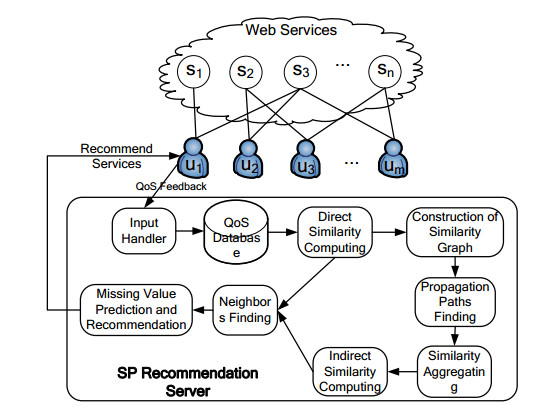
Citation: Kai Su, Xuan Zhang, Qing Liu, Bin Xiao. Strategies of similarity propagation in web service recommender systems[J]. Mathematical Biosciences and Engineering, 2021, 18(1): 530-550. doi: 10.3934/mbe.2021029
[1] | Hao Song, Xiaonong Lu, Xu Zhang, Xiaoan Tang, Qiang Zhang . A HRGO approach for resilience enhancement service composition and optimal selection in cloud manufacturing. Mathematical Biosciences and Engineering, 2020, 17(6): 6838-6872. doi: 10.3934/mbe.2020355 |
[2] | Hossein Habibi, Abbas Rasoolzadegan, Amir Mashmool, Shahab S. Band, Anthony Theodore Chronopoulos, Amir Mosavi . SaaSRec+: a new context-aware recommendation method for SaaS services. Mathematical Biosciences and Engineering, 2022, 19(2): 1471-1495. doi: 10.3934/mbe.2022068 |
[3] | Xin Liu, Yingyuan Xiao, Xu Jiao, Wenguang Zheng, Zihao Ling . A novel Kalman Filter based shilling attack detection algorithm. Mathematical Biosciences and Engineering, 2020, 17(2): 1558-1577. doi: 10.3934/mbe.2020081 |
[4] | Wenjing Lv, Jue Chen, Songlin Cheng, Xihe Qiu, Dongmei Li . QoS-driven resource allocation in fog radio access network: A VR service perspective. Mathematical Biosciences and Engineering, 2024, 21(1): 1573-1589. doi: 10.3934/mbe.2024068 |
[5] | Liang Xiao, Hao Zhou, John Fox . Towards a systematic approach for argumentation, recommendation, and explanation in clinical decision support. Mathematical Biosciences and Engineering, 2022, 19(10): 10445-10473. doi: 10.3934/mbe.2022489 |
[6] | Abhishek Savaliya, Rutvij H. Jhaveri, Qin Xin, Saad Alqithami, Sagar Ramani, Tariq Ahamed Ahanger . Securing industrial communication with software-defined networking. Mathematical Biosciences and Engineering, 2021, 18(6): 8298-8313. doi: 10.3934/mbe.2021411 |
[7] | Meili Tang, Qian Pan, Yurong Qian, Yuan Tian, Najla Al-Nabhan, Xin Wang . Parallel label propagation algorithm based on weight and random walk. Mathematical Biosciences and Engineering, 2021, 18(2): 1609-1628. doi: 10.3934/mbe.2021083 |
[8] | Abdullah Lakhan, Mazhar Ali Dootio, Ali Hassan Sodhro, Sandeep Pirbhulal, Tor Morten Groenli, Muhammad Saddam Khokhar, Lei Wang . Cost-efficient service selection and execution and blockchain-enabled serverless network for internet of medical things. Mathematical Biosciences and Engineering, 2021, 18(6): 7344-7362. doi: 10.3934/mbe.2021363 |
[9] | Chen Shao, Yue zhong yi Sun . Shilling attack detection for collaborative recommender systems: a gradient boosting method. Mathematical Biosciences and Engineering, 2022, 19(7): 7248-7271. doi: 10.3934/mbe.2022342 |
[10] | Xiang Nan, Kayo kanato . Role of information security-based tourism management system in the intelligent recommendation of tourism resources. Mathematical Biosciences and Engineering, 2021, 18(6): 7955-7964. doi: 10.3934/mbe.2021394 |
With the rapid development of Cloud Computing and Service-Oriented Computing technologies, a large number of web services appear on the network. When customers need to choose the best service to build service-oriented applications, quality of Service (QoS) becomes their primary concern to distinguish functionally equivalent services. Due to the influence of objective environment like physical location, network conditions and other factors, different users will experience different QoS (e.g., reliability, throughput, response time, etc.) on the same web service. Therefore, personalized web service QoS prediction and recommendation turn to be a hot issue in service computing [1,2,3,4].
In recent years, Collaborative Filtering (CF) has attracted much attention and becomes the most popular technology to realize a web service recommender system [5,6,7,8,9,10]. In General, the CF based approaches can be divided into two categories: Memory-based CF [5,6,7,11,12,13,14] and Model-based CF [8,9,10] approaches. Memory-based CF methods mainly mine the neighbor relation between users or services contained in the historical QoS data contributed by customers and utilize the neighbor information to predict the unknown service QoS for active users. Memory-based CF approaches are based on the assumption that the users who experienced similar QoS on past commonly-invoked services will have similar experience on other services. Obviously, the accuracy of Memory-based CF highly relies on the accuracy of similarity calculation. In real-world situation, most recommender systems commonly suffer from the problem of lacking of users' feedback data, which is known as the data sparse problem [15]. Data sparse problem highly affects the accuracy of similarities calculation and the performance of recommender system. In the web service recommender system, most users only invoked a small set of web services, causing to the data sparse problem of the user-service QoS matrix [10]. Thus the common set between two users or two services may not be found, and the similarity of users or services are failed to be computed. In another case, even the common set with few elements are discovered, the similarity may be overestimated or underestimated due to the amount of common items is small. Most existing literatures ignore the problem of inaccurate similarity calculation and thus fail to obtain high prediction accuracy under a sparse data condition.
In this paper, we propose two similarity propagation (SP) strategies to overcome the inaccurate similarity calculation problem in memory-based CF for web service recommendation. The SP strategies are inspired by the idea of trust propagation in Social Network (SN) [16,17,18]. In a SN, the trust of two indirect users who have not direct interaction can be inferred by a third user. We believe that the similarities between users or services could also be transitive, which means that "if A and B are similar, and B and C are similar, then A and C will be similar to some extent". Since the neighbor set of users or services is hard to find under a sparse user-service QoS data condition, it is crucial to discover a set of indirect similar users or services for prediction by similarity propagation. In our approach, we firstly propose an extended Pearson Correlation Coefficient (PCC) to compute the direct similarity between users or services and then construct an undirected similarity graph based on the computed direct similarities. Secondly, the similarity propagation paths are discovered for each pair of users or services on the graph. Thirdly, the similarity of each path is evaluated and the indirect similarity is computed by agammaegating the similarities of different paths. Finally, we integrate the direct similarity and indirect similarity to discover a set of similar users or similar services to predict the missing QoS value. The main contributions of this research work can be summarized as three-fold:
(1) We study the transitivity of similarity which is generally ignored by the existing literatures to our best knowledge. Two similarity propagation strategies are proposed to compute the indirect similarity between users or web services: Min-max similarity propagation among shortest paths (SPaS) and Min-max similarity propagation among all paths (SPaA).
(2) We design a Flyod based algorithm to implement the strategies of SPaS and SPaA. The proposed algorithm is very simple and effective.
(3) We conduct several experiments based on real-world dataset to verify the effectiveness of our similarity propagation strategies.
The rest of this paper is organized as follows. Section 2 reviews the related work. Section 3 describes our similarity propagation strategies. Section 4 conducts several experiments to evaluate the approach by real-world datasets. Section 5 is the conclusion of this paper.
In recent years, many CF based methods are developed to solve the web service recommendation problems, in which the memory-based CF plays a crucial role. The memory-based CF approaches mainly use the information of a group of similar users or similar services to predict the unknown QoS of target services. Zheng et al. [11] proposed a CF based approach called WSRec for predicting QoS values of web services and making web service recommendation by taking advantages of past usage experiences of users. In WSRec, the similarities between users or services are calculated by PCC method and TopK similar users combined with TopK similar services are found to predict the unknown QoS. Chen et al. [12] proposed an improved memory-based CF method, in which the physical location of users is integrated in the process of neighbor finding and QoS values prediction. They insisted that the closely located users would experience similar QoS and then used PCC to compute the similarities between different regions. Fletcher et al. [13] proposed a memory-based approach, where the satisfaction of users' personalized preferences on nonfunctional attributes were took into consideration by extending the tradition PCC method. Ma et al. [14] proposed a PCC and linear regression based approach HAPA to make highly accurate prediction for unknown QoS values. They considered that the similarity derived from the objective QoS data should not be directly used to make prediction. Based on the observation that a high similarity will hardly fluctuates with the growing items of the common set, they designed a linear regression model to make prediction. The above mentioned memory-based approaches commonly utilize the traditional or extended PCC to compute the similarities between different users or web services. However, they ignore the inaccuracy problem of PCC under a sparse QoS data condition. Idrissi et al. [15] reviewed the recent research works on alleviating the sparsity issues in recommender systems and analyzed several popular similarity measures. In this paper, the global similarity measures were proposed to measure the similarities between users who have not many direct relations. However, the authors did not depict how to find the global neighbors and calculate the global similarities between them.
As referred before, the memory-based method mainly relies on the neighbor information, where as the model-based method mainly rely on the latent feature information included in the historical QoS data. Each method may ignore the valuable information contained in the QoS data. To make fully utilization of this two type of information, several integrations of memory-based and model-based approaches are recently proposed. Yin et al. [19] proposed a service neighborhood enhanced probabilistic matrix factorization (MF) model where the feature vectors of the similar services were integrated into the learning process. Qi et al. [20] made use of the users' network locations to find the network neighbors. Then, the users' network neighbors and the services' neighbors were both integrated into the MF model for QoS prediction. In reference [21], the authors proposed an improved similarity computation method to discover a set of similar users and similar services. Then the deep latent features of neighbors were learned by a convolutional neural network model and integrated into the learning process of matrix factorization. Ryu et al. [22] argued that users located in same region may share similar QoS experiences and web services located in same region may have similar QoS. Based on the assumption, they described a location-based MF approach where both of the locations of users and web services are considered into similarities computation to overcome the cold start problem. Similarly, Zhu et al. [23] proposed a location-aware low-rank MF approach to realize a web service recommender system, in which a similarity-maintaining privacy preservation strategy was designed to protect the users' privacy. Nevertheless, the similarity computation method of these research works are also vulnerable to the sparse QoS data, since the direct common set among users or services are hard to find.
To address the inaccurate similarity calculation problem, we propose a similarity propagation approach to fully mine the neighbor information included in the QoS data. Since the direct interactions between users or services are limited, it makes sense to mine the implicit indirect relations of users or services. Propagation is recently studied to measure the indirect trust between two users who have not direct interactions in the Social Network [16,17,18]. In our previous work [24], we propose an indirect similarity computation approach in which only one intermediate node on the transitive path is considered. However, this strategy may ignore much valuable information to obtain more accurate similarities. In this paper, we propose two strategies of similarity propagation to compute the indirect similarity between users or services who are not directly connected. Then the indirect similarity and direct similarity are integrated to find the neighbors to make prediction. The extensive experiments conducted in Section 4 demonstrate that the proposed similarity propagation strategies can outstandingly improve the accuracy of memory-based CF approaches.
In this section, we will introduce the proposed SP strategies. Firstly, the SP service recommendation framework is given. Then, we give a motivating example to interpret why the traditional PCC may obtain inaccurate similarities under a sparse data condition. Moreover, the details of direct similarity computation and indirect similarity computation based on the similarity propagation strategies are introduced. Finally, we analyze the time complexity of our proposed approaches.
In recent years, several web service recommendation frameworks like WSRec [11], NIMF [25] are proposed to collect the QoS data submitted by customers and predict the missing QoS values for customers. However, most of them ignore the inaccurate similarity computing problem under the sparse QoS data condition. To tackle this problem, we propose a SP based service recommendation framework as shown in Figure 1, which mainly includes the following procedures.
(1) Users invoke the remote web services on the Internet, where the edges between users and services indicate the invocation records. Then users could submit the observed QoS data of the services to the SP recommendation server. In Figure 1, two users who invoked common services are considered as having direct interaction relation. E.g., both of u1 and u2 invoked s3, so they have direct interaction with each other. Similarly, two services which were invoked by common users are considered as having direct interaction relation.
(2) The Input Handler module process the QoS feedback data submitted by users and the processed data are stored in the QoS Database. The Direct Similarity Computing module compute the direct similarity between users or services by an extended PCC method.
(3) The similarity graph is constructed according to the direct interactions between users or services in the Construction of Similarity Graph module. Then, the similarity propagation paths on the graph are found by the Propagation Paths Finding module.
(4) The similarity along each propagation path is measured in the Similarity Agammaegating module. Then the indirect similarity among users or services are evaluated by agammaegating the similarities of different paths in the Indirect Similarity Computing module.
(5) The module of Missing Value Prediction and Recommendation make personalized web service QoS prediction for users and recommend the best services to users.
Given a web service recommender system containing m service users and n services, the user invocations data can be denoted as a m×n QoS matrix R, where the entry Rij in R represents the QoS value of web service sj experienced by user ui. If there is no invocation record of service sj by user ui, then Rij=null. A simple instance of user-service QoS matrix is shown in Table 1, in which each entry is the value of response time of a service experienced by a user. The goal of the service recommender system is to predict the missing entries in the matrix by using the available data.
s1 | s2 | s3 | s4 | s5 | |
u1 | 0.5 | 0.4 | 0.3 | ||
u2 | 0.8 | 0.7 | 0.7 | ||
u3 | 0.3 | 0.8 | |||
u4 | 0.6 | 0.2 | 0.1 | 0.5 | |
u5 | 0.7 | 0.2 | 0.6 | 0.3 |
Most existing memory-based approaches [11,12,13,14] employ PCC method to compute the similarities between users or services. PCC mainly uses the data of the common set between two users or services to compute the similarity. E.g., user u2 and u4 commonly invoked service s1, s3 and s5, so the common set including s1, s3 and s5 is used to compute the PCC similarity between u2 and u4. However, since the amount of users and services are quite large, most users only have invocation records on a small set of services, causing to the data sparse problem. As we know, many recommender systems face the challenge of data sparse problem. E.g., the density of two famous open datasets of Netflix and Movielens in movie recommender system are both below 5%. In this case, the similarity may not be accurately calculated as the situation listed below:
(1) Common set is not existed between two users or services; thus the similarity cannot be computed by PCC method. E.g., u2 and u3 have not commonly-invoked services, so their similarity cannot be evaluated.
(2) The common set with few items is found, the similarity may be overestimated. E.g., only one service s3 is commonly invoked by u1 and u2, the similarity between them is overestimated as 1 by PCC method.
(3) The common set with few items is found, the similarity may be underestimated who are actually similar but accidentally have dissimilar QoS experience on several co-invoked Web services. E.g, u1 and u3 have commonly invoked s2 and s4, due to the dissimilar QoS experience on service s4, their similarity is underestimated as −1.
To tackle the above problem, we propose a similarity propagation approach to accurately compute the similarities. In our approach, not only the direct interaction information between users or services is employed, but also the indirect relations inferred by the propagation paths are utilized. E.g., the similarity between u2 and u3 can be inferred from the path of u2→u5→u3, since u2 have direct interaction with u5 and u5 have direct interaction with u3 either. To discover the indirect relations among users or services is crucial for fully mining the neighbor information in the sparse QoS data. The main point of the similarity propagation is how to find the propagation paths and how to agammaegate the similarities on different paths. The details are presented in the following sections.
PCC is usually utilized to compute the similarities between different items in many recommender systems, because of its high accuracy and simple calculation [11]. The similarity between two users is calculated based on their commonly-invoked services as follows:
Sim(u,v)=∑i∈Suv(Rui−¯Ru)(Rvi−¯Rv)√∑i∈Suv(Rui−¯Ru)2√∑i∈Suv(Rvi−¯Rv)2 | (1) |
where Sim(u,v) is the similarity between user u and v. Suv=Su∩Sv is a collection of web services that are invoked by both user u and user v. Rui is the QoS value of service i experienced by user u. ¯Ru denote the average QoS values of all the services experienced by user u. ¯Rv denote the average QoS values of all the services experienced by user v. Sim(u,v) is in the interval of [−1, 1], where a larger value indicates a higher user similarity.
The similarity between two services is computed based on the users who invoked both of them:
Sim(i,j)=∑u∈Uij(Rui−¯Ri)(Ruj−¯Rj)√∑u∈Uij(Rui−¯Ri)2√∑u∈Uij(Ruj−¯Rj)2 | (2) |
where Sim(i,j) is the similarity between service i and j. Uij=Ui∩Uj is a collection of users that invoked both service i and service j. Rui is the QoS value of web service i experienced by user u. ¯Ri represents the average QoS value of service i experienced by all the users. Sim(i,j) is also in the interval of [−1, 1], where a larger value means a higher service similarity.
As referred in section 3.2, PCC may overestimate or underestimate the similarities under a sparse data condition. To address this problem, we extend the traditional PCC method by employing the sigmoid function as a damping factor related with the amount of common items. The direct similarity between two users is computed as follows:
SimD(u,v)={11+e−|Suv|Sim(u,v)|Suv|⩾20 |Suv|<2 | (3) |
In this equation, the similarity is related to the size of the common set. A larger common set will obtain a stronger similarity. This method can overcome the problem of underestimate or overestimate caused by the lack of common items to some extent. If only one commonly invoked service is found, the traditional PCC will obtain 1 as the similarity which is meaningless. In the extended PCC, the similarity between two users who only commonly invoked one service is equal to 0.
In the same way, the direct similarity between two web services can be computed as follows:
SimD(i,j)={11+e−|Uij|Sim(i,j)|Uij|⩾20 |Uij|<2 | (4) |
As referred in section 3.2, PCC may not achieve accurate similarity under a sparse data condition. In this section, we present the indirect similarity computing method.
Suppose the user similarity matrix derived from the QoS data is denoted as SU, where each entry SUuv denotes the direct similarity between user u and v obtained from Eq (3). The service similarity matrix derived from the QoS data is denoted as SS, where each entry SSij denotes the direct similarity between web service i and web service j obtained from Eq (4). Table 2 give a simple instance of user similarity matrix, in which SU12=0.5 means that the direct similarity between u1 and u2 is equal to 0.5, SU23=0 means that u2 and u3 have not direct experience. The users similarity matrix can be considered as an adjacent matrix to construct the user similarity graph, which is shown in Figure 2.
u1 | u2 | u3 | u4 | u5 | u6 | u7 | |
u1 | 0 | 0.5 | 0 | 0.1 | 0 | 0.6 | 0.3 |
u2 | 0.5 | 0 | 0 | 0.4 | 0 | 0 | 0 |
u3 | 0 | 0 | 0 | 0 | 0 | 0.2 | 0.6 |
u4 | 0.1 | 0.4 | 0 | 0 | 0.4 | 0 | 0 |
u5 | 0 | 0 | 0 | 0.4 | 0 | 0.2 | 0.5 |
u6 | 0.6 | 0 | 0.2 | 0 | 0.2 | 0 | 0 |
u7 | 0.3 | 0 | 0.6 | 0 | 0.5 | 0 | 0 |
The user similarity graph is an undirected weighted graph where the nodes indicate the users and the edges indicate the direct interactions between users. The weight on each edge represents the direct similarity of two users who are linked by it. For two users who are not directly connected such as u1 and u5, their indirect similarity can be inferred by other users. E.g., the indirect similarity of u1 and u5 can be inferred from the propagation paths like u1→u6→u5, u1→u7→u5, u1→u2→u4→u5, u1→u6→u3→u7→u5 and so on. The key point of indirect similarity computation includes two step: finding propagation paths and agammaegating the similarities on different paths.
In this paper, we study two strategies to compute the indirect similarity: Min-max similarity propagation among shortest paths (SPaS) and Min-max similarity propagation among all paths (SPaA). In SPaS, only the shortest propagation paths among users or services are considered, since the strength of similarity may damp with the increase of the transitive nodes and the shortest path could obtain a stronger and more convincing similarity [26]. In SPaA, all the propagation paths among users or services are took into consideration to avoid ignoring the valuable neighbor information contained in the QoS data. Both of SPaS and SPaA employ the min-max agammaegation method [27] to measure the similarity of a path and agammaegate the similarities of different paths to obtain the final indirect similarity. The min-max agammaegation method selects the minimum similarity value along the propagation path as the strength of this path. This approach makes sense to some extent, because the longer the path is, the lower the strength is likely to be. If multiple propagation paths have been searched out, it is reasonable to select the strongest propagation path as the optimal path.
Suppose that the user similarity graph GU(U,E,SimD) is already obtained from the QoS data, where U denotes all the users, E denotes all the edges among users, SimD denotes the direct similarities among users. In SPaA, the strength of a propagation path from source user u to target user v is measured as:
Str(Pk(u→v))=min(a,b)∈E(u→v){SimD(a,b)} | (5) |
where Pk(u→v) is the k th propagation path from user u to v. Str(Pk(u→v)) is the strength of Pk(u→v). E(u→v) is a set of edges along the path from u to v. (a,b) is an edge in E(u→v). E.g., if there is a propagation path from u to v : u→a→b→v, then E(u→v)={(u,a),(a,b),(b,v)}.
The indirect similarity between user u and user v is computed by agammaegating all the paths between them as follows:
SimI(u,v)=maxk∈P{Str(Pk(u→v))} | (6) |
where SimI(u,v) is the indirect similarity between user u and user v. P is a set of paths between u and v by searching on the user similarity graph GU. The method of SPaS is similar with SPaA except for their candidate propagation path sets. SPaS only search the shortest path from source user u to target user v and select the optimal shortest path with maximum similarity to obtain the final indirect similarity. It is worth noting that the shortest propagation path needs to include at least one intermediate node.
Similarly, suppose that the service similarity graph GS(S,E,SimD) is already obtained from the QoS data, where S denotes all the services, E denotes all the edges among services, SimD denotes the direct similarities among services. In SPaA, the strength of a propagation path from source service i to target service j is measured as:
Str(Pk(i→j))=min(a,b)∈E(i→j){SimD(a,b)} | (7) |
where Pk(i→j) is the k th propagation path from service i to j. Str(Pk(i→j)) is the strength of Pk(i→j). E(i→j) is a set of edges along the path from i to j. (a,b) is an edge in E(i→j).
The indirect similarity between service i and service j is calculated by agammaegating all the paths between them as follows:
SimI(i,j)=maxk∈P{Str(Pk(i→j))} | (8) |
where SimI(i,j) is the indirect similarity between service i and j. P is a set of paths between service i and j by searching on the service similarity graph GS. In the same way, the method of SPaS is similar with SPaA except for their candidate propagation path sets. SPaS only search the shortest path from source service i to target service j and select the optimal shortest path with maximum similarity to obtain the final indirect similarity.
In this paper, we design a Flyod based algorithm to implement the strategies of SPaS and SPaA. Floyd algorithm is a well-known dynamic programming based method to solve the multi-source shortest path searching problem [28]. Table 3 shows the details of our algorithm.
Algorithm: SPaA (min-max similarity propagation among all paths) | Algorithm: SPaS (min-max similarity propagation among shortest paths) |
Input: user direct similarity matrix SimD, the number of users m, distance matrix L, where each entry Lij denotes the distance between node i and j Output: user indirect similarity matrix SimI, where SimIij denotes the indirect similarity between user i and j 1 SimI=SimD; 2 For each entry Lij in L do 3 If SimIij=0 then Lij=0, else Lij=1; 4 End for 5 For (k=1;k⩽m;k++) do 6 For (i=1;i⩽m;i++) do 7 For (j=1;j⩽m;j++) do 8 If (k≠i&&k≠j&&i≠j) then 9 If ((Lik+Lkj)⩽6) then 10 If (SimIij<min{SimIik,SimIkj}) then 11 SimIij=min{SimIik,SimIkj}; 12 Lij=Lik+Lkj; 13 End if 14 End if 15 End if 16 End for 17 End for 18 End for 19 Return SimI |
Input: user direct similarity matrix SimD, the number of users m, distance matrix L, where each entry Lij denotes the distance between node i and j Output: user indirect similarity matrix SimI, where SimIij denotes the indirect similarity between user i and j 1 Each entry SimIij in SimI is initialized with the indirect similarity propagated from only one intermediate node; 2 For each entry Lij in L do 3 If SimIij=0 then Lij=0, else Lij=2; 4 End for 5 For (k=1;k⩽m;k++) do 6 For (i=1;i⩽m;i++) do 7 For (j=1;j⩽m;j++) do 8 If (k≠i&&k≠j&&i≠j) then 9 If (SimIij<min{SimIik,SimIkj}) then 10 If ((Lik+Lkj)⩽Lij P Lij==0) then 11 SimIij=min{SimIik,SimIkj}; 12 Lij=Lik+Lkj; 13 End if 14 End if 15 End if 16 End for 17 End for 18 End for 19 Return SimI |
In the 9th step in SPaA, the distance between two nodes can't be more than 6 to avoid too long walks on the similarity graph, according to the principle of "six degrees of separation" in Social Network [29]. In the 1st step in SPaS, the indirect similarity matrix SimI is initialized with the indirect similarity propagated from one intermediate node since the shortest path needs to include at least one intermediate node. The indirect similarities among services can also be computed by replacing the input parameter "user direct similarity matrix" with "service direct similarity matrix".
Since the natures of different datasets may be different, a similarity weight α(0⩽α⩽1) is designed to integrate the direct similarity and indirect similarity in evaluating the similarities among users or services.
The integrated similarity between user u and user v is defined as:
sim′(u,v)=αuvsimD(u,v)+(1−αuv)simI(u,v) | (9) |
where the similarity weight αuv is computed as:
αuv=|Su∩Sv||Su∪Sv| | (10) |
where Su∩Sv is the number of services that both u and v have invoked. Su∪Sv is the number of services that have been invoked by either u or v. Formula (10) means that if u and v rarely invoked same services, the similarity weight αuv will reduce the proportion of direct similarity and increase the proportion of indirect similarity. Since the value of αuv is between [0, 1], and both simD(u,v) and simI(u,v) are between [−1, 1], the value of sim′(u,v) is within the range of [−1, 1].
Similarly, the integrated similarity between service i and j is defined as:
sim′(i,j)=αijsimD(i,j)+(1−αij)simI(i,j) | (11) |
where the weight αij is computed as:
αij=|Ui∩Uj||Ui∪Uj| | (12) |
where Ui∩Uj denotes the number of users who have invoked both service i and service j, Ui∪Uj denotes the number of users who have either invoked service i or j. The value of sim′(i,j) is also within the range of [−1, 1].
After similarity computation, a set of TopK similar users or a set of similar services can be found to predict the missing QoS values.
In user-based PCC methods (UPCC), TopK similar users are found to make QoS value prediction. To distinguish with the traditional method, UPCC with similarity propagation by SPaS and SPaA are named UPCC-SPaS and UPCC-SPaA, respectively.
Rui=¯Ru+∑v∈T(u)Sim′(u,v)(Rvi−¯Rv)∑v∈T(u)Sim′(u,v) | (13) |
where Rui is the predicted QoS value, Sim′(u,v) is the integrated similarity between user u and user v, User v is the neighbor of user u, T(u) is a set of the TopK similar users of user u, Rvi is the QoS value of service i experienced by user v.
In item-based PCC methods (IPCC), TopK similar services are found to make QoS value prediction. Similarly, to distinguish with the traditional method, IPCC with similarity propagation by SPaS and SPaA are named IPCC-SPaS and IPCC-SPaA, respectively.
Rui=¯Ri+∑j∈T(i)Sim′(i,j)(Ruj−¯Rj)∑j∈T(i)Sim′(i,j) | (14) |
where Rui is the predicted QoS value, Sim′(i,j) is the integrated similarity between service i and j, Service i is the neighbor of service j, T(i) is a set of the TopK similar services of service i, Ruj is the QoS of service j observed by user u.
After the missing QoS values be predicted, the optimal services with the highest QoS value can be recommended to the active users.
The time complexity of our approach includes three parts: direct similarity computation, indirect similarity computation based on similarity propagation and QoS value prediction based on the TopK nearest neighbors.
In UPCC-SPaA or UPCC-SPaS, the complexity of direct similarity computation for one user pair is O(n). Since we need to compute the similarities of m(m−1)/2 user pairs for all the users, the complexity of direct similarity computation is O(m2n). In indirect similarity computation, the complexity of similarity propagation based on Floyd algorithm is O(m3). To choose the TopK similar users of the active user, O(mlogm) is need to sort the similarities between the active user and the other users. In addition, we need O(TopK∗n) to make TopK prediction for one active user. Hence, the complexity of predicting the missing values for one active user is O(mlogm+TopK∗n). The complexity of predicting the missing values for all the users is thus O(m2logm+TopK∗mn). Therefore, the total complexity of UPCC-SPaA or UPCC-SPaS is O(m2n+m3+m2logm+TopK∗mn)=O(m2n+m3).
Similarly, in IPCC-SPaA or IPCC-SPaS, the complexity of direct similarity computation for one service pair is O(m). Since we need to compute the similarities of n(n−1)/2 service pairs for all the services, the complexity of direct similarity computation is O(mn2). In indirect similarity computation, the complexity of similarity propagation based on Floyd algorithm is O(n3). To choose the TopK neighbors of the target service, O(nlogn) is need to sort the similarities between the target service and the other services. In addition, we need O(TopK∗m) to make TopK prediction for one target service. Hence, the complexity of predicting the missing values of one target service is O(nlogn+TopK∗m). The complexity of predicting the missing values of all the services is thus O(n2logn+TopK∗mn). Therefore, the total complexity of IPCC-SPaA or IPCC-SPaS is O(mn2+n3+n2logn+TopK∗mn)=O(mn2+n3).
In real-world, direct or indirect similarities can be calculated offline and stored in a database. The real-time prediction performance is only relevant to the online time complexity, which is O(m2logm+TopK∗mn) in user based approach and O(n2logn+TopK∗mn) in service based approach, respectively.
In this section, we use WSDream [11], a well-known web service QoS dataset, to conduct several real-world experiments to investigate the performance of our method. The performance studies include three aspects: the prediction accuracy, time efficiency and the impact of the parameter TopK. WSDream mainly contains two matrices: response time matrix (rt-Matrix) and throughput matrix (tp-Matrix), wherein the QoS data of 5825 web services invoked by 339 users are recorded. These experiments were all conducted on a ThinkPad T490 machine with Intel Core i5-8265U processor and 8 GB RAM. To simulate the real-world environment, the entries of the QoS matrix are randomly removed to a certain density. Then we compare the values of these removed entries with the predicted values to study the prediction accuracy of our approach. Each experiment is conducted for ten times, where different entries are randomly removed in each time. Finally, the mean values are recorded as the experimental results.
In many recommender systems, mean absolute error (MAE) is often used to measure the prediction accuracy [30]. MAE denotes the average absolute deviation between the actual value and the predicted value. The MAE value is calculated by the following formula:
MAE=∑u,i(Rui−ˆRui)W | (15) |
where W is the number of missing items in the matrix, Rui is the actual QoS value of web service i experienced by user u, ˆRui is the predicted QoS value of our approach. Since the value ranges of different QoS attributes are different, we utilize the Normalized Mean Absolute Error (NMAE) metric to evaluate the accuracy of the prediction results. The NMAE value is calculated by the following formula:
NMAE=MAE∑u,iRui/W | (16) |
In the above equation, a larger NMAE value indicates worse prediction accuracy. Therefore, the target of a web service recommender system is to achieve a lower NMAE value.
In this section, we evaluate the prediction accuracy of our approach by comparing with other approaches. In order to study whether our approach is effective in improving the accuracy of similarity computation, traditional memory-based approach such as IPCC and UPCC are compared with IPCC-SPaS and UPCC-SPaS, respectively. We also study the accuracy our approach by comparing with other state-of-the-arts approaches. The approaches evaluated in this section are listed as following:
UPCC (user-based CF method using PCC): UPCC employs the data of similar users for the QoS value prediction [31].
UPCC-SPaS: UPCC-SPaS employs SPaS user similarity propagation strategy to extend the UPCC approach to make prediction.
IPCC (item-based CF method using PCC): IPCC employs the data of similar web services (items) for the QoS value prediction [32].
IPCC-SPaS: IPCC-SPaS employs SPaS service similarity propagation strategy to extend the IPCC approach to make prediction.
WSRec: WSRec [11] is an improved hybrid CF approach, in which both the similar users and the similar services are found to make prediction.
IPCC-ST: IPCC-ST [24] is an IPCC based approach where the indirect similarity is considered and computed by one intermediate service on the similarity transitive path. The similarities of different transitive path are agammaegated by weighted mean method.
IPCC-SPaA: IPCC-SPaA employs SPaA service similarity propagation strategy to extend the IPCC approach to make prediction.
Due to the sparse QoS data in reality, we gradually increase the QoS matrix density from 5 to 14% with the step size of 1%. Then the prediction accuracy of these methods are evaluated by using these matrices with different sparsity. In all the approaches, the parameter TopK is set to 10, meaning that 10 most similar neighbors will be chosen to predict the missing QoS values. Table 4 shows the NMAE values of all the above mentioned methods under different QoS data sparsity conditions. In order to observe the experimental results more intuitively, Figure 3 presents the results in Table 4 in the form of graphs.
Matrices | Methods | Density of Matrix | |||||||||
5% | 6% | 7% | 8% | 9% | 10% | 11% | 12% | 13% | 14% | ||
rt-Matrix | UPCC | 0.9116 | 0.8650 | 0.8154 | 0.7859 | 0.7594 | 0.7245 | 0.7035 | 0.6556 | 0.6309 | 0.6020 |
UPCC-SPaS | 0.8915 | 0.8343 | 0.7733 | 0.7492 | 0.7135 | 0.6864 | 0.6576 | 0.6371 | 0.6220 | 0.5950 | |
IPCC | 0.6384 | 0.6327 | 0.6223 | 0.6266 | 0.6310 | 0.6222 | 0.6208 | 0.6117 | 0.5970 | 0.5821 | |
IPCC-SPaS | 0.5823 | 0.5781 | 0.5653 | 0.5638 | 0.5664 | 0.5540 | 0.5479 | 0.5412 | 0.5360 | 0.5311 | |
WSRec | 0.6440 | 0.6329 | 0.6110 | 0.6041 | 0.6038 | 0.5828 | 0.5717 | 0.5591 | 0.5494 | 0.5388 | |
IPCC-ST | 0.6124 | 0.5994 | 0.5815 | 0.5818 | 0.5836 | 0.5677 | 0.5639 | 0.5507 | 0.5437 | 0.5341 | |
IPCC-SPaA | 0.5884 | 0.5807 | 0.5697 | 0.5712 | 0.5753 | 0.5613 | 0.5550 | 0.5504 | 0.5424 | 0.5309 | |
Impro. of UPCC-SPaS vs UPCC | 2.2% | 3.5% | 5.2% | 4.7% | 6% | 5.3% | 6.5% | 2.8% | 1.4% | 1.2% | |
Impro. of IPCC-SPaS vs IPCC | 8.8% | 8.6% | 9.2% | 10% | 10.2% | 11% | 11.7% | 11.5% | 10.2% | 8.8% | |
Impro. of IPCC-SPaS vs IPCC-ST | 4.9% | 3.6% | 2.8% | 3.1% | 2.9% | 2.4% | 2.8% | 1.7% | 1.4% | 0.6% | |
tp-Matrix | UPCC | 0.7178 | 0.7030 | 0.6779 | 0.6762 | 0.6620 | 0.6414 | 0.6219 | 0.5973 | 0.5884 | 0.5705 |
UPCC-SPaS | 0.6611 | 0.6568 | 0.6351 | 0.6106 | 0.6008 | 0.5790 | 0.5661 | 0.5522 | 0.5538 | 0.5407 | |
IPCC | 0.6353 | 0.6416 | 0.6395 | 0.6454 | 0.6392 | 0.6341 | 0.6211 | 0.6278 | 0.6253 | 0.6176 | |
IPCC-SPaS | 0.5732 | 0.5751 | 0.5655 | 0.5609 | 0.5535 | 0.5433 | 0.5394 | 0.5366 | 0.5344 | 0.5290 | |
WSRec | 0.6063 | 0.6159 | 0.6147 | 0.6094 | 0.5997 | 0.5946 | 0.5920 | 0.5848 | 0.5811 | 0.5803 | |
IPCC-ST | 0.6097 | 0.6164 | 0.6092 | 0.6076 | 0.6015 | 0.5985 | 0.5932 | 0.5913 | 0.5913 | 0.5871 | |
IPCC-SPaA | 0.5733 | 0.5774 | 0.5669 | 0.5610 | 0.5555 | 0.5457 | 0.5400 | 0.5376 | 0.5344 | 0.5296 | |
Impro. of UPCC-SPaS vs UPCC | 7.9% | 6.6% | 6.3% | 9.7% | 9.2% | 9.7% | 9% | 7.6% | 5.9% | 5.2% | |
Impro. of IPCC-SPaS vs IPCC | 9.8% | 10.4% | 11.6% | 13.1% | 13.4% | 14.3% | 13.1% | 14.5% | 14.5% | 14.3% | |
Impro. of IPCC-SPaS vs IPCC-ST | 6% | 6.7% | 7.2% | 7.7% | 8% | 9.2% | 9.1% | 9.3% | 9.6% | 9.9% |
The experimental results demonstrate that:
(1) All methods can achieve smaller NMAE value when the density of QoS matrix increase, which means that we can improve the prediction accuracy of web service recommender systems by obtaining more historical QoS data. Under all matrix density conditions, the methods with similarity propagation can achieve smaller NMAE values than the corresponding methods without similarity propagation. Concretely, compared with UPCC, UPCC-SPAS improves the prediction accuracy of response time values by 4% on average and throughput values by 7% on average, respectively. Compared with IPCC, IPCC-SPAS improves the prediction accuracy of response time values by 10% on average and throughput values by 13% on average, respectively.
(2) Compared with IPCC-ST, IPCC-SPaS can achieve better accuracy both in response time values prediction and throughput values prediction. Concretely, compared with IPCC-ST, IPCC-SPAS improves the prediction accuracy of response time values by 2.6% on average and throughput values by 8.3% on average, respectively. This result indicate that the indirect similarity computation method used in IPCC-ST, which only consider one intermediate service on the similarity transitive path, may ignore much valuable neighbor information. According to find more propagation paths, the similarity can be more precisely calculated. In addition, IPCC-SPaS achieve better accuracy than IPCC-SPaA under all the data conditions, which indicate that the shortest propagation paths can obtain a stronger and more convincing similarity than the long chain paths.
(3) Under all matrix density conditions, the IPCC-SPaS method is superior to other methods in both response time values prediction and throughput values prediction. The advantage is very obvious when the QoS data is extremely sparse, e.g., under 5% matrix density condition. This observation indicates that the similarity propagation strategy is important for discovering more implicit neighbor information in memory-based approaches, especially in the real environment where QoS data is very sparse.
In this section, the time efficiency of different methods is studied by using QoS matrices of different scales. Firstly, the number of users is set to 300 and the number of services is increased from 100 to 500 with the step size of 50. Then, the number of services is set to 300 and the number of users is increased from 100 to 300 with the step size of 20. Therefore, the time efficiency of these methods may be evaluated by the QoS matrices with different scale. In all the approaches, the parameter TopK is set to 10 and the density of QoS matrix is set to 10%.
Figure 4 shows that the computation time of all approaches increase with the amount of QoS data. Our proposed similarity propagation approaches are more efficient than WSRec but more time consuming than the corresponding traditional memory-based approaches. Figure 4 also shows that the computation time of IPCC-SPaS is near to IPCC-SPaA. This result indicates that the time performance of the similarity propagation strategy based on shortest paths is approximate to the strategy based on all paths.
The parameter TopK determines how many similar neighbors should be selected in the QoS prediction algorithm. In order to evaluate the impact of parameter TopK on the accuracy of QoS prediction, we increase the value of TopK from 3 to 30 with the step size of 3. Figures 5 and 6 show the NMAE values of IPCC-SPaS and IPCC-SPaA in response time and throughput values prediction, under three different matrix densities of 5, 10 and 15%.
The experimental results demonstrate that with the increase of TopK, the NMAE value will gradually decrease at the beginning and then become stable or worse after TopK reaching to an optimal value. E.g., the NMAE value become worse when the TopK is larger than 12 in the response time prediction by IPCC-SPaS under 15% matrix density condition. This observation indicates that an appropriate value of TopK is crucial for ensuring the prediction accuracy. This result is interpretable, since too small TopK may cause too few neighbors be selected to make prediction which may ignore the valuable neighbor information, whereas too large TopK may cause many dissimilar neighbors be selected. As shown in Figures 5 and 6, all curves achieve the best results when TopK is between 10 to 15, which means that the optimal value of TopK is stable to some extent and not easily affected by the natures of QoS dataset or the density of matrix both in SPaS and SPaA strategies.
With the rapid growth of web services on the network, web service recommendation becomes essential for customers to select appropriate services to build service-oriented applications. Recently, memory-based CF approaches have been widely studied to realize the web service recommender systems. In these approaches, similarity calculation plays an important role. However, the similarity calculation in most existing approaches are vulnerable to the data sparse problem. In this paper, we propose a similarity propagation approach to evaluate the indirect similarities between users or services. Firstly, the similarity graph of users or services is constructed by using the QoS data. Secondly, the similarity propagation paths among users or services on the similarity graph are found. Moreover, the indirect similarities among users or services are agammaegated by two different strategies and the Flyod based algorithm are designed to implement the similarity propagation strategies. Finally, we integrate the direct similarity and indirect similarity to precisely compute the similarity and make accurate prediction. Extensive real-world experimental evaluations show that our approach can outstandingly improve the prediction accuracy of memory-based CF approaches and not susceptible to the parameters.
It is worth to mention that our similarity propagation strategies are also applicable to other recommender systems, e.g., E-commerce or movie recommender systems. In the future work, we will optimize the similarity propagation strategies in aspect of time performance and prediction accuracy. We also plan to study the applicability of similarity propagation to other memory-based approaches.
This research work is supported by the National Natural Science Foundation of China (NSFC) under Grant No. 61802425 and NSFC under Grant No. 61902422.
The authors declare there is no conflict of interest.
Term | Meaning of term |
SOC | Service-Oriented Computing |
QoS | Quality of Service |
CF | Collaborative Filtering |
SP | Similarity Propagation |
SN | Social Network |
PCC | Pearson Correlation Coefficient |
SPaS | Min-max similarity propagation among shortest paths |
SPaA | Min-max similarity propagation among all paths |
MF | Matrix Factorization |
UPCC | User based method using Pearson Correlation Coefficient |
IPCC | Item based method using Pearson Correlation Coefficient |
UPCC-SPaS | UPCC with similarity propagation by SPaS |
UPCC-SPaA | UPCC with similarity propagation by SPaA |
IPCC-SPaS | IPCC with similarity propagation by SPaS |
IPCC-SPaA | IPCC with similarity propagation by SPaA |
MAE | mean absolute error |
NMAE | Normalized Mean Absolute Error |
[1] | A. Abdullah, X. Li, An integrated-model QoS-based graph for web service recommendation, IEEE International Conference on Web Services, 2015. |
[2] | W. Shi, X. Liu, Q. Yu, Correlation-Aware Multi-Label Active Learning for Web Service Tag Recommendation, IEEE International Conference on Web Services, 2017. |
[3] |
X. Luo, M. Zhou, Z. Wang, Y. Xia, Q. Zhu, An Effective Scheme for QoS Estimation via Alternating Direction Method-Based Matrix Factorization, IEEE Trans. Serv. Comput., 12 (2019), 503–518. doi: 10.1109/TSC.2016.2597829
![]() |
[4] |
Y. Zhang, Y. Fan, W. Tan, J. Zhang, Web Service Recommendation with Reconstructed Profile from Mashup Descriptions, IEEE Trans. Autom. Sci. Eng., 15 (2018), 468–478. doi: 10.1109/TASE.2016.2624310
![]() |
[5] |
K. Su, B. Xiao, B. Liu, Z. Zhang, TAP: A personalized trust-aware QoS prediction approach for web service recommendation, Knowl. Based Syst., 115 (2017), 55–65. doi: 10.1016/j.knosys.2016.09.033
![]() |
[6] |
J. Liu, Y. Chen, A personalized clustering-based and reliable trust-aware QoS prediction approach for cloud service recommendation in cloud manufacturing, Knowl. Based Syst., 174 (2019), 43–56. doi: 10.1016/j.knosys.2019.02.032
![]() |
[7] |
C. Yu, L. Huang, A Web service QoS prediction approach based on time and location aware collaborative filtering, Serv. Oriented Comput. Appl., 10 (2016), 135–149. doi: 10.1007/s11761-014-0168-4
![]() |
[8] |
R. Xiong, J. Wang, N. Zhang, Y. Ma, Deep hybrid collaborative filtering for web service recommendation, Expert Syst. Appl., 110 (2018), 191–205. doi: 10.1016/j.eswa.2018.05.039
![]() |
[9] |
L. Ren, W. Wang, An SVM-based collaborative filtering approach for Top-N web services recommendation, Future Gener. Comput. Syst., 78 (2018), 531–543. doi: 10.1016/j.future.2017.07.027
![]() |
[10] |
Z. Chen, L. Shen, F. Li, Exploiting Web service geographical neighborhood for collaborative QoS prediction, Future Gener. Comput. Syst., 68 (2017), 248–259. doi: 10.1016/j.future.2016.09.022
![]() |
[11] |
Z. Zheng, H. Ma, M. Hao, M. R. Lyu, I. King, QoS-aware web service recommendation by collaborative filtering, IEEE Trans. Serv. Comput., 4 (2011), 140–152. doi: 10.1109/TSC.2010.52
![]() |
[12] |
X. Chen, Z. Zheng, X. Liu, Z. Huang, Personalized QoS-aware web service recommendation and visualization, IEEE Trans. Serv. Comput., 6 (2013), 35–47. doi: 10.1109/TSC.2011.35
![]() |
[13] | L. Fletcher, X. Liu, A Collaborative Filtering Method for Personalized Preference-based Service Recommendation, IEEE International Conference on Web Services, 2015. |
[14] |
Y. Ma, S. Wang, P. Hung, C. Hsu, Q. Sun, F. Yang, A Highly Accurate Prediction Algorithm for Unknown Web Service QoS Values, IEEE Trans. Serv. Comput., 9 (2016), 511–523. doi: 10.1109/TSC.2015.2407877
![]() |
[15] |
N. Idrissi, A. Zellou, A systematic literature review of sparsity issues in recommender systems, Soc. Network Anal. Min., 10 (2020), 1–23. doi: 10.1007/s13278-019-0612-8
![]() |
[16] | F. Gohari, F. Aliee, H. Haghighi, A Dynamic Local-Global Trust-aware Recommendation approach, Electron. Commer. Res. Appl., 34 (2019), 1–23. |
[17] |
Y. Kim, An enhanced trust propagation approach with expertise and homophily-based trust networks, Knowl. Based Syst., 82 (2015), 20–28. doi: 10.1016/j.knosys.2015.02.023
![]() |
[18] |
S. Lyu, J. Liu, M. Tang, Y. Xu, J. Chen, Efficiently Predicting Trustworthiness of Mobile Services Based on Trust Propagation in Social Networks, Mobile Networks Appl., 20 (2015), 840–852. doi: 10.1007/s11036-015-0619-y
![]() |
[19] |
J. Yin, Y. Xu, Personalized QoS-based Web Service Recommendation with Service Neighborhood-Enhanced Matrix Factorization, Int. J. Web Grid Serv., 11 (2015), 39–56. doi: 10.1504/IJWGS.2015.067156
![]() |
[20] | K. Qi, H. Hu, W. Song, J. Ge, J. Lü, Personalized QoS Prediction via Matrix Factorization Integrated with Neighborhood Information, IEEE International Conference on Services Computing, 2015. |
[21] | Y. Yin, L. Chen, Y. Xu, J. Wan, H. Zhang, Z. Mai, QoS Prediction for Service Recommendation with Deep Feature Learning in Edge Computing Environment, Mobile Networks Appl., 2019 (2019), 1–11. |
[22] | D. Ryu, K. Lee, J. Baik, Location-based Web Service QoS Prediction via Preference Propagation to address Cold Start Problem, IEEE Trans. Serv. Comput., 2018 (2018). |
[23] | X. Zhu, X. Jing, D. Wu, Z. He, J. Cao, D. Yue, et al., Similarity-maintaining Privacy Preservation and Location-aware Low-rank Matrix Factorization for QoS Prediction based Web Service Recommendation, IEEE Trans. Serv. Comput., 2018 (2018). |
[24] | K. Su, L. Ma, B. Xiao, H. Zhang, Web service QoS prediction by neighbor information combined non-negative matrix factorization, J. Int. Fuzzy Syst., 30 (2016), 3593–3604. |
[25] |
Z. Zheng, H. Ma, M. Hao, M. R. Lyu, I. King, Collaborative web service QoS prediction via neighborhood integrated matrix factorization, IEEE Trans. Serv. Comput., 6 (2013), 289–299. doi: 10.1109/TSC.2011.59
![]() |
[26] | J. Golbeck, J. Hendler, Inferring binary trust relationships in web-based social networks. ACM Trans. Int. Technol., 6 (2006), 497–529. |
[27] |
Y. Kim, H. Song, Strategies for predicting local trust based on trust propagation in social networks, Knowl. Based Syst., 24 (2011), 1360–1371. doi: 10.1016/j.knosys.2011.06.009
![]() |
[28] | D. Wei, An Optimized Floyd Algorithm for the Shortest Path Problem, J. Networks, 12 (2010), 1469–1504. |
[29] | D. Rafailidis, F. Crestani, A Regularization Method with Inference of Trust and Distrust in Recommender Systems, Joint European Conference on Machine Learning and Knowledge Discovery in Databases, 2017. |
[30] | H. Rong, P. Pearl, Enhancing collaborative filtering systems with personality information, Proceedings of The 5th ACM Conference on Recommender Systems, 2011. |
[31] | L. Shao, J. Zhang, Y. Wei, J. Zhao, B. Xie, H. Mei, Personalized QoS prediction for Web services via collaborative filtering, IEEE International Conference on Web Services, 2007. |
[32] | B. Sarwar, G. Karypis, J. Konstan, J. Riedl, Item-based collaborative filtering recommendation algorithms, Proceedings of the 10th international conference on World Wide Web, 2001. |
1. | Junwei Zhang, Deyu Li, A Comprehensive and Unified Approach to Web Service Trust Evaluation Based on Uncertainty Methodology, 2022, 24, 1099-4300, 243, 10.3390/e24020243 |
s1 | s2 | s3 | s4 | s5 | |
u1 | 0.5 | 0.4 | 0.3 | ||
u2 | 0.8 | 0.7 | 0.7 | ||
u3 | 0.3 | 0.8 | |||
u4 | 0.6 | 0.2 | 0.1 | 0.5 | |
u5 | 0.7 | 0.2 | 0.6 | 0.3 |
u1 | u2 | u3 | u4 | u5 | u6 | u7 | |
u1 | 0 | 0.5 | 0 | 0.1 | 0 | 0.6 | 0.3 |
u2 | 0.5 | 0 | 0 | 0.4 | 0 | 0 | 0 |
u3 | 0 | 0 | 0 | 0 | 0 | 0.2 | 0.6 |
u4 | 0.1 | 0.4 | 0 | 0 | 0.4 | 0 | 0 |
u5 | 0 | 0 | 0 | 0.4 | 0 | 0.2 | 0.5 |
u6 | 0.6 | 0 | 0.2 | 0 | 0.2 | 0 | 0 |
u7 | 0.3 | 0 | 0.6 | 0 | 0.5 | 0 | 0 |
Algorithm: SPaA (min-max similarity propagation among all paths) | Algorithm: SPaS (min-max similarity propagation among shortest paths) |
Input: user direct similarity matrix SimD, the number of users m, distance matrix L, where each entry Lij denotes the distance between node i and j Output: user indirect similarity matrix SimI, where SimIij denotes the indirect similarity between user i and j 1 SimI=SimD; 2 For each entry Lij in L do 3 If SimIij=0 then Lij=0, else Lij=1; 4 End for 5 For (k=1;k⩽m;k++) do 6 For (i=1;i⩽m;i++) do 7 For (j=1;j⩽m;j++) do 8 If (k≠i&&k≠j&&i≠j) then 9 If ((Lik+Lkj)⩽6) then 10 If (SimIij<min{SimIik,SimIkj}) then 11 SimIij=min{SimIik,SimIkj}; 12 Lij=Lik+Lkj; 13 End if 14 End if 15 End if 16 End for 17 End for 18 End for 19 Return SimI |
Input: user direct similarity matrix SimD, the number of users m, distance matrix L, where each entry Lij denotes the distance between node i and j Output: user indirect similarity matrix SimI, where SimIij denotes the indirect similarity between user i and j 1 Each entry SimIij in SimI is initialized with the indirect similarity propagated from only one intermediate node; 2 For each entry Lij in L do 3 If SimIij=0 then Lij=0, else Lij=2; 4 End for 5 For (k=1;k⩽m;k++) do 6 For (i=1;i⩽m;i++) do 7 For (j=1;j⩽m;j++) do 8 If (k≠i&&k≠j&&i≠j) then 9 If (SimIij<min{SimIik,SimIkj}) then 10 If ((Lik+Lkj)⩽Lij P Lij==0) then 11 SimIij=min{SimIik,SimIkj}; 12 Lij=Lik+Lkj; 13 End if 14 End if 15 End if 16 End for 17 End for 18 End for 19 Return SimI |
Matrices | Methods | Density of Matrix | |||||||||
5% | 6% | 7% | 8% | 9% | 10% | 11% | 12% | 13% | 14% | ||
rt-Matrix | UPCC | 0.9116 | 0.8650 | 0.8154 | 0.7859 | 0.7594 | 0.7245 | 0.7035 | 0.6556 | 0.6309 | 0.6020 |
UPCC-SPaS | 0.8915 | 0.8343 | 0.7733 | 0.7492 | 0.7135 | 0.6864 | 0.6576 | 0.6371 | 0.6220 | 0.5950 | |
IPCC | 0.6384 | 0.6327 | 0.6223 | 0.6266 | 0.6310 | 0.6222 | 0.6208 | 0.6117 | 0.5970 | 0.5821 | |
IPCC-SPaS | 0.5823 | 0.5781 | 0.5653 | 0.5638 | 0.5664 | 0.5540 | 0.5479 | 0.5412 | 0.5360 | 0.5311 | |
WSRec | 0.6440 | 0.6329 | 0.6110 | 0.6041 | 0.6038 | 0.5828 | 0.5717 | 0.5591 | 0.5494 | 0.5388 | |
IPCC-ST | 0.6124 | 0.5994 | 0.5815 | 0.5818 | 0.5836 | 0.5677 | 0.5639 | 0.5507 | 0.5437 | 0.5341 | |
IPCC-SPaA | 0.5884 | 0.5807 | 0.5697 | 0.5712 | 0.5753 | 0.5613 | 0.5550 | 0.5504 | 0.5424 | 0.5309 | |
Impro. of UPCC-SPaS vs UPCC | 2.2% | 3.5% | 5.2% | 4.7% | 6% | 5.3% | 6.5% | 2.8% | 1.4% | 1.2% | |
Impro. of IPCC-SPaS vs IPCC | 8.8% | 8.6% | 9.2% | 10% | 10.2% | 11% | 11.7% | 11.5% | 10.2% | 8.8% | |
Impro. of IPCC-SPaS vs IPCC-ST | 4.9% | 3.6% | 2.8% | 3.1% | 2.9% | 2.4% | 2.8% | 1.7% | 1.4% | 0.6% | |
tp-Matrix | UPCC | 0.7178 | 0.7030 | 0.6779 | 0.6762 | 0.6620 | 0.6414 | 0.6219 | 0.5973 | 0.5884 | 0.5705 |
UPCC-SPaS | 0.6611 | 0.6568 | 0.6351 | 0.6106 | 0.6008 | 0.5790 | 0.5661 | 0.5522 | 0.5538 | 0.5407 | |
IPCC | 0.6353 | 0.6416 | 0.6395 | 0.6454 | 0.6392 | 0.6341 | 0.6211 | 0.6278 | 0.6253 | 0.6176 | |
IPCC-SPaS | 0.5732 | 0.5751 | 0.5655 | 0.5609 | 0.5535 | 0.5433 | 0.5394 | 0.5366 | 0.5344 | 0.5290 | |
WSRec | 0.6063 | 0.6159 | 0.6147 | 0.6094 | 0.5997 | 0.5946 | 0.5920 | 0.5848 | 0.5811 | 0.5803 | |
IPCC-ST | 0.6097 | 0.6164 | 0.6092 | 0.6076 | 0.6015 | 0.5985 | 0.5932 | 0.5913 | 0.5913 | 0.5871 | |
IPCC-SPaA | 0.5733 | 0.5774 | 0.5669 | 0.5610 | 0.5555 | 0.5457 | 0.5400 | 0.5376 | 0.5344 | 0.5296 | |
Impro. of UPCC-SPaS vs UPCC | 7.9% | 6.6% | 6.3% | 9.7% | 9.2% | 9.7% | 9% | 7.6% | 5.9% | 5.2% | |
Impro. of IPCC-SPaS vs IPCC | 9.8% | 10.4% | 11.6% | 13.1% | 13.4% | 14.3% | 13.1% | 14.5% | 14.5% | 14.3% | |
Impro. of IPCC-SPaS vs IPCC-ST | 6% | 6.7% | 7.2% | 7.7% | 8% | 9.2% | 9.1% | 9.3% | 9.6% | 9.9% |
Term | Meaning of term |
SOC | Service-Oriented Computing |
QoS | Quality of Service |
CF | Collaborative Filtering |
SP | Similarity Propagation |
SN | Social Network |
PCC | Pearson Correlation Coefficient |
SPaS | Min-max similarity propagation among shortest paths |
SPaA | Min-max similarity propagation among all paths |
MF | Matrix Factorization |
UPCC | User based method using Pearson Correlation Coefficient |
IPCC | Item based method using Pearson Correlation Coefficient |
UPCC-SPaS | UPCC with similarity propagation by SPaS |
UPCC-SPaA | UPCC with similarity propagation by SPaA |
IPCC-SPaS | IPCC with similarity propagation by SPaS |
IPCC-SPaA | IPCC with similarity propagation by SPaA |
MAE | mean absolute error |
NMAE | Normalized Mean Absolute Error |
s1 | s2 | s3 | s4 | s5 | |
u1 | 0.5 | 0.4 | 0.3 | ||
u2 | 0.8 | 0.7 | 0.7 | ||
u3 | 0.3 | 0.8 | |||
u4 | 0.6 | 0.2 | 0.1 | 0.5 | |
u5 | 0.7 | 0.2 | 0.6 | 0.3 |
u1 | u2 | u3 | u4 | u5 | u6 | u7 | |
u1 | 0 | 0.5 | 0 | 0.1 | 0 | 0.6 | 0.3 |
u2 | 0.5 | 0 | 0 | 0.4 | 0 | 0 | 0 |
u3 | 0 | 0 | 0 | 0 | 0 | 0.2 | 0.6 |
u4 | 0.1 | 0.4 | 0 | 0 | 0.4 | 0 | 0 |
u5 | 0 | 0 | 0 | 0.4 | 0 | 0.2 | 0.5 |
u6 | 0.6 | 0 | 0.2 | 0 | 0.2 | 0 | 0 |
u7 | 0.3 | 0 | 0.6 | 0 | 0.5 | 0 | 0 |
Algorithm: SPaA (min-max similarity propagation among all paths) | Algorithm: SPaS (min-max similarity propagation among shortest paths) |
Input: user direct similarity matrix SimD, the number of users m, distance matrix L, where each entry Lij denotes the distance between node i and j Output: user indirect similarity matrix SimI, where SimIij denotes the indirect similarity between user i and j 1 SimI=SimD; 2 For each entry Lij in L do 3 If SimIij=0 then Lij=0, else Lij=1; 4 End for 5 For (k=1;k⩽m;k++) do 6 For (i=1;i⩽m;i++) do 7 For (j=1;j⩽m;j++) do 8 If (k≠i&&k≠j&&i≠j) then 9 If ((Lik+Lkj)⩽6) then 10 If (SimIij<min{SimIik,SimIkj}) then 11 SimIij=min{SimIik,SimIkj}; 12 Lij=Lik+Lkj; 13 End if 14 End if 15 End if 16 End for 17 End for 18 End for 19 Return SimI |
Input: user direct similarity matrix SimD, the number of users m, distance matrix L, where each entry Lij denotes the distance between node i and j Output: user indirect similarity matrix SimI, where SimIij denotes the indirect similarity between user i and j 1 Each entry SimIij in SimI is initialized with the indirect similarity propagated from only one intermediate node; 2 For each entry Lij in L do 3 If SimIij=0 then Lij=0, else Lij=2; 4 End for 5 For (k=1;k⩽m;k++) do 6 For (i=1;i⩽m;i++) do 7 For (j=1;j⩽m;j++) do 8 If (k≠i&&k≠j&&i≠j) then 9 If (SimIij<min{SimIik,SimIkj}) then 10 If ((Lik+Lkj)⩽Lij P Lij==0) then 11 SimIij=min{SimIik,SimIkj}; 12 Lij=Lik+Lkj; 13 End if 14 End if 15 End if 16 End for 17 End for 18 End for 19 Return SimI |
Matrices | Methods | Density of Matrix | |||||||||
5% | 6% | 7% | 8% | 9% | 10% | 11% | 12% | 13% | 14% | ||
rt-Matrix | UPCC | 0.9116 | 0.8650 | 0.8154 | 0.7859 | 0.7594 | 0.7245 | 0.7035 | 0.6556 | 0.6309 | 0.6020 |
UPCC-SPaS | 0.8915 | 0.8343 | 0.7733 | 0.7492 | 0.7135 | 0.6864 | 0.6576 | 0.6371 | 0.6220 | 0.5950 | |
IPCC | 0.6384 | 0.6327 | 0.6223 | 0.6266 | 0.6310 | 0.6222 | 0.6208 | 0.6117 | 0.5970 | 0.5821 | |
IPCC-SPaS | 0.5823 | 0.5781 | 0.5653 | 0.5638 | 0.5664 | 0.5540 | 0.5479 | 0.5412 | 0.5360 | 0.5311 | |
WSRec | 0.6440 | 0.6329 | 0.6110 | 0.6041 | 0.6038 | 0.5828 | 0.5717 | 0.5591 | 0.5494 | 0.5388 | |
IPCC-ST | 0.6124 | 0.5994 | 0.5815 | 0.5818 | 0.5836 | 0.5677 | 0.5639 | 0.5507 | 0.5437 | 0.5341 | |
IPCC-SPaA | 0.5884 | 0.5807 | 0.5697 | 0.5712 | 0.5753 | 0.5613 | 0.5550 | 0.5504 | 0.5424 | 0.5309 | |
Impro. of UPCC-SPaS vs UPCC | 2.2% | 3.5% | 5.2% | 4.7% | 6% | 5.3% | 6.5% | 2.8% | 1.4% | 1.2% | |
Impro. of IPCC-SPaS vs IPCC | 8.8% | 8.6% | 9.2% | 10% | 10.2% | 11% | 11.7% | 11.5% | 10.2% | 8.8% | |
Impro. of IPCC-SPaS vs IPCC-ST | 4.9% | 3.6% | 2.8% | 3.1% | 2.9% | 2.4% | 2.8% | 1.7% | 1.4% | 0.6% | |
tp-Matrix | UPCC | 0.7178 | 0.7030 | 0.6779 | 0.6762 | 0.6620 | 0.6414 | 0.6219 | 0.5973 | 0.5884 | 0.5705 |
UPCC-SPaS | 0.6611 | 0.6568 | 0.6351 | 0.6106 | 0.6008 | 0.5790 | 0.5661 | 0.5522 | 0.5538 | 0.5407 | |
IPCC | 0.6353 | 0.6416 | 0.6395 | 0.6454 | 0.6392 | 0.6341 | 0.6211 | 0.6278 | 0.6253 | 0.6176 | |
IPCC-SPaS | 0.5732 | 0.5751 | 0.5655 | 0.5609 | 0.5535 | 0.5433 | 0.5394 | 0.5366 | 0.5344 | 0.5290 | |
WSRec | 0.6063 | 0.6159 | 0.6147 | 0.6094 | 0.5997 | 0.5946 | 0.5920 | 0.5848 | 0.5811 | 0.5803 | |
IPCC-ST | 0.6097 | 0.6164 | 0.6092 | 0.6076 | 0.6015 | 0.5985 | 0.5932 | 0.5913 | 0.5913 | 0.5871 | |
IPCC-SPaA | 0.5733 | 0.5774 | 0.5669 | 0.5610 | 0.5555 | 0.5457 | 0.5400 | 0.5376 | 0.5344 | 0.5296 | |
Impro. of UPCC-SPaS vs UPCC | 7.9% | 6.6% | 6.3% | 9.7% | 9.2% | 9.7% | 9% | 7.6% | 5.9% | 5.2% | |
Impro. of IPCC-SPaS vs IPCC | 9.8% | 10.4% | 11.6% | 13.1% | 13.4% | 14.3% | 13.1% | 14.5% | 14.5% | 14.3% | |
Impro. of IPCC-SPaS vs IPCC-ST | 6% | 6.7% | 7.2% | 7.7% | 8% | 9.2% | 9.1% | 9.3% | 9.6% | 9.9% |
Term | Meaning of term |
SOC | Service-Oriented Computing |
QoS | Quality of Service |
CF | Collaborative Filtering |
SP | Similarity Propagation |
SN | Social Network |
PCC | Pearson Correlation Coefficient |
SPaS | Min-max similarity propagation among shortest paths |
SPaA | Min-max similarity propagation among all paths |
MF | Matrix Factorization |
UPCC | User based method using Pearson Correlation Coefficient |
IPCC | Item based method using Pearson Correlation Coefficient |
UPCC-SPaS | UPCC with similarity propagation by SPaS |
UPCC-SPaA | UPCC with similarity propagation by SPaA |
IPCC-SPaS | IPCC with similarity propagation by SPaS |
IPCC-SPaA | IPCC with similarity propagation by SPaA |
MAE | mean absolute error |
NMAE | Normalized Mean Absolute Error |