eqnarray*} && 2TLBFWA (\mathfrak{F}_{1}, \mathfrak{F}_{2}, \ldots, \mathfrak{F}_{n}) = \mathfrak{F}. \end{eqnarray*} $ |
(2) (Monotonicity)If $ \mathfrak{F}_{{k}}\leq \mathfrak{F}'_{{k}} $, for all $ {{k}} $, then
$ \begin{eqnarray*} 2TLBFWA (\mathfrak{F}_{1}, \mathfrak{F}_{2}, \ldots, \mathfrak{F}_{n})\leq 2TLBFWA (\mathfrak{F}'_{1}, \mathfrak{F}'_{2}, \ldots, \mathfrak{F}'_{n}). \end{eqnarray*} $ |
(3) (Boundedness)If $ \mathfrak{F}_{{k}} = ((\flat_{l_{{{k}}}}, £_{{k}}), (\flat_{n_{{{k}}}}, \aleph_{{k}}))({{k}} = 1, 2, \ldots, \mathfrak{n}) $ be a collection of 2TLBFNs, and let $ \mathfrak{F}^{-} = (\min_{{k}}(\flat_{l_{{{k}}}}, £_{{k}}), \max_{{k}}(\flat_{n_{{{k}}}}, \aleph_{{k}})) $ and $ \mathfrak{F}^{+} = (\max_{{k}}(\flat_{l_{{{k}}}}, £_{{k}}), \min_{{k}}(\flat_{n_{{{k}}}}, \aleph_{{k}})) $, then
$ \begin{eqnarray*} &&\mathfrak{F}^{-}\leq 2TLBFWA (\mathfrak{F}_{1}, \mathfrak{F}_{2}, \ldots, \mathfrak{F}_{n})\leq \mathfrak{F}^{+}. \end{eqnarray*} $ |
Definition 3.6. Let $ \mathfrak{F}_{{k}} = ((\flat_{l_{{{k}}}}, £_{{k}}), (\flat_{n_{{{k}}}}, \aleph_{{k}}))({{k}} = 1, 2, \ldots, \mathfrak{n}) $ be a collection of 2TLBFNs. The 2TLBFWG operator is a mapping $ \mathcal{P}^{n}\rightarrow \mathcal{P}, $ such that
$ \begin{eqnarray*} \text{2TLBFWG}(\mathfrak{F}_{1}, \mathfrak{F}_{2}, \ldots, \mathfrak{F}_{n}) = \otimes_{{{k}} = 1}^{n}\mathfrak{F}_{{k}}^{\varkappa^{\star}_{{{k}}}} \end{eqnarray*} $ |
where $ \varkappa^{\star} = (\varkappa^{\star}_{1}, \varkappa^{\star}_{2}, \ldots, \varkappa^{\star}_{n})^{T} $ is the weight vector of $ \mathfrak{F}_{{k}} ({{k}} = 1, 2, \ldots, \mathfrak{n}) $, such that $ \varkappa^{\star}_{{k}}\in[0, 1] $ and $ \sum\limits_{{{k}} = 1}^{n}\varkappa^{\star}_{{k}} = 1. $
Theorem 3.3. Let $ \mathfrak{F}_{{k}} = ((\flat_{l_{{{k}}}}, £_{{k}}), (\flat_{n_{{{k}}}}, \aleph_{{k}}))({{k}} = 1, 2, \ldots, \mathfrak{n}) $ be a collection of 2TLBFNs with weight vector $ \varkappa^{\star} = (\varkappa^{\star}_{1}, \varkappa^{\star}_{2}, \ldots, \varkappa^{\star}_{n})^{T} $, thereby satisfying $ \varkappa^{\star}_{{k}} \in [0, 1] $ and $ \sum\limits^{n}_{{{k}} = 1}\varkappa^{\star}_{{k}} = 1 $ $ ({{k}} = 1, 2, \ldots, \mathfrak{n}) $. Then, their aggregation value by the 2TLBFWG operator is still a 2TLBFN, and
$ \begin{eqnarray} 2TLBFWG (\mathfrak{F}_{1}, \mathfrak{F}_{2}, \ldots, \mathfrak{F}_{n}) = \left(\begin{array}{l} \Delta\left({\sigma}\prod\limits_{{{k}} = 1}^{n}\left(\frac{\Delta^{-1}(\flat_{l_{{{k}}}}, £_{{k}})}{\sigma}\right)^{\varkappa^{\star}_{{{k}}}}\right), \Delta\left(\sigma\left(1-\prod\limits_{{{k}} = 1}^{n}\left(1-\left(\frac{\Delta^{-1}(\flat_{n_{{{k}}}}, \aleph_{{k}})}{\sigma}\right)\right)^{\varkappa^{\star}_{{{k}}}}\right)\right) \end{array}\right). \end{eqnarray} $ | (3.4) |
Theorem 3.4. Let $ \mathfrak{F}_{{k}} = ((\flat_{l_{{{k}}}}, £_{{k}}), (\flat_{n_{{{k}}}}, \aleph_{{k}})), \mathfrak{F}'_{{k}} = ((\flat'_{l_{{{k}}}}, £'_{{k}}), (\flat'_{n_{{{k}}}}, \aleph'_{{k}})) ({{k}} = 1, 2, \ldots, \mathfrak{n}) $ be two sets of 2TLBFNs, then the 2TLBFWG operator has the following properties:
(1) (Idempotency) If all $ \mathfrak{F}_{{k}} = ((\flat_{l_{{{k}}}}, £_{{k}}), (\flat_{n_{{{k}}}}, \aleph_{{k}}))({{k}} = 1, 2, \ldots, \mathfrak{n}) $ are equal, for all $ {{k}} = 1, 2, \ldots, \mathfrak{n} $, then
$ \begin{eqnarray*} && 2TLBFWG (\mathfrak{F}_{1}, \mathfrak{F}_{2}, \ldots, \mathfrak{F}_{n}) = \mathfrak{F}. \end{eqnarray*} $ |
(2) (Monotonicity)If $ \mathfrak{F}_{{k}}\leq \mathfrak{F}'_{{k}} $, for all $ {{k}} $, then
$ \begin{eqnarray*} 2TLBFWG (\mathfrak{F}_{1}, \mathfrak{F}_{2}, \ldots, \mathfrak{F}_{n})\leq 2TLBFWG (\mathfrak{F}'_{1}, \mathfrak{F}'_{2}, \ldots, \mathfrak{F}'_{n}). \end{eqnarray*} $ |
(3) (Boundedness)If $ \mathfrak{F}_{{{k}}} = ((\flat_{l_{{{k}}}}, £_{{{k}}}), (\flat_{n_{{{k}}}}, \aleph_{{{k}}}))({{k}} = 1, 2, \ldots, \mathfrak{n}) $ be a collection of 2TLBFNs, and let $ \mathfrak{F}^{-} = (\min_{{{k}}}(\flat_{l_{{{k}}}}, £_{{{k}}}), \max_{{k}}(\flat_{n_{{{k}}}}, \aleph_{{{k}}})) $ and$ \mathfrak{F}^{+} = (\max_{{k}}(\flat_{l_{{{k}}}}, £_{{{k}}}), \min_{{k}}(\flat_{n_{{{k}}}}, \aleph_{{{k}}})) $, then
$ \begin{eqnarray*} &&\mathfrak{F}^{-}\leq 2TLBFWG (\mathfrak{F}_{1}, \mathfrak{F}_{2}, \ldots, \mathfrak{F}_{n})\leq \mathfrak{F}^{+}. \end{eqnarray*} $ |
The generalized Heronian mean (GHM) operator and its weighted form in the 2TLBF environment are introduced in this subsection. We also look into some of the properties of these operators.
In this subsection, we combine GHM with 2TLBFNs and propose the generalized 2TL bipolar fuzzy Heronian mean (G2TLBFHM) operator.
Definition 4.1. Let $ \mathfrak{F}_{{{k}}} = ((\flat_{l_{{{k}}}}, £_{{{k}}}), (\flat_{n_{{{k}}}}, \aleph_{{{k}}}))({{k}} = 1, 2, \ldots, \mathfrak{n}) $ be a collection of 2TLBFNs. The G2TLBFHM ia a mapping $ \mathcal{P}^{n}\rightarrow \mathcal{P} $ such that
$ \begin{eqnarray*} \label{eq-1} && \text{G2TLBFHM}^{s, t}{(\mathfrak{F}_{1}, \mathfrak{F}_{2}, \ldots, \mathfrak{F}_{n})} = \left(\frac{2}{n(n+1)} \oplus_{{{j}} = 1}^{n}\oplus_{{{k}} = {{j}}}^{n}{(\mathfrak{F}^{s}_{{{j}}} \otimes \mathfrak{F}^{t}_{{{k}}})}\right)^{\frac{1}{s+t}} \end{eqnarray*} $ |
where $ s, t\geq 0 $.
Theorem 4.1. The aggregated value by using G2TLBFHM operator is also 2TLBFN, where
$ \begin{eqnarray*} \label{eq-2} && G2TLBFHM ^{s, t}{(\mathfrak{F}_{1}, \mathfrak{F}_{2}, \ldots, \mathfrak{F}_{n})}\\ && = \left( \begin{array}{l}\Delta\left(\sigma{\left(1-\prod\limits^{n}_{{{j}} = 1, {{k}} = {{j}}}\left(1-\left(\frac{\Delta^{-1}(\flat_{l_{{{j}}}}, £_{{{j}}})}{\sigma}\right)^{s} \left(\frac{\Delta^{-1}(\flat_{l_{{{k}}}}, £_{{{k}}})}{\sigma}\right)^{t}\right)^ {\frac{2}{n(n+1)}}\right)^{\frac{1}{s+t}}}\right), \\ \Delta\left(\sigma\left(1-\left(1-\prod\limits^{n}_{{{j}} = 1, {{k}} = {{j}}}\left(1-\left(1-\left(\frac{\Delta^{-1}(\flat_{n_{{{j}}}}, \aleph_{{{j}}})}{\sigma}\right) \right)^{s}\left(1-\left(\frac{\Delta^{-1}(\flat_{n_{{{k}}}}, \aleph_{{{k}}})}{\sigma}\right)\right)^{t}\right)^{\frac{2}{n(n+1)}}\right)^ {\frac{1}{s+t}}\right)\right)\end{array}\right) \end{eqnarray*} $ |
where $ s, t > 0. $
Proof. Utilizing Def. 3.4, we have
$ \begin{eqnarray*} (\mathfrak{F}_{{{j}}})^{s}& = &\left(\Delta\left(\sigma\left(\frac{\Delta^{-1}(\flat_{l_{{{j}}}}, £_{{{j}}})}{\sigma}\right)^{s}\right) , \Delta\left(\sigma\left(1-\left(1-{\left(\frac{\Delta^{-1}(\flat_{n_{{{j}}}}, \aleph_{{{j}}})}{\sigma}\right)}\right)^{s}\right)\right)\right), \\ (\mathfrak{F}_{{{k}}})^{t}& = &\left(\Delta\left(\sigma\left(\frac{\Delta^{-1}(\flat_{l_{{{k}}}}, £_{{{k}}})}{\sigma}\right)^{t}\right), \Delta\left(\sigma\left(1-\left(1-{\left(\frac{\Delta^{-1}(\flat_{n_{{{k}}}}, \aleph_{{{k}}})}{\sigma}\right)}\right)^{t}\right)\right) \right). \end{eqnarray*} $ |
Thus,
$ \begin{eqnarray*} (\mathfrak{F}_{{{j}}})^{s}\otimes(\mathfrak{F}_{{{k}}})^{t} = \left(\begin{array}{l}\Delta\left(\sigma\left(\frac{\Delta^{-1}(\flat_{l_{{{j}}}}, £_{{{j}}})}{\sigma}\right)^{s} \left(\frac{\Delta^{-1}(\flat_{l_{{{k}}}}, £_{{{k}}})}{\sigma}\right)^{t}\right), \\ \Delta\left(\sigma\left(1-\left(1-\left(\frac{\Delta^{-1}(\flat_{n_{{{j}}}}, \aleph_{{{j}}})}{\sigma}\right)\right) ^{s}\left(1-\left(\frac{\Delta^{-1}(\flat_{n_{{{k}}}}, \aleph_{{{k}}})}{\sigma}\right)\right)^{t}\right)\right) \end{array}\right). \end{eqnarray*} $ |
Therefore,
$ \begin{eqnarray*} \oplus_{{{j}} = 1}^{n}\oplus_{{{k}} = {{j}}}^{n}{(\mathfrak{F}^{s}_{{{j}}} \otimes \mathfrak{F}^{t}_{{{k}}})} = & \left(\begin{array}{l}\Delta\left(\sigma\left(1-\prod\limits^{n}_{{{j}} = 1, {{k}} = {{j}}}\left(1-\left(\frac{\Delta^{-1}(\flat_{l_{{{j}}}}, £_{{{j}}})}{\sigma}\right)^{s}\left(\frac{\Delta^{-1}(\flat_{l_{{{k}}}}, £_{{{k}}})}{\sigma}\right)^{t}\right)\right)\right), \\ \Delta\left(\sigma\left(\prod\limits^{n}_{{{j}} = 1, {{k}} = {{j}}}\left(1-\left(1-\left(\frac{\Delta^{-1}(\flat_{n_{{{j}}}}, \aleph_{{{j}}})}{\sigma}\right)\right)^{s}\left(1-\left(\frac{\Delta^{-1}(\flat_{n_{{{k}}}}, \aleph_{{{k}}})}{\sigma}\right)\right)^{t}\right)\right)\right) \end{array}\right). \end{eqnarray*} $ |
Furthermore,
$ \begin{eqnarray*} &&\frac{2}{n(n+1)}\oplus_{{{j}} = 1}^{n}\oplus_{{{k}} = {{j}}}^{n}{(\mathfrak{F}^{s}_{{{j}}} \otimes \mathfrak{F}^{t}_{{{k}}})}\\ && = \left(\begin{array}{l}\Delta\left(\sigma\left(1-\prod\limits^{n}_{{{j}} = 1, {{k}} = {{j}}}\left(1-\left(\frac{\Delta^{-1}(\flat_{l_{{{j}}}}, £_{{{j}}})}{\sigma}\right)^{s}\left(\frac{\Delta^{-1}(\flat_{l_{{{k}}}}, £_{{{k}}})}{\sigma}\right)^{t}\right)^{\frac{2}{n(n+1)}}\right)\right), \\ \Delta\left(\sigma\left(\prod\limits^{n}_{{{j}} = 1, {{k}} = {{j}}}\left(1-\left(1-\left(\frac{\Delta^{-1}(\flat_{n_{{{j}}}}, \aleph_{{{j}}})}{\sigma}\right)\right)^{s}\left(1-\left(\frac {\Delta^{-1}(\flat_{n_{{{k}}}}, \aleph_{{{k}}})}{\sigma}\right)\right)^{t}\right)^{{\frac{2}{n(n+1)}}}\right)\right) \end{array}\right). \end{eqnarray*} $ |
$ \begin{eqnarray*} && \text{G2TLBFHM}^{s, t}{(\mathfrak{F}_{1}, \mathfrak{F}_{2}, \ldots, \mathfrak{F}_{n})} = \left(\frac{2}{n(n+1)} \oplus_{{{j}} = 1}^{n}\oplus_{{{k}} = {{j}}}^{n}{(\mathfrak{F}^{s}_{{{j}}} \otimes \mathfrak{F}^{t}_{{{k}}})}\right)^{\frac{1}{s+t}}\nonumber\\&& = \left(\begin{array}{l}\Delta\left(\sigma{\left(1-\prod\limits^{n}_{{{j}} = 1, {{k}} = {{j}}}\left(1-\left(\frac{\Delta^{-1}(\flat_{l_{{{j}}}}, £_{{{j}}})}{\sigma}\right)^{s} \left(\frac{\Delta^{-1}(\flat_{l_{{{k}}}}, £_{{{k}}})}{\sigma}\right)^{t}\right)^ {\frac{2}{n(n+1)}}\right)^{\frac{1}{s+t}}}\right), \\\Delta\left(\sigma\left(1-\left(1-\prod\limits^{n}_{{{j}} = 1, {{k}} = {{j}}}\left(1-\left(1-\left(\frac{\Delta^{-1}(\flat_{n_{{{j}}}}, \aleph_{{{j}}})}{\sigma}\right) \right)^{s}\left(1-\left(\frac{\Delta^{-1}(\flat_{n_{{{k}}}}, \aleph_{{{k}}})}{\sigma}\right)\right)^{t}\right)^{\frac{2}{n(n+1)}}\right)^ {\frac{1}{s+t}}\right)\right)\end{array}\right). \end{eqnarray*} $ |
Example 4.1. Let $ \mathfrak{F}_{1} = ((\flat_{3}, 0.4), (\flat_{5}, -0.2)) $, $ \mathfrak{F}_{2} = ((\flat_{4}, 0.3), (\flat_{2}, -0.5)) $, $ \mathfrak{F}_{3} = ((\flat_{3}, 0.1), (\flat_{4}, -0.4)) $, and $ \mathfrak{F}_{4} = ((\flat_{5}, 0.2), (\flat_{6}, -0.3)) $ be four 2TLBFNs, and suppose $ s = 2 $ and $ t = 3 $, then according to Theorem 4.1 we have
$ \begin{eqnarray*} && \text{G2TLBFHM}^{s, t}{(\mathfrak{F}_{1}, \mathfrak{F}_{2}, \ldots, \mathfrak{F}_{n})} = \left(\frac{2}{n(n+1)} \oplus_{{{j}} = 1}^{n}\oplus_{{{k}} = {{j}}}^{n}{(\mathfrak{F}^{s}_{{{j}}} \otimes \mathfrak{F}^{t}_{{{k}}})}\right)^{\frac{1}{s+t}}\\ && = \left(\begin{array}{l} \Delta\left(6\left({1- \left( \begin{array}{l} \; \; (1-(\frac{3.4}{6})^{2}\times(\frac{3.4}{6})^{3})\times(1-(\frac{3.4}{6})^{2}\times(\frac{4.3}{6})^{3})\\ \times(1-(\frac{3.4}{6})^{2}\times(\frac{3.1}{6})^{3})\times (1-(\frac{3.4}{6})^{2}\times(\frac{5.2}{6})^{3})\\ \times(1-(\frac{4.3}{6})^{2}\times(\frac{4.3}{6})^{3})\times(1-(\frac{4.3}{6})^{2}\times(\frac{3.1}{6})^{3})\\ \times(1-(\frac{4.3}{6})^{2}\times(\frac{5.2}{6})^{3}) \times(1-(\frac{3.1}{6})^{2}\times(\frac{3.1}{6})^{3})\\\times(1-(\frac{3.1}{6})^{2}\times(\frac{5.2}{6})^{3})\times(1-(\frac{5.2}{6})^{2}\times(\frac{5.2}{6})^{3}) \end{array} \right) ^{\frac{2}{4(4+1)}}}\right)^{\frac{1}{2+3}}\right), \\ \Delta\left(6\left(1-\left( {1- \left( \begin{array}{l} \; \; (1-(1-\frac{4.8}{6})^{2}\times(1-\frac{4.8}{6})^{3})\times(1-(1-\frac{4.8}{6})^{2}\times(1-\frac{1.5}{6})^{3})\\ \times(1-(1-\frac{4.8}{6})^{2}\times(1-\frac{3.6}{6})^{3})\times (1-(1-\frac{4.8}{6})^{2}\times(1-\frac{5.7}{6})^{3}) \\ \times(1-(1-\frac{1.5}{6})^{2}\times(1-\frac{1.5}{6})^{3})\times(1-(1-\frac{1.5}{6})^{2}\times(1-\frac{3.6}{6})^{3})\\ \times(1-(1-\frac{1.5}{6})^{2}\times(1-\frac{5.7}{6})^{3}) \times(1-(1-\frac{3.6}{6})^{2}\times(1-\frac{3.6}{6})^{3})\\ \times(1-(1-\frac{3.6}{6})^{2}\times(1-\frac{5.7}{6})^{3})\times(1-(1-\frac{5.7}{6})^{2}\times(1-\frac{5.7}{6})^{3}) \end{array}\right) ^{\frac{2}{4(4+1)}}} \right)^{\frac{1}{2+3}}\right)\right) \end{array} \right)\\ && = ((\flat_{3}, 0.7626), (\flat_{3}, 0.5081)). \end{eqnarray*} $ |
Property 4.1. (Idempotency) Let all $ \mathfrak{F}_{{{k}}}({{k}} = 1, 2, \ldots, \mathfrak{n}) $ are equal, i.e., $ \mathfrak{F}_{{{k}}} = \mathfrak{F} $ for all $ {{k}} $, then
$ G2TLBFHM ^{s, t}{(\mathfrak{F}_{1}, \mathfrak{F}_{2}, \ldots, \mathfrak{F}_{n})} = \mathfrak{F}. $ |
Proof. Since $ \mathfrak{F}_{{{k}}} = \mathfrak{F} = \left((\flat_{l}, £), (\flat_{n}, \aleph)\right) $, then
$ \begin{eqnarray*} && \text{G2TLBFHM}^{s, t}{(\mathfrak{F}_{1}, \mathfrak{F}_{2}, \ldots, \mathfrak{F}_{n})}\\ && = \left(\begin{array}{l}\Delta\left(\sigma\left(\left(1-\prod\limits^{n}_{{{j}} = 1, {{k}} = {{j}}}\left(1-\left(\frac{\Delta^{-1}(\flat_{l_{{{j}}}}, £_{{{j}}})}{\sigma}\right)^{s}\left(\frac{\Delta^{-1}(\flat_{l_{{{k}}}}, £_{{{k}}})}{\sigma}\right)^{t}\right)^ {\frac{2}{n(n+1)}}\right)^{\frac{1}{s+t}}\right)\right), \\\Delta\left(\sigma\left(1-\left(1-\prod\limits^{n}_{{{j}} = 1, {{k}} = {{j}}}\left(1-\left(1-\left(\frac{\Delta^{-1}(\flat_{n_{{{j}}}}, \aleph_{{{j}}})}{\sigma}\right) \right)^{s}\left(1-\left(\frac{\Delta^{-1}(\flat_{n_{{{k}}}}, \aleph_{{{k}}})}{\sigma}\right)\right)^{t}\right)^{\frac{2}{n(n+1)}}\right)^ {\frac{1}{s+t}}\right)\right)\end{array}\right).\\ && = \left(\begin{array}{l}\Delta\left(\sigma\left(1-\left(1-\left(\frac{\Delta^{-1}(\flat_{l}, £)}{\sigma}\right)^{s}\left(\frac{\Delta^{-1}(\flat_{l}, £)}{\sigma}\right)^{t}\right)^{\frac{1}{s+t}}\right)\right), \\\Delta\left(\sigma\left(1-\left(1-\left(\frac{\Delta^{-1}(\flat_{n}, \aleph)}{\sigma}\right) \right)^{s}\left(1-\left(\frac{\Delta^{-1}(\flat_{n}, \aleph)}{\sigma}\right)\right)^{t}\right)^ {\frac{1}{s+t}}\right)\end{array}\right).\\ && = \left(\begin{array}{l}\Delta\left(\sigma\left(1-\left(1-\left(\frac{\Delta^{-1}(\flat_{l}, £)}{\sigma}\right)\right)\right)\right), \Delta\left(\sigma\left(1-\left(1-\left(\frac{\Delta^{-1}(\flat_{n}, \aleph)}{\sigma}\right) \right)\right)\right)\end{array}\right).\\ && = \left((\flat_{l}, £), (\flat_{n}, \aleph)\right) = \mathfrak{F}. \end{eqnarray*} $ |
Property 4.2. (Monotonicity) Let $ \mathfrak{F}_{{{k}}}({{k}} = 1, 2, \ldots, \mathfrak{n}) $ and $ \mathfrak{F}'_{{{k}}}({{k}} = 1, 2, \ldots, \mathfrak{n}) $ be two sets of 2TLBFNs. If $ \mathfrak{F}_{{{k}}}\geq \mathfrak{F}'_{{{k}}} $ for all $ {{k}} $, then
$ G2TLBFHM^{s, t}(\mathfrak{F}_{1}, \mathfrak{F}_{2}, \ldots, \mathfrak{F}_{n})\geq G2TLBFHM^{s, t}(\mathfrak{F}'_{1}, \mathfrak{F}'_{2}, \ldots, \mathfrak{F}'_{n}). $ |
Proof. Let $ \mathfrak{F} = \left((\flat_{l_{{{j}}}}, £_{{{j}}}), (\flat_{n_{{{j}}}}, \aleph_{{{j}}})\right) $ and $ \mathfrak{F}' = \left((\flat'_{l_{{{j}}}}, £'_{{{j}}}), (\flat'_{n_{{{j}}}}, \aleph'_{{{j}}})\right) $ be two sets of 2TLBFNs. Since
$ \begin{eqnarray*} &(\flat_{l_{{{j}}}}, £_{{{j}}}) \geq (\flat'_{l_{{{j}}}}, £'_{{{j}}})\\ & \Delta^{-1}(\flat_{l_{{{j}}}}, £_{{{j}}}) \geq \Delta^{-1} (\flat'_{l_{{{j}}}}, £'_{{{j}}})\\ &\left(\frac{\Delta^{-1}(\flat_{l_{{{j}}}}, £_{{{j}}})}{\sigma}\right)^{s}\left(\frac{\Delta^{-1}(\flat_{l_{{{k}}}}, £_{{{k}}})}{\sigma}\right)^{t}\geq\left(\frac{\Delta^{-1}(\flat'_{l_{{{j}}}}, £'_{{{j}}})}{\sigma}\right)^{s} \left(\frac{\Delta^{-1}(\flat'_{l_{{{k}}}}, £'_{{{k}}})}{\sigma}\right)^{t}\\ &\prod\limits^{n}_{{{j}} = 1, {{k}} = {{j}}}\left(1-\left(\frac{\Delta^{-1}(\flat_{l_{{{j}}}}, £_{{{j}}})}{\sigma}\right)^{s}\left(\frac{\Delta^{-1}(\flat_{l_{{{k}}}}, £_{{{k}}})}{\sigma}\right)^{t}\right)^{\frac{2}{n(n+1)}}\leq\prod\limits^{n}_{{{j}} = 1, {{k}} = {{j}}}\left(1-\left(\frac{\Delta^{-1}(\flat'_{l_{{{j}}}}, £'_{{{j}}})}{\sigma}\right)'^{s} \left(\frac{\Delta^{-1}(\flat'_{l_{{{k}}}}, £'_{{{k}}})}{\sigma}\right)^{t}\right)^{\frac{2}{n(n+1)}}.\\ &1-\prod\limits^{n}_{{{j}} = 1, {{k}} = {{j}}}\left(1-\left(\frac{\Delta^{-1}(\flat_{l_{{{j}}}}, £_{{{j}}})}{\sigma}\right)^{s}\left(\frac{\Delta^{-1}(\flat_{l_{{{k}}}}, £_{{{k}}})}{\sigma}\right)^{t}\right)^{\frac{2}{n(n+1)}}\geq 1-\prod\limits^{n}_{{{j}} = 1, {{k}} = {{j}}}\left(1-\left(\frac{\Delta^{-1}(\flat'_{l_{{{j}}}}, £'_{{{j}}})}{\sigma}\right)^{s} \left(\frac{\Delta^{-1}(\flat'_{l_{{{k}}}}, £'_{{{k}}})}{\sigma}\right)^{t}\right)^{\frac{2}{n(n+1)}}.\\ &\Delta\left(\sigma\left(1-\prod\limits^{n}_{{{j}} = 1, {{k}} = {{j}}}\left(1-\left(\frac{\Delta^{-1}(\flat_{l_{{{j}}}}, £_{{{j}}})}{\sigma}\right)^{s}\left(\frac{\Delta^{-1}(\flat_{l_{{{k}}}}, £_{{{k}}})}{\sigma}\right)^{t}\right)^{\frac{2}{n(n+1)}}\right)^\frac{1}{s+t}\right) \geq \\ & \Delta\left(\sigma\left(1-\prod\limits^{n}_{{{j}} = 1, {{k}} = {{j}}}\left(1-\left(\frac{\Delta^{-1}(\flat'_{l_{{{j}}}}, £'_{{{j}}})}{\sigma}\right)^{s} \left(\frac{\Delta^{-1}(\flat'_{l_{{{k}}}}, £'_{{{k}}})}{\sigma}\right)^{t}\right)^{\frac{2}{n(n+1)}}\right)^\frac{1}{s+t}\right). \end{eqnarray*} $ |
Similarly, we can prove that $ (\flat_{n_{{{j}}}}, \aleph_{{{j}}}) \leq (\flat'_{n_{{{j}}}}, \aleph'_{{{j}}}) $.
Property 4.3. (Boundedness)Let $ \mathfrak{F}_{{{k}}}({{k}} = 1, 2, \ldots, \mathfrak{n}) $ be a collection of 2TLBFNs, and let$ \mathfrak{F}^{-} = \min_{{{k}}}\mathfrak{F}_{{{k}}}, \; \mathfrak{F}^{+} = \max_{{{k}}}\mathfrak{F}_{{{k}}} $, then
$ \mathfrak{F}^{-}\leq G2TLBFHM^{s, t}(\mathfrak{F}_{1}, \mathfrak{F}_{2}, \ldots, \mathfrak{F}_{n})\leq \mathfrak{F}^{+}. $ |
Proof. According to Property 4.1
$ G2TLBFHM^{s, t}(\mathfrak{F}_{1}^{-}, \mathfrak{F}_{2}^{-}, \ldots, \mathfrak{F}_{n}^{-}) = \mathfrak{F}^{-} $ |
and
$ G2TLBFHM^{s, t}(\mathfrak{F}_{1}^{+}, \mathfrak{F}_{2}^{+}, \ldots, \mathfrak{F}_{n}^{+}) = \mathfrak{F}^{+} $ |
From Property 4.2
$ \mathfrak{F}^{-}\leq G2TLBFHM^{s, t}(\mathfrak{F}_{1}, \mathfrak{F}_{2}, \ldots, \mathfrak{F}_{n})\leq \mathfrak{F}^{+}. $ |
Definition 4.2. Let $ s, t > 0, \mathfrak{F}_{{{k}}} = ((\flat_{l_{{{k}}}}, £_{{{k}}}), (\flat_{n_{{{k}}}}, \aleph_{{{k}}}))({{k}} = 1, 2, \ldots, \mathfrak{n}) $ be a collection of 2TLBFNs, $ \varkappa^{\star} = (\varkappa^{\star}_{1}, \varkappa^{\star}_{2}, \ldots, \varkappa^{\star}_{n})^{T} $ is the weight vector of $ \mathfrak{F}_{{k}} $, satisfying $ \varkappa^{\star}_{{{k}}} > 0 $ and $ \sum^{n}_{{{k}} = 1}\varkappa^{\star}_{{{k}}} = 1\; ({{k}} = 1, 2, \ldots, \mathfrak{n}) $. The G2TLBFWHM operator is defined as follows:
$ \begin{equation} \text{G2TLBFWHM}_{\varkappa^{\star}}^{s, t}(\mathfrak{F}_{1}, \mathfrak{F}_{2}, \ldots, \mathfrak{F}_{n}) = \left(\oplus_{{{j}} = 1}^{n}\oplus_{{{k}} = {{j}}}^{n}\left(\varkappa^{\star}_{{{j}}}\varkappa^{\star}_{{{k}}}(\mathfrak{F}_{{{j}}})^s\otimes(\mathfrak{F}_{{{k}}})^{t}\right)\right)^{\frac{1}{s+t}}. \end{equation} $ | (4.1) |
Theorem 4.2. Let $ s, t > 0 $, $ \mathfrak{F}_{{{k}}} = ((\flat_{l_{{{k}}}}, £_{{{k}}}), (\flat_{n_{{{k}}}}, \aleph_{{{k}}}))({{k}} = 1, 2, \ldots, \mathfrak{n}) $ be a collection of 2TLBFNs. Then the aggregated value using by G2TLBFWHM operator is also a 2TLBFN, and
$ \begin{eqnarray} && G2TLBFWHM _{\varkappa^{\star}}^{s, t}{(\mathfrak{F}_{1}, \mathfrak{F}_{2}, \ldots, \mathfrak{F}_{n})}\\&& = \left(\begin{array}{l}\Delta\left(\sigma\left(\left({1-\prod\limits^{n}_{{{j}} = 1, {{k}} = {{j}}}\left(1-\left(\frac{\Delta^{-1}(\flat_{l_{{{j}}}}, £_{{{j}}})}{\sigma}\right)^{s}\left(\frac{\Delta^{-1}(\flat_{l_{{{k}}}}, £_{{{k}}})}{\sigma}\right)^{t} \right)^{\varkappa^{\star}_{{{j}}}\varkappa^{\star}_{{{k}}}}}\right)^{\frac{1}{s+t}}\right)\right), \\ \Delta\left(\sigma\left(1-\left(1-\prod\limits^{n}_{{{j}} = 1, {{k}} = {{j}}}\left(1-\left(1-\left(\frac{\Delta^{-1}(\flat_{n_{{{j}}}}, \aleph_{{{j}}})}{\sigma}\right) \right)^{s}\left(1-\left(\frac{\Delta^ {-1}(\flat_{n_{{{k}}}}, \aleph_{{{k}}})}{\sigma}\right)\right)^{t}\right)^{{\varkappa^{\star}_{{{j}}}\varkappa^{\star}_{{{k}}}}}\right)^{\frac{1}{s+t}}\right)\right)\end{array}\right). \end{eqnarray} $ | (4.2) |
Proof. According to Definition 3.4, we can derive
$ \begin{eqnarray*} (\mathfrak{F}_{{{j}}})^{s}& = &\left(\Delta\left(\sigma\left(\frac{\Delta^{-1}(\flat_{l_{{{j}}}}, £_{{{j}}})}{\sigma}\right)^{s}\right) , \Delta\left(\sigma\left(1-\left(1-{\left(\frac{\Delta^{-1}(\flat_{n_{{{j}}}}, \aleph_{{{j}}})}{\sigma}\right)}\right)^{s}\right)\right)\right), \\ (\mathfrak{F}_{{{k}}})^{t}& = &\left(\Delta\left(\sigma\left(\frac{\Delta^{-1}(\flat_{l_{{{k}}}}, £_{{{k}}})}{\sigma}\right)^{t}\right), \Delta\left(\sigma\left(1-\left(1-{\left(\frac{\Delta^{-1}(\flat_{n_{{{k}}}}, \aleph_{{{k}}})}{\sigma}\right)}\right)^{t}\right)\right) \right). \end{eqnarray*} $ |
Thus,
$ \begin{eqnarray*} (\mathfrak{F}_{{{j}}})^{s}\otimes(\mathfrak{F}_{{{k}}})^{t} = \left(\begin{array}{l}\Delta\left(\sigma\left(\frac{\Delta^{-1}(\flat_{l_{{{j}}}}, £_{{{j}}})}{\sigma}\right)^{s} \left(\frac{\Delta^{-1}(\flat_{l_{{{k}}}}, £_{{{k}}})}{\sigma}\right)^{t}\right), \\ \Delta\left(\sigma\left(1-\left(1-\left(\frac{\Delta^{-1}(\flat_{n_{{{j}}}}, \aleph_{{{j}}})}{\sigma}\right)\right)^{s}\left(1-\left(\frac{\Delta^{-1}(\flat_{n_{{{k}}}}, \aleph_{{{k}}})}{\sigma}\right)\right)^{t}\right)\right) \end{array}\right). \end{eqnarray*} $ |
Therefore,
$ \begin{eqnarray*} &\varkappa^{\star}_{{{j}}}\varkappa^{\star}_{{{k}}}{(\mathfrak{F}_{{{j}}})^{s} \otimes (\mathfrak{F}_{{{k}}})^{t}} = & \left(\begin{array}{l}\Delta\left(\sigma\left(1-\left(1-\left(\frac{\Delta^{-1}(\flat_{l_{{{j}}}}, £_{{{j}}})}{\sigma}\right)^{s}\left(\frac{\Delta^{-1}(\flat_{l_{{{k}}}}, £_{{{k}}})}{\sigma}\right)^{t}\right)^{\varkappa^{\star}_{{{j}}}\varkappa^{\star}_{{{k}}}}\right)\right), \\ \Delta\left(\sigma\left(1-\left(1-\left(\frac{\Delta^{-1}(\flat_{n_{{{j}}}}, \aleph_{{{j}}})}{\sigma}\right)\right)^{s}\left(1-\left(\frac{\Delta^{-1}(\flat_{n_{{{k}}}}, \aleph_{{{k}}})}{\sigma}\right)\right)^{t}\right)^{\varkappa^{\star}_{{{j}}}\varkappa^{\star}_{{{k}}}}\right) \end{array}\right). \end{eqnarray*} $ |
Furthermore,
$ \begin{eqnarray*} &&\oplus_{{{j}} = 1}^{n}\oplus_{{{k}} = {{j}}}^{n}(\varkappa^{\star}_{{{j}}}\varkappa^{\star}_{{{k}}}{(\mathfrak{F}_{{{j}}})^{s} \otimes (\mathfrak{F}_{{{k}}})^{t}})\\&& = \left(\begin{array}{l}\Delta\left(\sigma\left(1-\prod\limits^{n}_{{{j}} = 1, {{k}} = {{j}}}\left(1-\left(\frac{\Delta^{-1}(\flat_{l_{{{j}}}}, £_{{{j}}})}{\sigma}\right)^{s}\left(\frac{\Delta^{-1}(\flat_{l_{{{k}}}}, £_{{{k}}})}{\sigma}\right)^{t} \right)^{\varkappa^{\star}_{{{j}}}\varkappa^{\star}_{{{k}}}}\right)\right), \\\Delta\left(\sigma\left(\prod\limits^{n}_{{{j}} = 1, {{k}} = {{j}}}\left(1-\left(1-\left(\frac{ \Delta^{-1}(\flat_{n_{{{j}}}}, \aleph_{{{j}}})}{\sigma}\right)\right)^{s}\left( 1-\left(\frac{\Delta^{-1}(\flat_{n_{{{k}}}}, \aleph_{{{k}}})}{\sigma}\right)\right)^{t}\right)^{\varkappa^{\star}_{{{j}}}\varkappa^{\star}_{{{k}}}}\right)\right)\end{array}\right). \end{eqnarray*} $ |
$ \begin{eqnarray*} && \text{G2TLBFWHM}_{\varkappa^{\star}}^{s, t}{(\mathfrak{F}_{1}, \mathfrak{F}_{2}, \ldots, \mathfrak{F}_{n})} = \left(\oplus_{{{j}} = 1}^{n}\oplus_{{{k}} = {{j}}}^{n}\left(\varkappa^{\star}_{{{j}}}\varkappa^{\star}_{{{k}}}(\mathfrak{F}_{{{j}}})^s\otimes(\mathfrak{F}_{{{k}}})^{t}\right)\right)^ {\frac{1}{s+t}}\\ && = \left(\begin{array}{l}\Delta\left(\sigma\left({1-\prod\limits^{n}_{{{j}} = 1, {{k}} = {{j}}}\left(1-\left(\frac{\Delta^{-1}(\flat_{l_{{{j}}}}, £_{{{j}}})}{\sigma}\right)^{s}\left(\frac{\Delta^{-1}(\flat_{l_{{{k}}}}, £_{{{k}}})}{\sigma}\right)^{t} \right)^{\varkappa^{\star}_{{{j}}}\varkappa^{\star}_{{{k}}}}}\right)^{\frac{1}{s+t}}\right) , \\\Delta\left(\sigma\left(1-\left(1-\prod\limits^{n}_{{{j}} = 1, {{k}} = {{j}}}\left(1-\left(1-\left(\frac{\Delta^{-1}(\flat_{n_{{{j}}}}, \aleph_{{{j}}})}{\sigma}\right)\right)^{s}\left(1-\left(\frac{\Delta^ {-1}(\flat_{n_{{{k}}}}, \aleph_{{{k}}})}{\sigma}\right)\right)^{t}\right)^{{\varkappa^{\star}_{{{j}}}\varkappa^{\star}_{{{k}}}}}\right)^{\frac{1}{s+t}}\right)\right)\end{array}\right). \end{eqnarray*} $ |
Example 4.2. Let $ \mathfrak{F}_{1} = ((\flat_{3}, 0.4), (\flat_{5}, 0.2)) $, $ \mathfrak{F}_{2} = ((\flat_{4}, 0.3), (\flat_{2}, -0.5)) $, $ \mathfrak{F}_{3} = ((\flat_{3}, 0.1), (\flat_{4}, -0.4)) $, and $ \mathfrak{F}_{4} = ((\flat_{5}, 0.2), (\flat_{6}, -0.3)) $ be four 2TLBFNs, and suppose $ s = 2 $ and $ t = 3 $, $ \varkappa^{\star} = (0.17, 0.32, 0.38, 0.13) $ then according to Theorem 4.2 we have
$ \begin{eqnarray*} && \text{G2TLBFWHM}_{\varkappa^{\star}}^{s, t}{(\mathfrak{F}_{1}, \mathfrak{F}_{2}, \ldots, \mathfrak{F}_{n})} = \left(\oplus_{{{j}} = 1}^{n}\oplus_{{{k}} = {{j}}}^{n}{\left(\varkappa^{\star}_{{{j}}}\varkappa^{\star}_{{{k}}}(\mathfrak{F}_{{{j}}})^{s} \otimes (\mathfrak{F}_{{{k}}})^{t}\right)}\right)^{\frac{1}{s+t}}\\ && = \left(\begin{array}{l} \Delta\left(6\left({1- \left( \begin{array}{l} \; \; (1-(\frac{3.4}{6})^{2}\times(\frac{3.4}{6})^{3})^{0.17\times0.17}\times(1-(\frac{3.4}{6})^{2}\times(\frac{4.3}{6})^{3})^{0.17\times0.32}\\ \times(1-(\frac{3.4}{6})^{2}\times(\frac{3.1}{6})^{3})^{0.17\times0.38}\times (1-(\frac{3.4}{6})^{2}\times(\frac{5.2}{6})^{3})^{0.17\times0.13} \\ \times(1-(\frac{4.3}{6})^{2}\times(\frac{4.3}{6})^{3})^{0.32\times0.32}\times(1-(\frac{4.3}{6})^{2}\times(\frac{3.1}{6})^{3})^{0.32\times0.38}\\ \times(1-(\frac{4.3}{6})^{2}\times(\frac{5.2}{6})^{3})^{0.32\times0.13} \times(1-(\frac{3.1}{6})^{2}\times(\frac{3.1}{6})^{3})^{0.38\times0.38}\\ \times(1-(\frac{3.1}{6})^{2}\times(\frac{5.2}{6})^{3})^{0.38\times0.13}\times(1-(\frac{5.2}{6})^{2}\times(\frac{5.2}{6})^{3})^{0.13\times0.13} \end{array} \right) }\right)^{\frac{1}{2+3}}\right), \\ \Delta\left(6\left(1-\left( {1- \left( \begin{array}{l} \; \; \left(1-(1-\frac{5.2}{6})^{2}\times(1-\frac{5.2}{6})^{3}\right)^{0.17\times0.17}\times \left(1-(1-\frac{5.2}{6})^{2}\times(1-\frac{1.5}{6})^{3}\right)^{0.17\times0.32}\\ \times \left(1-(1-\frac{5.2}{6})^{2}\times(1-\frac{3.6}{6})^{3}\right)^{0.17\times0.38}\times \left(1-(1-\frac{5.2}{6})^{2}\times(1-\frac{5.7}{6})^{3}\right)^{0.17\times0.13} \\ \times\left(1-(1-\frac{1.5}{6})^{2}\times(1-\frac{1.5}{6})^{3}\right)^{0.32\times0.32}\times \left(1-(1-\frac{1.5}{6})^{2}\times(1-\frac{3.6}{6})^{3}\right)^{0.32\times0.38}\\ \times\left(1-(1-\frac{1.5}{6})^{2} \times(1-\frac{5.7}{6})^{3}\right)^{0.32\times0.13} \times\left(1-(1-\frac{3.6}{6})^{2} \times(1-\frac{3.6}{6})^{3}\right)^{0.38\times0.38}\\ \times \left(1-(1-\frac{3.6}{6})^{2}\times(1-\frac{5.7}{6})^{3}\right)^{0.38\times0.13}\times \left(1-(1-\frac{5.7}{6})^{2}\times(1-\frac{5.7}{6})^{3}\right)^{0.13\times0.13} \end{array} \right)}\right)^{\frac{1}{2+3}}\right)\right) \end{array}\right).\\ && = ((\flat_{3}, 0.2487), (\flat_{2}, 0.9455)). \end{eqnarray*} $ |
The G2TLBFWHM operator has the following features, which are easily shown.
Property 4.4. (Idempotancy) Let all $ \mathfrak{F}_{{{k}}}({{k}} = 1, 2, \ldots, \mathfrak{n}) $ are equal, i.e., $ \mathfrak{F}_{{{k}}} = \mathfrak{F} $ for all $ {{k}} $, then
$ {G2TLBFWHM}_{\varkappa^{\star}}^{s, t}{(\mathfrak{F}_{1}, \mathfrak{F}_{2}, \ldots, \mathfrak{F}_{n})} = \mathfrak{F}. $ |
Property 4.5. (Monotonicity) Let $ \mathfrak{F}_{{{k}}}({{k}} = 1, 2, \ldots, \mathfrak{n}) $ and $ \mathfrak{F}'_{{{k}}}({{k}} = 1, 2, \ldots, \mathfrak{n}) $ be two sets of 2TLBFNs. If $ \mathfrak{F}_{{{k}}}\geq \mathfrak{F}'_{{{k}}} $ for all $ {{k}} $, then
$ G2TLBFWHM _{\varkappa^{\star}}^{s, t}(\mathfrak{F}_{1}, \mathfrak{F}_{2}, \ldots, \mathfrak{F}_{n})\geq {G2TLBFWHM}_{\varkappa^{\star}}^{s, t}(\mathfrak{F}'_{1}, \mathfrak{F}'_{2}, \ldots, \mathfrak{F}'_{n}). $ |
Property 4.6. (Boundedness)Let $ \mathfrak{F}_{{{k}}}({{k}} = 1, 2, \ldots, \mathfrak{n}) $ be a collection of 2TLBFNs, and let $ \mathfrak{F}^{-} = \min_{{{k}}}\mathfrak{F}_{{{k}}}, \; \mathfrak{F}^{+} = \max_{{{k}}}\mathfrak{F}_{{{k}}} $, then
$ \mathfrak{F}^{-}\leq G2TLBFWHM _{\varkappa^{\star}}^{s, t}(\mathfrak{F}_{1}, \mathfrak{F}_{2}, \ldots, \mathfrak{F}_{n})\leq \mathfrak{F}^{+}. $ |
In this subsection, we introduced the geometric Heronian mean (GHM) operator and its weighted form. In addition, we investigate some properties of these operators.
In this subsection, we combine GHM with 2TLBFNs and propose the 2TL bipolar fuzzy geometric Heronian mean (2TLBFGHM) operator.
Definition 4.3. Let $ \mathfrak{F}_{{{k}}} = ((\flat_{l_{{{k}}}}, £_{{{k}}}), (\flat_{n_{{{k}}}}, \aleph_{{{k}}}))({{k}} = 1, 2, \ldots, \mathfrak{n}) $ be a collection of 2TLBFNs. The 2TLBFGHM ia a mapping $ \mathcal{P}^{n}\rightarrow \mathcal{P} $ such that
$ \begin{equation} \text{2TLBFGHM}^{s, t}{(\mathfrak{F}_{1}, \mathfrak{F}_{2}, \ldots, \mathfrak{F}_{n})} = \frac{1}{s+t}\left( \otimes_{{{j}} = 1}^{n}\otimes_{{{k}} = {{j}}}^{n}{({s}\mathfrak{F}_{{{j}}} \oplus {t}\mathfrak{F}_{{{k}}})}\right)^{\frac{2}{n(n+1)}} \end{equation} $ | (4.3) |
where $ s, t\geq 0 $.
Theorem 4.3. The aggregated value by using 2TLBFGHM operator is also 2TLBFN, where
$ \begin{eqnarray*} && 2TLBFGHM ^{s, t}{(\mathfrak{F}_{1}, \mathfrak{F}_{2}, \ldots, \mathfrak{F}_{n})}\\ && = \left(\begin{array}{l}\Delta\left(\sigma\left(1-\left(1-\left(\prod\limits^{n}_{{{j}} = 1, {{k}} = {{j}}}\left(1-\left(1-\left(\frac{\Delta^{-1}(\flat_{l_{{{j}}}}, £_{{{j}}})}{\sigma}\right)\right)\right)^{s}\left(1-\left(\frac {\Delta^{-1}(\flat_{l_{{{k}}}}, £_{{{k}}})}{\sigma}\right)\right)^{t}\right)^{{\frac{2}{n(n+1)}}}\right)^{\frac{1}{s+t}}\right)\right), \\ \Delta\left(\sigma\left(\left( 1-\prod\limits^{n}_{{{j}} = 1, {{k}} = {{j}}}\left(1-\left(\frac{\Delta^{-1}(\flat_{n_{{{j}}}}, \aleph_{{{j}}})}{\sigma}\right)^{s}\left(\frac{\Delta^{-1}(\flat_{n_{{{k}}}}, \aleph_{{{k}}})}{\sigma}\right)^{t}\right)^{\frac{2}{n(n+1)}}\right)^{\frac{1}{s+t}}\right)\right) \end{array}\right) \end{eqnarray*} $ |
where $ s, t > 0. $
Example 4.3. Let $ \mathfrak{F}_{1} = ((\flat_{3}, 0.4), (\flat_{5}, -0.2)) $, $ \mathfrak{F}_{2} = ((\flat_{4}, 0.3), (\flat_{2}, -0.5)) $, $ \mathfrak{F}_{3} = ((\flat_{3}, 0.1), (\flat_{4}, -0.4)) $, and $ \mathfrak{F}_{4} = ((\flat_{5}, 0.2), (\flat_{6}, -0.3)) $ be four 2TLBFNs, and suppose $ s = 2 $ and $ t = 3 $, then according to Theorem 4.3 we have
$ \begin{eqnarray*} && \text{2TLBFGHM}^{s, t}{(\mathfrak{F}_{1}, \mathfrak{F}_{2}, \ldots, \mathfrak{F}_{n})} = {\frac{1}{s+t}}\left( \otimes_{{{j}} = 1}^{n}\otimes_{{{k}} = {{j}}}^{n}{({s}\mathfrak{F}_{{{j}}} \oplus {t}\mathfrak{F}_{{{k}}})}\right)^{\frac{2}{n(n+1)}}\\ && = \left(\begin{array}{l} \Delta\left(6\left(1-\left( {1- \left( \begin{array}{l} \; \; (1-(1-\frac{3.4}{6})^{2}\times(1-\frac{3.4}{6})^{3})\times(1-(1-\frac{3.4}{6})^{2}\times(1-\frac{4.3}{6})^{3})\\\times(1-(1-\frac{3.4}{6})^{2}\times(1-\frac{3.1}{6})^{3})\times (1-(1-\frac{3.4}{6})^{2}\times(1-\frac{5.2}{6})^{3}) \\\times(1-(1-\frac{4.3}{6})^{2}\times(1-\frac{4.3}{6})^{3})\times(1-(1-\frac{4.3}{6})^{2}\times(1-\frac{3.1}{6})^{3})\\\times(1-(1-\frac{4.3}{6})^{2}\times(1-\frac{5.2}{6})^{3}) \times(1-(1-\frac{3.1}{6})^{2}\times(1-\frac{3.1}{6})^{3})\\\times(1-(1-\frac{3.1}{6})^{2}\times(1-\frac{5.2}{6})^{3})\times(1-(1-\frac{5.2}{6})^{2}\times(1-\frac{5.2}{6})^{3}) \end{array}\right) ^{\frac{2}{4(4+1)}}} \right)^{\frac{1}{2+3}}\right)\right), \\ \left(\Delta\left(6\left({1- \left( \begin{array}{l} \; \; (1-(\frac{4.8}{6})^{2}\times(\frac{4.8}{6})^{3})\times(1-(\frac{4.8}{6})^{2}\times(\frac{1.5}{6})^{3})\\\times(1-(\frac{4.8}{6})^{2}\times(\frac{3.6}{6})^{3})\times (1-(\frac{4.8}{6})^{2}\times(\frac{5.7}{6})^{3})\\ \times(1-(\frac{1.5}{6})^{2}\times(\frac{1.5}{6})^{3})\times(1-(\frac{1.5}{6})^{2}\times(\frac{3.6}{6})^{3})\\\times(1-(\frac{1.5}{6})^{2}\times(\frac{5.7}{6})^{3}) \times(1-(\frac{3.6}{6})^{2}\times(\frac{3.6}{6})^{3})\\\times(1-(\frac{3.6}{6})^{2}\times(\frac{5.7}{6})^{3})\times(1-(\frac{5.7}{6})^{2}\times(\frac{5.7}{6})^{3}) \end{array} \right) ^{\frac{2}{4(4+1)}}}\right)^{\frac{1}{2+3}}\right)\right) \end{array} \right)\\ && = ((\flat_{2}, 0.9433), (\flat_{4}, 0.6685)). \end{eqnarray*} $ |
Property 4.7. (Idempotency) Let all $ \mathfrak{F}_{{{k}}}({{k}} = 1, 2, \ldots, \mathfrak{n}) $ are equal, i.e., $ \mathfrak{F}_{{{k}}} = \mathfrak{F} $ for all $ {{k}} $, then
$ 2TLBFGHM ^{\; s, t}{(\mathfrak{F}_{1}, \mathfrak{F}_{2}, \ldots, \mathfrak{F}_{n})} = \mathfrak{F}. $ |
Property 4.8. (Monotonicity) Let $ \mathfrak{F}_{{{k}}}({{k}} = 1, 2, \ldots, \mathfrak{n}) $ and $ \mathfrak{F}'_{{{k}}}({{k}} = 1, 2, \ldots, \mathfrak{n}) $ be two sets of 2TLBFNs. If $ \mathfrak{F}_{{{k}}}\geq \mathfrak{F}'_{{{k}}} $ for all $ {{k}} $, then
$ 2TLBFGHM ^{\; s, t}(\mathfrak{F}_{1}, \mathfrak{F}_{2}, \ldots, \mathfrak{F}_{n})\geq 2TLBFGHM^{\; s, t}(\mathfrak{F}'_{1}, \mathfrak{F}'_{2}, \ldots, \mathfrak{F}'_{n}). $ |
Property 4.9. (Boundedness)Let $ \mathfrak{F}_{{{k}}}({{k}} = 1, 2, \ldots, \mathfrak{n}) $ be a collection of 2TLBFNs, and let $ \mathfrak{F}^{-} = \min_{{{k}}}\mathfrak{F}_{{{k}}}, \; \mathfrak{F}^{+} = \max_{{{k}}}\mathfrak{F}_{{{k}}} $, then
$ \mathfrak{F}^{-}\leq 2TLBFGHM ^{\; s, t}(\mathfrak{F}_{1}, \mathfrak{F}_{2}, \ldots, \mathfrak{F}_{n})\leq \mathfrak{F}^{+}. $ |
Definition 4.4. Let $ s, t > 0, \mathfrak{F}_{{{k}}} = ((\flat_{l_{{{k}}}}, £_{{{k}}}), (\flat_{n_{{{k}}}}, \aleph_{{{k}}}))({{k}} = 1, 2, \ldots, \mathfrak{n}) $ be a collection of 2TLBFNs, $ \varkappa^{\star} = (\varkappa^{\star}_{1}, \varkappa^{\star}_{2}, \ldots, \varkappa^{\star}_{n})^{T} $ is the weight vector of $ \mathfrak{F}_{{k}} $, satisfying $ \varkappa^{\star}_{{{k}}} > 0 $ and $ \sum^{n}_{{{k}} = 1}\varkappa^{\star}_{{{k}}} = 1\; ({{k}} = 1, 2, \ldots, \mathfrak{n}) $. The 2TLBFWGHM operator is defined as follows:
$ \begin{equation} \text{2TLBFWGHM}_{\varkappa^{\star}}^{s, t}(\mathfrak{F}_{1}, \mathfrak{F}_{2}, \ldots, \mathfrak{F}_{n}) = \frac{1}{s+t} \left(\otimes_{{{j}} = 1}^{n}\otimes_{{{k}} = {{j}}}^{n}{(s \mathfrak{F}_{{{j}}} \oplus t \mathfrak{F}_{{{k}}})}^{\varkappa^{\star}_{{{j}}}\varkappa^{\star}_{{{k}}}}\right). \end{equation} $ | (4.4) |
Theorem 4.4. Let $ s, t > 0, \mathfrak{F}_{{{k}}} = ((\flat_{l_{{{k}}}}, £_{{{k}}}), (\flat_{n_{{{k}}}}, \aleph_{{{k}}}))({{k}} = 1, 2, \ldots, \mathfrak{n}) $ be a collection of 2TLBFNs. Then, the aggregated value by using the 2TLBFWGHM operator is also a 2TLBFN, and
$ \begin{eqnarray} && 2TLBFGWHM _{\varkappa^{\star}}^{s, t}{(\mathfrak{F}_{1}, \mathfrak{F}_{2}, \ldots, \mathfrak{F}_{n})} \\ && = \left(\begin{array}{l}\Delta\left(\sigma\left(1-\left(1-\prod\limits^{n}_{{{j}} = 1, {{k}} = {{j}}}\left(1-\left(1-\left(\frac{\Delta^{-1}(\flat_{l_{{{j}}}}, £_{{{j}}})}{\sigma}\right) \right)^{s}\left(1-\left(\frac{\Delta^{-1}(\flat_{l_{{{k}}}}, £_{{{k}}})}{\sigma}\right)\right)^{t}\right)^{\varkappa^{\star}_{{{j}}}\varkappa^{\star}_{{{k}}}}\right)^ {\frac{1}{s+t}}\right)\right), \\\Delta\left(\sigma{\left(1-\prod\limits^{n}_{{{j}} = 1, {{k}} = {{j}}}\left(1-\left(\frac{\Delta^{-1}(\flat_{n_{{{j}}}}, \aleph_{{{j}}})}{\sigma}\right)^{s} \left(\frac{\Delta^{-1}(\flat_{n_{{{k}}}}, \aleph_{{{k}}})}{\sigma}\right)^{t}\right)^ {\varkappa^{\star}_{{{j}}}\varkappa^{\star}_{{{k}}}}\right)^{\frac{1}{s+t}}}\right)\end{array}\right). \end{eqnarray} $ | (4.5) |
Example 4.4. Let $ \mathfrak{F}_{1} = ((\flat_{3}, 0.4), (\flat_{5}, 0.2)) $, $ \mathfrak{F}_{2} = ((\flat_{4}, 0.3), (\flat_{2}, -0.5)) $, $ \mathfrak{F}_{3} = ((\flat_{3}, 0.1), (\flat_{4}, -0.4)) $, and $ \mathfrak{F}_{4} = ((\flat_{5}, 0.2), (\flat_{6}, -0.3)) $ be four 2TLBFNs, and suppose $ s = 2 $ and $ t = 3 $, $ \varkappa^{\star} = (0.17, 0.32, 0.38, 0.13) $, then according to Theorem 4.4 we have
$ \begin{eqnarray*} && \text{2TLBFWGHM}_{\varkappa^{\star}}^{s, t}{(\mathfrak{F}_{1}, \mathfrak{F}_{2}, \ldots, \mathfrak{F}_{n})} = {\frac{1}{s+t}}\left( \otimes_{{{j}} = 1}^{n}\otimes_{{{k}} = {{j}}}^{n}{({s}\mathfrak{F}_{{{j}}} \oplus {t}\mathfrak{F}_{{{k}}})}^{\varkappa^{\star}_{{{j}}}\varkappa^{\star}_{{{k}}}}\right)\\ && = \left(\begin{array}{l} \Delta\left(6\left(1-\left( {1- \left( \begin{array}{l} \; \; \left(1-(1-\frac{3.4}{6})^{2}\times(1-\frac{3.4}{6})^{3}\right)^{0.17\times0.17}\times\left(1-(1-\frac{3.4}{6})^{2}\times(1-\frac{4.3}{6})^{3}\right)^{0.17\times0.32}\\\times\left(1-(1-\frac{3.4}{6})^{2}\times(1-\frac{3.1}{6})^{3}\right)^{0.17\times0.38}\times \left(1-(1-\frac{3.4}{6})^{2}\times(1-\frac{5.2}{6})^{3}\right)^{0.17\times0.13} \\\times\left(1-(1-\frac{4.3}{6})^{2}\times(1-\frac{4.3}{6})^{3}\right)^{0.32\times0.32}\times\left(1-(1-\frac{4.3}{6})^{2}\times(1-\frac{3.1}{6})^{3}\right)^{0.32\times0.38}\\\times\left(1-(1-\frac{4.3}{6})^{2}\times(1-\frac{5.2}{6})^{3}\right)^{0.32\times0.13} \times\left(1-(1-\frac{3.1}{6})^{2} \times(1-\frac{3.1}{6})^{3}\right)^{0.38\times0.38}\\\times\left(1-(1-\frac{3.1}{6})^{2}\times(1-\frac{5.2}{6})^{3}\right)^{0.38\times0.13}\times\left(1-(1-\frac{5.2}{6})^{2}\times(1-\frac{5.2}{6})^{3}\right)^{0.13\times0.13} \end{array}\right)} \right)^{\frac{1}{2+3}}\right)\right), \\ \Delta\left(6\left({1- \left( \begin{array}{l} \; \; (1-(\frac{5.2}{6})^{2}\times(\frac{5.2}{6})^{3})^{0.17\times0.17}\times(1-(\frac{5.2}{6})^{2}\times(\frac{1.5}{6})^{3})^{0.17\times0.32}\\\times(1-(\frac{5.2}{6})^{2}\times(\frac{3.6}{6})^{3})^{0.17\times0.38}\times (1-(\frac{5.2}{6})^{2}\times(\frac{5.7}{6})^{3})^{0.17\times0.13} \\\times(1-(\frac{1.5}{6})^{2}\times(\frac{1.5}{6})^{3})^{0.32\times0.32}\times(1-(\frac{1.5}{6})^{2}\times(\frac{3.6}{6})^{3})^{0.32\times0.38}\\\times(1-(\frac{1.5}{6})^{2}\times(\frac{5.7}{6})^{3})^{0.32\times0.13} \times(1-(\frac{3.6}{6})^{2}\times(\frac{3.6}{6})^{3})^{0.38\times0.38}\\\times(1-(\frac{3.6}{6})^{2}\times(\frac{5.7}{6})^{3})^{0.38\times0.13}\times(1-(\frac{5.7}{6})^{2}\times(\frac{5.7}{6})^{3})^{0.13\times0.13} \end{array} \right) }\right)^{\frac{1}{2+3}}\right) \end{array} \right).\\ && = ((\flat_{3}, 0.1564), (\flat_{3}, 0.7362)). \end{eqnarray*} $ |
Property 4.10. (Idempotency) Let all $ \mathfrak{F}_{{{k}}}({{k}} = 1, 2, \ldots, \mathfrak{n}) $ are equal, i.e., $ \mathfrak{F}_{{{k}}} = \mathfrak{F} $ for all $ {{k}} $, then
$ 2TLBFWGHM _{\varkappa^{\star}}^{s, t}{(\mathfrak{F}_{1}, \mathfrak{F}_{2}, \ldots, \mathfrak{F}_{n})} = \mathfrak{F}. $ |
Property 4.11. (Monotonicity) Let $ \mathfrak{F}_{{{k}}}({{k}} = 1, 2, \ldots, \mathfrak{n}) $ and $ \mathfrak{F}'_{{{k}}}({{k}} = 1, 2, \ldots, \mathfrak{n}) $ be two sets of 2TLBFNs. If $ \mathfrak{F}_{{{k}}}\geq \mathfrak{F}'_{{{k}}} $ for all $ {{k}} $, then
$ 2TLBFWGHM _{\varkappa^{\star}}^{s, t}(\mathfrak{F}_{1}, \mathfrak{F}_{2}, \ldots, \mathfrak{F}_{n})\geq {2TLBFWGHM}_{\varkappa^{\star}}^{s, t}(\mathfrak{F}'_{1}, \mathfrak{F}'_{2}, \ldots, \mathfrak{F}'_{n}). $ |
Property 4.12. (Boundedness)Let $ \mathfrak{F}_{{{k}}}({{k}} = 1, 2, \ldots, \mathfrak{n}) $ be a collection of 2TLBFNs, and let $ \mathfrak{F}^{-} = \min_{{{k}}}\mathfrak{F}_{{{k}}}, \; \mathfrak{F}^{+} = \max_{{{k}}}\mathfrak{F}_{{{k}}} $, then
$ \mathfrak{F}^{-}\leq {2TLBFWGHM}_{\varkappa^{\star}}^{s, t}(\mathfrak{F}_{1}, \mathfrak{F}_{2}, \ldots, \mathfrak{F}_{n})\leq \mathfrak{F}^{+}. $ |
We provide a novel approach to MAGDM in this section, based on the suggested G2TLBFWHM and 2TLBFWGHM operators. Consider $ \digamma = \{\digamma_{1}, \digamma_{2}, \ldots, \digamma_{m}\} $ be a set of alternatives, $ Q = \{Q_{1}, Q_{2}, \ldots, Q_{n}\} $ be the set of attributes, and $ D = \{D_{1}, D_{2}, \ldots, D_{l}\} $ be a set of experts. For attribute $ Q_{{{k}}}({{k}} = 1, 2, \ldots, \mathfrak{n}) $ of alternative $ \digamma_{{{j}}}({{j}} = 1, 2, \ldots, m) $, the DM $ D_{h}(h = 1, 2, \ldots, l) $ expresses his assessment by $ \mathfrak{F}_{{{j}} {{k}}}^{h} = ((\flat_{l_{{{j}}{{k}}}}^{h}, £_{{{j}}{{k}}}^{h}), (\flat_{n_{{{j}}{{k}}}}^{h}, \aleph_{{{j}}{{k}}}^{h})) $, which is a 2TLBFN defined on the 2TL term set $ \flat_{{{j}} {{k}}}^{h}\in S = (\flat_{0}, \flat_{1}, \flat_{2}, \ldots, \flat_{\sigma}) $. Thus, for each DM's an individual 2TLBF assessment matrix can be derived, which can be denoted as $ F^{h} = (\mathfrak{F}_{{{j}} {{k}}}^{h})_{m\times n} $. Let $ \varkappa^{\star} = (\varkappa^{\star}_{1}, \varkappa^{\star}_{2}, \ldots, \varkappa^{\star}_{n})^{T} $ be the weight vector of attributes, such that $ \varkappa^{\star}_{{{k}}}\in[0, 1] $, $ \sum\limits_{{{k}} = 1}^{n} \varkappa^{\star}_{{{k}}} = 1 $. Let $ \varkappa^{\star\star} = (\varkappa^{\star\star}_{1}, \varkappa^{\star\star}_{2}, \ldots, \varkappa^{\star\star}_{l})^{T} $ be the weight vector of DMs, satisfying $ \varkappa^{\star\star}_{h}\in[0, 1] $, $ \sum\limits_{h = 1}^{l} \varkappa^{\star\star}_{h} = 1 $. The essential steps for addressing the 2TLBF-MAGDM problem are described below:
$ \bf Step \ 1. $ Utilize the 2TLBFWA operator from Eq (3.3) and the 2TLBFWG operator from Eq (3.4) to aggregate all individual 2TLBF decision matrices $ F^{h} = (\mathfrak{F}_{{{j}}{{k}}}^{h})_{m \times n}(h = 1, 2, \ldots, l) $ into a collective 2TLBF decision matrix $ F = (\mathfrak{F}_{{{j}}{{k}}})_{m \times n} $.
$ \bf Step \ 2. $ Aggregate the 2TLBF evaluation values of alternative $ \digamma_{{{j}}} $ on all attributes $ Q_{{{k}}}({{k}} = 1, 2, \ldots, n) $ into the overall evaluation value of the alternative $ \digamma_{{{j}}}({{j}} = 1, 2, \ldots, m) $ by using the G2TLBFWHM operator from Eq (4.2) and the 2TLBFWGHM operator from Eq (4.5) to derive the overall preference values of the alternatives $ \digamma_{{{j}}}({{j}} = 1, 2, \ldots, m) $.
$\bf Step \ 3. $ Determine the score $ \mathcal{S}(\mathfrak{F}_{j}) $ of overall assessment value $ \mathfrak{F}_{{j}}({{j}} = 1, 2, \ldots, m) $ according to Def. 3.1.
$\bf Step \ 4. $ By ranking the alternatives $ \digamma_{{{j}}}({{j}} = 1, 2, \ldots, m) $ based on their score values, select the best alternative.
$\bf Step \ 5. $ End.
We give the following flowchart (Figure 1) to better explain the steps of the developed MAGDM approach in this paper.
Due to population growth and industrial development, the need for electrical energy is increasing rapidly. A long time ago, traditional energy sources like crude oil, natural gas, and coal were thought to be the main sources of generating electricity. Electricity prices are increasing rapidly over the last decade. Solar photovoltaic development is extending, as non-renewable resources are limited and pollution is increasing rapidly. Photovoltaic cells are commonly used as an economical and reliable energy source in different fields all across the world. As a result, academics and researchers work with government and different companies to enhance renewable energy standards and decreasing $ CO_2 $ emissions. Photovoltaic cells provide a cost-effective solution to this problem because it is close to demand areas and does not require extra transmission routes. Photovoltaic cells are essential for investors to conduct appropriate risk preference measures to guarantee that the project is implemented smoothly and that the expected benefits are achieved. Renewable energy sources have a rapid increase in electricity output, contributing 181 GW in 2018. Solar photovoltaic systems have the maximum capacity $ 55 $ percent, followed by wind power $ 28 $ percent, and hydropower $ 11 $ percent. The implementation of this technology provides a huge opportunity to increase system efficiency while reducing costs. A company's board of directors decided to reduce costs in order to increase profit. They observe that electricity is a major expenditure that can be reduced if solar energy is used to generate electricity. They have five alternatives of photovoltaic cells for their solar plant: Mono-crystalline photovoltaic cell $ (\digamma_{1}) $, Poly-crystalline photovoltaic cell $ (\digamma_{2}) $, Thin-film photovoltaic cell $ (\digamma_{3}) $, Amorphous silicon $ (\digamma_{4}) $, Copper indium diselenide $ (\digamma_{5}) $. They select a photovoltaic cell based on the following attributes: Heat absorption $ (Q_{1}) $, Expenditure $ (Q_{2}) $, Efficiency and reliability $ (Q_{3}) $, Ability of charge separation $ (Q_{4}) $. Furthermore, four attributes have weight vector that is $ \varkappa^{\star} = (0.23, 0.31, 0.27, 0.19)^{T} $, and $ \sum\limits_{{{k}} = 1}^{n} \varkappa^{\star}_{{{k}}} = 1 $. Whereas the experts believed that 2TL information is better choice for them. To select the optimal photovoltaic cell, three experts $ D_h(h = 1, 2, 3) $ are invited to give their assessments using LTS S = $\{ \flat_{0} = \text{extremely poor}, \; \flat_{1} = \text{very poor}, \; \flat_{2} = \text{poor}, \; \flat_{3} = \text{fair}, \; \flat_{4} = \text{good}, \; \flat_{5} = \text{very good}, \; \flat_{6} = \text{extremely good} \}$. The weight vector of these experts is $ \varkappa^{\star\star} = (0.3, 0.5, 0.2) $. The assessment values provided by the three experts for each attribute of each alternative are represented in the decision matrix $ F^{h} $ = $ (\mathfrak{F}_{{{j}}{{k}}}^{h})_{5 \times 4}(h = 1, 2, 3) $, as in Tables 1, 2, and 3, respectively.
$ Q_{1} $ | $ Q_{2} $ | $ Q_{3} $ | $ Q_{4} $ | |
$ \digamma_{1} $ | $ ((\flat_{3}, 0), (\flat_{5}, 0)) $ | $ ((\flat_{1}, 0), (\flat_{3}, 0)) $ | $ ((\flat_{2}, 0), (\flat_{4}, 0)) $ | $ ((\flat_{3}, 0), (\flat_{2}, 0)) $ |
$ \digamma_{2} $ | $ ((\flat_{4}, 0), (\flat_{3}, 0)) $ | $ ((\flat_{5}, 0), (\flat_{2}, 0)) $ | $ ((\flat_{2}, 0), (\flat_{3}, 0)) $ | $ ((\flat_{4}, 0), (\flat_{2}, 0)) $ |
$ \digamma_{3} $ | $ ((\flat_{1}, 0), (\flat_{4}, 0)) $ | $ ((\flat_{3}, 0), (\flat_{3}, 0)) $ | $ ((\flat_{1}, 0), (\flat_{3}, 0)) $ | $ ((\flat_{4}, 0), (\flat_{3}, 0)) $ |
$ \digamma_{4} $ | $ ((\flat_{2}, 0), (\flat_{6}, 0)) $ | $ ((\flat_{3}, 0), (\flat_{4}, 0)) $ | $ ((\flat_{5}, 0), (\flat_{3}, 0)) $ | $ ((\flat_{1}, 0), (\flat_{5}, 0)) $ |
$ \digamma_{5} $ | $ ((\flat_{4}, 0), (\flat_{1}, 0)) $ | $ ((\flat_{1}, 0), (\flat_{7}, 0)) $ | $ ((\flat_{6}, 0), (\flat_{1}, 0)) $ | $ ((\flat_{2}, 0), (\flat_{2}, 0)) $ |
$ Q_{1} $ | $ Q_{2} $ | $ Q_{3} $ | $ Q_{4} $ | |
$ \digamma_{1} $ | $ ((\flat_{2}, 0), (\flat_{5}, 0)) $ | $ ((\flat_{4}, 0), (\flat_{2}, 0)) $ | $ ((\flat_{3}, 0), (\flat_{5}, 0)) $ | $ ((\flat_{4}, 0), (\flat_{4}, 0)) $ |
$ \digamma_{2} $ | $ ((\flat_{4}, 0), (\flat_{1}, 0)) $ | $ ((\flat_{5}, 0), (\flat_{2}, 0)) $ | $ ((\flat_{6}, 0), (\flat_{1}, 0)) $ | $ ((\flat_{2}, 0), (\flat_{6}, 0)) $ |
$ \digamma_{3} $ | $ ((\flat_{3}, 0), (\flat_{2}, 0)) $ | $ ((\flat_{3}, 0), (\flat_{5}, 0)) $ | $ ((\flat_{2}, 0), (\flat_{3}, 0)) $ | $ ((\flat_{3}, 0), (\flat_{4}, 0)) $ |
$ \digamma_{4} $ | $ ((\flat_{2}, 0), (\flat_{6}, 0)) $ | $ ((\flat_{1}, 0), (\flat_{4}, 0)) $ | $ ((\flat_{3}, 0), (\flat_{5}, 0)) $ | $ ((\flat_{5}, 0), (\flat_{3}, 0)) $ |
$ \digamma_{5} $ | $ ((\flat_{5}, 0), (\flat_{1}, 0)) $ | $ ((\flat_{2}, 0), (\flat_{2}, 0)) $ | $ ((\flat_{3}, 0), (\flat_{1}, 0)) $ | $ ((\flat_{1}, 0), (\flat_{7}, 0)) $ |
$ Q_{1} $ | $ Q_{2} $ | $ Q_{3} $ | $ Q_{4} $ | |
$ \digamma_{1} $ | $ ((\flat_{1}, 0), (\flat_{6}, 0)) $ | $ ((\flat_{3}, 0), (\flat_{4}, 0)) $ | $ ((\flat_{2}, 0), (\flat_{5}, 0)) $ | $ ((\flat_{1}, 0), (\flat_{5}, 0)) $ |
$ \digamma_{2} $ | $ ((\flat_{4}, 0), (\flat_{4}, 0)) $ | $ ((\flat_{2}, 0), (\flat_{6}, 0)) $ | $ ((\flat_{1}, 0), (\flat_{7}, 0)) $ | $ ((\flat_{4}, 0), (\flat_{2}, 0)) $ |
$ \digamma_{3} $ | $ ((\flat_{2}, 0), (\flat_{3}, 0)) $ | $ ((\flat_{4}, 0), (\flat_{3}, 0)) $ | $ ((\flat_{3}, 0), (\flat_{3}, 0)) $ | $ ((\flat_{4}, 0), (\flat_{1}, 0)) $ |
$ \digamma_{4} $ | $ ((\flat_{3}, 0), (\flat_{5}, 0)) $ | $ ((\flat_{2}, 0), (\flat_{4}, 0)) $ | $ ((\flat_{6}, 0), (\flat_{1}, 0)) $ | $ ((\flat_{4}, 0), (\flat_{3}, 0)) $ |
$ \digamma_{5} $ | $ ((\flat_{7}, 0), (\flat_{1}, 0)) $ | $ ((\flat_{5}, 0), (\flat_{1}, 0)) $ | $ ((\flat_{4}, 0), (\flat_{1}, 0)) $ | $ ((\flat_{5}, 0), (\flat_{3}, 0)) $ |
In order to choose the most desirable photovoltaic cell, the G2TLBFWHM and 2TLBFWGHM operators are used to solve the MAGDM problem with 2TLBFNs, which involves the following computing steps:
$\bf Step \ 1. $ Utilizing the 2TLBFWA from Eq (3.3) and the 2TLBFWG from Eq (3.4), we fuse all assessment values to get the overall 2TLBFNs $ \mathfrak{F}_{{{j}}}({{j}} = 1, 2, 3, 4, 5) $ of the alternatives. The fused result is shown in Table 4.
$ Q_{1} $ | $ Q_{2} $ | $ Q_{3} $ | $ Q_{4} $ | |
G2TLBFWA operator | ||||
$ \digamma_1 $ | $ ((\flat_2, 0.1415), (\flat_5, 0.1857)) $ | $ ((\flat_3, 0.0529), (\flat_3, -0.4054)) $ | $ ((\flat_3, -0.4772), (\flat_5, -0.3238)) $ | $ ((\flat_3, 0.2166), (\flat_3, 0.3973)) $ |
$ \digamma_2 $ | $ ((\flat_4, 0.0000), (\flat_2, -0.1654)) $ | $ ((\flat_5, -0.4461), (\flat_2, 0.4915)) $ | $ ((\flat_4, 0.4274), (\flat_2, 0.0519)) $ | $ ((\flat_3, 0.1010), (\flat_3, 0.4641)) $ |
$ \digamma_3 $ | $ ((\flat_2, 0.2635), (\flat_3, -0.3297)) $ | $ ((\flat_3, 0.2182), (\flat_4, -0.1270)) $ | $ ((\flat_2, -0.0590), (\flat_3, 0.0000)) $ | $ ((\flat_4, -0.4721), (\flat_3, -0.2192)) $ |
$ \digamma_4 $ | $ ((\flat_2, 0.2148), (\flat_6, -0.2148)) $ | $ ((\flat_2, -0.1358), (\flat_4, 0.0000)) $ | $ ((\flat_4, 0.4287), (\flat_3, 0.1090)) $ | $ ((\flat_4, -0.0973), (\flat_3, 0.4968)) $ |
$ \digamma_5 $ | $ ((\flat_5, 0.3747), (\flat_1, 0.0000)) $ | $ ((\flat_3, -0.4705), (\flat_3, -0.4646)) $ | $ ((\flat_4, 0.3675), (\flat_1, 0.0000)) $ | $ ((\flat_2, 0.3582), (\flat_4, 0.0577)) $ |
2TLBFWG operator | ||||
$\digamma_1$ | $((\flat_2, -0.0337), (\flat_5, 0.2337))$ | $((\flat_2, 0.4915), (\flat_3, -0.2382))$ | $((\flat_2, 0.4495), (\flat_5, -0.2704))$ | $((\flat_3, -0.2192), (\flat_4, -0.2648))$ |
$\digamma_2$ | $((\flat_4, 0.0000), (\flat_2, 0.3421))$ | $((\flat_4, 0.1628), (\flat_3, 0.1836))$ | $((\flat_3, 0.0157), (\flat_4, -0.2879))$ | $((\flat_3, -0.1716), (\flat_5, -0.4641))$ |
$\digamma_3$ | $((\flat_2, -0.0104), (\flat_3, -0.1226))$ | $((\flat_3, 0.1777), (\flat_4, 0.1270))$ | $((\flat_2, -0.2383), (\flat_3, 0.0000))$ | $((\flat_3, 0.4641), (\flat_3, 0.2166))$ |
$\digamma_4$ | $((\flat_2, 0.1689), (\flat_6, -0.1689))$ | $((\flat_2, -0.4029), (\flat_4, 0.0000))$ | $((\flat_4, 0.0168), (\flat_4, -0.1426))$ | $((\flat_3, -0.0495), (\flat_4, -0.2896))$ |
$\digamma_5$ | $((\flat_5, 0.0018), (\flat_1, 0.0000))$ | $((\flat_2, -0.0488), (\flat_4, 0.3851))$ | $((\flat_4, -0.0878), (\flat_1, 0.0000))$ | $((\flat_2, -0.3014), (\flat_6, -0.3618))$ |
$\bf Step \ 2. $ Using Eqs (4.2) and (4.5) to aggregate the 2TLBF assessment values $ \mathfrak{F}_{{{j}}} $ of alternative $ \digamma_{{{j}}} $ on all attributes $ Q_{{{k}}} ({{k}} = 1, 2, 3, 4) $, into the overall assessment value $ \mathfrak{F}_{{{j}}} $ of the alternative $ \digamma_{{{j}}} \; ({{j}} = 1, 2, 3, 4, 5) $ (take $ s = 2 $ and $ t = 3 $). The overall assessment values of alternatives $ \digamma_{{{j}}}\; ({{j}} = 1, 2, 3, 4, 5) $ are shown in Table 5.
Alternatives | Overall assessment values by G2TLBFWHM | Overall assessment values by 2TLBFWGHM |
$ \digamma_{1} $ | $ ((\flat_3, -0.4392), (\flat_4, -0.0151)) $ | $ ((\flat_3, -0.1204), (\flat_4, -0.1222)) $ |
$ \digamma_{2} $ | $ ((\flat_4, -0.1760), (\flat_3, -0.1781)) $ | $ ((\flat_4, -0.1615), (\flat_3, 0.2750)) $ |
$ \digamma_{3} $ | $ ((\flat_3, -0.3760), (\flat_4, -0.4739)) $ | $ ((\flat_3, -0.0786), (\flat_3, 0.1544)) $ |
$ \digamma_{4} $ | $ ((\flat_3, 0.1383), (\flat_4, 0.1756)) $ | $ ((\flat_3, -0.0911), (\flat_4, 0.0569)) $ |
$ \digamma_{5} $ | $ ((\flat_4, -0.3497), (\flat_2, 0.2376)) $ | $ ((\flat_3, 0.1549), (\flat_4, -0.3829)) $ |
$ \bf Step \ 3. $ Determine the score function $ \mathcal{S}(\mathfrak{F}_{{{j}}}) $ of overall assessment value $ \mathfrak{F}_{{{j}}}\; ({{j}} = 1, 2, 3, 4, 5) $ utilizing Eq (3.1). Score function of alternatives by G2TLBFWHM and 2TLBFWGHM operators are as follows:
$ \begin{eqnarray*} \mathcal{S}^{HM}(\digamma_{1})& = &(\flat_3, 0.2880), \mathcal{S}^{HM}(\digamma_{2}) = (\flat_5, -0.4990), \mathcal{S}^{HM}(\digamma_{3}) = (\flat_4, -0.4510), \\ \mathcal{S}^{HM}(\digamma_{4})& = &(\flat_3, 0.4814), \mathcal{S}^{HM}(\digamma_{5}) = (\flat_5, -0.2937).\\\\ \mathcal{S}^{GHM}(\digamma_{1})& = &(\flat_4, -0.4991), \mathcal{S}^{GHM}(\digamma_{2}) = (\flat_4, 0.2817), \mathcal{S}^{GHM}(\digamma_{3}) = (\flat_4, -0.1164), \\ \mathcal{S}^{GHM}(\digamma_{4})& = &(\flat_3, 0.4260), \mathcal{S}^{GHM}(\digamma_{5}) = (\flat_4, -0.2311). \end{eqnarray*} $ |
$ \bf Step \ 4. $ Rank all alternatives $ \digamma_{{{j}}} \; ({{j}} = 1, 2, 3, 4, 5) $ according to the score index. The ranking of all alternatives is as: $ \digamma_{5} > \digamma_{2} > \digamma_{3} > \digamma_{4} > \digamma_{1} $ and $ \digamma_{2} > \digamma_{3} > \digamma_{5} > \digamma_{1} > \digamma_{4} $ utilizing G2TLBFWHM and 2TLBFWGHM operators, respectively. Therefore, $ \digamma_{5} $ or $ \digamma_{2} $ is the best choice.
$ \bf Step \ 5. $ End.
Different attribute weight values have a significant effect on the ranking of alternatives, as demonstrated in Tables 6 and 7. When $ \varkappa^{\star}_1 = 0.1, \varkappa^{\star}_2 = 0.2, \varkappa^{\star}_3 = 0.3, \; and \; \varkappa^{\star}_4 = 0.4 $, the five alternatives are ranked $ \digamma_5 > \digamma_2 > \digamma_4 > \digamma_3 > \digamma_1 $ in order of preference. In other words, $ \digamma_5 $ is the best alternative. When $ \varkappa^{\star}_1 = 0.2, \varkappa^{\star}_2 = 0.1, \varkappa^{\star}_3 = 0.3 $, and $ \varkappa^{\star}_4 = 0.4 $, than the ranking is $ \digamma_5 > \digamma_2 > \digamma_4 > \digamma_3 > \digamma_1 $, and still $ \digamma_5 $ is the best alternative. When $ \varkappa^{\star}_1 = 0.3, \varkappa^{\star}_2 = 0.1, \varkappa^{\star}_3 = 0.2 $, and $ \varkappa^{\star}_4 = 0.4 $, than the ranking is $ \digamma_5 > \digamma_2 > \digamma_3 > \digamma_4 > \digamma_1 $. Here is the slightest difference in the ranking of alternative, however $ \digamma_5 $ is the best alternative. When $ \varkappa^{\star}_1 = 0.4, \varkappa^{\star}_2 = 0.1, \varkappa^{\star}_3 = 0.2 $, and $ \varkappa^{\star}_4 = 0.3 $, than the ranking is $ \digamma_5 > \digamma_2 > \digamma_3 > \digamma_4 > \digamma_1 $ and $ \digamma_5 $ is the best alternative, as shown in Table 6 based on G2TLBFWHM operator. As a consequence, in the decision-making process the attributes weight can be varied to get appropriate decision results. Similarly the different outcomes by utilizing 2TLBFWGHM operator are shown in Table 7.
Weights | Scores | Ranking |
$ \varkappa^{\star}_{1}=0.1, \varkappa^{\star}_{2}=0.2, $ | $ \mathcal{S}^{HM}_{1}=(\flat_3, 0.3689), \mathcal{S}^{HM}_{2}=(\flat_4, 0.2697), \mathcal{S}^{HM}_{3}=(\flat_4, -0.2814), $ | $ {\digamma_{5} > \digamma_{2} > \digamma_{4} > \digamma_{3} > \digamma_{1}} $ |
$ \varkappa^{\star}_{3}=0.3, \varkappa^{\star}_{4}=0.4 $ | $ \mathcal{S}^{HM}_{4}=(\flat_4, -0.2213), \mathcal{S}^{HM}_{5}=(\flat_4, 0.3436) $ | |
$ \varkappa^{\star}_{1}=0.2, \varkappa^{\star}_{2}=0.1, $ | $ \mathcal{S}^{HM}_{1}=(\flat_3, 0.2348), \mathcal{S}^{HM}_{2}=(\flat_4, 0.2770), \mathcal{S}^{HM}_{3}=(\flat_4, -0.2690), $ | $ {\digamma_{5} > \digamma_{2} > \digamma_{4} > \digamma_{3} > \digamma_{1}} $ |
$ \varkappa^{\star}_{3}=0.3, \varkappa^{\star}_{4}=0.4 $ | $ \mathcal{S}^{HM}_{4}=(\flat_4, -0.2562), \mathcal{S}^{HM}_{5}=(\flat_5, -0.4185) $ | |
$ \varkappa^{\star}_{1}=0.3, \varkappa^{\star}_{2}=0.1, $ | $ \mathcal{S}^{HM}_{1}=(\flat_3, 0.2133), \mathcal{S}^{HM}_{2}=(\flat_4, 0.2652) \mathcal{S}^{HM}_{3}=(\flat_4, -0.2444), $ | $ {\digamma_{5} > \digamma_{2} > \digamma_{3} > \digamma_{4} > \digamma_{1}} $ |
$ \varkappa^{\star}_{3}=0.2, \varkappa^{\star}_{4}=0.4, $ | $ \mathcal{S}^{HM}_{4}=(\flat_4, -0.4446), \mathcal{S}^{HM}_{5}=(\flat_5, -0.3323) $ | |
$ \varkappa^{\star}_{1}=0.4, \varkappa^{\star}_{2}=0.1, $ | $ \mathcal{S}^{HM}_{1}=(\flat_3, 0.0671), \mathcal{S}^{HM}_{2}=(\flat_4, 0.4098), \mathcal{S}^{HM}_{3}=(\flat_4, -0.3131), $ | $ {\digamma_{5} > \digamma_{2} > \digamma_{3} > \digamma_{4} > \digamma_{1}} $ |
$ \varkappa^{\star}_{3}=0.2, \varkappa^{\star}_{4}=0.3, $ | $ \mathcal{S}^{HM}_{4}=(\flat_3, 0.3958), \mathcal{S}^{HM}_{5}=(\flat_5, -0.0206) $ |
Weights | Scores | Ranking |
$ \varkappa^{\star}_{1}=0.1, \varkappa^{\star}_{2}=0.2, $ | $ \mathcal{S}^{GHM}_{1}=(\flat_4, -0.3918), \mathcal{S}^{GHM}_{2}=(\flat_4, -0.0843), \mathcal{S}^{GHM}_{3}=(\flat_4, 0.0048), $ | $ {\digamma_{3} > \digamma_{2} > \digamma_{4} > \digamma_{1} > \digamma_{5}} $ |
$ \varkappa^{\star}_{3}=0.3, \varkappa^{\star}_{4}=0.4 $ | $ \mathcal{S}^{GHM}_{4}=(\flat_4, -0.2370), \mathcal{S}^{GHM}_{5}=(\flat_3, 0.2781) $ | |
$ \varkappa^{\star}_{1}=0.2, \varkappa^{\star}_{2}=0.1, $ | $ \mathcal{S}^{GHM}_{1}=(\flat_3, 0.4825), \mathcal{S}^{GHM}_{2}=(\flat_4, -0.0710), \mathcal{S}^{GHM}_{3}=(\flat_4, 0.0133), $ | $ {\digamma_{3} > \digamma_{2} > \digamma_{4} > \digamma_{1} > \digamma_{5}} $ |
$ \varkappa^{\star}_{3}=0.3, \varkappa^{\star}_{4}=0.4 $ | $ \mathcal{S}^{GHM}_{4}=(\flat_4, -0.3175), \mathcal{S}^{GHM}_{5}=(\flat_3, 0.4242) $ | |
$ \varkappa^{\star}_{1}=0.3, \varkappa^{\star}_{2}=0.1, $ | $ \mathcal{S}^{GHM}_{1}=(\flat_3, 0.4177), \mathcal{S}^{GHM}_{2}=(\flat_4, 0.0094), \mathcal{S}^{GHM}_{3}=(\flat_4, 0.0358), $ | $ {\digamma_{3} > \digamma_{2} > \digamma_{4} > \digamma_{5} > \digamma_{1}} $ |
$ \varkappa^{\star}_{3}=0.2, \varkappa^{\star}_{4}=0.4, $ | $ \mathcal{S}^{GHM}_{4}=(\flat_3, 0.4578), \mathcal{S}^{GHM}_{5}=(\flat_3, 0.4436) $ | |
$ \varkappa^{\star}_{1}=0.4, \varkappa^{\star}_{2}=0.1, $ | $ \mathcal{S}^{GHM}_{1}=(\flat_3, 0.2737), \mathcal{S}^{GHM}_{2}=(\flat_4, 0.1855), \mathcal{S}^{GHM}_{3}=(\flat_4, -0.0331), $ | $ {\digamma_{2} > \digamma_{3} > \digamma_{5} > \digamma_{1} > \digamma_{4}} $ |
$ \varkappa^{\star}_{3}=0.2, \varkappa^{\star}_{4}=0.3, $ | $ \mathcal{S}^{GHM}_{4}=(\flat_3, 0.2684), \mathcal{S}^{GHM}_{5}=(\flat_4, -0.1974) $ |
Surely, the parameters $ s $ and $ t $ have a great influence on the ranking results. The influence of parameters on score functions and ranking results based on G2TLBFWHM and 2TLBFWGHM operators are evaluated in this subsection. We fix the several values of $ s $ and $ t $, and evaluate the scores of the overall aggregation. Further, scores are used to rank the alternatives. In Tables 8 and 9 score values are evaluated by varying $ s $ and $ t $ based on G2TLBFWHM and 2TLBFGWHM operators, respectively. Then these scores are used to rank the alternatives. Ranking results from Table 10 are used to select the best alternative, $ \digamma_{5} $ and $ \digamma_{2} $ are the best alternatives based on G2TLBFWHM and 2TLBFWGHM operators, respectively.
Parameter values | Score functions |
$ s = t = 0.5 $ | $ \mathcal{S}^{HM}_{1} = (\flat_{2}, \; 0.3985), \; \; \mathcal{S}^{HM}_{2} = (\flat_{4}, -0.3920), \; \mathcal{S}^{HM}_{3} = (\flat_{3}, -0.3138), \; \mathcal{S}^{HM}_{4} = (\flat_{2}, \; 0.4184), \; \; \; \mathcal{S}^{HM}_{5} = (\flat_{4}, -0.3323) $ |
$ s = t = 1 $ | $ \mathcal{S}^{HM}_{1} = (\flat_{3}, -0.1830), \; \mathcal{S}^{HM}_{2} = (\flat_{4}, \; 0.0610), \; \; \mathcal{S}^{HM}_{3} = (\flat_{3}, \; 0.1018), \; \; \mathcal{S}^{HM}_{4} = (\flat_{3}, -0.1253), \; \; \mathcal{S}^{HM}_{5} = (\flat_{4}, \; 0.1457) $ |
$ s = t = 2 $ | $ \mathcal{S}^{HM}_{1} = (\flat_{3}, \; 0.1731), \; \; \mathcal{S}^{HM}_{2} = (\flat_{4}, \; 0.4197), \; \; \mathcal{S}^{HM}_{3} = (\flat_{3}, \; 0.4407), \; \; \; \mathcal{S}^{HM}_{4} = (\flat_{3}, \; 0.3053), \; \; \; \mathcal{S}^{HM}_{5} = (\flat_{5}, -0.4105) $ |
$ s = 3, t = 4 $ | $ \mathcal{S}^{HM}_{1} = (\flat_{3}, \; 0.4369), \; \; \mathcal{S}^{HM}_{2} = (\flat_{5}, -0.3629), \; \mathcal{S}^{HM}_{3} = (\flat_{4}, -0.3229), \; \mathcal{S}^{HM}_{4} = (\flat_{4}, -0.3379), \; \mathcal{S}^{HM}_{5} = (\flat_{5}, -0.0659) $ |
$ s = 4, t = 3 $ | $ \mathcal{S}^{HM}_{1} = (\flat_{3}, \; 0.4359), \; \; \mathcal{S}^{HM}_{2} = (\flat_{5}, -0.3434), \; \mathcal{S}^{HM}_{3} = (\flat_{4}, -0.3369), \; \mathcal{S}^{HM}_{4} = (\flat_{4}, -0.3654), \; \mathcal{S}^{HM}_{5} = (\flat_{5}, -0.0288) $ |
$ s = 5, t = 6 $ | $ \mathcal{S}^{HM}_{1} = (\flat_{4}, -0.3663), \; \mathcal{S}^{HM}_{2} = (\flat_{5}, -0.2087), \; \mathcal{S}^{HM}_{3} = (\flat_{4}, -0.1665), \; \mathcal{S}^{HM}_{4} = (\flat_{4}, -0.1007), \; \mathcal{S}^{HM}_{5} = (\flat_{5}, \; 0.2306) $ |
$ s = 6, t = 5 $ | $ \mathcal{S}^{HM}_{1} = (\flat_{4}, -0.3639), \; \mathcal{S}^{HM}_{2} = (\flat_{5}, -0.2005), \; \mathcal{S}^{HM}_{3} = (\flat_{4}, -0.1730), \; \mathcal{S}^{HM}_{4} = (\flat_{4}, -0.1050), \; \mathcal{S}^{HM}_{5} = (\flat_{5}, \; 0.2448) $ |
$ s = 7, t = 8 $ | $ \mathcal{S}^{HM}_{1} = (\flat_{4}, -0.2402), \; \mathcal{S}^{HM}_{2} = (\flat_{5}, -0.1187), \; \mathcal{S}^{HM}_{3} = (\flat_{4}, -0.0700), \; \mathcal{S}^{HM}_{4} = (\flat_{4}, \; 0.0445), \; \; \mathcal{S}^{HM}_{5} = (\flat_{5}, \; 0.4174) $ |
$ s = 8, t = 7 $ | $ \mathcal{S}^{HM}_{1} = (\flat_{4}, -0.2385), \; \mathcal{S}^{HM}_{2} = (\flat_{5}, -0.1144), \; \mathcal{S}^{HM}_{3} = (\flat_{4}, -0.0732), \; \mathcal{S}^{HM}_{4} = (\flat_{4}, \; 0.0446), \; \; \mathcal{S}^{HM}_{5} = (\flat_{5}, \; 0.4241) $ |
$ s = 1, t = 9 $ | $ \mathcal{S}^{HM}_{1} = (\flat_{4}, -0.3257), \; \mathcal{S}^{HM}_{2} = (\flat_{5}, -0.2461), \; \mathcal{S}^{HM}_{3} = (\flat_{4}, -0.1150), \; \mathcal{S}^{HM}_{4} = (\flat_{4}, \; 0.0041), \; \; \mathcal{S}^{HM}_{5} = (\flat_{5}, \; 0.1821) $ |
$ s = 9, t = 1 $ | $ \mathcal{S}^{HM}_{1} = (\flat_{4}, -0.3293), \; \mathcal{S}^{HM}_{2} = (\flat_{5}, -0.1503), \; \mathcal{S}^{HM}_{3} = (\flat_{4}, -0.1849), \; \mathcal{S}^{HM}_{4} = (\flat_{4}, -0.1453), \; \mathcal{S}^{HM}_{5} = (\flat_{5}, 0.3953) $ |
$ s = t = 10 $ | $ \mathcal{S}^{HM}_{1} = (\flat_{4}, -0.1355), \; \mathcal{S}^{HM}_{2} = (\flat_{5}, -0.0439), \; \mathcal{S}^{HM}_{3} = (\flat_{4}, 0.0097), \; \; \; \mathcal{S}^{HM}_{4} = (\flat_{4}, 0.1619), \; \; \; \mathcal{S}^{HM}_{5} = (\flat_{6}, -0.4265) $ |
Parameter values | Score functions |
$ s = t = 0.5 $ | $ \mathcal{S}^{GHM}_{1} = (\flat_{4}, 0.4425), \; \; \mathcal{S}^{GHM}_{2} = (\flat_{5}, 0.2342), \; \; \mathcal{S}^{GHM}_{3} = (\flat_{5}, -0.2274), \mathcal{S}^{GHM}_{4} = (\flat_{4}, 0.3902), \; \; \; \mathcal{S}^{GHM}_{5} = (\flat_{5}, 0.1811) $ |
$ s = t = 1 $ | $ \mathcal{S}^{GHM}_{1} = (\flat_{4}, -0.0219), \mathcal{S}^{GHM}_{2} = (\flat_{5}, -0.2048), \mathcal{S}^{GHM}_{3} = (\flat_{4}, 0.3335), \; \; \mathcal{S}^{GHM}_{4} = (\flat_{4}, -0.0868), \mathcal{S}^{GHM}_{5} = (\flat_{5}, -0.3859) $ |
$ s = t = 2 $ | $ \mathcal{S}^{GHM}_{1} = (\flat_{4}, -0.4094), \mathcal{S}^{GHM}_{2} = (\flat_{4}, 0.4340), \; \; \mathcal{S}^{GHM}_{3} = (\flat_{4}, -0.0223), \mathcal{S}^{GHM}_{4} = (\flat_{4}, -0.4951), \mathcal{S}^{GHM}_{5} = (\flat_{4}, 0.0061) $ |
$ s = 3, t = 4 $ | $ \mathcal{S}^{GHM}_{1} = (\flat_{3}, 0.3385), \; \; \mathcal{S}^{GHM}_{2} = (\flat_{4}, 0.1488), \; \; \mathcal{S}^{GHM}_{3} = (\flat_{4}, -0.2615), \mathcal{S}^{GHM}_{4} = (\flat_{3}, 0.2222), \; \; \mathcal{S}^{GHM}_{5} = (\flat_{3}, 0.4955) $ |
$ s = 4, t = 3 $ | $ \mathcal{S}^{GHM}_{1} = (\flat_{3}, 0.3027), \; \; \mathcal{S}^{GHM}_{2} = (\flat_{4}, 0.2111), \; \; \mathcal{S}^{GHM}_{3} = (\flat_{4}, -0.2736), \mathcal{S}^{GHM}_{4} = (\flat_{3}, 0.1584), \; \; \mathcal{S}^{GHM}_{5} = (\flat_{4}, -0.4688) $ |
$ s = 5, t = 6 $ | $ \mathcal{S}^{GHM}_{1} = (\flat_{3}, 0.1412), \; \; \mathcal{S}^{GHM}_{2} = (\flat_{4}, -0.0319), \mathcal{S}^{GHM}_{3} = (\flat_{4}, -0.4497), \mathcal{S}^{GHM}_{4} = (\flat_{3}, -0.0616), \mathcal{S}^{GHM}_{5} = (\flat_{3}, 0.1477) $ |
$ s = 6, t = 5 $ | $ \mathcal{S}^{GHM}_{1} = (\flat_{3}, 0.1198), \; \; \mathcal{S}^{GHM}_{2} = (\flat_{4}, 0.0002), \; \; \mathcal{S}^{GHM}_{3} = (\flat_{4}, -0.4552), \mathcal{S}^{GHM}_{4} = (\flat_{3}, -0.0884), \mathcal{S}^{GHM}_{5} = (\flat_{3}, 0.1650) $ |
$ s = 7, t = 8 $ | $ \mathcal{S}^{GHM}_{1} = (\flat_{3}, 0.0215), \; \; \mathcal{S}^{GHM}_{2} = (\flat_{4}, -0.1589), \mathcal{S}^{GHM}_{3} = (\flat_{3}, 0.4237), \; \; \mathcal{S}^{GHM}_{4} = (\flat_{3}, -0.2577), \mathcal{S}^{GHM}_{5} = (\flat_{3}, -0.0660) $ |
$ s = 8, t = 7 $ | $ \mathcal{S}^{GHM}_{1} = (\flat_{3}, 0.0074), \; \; \mathcal{S}^{GHM}_{2} = (\flat_{4}, -0.1413), \mathcal{S}^{GHM}_{3} = (\flat_{3}, 0.4217), \; \; \mathcal{S}^{GHM}_{4} = (\flat_{3}, -0.2677), \mathcal{S}^{GHM}_{5} = (\flat_{3}, -0.0561) $ |
$ s = 1, t = 9 $ | $ \mathcal{S}^{GHM}_{1} = (\flat_{3}, 0.2199), \; \; \mathcal{S}^{GHM}_{2} = (\flat_{4}, -0.1981), \mathcal{S}^{GHM}_{3} = (\flat_{4}, -0.4622), \mathcal{S}^{GHM}_{4} = (\flat_{3}, 0.0421), \; \; \mathcal{S}^{GHM}_{5} = (\flat_{3}, 0.0067) $ |
$ s = 9, t = 1 $ | $ \mathcal{S}^{GHM}_{1} = (\flat_{3}, 0.0159), \; \; \mathcal{S}^{GHM}_{2} = (\flat_{4}, 0.1304), \; \; \mathcal{S}^{GHM}_{3} = (\flat_{3}, 0.4816), \; \; \mathcal{S}^{GHM}_{4} = (\flat_{3}, -0.2845), \mathcal{S}^{GHM}_{5} = (\flat_{3}, 0.2408) $ |
$ s = t = 10 $ | $ \mathcal{S}^{GHM}_{1} = (\flat_{3}, -0.0821), \mathcal{S}^{GHM}_{2} = (\flat_{4}, -0.2708), \mathcal{S}^{GHM}_{3} = (\flat_{3}, 0.3125), \; \mathcal{S}^{GHM}_{4} = (\flat_{3}, -0.4277), \mathcal{S}^{GHM}_{5} = (\flat_{3}, -0.2376) $ |
Parameters | G2TLBFWHM operator | 2TLBFWGHM operator |
$ s = t = 0.5 $ | $ \digamma_{5} > \digamma_{2} > \digamma_{3} > \digamma_{4} > \digamma_{1} $ | $ \digamma_{2} > \digamma_{5} > \digamma_{3} > \digamma_{1} > \digamma_{4} $ |
$ s = t = 1 $ | $ \digamma_{5} > \digamma_{2} > \digamma_{3} > \digamma_{4} > \digamma_{1} $ | $ \digamma_{2} > \digamma_{5} > \digamma_{3} > \digamma_{1} > \digamma_{4} $ |
$ s = t = 2 $ | $ \digamma_{5} > \digamma_{2} > \digamma_{3} > \digamma_{4} > \digamma_{1} $ | $ \digamma_{2} > \digamma_{5} > \digamma_{3} > \digamma_{1} > \digamma_{4} $ |
$ s = 3, t = 4 $ | $ \digamma_{5} > \digamma_{2} > \digamma_{3} > \digamma_{4} > \digamma_{1} $ | $ \digamma_{2} > \digamma_{3} > \digamma_{5} > \digamma_{1} > \digamma_{4} $ |
$ s = 4, t = 3 $ | $ \digamma_{5} > \digamma_{2} > \digamma_{3} > \digamma_{4} > \digamma_{1} $ | $ \digamma_{2} > \digamma_{3} > \digamma_{5} > \digamma_{1} > \digamma_{4} $ |
$ s = 5, t = 6 $ | $ \digamma_{5} > \digamma_{2} > \digamma_{4} > \digamma_{3} > \digamma_{1} $ | $ \digamma_{2} > \digamma_{3} > \digamma_{5} > \digamma_{1} > \digamma_{4} $ |
$ s = 6, t = 5 $ | $ \digamma_{5} > \digamma_{2} > \digamma_{4} > \digamma_{3} > \digamma_{1} $ | $ \digamma_{2} > \digamma_{3} > \digamma_{5} > \digamma_{1} > \digamma_{4} $ |
$ s = 7, t = 8 $ | $ \digamma_{5} > \digamma_{2} > \digamma_{4} > \digamma_{3} > \digamma_{1} $ | $ \digamma_{2} > \digamma_{3} > \digamma_{1} > \digamma_{5} > \digamma_{4} $ |
$ s = 8, t = 7 $ | $ \digamma_{5} > \digamma_{2} > \digamma_{4} > \digamma_{3} > \digamma_{1} $ | $ \digamma_{2} > \digamma_{3} > \digamma_{1} > \digamma_{5} > \digamma_{4} $ |
$ s = 1, t = 9 $ | $ \digamma_{5} > \digamma_{2} > \digamma_{4} > \digamma_{3} > \digamma_{1} $ | $ \digamma_{2} > \digamma_{3} > \digamma_{1} > \digamma_{4} > \digamma_{5} $ |
$ s = 9, t = 1 $ | $ \digamma_{5} > \digamma_{2} > \digamma_{4} > \digamma_{3} > \digamma_{1} $ | $ \digamma_{2} > \digamma_{3} > \digamma_{5} > \digamma_{1} > \digamma_{4} $ |
$ s = t = 10 $ | $ \digamma_{5} > \digamma_{2} > \digamma_{4} > \digamma_{3} > \digamma_{1} $ | $ \digamma_{2} > \digamma_{3} > \digamma_{1} > \digamma_{5} > \digamma_{4} $ |
As the values of $ s $ and $ t $ vary at the same time, the scores of the five alternatives change as well, resulting in an irregular change accordingly, as shown in Tables 8 and 9 based on G2TLBFWHM and 2TLBFWGHM operators. The change in values of $ s $ and $ t $ have a significant influence on the results of the alternatives ranking. Table 10 demonstrates that when $ s $ and $ t $ are changed, the ranking results are relatively stable, and the best alternative remained unchanged. The decision preference can be represented in the actual decision-making process by varying the values of $ s $ and $ t $ to obtain the best decision results.
Based on the G2TLBFWHM operator, the scores vary accordingly, corresponding to different values of $ s $ and $ t $ for alternatives $ \digamma_{{{j}}}({{j}} = 1, 2, 3, 4, 5) $ as shown in Figures 2–6. In Figure 7 the value of parameter $ s = 3 $ is fixed, and $ t $ is varied from 1 to 10. The value of parameter $ t = 3 $ is fixed, and $ s $ is varied from 1 to 10 in Figure 8.
Based on the 2TLBFWGHM operator, the scores vary accordingly, corresponding to different values of $ s $ and $ t $ for alternatives $ \digamma_{{{j}}}({{j}} = 1, 2, 3, 4, 5) $ as shown in Figures 9–13. In Figure 14 the value of parameter $ s = 3 $ is fixed, and $ t $ is varied from 1 to 10. In Figure 15 the value of parameter $ t = 3 $ is fixed, and $ s $ is varied from 1 to 10.
When the parameters $ s $ and $ t $ are assigned the same values, the ranking results of the G2TLBFWHM and 2TLBFWGHM operators are not the same, and the score values vary differently. G2TLBFWHM and 2TLBFWGHM operators consider the relationship between two input arguments. These results indicate that the proposed methods are flexible. In actual decision-making, the parameters could be varied based on DM's preferences. The gloomy decision-maker could choose smaller parameters, whereas the optimistic decision-maker could choose larger parameters. Consequently, these results indicate that the suggested G2TLBFWHM and 2TLBFWGHM operators are more flexible and adaptable. Also the proposed strategy are most effective, realistic, and sufficient to address real-world MAGDM problems.
The ability to consider the interrelationship among the 2TLBFNs is a unique feature of the G2TLBFWHM and 2TLBFWGHM operators. To demonstrate the effectiveness of the suggested operators, we provide comparative analysis. To verify the validity of the developed approach, we utilize different approaches to solve the above mentioned MAGDM problem in Subsection 6.1. These methods include the 2TLBFWA operator, 2TLBFWG operator, the 2TL bipolar fuzzy weighted Hamy mean [37] (2TLBFWHM) operator, the 2TL bipolar fuzzy weighted dual Hamy mean [38] (2TLBFWDHM) operator, the 2TL bipolar fuzzy weighted Maclaurin symmetric mean [39] (2TLBFWMSM) operator, and the 2TL bipolar fuzzy weighted dual Maclaurin symmetric mean [40] (2TLBFWDMSM) operator. Detailed evaluation results gained using different MAGDM approaches are given in Tables 11–16.
2TLBFWA | Scores values | The order | |
$ \digamma_{1} $ | $ ((\flat_3, -0.2530), (\flat_4, -0.2456)) $ | $ (\flat_3, 0.4963) $ | $ {\digamma_{5} > \digamma_{2} > \digamma_{3} > f \digamma_{4} > \digamma_{1}} $ |
$ \digamma_{2} $ | $ ((\flat_4, 0.1497), (\flat_2, 0.3458)) $ | $ (\flat_5, -0.0981) $ | |
$ \digamma_{3} $ | $ ((\flat_3, -0.2482), (\flat_3, 0.1161)) $ | $ (\flat_4, -0.1822) $ | |
$ \digamma_{4} $ | $ ((\flat_3, 0.1559), (\flat_4, -0.0348)) $ | $ (\flat_4, -0.4046) $ | |
$ \digamma_{5} $ | $ ((\flat_4, -0.1614), (\flat_2, -0.2589)) $ | $ (\flat_5, 0.0488) $ |
2TLBFWG | Scores values | The order | |
$ \digamma_{1} $ | $ ((\flat_2, 0.3982), (\flat_4, 0.1699)) $ | $ (\flat_3, 0.1142) $ | $ {\digamma_{2} > \digamma_{5} > \digamma_{3} > \digamma_{1} > \digamma_{2}} $ |
$ \digamma_{2} $ | $ ((\flat_4, -0.4867), (\flat_3, 0.4502)) $ | $ (\flat_4, 0.0316) $ | |
$ \digamma_{3} $ | $ ((\flat_2, 0.4733), (\flat_3, 0.3939)) $ | $ (\flat_4, -0.4603) $ | |
$ \digamma_{4} $ | $ ((\flat_2, 0.4698), (\flat_4, 0.4454)) $ | $ (\flat_3, 0.0122) $ | |
$ \digamma_{5} $ | $ ((\flat_3, -0.1526), (\flat_3, 0.3604)) $ | $ (\flat_4, -0.2565) $ |
2TLBFWHM | Scores values | The order | |
$ \digamma_{1} $ | $ ((\flat_6, 0.1111), (\flat_1 0.2620)) $ | $ (\flat_6, 0.4246) $ | $ {\digamma_{2} > \digamma_{5} > \digamma_{3} > \digamma_{4} > \digamma_{1}} $ |
$ \digamma_{2} $ | $ ((\flat_7, -0.2399), (\flat_1-0.3123)) $ | $ (\flat_7, 0.0362) $ | |
$ \digamma_{3} $ | $ ((\flat_6, 0.0880), (\flat_1-0.0719)) $ | $ (\flat_7, -0.4201) $ | |
$ \digamma_{4} $ | $ ((\flat_6, 0.2394), (\flat_1 0.3353)) $ | $ (\flat_6, 0.4520) $ | |
$ \digamma_{5} $ | $ ((\flat_7, -0.4646), (\flat_1-0.4275)) $ | $ (\flat_7, -0.0185) $ |
2TLBFWDHM | Scores values | The order | |
$ \digamma_{1} $ | $ ((\flat_1, -0.3159), (\flat_7, -0.2518)) $ | $ (\flat_1, -0.0321) $ | $ {\digamma_{5} > \digamma_{2} > \digamma_{3} > \digamma_{1} > \digamma_{4}} $ |
$ \digamma_{2} $ | $ ((\flat_1, 0.0870), (\flat_6, 0.4438)) $ | $ (\flat_1, 0.3216) $ | |
$ \digamma_{3} $ | $ ((\flat_1, -0.2613), (\flat_6, 0.4293)) $ | $ (\flat_1, 0.1547) $ | |
$ \digamma_{4} $ | $ ((\flat_1, -0.2369), (\flat_7, -0.1422)) $ | $ (\flat_1, -0.0473) $ | |
$ \digamma_{5} $ | $ ((\flat_1, -0.0449), (\flat_6, -0.0374)) $ | $ (\flat_1, 0.4962) $ |
2TLBFWMSM | Scores values | The order | |
$ \digamma_{1} $ | $ ((\flat_6, 0.1130), (\flat_1, 0.2595)) $ | $ (\flat_6, 0.4267) $ | $ {\digamma_{2} > \digamma_{5} > \digamma_{3} > \digamma_{4} > \digamma_{1}} $ |
$ \digamma_{2} $ | $ ((\flat_7, -0.2398), (\flat_1, -0.3126)) $ | $ (\flat_7, 0.0364) $ | |
$ \digamma_{3} $ | $ ((\flat_6, 0.0917), (\flat_1, -0.0734)) $ | $ (\flat_7, -0.4175) $ | |
$ \digamma_{4} $ | $ ((\flat_6, 0.2487), (\flat_1, 0.3320)) $ | $ (\flat_6, 0.4584) $ | |
$ \digamma_{5} $ | $ ((\flat_7, -0.4588), (\flat_1, -0.4291)) $ | $ (\flat_7, -0.0149) $ |
2TLBFWDMSM | Scores values | The order | |
$ \digamma_{1} $ | $ ((\flat_1, -0.3161), (\flat_7, -0.2470)) $ | $ (\flat_1, -0.0346) $ | $ {\digamma_{5} > \digamma_{2} > \digamma_{3} > \digamma_{1} > \digamma_{4}} $ |
$ \digamma_{2} $ | $ ((\flat_1, 0.0852), (\flat_6, 0.4470)) $ | $ (\flat_1, 0.3191) $ | |
$ \digamma_{3} $ | $ ((\flat_1, -0.2622), (\flat_6, 0.4300)) $ | $ (\flat_1, 0.1539) $ | |
$ \digamma_{4} $ | $ ((\flat_1, -0.2383), (\flat_7, -0.1403)) $ | $ (\flat_1, -0.0490) $ | |
$ \digamma_{5} $ | $ ((\flat_1, -0.0483), (\flat_6, -0.0121)) $ | $ (\flat_1, 0.4819) $ |
We compare our suggested approach to other approaches such as the 2TLBFWA and 2TLBFWG operators. Tables 11, 12 indicates that the 2TLBFWA and 2TLBFWG operators are unable to provide the interrelationship between the 2TLBFNs. In the above example, we should consider not only the attribute values of each photovoltaic cells, but also the correlations between these attributes while selecting the most optimal photovoltaic cell. Approaches based on 2TLBFWA and 2TLBFWG operators are ineffective in dealing with this issue. Our approach is more appropriate for dealing with this issue than, 2TLBFWA and 2TLBFWG operators as it can capture parameter correlations. The interrelationship between the 2TLBFNs is evaluated by the G2TLBFHM, G2TLBFWHM, 2TLBFGHM, and 2TLBFWGHM operators. In addition, 2TLBFWA and 2TLBFWG operators do not have any parameters, but our purposed operator has two parameters, that make our operator more flexible and adaptable. Here, we propose a novel approach for MAGDM problems based on 2TLBFS, which is an influential approach for demonstrating and indicating DM's assessments. As a result, compared with different approaches, our approach has some benefits and superiorities.
The ranking effects of the above approaches are slightly different, as shown in the above calculations, but still, the best alternative is $ \digamma_{2} $ or $ \digamma_{5} $. It shows that the G2TLBFWHM and 2TLBFWGHM operators are more effective and appropriate with 2TLBFNs for MAGDM problems. The 2TLBFS is more general and contains more information in the MAGDM process. As a result, in MAGDM, our proposed method gives more general and powerful information.
$ \bf(1) $ The drawback of 2TLBFWA and 2TLBFWG is that in the process of fusion the interrelationship between arguments is not taken into account. In other words, they assume that all attributes are identical, which is to some extent inaccurate. To select the most desirable photovoltaic cell, in an illustrative example, we not only take into account the attribute values of each alternative but also their interrelationship. As a result, the 2TLBFWA and 2TLBFWG techniques are inapplicable to this situation. Our technique is more practical to tackle this problem than 2TLBFWA and 2TLBFWG techniques because it can easily capture interrelationships among multi-input arguments.
$ \bf(2) $ Since both G2TLBFWHM and 2TLBFWGHM operators can consider the correlation among multi-input arguments, the optimal alternative is selected in the same way, demonstrating the rationality and validity of the proposed operators. Furthermore, 2TLBFWHM, 2TLBFWDHM, 2TLBFWMSM, and 2TLBFWDMSM operators do not reduce the effects of extremely strong or weak ranking outcomes, whereas our suggested method does. This shows our proposed technique is more straightforward and superior.
Choosing the optimal photovoltaic cell is essential for a company's board of directors. The selection of an appropriate photovoltaic cell not only increases profit but also reduces expenditures. As a result, choosing the best photovoltaic cell is an important MAGDM problem. In comparison to the classical and FSs, a BFS gives better precision, flexibility, and compatibility for the system. In this article, we investigated the MAGDM problem by combining the concepts of 2TL terms and HM operators with BF numbers. As a result of being inspired by the generalized HM operator and the geometric HM operator, we introduced some G2TLBFHM and 2TLBFGHM aggregation operators, as well as their weighted forms. The G2TLBFWHM and 2TLBFWGHM operators were different from other operators not only by accommodating the 2TLBFNs as well as by considering the interdependent phenomena among the arguments. Providing our operators to have a broader range of practical application potentials, a numerical example has been provided to evaluate the developed approach and demonstrate the feasibility and usefulness of the suggested method. By using the illustrated example, we can see that the parameters of the aggregation operators influence the ranking of alternatives. The significance of the parameters $ s $ and $ t $ on decision-making results and comparative analysis have been demonstrated.
On the other hand, there are still some limitations in this research study. Firstly, this work only deals with the aggregation of 2TLBFNs. Secondly, this research is only applied to the evaluation of photovoltaic cells with 2TLBFS. In fact, it has broad implications for assessment methods and related fields such as cognitive computation, engineering, natural and artificial cognitive systems, and management applications.s To overcome discussed limitations, in the future work, we will integrate this approach with other generalized FSs, including dual hesitant $ q $-ROF 2-tuple linguistic sets, probability hesitant FSs, cloud approaches, and so on, to enlarge the area for expression of assessment results, adapt to a wider range of assessment environments, and increase the versatility of the strategy. Furthermore, we will investigate more aggregation operators for fusing 2TLBFNs, such as the 2TLBF Bonferroni operators, the 2TLBF power Bonferroni operators, and the 2TLBF Muirhead mean operators, which can capture the interrelationships among given input attributes.
The authors extend their appreciation to the Deanship of Scientific Research at King Khalid University for funding this work through General Research Project under grant number (R.G.P.1./258/43).
The authors declare there is no conflict of interest.
[1] |
Z. Ali, T. Mahmood, K. Ullah, Q. Khan, Einstein geometric aggregation operators using a novel complex interval-valued pythagorean fuzzy setting with application in green supplier chain management, Rep. Mech. Eng., 2 (2021), 105–134. https://doi.org/10.31181/rme2001020105t doi: 10.31181/rme2001020105t
![]() |
[2] |
R. Sahu, S. R. Dash, S. Das, Career selection of students using hybridized distance measure based on picture fuzzy set and rough set theory, Decis. Making Appl. Manage. Eng., 4 (2021), 104–126. https://doi.org/10.31181/dmame2104104s doi: 10.31181/dmame2104104s
![]() |
[3] | L. A. Zadeh, Fuzzy sets, Inf. Control, 8 (1996), 338–353. https://doi.org/10.1016/S0019-9958(65)90241-X |
[4] | K. T. Atanassov, Intuitionistic fuzzy sets, in Intuitionistic Fuzzy Sets, Physica, Heidelberg, (1999), 1–137. https://doi.org/10.1007/978-3-7908-1870-3_1 |
[5] | W. R. Zhang, Bipolar fuzzy sets and relations: a computational framework for cognitive modeling and multiagent decision analysis, in NAFIPS/IFIS/NASA'94. Proceedings of the First International Joint Conference of The North American Fuzzy Information Processing Society Biannual Conference. The Industrial Fuzzy Control and Intellige, (1994), 305–309. https://doi.org/10.1109/IJCF.1994.375115 |
[6] |
M. Akram, U. Amjad, B. Davvaz, Decision-making analysis based on bipolar fuzzy N-soft information, Comput. Appl. Math., 40 (2021), 1–39. https://doi.org/10.1007/s40314-021-01570-y doi: 10.1007/s40314-021-01570-y
![]() |
[7] |
M. Zhao, G. Wei, C. Wei, Y. Guo, CPT-TODIM method for bipolar fuzzy multi-attribute group decision making and its application to network security service provider selection, Int. J. Intell. Syst., 36 (2021), 1943–1969. https://doi.org/10.1002/int.22367 doi: 10.1002/int.22367
![]() |
[8] | M. Akram, M. Sarwar, W. A. Dudek, Graphs for the analysis of bipolar fuzzy information, Springer, 2021. https://doi.org/10.1007/978-981-15-8756-6 |
[9] |
S. Poulik, G. Ghorai, Determination of journeys order based on graph's Wiener absolute index with bipolar fuzzy information, Inf. Sci., 545 (2021), 608–619. https://doi.org/10.1016/j.ins.2020.09.050 doi: 10.1016/j.ins.2020.09.050
![]() |
[10] |
M. Akram, T. Allahviranloo, W. Pedrycz, M. Ali, Methods for solving LR-bipolar fuzzy linear systems, Soft Comput., 25 (2021), 85–108. https://doi.org/10.1007/s00500-020-05460-z doi: 10.1007/s00500-020-05460-z
![]() |
[11] |
G. Ali, M. Akram, J. C. R. Alcantud, Attributes reductions of bipolar fuzzy relation decision systems, Neural Comput. Appl., 32 (2020), 10051–10071. https://doi.org/10.1007/s00521-019-04536-8 doi: 10.1007/s00521-019-04536-8
![]() |
[12] |
M. Akram, Shumaiza, A. N. Al-Kenani, Multi-criteria group decision-making for selection of green suppliers under bipolar fuzzy PROMETHEE process, Symmetry, 12 (2020), 77. https://doi.org/10.3390/sym12010077 doi: 10.3390/sym12010077
![]() |
[13] |
M. Akram, M. Arshad, Bipolar fuzzy TOPSIS and bipolar fuzzy ELECTRE-I methods to diagnosis, Comput. Appl. Math., 39 (2020), 1–21. https://doi.org/10.1007/s40314-019-0980-8 doi: 10.1007/s40314-019-0980-8
![]() |
[14] |
S. Naz, M. Akram, Novel decision-making approach based on hesitant fuzzy sets and graph theory, Comput. Appl. Math., 38 (2019), 1–26. https://doi.org/10.1007/s40314-019-0773-0 doi: 10.1007/s40314-019-0773-0
![]() |
[15] |
M. Akram, S. Naz, S. A. Edalatpanah, R. Mehreen, Group decision-making framework under linguistic q-rung orthopair fuzzy Einstein models, Soft Comput., 25 (2021), 10309–10334. https://doi.org/10.1007/s00500-021-05771-9 doi: 10.1007/s00500-021-05771-9
![]() |
[16] |
P. Liu, S. Naz, M. Akram, M. Muzammal, Group decision-making analysis based on linguistic q-rung orthopair fuzzy generalized point weighted aggregation operators, Int. J. Mach. Learn. Cybern., 2021 (2021), 1–24. https://doi.org/10.1007/s13042-021-01425-2 doi: 10.1007/s13042-021-01425-2
![]() |
[17] |
M. Akram, S. Naz, F. Ziaa, Novel decision-making framework based on complex q-rung orthopair fuzzy information, Scientia Iran., 2021 (2021), 1–34. https://doi.org/10.24200/SCI doi: 10.24200/SCI
![]() |
[18] |
S. Naz, M. Akram, S. Alsulami, F. Ziaa, Decision-making analysis under interval-valued q-rung orthopair dual hesitant fuzzy environment, Int. J. Comput. Intell. Syst., 14 (2021), 332–357. https://doi.org/10.2991/ijcis.d.201204.001 doi: 10.2991/ijcis.d.201204.001
![]() |
[19] | M. Akram, S. Naz, F. Smarandache, Generalization of maximizing deviation and TOPSIS method for MADM in simplified neutrosophic hesitant fuzzy environment, Symmetry, 11 (8), 1058. https://doi.org/10.3390/sym11081058 |
[20] | H. Garg, S. Naz, F. Ziaa, Z. Shoukat, A ranking method based on Muirhead mean operator for group decision making with complex interval-valued q-rung orthopair fuzzy numbers, Soft Comput., 25 (2021), 14001–14027. https://doi.org/10.1007/s00500-021-06231-0 |
[21] |
F. Herrera, L. Martínez, A 2-tuple fuzzy linguistic representation model for computing with words, IEEE Trans. Fuzzy Syst., 8 (2000), 746–752. https://doi.org/10.1109/91.890332 doi: 10.1109/91.890332
![]() |
[22] |
L. Martı, F. Herrera, An overview on the 2-tuple linguistic model for computing with words in decision making: extensions, applications and challenges, Inf. Sci., 207 (2012), 1–18. https://doi.org/10.1016/j.ins.2012.04.025 doi: 10.1016/j.ins.2012.04.025
![]() |
[23] |
Y. Zhang, G. Wei, Y. Guo, C. Wei, TODIM method based on cumulative prospect theory for multiple attribute group decision-making under 2-tuple linguistic pythagorean fuzzy environment, Int. J. Intell. Syst., 36 (2021), 2548–2571. https://doi.org/10.1002/int.22393 doi: 10.1002/int.22393
![]() |
[24] |
S. Faizi, W. Sacabun, S. Nawaz, A. Rehman, J. Watróbski, Best-worst method and Hamacher aggregation operations for intuitionistic 2-tuple linguistic sets, Expert Syst. Appl., 181 (2021), 115088. https://doi.org/10.1016/j.eswa.2021.115088 doi: 10.1016/j.eswa.2021.115088
![]() |
[25] |
A. Labella, B. Dutta, L. Martinez, An optimal best-worst prioritization method under a 2-tuple linguistic environment in decision making, Comput. Ind. Eng., 155 (2021), 107141. https://doi.org/10.1016/j.cie.2021.107141 doi: 10.1016/j.cie.2021.107141
![]() |
[26] |
M. Zhao, G. Wei, J. Wu, Y. Guo, TODIM method for multiple attribute group decision making based on cumulative prospect theory with 2-tuple linguistic neutrosophic sets, Int. J. Intell. Syst., 36 (2020), 1199–1222. https://doi.org/10.1002/int.22338 doi: 10.1002/int.22338
![]() |
[27] |
T. He, G. Wei, J. Wu, C. Wei, QUALIFLEX method for evaluating human factors in construction project management with Pythagorean 2-tuple linguistic information, J. Intell. Fuzzy Syst., 40 (2021), 1–12. https://doi.org/10.3233/JIFS-200379 doi: 10.3233/JIFS-200379
![]() |
[28] | G. Beliakov, A. Pradera, T. Calvo, Aggregation Functions: Aguide for Practitioners, Springer, 2007. |
[29] |
S. Ayub, S. Abdullah, F. Ghani, M. Qiyas, M. Yaqub Khan, Cubic fuzzy Heronian mean dombi aggregation operators and their application on multi-attribute decision-making problems, Soft Comput., 25 (2021), 4175–4189. https://doi.org/10.1007/s00500-020-05512-4 doi: 10.1007/s00500-020-05512-4
![]() |
[30] |
M. Lin, X. Li, R. Chen, H. Fujita, J. Lin, Picture fuzzy interactional partitioned heronian mean aggregation operators: an application to MADM process, Artif. Intell. Rev., 2021 (2021), 1–38. https://doi.org/10.1007/s10462-021-09953-7 doi: 10.1007/s10462-021-09953-7
![]() |
[31] |
M. Deveci, D. Pamucar, I. Gokasar, Fuzzy power heronian function-based CoCoSo method for the advantage prioritization of autonomous vehicles in real-time traffic management, Sustainable Cities Soc., 69 (2021), 102846. https://doi.org/10.1016/j.scs.2021.102846 doi: 10.1016/j.scs.2021.102846
![]() |
[32] |
D. Pamucar, M. Behzad, D. Bozanic, M. Behzad, Decision making to support sustainable energy policies corresponding to agriculture sector: a case study in iran's caspian sea coastline, J. Cleaner Prod., 292 (2021), 125302. https://doi.org/10.1016/j.jclepro.2020.125302 doi: 10.1016/j.jclepro.2020.125302
![]() |
[33] | H. Garg, Z. Ali, J. Gwak, T. Mahmood, S. Aljahdali, Some complex intuitionistic uncertain linguistic heronian mean operators and their application in multiattribute group decision making, J. Math., 2021 (2021). https://doi.org/10.1155/2021/9986704 |
[34] |
P. Liu, Q. Khan, T. Mahmood, Group decision-making based on power Heronian aggregation operators under neutrosophic cubic environment, Soft Comput., 24 (2020), 1971–1997. https://doi.org/10.1007/s00500-019-04025-z doi: 10.1007/s00500-019-04025-z
![]() |
[35] |
F. Herrera, E. Herrera-Viedma, Linguistic decision analysis: steps for solving decision problems under linguistic information, Fuzzy Sets Syst., 115 (2000), 67–82. https://doi.org/10.1016/S0165-0114(99)00024-X doi: 10.1016/S0165-0114(99)00024-X
![]() |
[36] |
D. Yu, Intuitionistic fuzzy geometric Heronian mean aggregation operators, Appl. Soft Comput., 13 (2013), 1235–1246. https://doi.org/10.1016/j.asoc.2012.09.021 doi: 10.1016/j.asoc.2012.09.021
![]() |
[37] | T. Hara, M. Uchiyama, S. E. Takahasi, A refinement of various mean inequalities, J. Inequalities Appl., 4 (1998), 387–395. |
[38] |
S. Wu, J. Wang, G. Wei, Y. Wei, Research on construction engineering project risk assessment with some 2-tuple linguistic neutrosophic Hamy mean operators, Sustainability, 10 (2018), 1536. https://doi.org/10.3390/su10051536 doi: 10.3390/su10051536
![]() |
[39] | C. Maclaurin, A second letter to Martin Folkes, Esq.; concerning the roots of equations, with demonstration of other rules of algebra, Philos. Trans. R. Soc. Lond. Ser. A, 36 (1729), 59–96. |
[40] |
J. Qin, X. Liu, Approaches to uncertain linguistic multiple attribute decision making based on dual Maclaurin symmetric mean, J. Intell. Fuzzy Syst., 29 (2015), 171–186. https://doi.org/10.3233/IFS-151584 doi: 10.3233/IFS-151584
![]() |
1. | Muhammad Akram, Sumera Naz, Tahir Abbas, Complex q-rung orthopair fuzzy 2-tuple linguistic group decision-making framework with Muirhead mean operators, 2023, 0269-2821, 10.1007/s10462-023-10408-4 | |
2. | Tahir Mahmood, Ubaid ur Rehman, Zeeshan Ali, Analysis and application of Aczel-Alsina aggregation operators based on bipolar complex fuzzy information in multiple attribute decision making, 2023, 619, 00200255, 817, 10.1016/j.ins.2022.11.067 | |
3. | Sumera Naz, Muhammad Akram, Mamoona Muzammal, Group decision-making based on 2-tuple linguistic T-spherical fuzzy COPRAS method, 2023, 27, 1432-7643, 2873, 10.1007/s00500-022-07644-1 | |
4. | Ghous Ali, Adeel Farooq, Mohammed M. Ali Al-Shamiri, Novel multiple criteria decision-making analysis under $ m $-polar fuzzy aggregation operators with application, 2022, 20, 1551-0018, 3566, 10.3934/mbe.2023166 | |
5. | Muhammad Akram, Ayesha Khan, Uzma Ahmad, José Carlos R. Alcantud, Mohammed M. Ali Al-Shamiri, A new group decision-making framework based on 2-tuple linguistic complex $ q $-rung picture fuzzy sets, 2022, 19, 1551-0018, 11281, 10.3934/mbe.2022526 | |
6. | Tahir Mahmood, Ubaid ur Rehman, Zeeshan Ali, Muhammad Aslam, Ronnason Chinram, Identification and Classification of Aggregation Operators Using Bipolar Complex Fuzzy Settings and Their Application in Decision Support Systems, 2022, 10, 2227-7390, 1726, 10.3390/math10101726 | |
7. | Muhammad Akram, Uzma Noreen, Mohammed M. Ali Al-Shamiri, Zaoli Yang, Decision Analysis Approach Based on 2-Tuple Linguistic m -Polar Fuzzy Hamacher Aggregation Operators, 2022, 2022, 1607-887X, 1, 10.1155/2022/6269115 | |
8. | Muhammad Akram, Sumera Naz, Feng Feng, Aqsa Shafiq, Assessment of Hydropower Plants in Pakistan: Muirhead Mean-Based 2-Tuple Linguistic T-spherical Fuzzy Model Combining SWARA with COPRAS, 2022, 2193-567X, 10.1007/s13369-022-07081-0 | |
9. | Muhammad Akram, Uzma Noreen, Mohammed M. Ali Al-Shamiri, Dragan Pamucar, Integrated decision-making methods based on 2-tuple linguistic $ m $-polar fuzzy information, 2022, 7, 2473-6988, 14557, 10.3934/math.2022802 | |
10. | Ubaid ur Rehman, Tahir Mahmood, A study and performance evaluation of computer network under the environment of bipolar complex fuzzy partition Heronian mean operators, 2023, 180, 09659978, 103443, 10.1016/j.advengsoft.2023.103443 | |
11. | Muhammad Akram, Sumera Naz, Gustavo Santos-García, Muhammad Ramzan Saeed, Extended CODAS method for MAGDM with $ 2 $-tuple linguistic $ T $-spherical fuzzy sets, 2023, 8, 2473-6988, 3428, 10.3934/math.2023176 | |
12. | Sumera Naz, Muhammad Akram, G. Muhiuddin, Aqsa Shafiq, Salman Ahmad, Modified EDAS Method for MAGDM Based on MSM Operators with 2-Tuple Linguistic T -Spherical Fuzzy Sets, 2022, 2022, 1563-5147, 1, 10.1155/2022/5075998 | |
13. | Ubaid Ur Rehman, Tahir Mahmood, Majed Albaity, Khizar Hayat, Zeeshan Ali, Identification and Prioritization of DevOps Success Factors Using Bipolar Complex Fuzzy Setting With Frank Aggregation Operators and Analytical Hierarchy Process, 2022, 10, 2169-3536, 74702, 10.1109/ACCESS.2022.3190611 | |
14. | Muhammad Akram, Ayesha Khan, Uzma Ahmad, Extended MULTIMOORA method based on 2-tuple linguistic Pythagorean fuzzy sets for multi-attribute group decision-making, 2023, 8, 2364-4966, 311, 10.1007/s41066-022-00330-5 | |
15. | Sumera Naz, Muhammad Akram, Afia Sattar, Mohammed M. Ali Al-Shamiri, 2-tuple linguistic $ q $-rung orthopair fuzzy CODAS approach and its application in arc welding robot selection, 2022, 7, 2473-6988, 17529, 10.3934/math.2022966 | |
16. | Hui Liu, Performance evaluation of family business strategic transition based on the 2-tuple linguistic neutrosophic number multiple attribute group decision making, 2023, 44, 10641246, 3271, 10.3233/JIFS-221514 | |
17. | Sumera Naz, Muhammad Akram, Mohammed M. Ali Al-Shamiri, Muhammad Ramzan Saeed, Yu Zhou, Evaluation of Network Security Service Provider Using 2-Tuple Linguistic Complex q -Rung Orthopair Fuzzy COPRAS Method, 2022, 2022, 1099-0526, 1, 10.1155/2022/4523287 | |
18. | Ayesha Khan, Muhammad Akram, Uzma Ahmad, Mohammed M. Ali Al-Shamiri, A new multi-objective optimization ratio analysis plus full multiplication form method for the selection of an appropriate mining method based on 2-tuple spherical fuzzy linguistic sets, 2022, 20, 1551-0018, 456, 10.3934/mbe.2023021 | |
19. | Muhammad Akram, Sumera Naz, S. A. Edalatpanah, Shamaila Samreen, A hybrid decision-making framework under 2-tuple linguistic complex q-rung orthopair fuzzy Hamy mean aggregation operators, 2023, 42, 2238-3603, 10.1007/s40314-023-02254-5 | |
20. | Sumera Naz, Aqsa Shafiq, Shariq Aziz Butt, Rabia Ijaz, A new approach to sentiment analysis algorithms: Extended SWARA-MABAC method with 2-tuple linguistic q-rung orthopair fuzzy information, 2023, 126, 09521976, 106943, 10.1016/j.engappai.2023.106943 | |
21. | Muhammad Akram, José Carlos Rodríguez Alcantud, 2023, Chapter 7, 978-981-99-0568-3, 177, 10.1007/978-981-99-0569-0_7 | |
22. | Lijun Ma, Zeeshan Ali, Shi Yin, Van Thanh Tien Nguyen, Implementation of Learning Management Systems (LMS) in higher education systems through bipolar complex hesitant fuzzy Aczel-Alsina power aggregation operators: A case review for China, 2024, 19, 1932-6203, e0300317, 10.1371/journal.pone.0300317 | |
23. | Mengran Sun, Yushui Geng, Jing Zhao, Multi-Attribute Group Decision-Making Methods Based on Entropy Weights with q-Rung Picture Uncertain Linguistic Fuzzy Information, 2023, 15, 2073-8994, 2027, 10.3390/sym15112027 | |
24. | Junwei Li, Mengmeng Lian, Yong Jin, Miaomiao Xia, Huaibin Hou, Research on probabilistic language multi-attribute group decision-making method based on correlation coefficient and improved entropy, 2024, 46, 10641246, 9465, 10.3233/JIFS-235593 | |
25. | Tahir Mahmood, Hafiz Muhammad Waqas, Ubaid ur Rehman, Selection of optimal encryption algorithm based on hesitant bipolar complex fuzzy frank aggregation operators, 2024, 1598-5865, 10.1007/s12190-024-02239-5 | |
26. | Sumera Naz, Muhammad Akram, Aqsa Shafiq, Kiran Akhtar, Optimal airport selection utilizing power Muirhead mean based group decision model with 2-tuple linguistic q-rung orthopair fuzzy information, 2024, 15, 1868-8071, 303, 10.1007/s13042-023-01911-9 | |
27. | Zia Ur Rahman, Ghous Ali, Muhammad Asif, Yufeng Chen, Muhammad Zain Ul Abidin, Identification of desalination and wind power plants sites using m-polar fuzzy Aczel–Alsina aggregation information, 2024, 14, 2045-2322, 10.1038/s41598-023-50397-6 | |
28. | Sumera Naz, Aqsa Tasawar, Shariq Aziz Butt, Jorge Diaz-Martinez, Emiro De-La-Hoz-Franco, An integrated CRITIC-MABAC model under 2-tuple linguistic cubic q-rung orthopair fuzzy information with advanced aggregation operators, designed for multiple attribute group decision-making, 2024, 80, 0920-8542, 27244, 10.1007/s11227-024-06419-9 | |
29. | Sumera Naz, Areej Fatima, Shariq Aziz But, Arooj Ashiq, 2024, Chapter 17, 978-981-97-6971-1, 353, 10.1007/978-981-97-6972-8_17 | |
30. | Sumera Naz, Muhammad Muneeb ul Hassan, Areej Fatima, Diaz Jorge Martinez, Elisa Ospino Mendoza, Shariq Aziz Butt, A decision-making mechanism for multi-attribute group decision-making using 2-tuple linguistic T-spherical fuzzy maximizing deviation method, 2023, 8, 2364-4966, 1659, 10.1007/s41066-023-00388-9 | |
31. | Ubaid ur Rehman, Tahir Mahmood, Prioritization of types of wireless sensor networks by applying decision-making technique based on bipolar complex fuzzy linguistic heronian mean operators, 2024, 46, 10641246, 967, 10.3233/JIFS-232167 | |
32. | Muhammad Aslam, Hafiz Muhammad Waqas, Ubaid Ur Rehman, Tahir Mahmood, Selection of Cloud Services Provider by Utilizing Multi-Attribute Decision-Making Based on Hesitant Bipolar Complex Fuzzy Dombi Aggregation Operators, 2024, 12, 2169-3536, 35417, 10.1109/ACCESS.2024.3369893 | |
33. | Muhammad Akram, Arooj Adeel, 2023, Chapter 9, 978-3-031-43635-2, 437, 10.1007/978-3-031-43636-9_9 | |
34. | Fan Lei, Qiang Cai, Hongjun Wang, Guiwu Wei, Zhiwen Mo, An integrated group decision-making framework for evaluating the urban fire emergency management capability based on PDHLWPHM and PDHLWPGHM operator, 2024, 46, 10641246, 3713, 10.3233/JIFS-230485 | |
35. | Sumera Naz, Aqsa Tasawar, Areej Fatima, Shariq Aziz Butt, Zhoe Comas Gonzalez, An efficient 2-tuple linguistic cubic q-rung orthopair fuzzy CILOS-TOPSIS method: evaluating the hydrological geographical regions for watershed management in Pakistan, 2025, 81, 0920-8542, 10.1007/s11227-024-06505-y | |
36. | Tahir Mahmood, Ubaid Ur Rehman, Walid Emam, Yusra Tashkandy, Zeeshan Ali, Shi Yin, A Knowledge-Based System for the Classification of Cancer Diseases in Human Body Using Hybrid Approaches Based on Bipolar Complex Fuzzy Information, 2023, 11, 2169-3536, 96971, 10.1109/ACCESS.2023.3310568 | |
37. | Sumera Naz, Areej Fatima, Shariq Aziz But, Dragan Pamucar, Ronald Zamora-Musa, Melisa Acosta-Coll, Effective multi-attribute group decision-making approach to study astronomy in the probabilistic linguistic q-rung orthopair fuzzy VIKOR framework, 2024, 10, 24058440, e33004, 10.1016/j.heliyon.2024.e33004 | |
38. | Ghous Ali, Kholood Alsager, Novel Heronian mean based $ m $-polar fuzzy power geometric aggregation operators and their application to urban transportation management, 2024, 9, 2473-6988, 34109, 10.3934/math.20241626 | |
39. | Bin Xie, Hongmiao Yuan, Enhancing the combined compromise solution method for group decision-making under intuitionistic fuzziness: an application to blended English teaching quality evaluation in vocational colleges, 2025, 1432-7643, 10.1007/s00500-025-10417-1 | |
40. | Jing Guo, Xianjun Zhu, Wenfeng Li, Hui Li, A noval approach for dual probabilistic linguistic multi-criteria decision making based on prioritized Maclaurin symmetric mean operators and VIKOR, 2025, 48, 1064-1246, 447, 10.3233/JIFS-224232 | |
41. | Jie Li, Hind Y. Saleh, Areen A. Salih, M. Waheed Rasheed, Muhammad Bilal, Nimra Shabbir, Fuzzy-based group decision-making approach utilizing a 2-tuple linguistic q-rung orthopair set for the selection of optimal watershed system model, 2025, 12, 2296-665X, 10.3389/fenvs.2024.1502216 |
$ Q_{1} $ | $ Q_{2} $ | $ Q_{3} $ | $ Q_{4} $ | |
$ \digamma_{1} $ | $ ((\flat_{3}, 0), (\flat_{5}, 0)) $ | $ ((\flat_{1}, 0), (\flat_{3}, 0)) $ | $ ((\flat_{2}, 0), (\flat_{4}, 0)) $ | $ ((\flat_{3}, 0), (\flat_{2}, 0)) $ |
$ \digamma_{2} $ | $ ((\flat_{4}, 0), (\flat_{3}, 0)) $ | $ ((\flat_{5}, 0), (\flat_{2}, 0)) $ | $ ((\flat_{2}, 0), (\flat_{3}, 0)) $ | $ ((\flat_{4}, 0), (\flat_{2}, 0)) $ |
$ \digamma_{3} $ | $ ((\flat_{1}, 0), (\flat_{4}, 0)) $ | $ ((\flat_{3}, 0), (\flat_{3}, 0)) $ | $ ((\flat_{1}, 0), (\flat_{3}, 0)) $ | $ ((\flat_{4}, 0), (\flat_{3}, 0)) $ |
$ \digamma_{4} $ | $ ((\flat_{2}, 0), (\flat_{6}, 0)) $ | $ ((\flat_{3}, 0), (\flat_{4}, 0)) $ | $ ((\flat_{5}, 0), (\flat_{3}, 0)) $ | $ ((\flat_{1}, 0), (\flat_{5}, 0)) $ |
$ \digamma_{5} $ | $ ((\flat_{4}, 0), (\flat_{1}, 0)) $ | $ ((\flat_{1}, 0), (\flat_{7}, 0)) $ | $ ((\flat_{6}, 0), (\flat_{1}, 0)) $ | $ ((\flat_{2}, 0), (\flat_{2}, 0)) $ |
$ Q_{1} $ | $ Q_{2} $ | $ Q_{3} $ | $ Q_{4} $ | |
$ \digamma_{1} $ | $ ((\flat_{2}, 0), (\flat_{5}, 0)) $ | $ ((\flat_{4}, 0), (\flat_{2}, 0)) $ | $ ((\flat_{3}, 0), (\flat_{5}, 0)) $ | $ ((\flat_{4}, 0), (\flat_{4}, 0)) $ |
$ \digamma_{2} $ | $ ((\flat_{4}, 0), (\flat_{1}, 0)) $ | $ ((\flat_{5}, 0), (\flat_{2}, 0)) $ | $ ((\flat_{6}, 0), (\flat_{1}, 0)) $ | $ ((\flat_{2}, 0), (\flat_{6}, 0)) $ |
$ \digamma_{3} $ | $ ((\flat_{3}, 0), (\flat_{2}, 0)) $ | $ ((\flat_{3}, 0), (\flat_{5}, 0)) $ | $ ((\flat_{2}, 0), (\flat_{3}, 0)) $ | $ ((\flat_{3}, 0), (\flat_{4}, 0)) $ |
$ \digamma_{4} $ | $ ((\flat_{2}, 0), (\flat_{6}, 0)) $ | $ ((\flat_{1}, 0), (\flat_{4}, 0)) $ | $ ((\flat_{3}, 0), (\flat_{5}, 0)) $ | $ ((\flat_{5}, 0), (\flat_{3}, 0)) $ |
$ \digamma_{5} $ | $ ((\flat_{5}, 0), (\flat_{1}, 0)) $ | $ ((\flat_{2}, 0), (\flat_{2}, 0)) $ | $ ((\flat_{3}, 0), (\flat_{1}, 0)) $ | $ ((\flat_{1}, 0), (\flat_{7}, 0)) $ |
$ Q_{1} $ | $ Q_{2} $ | $ Q_{3} $ | $ Q_{4} $ | |
$ \digamma_{1} $ | $ ((\flat_{1}, 0), (\flat_{6}, 0)) $ | $ ((\flat_{3}, 0), (\flat_{4}, 0)) $ | $ ((\flat_{2}, 0), (\flat_{5}, 0)) $ | $ ((\flat_{1}, 0), (\flat_{5}, 0)) $ |
$ \digamma_{2} $ | $ ((\flat_{4}, 0), (\flat_{4}, 0)) $ | $ ((\flat_{2}, 0), (\flat_{6}, 0)) $ | $ ((\flat_{1}, 0), (\flat_{7}, 0)) $ | $ ((\flat_{4}, 0), (\flat_{2}, 0)) $ |
$ \digamma_{3} $ | $ ((\flat_{2}, 0), (\flat_{3}, 0)) $ | $ ((\flat_{4}, 0), (\flat_{3}, 0)) $ | $ ((\flat_{3}, 0), (\flat_{3}, 0)) $ | $ ((\flat_{4}, 0), (\flat_{1}, 0)) $ |
$ \digamma_{4} $ | $ ((\flat_{3}, 0), (\flat_{5}, 0)) $ | $ ((\flat_{2}, 0), (\flat_{4}, 0)) $ | $ ((\flat_{6}, 0), (\flat_{1}, 0)) $ | $ ((\flat_{4}, 0), (\flat_{3}, 0)) $ |
$ \digamma_{5} $ | $ ((\flat_{7}, 0), (\flat_{1}, 0)) $ | $ ((\flat_{5}, 0), (\flat_{1}, 0)) $ | $ ((\flat_{4}, 0), (\flat_{1}, 0)) $ | $ ((\flat_{5}, 0), (\flat_{3}, 0)) $ |
$ Q_{1} $ | $ Q_{2} $ | $ Q_{3} $ | $ Q_{4} $ | |
G2TLBFWA operator | ||||
$ \digamma_1 $ | $ ((\flat_2, 0.1415), (\flat_5, 0.1857)) $ | $ ((\flat_3, 0.0529), (\flat_3, -0.4054)) $ | $ ((\flat_3, -0.4772), (\flat_5, -0.3238)) $ | $ ((\flat_3, 0.2166), (\flat_3, 0.3973)) $ |
$ \digamma_2 $ | $ ((\flat_4, 0.0000), (\flat_2, -0.1654)) $ | $ ((\flat_5, -0.4461), (\flat_2, 0.4915)) $ | $ ((\flat_4, 0.4274), (\flat_2, 0.0519)) $ | $ ((\flat_3, 0.1010), (\flat_3, 0.4641)) $ |
$ \digamma_3 $ | $ ((\flat_2, 0.2635), (\flat_3, -0.3297)) $ | $ ((\flat_3, 0.2182), (\flat_4, -0.1270)) $ | $ ((\flat_2, -0.0590), (\flat_3, 0.0000)) $ | $ ((\flat_4, -0.4721), (\flat_3, -0.2192)) $ |
$ \digamma_4 $ | $ ((\flat_2, 0.2148), (\flat_6, -0.2148)) $ | $ ((\flat_2, -0.1358), (\flat_4, 0.0000)) $ | $ ((\flat_4, 0.4287), (\flat_3, 0.1090)) $ | $ ((\flat_4, -0.0973), (\flat_3, 0.4968)) $ |
$ \digamma_5 $ | $ ((\flat_5, 0.3747), (\flat_1, 0.0000)) $ | $ ((\flat_3, -0.4705), (\flat_3, -0.4646)) $ | $ ((\flat_4, 0.3675), (\flat_1, 0.0000)) $ | $ ((\flat_2, 0.3582), (\flat_4, 0.0577)) $ |
2TLBFWG operator | ||||
$\digamma_1$ | $((\flat_2, -0.0337), (\flat_5, 0.2337))$ | $((\flat_2, 0.4915), (\flat_3, -0.2382))$ | $((\flat_2, 0.4495), (\flat_5, -0.2704))$ | $((\flat_3, -0.2192), (\flat_4, -0.2648))$ |
$\digamma_2$ | $((\flat_4, 0.0000), (\flat_2, 0.3421))$ | $((\flat_4, 0.1628), (\flat_3, 0.1836))$ | $((\flat_3, 0.0157), (\flat_4, -0.2879))$ | $((\flat_3, -0.1716), (\flat_5, -0.4641))$ |
$\digamma_3$ | $((\flat_2, -0.0104), (\flat_3, -0.1226))$ | $((\flat_3, 0.1777), (\flat_4, 0.1270))$ | $((\flat_2, -0.2383), (\flat_3, 0.0000))$ | $((\flat_3, 0.4641), (\flat_3, 0.2166))$ |
$\digamma_4$ | $((\flat_2, 0.1689), (\flat_6, -0.1689))$ | $((\flat_2, -0.4029), (\flat_4, 0.0000))$ | $((\flat_4, 0.0168), (\flat_4, -0.1426))$ | $((\flat_3, -0.0495), (\flat_4, -0.2896))$ |
$\digamma_5$ | $((\flat_5, 0.0018), (\flat_1, 0.0000))$ | $((\flat_2, -0.0488), (\flat_4, 0.3851))$ | $((\flat_4, -0.0878), (\flat_1, 0.0000))$ | $((\flat_2, -0.3014), (\flat_6, -0.3618))$ |
Alternatives | Overall assessment values by G2TLBFWHM | Overall assessment values by 2TLBFWGHM |
$ \digamma_{1} $ | $ ((\flat_3, -0.4392), (\flat_4, -0.0151)) $ | $ ((\flat_3, -0.1204), (\flat_4, -0.1222)) $ |
$ \digamma_{2} $ | $ ((\flat_4, -0.1760), (\flat_3, -0.1781)) $ | $ ((\flat_4, -0.1615), (\flat_3, 0.2750)) $ |
$ \digamma_{3} $ | $ ((\flat_3, -0.3760), (\flat_4, -0.4739)) $ | $ ((\flat_3, -0.0786), (\flat_3, 0.1544)) $ |
$ \digamma_{4} $ | $ ((\flat_3, 0.1383), (\flat_4, 0.1756)) $ | $ ((\flat_3, -0.0911), (\flat_4, 0.0569)) $ |
$ \digamma_{5} $ | $ ((\flat_4, -0.3497), (\flat_2, 0.2376)) $ | $ ((\flat_3, 0.1549), (\flat_4, -0.3829)) $ |
Weights | Scores | Ranking |
$ \varkappa^{\star}_{1}=0.1, \varkappa^{\star}_{2}=0.2, $ | $ \mathcal{S}^{HM}_{1}=(\flat_3, 0.3689), \mathcal{S}^{HM}_{2}=(\flat_4, 0.2697), \mathcal{S}^{HM}_{3}=(\flat_4, -0.2814), $ | $ {\digamma_{5} > \digamma_{2} > \digamma_{4} > \digamma_{3} > \digamma_{1}} $ |
$ \varkappa^{\star}_{3}=0.3, \varkappa^{\star}_{4}=0.4 $ | $ \mathcal{S}^{HM}_{4}=(\flat_4, -0.2213), \mathcal{S}^{HM}_{5}=(\flat_4, 0.3436) $ | |
$ \varkappa^{\star}_{1}=0.2, \varkappa^{\star}_{2}=0.1, $ | $ \mathcal{S}^{HM}_{1}=(\flat_3, 0.2348), \mathcal{S}^{HM}_{2}=(\flat_4, 0.2770), \mathcal{S}^{HM}_{3}=(\flat_4, -0.2690), $ | $ {\digamma_{5} > \digamma_{2} > \digamma_{4} > \digamma_{3} > \digamma_{1}} $ |
$ \varkappa^{\star}_{3}=0.3, \varkappa^{\star}_{4}=0.4 $ | $ \mathcal{S}^{HM}_{4}=(\flat_4, -0.2562), \mathcal{S}^{HM}_{5}=(\flat_5, -0.4185) $ | |
$ \varkappa^{\star}_{1}=0.3, \varkappa^{\star}_{2}=0.1, $ | $ \mathcal{S}^{HM}_{1}=(\flat_3, 0.2133), \mathcal{S}^{HM}_{2}=(\flat_4, 0.2652) \mathcal{S}^{HM}_{3}=(\flat_4, -0.2444), $ | $ {\digamma_{5} > \digamma_{2} > \digamma_{3} > \digamma_{4} > \digamma_{1}} $ |
$ \varkappa^{\star}_{3}=0.2, \varkappa^{\star}_{4}=0.4, $ | $ \mathcal{S}^{HM}_{4}=(\flat_4, -0.4446), \mathcal{S}^{HM}_{5}=(\flat_5, -0.3323) $ | |
$ \varkappa^{\star}_{1}=0.4, \varkappa^{\star}_{2}=0.1, $ | $ \mathcal{S}^{HM}_{1}=(\flat_3, 0.0671), \mathcal{S}^{HM}_{2}=(\flat_4, 0.4098), \mathcal{S}^{HM}_{3}=(\flat_4, -0.3131), $ | $ {\digamma_{5} > \digamma_{2} > \digamma_{3} > \digamma_{4} > \digamma_{1}} $ |
$ \varkappa^{\star}_{3}=0.2, \varkappa^{\star}_{4}=0.3, $ | $ \mathcal{S}^{HM}_{4}=(\flat_3, 0.3958), \mathcal{S}^{HM}_{5}=(\flat_5, -0.0206) $ |
Weights | Scores | Ranking |
$ \varkappa^{\star}_{1}=0.1, \varkappa^{\star}_{2}=0.2, $ | $ \mathcal{S}^{GHM}_{1}=(\flat_4, -0.3918), \mathcal{S}^{GHM}_{2}=(\flat_4, -0.0843), \mathcal{S}^{GHM}_{3}=(\flat_4, 0.0048), $ | $ {\digamma_{3} > \digamma_{2} > \digamma_{4} > \digamma_{1} > \digamma_{5}} $ |
$ \varkappa^{\star}_{3}=0.3, \varkappa^{\star}_{4}=0.4 $ | $ \mathcal{S}^{GHM}_{4}=(\flat_4, -0.2370), \mathcal{S}^{GHM}_{5}=(\flat_3, 0.2781) $ | |
$ \varkappa^{\star}_{1}=0.2, \varkappa^{\star}_{2}=0.1, $ | $ \mathcal{S}^{GHM}_{1}=(\flat_3, 0.4825), \mathcal{S}^{GHM}_{2}=(\flat_4, -0.0710), \mathcal{S}^{GHM}_{3}=(\flat_4, 0.0133), $ | $ {\digamma_{3} > \digamma_{2} > \digamma_{4} > \digamma_{1} > \digamma_{5}} $ |
$ \varkappa^{\star}_{3}=0.3, \varkappa^{\star}_{4}=0.4 $ | $ \mathcal{S}^{GHM}_{4}=(\flat_4, -0.3175), \mathcal{S}^{GHM}_{5}=(\flat_3, 0.4242) $ | |
$ \varkappa^{\star}_{1}=0.3, \varkappa^{\star}_{2}=0.1, $ | $ \mathcal{S}^{GHM}_{1}=(\flat_3, 0.4177), \mathcal{S}^{GHM}_{2}=(\flat_4, 0.0094), \mathcal{S}^{GHM}_{3}=(\flat_4, 0.0358), $ | $ {\digamma_{3} > \digamma_{2} > \digamma_{4} > \digamma_{5} > \digamma_{1}} $ |
$ \varkappa^{\star}_{3}=0.2, \varkappa^{\star}_{4}=0.4, $ | $ \mathcal{S}^{GHM}_{4}=(\flat_3, 0.4578), \mathcal{S}^{GHM}_{5}=(\flat_3, 0.4436) $ | |
$ \varkappa^{\star}_{1}=0.4, \varkappa^{\star}_{2}=0.1, $ | $ \mathcal{S}^{GHM}_{1}=(\flat_3, 0.2737), \mathcal{S}^{GHM}_{2}=(\flat_4, 0.1855), \mathcal{S}^{GHM}_{3}=(\flat_4, -0.0331), $ | $ {\digamma_{2} > \digamma_{3} > \digamma_{5} > \digamma_{1} > \digamma_{4}} $ |
$ \varkappa^{\star}_{3}=0.2, \varkappa^{\star}_{4}=0.3, $ | $ \mathcal{S}^{GHM}_{4}=(\flat_3, 0.2684), \mathcal{S}^{GHM}_{5}=(\flat_4, -0.1974) $ |
Parameter values | Score functions |
$ s = t = 0.5 $ | $ \mathcal{S}^{HM}_{1} = (\flat_{2}, \; 0.3985), \; \; \mathcal{S}^{HM}_{2} = (\flat_{4}, -0.3920), \; \mathcal{S}^{HM}_{3} = (\flat_{3}, -0.3138), \; \mathcal{S}^{HM}_{4} = (\flat_{2}, \; 0.4184), \; \; \; \mathcal{S}^{HM}_{5} = (\flat_{4}, -0.3323) $ |
$ s = t = 1 $ | $ \mathcal{S}^{HM}_{1} = (\flat_{3}, -0.1830), \; \mathcal{S}^{HM}_{2} = (\flat_{4}, \; 0.0610), \; \; \mathcal{S}^{HM}_{3} = (\flat_{3}, \; 0.1018), \; \; \mathcal{S}^{HM}_{4} = (\flat_{3}, -0.1253), \; \; \mathcal{S}^{HM}_{5} = (\flat_{4}, \; 0.1457) $ |
$ s = t = 2 $ | $ \mathcal{S}^{HM}_{1} = (\flat_{3}, \; 0.1731), \; \; \mathcal{S}^{HM}_{2} = (\flat_{4}, \; 0.4197), \; \; \mathcal{S}^{HM}_{3} = (\flat_{3}, \; 0.4407), \; \; \; \mathcal{S}^{HM}_{4} = (\flat_{3}, \; 0.3053), \; \; \; \mathcal{S}^{HM}_{5} = (\flat_{5}, -0.4105) $ |
$ s = 3, t = 4 $ | $ \mathcal{S}^{HM}_{1} = (\flat_{3}, \; 0.4369), \; \; \mathcal{S}^{HM}_{2} = (\flat_{5}, -0.3629), \; \mathcal{S}^{HM}_{3} = (\flat_{4}, -0.3229), \; \mathcal{S}^{HM}_{4} = (\flat_{4}, -0.3379), \; \mathcal{S}^{HM}_{5} = (\flat_{5}, -0.0659) $ |
$ s = 4, t = 3 $ | $ \mathcal{S}^{HM}_{1} = (\flat_{3}, \; 0.4359), \; \; \mathcal{S}^{HM}_{2} = (\flat_{5}, -0.3434), \; \mathcal{S}^{HM}_{3} = (\flat_{4}, -0.3369), \; \mathcal{S}^{HM}_{4} = (\flat_{4}, -0.3654), \; \mathcal{S}^{HM}_{5} = (\flat_{5}, -0.0288) $ |
$ s = 5, t = 6 $ | $ \mathcal{S}^{HM}_{1} = (\flat_{4}, -0.3663), \; \mathcal{S}^{HM}_{2} = (\flat_{5}, -0.2087), \; \mathcal{S}^{HM}_{3} = (\flat_{4}, -0.1665), \; \mathcal{S}^{HM}_{4} = (\flat_{4}, -0.1007), \; \mathcal{S}^{HM}_{5} = (\flat_{5}, \; 0.2306) $ |
$ s = 6, t = 5 $ | $ \mathcal{S}^{HM}_{1} = (\flat_{4}, -0.3639), \; \mathcal{S}^{HM}_{2} = (\flat_{5}, -0.2005), \; \mathcal{S}^{HM}_{3} = (\flat_{4}, -0.1730), \; \mathcal{S}^{HM}_{4} = (\flat_{4}, -0.1050), \; \mathcal{S}^{HM}_{5} = (\flat_{5}, \; 0.2448) $ |
$ s = 7, t = 8 $ | $ \mathcal{S}^{HM}_{1} = (\flat_{4}, -0.2402), \; \mathcal{S}^{HM}_{2} = (\flat_{5}, -0.1187), \; \mathcal{S}^{HM}_{3} = (\flat_{4}, -0.0700), \; \mathcal{S}^{HM}_{4} = (\flat_{4}, \; 0.0445), \; \; \mathcal{S}^{HM}_{5} = (\flat_{5}, \; 0.4174) $ |
$ s = 8, t = 7 $ | $ \mathcal{S}^{HM}_{1} = (\flat_{4}, -0.2385), \; \mathcal{S}^{HM}_{2} = (\flat_{5}, -0.1144), \; \mathcal{S}^{HM}_{3} = (\flat_{4}, -0.0732), \; \mathcal{S}^{HM}_{4} = (\flat_{4}, \; 0.0446), \; \; \mathcal{S}^{HM}_{5} = (\flat_{5}, \; 0.4241) $ |
$ s = 1, t = 9 $ | $ \mathcal{S}^{HM}_{1} = (\flat_{4}, -0.3257), \; \mathcal{S}^{HM}_{2} = (\flat_{5}, -0.2461), \; \mathcal{S}^{HM}_{3} = (\flat_{4}, -0.1150), \; \mathcal{S}^{HM}_{4} = (\flat_{4}, \; 0.0041), \; \; \mathcal{S}^{HM}_{5} = (\flat_{5}, \; 0.1821) $ |
$ s = 9, t = 1 $ | $ \mathcal{S}^{HM}_{1} = (\flat_{4}, -0.3293), \; \mathcal{S}^{HM}_{2} = (\flat_{5}, -0.1503), \; \mathcal{S}^{HM}_{3} = (\flat_{4}, -0.1849), \; \mathcal{S}^{HM}_{4} = (\flat_{4}, -0.1453), \; \mathcal{S}^{HM}_{5} = (\flat_{5}, 0.3953) $ |
$ s = t = 10 $ | $ \mathcal{S}^{HM}_{1} = (\flat_{4}, -0.1355), \; \mathcal{S}^{HM}_{2} = (\flat_{5}, -0.0439), \; \mathcal{S}^{HM}_{3} = (\flat_{4}, 0.0097), \; \; \; \mathcal{S}^{HM}_{4} = (\flat_{4}, 0.1619), \; \; \; \mathcal{S}^{HM}_{5} = (\flat_{6}, -0.4265) $ |
Parameter values | Score functions |
$ s = t = 0.5 $ | $ \mathcal{S}^{GHM}_{1} = (\flat_{4}, 0.4425), \; \; \mathcal{S}^{GHM}_{2} = (\flat_{5}, 0.2342), \; \; \mathcal{S}^{GHM}_{3} = (\flat_{5}, -0.2274), \mathcal{S}^{GHM}_{4} = (\flat_{4}, 0.3902), \; \; \; \mathcal{S}^{GHM}_{5} = (\flat_{5}, 0.1811) $ |
$ s = t = 1 $ | $ \mathcal{S}^{GHM}_{1} = (\flat_{4}, -0.0219), \mathcal{S}^{GHM}_{2} = (\flat_{5}, -0.2048), \mathcal{S}^{GHM}_{3} = (\flat_{4}, 0.3335), \; \; \mathcal{S}^{GHM}_{4} = (\flat_{4}, -0.0868), \mathcal{S}^{GHM}_{5} = (\flat_{5}, -0.3859) $ |
$ s = t = 2 $ | $ \mathcal{S}^{GHM}_{1} = (\flat_{4}, -0.4094), \mathcal{S}^{GHM}_{2} = (\flat_{4}, 0.4340), \; \; \mathcal{S}^{GHM}_{3} = (\flat_{4}, -0.0223), \mathcal{S}^{GHM}_{4} = (\flat_{4}, -0.4951), \mathcal{S}^{GHM}_{5} = (\flat_{4}, 0.0061) $ |
$ s = 3, t = 4 $ | $ \mathcal{S}^{GHM}_{1} = (\flat_{3}, 0.3385), \; \; \mathcal{S}^{GHM}_{2} = (\flat_{4}, 0.1488), \; \; \mathcal{S}^{GHM}_{3} = (\flat_{4}, -0.2615), \mathcal{S}^{GHM}_{4} = (\flat_{3}, 0.2222), \; \; \mathcal{S}^{GHM}_{5} = (\flat_{3}, 0.4955) $ |
$ s = 4, t = 3 $ | $ \mathcal{S}^{GHM}_{1} = (\flat_{3}, 0.3027), \; \; \mathcal{S}^{GHM}_{2} = (\flat_{4}, 0.2111), \; \; \mathcal{S}^{GHM}_{3} = (\flat_{4}, -0.2736), \mathcal{S}^{GHM}_{4} = (\flat_{3}, 0.1584), \; \; \mathcal{S}^{GHM}_{5} = (\flat_{4}, -0.4688) $ |
$ s = 5, t = 6 $ | $ \mathcal{S}^{GHM}_{1} = (\flat_{3}, 0.1412), \; \; \mathcal{S}^{GHM}_{2} = (\flat_{4}, -0.0319), \mathcal{S}^{GHM}_{3} = (\flat_{4}, -0.4497), \mathcal{S}^{GHM}_{4} = (\flat_{3}, -0.0616), \mathcal{S}^{GHM}_{5} = (\flat_{3}, 0.1477) $ |
$ s = 6, t = 5 $ | $ \mathcal{S}^{GHM}_{1} = (\flat_{3}, 0.1198), \; \; \mathcal{S}^{GHM}_{2} = (\flat_{4}, 0.0002), \; \; \mathcal{S}^{GHM}_{3} = (\flat_{4}, -0.4552), \mathcal{S}^{GHM}_{4} = (\flat_{3}, -0.0884), \mathcal{S}^{GHM}_{5} = (\flat_{3}, 0.1650) $ |
$ s = 7, t = 8 $ | $ \mathcal{S}^{GHM}_{1} = (\flat_{3}, 0.0215), \; \; \mathcal{S}^{GHM}_{2} = (\flat_{4}, -0.1589), \mathcal{S}^{GHM}_{3} = (\flat_{3}, 0.4237), \; \; \mathcal{S}^{GHM}_{4} = (\flat_{3}, -0.2577), \mathcal{S}^{GHM}_{5} = (\flat_{3}, -0.0660) $ |
$ s = 8, t = 7 $ | $ \mathcal{S}^{GHM}_{1} = (\flat_{3}, 0.0074), \; \; \mathcal{S}^{GHM}_{2} = (\flat_{4}, -0.1413), \mathcal{S}^{GHM}_{3} = (\flat_{3}, 0.4217), \; \; \mathcal{S}^{GHM}_{4} = (\flat_{3}, -0.2677), \mathcal{S}^{GHM}_{5} = (\flat_{3}, -0.0561) $ |
$ s = 1, t = 9 $ | $ \mathcal{S}^{GHM}_{1} = (\flat_{3}, 0.2199), \; \; \mathcal{S}^{GHM}_{2} = (\flat_{4}, -0.1981), \mathcal{S}^{GHM}_{3} = (\flat_{4}, -0.4622), \mathcal{S}^{GHM}_{4} = (\flat_{3}, 0.0421), \; \; \mathcal{S}^{GHM}_{5} = (\flat_{3}, 0.0067) $ |
$ s = 9, t = 1 $ | $ \mathcal{S}^{GHM}_{1} = (\flat_{3}, 0.0159), \; \; \mathcal{S}^{GHM}_{2} = (\flat_{4}, 0.1304), \; \; \mathcal{S}^{GHM}_{3} = (\flat_{3}, 0.4816), \; \; \mathcal{S}^{GHM}_{4} = (\flat_{3}, -0.2845), \mathcal{S}^{GHM}_{5} = (\flat_{3}, 0.2408) $ |
$ s = t = 10 $ | $ \mathcal{S}^{GHM}_{1} = (\flat_{3}, -0.0821), \mathcal{S}^{GHM}_{2} = (\flat_{4}, -0.2708), \mathcal{S}^{GHM}_{3} = (\flat_{3}, 0.3125), \; \mathcal{S}^{GHM}_{4} = (\flat_{3}, -0.4277), \mathcal{S}^{GHM}_{5} = (\flat_{3}, -0.2376) $ |
Parameters | G2TLBFWHM operator | 2TLBFWGHM operator |
$ s = t = 0.5 $ | $ \digamma_{5} > \digamma_{2} > \digamma_{3} > \digamma_{4} > \digamma_{1} $ | $ \digamma_{2} > \digamma_{5} > \digamma_{3} > \digamma_{1} > \digamma_{4} $ |
$ s = t = 1 $ | $ \digamma_{5} > \digamma_{2} > \digamma_{3} > \digamma_{4} > \digamma_{1} $ | $ \digamma_{2} > \digamma_{5} > \digamma_{3} > \digamma_{1} > \digamma_{4} $ |
$ s = t = 2 $ | $ \digamma_{5} > \digamma_{2} > \digamma_{3} > \digamma_{4} > \digamma_{1} $ | $ \digamma_{2} > \digamma_{5} > \digamma_{3} > \digamma_{1} > \digamma_{4} $ |
$ s = 3, t = 4 $ | $ \digamma_{5} > \digamma_{2} > \digamma_{3} > \digamma_{4} > \digamma_{1} $ | $ \digamma_{2} > \digamma_{3} > \digamma_{5} > \digamma_{1} > \digamma_{4} $ |
$ s = 4, t = 3 $ | $ \digamma_{5} > \digamma_{2} > \digamma_{3} > \digamma_{4} > \digamma_{1} $ | $ \digamma_{2} > \digamma_{3} > \digamma_{5} > \digamma_{1} > \digamma_{4} $ |
$ s = 5, t = 6 $ | $ \digamma_{5} > \digamma_{2} > \digamma_{4} > \digamma_{3} > \digamma_{1} $ | $ \digamma_{2} > \digamma_{3} > \digamma_{5} > \digamma_{1} > \digamma_{4} $ |
$ s = 6, t = 5 $ | $ \digamma_{5} > \digamma_{2} > \digamma_{4} > \digamma_{3} > \digamma_{1} $ | $ \digamma_{2} > \digamma_{3} > \digamma_{5} > \digamma_{1} > \digamma_{4} $ |
$ s = 7, t = 8 $ | $ \digamma_{5} > \digamma_{2} > \digamma_{4} > \digamma_{3} > \digamma_{1} $ | $ \digamma_{2} > \digamma_{3} > \digamma_{1} > \digamma_{5} > \digamma_{4} $ |
$ s = 8, t = 7 $ | $ \digamma_{5} > \digamma_{2} > \digamma_{4} > \digamma_{3} > \digamma_{1} $ | $ \digamma_{2} > \digamma_{3} > \digamma_{1} > \digamma_{5} > \digamma_{4} $ |
$ s = 1, t = 9 $ | $ \digamma_{5} > \digamma_{2} > \digamma_{4} > \digamma_{3} > \digamma_{1} $ | $ \digamma_{2} > \digamma_{3} > \digamma_{1} > \digamma_{4} > \digamma_{5} $ |
$ s = 9, t = 1 $ | $ \digamma_{5} > \digamma_{2} > \digamma_{4} > \digamma_{3} > \digamma_{1} $ | $ \digamma_{2} > \digamma_{3} > \digamma_{5} > \digamma_{1} > \digamma_{4} $ |
$ s = t = 10 $ | $ \digamma_{5} > \digamma_{2} > \digamma_{4} > \digamma_{3} > \digamma_{1} $ | $ \digamma_{2} > \digamma_{3} > \digamma_{1} > \digamma_{5} > \digamma_{4} $ |
2TLBFWA | Scores values | The order | |
$ \digamma_{1} $ | $ ((\flat_3, -0.2530), (\flat_4, -0.2456)) $ | $ (\flat_3, 0.4963) $ | $ {\digamma_{5} > \digamma_{2} > \digamma_{3} > f \digamma_{4} > \digamma_{1}} $ |
$ \digamma_{2} $ | $ ((\flat_4, 0.1497), (\flat_2, 0.3458)) $ | $ (\flat_5, -0.0981) $ | |
$ \digamma_{3} $ | $ ((\flat_3, -0.2482), (\flat_3, 0.1161)) $ | $ (\flat_4, -0.1822) $ | |
$ \digamma_{4} $ | $ ((\flat_3, 0.1559), (\flat_4, -0.0348)) $ | $ (\flat_4, -0.4046) $ | |
$ \digamma_{5} $ | $ ((\flat_4, -0.1614), (\flat_2, -0.2589)) $ | $ (\flat_5, 0.0488) $ |
2TLBFWG | Scores values | The order | |
$ \digamma_{1} $ | $ ((\flat_2, 0.3982), (\flat_4, 0.1699)) $ | $ (\flat_3, 0.1142) $ | $ {\digamma_{2} > \digamma_{5} > \digamma_{3} > \digamma_{1} > \digamma_{2}} $ |
$ \digamma_{2} $ | $ ((\flat_4, -0.4867), (\flat_3, 0.4502)) $ | $ (\flat_4, 0.0316) $ | |
$ \digamma_{3} $ | $ ((\flat_2, 0.4733), (\flat_3, 0.3939)) $ | $ (\flat_4, -0.4603) $ | |
$ \digamma_{4} $ | $ ((\flat_2, 0.4698), (\flat_4, 0.4454)) $ | $ (\flat_3, 0.0122) $ | |
$ \digamma_{5} $ | $ ((\flat_3, -0.1526), (\flat_3, 0.3604)) $ | $ (\flat_4, -0.2565) $ |
2TLBFWHM | Scores values | The order | |
$ \digamma_{1} $ | $ ((\flat_6, 0.1111), (\flat_1 0.2620)) $ | $ (\flat_6, 0.4246) $ | $ {\digamma_{2} > \digamma_{5} > \digamma_{3} > \digamma_{4} > \digamma_{1}} $ |
$ \digamma_{2} $ | $ ((\flat_7, -0.2399), (\flat_1-0.3123)) $ | $ (\flat_7, 0.0362) $ | |
$ \digamma_{3} $ | $ ((\flat_6, 0.0880), (\flat_1-0.0719)) $ | $ (\flat_7, -0.4201) $ | |
$ \digamma_{4} $ | $ ((\flat_6, 0.2394), (\flat_1 0.3353)) $ | $ (\flat_6, 0.4520) $ | |
$ \digamma_{5} $ | $ ((\flat_7, -0.4646), (\flat_1-0.4275)) $ | $ (\flat_7, -0.0185) $ |
2TLBFWDHM | Scores values | The order | |
$ \digamma_{1} $ | $ ((\flat_1, -0.3159), (\flat_7, -0.2518)) $ | $ (\flat_1, -0.0321) $ | $ {\digamma_{5} > \digamma_{2} > \digamma_{3} > \digamma_{1} > \digamma_{4}} $ |
$ \digamma_{2} $ | $ ((\flat_1, 0.0870), (\flat_6, 0.4438)) $ | $ (\flat_1, 0.3216) $ | |
$ \digamma_{3} $ | $ ((\flat_1, -0.2613), (\flat_6, 0.4293)) $ | $ (\flat_1, 0.1547) $ | |
$ \digamma_{4} $ | $ ((\flat_1, -0.2369), (\flat_7, -0.1422)) $ | $ (\flat_1, -0.0473) $ | |
$ \digamma_{5} $ | $ ((\flat_1, -0.0449), (\flat_6, -0.0374)) $ | $ (\flat_1, 0.4962) $ |
2TLBFWMSM | Scores values | The order | |
$ \digamma_{1} $ | $ ((\flat_6, 0.1130), (\flat_1, 0.2595)) $ | $ (\flat_6, 0.4267) $ | $ {\digamma_{2} > \digamma_{5} > \digamma_{3} > \digamma_{4} > \digamma_{1}} $ |
$ \digamma_{2} $ | $ ((\flat_7, -0.2398), (\flat_1, -0.3126)) $ | $ (\flat_7, 0.0364) $ | |
$ \digamma_{3} $ | $ ((\flat_6, 0.0917), (\flat_1, -0.0734)) $ | $ (\flat_7, -0.4175) $ | |
$ \digamma_{4} $ | $ ((\flat_6, 0.2487), (\flat_1, 0.3320)) $ | $ (\flat_6, 0.4584) $ | |
$ \digamma_{5} $ | $ ((\flat_7, -0.4588), (\flat_1, -0.4291)) $ | $ (\flat_7, -0.0149) $ |
2TLBFWDMSM | Scores values | The order | |
$ \digamma_{1} $ | $ ((\flat_1, -0.3161), (\flat_7, -0.2470)) $ | $ (\flat_1, -0.0346) $ | $ {\digamma_{5} > \digamma_{2} > \digamma_{3} > \digamma_{1} > \digamma_{4}} $ |
$ \digamma_{2} $ | $ ((\flat_1, 0.0852), (\flat_6, 0.4470)) $ | $ (\flat_1, 0.3191) $ | |
$ \digamma_{3} $ | $ ((\flat_1, -0.2622), (\flat_6, 0.4300)) $ | $ (\flat_1, 0.1539) $ | |
$ \digamma_{4} $ | $ ((\flat_1, -0.2383), (\flat_7, -0.1403)) $ | $ (\flat_1, -0.0490) $ | |
$ \digamma_{5} $ | $ ((\flat_1, -0.0483), (\flat_6, -0.0121)) $ | $ (\flat_1, 0.4819) $ |
$ Q_{1} $ | $ Q_{2} $ | $ Q_{3} $ | $ Q_{4} $ | |
$ \digamma_{1} $ | $ ((\flat_{3}, 0), (\flat_{5}, 0)) $ | $ ((\flat_{1}, 0), (\flat_{3}, 0)) $ | $ ((\flat_{2}, 0), (\flat_{4}, 0)) $ | $ ((\flat_{3}, 0), (\flat_{2}, 0)) $ |
$ \digamma_{2} $ | $ ((\flat_{4}, 0), (\flat_{3}, 0)) $ | $ ((\flat_{5}, 0), (\flat_{2}, 0)) $ | $ ((\flat_{2}, 0), (\flat_{3}, 0)) $ | $ ((\flat_{4}, 0), (\flat_{2}, 0)) $ |
$ \digamma_{3} $ | $ ((\flat_{1}, 0), (\flat_{4}, 0)) $ | $ ((\flat_{3}, 0), (\flat_{3}, 0)) $ | $ ((\flat_{1}, 0), (\flat_{3}, 0)) $ | $ ((\flat_{4}, 0), (\flat_{3}, 0)) $ |
$ \digamma_{4} $ | $ ((\flat_{2}, 0), (\flat_{6}, 0)) $ | $ ((\flat_{3}, 0), (\flat_{4}, 0)) $ | $ ((\flat_{5}, 0), (\flat_{3}, 0)) $ | $ ((\flat_{1}, 0), (\flat_{5}, 0)) $ |
$ \digamma_{5} $ | $ ((\flat_{4}, 0), (\flat_{1}, 0)) $ | $ ((\flat_{1}, 0), (\flat_{7}, 0)) $ | $ ((\flat_{6}, 0), (\flat_{1}, 0)) $ | $ ((\flat_{2}, 0), (\flat_{2}, 0)) $ |
$ Q_{1} $ | $ Q_{2} $ | $ Q_{3} $ | $ Q_{4} $ | |
$ \digamma_{1} $ | $ ((\flat_{2}, 0), (\flat_{5}, 0)) $ | $ ((\flat_{4}, 0), (\flat_{2}, 0)) $ | $ ((\flat_{3}, 0), (\flat_{5}, 0)) $ | $ ((\flat_{4}, 0), (\flat_{4}, 0)) $ |
$ \digamma_{2} $ | $ ((\flat_{4}, 0), (\flat_{1}, 0)) $ | $ ((\flat_{5}, 0), (\flat_{2}, 0)) $ | $ ((\flat_{6}, 0), (\flat_{1}, 0)) $ | $ ((\flat_{2}, 0), (\flat_{6}, 0)) $ |
$ \digamma_{3} $ | $ ((\flat_{3}, 0), (\flat_{2}, 0)) $ | $ ((\flat_{3}, 0), (\flat_{5}, 0)) $ | $ ((\flat_{2}, 0), (\flat_{3}, 0)) $ | $ ((\flat_{3}, 0), (\flat_{4}, 0)) $ |
$ \digamma_{4} $ | $ ((\flat_{2}, 0), (\flat_{6}, 0)) $ | $ ((\flat_{1}, 0), (\flat_{4}, 0)) $ | $ ((\flat_{3}, 0), (\flat_{5}, 0)) $ | $ ((\flat_{5}, 0), (\flat_{3}, 0)) $ |
$ \digamma_{5} $ | $ ((\flat_{5}, 0), (\flat_{1}, 0)) $ | $ ((\flat_{2}, 0), (\flat_{2}, 0)) $ | $ ((\flat_{3}, 0), (\flat_{1}, 0)) $ | $ ((\flat_{1}, 0), (\flat_{7}, 0)) $ |
$ Q_{1} $ | $ Q_{2} $ | $ Q_{3} $ | $ Q_{4} $ | |
$ \digamma_{1} $ | $ ((\flat_{1}, 0), (\flat_{6}, 0)) $ | $ ((\flat_{3}, 0), (\flat_{4}, 0)) $ | $ ((\flat_{2}, 0), (\flat_{5}, 0)) $ | $ ((\flat_{1}, 0), (\flat_{5}, 0)) $ |
$ \digamma_{2} $ | $ ((\flat_{4}, 0), (\flat_{4}, 0)) $ | $ ((\flat_{2}, 0), (\flat_{6}, 0)) $ | $ ((\flat_{1}, 0), (\flat_{7}, 0)) $ | $ ((\flat_{4}, 0), (\flat_{2}, 0)) $ |
$ \digamma_{3} $ | $ ((\flat_{2}, 0), (\flat_{3}, 0)) $ | $ ((\flat_{4}, 0), (\flat_{3}, 0)) $ | $ ((\flat_{3}, 0), (\flat_{3}, 0)) $ | $ ((\flat_{4}, 0), (\flat_{1}, 0)) $ |
$ \digamma_{4} $ | $ ((\flat_{3}, 0), (\flat_{5}, 0)) $ | $ ((\flat_{2}, 0), (\flat_{4}, 0)) $ | $ ((\flat_{6}, 0), (\flat_{1}, 0)) $ | $ ((\flat_{4}, 0), (\flat_{3}, 0)) $ |
$ \digamma_{5} $ | $ ((\flat_{7}, 0), (\flat_{1}, 0)) $ | $ ((\flat_{5}, 0), (\flat_{1}, 0)) $ | $ ((\flat_{4}, 0), (\flat_{1}, 0)) $ | $ ((\flat_{5}, 0), (\flat_{3}, 0)) $ |
$ Q_{1} $ | $ Q_{2} $ | $ Q_{3} $ | $ Q_{4} $ | |
G2TLBFWA operator | ||||
$ \digamma_1 $ | $ ((\flat_2, 0.1415), (\flat_5, 0.1857)) $ | $ ((\flat_3, 0.0529), (\flat_3, -0.4054)) $ | $ ((\flat_3, -0.4772), (\flat_5, -0.3238)) $ | $ ((\flat_3, 0.2166), (\flat_3, 0.3973)) $ |
$ \digamma_2 $ | $ ((\flat_4, 0.0000), (\flat_2, -0.1654)) $ | $ ((\flat_5, -0.4461), (\flat_2, 0.4915)) $ | $ ((\flat_4, 0.4274), (\flat_2, 0.0519)) $ | $ ((\flat_3, 0.1010), (\flat_3, 0.4641)) $ |
$ \digamma_3 $ | $ ((\flat_2, 0.2635), (\flat_3, -0.3297)) $ | $ ((\flat_3, 0.2182), (\flat_4, -0.1270)) $ | $ ((\flat_2, -0.0590), (\flat_3, 0.0000)) $ | $ ((\flat_4, -0.4721), (\flat_3, -0.2192)) $ |
$ \digamma_4 $ | $ ((\flat_2, 0.2148), (\flat_6, -0.2148)) $ | $ ((\flat_2, -0.1358), (\flat_4, 0.0000)) $ | $ ((\flat_4, 0.4287), (\flat_3, 0.1090)) $ | $ ((\flat_4, -0.0973), (\flat_3, 0.4968)) $ |
$ \digamma_5 $ | $ ((\flat_5, 0.3747), (\flat_1, 0.0000)) $ | $ ((\flat_3, -0.4705), (\flat_3, -0.4646)) $ | $ ((\flat_4, 0.3675), (\flat_1, 0.0000)) $ | $ ((\flat_2, 0.3582), (\flat_4, 0.0577)) $ |
2TLBFWG operator | ||||
$\digamma_1$ | $((\flat_2, -0.0337), (\flat_5, 0.2337))$ | $((\flat_2, 0.4915), (\flat_3, -0.2382))$ | $((\flat_2, 0.4495), (\flat_5, -0.2704))$ | $((\flat_3, -0.2192), (\flat_4, -0.2648))$ |
$\digamma_2$ | $((\flat_4, 0.0000), (\flat_2, 0.3421))$ | $((\flat_4, 0.1628), (\flat_3, 0.1836))$ | $((\flat_3, 0.0157), (\flat_4, -0.2879))$ | $((\flat_3, -0.1716), (\flat_5, -0.4641))$ |
$\digamma_3$ | $((\flat_2, -0.0104), (\flat_3, -0.1226))$ | $((\flat_3, 0.1777), (\flat_4, 0.1270))$ | $((\flat_2, -0.2383), (\flat_3, 0.0000))$ | $((\flat_3, 0.4641), (\flat_3, 0.2166))$ |
$\digamma_4$ | $((\flat_2, 0.1689), (\flat_6, -0.1689))$ | $((\flat_2, -0.4029), (\flat_4, 0.0000))$ | $((\flat_4, 0.0168), (\flat_4, -0.1426))$ | $((\flat_3, -0.0495), (\flat_4, -0.2896))$ |
$\digamma_5$ | $((\flat_5, 0.0018), (\flat_1, 0.0000))$ | $((\flat_2, -0.0488), (\flat_4, 0.3851))$ | $((\flat_4, -0.0878), (\flat_1, 0.0000))$ | $((\flat_2, -0.3014), (\flat_6, -0.3618))$ |
Alternatives | Overall assessment values by G2TLBFWHM | Overall assessment values by 2TLBFWGHM |
$ \digamma_{1} $ | $ ((\flat_3, -0.4392), (\flat_4, -0.0151)) $ | $ ((\flat_3, -0.1204), (\flat_4, -0.1222)) $ |
$ \digamma_{2} $ | $ ((\flat_4, -0.1760), (\flat_3, -0.1781)) $ | $ ((\flat_4, -0.1615), (\flat_3, 0.2750)) $ |
$ \digamma_{3} $ | $ ((\flat_3, -0.3760), (\flat_4, -0.4739)) $ | $ ((\flat_3, -0.0786), (\flat_3, 0.1544)) $ |
$ \digamma_{4} $ | $ ((\flat_3, 0.1383), (\flat_4, 0.1756)) $ | $ ((\flat_3, -0.0911), (\flat_4, 0.0569)) $ |
$ \digamma_{5} $ | $ ((\flat_4, -0.3497), (\flat_2, 0.2376)) $ | $ ((\flat_3, 0.1549), (\flat_4, -0.3829)) $ |
Weights | Scores | Ranking |
$ \varkappa^{\star}_{1}=0.1, \varkappa^{\star}_{2}=0.2, $ | $ \mathcal{S}^{HM}_{1}=(\flat_3, 0.3689), \mathcal{S}^{HM}_{2}=(\flat_4, 0.2697), \mathcal{S}^{HM}_{3}=(\flat_4, -0.2814), $ | $ {\digamma_{5} > \digamma_{2} > \digamma_{4} > \digamma_{3} > \digamma_{1}} $ |
$ \varkappa^{\star}_{3}=0.3, \varkappa^{\star}_{4}=0.4 $ | $ \mathcal{S}^{HM}_{4}=(\flat_4, -0.2213), \mathcal{S}^{HM}_{5}=(\flat_4, 0.3436) $ | |
$ \varkappa^{\star}_{1}=0.2, \varkappa^{\star}_{2}=0.1, $ | $ \mathcal{S}^{HM}_{1}=(\flat_3, 0.2348), \mathcal{S}^{HM}_{2}=(\flat_4, 0.2770), \mathcal{S}^{HM}_{3}=(\flat_4, -0.2690), $ | $ {\digamma_{5} > \digamma_{2} > \digamma_{4} > \digamma_{3} > \digamma_{1}} $ |
$ \varkappa^{\star}_{3}=0.3, \varkappa^{\star}_{4}=0.4 $ | $ \mathcal{S}^{HM}_{4}=(\flat_4, -0.2562), \mathcal{S}^{HM}_{5}=(\flat_5, -0.4185) $ | |
$ \varkappa^{\star}_{1}=0.3, \varkappa^{\star}_{2}=0.1, $ | $ \mathcal{S}^{HM}_{1}=(\flat_3, 0.2133), \mathcal{S}^{HM}_{2}=(\flat_4, 0.2652) \mathcal{S}^{HM}_{3}=(\flat_4, -0.2444), $ | $ {\digamma_{5} > \digamma_{2} > \digamma_{3} > \digamma_{4} > \digamma_{1}} $ |
$ \varkappa^{\star}_{3}=0.2, \varkappa^{\star}_{4}=0.4, $ | $ \mathcal{S}^{HM}_{4}=(\flat_4, -0.4446), \mathcal{S}^{HM}_{5}=(\flat_5, -0.3323) $ | |
$ \varkappa^{\star}_{1}=0.4, \varkappa^{\star}_{2}=0.1, $ | $ \mathcal{S}^{HM}_{1}=(\flat_3, 0.0671), \mathcal{S}^{HM}_{2}=(\flat_4, 0.4098), \mathcal{S}^{HM}_{3}=(\flat_4, -0.3131), $ | $ {\digamma_{5} > \digamma_{2} > \digamma_{3} > \digamma_{4} > \digamma_{1}} $ |
$ \varkappa^{\star}_{3}=0.2, \varkappa^{\star}_{4}=0.3, $ | $ \mathcal{S}^{HM}_{4}=(\flat_3, 0.3958), \mathcal{S}^{HM}_{5}=(\flat_5, -0.0206) $ |
Weights | Scores | Ranking |
$ \varkappa^{\star}_{1}=0.1, \varkappa^{\star}_{2}=0.2, $ | $ \mathcal{S}^{GHM}_{1}=(\flat_4, -0.3918), \mathcal{S}^{GHM}_{2}=(\flat_4, -0.0843), \mathcal{S}^{GHM}_{3}=(\flat_4, 0.0048), $ | $ {\digamma_{3} > \digamma_{2} > \digamma_{4} > \digamma_{1} > \digamma_{5}} $ |
$ \varkappa^{\star}_{3}=0.3, \varkappa^{\star}_{4}=0.4 $ | $ \mathcal{S}^{GHM}_{4}=(\flat_4, -0.2370), \mathcal{S}^{GHM}_{5}=(\flat_3, 0.2781) $ | |
$ \varkappa^{\star}_{1}=0.2, \varkappa^{\star}_{2}=0.1, $ | $ \mathcal{S}^{GHM}_{1}=(\flat_3, 0.4825), \mathcal{S}^{GHM}_{2}=(\flat_4, -0.0710), \mathcal{S}^{GHM}_{3}=(\flat_4, 0.0133), $ | $ {\digamma_{3} > \digamma_{2} > \digamma_{4} > \digamma_{1} > \digamma_{5}} $ |
$ \varkappa^{\star}_{3}=0.3, \varkappa^{\star}_{4}=0.4 $ | $ \mathcal{S}^{GHM}_{4}=(\flat_4, -0.3175), \mathcal{S}^{GHM}_{5}=(\flat_3, 0.4242) $ | |
$ \varkappa^{\star}_{1}=0.3, \varkappa^{\star}_{2}=0.1, $ | $ \mathcal{S}^{GHM}_{1}=(\flat_3, 0.4177), \mathcal{S}^{GHM}_{2}=(\flat_4, 0.0094), \mathcal{S}^{GHM}_{3}=(\flat_4, 0.0358), $ | $ {\digamma_{3} > \digamma_{2} > \digamma_{4} > \digamma_{5} > \digamma_{1}} $ |
$ \varkappa^{\star}_{3}=0.2, \varkappa^{\star}_{4}=0.4, $ | $ \mathcal{S}^{GHM}_{4}=(\flat_3, 0.4578), \mathcal{S}^{GHM}_{5}=(\flat_3, 0.4436) $ | |
$ \varkappa^{\star}_{1}=0.4, \varkappa^{\star}_{2}=0.1, $ | $ \mathcal{S}^{GHM}_{1}=(\flat_3, 0.2737), \mathcal{S}^{GHM}_{2}=(\flat_4, 0.1855), \mathcal{S}^{GHM}_{3}=(\flat_4, -0.0331), $ | $ {\digamma_{2} > \digamma_{3} > \digamma_{5} > \digamma_{1} > \digamma_{4}} $ |
$ \varkappa^{\star}_{3}=0.2, \varkappa^{\star}_{4}=0.3, $ | $ \mathcal{S}^{GHM}_{4}=(\flat_3, 0.2684), \mathcal{S}^{GHM}_{5}=(\flat_4, -0.1974) $ |
Parameter values | Score functions |
$ s = t = 0.5 $ | $ \mathcal{S}^{HM}_{1} = (\flat_{2}, \; 0.3985), \; \; \mathcal{S}^{HM}_{2} = (\flat_{4}, -0.3920), \; \mathcal{S}^{HM}_{3} = (\flat_{3}, -0.3138), \; \mathcal{S}^{HM}_{4} = (\flat_{2}, \; 0.4184), \; \; \; \mathcal{S}^{HM}_{5} = (\flat_{4}, -0.3323) $ |
$ s = t = 1 $ | $ \mathcal{S}^{HM}_{1} = (\flat_{3}, -0.1830), \; \mathcal{S}^{HM}_{2} = (\flat_{4}, \; 0.0610), \; \; \mathcal{S}^{HM}_{3} = (\flat_{3}, \; 0.1018), \; \; \mathcal{S}^{HM}_{4} = (\flat_{3}, -0.1253), \; \; \mathcal{S}^{HM}_{5} = (\flat_{4}, \; 0.1457) $ |
$ s = t = 2 $ | $ \mathcal{S}^{HM}_{1} = (\flat_{3}, \; 0.1731), \; \; \mathcal{S}^{HM}_{2} = (\flat_{4}, \; 0.4197), \; \; \mathcal{S}^{HM}_{3} = (\flat_{3}, \; 0.4407), \; \; \; \mathcal{S}^{HM}_{4} = (\flat_{3}, \; 0.3053), \; \; \; \mathcal{S}^{HM}_{5} = (\flat_{5}, -0.4105) $ |
$ s = 3, t = 4 $ | $ \mathcal{S}^{HM}_{1} = (\flat_{3}, \; 0.4369), \; \; \mathcal{S}^{HM}_{2} = (\flat_{5}, -0.3629), \; \mathcal{S}^{HM}_{3} = (\flat_{4}, -0.3229), \; \mathcal{S}^{HM}_{4} = (\flat_{4}, -0.3379), \; \mathcal{S}^{HM}_{5} = (\flat_{5}, -0.0659) $ |
$ s = 4, t = 3 $ | $ \mathcal{S}^{HM}_{1} = (\flat_{3}, \; 0.4359), \; \; \mathcal{S}^{HM}_{2} = (\flat_{5}, -0.3434), \; \mathcal{S}^{HM}_{3} = (\flat_{4}, -0.3369), \; \mathcal{S}^{HM}_{4} = (\flat_{4}, -0.3654), \; \mathcal{S}^{HM}_{5} = (\flat_{5}, -0.0288) $ |
$ s = 5, t = 6 $ | $ \mathcal{S}^{HM}_{1} = (\flat_{4}, -0.3663), \; \mathcal{S}^{HM}_{2} = (\flat_{5}, -0.2087), \; \mathcal{S}^{HM}_{3} = (\flat_{4}, -0.1665), \; \mathcal{S}^{HM}_{4} = (\flat_{4}, -0.1007), \; \mathcal{S}^{HM}_{5} = (\flat_{5}, \; 0.2306) $ |
$ s = 6, t = 5 $ | $ \mathcal{S}^{HM}_{1} = (\flat_{4}, -0.3639), \; \mathcal{S}^{HM}_{2} = (\flat_{5}, -0.2005), \; \mathcal{S}^{HM}_{3} = (\flat_{4}, -0.1730), \; \mathcal{S}^{HM}_{4} = (\flat_{4}, -0.1050), \; \mathcal{S}^{HM}_{5} = (\flat_{5}, \; 0.2448) $ |
$ s = 7, t = 8 $ | $ \mathcal{S}^{HM}_{1} = (\flat_{4}, -0.2402), \; \mathcal{S}^{HM}_{2} = (\flat_{5}, -0.1187), \; \mathcal{S}^{HM}_{3} = (\flat_{4}, -0.0700), \; \mathcal{S}^{HM}_{4} = (\flat_{4}, \; 0.0445), \; \; \mathcal{S}^{HM}_{5} = (\flat_{5}, \; 0.4174) $ |
$ s = 8, t = 7 $ | $ \mathcal{S}^{HM}_{1} = (\flat_{4}, -0.2385), \; \mathcal{S}^{HM}_{2} = (\flat_{5}, -0.1144), \; \mathcal{S}^{HM}_{3} = (\flat_{4}, -0.0732), \; \mathcal{S}^{HM}_{4} = (\flat_{4}, \; 0.0446), \; \; \mathcal{S}^{HM}_{5} = (\flat_{5}, \; 0.4241) $ |
$ s = 1, t = 9 $ | $ \mathcal{S}^{HM}_{1} = (\flat_{4}, -0.3257), \; \mathcal{S}^{HM}_{2} = (\flat_{5}, -0.2461), \; \mathcal{S}^{HM}_{3} = (\flat_{4}, -0.1150), \; \mathcal{S}^{HM}_{4} = (\flat_{4}, \; 0.0041), \; \; \mathcal{S}^{HM}_{5} = (\flat_{5}, \; 0.1821) $ |
$ s = 9, t = 1 $ | $ \mathcal{S}^{HM}_{1} = (\flat_{4}, -0.3293), \; \mathcal{S}^{HM}_{2} = (\flat_{5}, -0.1503), \; \mathcal{S}^{HM}_{3} = (\flat_{4}, -0.1849), \; \mathcal{S}^{HM}_{4} = (\flat_{4}, -0.1453), \; \mathcal{S}^{HM}_{5} = (\flat_{5}, 0.3953) $ |
$ s = t = 10 $ | $ \mathcal{S}^{HM}_{1} = (\flat_{4}, -0.1355), \; \mathcal{S}^{HM}_{2} = (\flat_{5}, -0.0439), \; \mathcal{S}^{HM}_{3} = (\flat_{4}, 0.0097), \; \; \; \mathcal{S}^{HM}_{4} = (\flat_{4}, 0.1619), \; \; \; \mathcal{S}^{HM}_{5} = (\flat_{6}, -0.4265) $ |
Parameter values | Score functions |
$ s = t = 0.5 $ | $ \mathcal{S}^{GHM}_{1} = (\flat_{4}, 0.4425), \; \; \mathcal{S}^{GHM}_{2} = (\flat_{5}, 0.2342), \; \; \mathcal{S}^{GHM}_{3} = (\flat_{5}, -0.2274), \mathcal{S}^{GHM}_{4} = (\flat_{4}, 0.3902), \; \; \; \mathcal{S}^{GHM}_{5} = (\flat_{5}, 0.1811) $ |
$ s = t = 1 $ | $ \mathcal{S}^{GHM}_{1} = (\flat_{4}, -0.0219), \mathcal{S}^{GHM}_{2} = (\flat_{5}, -0.2048), \mathcal{S}^{GHM}_{3} = (\flat_{4}, 0.3335), \; \; \mathcal{S}^{GHM}_{4} = (\flat_{4}, -0.0868), \mathcal{S}^{GHM}_{5} = (\flat_{5}, -0.3859) $ |
$ s = t = 2 $ | $ \mathcal{S}^{GHM}_{1} = (\flat_{4}, -0.4094), \mathcal{S}^{GHM}_{2} = (\flat_{4}, 0.4340), \; \; \mathcal{S}^{GHM}_{3} = (\flat_{4}, -0.0223), \mathcal{S}^{GHM}_{4} = (\flat_{4}, -0.4951), \mathcal{S}^{GHM}_{5} = (\flat_{4}, 0.0061) $ |
$ s = 3, t = 4 $ | $ \mathcal{S}^{GHM}_{1} = (\flat_{3}, 0.3385), \; \; \mathcal{S}^{GHM}_{2} = (\flat_{4}, 0.1488), \; \; \mathcal{S}^{GHM}_{3} = (\flat_{4}, -0.2615), \mathcal{S}^{GHM}_{4} = (\flat_{3}, 0.2222), \; \; \mathcal{S}^{GHM}_{5} = (\flat_{3}, 0.4955) $ |
$ s = 4, t = 3 $ | $ \mathcal{S}^{GHM}_{1} = (\flat_{3}, 0.3027), \; \; \mathcal{S}^{GHM}_{2} = (\flat_{4}, 0.2111), \; \; \mathcal{S}^{GHM}_{3} = (\flat_{4}, -0.2736), \mathcal{S}^{GHM}_{4} = (\flat_{3}, 0.1584), \; \; \mathcal{S}^{GHM}_{5} = (\flat_{4}, -0.4688) $ |
$ s = 5, t = 6 $ | $ \mathcal{S}^{GHM}_{1} = (\flat_{3}, 0.1412), \; \; \mathcal{S}^{GHM}_{2} = (\flat_{4}, -0.0319), \mathcal{S}^{GHM}_{3} = (\flat_{4}, -0.4497), \mathcal{S}^{GHM}_{4} = (\flat_{3}, -0.0616), \mathcal{S}^{GHM}_{5} = (\flat_{3}, 0.1477) $ |
$ s = 6, t = 5 $ | $ \mathcal{S}^{GHM}_{1} = (\flat_{3}, 0.1198), \; \; \mathcal{S}^{GHM}_{2} = (\flat_{4}, 0.0002), \; \; \mathcal{S}^{GHM}_{3} = (\flat_{4}, -0.4552), \mathcal{S}^{GHM}_{4} = (\flat_{3}, -0.0884), \mathcal{S}^{GHM}_{5} = (\flat_{3}, 0.1650) $ |
$ s = 7, t = 8 $ | $ \mathcal{S}^{GHM}_{1} = (\flat_{3}, 0.0215), \; \; \mathcal{S}^{GHM}_{2} = (\flat_{4}, -0.1589), \mathcal{S}^{GHM}_{3} = (\flat_{3}, 0.4237), \; \; \mathcal{S}^{GHM}_{4} = (\flat_{3}, -0.2577), \mathcal{S}^{GHM}_{5} = (\flat_{3}, -0.0660) $ |
$ s = 8, t = 7 $ | $ \mathcal{S}^{GHM}_{1} = (\flat_{3}, 0.0074), \; \; \mathcal{S}^{GHM}_{2} = (\flat_{4}, -0.1413), \mathcal{S}^{GHM}_{3} = (\flat_{3}, 0.4217), \; \; \mathcal{S}^{GHM}_{4} = (\flat_{3}, -0.2677), \mathcal{S}^{GHM}_{5} = (\flat_{3}, -0.0561) $ |
$ s = 1, t = 9 $ | $ \mathcal{S}^{GHM}_{1} = (\flat_{3}, 0.2199), \; \; \mathcal{S}^{GHM}_{2} = (\flat_{4}, -0.1981), \mathcal{S}^{GHM}_{3} = (\flat_{4}, -0.4622), \mathcal{S}^{GHM}_{4} = (\flat_{3}, 0.0421), \; \; \mathcal{S}^{GHM}_{5} = (\flat_{3}, 0.0067) $ |
$ s = 9, t = 1 $ | $ \mathcal{S}^{GHM}_{1} = (\flat_{3}, 0.0159), \; \; \mathcal{S}^{GHM}_{2} = (\flat_{4}, 0.1304), \; \; \mathcal{S}^{GHM}_{3} = (\flat_{3}, 0.4816), \; \; \mathcal{S}^{GHM}_{4} = (\flat_{3}, -0.2845), \mathcal{S}^{GHM}_{5} = (\flat_{3}, 0.2408) $ |
$ s = t = 10 $ | $ \mathcal{S}^{GHM}_{1} = (\flat_{3}, -0.0821), \mathcal{S}^{GHM}_{2} = (\flat_{4}, -0.2708), \mathcal{S}^{GHM}_{3} = (\flat_{3}, 0.3125), \; \mathcal{S}^{GHM}_{4} = (\flat_{3}, -0.4277), \mathcal{S}^{GHM}_{5} = (\flat_{3}, -0.2376) $ |
Parameters | G2TLBFWHM operator | 2TLBFWGHM operator |
$ s = t = 0.5 $ | $ \digamma_{5} > \digamma_{2} > \digamma_{3} > \digamma_{4} > \digamma_{1} $ | $ \digamma_{2} > \digamma_{5} > \digamma_{3} > \digamma_{1} > \digamma_{4} $ |
$ s = t = 1 $ | $ \digamma_{5} > \digamma_{2} > \digamma_{3} > \digamma_{4} > \digamma_{1} $ | $ \digamma_{2} > \digamma_{5} > \digamma_{3} > \digamma_{1} > \digamma_{4} $ |
$ s = t = 2 $ | $ \digamma_{5} > \digamma_{2} > \digamma_{3} > \digamma_{4} > \digamma_{1} $ | $ \digamma_{2} > \digamma_{5} > \digamma_{3} > \digamma_{1} > \digamma_{4} $ |
$ s = 3, t = 4 $ | $ \digamma_{5} > \digamma_{2} > \digamma_{3} > \digamma_{4} > \digamma_{1} $ | $ \digamma_{2} > \digamma_{3} > \digamma_{5} > \digamma_{1} > \digamma_{4} $ |
$ s = 4, t = 3 $ | $ \digamma_{5} > \digamma_{2} > \digamma_{3} > \digamma_{4} > \digamma_{1} $ | $ \digamma_{2} > \digamma_{3} > \digamma_{5} > \digamma_{1} > \digamma_{4} $ |
$ s = 5, t = 6 $ | $ \digamma_{5} > \digamma_{2} > \digamma_{4} > \digamma_{3} > \digamma_{1} $ | $ \digamma_{2} > \digamma_{3} > \digamma_{5} > \digamma_{1} > \digamma_{4} $ |
$ s = 6, t = 5 $ | $ \digamma_{5} > \digamma_{2} > \digamma_{4} > \digamma_{3} > \digamma_{1} $ | $ \digamma_{2} > \digamma_{3} > \digamma_{5} > \digamma_{1} > \digamma_{4} $ |
$ s = 7, t = 8 $ | $ \digamma_{5} > \digamma_{2} > \digamma_{4} > \digamma_{3} > \digamma_{1} $ | $ \digamma_{2} > \digamma_{3} > \digamma_{1} > \digamma_{5} > \digamma_{4} $ |
$ s = 8, t = 7 $ | $ \digamma_{5} > \digamma_{2} > \digamma_{4} > \digamma_{3} > \digamma_{1} $ | $ \digamma_{2} > \digamma_{3} > \digamma_{1} > \digamma_{5} > \digamma_{4} $ |
$ s = 1, t = 9 $ | $ \digamma_{5} > \digamma_{2} > \digamma_{4} > \digamma_{3} > \digamma_{1} $ | $ \digamma_{2} > \digamma_{3} > \digamma_{1} > \digamma_{4} > \digamma_{5} $ |
$ s = 9, t = 1 $ | $ \digamma_{5} > \digamma_{2} > \digamma_{4} > \digamma_{3} > \digamma_{1} $ | $ \digamma_{2} > \digamma_{3} > \digamma_{5} > \digamma_{1} > \digamma_{4} $ |
$ s = t = 10 $ | $ \digamma_{5} > \digamma_{2} > \digamma_{4} > \digamma_{3} > \digamma_{1} $ | $ \digamma_{2} > \digamma_{3} > \digamma_{1} > \digamma_{5} > \digamma_{4} $ |
2TLBFWA | Scores values | The order | |
$ \digamma_{1} $ | $ ((\flat_3, -0.2530), (\flat_4, -0.2456)) $ | $ (\flat_3, 0.4963) $ | $ {\digamma_{5} > \digamma_{2} > \digamma_{3} > f \digamma_{4} > \digamma_{1}} $ |
$ \digamma_{2} $ | $ ((\flat_4, 0.1497), (\flat_2, 0.3458)) $ | $ (\flat_5, -0.0981) $ | |
$ \digamma_{3} $ | $ ((\flat_3, -0.2482), (\flat_3, 0.1161)) $ | $ (\flat_4, -0.1822) $ | |
$ \digamma_{4} $ | $ ((\flat_3, 0.1559), (\flat_4, -0.0348)) $ | $ (\flat_4, -0.4046) $ | |
$ \digamma_{5} $ | $ ((\flat_4, -0.1614), (\flat_2, -0.2589)) $ | $ (\flat_5, 0.0488) $ |
2TLBFWG | Scores values | The order | |
$ \digamma_{1} $ | $ ((\flat_2, 0.3982), (\flat_4, 0.1699)) $ | $ (\flat_3, 0.1142) $ | $ {\digamma_{2} > \digamma_{5} > \digamma_{3} > \digamma_{1} > \digamma_{2}} $ |
$ \digamma_{2} $ | $ ((\flat_4, -0.4867), (\flat_3, 0.4502)) $ | $ (\flat_4, 0.0316) $ | |
$ \digamma_{3} $ | $ ((\flat_2, 0.4733), (\flat_3, 0.3939)) $ | $ (\flat_4, -0.4603) $ | |
$ \digamma_{4} $ | $ ((\flat_2, 0.4698), (\flat_4, 0.4454)) $ | $ (\flat_3, 0.0122) $ | |
$ \digamma_{5} $ | $ ((\flat_3, -0.1526), (\flat_3, 0.3604)) $ | $ (\flat_4, -0.2565) $ |
2TLBFWHM | Scores values | The order | |
$ \digamma_{1} $ | $ ((\flat_6, 0.1111), (\flat_1 0.2620)) $ | $ (\flat_6, 0.4246) $ | $ {\digamma_{2} > \digamma_{5} > \digamma_{3} > \digamma_{4} > \digamma_{1}} $ |
$ \digamma_{2} $ | $ ((\flat_7, -0.2399), (\flat_1-0.3123)) $ | $ (\flat_7, 0.0362) $ | |
$ \digamma_{3} $ | $ ((\flat_6, 0.0880), (\flat_1-0.0719)) $ | $ (\flat_7, -0.4201) $ | |
$ \digamma_{4} $ | $ ((\flat_6, 0.2394), (\flat_1 0.3353)) $ | $ (\flat_6, 0.4520) $ | |
$ \digamma_{5} $ | $ ((\flat_7, -0.4646), (\flat_1-0.4275)) $ | $ (\flat_7, -0.0185) $ |
2TLBFWDHM | Scores values | The order | |
$ \digamma_{1} $ | $ ((\flat_1, -0.3159), (\flat_7, -0.2518)) $ | $ (\flat_1, -0.0321) $ | $ {\digamma_{5} > \digamma_{2} > \digamma_{3} > \digamma_{1} > \digamma_{4}} $ |
$ \digamma_{2} $ | $ ((\flat_1, 0.0870), (\flat_6, 0.4438)) $ | $ (\flat_1, 0.3216) $ | |
$ \digamma_{3} $ | $ ((\flat_1, -0.2613), (\flat_6, 0.4293)) $ | $ (\flat_1, 0.1547) $ | |
$ \digamma_{4} $ | $ ((\flat_1, -0.2369), (\flat_7, -0.1422)) $ | $ (\flat_1, -0.0473) $ | |
$ \digamma_{5} $ | $ ((\flat_1, -0.0449), (\flat_6, -0.0374)) $ | $ (\flat_1, 0.4962) $ |
2TLBFWMSM | Scores values | The order | |
$ \digamma_{1} $ | $ ((\flat_6, 0.1130), (\flat_1, 0.2595)) $ | $ (\flat_6, 0.4267) $ | $ {\digamma_{2} > \digamma_{5} > \digamma_{3} > \digamma_{4} > \digamma_{1}} $ |
$ \digamma_{2} $ | $ ((\flat_7, -0.2398), (\flat_1, -0.3126)) $ | $ (\flat_7, 0.0364) $ | |
$ \digamma_{3} $ | $ ((\flat_6, 0.0917), (\flat_1, -0.0734)) $ | $ (\flat_7, -0.4175) $ | |
$ \digamma_{4} $ | $ ((\flat_6, 0.2487), (\flat_1, 0.3320)) $ | $ (\flat_6, 0.4584) $ | |
$ \digamma_{5} $ | $ ((\flat_7, -0.4588), (\flat_1, -0.4291)) $ | $ (\flat_7, -0.0149) $ |
2TLBFWDMSM | Scores values | The order | |
$ \digamma_{1} $ | $ ((\flat_1, -0.3161), (\flat_7, -0.2470)) $ | $ (\flat_1, -0.0346) $ | $ {\digamma_{5} > \digamma_{2} > \digamma_{3} > \digamma_{1} > \digamma_{4}} $ |
$ \digamma_{2} $ | $ ((\flat_1, 0.0852), (\flat_6, 0.4470)) $ | $ (\flat_1, 0.3191) $ | |
$ \digamma_{3} $ | $ ((\flat_1, -0.2622), (\flat_6, 0.4300)) $ | $ (\flat_1, 0.1539) $ | |
$ \digamma_{4} $ | $ ((\flat_1, -0.2383), (\flat_7, -0.1403)) $ | $ (\flat_1, -0.0490) $ | |
$ \digamma_{5} $ | $ ((\flat_1, -0.0483), (\flat_6, -0.0121)) $ | $ (\flat_1, 0.4819) $ |