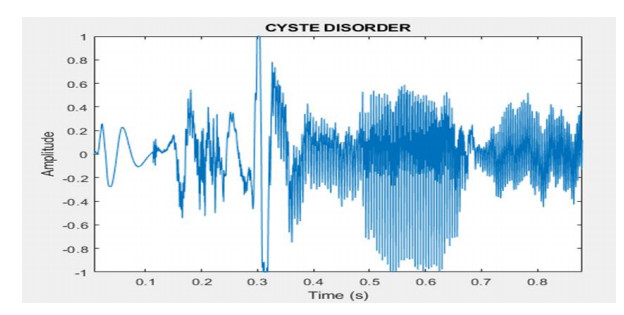
Voice pathologies are irregular vibrations produced due to vocal folds and various factors malfunctioning. In medical science, novel machine learning algorithms are applied to construct a system to identify disorders that occur invoice. This study aims to extract the features from the audio signals of four chosen diseases from the SVD dataset, such as laryngitis, cyst, non-fluency syndrome, and dysphonia, and then compare the four results of machine learning algorithms, i.e., SVM, Naïve Byes, decision tree and ensemble classifier. In this project, we have used a comparative approach along with the new combination of features to detect voice pathologies which are laryngitis, cyst, non-fluency syndrome, and dysphonia from the SVD dataset. The combination of specific 13 MFCC (mel-frequency cepstral coefficients) features along with pitch, zero crossing rate (ZCR), spectral flux, spectral entropy, spectral centroid, spectral roll-off, and short term energy for more accurate detection of voice pathologies. It is proven that the combination of features extracted gives the best product on the audio, which split into 10 ms. Four machine learning classifiers, SVM, Naïve Bayes, decision tree and ensemble classifier for the inter classifier comparison, give 93.18, 99.45,100 and 51%, respectively. Out of these accuracies, both Naïve Bayes and the decision tree show the most promising results with a higher detection rate. Naïve Bayes and decision tree gives the highest reported outcomes on the selected set of features in the proposed methodology. The SVM has also been concluded to be the commonly used voice condition identification algorithm.
Citation: Sidra Abid Syed, Munaf Rashid, Samreen Hussain, Anoshia Imtiaz, Hamnah Abid, Hira Zahid. Inter classifier comparison to detect voice pathologies[J]. Mathematical Biosciences and Engineering, 2021, 18(3): 2258-2273. doi: 10.3934/mbe.2021114
[1] | Sidra Abid Syed, Munaf Rashid, Samreen Hussain . Meta-analysis of voice disorders databases and applied machine learning techniques. Mathematical Biosciences and Engineering, 2020, 17(6): 7958-7979. doi: 10.3934/mbe.2020404 |
[2] | Lal Hussain, Wajid Aziz, Ishtiaq Rasool Khan, Monagi H. Alkinani, Jalal S. Alowibdi . Machine learning based congestive heart failure detection using feature importance ranking of multimodal features. Mathematical Biosciences and Engineering, 2021, 18(1): 69-91. doi: 10.3934/mbe.2021004 |
[3] | Desire Ngabo, Wang Dong, Ebuka Ibeke, Celestine Iwendi, Emmanuel Masabo . Tackling pandemics in smart cities using machine learning architecture. Mathematical Biosciences and Engineering, 2021, 18(6): 8444-8461. doi: 10.3934/mbe.2021418 |
[4] | Wajid Aziz, Lal Hussain, Ishtiaq Rasool Khan, Jalal S. Alowibdi, Monagi H. Alkinani . Machine learning based classification of normal, slow and fast walking by extracting multimodal features from stride interval time series. Mathematical Biosciences and Engineering, 2021, 18(1): 495-517. doi: 10.3934/mbe.2021027 |
[5] | Liangyu Yang, Tianyu Shi, Jidong Lv, Yan Liu, Yakang Dai, Ling Zou . A multi-feature fusion decoding study for unilateral upper-limb fine motor imagery. Mathematical Biosciences and Engineering, 2023, 20(2): 2482-2500. doi: 10.3934/mbe.2023116 |
[6] | Natalya Shakhovska, Vitaliy Yakovyna, Valentyna Chopyak . A new hybrid ensemble machine-learning model for severity risk assessment and post-COVID prediction system. Mathematical Biosciences and Engineering, 2022, 19(6): 6102-6123. doi: 10.3934/mbe.2022285 |
[7] | Xiao Wang, Jianbiao Zhang, Ai Zhang, Jinchang Ren . TKRD: Trusted kernel rootkit detection for cybersecurity of VMs based on machine learning and memory forensic analysis. Mathematical Biosciences and Engineering, 2019, 16(4): 2650-2667. doi: 10.3934/mbe.2019132 |
[8] | Lili Jiang, Sirong Chen, Yuanhui Wu, Da Zhou, Lihua Duan . Prediction of coronary heart disease in gout patients using machine learning models. Mathematical Biosciences and Engineering, 2023, 20(3): 4574-4591. doi: 10.3934/mbe.2023212 |
[9] | Yue Li, Wusheng Xu, Wei Li, Ang Li, Zengjin Liu . Research on hybrid intrusion detection method based on the ADASYN and ID3 algorithms. Mathematical Biosciences and Engineering, 2022, 19(2): 2030-2042. doi: 10.3934/mbe.2022095 |
[10] | Jian-xue Tian, Jue Zhang . Breast cancer diagnosis using feature extraction and boosted C5.0 decision tree algorithm with penalty factor. Mathematical Biosciences and Engineering, 2022, 19(3): 2193-2205. doi: 10.3934/mbe.2022102 |
Voice pathologies are irregular vibrations produced due to vocal folds and various factors malfunctioning. In medical science, novel machine learning algorithms are applied to construct a system to identify disorders that occur invoice. This study aims to extract the features from the audio signals of four chosen diseases from the SVD dataset, such as laryngitis, cyst, non-fluency syndrome, and dysphonia, and then compare the four results of machine learning algorithms, i.e., SVM, Naïve Byes, decision tree and ensemble classifier. In this project, we have used a comparative approach along with the new combination of features to detect voice pathologies which are laryngitis, cyst, non-fluency syndrome, and dysphonia from the SVD dataset. The combination of specific 13 MFCC (mel-frequency cepstral coefficients) features along with pitch, zero crossing rate (ZCR), spectral flux, spectral entropy, spectral centroid, spectral roll-off, and short term energy for more accurate detection of voice pathologies. It is proven that the combination of features extracted gives the best product on the audio, which split into 10 ms. Four machine learning classifiers, SVM, Naïve Bayes, decision tree and ensemble classifier for the inter classifier comparison, give 93.18, 99.45,100 and 51%, respectively. Out of these accuracies, both Naïve Bayes and the decision tree show the most promising results with a higher detection rate. Naïve Bayes and decision tree gives the highest reported outcomes on the selected set of features in the proposed methodology. The SVM has also been concluded to be the commonly used voice condition identification algorithm.
Voice pathology influences the patient's speaking ability, affecting disorders, including agitation, voice tiredness, trouble pronunciation the phrases. There are, in total, three instances of associated illnesses used in this work: dysphonia, laryngitis, and vocal cord paralysis [1]. The trouble in sustaining the voice is linked to dysphonia and primarily to the heartbeat. Chronic laryngitis often includes hoarseness effects, triggered by larynx irritation for a lengthy period of time. Vocal cord paralysis impacts the patient's speech and breathing and frequently contributes to a lack of vocal capacity as all cords are paralyzed. The diagnostic test to classify these language pathologies is rather intrusive for the patient. Thus, the research and production of machine learning approaches have risen in recent years [2]. In the case of various pathologies, most works have created, using audio with a steady voice, mostly vowel /a/ [3] or other vows such as /i/ and /u/ [4,5], as well as obtaining satisfactory results. However, there are still very few continuous voice databases with medical diagnostic annotations accessible to study, notwithstanding the scientific interests. Based on the previous record the specialized doctors or physician detects that whether it's a pathological or normal voice in subjective detection. Now the objective detection is getting more attention in voice diseases detection in medical diagnosis. The risk of voice pathologies is increasing in individuals [6]. In medical science, engineering and programming are induced to construct a system to identify voice disorders at the beginning of these diseases. One of the open problems is voice disease identification and detection. It is about detecting how to sever the voice disease because when the severity of the disease is low, the patient can easily pronounce the vowel.
Vocal folds are affected during phonation in voice disorders, due to which the vocal folds produce asymmetrical vibrations. The asymmetrical vibrations may be due to the malfunctioning factors responsible for producing vibrations [7]. There is not any kind of tracheobronchial effect on the vocal tract when the vowels are produced. The vocal tract is influenced by the structure of infra laryngeal and articulatory interaction in the vocal tract and articulatory in vocal folds [8]. Information related to the vocal tract is predictable and allows vocal folds identification characteristics, especially in phonation [9]. It depends on the type and location of voice disease in vocal folds, affecting the vibration production during phonation. There are certain factors upon which the vibration of vocal folds depends, and they're as follow.
● Presence of mucous on the tissue of vocal fold.
● The stiffness of vocal fold
● The tension created in the vocal fold
● The muscles of the larynx
● Folds opening and closing
As certain different types of voice diseases affect these factors differently. The closure of vocal folds is different in vibration because of the disease's size and location; due to this, there is variation in vibrations the diseases are differentiated. The research fields which are present in speech are speech and speaker recognition, synthesis of speech, coding of speech. The automatic identification of the speaker has the following objectives: feature extraction, feature characterization, and recognition of speaker identity. Dysphonia and other voice pathological problems were increasing drastically in the US; about 7.5 million people were having voice disease [10]. The main aim and main concern are to establish a system that will identify the disease of voice correctly and accurately. The acoustic analysis is not dependent upon the interventions of humans and will help in making the decision to the clinicians. However, the doctors know the decision according to the diagnosis, and this system will act as an assistive tool to them. The proposed methodology is the continuity of our research work [11] that was the meta-analysis of classifiers used for voice disorders. The gaps that we have identified in [11] tried to full fill in the proposed methodology. In our research, we have used the free access database of voice pathology, SVD. This database is recorded by the institute of phonetics of Saarland University. The noninvasive technique which is based on digital processing of given audio signals is acoustic analysis. It is an efficient technique used in the diagnosis and identification of voice diseases. Two types of analysis are used in automatic voice disorder identification i.e., short-term analysis and long-term analysis. The long-term analysis takes the parameters which are yield through acoustic analysis of an audio signal [12] whereas the parameters of short-term analysis are yield from MFCC (Mel-frequency cepstral coefficients), LPCC, and LPC [8]. MFCC is based on short time spectral observations. Mel frequency cepstral coefficient act on the human auditory system. That's why it is used to extract the Features from the given audio signal and is very useful in identifying voice diseases [13].
In [14], the key goal of Nasheri et al. works is to develop accurate and robust feature extraction to classify and differentiate voice disease by autocorrelation and entropy analysis of several frequency bands. From each frame, maximal peak values and corresponding lag values were obtained, utilizing autocorrelation as a function for the identification and differentiation of pathological samples. After normalizing the values to be used as functions, we have obtained entropy for and frame of the talk signal. These features were tested in various frequency bands for the recognition and classification schemes to assess the contributions of each unit. Various continuous vocal examples were obtained for both regular and irregular voices in English, German and Arabic from three distinct datasets. As a classifier, the support vector machine was used. 92% for SVD is the best-recorded accuracy [15]. In [15], the paper's main objective is to evaluate multidimensional voice parameters (MDVP) such that voice pathologies can be automatically defined and separated in multiple data sets before deciding the parameters of the two processes, which are well-compared. The experimental results indicate that the utility of the MDPV parameters using these databases is clearly different. Substantially rated parameters differ from database to database. The three top-rated MDVPs organized with the fisher prejudice ratio were used to obtain optimum accuracy: 99.98 percent for SVD. The article [16] utilizes a correlation approach in order to define and identify pathological materials to extract optimum pick values and their corresponding lag values from each frame of the spoken signal. In different frequency bands, these features are tested to assess the contribution of each band to processes of recognition and classification. The most contributing bands are between 1000 and 8000 Hz for detection and classification. Maximum accurate consistency in Massachusetts Eye and Ear Infirmary, the Saarbrücken Expression Database, and the Arabic Voice Pathology Database was 99.809, 90.979 and 91.16%. However, 99.255, 98.941 and 95.188% respectively in three datasets reached full accuracy by cross-correlation. Teixeira proposed the voice recognition device and, in both, his publications retained the same features but modified the classifications. In [17] SVM was used with Jitter, Glitter, and HNR, and the accuracy recorded was 71%. The recorded accuracy was 100% but only for female voices in the [18] MLP-ANN used for jitter, glitter, and HNR. In [19], the recorded accuracy of Fonseca et al. using SVM with SE, ZCRs, SH was 95%. Many automated disorders detection systems were established by applying various types of traditional voice characteristics such as linear prediction coefficients, linear cepstral coefficients, and cepstral coefficients of Mel-frequency (MFCCs). The aim of the research is to see whether traditional voice features accurately identify voice pathology and can compare it with voice quality [20].In order for this research to be investigated, an automated MFCC method of detection was developed, and three separate databases of voice impairment were used. The findings of the experiment indicate that the exactness of the MFCC dependent method differs between the database and the database. The intra-database detections vary from 72 to 95%, but for the SVD database, the highest reported accuracy was 80% [20]. In [21], while assessing voice pathologies, when 13 conventional MFCC features were extracted, the highest reported accuracy is 72% using the SVM as a machine learning classifier.
SVD stands for Saarbrücken Voice Database. Basically, SVD is a freely accessible archive, a compilation of voice recordings from more than 2000 individuals. Vocal register provided at normal, high, and low pitch. The reality has been documented in a recording session-Voice documentation for increasing pitch. The voice signal and the EGG signal have been stored in individual files for the specified components [22]. The database has a text file containing all applicable dataset material. These features make it a reasonable alternative for the experimenters to use. Both captured SVD voices were sampled at a resolution of 16-bit at 50 kHz. The Saarbruecken Voice Server is accessible via this web interface. It includes several internet pages that are used to choose the parameters for the database program, to play directly and to record, and to select the listening session files that are to be exported from the SVD database to the required parameter [14]. From the SVD index, the diseases we picked are laryngitis, cyst, non-fluency syndrome, and dysphonia and we have also used audio samples of vowels (a, e, i, o, u) [22].
SVM is a good way to build a classifier. It seeks to establish a decision limit between two groups, which will enable labels to be predicted from one or more vectors. This choice, called the hyper-plane, is so directed that from the nearest data points in each class, it is as far as possible. The nearest points are regarded as support vectors [23]. An SVM classifier generates the most advanced hyperplane in the transformed entrance space and separates the outstanding groups, and maximizes the gap to cleanly separated instances nearby. A quadratic optimization problem is the parameters of the hyperplane approach [24]. So to label a dataset:
(x1,y1),…,(xn,yn),xi∈Rdandyi∈(−1,+1) |
where xi is a characteristic vector representation and yi is a class mark (negative or positive) of a training formula i. The optimum hyperplane can then be described as:
wxT+b=0 |
where w is the weight matrix, x is the input vector, and b is the bias. For all the elements of the training collection, w and b must fulfill the following inequalities:
wxT+b≥+1ifyi=1 |
wxT+b≤−1ifyi=−1 |
The main aim is to find w and b so that we can form a hyperplane and increase the margin 1/‖w‖2. Vectors xi for which |yi|(wxiT+b)=1 will be termed support-vector. In the proposed methodology, as shown in graph 1, linear SVM, quadratic SVM, cubic SVM, fine Gaussian SVM, medium Gaussian SVM, and coarse Gaussian have been used to check the trained model.
In the Naive Bayes classification, the conditional likelihood is centered on the features in one class after the collection of features utilizing current processes. It identifies functions by an established system of selection and selects a supplementary functionality that can reclassify the class space for the features selected [25]. Denote a vector of variables D=(di),i=1,2,…,n, where di is corresponding to a letter, a word, or other attributes about some text in reality, and a set of C={C1,C2,…,Ck} is predefined classes. Text classification is to assign a class label Cj,j=1,2,…,k from C to a document. In essence, the Bayes Classifier is a hybrid probability model parameter:
P(cj|D)=P(cj)P(D|cj)P(D) |
where P(cj) is previous details on the obvious likelihood of a class is the information from the observations, which is the experience of the text itself to be graded, and P(D|cj) is the probability of the distribution of document D in class space. As the naive Bayes assigns the most likelihood text to the class, the Naive Bayes is perfect in the context of probability. As speech recognition has the problem of multiple classes' classification so, Naïve Bayes can handle such problems and works well. Naïve Bayes works on Bayesian theorem along with naïve assumption, i.e., the pair of features are independent of each other. Naïve Bayes classifier works well in real world conditions. Naïve Bayes classifier has good performance and also works fast. The benefits of using naïve Bayes classifier are situational unconventional assumption that helps to get fast classification results, probabilistic assumption, and high accuracy [26,27]. By the trained data, the Naïve Bayes classifier is made while on the basis of the dataset, the algorithm is formed. The trained data is classified under four classes' dysphonia, cyst, laryngitis, and non-fluency syndrome. Gaussian and kernel approaches have been used to test the model.
A decision tree categorizes data objects by answering a range of questions about their characteristics. Each query is embedded in an internal node, and with each potential answer to its question, each inner node points to a child node. The questions shape a tree-encoded hierarchy. In the simplest type, we pose questions of yes or no, and there is a yes child and no child in an inner node. An object is sorted into a class in conjunction with the answers to the item in question by following the way from the top node, the root, to a child-loss node. The class associated with the leaf that reaches is allocated an object. In certain combinations, each leaf includes a distribution of probabilities around the groups, which calculates the likelihood that an object that enters the leaf is of a specific class. However, it may be challenging to quantify impartial odds [28].
Ensemble methods are algorithms that build up a group of classifiers and then categories new data points via a (weighted) majority of their correlations. The initial ensemble approach is averaging in Bayesian but modern algorithms provide error correction, bagging, and boosting performance [29]. Ensemble learning is about multiple classifiers systems. Training of distinguish classifier is included, and then predictions are combined to get better accuracy result of classification. The method of ensemble tries to gather a set of the learner as compared to ordinary approaches of learning, and this helps to make predictions based on a single learner [30]. Outstanding results of the stable classifier are received by the Bagged tree [31]. It deals with artificial and also real-world problems and also helps to improve the accuracy of some classifiers.
Speech signals are split into frames because speech signals are constant or non-varying for a short period of time, so the characteristics of the frame in the form of features are extracted where the speech signals remain unchanged [32]. We have used a supervised machine learning algorithm for which we have taken the audio signals from the SVD dataset and extracted the features that can be used in the classifier learner app as an input signal. Then the feature vector is constructed from each. The popular audio features are using the technique of Mel Frequency Cepstral coefficient. Other than 13 MFCC and pitch, we have six other features, which are spectral flux, spectral centroid, ZCR, roll-off, short-term energy, and spectral entropy. It has been observed in a literature review [20] and [21] that when MFCC was used alone, the reported accuracy was not better, i.e., 72 and 80%. So to increase the accuracy and for better detection of voice pathologies, we have added 7 more features and extracted them on audio features along with 13 conventional MFCC features.
Mel frequency cepstral coefficient works on the principle of the human auditory system. It is used to extract the features from the audio signal datasets. In MFCC, the bands of frequency using the Mel scale parameter there is the equal spacing that is very close to the human auditory system as compared to Cepstrum, which has linear spacing in bands of frequency. According to the psychophysical study, the human auditory system doesn't have a linear scale that it follows. Every incoming audio signal has an original frequency 'f', which is measured in Hz, and a pitch is measured on the Mel scale [33]. MFCC is said to be coefficients that are derived from audio signals. The speech input is actually the audio signal input that undergoes the process of framing. Before framing the audio signal undergoes the process of pre-emphasis, this is beneficial to achieve good accuracy and good efficiency level. Using this process, the compensation in higher frequency is achieved that is curbed in the human auditory system during sound production.
c2(n)=c(n)−d∗c(n−1) |
Here c2(n) shows the signal of output and d recommended value is 0.9 and 1 [33]. The filter's z transform is as follow,
H(z)=1−d∗z∧(−1) |
After the process of pre-emphasis now the aim is to split the entire audio signal into a number of frames to easily analyze and interpret every frame signal. The audio signal is split into a frame of around 10 ms while the Standard framing size is 25 ms [34]. It shows that the length of the frame for the 50 kHz audio signal will be 50 k * 0.01 = 500 samples. The step of framing allows the overlapping of the frames. The step of the frame is 10 ms for the first 500 samples. The 500 sample frame starts at 0 samples, and then another 500 sample frame will begin at sample 501 and so on until the last audio signal is reached. The reason for taking a 10 ms signal is that audio recordings in the SVD database [22] are very small, i.e., vowel recordings, so the suitable recording time as per the database is 10 ms. There is some effect of spectral artifacts after framing; these effects are reduced by applying Hamming window function. The combination of short-term spectrum and the transfer function of window (hamming) becomes convolution in the frequency domain. In order to maintain continuity between the first and last marks in the frame, each frame has to be multiplied with the function of hamming window [35]. The efficient function of the window has a narrow main lobe and low side lobe. The hamming window function is given below,
K(n)=0.54−0.46cos(2πn/N−1),0≤n≤N−1 |
K(n) represents the window, and the output is given by Q (n) below, whereas X (n) shows the input frame signal.
Q(n)=X(n)∗w(n) |
Now the signal needs to be converted into the frequency domain signal from the time domain signal. For that, FFT (Fast Fourier transformation) is applied to the signal to prepare it for the next stage of the Mel filter bank [35]. The mathematical expression is given below. The period gram of audio signal (CYSTE1.WAV) before the MFCC is shown below in Figure 1.
Q(ω)=FFT[k(t)∗X(t)]=k(ω)∗X(ω) |
Now the original frequency of the signal is converted into the Mel frequency using the Mel filter bank. The Mel filter bank consists of a group of triangular filters. There is not any uniform spacing between the filters, and fewer filters are present in the higher frequency region while a greater number of filters are present in the lower frequency region [34]. The unique property of Filter bank is that it can be applicable on time domain and also in frequency domain signals. In the processing of Mel frequency cepstral coefficient (MFCC), it is applicable in frequency domain signals.
In Figure 2, the filter applied in the region of lower and higher frequency range showing the change in frequency. The perceived frequency, the pitch of a tone, is related with the Mel scale to its original frequency measured. The human auditory system is capable of distinguishing the slight changes in pitch at low frequency as compared to a higher frequency. So the main reason for using the Mel scale is to do feature extracting on the basis of the human auditory system [32]. The mathematical formula that is used to convert the original frequency into the Mel Scale is as follow:
M(f)=1125xln(1+f/700) |
Here f represents the actual frequency of the audio signal.
The method of zero paddings is used if the frames of audio signals are not in even numbers. The upcoming steps are applicable on every frame. 13 MFC coefficients are extracted from every frame. The time-domain signal B(n) after framing we get Bi(n), here n shows the range from 1 to 500 (we have 500 samples for 50 kHz frequency audio signal) [36]. When the complex discrete Fourier transform (DFT) is calculated so, we haveBi(k), here 'i' represents a number of the frame corresponding to the time domain.Zi(k) is then the frame power spectrum. In order to apply DFT, we have the following equation,
Bi(k)=N∑n=1Bi(n)h(n)e−j2πkn/N |
here h(n) represents the Hamming window function, while K shows the length of DFT. The frame's Bi(n) power spectral estimation based on the period gram is given by:
zi(k)=1N|Bi(k)|2 |
It is called spectral power estimation based on the period gram. Take the absolute value of the complex Fourier transform, then the square of the result. Generally, N point FFT is performed, and first C coefficients are kept. Calculate Mel filter bank, which is the group of 20 to 40 triangular filters. These triangular filters are applied to spectral power estimation. In order to get energies of the filter bank, we need to multiply every bank of the filter with power spectrum and then add coefficients [34].
Signal changes from positive to negative at a specific rate; that rate is called zero crossing rates (ZCR). ZCR is about the extent of no. of times in a frame that audio signal's amplitude passes over zero value as shown in the equation below,
ZCR=1/(T−1)∑(t=1)∧(T−1) |
Before computation of zero crossing rate, the waveform is shifted to distinguish between unvoiced audio signals and environment & noise. When the noise is small, it is very useful. Zero-crossing rate has a high frequency of the signal and lowers for voiced audio signals than unvoiced signals. It is a useful feature used to detect endpoints and to classify voiced and unvoiced signals. Zero-crossing rate doesn't notice noise which is its drawback [37].
The spectral roll-off is the frequency under which cutoff frequency, i.e., some percent of total energy, is contained. Spectral roll-off is used to differentiate between noise signals and harmonic signals. (Noise is above roll off and harmonic is below roll-off). Similarly, in our project, we have used spectral roll-off to differentiate between voiced and unvoiced signals (normal and pathological signals). The frequency below 85% of spectrum magnitude distribution is concentrated in the spectral roll-off. In digital signal processing, the spectral centroid is widely used [38]. The spectrum's gravity is the spectral centroid, i.e., and it shows the center of the mass of the spectrum. Spectral flux is the square of the difference between a successive spectral distribution and successive frames of signal. It measures the rate of change of the power spectrum of a signal. It can be calculated by comparing the power spectrum of one frame with the previous frame. In audio signal classification, short-term energy is widely used. It helps to differentiate between voiced signals and unvoiced signals. In a high-quality audio signal case, it is used to distinguish between audio and silence. It allows the calculating amount of energy in the audio signal at a specific instance in time.
The spectral complexity is quantified of a system by entropy information, i.e., spectral entropy. An uncertain system assumes a variable Y as a system state [39]. Through Fast Fourier Transform, signals of time series become power spectrum, while the entropy information of power spectrum is power spectral entropy.
In the section on material and method, now we are going to cover the method we used for the extraction of features from audio signals. The feature extraction and classification of then those extracted features have been carried out on MATLAB. The MATLAB version used to implement the proposed methodology is R2018b. The extraction of features is the method to convert the audio signals into the sequence of the vector of features; it carries the information of characteristics in audio signals. Then these vectors are used in the analysis for the different algorithms. It is difficult for those analysis algorithms are based on features extracted from window sources. The features which are based on the window show the description of the signal for a short time. MATLAB has the function of MFCC (Mel-frequency cepstral coefficients), which is used to extract pitch and 13 MFCC features from the imported dataset. After the process of feature extraction, the classification is carried out using the classification learner App in MATLAB. The classification learner app is easily available in the version of MATLAB, which is used to implement the methodology. No extra downloads were required overall. Classification learner is used to training the model of classifiers including; linear discriminant analysis, support vector machine, k nearest neighbor, decision tree, etc., using the classification learner application several other functions as an exploration of data, feature selection, specification of validation scheme, and results from the evaluation. In order to use the model for other data also the model can be exported to the workspace. The model can be trained using the classification learner app on MATLAB. The procedure is as follows:
1) Open MATLAB and perform the feature extraction on the dataset and save the extracted feature in an excel file.
2) Open classification leaner app in MATLAB, create a new session and upload your excel file (extracted features).
3) After successfully uploading the file, select the workspace variable 'FEATURES', and in response, you need to select 'LABELS'.
4) Make sure that all the features are selected. Here we are using 20 audio features, which include 13 MFCC (Mel-frequency cepstral coefficients), pitch, ZCR, energy Entropy, Rolloff, Spectral Centroid, Spectral Flux, and Energy.
5) Provide the validation scheme the value of cross-validation. In the proposed methodology, we are opting for 5-fold validation to avoid error-based results and to train the model to avoid overfitting.
6) Now to train your model on SVM, KNN, LDA, and other models, you need to click on the MODEL TYPE drop-down and select the particular model you want to train. For example: if you want to train your data on SVM so, select SVM, you will get multiple options to click on 'ALL SVM'.
7) The types of models are enabled according to the data we have as we are using the audio dataset, so we are going to apply the Decision tree and its kernel, the Naïve Bayes classifier, and its kernel SVM and its kernel, and the ensemble classifier and its kernel.
8) After the selection of the particular type of MODEL, you need to click on 'TRAIN'. It depends on the data that how much time it will take to be trained.
9) After the training of the model, you can export the trained model in the workspace so that it can be used for other data as well. Before exiting the MATLAB, make sure that you have saved it as the trained model on your PC. This trained data is helpful in the real-time system also.
The audio features were extracted from the audio database; i.e., 20 features were extracted. After the feature extraction, the classification of features was carried out using the classification learner app. Among the several classifiers, we have used four classifiers, and their kernels which are support vector machine classifier (SVM), Naïve Bayes classifier, decision tree classifier, and ensemble classifier, and the results that we have generated through the classification app can be observed in Table 1. The reported accuracy of the individual classifier is same for all the samples of laryngitis, cyst, non-fluency syndrome, and dysphonia.
Classifiers | Accuracy (%) | Misclassification rate (%) |
SVM | 93.18 | 6.82 |
Decision Tree | 100 | 0 |
Naïve bayes | 99.45 | 0.55 |
Ensemble | 51 | 49 |
Among all voice data, 5-fold cross-validation has been used for testing. After pre-processing, the voice signals are disrupted into the short stream by implementing a moderate windowing technique, hamming window. Every segment is processed separately. Hamming window is commonly used in narrow-band applications, e.g., telephone signal spectrum. Fast Fourier transform is computed for every segmented signal. Correlation of these small segmented signals is carried. De-correlation is performed to reconstruct these short segmented signals. The cross-correlating values are eliminated. A permutation is applied to find out the relation between these independent segmented signals. These segmented values are combined on the basis of relation. The features that we extract from each frame for classification. Combining these futures forming a support vector. Table 2 shows the reported accuracy of types of SVM, decision tree, Naïve Bayes, and ensemble, which we have used in our intercomparison approach.
Classifiers | Accuracy rate |
SVM kernel | |
Linear SVM | 100% |
Quadratic SVM | 100% |
Cubic SVM | 100% |
Fine Gaussian SVM | 72.7% |
Medium Gaussian SVM | 97.8% |
Coarse Gaussian SVM | 88.6% |
Naïve Bayes | |
Gaussian Naive Bayes | 99.8% |
Kernel Naive Bayes | 99.1% |
Decision Tree | |
Fine Tree | 100% |
Medium Tree | 100% |
Coarse Tree | 100% |
Ensemble | |
Bagged Tree | 100% |
Bootstrapping | 26.5% |
RUSBoosted Tree | 26.5% |
In Figure 3 shows the comparison between six kernels of the SVM classifier. Among all of them, linear, quadratic, and cubic SVM gave an accuracy of 100% with 0% misclassification rate while fine Gaussian, medium Gaussian, and coarse Gaussian gave 72.70, 97.80 and 88.60% accuracy rare respectively, with a misclassification rate of 27.3, 2.2 and 11.4%. In Figure 4 shows the reported accuracy of the Naïve Bayes classifier. This graph clearly shows that the reported accuracy rate is 99.80 and 99.10% when classified by Gaussian Naïve -Bayes and kernel Naïve Bayes, respectively, with the misclassification rate of 0.2 and 0.9%. In Figure 5 shows the accuracy of the decision tree classifier with its three kernels. Fine decision tree, medium decision tree, and coarse decision tree gave an accuracy rate of 100% with 0% misclassification rate when applied to our audio database. This shows that the proposed technique of MFCC gives the best accuracy results when classified by Decision Tree. In Figure 6 shows the reported accuracy from the Ensemble classifier and its kernels. Bagged trees, Boosted trees, and RUSBoosted trees gave the accuracy of 100, 26.50 and 26.50%, respectively, with the misclassification rate of 0, 73.5 and 73.5%.
There are several studies based on the detection of voice pathologies. Vocal cord paralysis primarily impacts the patient's speech and breathing and often contributes to a lack of vocal capacity as all cords are paralyzed. The diagnostic test in order to classify these language pathologies is rather intrusive for the patient and, thus, the research and production of approaches to machine learning has risen in recent years. Similar to our approach but with different features, SVM was used as a classifier for SVD, and the reported accuracy 92% [15]. In [21], while assessing voice pathologies, when 13 conventional MFCC features were extracted, the highest reported accuracy is 72% using the SVM as a machine learning classifier. In the proposed methodology, we have used the freely accessible database of voice pathology, Saarbrucken Voice database (SVD). This database is recorded by the institute of phonetics of Saarland University, and it contains normal and pathological voice signals. We worked on four different voice disorders, which are laryngitis, cyst, non-fluency syndrome, and dysphonia, available on the SVD database. According to the database, we have extracted features that were required to perform training and testing have been extracted. As the voice signals are continuous signals, so we have split the signals into the frame of 10ms because the audio signal is itself is so small, so to increase the frames, we split them in 10ms and considered the voice signals to be stationary statistical. Twenty features were extracted from each frame of the voice signal. The features which were assumed to be extracted are 13 MFCC and pitch, spectral flux, spectral centroid, spectral roll-off, spectral entropy, short term energy, and zero crossing rates (ZCR). After the extraction of the feature, we applied support vector machine, decision tree, Naïve Bayes, and ensemble classifiers on the database by classification learner app on MATLAB software. A classification learner app was used to classify the extracted features. We applied each of the classifiers to know the results of every classifier on the publicly available database. The main focus of the study is to extract the seven new features along with 13 conventional MFCC features and to make such a combination that could give the best 10 ms audio signal as compared to the results discussed in the literature review. The inter classifier comparison concluded that the decision tree and Naïve Bayes give the highest accuracies, which to the best of our knowledge, have not been used for the selected set of features. The accuracies that classifiers show that using these twenty features, SVM gave 93.18% accuracy, Naïve Bayes gave 99.45% accuracy, decision tree gave 100% accuracy, and Ensemble Classifier gave 51% accuracy. The intercomparison approach provides accuracies that will enable future researchers to opt for the best fit classifier. Hence it is concluded that machine-based detection of voice disorders can be a breakthrough with such promising results. In the future, researchers can opt for artificial neural networks, and deep learning classifies with the same set of features used in the proposed methodology.
There is no conflict of interest between authors.
[1] | P. Harar, J. B. Alonso-Hernandezy, J. Mekyska, Z. Galaz, R. Burget, Z. Smekal, Voice pathology detection using deep learning: a preliminary study, in 2017 international conference and workshop on bioinspired intelligence (IWOBI), (2017), 1-4. |
[2] |
M. Alhussein, G. Muhammad, Voice pathology detection using deep learning on mobile healthcare framework, IEEE Access, 6 (2018), 41034-41041. doi: 10.1109/ACCESS.2018.2856238
![]() |
[3] |
F. Teixeira, J. Fernandes, V. Guedes, A. Junior, J. P. Teixeira, Classification of control/pathologic subjects with support vector machines, Procedia Comput. Sci., 138 (2018), 272-279. doi: 10.1016/j.procs.2018.10.039
![]() |
[4] |
V. Guedes, A. Junior, J. Fernandes, F. Teixeira, J. P. Teixeira, Long short term memory on chronic laryngitis classification, Procedia Comput. Sci., 138 (2018), 250-257. doi: 10.1016/j.procs.2018.10.036
![]() |
[5] |
J. P. Teixeira, P. O. Fernandes, N. Alves, Vocal acoustic analysis-classification of dysphonic voices with artificial neural networks, Procedia Comput. Sci., 121 (2017), 19-26. doi: 10.1016/j.procs.2017.11.004
![]() |
[6] |
J. Kreiman, B. R. Gerratt, K. Precoda, Listener experience and perception of voice quality, J. Speech, Lang., Hear. Res., 33 (1990), 103-115. doi: 10.1044/jshr.3301.103
![]() |
[7] |
G. Muhammad, G. Altuwaijri, M. Alsulaiman, Z. Ali, T. A. Mesallam, M. Farahat, et al., Automatic voice pathology detection and classification using vocal tract area irregularity, Biocybern. Biomed. Eng., 36 (2016), 309-317. doi: 10.1016/j.bbe.2016.01.004
![]() |
[8] | N. Rezaei, A. Salehi, An introduction to speech sciences (acoustic analysis of speech), Iran. Rehabil. J., 4 (2006), 5-14. |
[9] | J. W. Lee, H. G. Kang, J. Y. Choi, Y. I. Son, An investigation of vocal tract characteristics for acoustic discrimination of pathological voices, BioMed Res. Int., 2013 (2013). |
[10] | US Department of Health and Human Services, NIDCD fact sheet: Speech and language developmental milestones, NIH Publication, 2010. |
[11] |
S. A. Syed, M. Rashid, S. Hussain, Meta-analysis of voice disorders databases and applied machine learning techniques, Math. Biosci. Eng., 17 (2020), 7958-7979. doi: 10.3934/mbe.2020404
![]() |
[12] | B. Boyanov, S. Hadjitodorov, Acoustic analysis of pathological voices. A voice analysis system for the screening of laryngeal diseases, IEEE Eng. Med. Biol. Mag., 16 (1997), 74-82. |
[13] | A. Zulfiqar, A. Muhammad, A. M. Enriquez, A speaker identification system using MFCC features with VQ technique, in 2009 Third International Symposium on Intelligent Information Technology Application, IEEE, 3 (2009), 115-118. |
[14] | A. Al-Nasheri, G. Muhammad, M. Alsulaiman, Z. Ali, K. H. Malki, T. A. Mesallam, et al., Voice pathology detection and classification using auto-correlation and entropy features in different frequency regions, IEEE Access, 6 (2017), 6961-6974. |
[15] | A. Al-Nasheri, G. Muhammad, M. Alsulaiman, Z. Ali, T. A. Mesallam, M. Farahat, et al., An investigation of multidimensional voice program parameters in three different databases for voice pathology detection and classification, J. Voice, 31 (2017), 113.e9-e18. |
[16] |
A. Al-Nasheri, G. Muhammad, M. Alsulaiman, Z. Ali, Investigation of voice pathology detection and classification on different frequency regions using correlation functions, J. Voice, 31 (2017), 3-15. doi: 10.1016/j.jvoice.2016.01.014
![]() |
[17] |
F. Teixeira, J. Fernandes, V. Guedes, A. Junior, J. P. Teixeira, Classification of control/pathologic subjects with support vector machines, Proced. Comput. Sci., 138 (2018), 272-279. doi: 10.1016/j.procs.2018.10.039
![]() |
[18] |
J. P. Teixeira, P. O. Fernandes, N. Alves, Vocal acoustic analysis-classification of dysphonic voices with artificial neural networks, Proced. Comput. Sci., 121 (2017), 19-26. doi: 10.1016/j.procs.2017.11.004
![]() |
[19] | E. S. Fonseca, R. C. Guido, S. B. Junior, H. Dezani, R. R. Gati, D. C. Pereira, Acoustic investigation of speech pathologies based on the discriminative paraconsistent machine (DPM), Biomed. Signal Process. Control, 55 (2020). |
[20] | Z. Ali, M. Alsulaiman, G. Muhammad, I. Elamvazuthi, A. Al-nasheri, T. A. Mesallam, et al., Intra-and inter-database study for Arabic, English, and German databases: do conventional speech features detect voice pathology?, J. Voice, 31 (2017), 386.e1-e8. |
[21] | S. Kadiri, P. Alku, Analysis and detection of pathological voice using glottal source features, IEEE J. Sel. Top. Signal Process., 14 (2019), 367-379. |
[22] | B. Woldert-Jokisz, Saarbruecken Voice Database, 2007. Available from: http://www.stimmdatenbank.coli.uni-saarland.de/help_en.php4. |
[23] | S. Huang, N. Cai, P. P. Pacheco, S. Narrandes, Y. Wang, W. Xu, Applications of support vector machine (SVM) learning in cancer genomics, Cancer Genomics-Proteomics, 15 (2018), 41-51. |
[24] | A. Shmilovici, Support vector machines, in Data Mining and Knowledge Discovery Handbook, Springer, Boston, MA, (2009), 231-247. |
[25] |
W. Zhang, F. Gao, An improvement to naive bayes for text classification, Procedia Eng., 15 (2011), 2160-2164. doi: 10.1016/j.proeng.2011.08.404
![]() |
[26] | C. C. Aggarwal, Data Mining: The Textbook, Springer, 2015. |
[27] | L. Toth, A. Kocsor, J. Csirik, On naive Bayes in speech recognition, Int. J. Appl. Math. Comput. Sci., 15 (2005), 287-294. |
[28] |
C. Kingsford, S. Salzberg, What are decision trees?, Nat. Biotechnol., 26 (2008), 1011-1013. doi: 10.1038/nbt0908-1011
![]() |
[29] | T. G. Dietterich, Ensemble methods in machine learning, in International workshop on multiple classifier systems, Springer, Berlin, Heidelberg, (2000), 1-15. |
[30] |
E. Bauer, R. Kohavi, An empirical comparison of voting classification algorithms: Bagging, boosting, and variants, Mach. Learn., 36 (1999), 105-139. doi: 10.1023/A:1007515423169
![]() |
[31] | R. Sharma, K. Hara, H. Hirayama, A machine learning and cross-validation approach for the discrimination of vegetation physiognomic types using satellite based multispectral and multitemporal data, Scientifica, 2017 (2017), 9806479. |
[32] | R. O. Duda, P. E. Hart, D. G. Stork, Pattern Classification, 2nd edition, Wiley-Interscience, USA, 2000. |
[33] | S. Memon, M. Lech, L. He, Using information theoretic vector quantization for inverted MFCC based speaker verification, in 2009 2nd International Conference on Computer, Control and Communication, IEEE, (2009), 1-5. |
[34] | M. Sahidullah, G. Saha, On the use of distributed dct in speaker identification, in 2009 Annual IEEE India Conference, IEEE, (2009), 1-4. |
[35] | Ö. Eskidere, A. Gürhanlı, Voice disorder classification based on multitaper mel frequency cepstral coefficients features, Comput. Math. Methods Med., 2015 (2015), 956249. |
[36] | P. Mahesha, D. Vinod, Classification of speech dysfluencies using speech parameterization techniques and multiclass SVM, in International Conference on Heterogeneous Networking for Quality, Reliability, Security and Robustness, Springer, Berlin, Heidelberg, (2013), 298-308. |
[37] | M. M. Oo, Comparative study of MFCC feature with different machine learning techniques in acoustic scene classification, Int. J. Res. Eng., 5 (2018), 439-444. |
[38] | A. Mehler, S. Sharoff, M. Santini, Genres on the Web: Computational Models and Empirical Studies, Springer Science & Business Media, 2010. |
[39] | K. Prahallad, Speech technology: A practical introduction, topic: Spectrogram, cepstrum and mel-frequency analysis, Carnegie Mellon Univ. Int. Inst. Inf. Technol. Hyderabad, Slide, 2011. |
1. | Sidra Abid Syed, Munaf Rashid, Samreen Hussain, Hira Zahid, Wen Si, Comparative Analysis of CNN and RNN for Voice Pathology Detection, 2021, 2021, 2314-6141, 1, 10.1155/2021/6635964 | |
2. | Ebenhaeser Otto Janse van Rensburg, Reinhardt A. Botha, Rossouw von Solms, Utility indicator for emotion detection in a speaker authentication system, 2022, 30, 2056-4961, 672, 10.1108/ICS-07-2021-0097 | |
3. | Asli Nur Omeroglu, Hussein M.A. Mohammed, Emin Argun Oral, Multi-modal voice pathology detection architecture based on deep and handcrafted feature fusion, 2022, 36, 22150986, 101148, 10.1016/j.jestch.2022.101148 | |
4. | Elmoundher Hadjaidji, Mohamed Cherif Amara Korba, Khaled Khelil, 2021, Detection of precancerous laryngeal leukoplakia using sub-band based cepstral, 978-1-6654-2821-7, 1, 10.1109/ICNAS53565.2021.9628992 | |
5. | Nadhirah Johari, Mazlina Mamat, Ali Chekima, 2021, Performance of Machine Learning Classifiers in Distress Keywords Recognition for Audio Surveillance Applications, 978-1-6654-2899-6, 1, 10.1109/IICAIET51634.2021.9573852 | |
6. | Lei Geng, Yan Liang, Hongfeng Shan, Zhitao Xiao, Wei Wang, Mei Wei, Pathological Voice Detection and Classification Based on Multimodal Transmission Network, 2022, 08921997, 10.1016/j.jvoice.2022.11.018 | |
7. | Vadi Su Yılmaz, Kemal Efe Eseller, Ozgur Aslan, Emin Bayraktar, Classification of Different Recycled Rubber-Epoxy Composite Based on Their Hardness Using Laser-Induced Breakdown Spectroscopy (LIBS) with Comparison Machine Learning Algorithms, 2023, 8, 2411-5134, 54, 10.3390/inventions8020054 | |
8. | Erdal Özbay, Feyza Altunbey Özbay, Nima Khodadadi, Farhad Soleimanian Gharehchopogh, Seyedali Mirjalili, Multifeature Fusion Method with Metaheuristic Optimization for Automated Voice Pathology Detection, 2024, 08921997, 10.1016/j.jvoice.2024.08.018 | |
9. | Ioanna Miliaresi, Aggelos Pikrakis, Kyriakos Poutos, 2022, A Deep Multimodal Voice Pathology Classifier with Electroglottographic Signal Processing Capabilities, 978-1-6654-8158-8, 109, 10.1109/ICFSP55781.2022.9924745 | |
10. | Sharal Coelho, Hosahalli Lakshmaiah Shashirekha, 2023, Chapter 45, 978-3-031-48308-0, 565, 10.1007/978-3-031-48309-7_45 | |
11. | Laura Verde, Fiammetta Marulli, Roberta De Fazio, Lelio Campanile, Stefano Marrone, HEAR set: A ligHtwEight acoustic paRameters set to assess mental health from voice analysis, 2024, 182, 00104825, 109021, 10.1016/j.compbiomed.2024.109021 | |
12. | Manjit Singh Sidhu, Nur Atiqah Abdul Latib, Kirandeep Kaur Sidhu, MFCC in audio signal processing for voice disorder: a review, 2024, 1573-7721, 10.1007/s11042-024-19253-1 | |
13. | Ioanna Miliaresi, Aggelos Pikrakis, A Modular Deep Learning Architecture for Voice Pathology Classification, 2023, 11, 2169-3536, 80465, 10.1109/ACCESS.2023.3300795 | |
14. | Ebenhaeser Janse van Rensburg, Reinhardt A. Botha, Bertram Haskins, 2023, Identifying Duress Through Voice During Speaker Authentication, 979-8-3503-2781-6, 1, 10.1109/ICECET58911.2023.10389204 | |
15. | Jaycee M. Kaufman, Anirudh Thommandram, Yan Fossat, Acoustic Analysis and Prediction of Type 2 Diabetes Mellitus Using Smartphone-Recorded Voice Segments, 2023, 1, 29497612, 534, 10.1016/j.mcpdig.2023.08.005 | |
16. | Yuwei Yang, Youhua Lin, Chang Liu, Zhi Li, Kun Mei, Jingyue Wu, Yi Xiao, Jun Zhang, 2023, Speech Feature-Based Machine Learning Model and Smart Devices for Stroke Early Recognition, 979-8-3503-0017-8, 354, 10.1109/ICARM58088.2023.10218802 | |
17. | Shao-Hsuan Lee, Li-Chun Hsieh, Jia Long Shyu, Lun-Ping Hung, Guo-She Lee, Assessment of Vocal Characteristics Associated With Chronic Rhinosinusitis Using Machine-Learning Techniques Based on Long-Term Average Spectrum of Voice, 2024, 08921997, 10.1016/j.jvoice.2024.10.004 | |
18. | N. F. S. Md Sazihan, N. M. Abdul Latiff, N. N. N. Abd Malik, S. Mashohor, K. Alaghbari, F. T. AL-Dhief, 2024, The Effect of Balanced and Imbalanced Dataset on Voice Pathology Detection Using Online Sequential Extreme Learning Machine (OSELM), 979-8-3503-5350-1, 1, 10.1109/ATNT61688.2024.10719208 | |
19. | Anitha Sankaran, Lakshmi Sutha Kumar, Advances in Automated Voice Pathology Detection: A Comprehensive Review of Speech Signal Analysis Techniques, 2024, 12, 2169-3536, 181127, 10.1109/ACCESS.2024.3508884 | |
20. | J. Jayanthi, V. Upendran, Raga Recognition of Indian Classical Music using Meerkat Optimization Based MFCC and Fine Tuned BILSTM-XGBOOST, 2025, 0278-081X, 10.1007/s00034-025-02999-w |
Classifiers | Accuracy (%) | Misclassification rate (%) |
SVM | 93.18 | 6.82 |
Decision Tree | 100 | 0 |
Naïve bayes | 99.45 | 0.55 |
Ensemble | 51 | 49 |
Classifiers | Accuracy rate |
SVM kernel | |
Linear SVM | 100% |
Quadratic SVM | 100% |
Cubic SVM | 100% |
Fine Gaussian SVM | 72.7% |
Medium Gaussian SVM | 97.8% |
Coarse Gaussian SVM | 88.6% |
Naïve Bayes | |
Gaussian Naive Bayes | 99.8% |
Kernel Naive Bayes | 99.1% |
Decision Tree | |
Fine Tree | 100% |
Medium Tree | 100% |
Coarse Tree | 100% |
Ensemble | |
Bagged Tree | 100% |
Bootstrapping | 26.5% |
RUSBoosted Tree | 26.5% |
Classifiers | Accuracy (%) | Misclassification rate (%) |
SVM | 93.18 | 6.82 |
Decision Tree | 100 | 0 |
Naïve bayes | 99.45 | 0.55 |
Ensemble | 51 | 49 |
Classifiers | Accuracy rate |
SVM kernel | |
Linear SVM | 100% |
Quadratic SVM | 100% |
Cubic SVM | 100% |
Fine Gaussian SVM | 72.7% |
Medium Gaussian SVM | 97.8% |
Coarse Gaussian SVM | 88.6% |
Naïve Bayes | |
Gaussian Naive Bayes | 99.8% |
Kernel Naive Bayes | 99.1% |
Decision Tree | |
Fine Tree | 100% |
Medium Tree | 100% |
Coarse Tree | 100% |
Ensemble | |
Bagged Tree | 100% |
Bootstrapping | 26.5% |
RUSBoosted Tree | 26.5% |