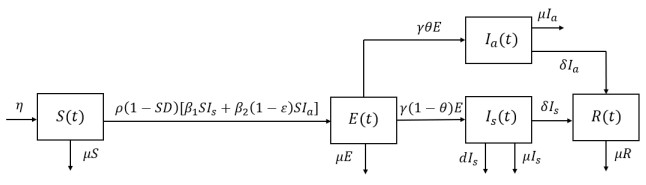
Citation: Bernhard Voelkl. Quantitative characterization of animal social organization: Applications for epidemiological modelling[J]. Mathematical Biosciences and Engineering, 2020, 17(5): 5005-5026. doi: 10.3934/mbe.2020271
[1] | Hamdy M. Youssef, Najat A. Alghamdi, Magdy A. Ezzat, Alaa A. El-Bary, Ahmed M. Shawky . A new dynamical modeling SEIR with global analysis applied to the real data of spreading COVID-19 in Saudi Arabia. Mathematical Biosciences and Engineering, 2020, 17(6): 7018-7044. doi: 10.3934/mbe.2020362 |
[2] | Adil Yousif, Awad Ali . The impact of intervention strategies and prevention measurements for controlling COVID-19 outbreak in Saudi Arabia. Mathematical Biosciences and Engineering, 2020, 17(6): 8123-8137. doi: 10.3934/mbe.2020412 |
[3] | Sarah R. Al-Dawsari, Khalaf S. Sultan . Modeling of daily confirmed Saudi COVID-19 cases using inverted exponential regression. Mathematical Biosciences and Engineering, 2021, 18(3): 2303-2330. doi: 10.3934/mbe.2021117 |
[4] | Mattia Zanella, Chiara Bardelli, Mara Azzi, Silvia Deandrea, Pietro Perotti, Santino Silva, Ennio Cadum, Silvia Figini, Giuseppe Toscani . Social contacts, epidemic spreading and health system. Mathematical modeling and applications to COVID-19 infection. Mathematical Biosciences and Engineering, 2021, 18(4): 3384-3403. doi: 10.3934/mbe.2021169 |
[5] | Enahoro A. Iboi, Oluwaseun Sharomi, Calistus N. Ngonghala, Abba B. Gumel . Mathematical modeling and analysis of COVID-19 pandemic in Nigeria. Mathematical Biosciences and Engineering, 2020, 17(6): 7192-7220. doi: 10.3934/mbe.2020369 |
[6] | Avinash Shankaranarayanan, Hsiu-Chuan Wei . Mathematical modeling of SARS-nCoV-2 virus in Tamil Nadu, South India. Mathematical Biosciences and Engineering, 2022, 19(11): 11324-11344. doi: 10.3934/mbe.2022527 |
[7] | Xinyu Bai, Shaojuan Ma . Stochastic dynamical behavior of COVID-19 model based on secondary vaccination. Mathematical Biosciences and Engineering, 2023, 20(2): 2980-2997. doi: 10.3934/mbe.2023141 |
[8] | Haiyan Wang, Nao Yamamoto . Using a partial differential equation with Google Mobility data to predict COVID-19 in Arizona. Mathematical Biosciences and Engineering, 2020, 17(5): 4891-4904. doi: 10.3934/mbe.2020266 |
[9] | Sarita Bugalia, Vijay Pal Bajiya, Jai Prakash Tripathi, Ming-Tao Li, Gui-Quan Sun . Mathematical modeling of COVID-19 transmission: the roles of intervention strategies and lockdown. Mathematical Biosciences and Engineering, 2020, 17(5): 5961-5986. doi: 10.3934/mbe.2020318 |
[10] | Javad Hassannataj Joloudari, Faezeh Azizi, Issa Nodehi, Mohammad Ali Nematollahi, Fateme Kamrannejhad, Edris Hassannatajjeloudari, Roohallah Alizadehsani, Sheikh Mohammed Shariful Islam . Developing a Deep Neural Network model for COVID-19 diagnosis based on CT scan images. Mathematical Biosciences and Engineering, 2023, 20(9): 16236-16258. doi: 10.3934/mbe.2023725 |
Coronavirus disease 2019, COVID-19, is a new infectious disease that emerged at the end of 2019. Within three months, became a global epidemic [1]. As of December 26, 2021, the number of confirmed cases around the world had reached 278,714,484 [2]. The epidemic affected various aspects of life, including the economic, health and social aspects.
Several researchers have discussed the disease dynamics and characteristics of COVID-19 patients in different regions of the world. Individuals infected with COVID-19 may or may not have symptoms that range from mild to severe. Individuals who experience a fever, dry cough, fatigue and headaches as mild symptoms or acute respiratory distress syndrome as a severe symptom [3] are called symptomatic individuals. Individuals who do not show any signs and their infection is confirmed through real-time reverse transcriptase-polymerase chain reaction (RT-PCR), i.e., a type of diagnostic test for COVID-19, are called asymptomatic individuals [4]. These patients can also transmit the disease to other individuals [5,6].
The presence of asymptomatic patients has led researchers to focus on studying this class of patients. Some research has described the clinical characteristics of asymptomatic and symptomatic patients of COVID-19 [7,8]. Kim et al. [7] determined the spread of asymptomatic cases in South Korea. Of 213 infected individuals with COVID-19, 41 (19.2%) were asymptomatic. They concluded that as much as one-fifth of infected individuals with COVID-19 are asymptomatic. Therefore, strict social distancing must be applied to prevent the transmission of the disease through these individuals. AlJishi et al. [8] described the spread of COVID-19 at the epicenter of its spread in Saudi Arabia, in the eastern region of the kingdom. They concluded that most COVID-19 cases were asymptomatic and returned from travel. In addition, Alsofayan et al. [9] emphasized the need to give attention to asymptomatic individuals and health care workers because they contribute to the spread of the disease.
Several studies have proposed a mathematical model that considers asymptomatic and symptomatic infected individuals [10,11,12,13,14,15,16,17,18]. A mathematical model was proposed in [10] to discover the effect of asymptomatic infected people and estimate the number of beds needed in hospitals and intensive care units in Saudi Arabia. They concluded that asymptomatic people are more effective in increasing the number of infections than symptomatic people. However, the increase in COVID-19 testing and social distancing reduces the infection by asymptomatic individuals. Sun and Weng [15] established a mathematical model to discuss the effect of asymptomatic and imported patients in the Jiangsu and Anhui provinces in China. Their analysis found that asymptomatic patients are more dangerous than imported patients. Moreover, asymptomatic patients can rapidly cause an outbreak of the disease without strict preventive measures.
Serhani and Labbardi [16] analyzed two mathematical models to study the spread of COVID-19 in Morocco without and with containment of the pandemic. They found that the free equilibrium point is asymptotically stable when the containment coefficient exceeds the basic reproduction number R0. Furthermore, increasing the containment rate decreases the number of asymptomatic and symptomatic individuals. Huo et al. [17] studied the spread of COVID-19 and the effect of non-pharmaceutical interventions in Wuhan. They estimated that 20% of patients remain asymptomatic during infection, and that their ability to transmit is around 70% of the symptomatic patients' ability.
Moreover, in [13,14], the authors analyzed mathematical models for COVID-19 in Saudi Arabia. The models assumed the transmission of infection is due to the environment and occurs through contact with exposed, asymptomatic and symptomatic individuals. Alzahrani et al. [13] dealt with a fractional model. Alqarni et al. [14] used a classical epidemic model and concluded that the cases in Saudi Arabia would decrease if contact with exposed individuals and the environment decreases.
With the increasing number of infections and the contribution of asymptomatic individuals to the transmission of the disease, there have been efforts in various countries to constrain the epidemic. Several precautions have been imposed to keep susceptible individuals away from infected individuals. Studies have demonstrated the effectiveness of preventive measures, such as lockdowns [19,20,21], social distancing [22,23,24], quarantining [12,16] and isolation [25,26]. Nevertheless, we need measures to detect asymptomatic patients, particularly at the beginning of the disease and before treatment is available, to limit its spread and reduce the number of deaths, an example of such a measure is diagnosis testing [27].
Al-Salti et al. [18] developed a mathematical model to analyze the optimal control strategies, especially diagnosis and quarantining, for the spread of COVID-19 in the Sultanate of Oman. It was concluded that diagnosis and quarantining are the most important strategies for controlling the disease. Similarly, in [11], Ahmed et al. confirmed the effectiveness of the diagnosis in reducing the infected cases. In addition, Khan et al. [28] studied the effectiveness of Saudi Arabia's experience in producing the diagnosis of COVID-19. From April 14 to mid-July of 2020, the Saudi's diagnostic program for COVID-19 was conducted in three stages [28,29]. The first stage was performing random diagnostic tests on people in crowded places such as workers' housing, which included 807 locations from April 16 to May 5, 2020 [30]. The second stage was conducting diagnostic tests in specific health centers through an application (Mawid) from May 3 to May 19, 2020. The third stage was conducting rapid tests at medical centers through drive-in vehicles, which started on May 29, 2020 [31,32]. Also, the government of Saudi Arabia has continued to expand the diagnostic procedures for COVID-19 [33,34,35,36].
This paper describe the study of the impact of detecting asymptomatic COVID-19 patients through random diagnostic testing in Saudi Arabia. Random diagnosis means diagnosing COVID-19 for suspected individuals at random, whether they show symptoms or not. In other words, officials in the Ministry of Health go to homes and diagnose individuals in places where there are factors that contribute to the spread of the disease, such as overpopulation.
We extend our model in [37] to include the asymptomatic COVID-19 patients and analyze the impact of random diagnostic tests. Therefore, this model contains three control measures that have been implemented in Saudi Arabia: lockdown, social distancing and random diagnostic testing. The analysis of this model attempts to determine if it is possible to rely on random diagnosis while reducing or eliminating lockdown and social distancing. This paper is organized as follows. In Section 2, we formulate the model and prove that it is well posed. In Section 3, we demonstrate the qualitative analysis, including the equilibrium points, basic and control reproduction numbers, and the local and global stability of the equilibrium points. Section 4 presents the numerical analysis, which includes the fitting of the model to COVID-19 cases in Saudi Arabia and the estimation of the model parameters. Furthermore, we describe numerical experiments that were conducted to confirm the qualitative analysis results and investigate the sensitivity analysis for the control reproduction number. Finally, we analyze different scenarios for control strategies, random diagnostic testing, lockdown and social distancing.
The model was formulated to examine the effect of the random diagnostic tests for asymptomatic patients on the spread of COVID-19 in Saudi Arabia. The Saudi population, N, comprises five compartments: susceptible individuals, S, exposed individuals, E, asymptomatic infected individuals (with no symptoms), Ia, symptomatic infected individuals (with symptoms), Is, and recovered individuals, R. Individuals move from the susceptible compartment to the exposed compartment after interacting with infected individuals, symptomatic or asymptomatic, at the transmission rates β1 and β2, respectively. The control measures, i.e., the lockdown (ρ(t)∈(0,1]) and the social distancing (SD(t)∈[0,1)), impact the transmission rates β1 and β2. However, the random diagnostic testing for asymptomatic individuals affects only β2. The function ε(t)∈[0,1] represents the effectiveness of the random diagnostic testing for Ia. If ε=1, then the random diagnostic test was administered to all asymptomatic individuals in the community. Conversely, no random diagnostic test is performed for Ia when ε=0. After completing the incubation period, 1/γ, individuals in the exposed compartment move to the asymptomatic compartment with the probability θ, where θ∈(0,1); and the rest of the individuals (1−θ) move to the symptomatic compartment. We assume that individuals in the exposed compartment may or may not show symptoms by the end of the incubation period. Also, individuals in the asymptomatic compartment can transmit the disease more than individuals in the symptomatic compartment, that is, β2>β1. Moreover, individuals die due to COVID-19 only from the symptomatic compartment at a rate d. Infected individuals, asymptomatic and symptomatic, recover and gain immunity to COVID-19 at a rate δ. The natural death rate for each compartment is μ, and η is the natural birth rate.
Figure 1 displays the model's dynamics. We express the model mathematically with the following nonlinear system of ordinary differential equations:
dSdt=η−ρ(1−SD)[β1SIs+β2(1−ε)SIa]−μS,dEdt=ρ(1−SD)[β1SIs+β2(1−ε)SIa]−(γ+μ)E,dIadt=γθE−(δ+μ)Ia,dIsdt=γ(1−θ)E−(δ+d+μ)Is,dRdt=δIa+δIs−μR, | (2.1) |
where all parameters belong to the interval (0,1] and N=S(t)+E(t)+Ia(t)+Is(t)+R(t).
We start the analysis by proving that the state variables of Model (2.1) are epidemiologically meaningful, that is, non-negative and bounded.
Theorem 1
If (S,E,Ia,Is,R)∈R5≥0, then the set
Ω={(S,E,Ia,Is,R)∈R5≥0:0≤N≤ημ} |
is positively invariant for Model (2.1).
Proof.
Let (S(0),E(0),Ia(0),Is(0),R(0))∈Ω. We have
dSdt|S=0=η>0,dEdt|E=0=ρ(1−SD)[β1SIs+β2(1−ε)SIa]≥0,forall S, Ia, Is≥0,dIadt|Ia=0=γθE≥0,forall E≥0,dIsdt|Is=0=γ(1−θ)E≥0,forall E≥0,dRdt|R=0=δIa+δIs≥0,forall Ia, Is≥0. |
Thus, all non-negative solutions remain non-negative for t≥0. By adding all of the equations of Model (2.1), we get
dNdt=η−dIs−μN≤η−μN. |
Multiplying the above inequality by the integrating factor (eμr), we have
ddr[eμrN(r)]≤ηeμr. |
By integrating both sides over the interval [0,t], we obtain
N(t)≤ημ+[N(0)−ημ]e−μt. |
Hence,
limt→∞Sup[N(t)]≤ημ. |
Therefore, for t≥0, the solutions of Model (2.1) are non-negative and bounded. Thus, Ω is positively invariant.
We qualitatively investigate Model (2.1). First, we determine the equilibrium points and the expression of the control reproduction number. Second, we examine the local and global stability of the equilibrium points.
The equilibrium points of the model are determined by setting the rates for Model (2.1) to zero:
η−ρ(1−SD)[β1SIs+β2(1−ε)SIa]−μS=0,ρ(1−SD)[β1SIs+β2(1−ε)SIa]−(γ+μ)E=0,γθE−(δ+μ)Ia=0,γ(1−θ)E−(δ+d+μ)Is=0,δIa+δIs−μR=0. | (3.1) |
System (3.1) produces two steady-state solutions: the COVID-19 free equilibrium point, P0=(η/μ,0,0,0,0), which always exists, and the COVID-19 endemic equilibrium point, P1=(S1,E1,Ia1,Is1,R1), where
S1=ημRc,Ia1=ηγθ(γ+μ)(δ+μ)(1−1Rc),E1=η(γ+μ)(1−1Rc),Is1=ηγ(1−θ)(γ+μ)(δ+d+μ)(1−1Rc),R1=ηγ(δθd+δ2+δμ)μ(γ+μ)(δ+μ)(δ+d+μ)(1−1Rc). |
Here,
Rc=ρ(1−SD)β1(1−θ)γημ(γ+μ)(δ+d+μ)+ρ(1−SD)β2θ(1−ε)γημ(γ+μ)(δ+μ). |
The endemic equilibrium P1 exists only if Rc>1.
Control reproduction number. We use the next-generation matrix method [38] to find the control reproduction number. Let the infected compartments O=(E,Ia,Is)T; then, the exposed class, asymptomatic class and symptomatic class equations of Model (2.1) can be rewritten as ˙O=F(O)−V(O), where
F=[ρ(1−SD)[β1SIs+β2(1−ε)SIa]00] |
and
V=[(γ+μ)E−γθE+(δ+μ)Ia−γ(1−θ)E+(δ+d+μ)Is]. |
Evaluating the Jacobian matrix of F and V at the COVID-19 free equilibrium point P0, we get, respectively,
F=[0ρ(1−SD)β2(1−ε)ημρ(1−SD)β1ημ000000] |
and
V=[γ+μ00−γθδ+μ0−γ(1−θ)0δ+d+μ]. |
The next-generation matrix is
FV−1=[K1ρ(1−SD)β2(1−ε)ημ(δ+μ)ρ(1−SD)β1ημ(δ+d+μ)000000], |
where
K1=ρ(1−SD)β1(1−θ)γημ(γ+μ)(δ+d+μ)+ρ(1−SD)β2θ(1−ε)γημ(γ+μ)(δ+μ). |
Hence, the control reproduction number is the spectral radius of the matrix FV−1, that is,
Rc=ρ(1−SD)β1(1−θ)γημ(γ+μ)(δ+d+μ)+ρ(1−SD)β2θ(1−ε)γημ(γ+μ)(δ+μ). | (3.2) |
Also, we can rewrite Expression (3.2) as follows: Rc=ρ(1−SD)(Rs+(1−ε)Ra), where Rs gives the secondary cases of COVID-19 by one symptomatic individuals, and Ra gives the secondary cases of COVID-19 by one asymptomatic individuals.
The terms in Rc are explained further as follows. First, for Rs, the term that expresses the incidence of new infections by symptomatic individuals is β1SIs. Thus, the number of secondary cases by one symptomatic individual (Is=1) in a population containing only susceptible individuals is β1S0, where S0=η/μ. Moreover, 1/(δ+d+μ) represents the average time spent by one symptomatic individual in the symptomatic compartment. Also, γ(1−θ)/(γ+μ) is the proportion of newly symptomatic individuals that survived the incubation period. Second, for Ra, the term that represents the incidence of new infections by asymptomatic individuals is β2SIa. Therefore, the number of secondary cases by one asymptomatic individual (Ia=1) in a population containing only susceptible individuals is β2S0, where S0=η/μ. Further, 1/(δ+μ) expresses the average time spent by one asymptomatic individual in the asymptomatic compartment. Again, γθ/(γ+μ) is the proportion of newly asymptomatic individuals that survived the incubation period.
We employ the linearization method [39] to examine the local stability of the equilibrium points of Model (2.1).
Theorem 2
The COVID-19 free equilibrium point P0 is locally asymptotically stable if Rc<1.
Proof.
The Jacobian matrix of Model (2.1) evaluated at P0 yields
J(P0)=[−μ0−ρ(1−SD)β2(1−ε)S0−ρ(1−SD)β1S000−(γ+μ)ρ(1−SD)β2(1−ε)S0ρ(1−SD)β1S000γθ−(δ+μ)000γ(1−θ)0−(δ+d+μ)000δδ−μ]. |
By solving the characteristic equation ∣J(P0)−λI∣=0, we obtain the eigenvalues λ1,2=−μ, and λ3,4,5 are roots of the following equation:
λ3+a1λ2+a2λ+a3=0, |
where
a1=γ+d+2δ+3μ,a2=(δ+μ)(δ+d+μ)+(γ+μ)(δ+μ)[1−ρ(1−SD)(1−ε)Ra]+(γ+μ)(δ+d+μ)[1−ρ(1−SD)Rs],a3=(γ+μ)(δ+μ)(δ+d+μ)(1−Rc). |
We use the Routh-Hurwitz criteria [39] to determine the signs of the remaining eigenvalues λ3,4,5. They are negative if a1>0,a3>0 and a1a2−a3>0. Clearly, a1>0. Also, a3>0 if Rc<1. Moreover,
a1a2−a3=2(γ+μ)(δ+μ)(δ+d+μ)+(δ+μ)2(δ+d+μ)+(δ+μ)(δ+d+μ)2+((γ+μ)2(δ+d+μ)+(γ+μ)(δ+d+μ)2)[1−ρ(1−SD)Rs]+((γ+μ)2(δ+μ)+(γ+μ)(δ+μ)2)[1−ρ(1−SD)(1−ε)Ra]. |
If Rc=ρ(1−SD)[Rs+(1−ε)Ra]<1, then ρ(1−SD)Rs<1 and ρ(1−SD)(1−ε)Ra<1. Thus, a1a2−a3>0. Hence, P0 is locally asymptotically stable if Rc<1.
Theorem 3
The COVID-19 endemic equilibrium point P1 is locally asymptotically stable if Rc>1.
Proof.
The Jacobian matrix of Model (2.1) evaluated at P1 yields
J(P1)=[J110−ρ(1−SD)β2(1−ε)S1−ρ(1−SD)β1S10J21−(γ+μ)ρ(1−SD)β2(1−ε)S1ρ(1−SD)β1S100γθ−(δ+μ)000γ(1−θ)0−(δ+d+μ)000δδ−μ], |
where
J11=−ρ(1−SD)[β1Is1+β2(1−ε)Ia1]−μ,J21=ρ(1−SD)[β1Is1+β2(1−ε)Ia1]. |
The characteristic equation, ∣J(P1)−λI∣=0, has the eigenvalues λ1=−μ, and λ2,3,4,5 are the roots of the following equation:
λ4+a1λ3+a2λ2+a3λ+a4=0, | (3.3) |
where
a1=μRc+γ+d+2δ+3μ,a2=μ(δ+d+μ)Rc+μ(γ+δ+2μ)Rc+(δ+μ)(δ+d+μ)+(γ+μ)(δ+μ)[1−ρ(1−SD)(1−ε)RaRc]+(γ+μ)(δ+d+μ)[1−ρ(1−SD)RsRc],a3=μ(γ+μ)(δ+μ)(δ+d+μ)Rc+μ(δ+μ)(δ+d+μ)Rc+ηγρ(1−SD)[β1(1−θ)+β2θ(1−ε)] (1−1Rc),a4=μ(γ+μ)(δ+μ)(δ+d+μ)Rc. |
Clearly, a1>0 and a4>0. Also, since Rc=ρ(1−SD)(Rs+(1−ε)Ra)>1, then a2>0 and a3>0. By the Descartes' rule [40], the characteristic equation given by Eq (3.3) has no positive roots since there is no change in the signs of the coefficients. When substituting for (λ) by (−λ) in Eq (3.3), the signs of the coefficients change as follows:
λ4−a1λ3+a2λ2−a3λ+a4=0. |
The number of sign changes is four; therefore, the characteristic Eq (3.3) has four negative eigenvalues. Hence, P1 is locally asymptotically stable if Rc>1.
We utilize the Lyapunov and Krasovkii–LaSalle stability theorems [41,42,43] to examine the global stability of the equilibrium points of Model (2.1). Also, we use the function W(u)=u−1−lnu, which is a positive function, in the following proofs.
Theorem 4
The COVID-19 free equilibrium point P0 is globally asymptotically stable if Rc<1.
Proof.
Define the Lyapunov function L0(S,E,Ia,Is,R) as follows:
L0=S0W(S/S0)+E+(γ+μ)γ[ρ(1−SD)(1−ε)θRa+(δ+d+μ)(θd+δ+μ)(1−Rc)]Ia+(γ+μ)γ[ρ(1−SD)(1−θ)Rs+(δ+μ)(θd+δ+μ)(1−Rc)]Is+(γ+μ)(δ+μ)(δ+d+μ)γδ(θd+δ+μ)(1−Rc)R. |
If Rc<1, then L0 is positive-definite since L0(S,E,Ia,Is,R)>0 for all (S,E,Ia,Is,R)∈Ω and L0(P0)=0. Computing the time derivative of L0 along the solutions of Model (2.1), we get
dL0dt=(1−S0S)[η−ρ(1−SD)[β1SIs+β2(1−ε)SIa]−μS]+[ρ(1−SD)[β1SIs+β2(1−ε)SIa]−(γ+μ)E]+(γ+μ)γ[ρ(1−SD)(1−ε)θRa+(δ+d+μ)(θd+δ+μ)(1−Rc)][γθE−(δ+μ)Ia]+(γ+μ)γ[ρ(1−SD)(1−θ)Rs+(δ+μ)(θd+δ+μ)(1−Rc)][γ(1−θ)E−(δ+d+μ)Is]+(γ+μ)(δ+μ)(δ+d+μ)γδ(θd+δ+μ)(1−Rc)[δIa+δIs−μR]. |
Since S0=η/μ, then η=μS0. Collecting terms, we get
dL0dt=(1−S0S)(μS0−μS)−μ(γ+μ)(δ+μ)(δ+d+μ)γδ(θd+δ+μ)(1−Rc)R−[(γ+μ)−θ(γ+μ)(ρ(1−SD)(1−ε)θRa+(δ+d+μ)(θd+δ+μ)(1−Rc))−(1−θ)(γ+μ)(ρ(1−SD)(1−θ)Rs+(δ+μ)(θd+δ+μ)(1−Rc))]E−[(γ+μ)(δ+μ)γ(ρ(1−SD)(1−ε)θRa+(δ+d+μ)(θd+δ+μ)(1−Rc))−ρ(1−SD)β2(1−ε)S0−(γ+μ)(δ+μ)(δ+d+μ)γ(θd+δ+μ)(1−Rc)]Ia−[(γ+μ)(δ+d+μ)γ(ρ(1−SD)(1−θ)Rs+(δ+μ)(θd+δ+μ)(1−Rc))−ρ(1−SD)β1S0−(γ+μ)(δ+μ)(δ+d+μ)γ(θd+δ+μ)(1−Rc)]Is. |
After simplifications by using expressions of Ra and Rs, we obtain
dL0dt=−μ(S−S0)2S−μ(γ+μ)(δ+μ)(δ+d+μ)γδ(θd+δ+μ)(1−Rc)R. |
If Rc<1, then dL0/dt≤0 for all S,R>0. Also, dL0/dt=0 when S(t)=S0, and R(t)=0. Applying the Krasovkii-Lasalle theorem, we suppose that
I0={(S(t),E(t),Ia(t),Is(t),R(t)):dL0dt=0}, |
and M0 is the largest invariant subset of I0, where all elements in it satisfy S(t)=S0 and R(t)=0. Then, from the fifth equation of Model (2.1), we get
dRdt=0=δ(Ia+Is)⟹Ia(t)=0 and Is(t)=0. |
Substituting this into the third equation of Model (2.1), we have
dIadt=0=γθE⟹E(t)=0. |
Hence, M0={P0}; thus, the equilibrium P0 is globally asymptotically stable if Rc<1.
Theorem 5
The COVID-19 endemic equilibrium point P1 is globally asymptotically stable if Rc>1.
Proof.
Define the Lyapunov function L1(S,E,Ia,Is,R) as follows:
L1=S1W(S/S1)+E1W(E/E1)+ηρ(1−SD)β2(1−ε)μ(δ+μ)Rc Ia1W(I/Ia1)+ηρ(1−SD)β1μ(δ+d+μ)Rc Is1W(I/Is1). |
Clearly, L1(S,E,Ia,Is,R) is a positive semi-definite function since L1≥0 for all (S,E,Ia,Is,R)∈Ω and L1(S1,E1,Ia1,Is1,R1)=0. The time derivative of L1 along the solutions of Model (2.1) is given by
dL1dt=(1−S1S)[η−ρ(1−SD)[β1SIs+β2(1−ε)SIa]−μS]+(1−E1E)[ρ(1−SD)[β1SIs+β2(1−ε)SIa]−(γ+μ)E]+(1−Ia1Ia)ηρ(1−SD)β2(1−ε)μ(δ+μ)Rc[γθE−(δ+μ)Ia]+(1−Is1Is)ηρ(1−SD)β1μ(δ+d+μ)Rc[γ(1−θ)E−(δ+d+μ)Is]. |
From the equilibrium described by Eq (3.1) for P1, we have
η=ρ(1−SD)[β1S1Is1+β2(1−ε)S1Ia1]+μS1,(γ+μ)E1=ρ(1−SD)[β1S1Is1+β2(1−ε)S1Ia1]. |
Then,
dL1dt=−μ(S−S1)2S+3ρ(1−SD)[β1S1Is1+β2(1−ε)S1Ia1]+[γ(1−θ)ρ(1−SD)β1S1(δ+d+μ)+γθρ(1−SD)β2(1−ε)S1(δ+μ)−(γ+μ)]E−ρ(1−SD)β1S1Is1S1S−ρ(1−SD)β2(1−ε)S1Ia1S1S−ρ(1−SD)β1SIsS1Is1E1S1Is1E−ρ(1−SD)β2(1−ε)SIaS1Ia1E1S1Ia1E−γθρ(1−SD)β2(1−ε)S1(δ+μ) E1Ia1EIa1E1Ia1Ia−γ(1−θ)ρ(1−SD)β1S1(δ+d+μ) E1Is1EIs1E1Is1Is. | (3.4) |
Now, we substitute for S1=η/μRc in the coefficient of E in Eq (3.4) and use the formula of Rc. Moreover, we substitute the values of E1, 1/Ia1 and 1/Is1 in the following terms:
(γθE1(δ+μ)Ia1)ρ(1−SD)β2(1−ε)S1Ia1EIa1E1Ia=ρ(1−SD)β2(1−ε)S1Ia1EIa1E1Ia,(γ(1−θ)E1(δ+d+μ)Is1)ρ(1−SD)β1S1Is1EIs1E1Is=ρ(1−SD)β1S1Is1EIs1E1Is. |
After simplifying Eq (3.4), we obtain
dL1dt=−μ(S−S1)2S+ρ(1−SD)β2(1−ε)S1Ia1(3−S1S−SIaE1S1Ia1E−EIa1E1Ia)+ρ(1−SD)β1S1Is1(3−S1S−SIsE1S1Is1E−EIs1E1Is). |
Since the arithmetic mean is greater than or equal to the geometric mean, we have
3−S1S−SIaE1S1Ia1E−EIa1E1Ia≤0,3−S1S−SIsE1S1Is1E−EIs1E1Is≤0. |
Hence, dL1/dt≤0 for all S,E,Ia,Is>0 and dL1/dt=0 when S(t)=S1, E(t)=E1, Ia(t)=Ia1 and Is(t)=Is1. Applying the Krasovkii-Lasalle theorem, we consider the set
I1={(S(t),E(t),Ia(t),Is(t),R(t)):dL1dt=0}, |
and M1 is the largest invariant subset of I1, where all elements satisfy S(t)=S1, E(t)=E1, Ia(t)=Ia1 and Is(t)=Is1; it remains to be proven that R(t)=R1. Assume that (S(t),E(t),Ia(t),Is(t),R(t)) is a solution to Model (2.1) belonging to the set M1; thus, we have
dRdt=δ(Ia1+Is1)−μR. | (3.5) |
Solving Eq (3.5) by using the integrating factor method, we obtain
R(t)=R1+(R(0)−R1)e−μt. | (3.6) |
Note that, δ(Ia1+Is1)/μ=R1. From Eq (3.6), as time increases, R(t) approaches R1, that is, limt→∞R(t)⟶R1. The solution (S(t),E(t),Ia(t),Is(t),R(t)) will stay at the set M1; hence, M1={P1}. Therefore, the equilibrium P1 is globally asymptotically stable if Rc>1.
Here, we fit Model (2.1) to the actual data of COVID-19 cases in Saudi Arabia and estimate the parameters that make it compatible with reality. Also, we perform numerical experiments to demonstrate the agreement with the qualitative results. Furthermore, we discuss the sensitivity analysis for the control reproduction number, Rc. Finally, we examine different scenarios for the control measures in the model, namely, lockdown, social distancing and the detection of asymptomatic individuals through random diagnostic testing.
We estimate the parameters of Model (2.1) to validate it with the actual data of infected cases in Saudi Arabia. The data of the infected cases were taken from the COVID-19 dashboard of the Saudi Ministry of Health for the period of March 12, 2020 to September 23, 2020 [45].
The health care workers in the Saudi Ministry of Health have applied random diagnostic testing for asymptomatic and symptomatic individuals in dense regions. The random diagnostic tests were done between April 16, 2020 and May 3, 2020, for 42,765 individuals. The number of confirmed cases (positive test result) was 7,776 (18.18%) individuals [28]. We estimate that the effectiveness of the random diagnostic tests for the asymptomatic individuals, ε, to be approximately zero because of the insufficient information about the number of asymptomatic individuals at the time of implementing these tests. Also, the period for these tests is small compared to the time interval of the data considered from the COVID-19 dashboard.
The parameters η, μ,δ, d, ρ, SD and γ have the same values as in [37]. As for the values of the remaining parameters of Model (2.1), we estimate the value of β2 to be greater than β1 based on the model's assumption, which is similar to [10,13].
The parameter values are presented in Table 1. In Table 2, we display the values of lockdown, ρ, social distancing, SD and the corresponding values of Rc. These values were taken from [37], where Saudi Arabia implemented different levels of lockdown and social distancing, which led to dividing the study period into seven phases.
Parameter | Description | Value | Unit | Source |
N | Population of Saudi Arabia | 34218169 | Individual | [44] |
η | Birth rate | 1250 | Individual×Day−1 | [37] |
μ | Natural death rate | 3.6529×10−5 | Day−1 | [37] |
β1 | Transmission rate by Is | 0.0554×10−7 | (Individual×Day)−1 | Estimated |
β2 | Transmission rate by Ia | 1.0596×10−7 | (Individual×Day)−1 | Estimated |
γ | Incubation rate | 1/6 | Day−1 | [9] |
θ | Probability of becoming Ia | 0.54 | − | Estimated |
ε | Effectiveness of random diagnostic testing for Ia | 0 | − | Estimated |
δ | Recovery rate | 3.2772×10−1 | Day−1 | [37] |
d | Death rate due to COVID-19 | 2.3724×10−1 | Day−1 | [37] |
Phase | Time | ρ | SD | Rc |
Phase 1 | t1=[12,22] | 0.85 | 0.15 | 4.4267 |
Phase 2 | t2=[22,25] | 0.75 | 0.30 | 3.2166 |
t3=[25,28] | 0.65 | 0.45 | 2.1904 | |
t4=[28,36] | 0.60 | 0.55 | 1.6543 | |
Phase 3 | t5=[36,56] | 0.55 | 0.55 | 1.5164 |
Phase 4 | t6=[56,67] | 0.55 | 0.60 | 1.3479 |
t7=[67,83] | 0.55 | 0.69 | 1.0446 | |
Phase 5 | t8=[83,88] | 0.40 | 0.80 | 0.4902 |
Phase 6 | t9=[88,92] | 0.65 | 0.70 | 1.1947 |
t10=[92,112] | 0.75 | 0.70 | 1.3785 | |
Phase 7 | t11=[112,120] | 0.80 | 0.76 | 1.1764 |
t12=[120,130] | 0.80 | 0.80 | 0.9803 | |
t13=[130,144] | 0.80 | 0.82 | 0.8823 | |
t14=[144,169] | 0.85 | 0.83 | 0.8853 | |
t15=[169,179] | 0.85 | 0.82 | 0.9374 | |
t16=[179,193] | 0.90 | 0.82 | 0.9926 | |
t17=[193,204] | 0.95 | 0.85 | 0.8731 | |
t18=[204,207] | 0.95 | 0.88 | 0.6985 |
Model (2.1) was solved numerically by using the MATLAB package ode45 with the following initial values: S(0)=34813577, E(0)=150, Ia(0)=95, IS(0)=44 and R(0)=1. We assumed that each initial value for the exposed class was set to be greater than those for the infected classes, as in [13,23,46]. Also, each initial value of the asymptomatic class was set to be greater than those for the symptomatic class based on the results in [8], which demonstrated that most of the COVID-19 cases at the beginning of the spread in Saudi Arabia were asymptomatic and returning from imported. Moreover, Li et al. [6] estimated the undocumented infections (infections with mild, limited or loss of symptoms) to be 86% of the infections in China before imposing travel restrictions on January 23, 2020.
The results of fitting Model (2.1) to the COVID-19 infected cases (symptomatic and asymptomatic) in Saudi Arabia from March 12, 2020 to September 23, 2020 are illustrated in Figure 2, which shows good agreement.
This section demonstrates the agreement between the numerical solution of Model (2.1) and the qualitative analysis offered in Section 3. We conducted numerical simulations of the model for different initial values in the feasible set to show the tendency of the solution curves to equilibrium P0 if Rc<1, or equilibrium P1 if Rc>1. We start by rescaling the state variables in the model. Let
S=ˉSN, E=ˉEN, Ia=¯IaN, Is=¯IsN, R=ˉRN. | (4.1) |
Thus, the rescaled Model (2.1) becomes (omitting the bar onward)
dSdt=μ−ρ(1−SD)ημ[β1SIs+β2(1−ε)SIa]−μS,dEdt=ρ(1−SD)ημ[β1SIs+β2(1−ε)SIa]−(γ+μ)E,dIadt=γθE−(δ+μ)Ia,dIsdt=γ(1−θ)E−(δ+d+μ)Is,dRdt=δ(Ia+Is)−μR. | (4.2) |
Note that we have used the limiting value of N in the model, i.e., N=η/μ. We numerically solve Model (4.2) with parameters chosen to satisfy the stability conditions from the qualitative analysis, and with the following different initial conditions belonging to the set Ω:
IC1: S(0)=0.8, E(0)=0.1, Ia(0)=0.05, Is(0)=0.02, R(0)=0.01,
IC2: S(0)=0.6, E(0)=0.2, Ia(0)=0.08, Is(0)=0.07, R(0)=0.05,
IC3: S(0)=0.4, E(0)=0.3, Ia(0)=0.12, Is(0)=0.10, R(0)=0.07.
Experiment1: Assume the parameters of Model (4.2) have the following values: μ=0.04, η=1250, γ=0.167, β1=0.0554×10−4, β2=1.0596×10−4, ε=0.50, θ=0.54, δ=3.2772×10−1, d=2.3724×10−1, ρ=0.5 and SD=0.75. Thus, Rc=0.2585<1. Therefore, we expect that the solution curves of the model tend to the COVID-19 free equilibrium point P0=(1, 0, 0, 0, 0). This is evident in Figure 3; the numerical solutions eventually reach P0 for different initial conditions. Accordingly, COVID-19 diminishes.
Experiment2: In this experiment, we increased the value of the parameter ρ and decreased the values of the parameters ε and SD. That is to say, the control measures of lockdown, social distancing and random diagnostic testing were reduced. We assume the parameters of Model (4.2) have the following values: μ=0.04, η=1250, γ=0.167, β1=0.0554×10−4, β2=1.0596×10−4, ε=0.10, θ=0.54, δ=3.2772×10−1, d=2.3724×10−1, ρ=0.85 and SD=0.30. Therefore, Rc=2.1639>1. Hence, we expect the solution curves of the model to approach the COVID-19 endemic equilibrium point P1. This is displayed in Figure 4; the numerical solutions eventually tend to P1=(0.4621, 0.1039, 0.0255, 0.0132, 0.3170) for different initial conditions. Consequently, COVID-19 remains at a specific percentage.
We conclude from the experiments that the numerical results are in good agreement with the qualitative results.
To determine which parameters influence the spread of COVID-19, we investigated the sensitivity of the control reproduction number for Model (2.1). The sensitivity of Rc was examined analytically by evaluating ∂Rc/∂P, where P=(η,β1,β2,ρ,SD,γ,δ,ε,θ,d,μ). The changes in Rc corresponding to one parameter at a time are as follows:
∂Rc∂ε=−ηγρ(1−SD)β2θμ(γ+μ)(δ+μ)<0,∂Rc∂β1=ηγρ(1−SD)(1−θ)μ(γ+μ)(δ+d+μ)>0,∂Rc∂β2=ηγρ(1−SD)θ(1−ε)μ(γ+μ)(δ+μ)>0,∂Rc∂d=−ηγρ(1−SD)β1(1−θ)μ(γ+μ)(δ+d+μ)2<0,∂Rc∂SD=−ηγρμ(γ+μ)[β1(1−θ)(δ+d+μ)+β2θ(1−ε)(δ+μ)]<0,∂Rc∂η=γρ(1−SD)μ(γ+μ)[β1(1−θ)(δ+d+μ)+β2θ(1−ε)(δ+μ)]>0,∂Rc∂γ=ηρ(1−SD)(γ+μ)2[β1(1−θ)(δ+d+μ)+β2θ(1−ε)(δ+μ)]>0,∂Rc∂ρ=ηγ(1−SD)μ(γ+μ)[β1(1−θ)(δ+d+μ)+β2θ(1−ε)(δ+μ)]>0,∂Rc∂δ=−ηγρ(1−SD)μ(γ+μ)[β1(1−θ)(δ+d+μ)2+β2θ(1−ε)(δ+μ)2]<0,∂Rc∂θ=ηγρ(1−SD)μ(γ+μ)[β2(1−ε)(δ+μ)−β1(δ+d+μ)]=ηγρ(1−SD)β2μ(γ+μ)(δ+μ)(1−ω)>0when ω<1(<0 when ω>1),∂Rc∂μ=−ηγρ(1−SD)(γ+2μ)μ2(γ+μ)2[β1(1−θ)(δ+d+μ)+β2θ(1−ε)(δ+μ)]−ηγρ(1−SD)μ(γ+μ)[β1(1−θ)(δ+d+μ)2+β2θ(1−ε)(δ+μ)2]<0, | (4.3) |
where ω=ε+(β1(δ+μ)/β2(δ+d+μ)). From Eq (4.3), we see that an increase in ε, SD, δ, d or μ leads to a decrease in Rc. Conversely, an increase in β1, β2, ρ, γ or η leads to an increase in Rc. Finally, the sensitivity of Rc with respect to θ depends on the value of ω. If ω>1, then Rc decreases, and if ω<1, Rc increases. Figure 5 illustrates these results.
The normalized sensitivity index (elasticity) of Rc with respect to the model parameters P is defined as follows [47]:
ΓPRc=∂Rc∂PPRc. | (4.4) |
By applying the formula in Eq (4.4), we get
ΓηRc=1,ΓρRc=1,ΓγRc=μγ+μ,ΓSDRc=−SD1−SD,ΓθRc=β2θ(1−ε)(δ+d+μ)−β1θ(δ+μ)β2θ(1−ε)(δ+d+μ)+β1(1−θ)(δ+μ),Γβ1Rc=β1(1−θ)(δ+μ)β1(1−θ)(δ+μ)+β2θ(1−ε)(δ+d+μ),Γβ2Rc=β2θ(1−ε)(δ+d+μ)β1(1−θ)(δ+μ)+β2θ(1−ε)(δ+d+μ),ΓεRc=−β2εθ(δ+d+μ)β1(1−θ)(δ+μ)+β2θ(1−ε)(δ+d+μ),ΓdRc=−dβ1(1−θ)(δ+μ)β1(1−θ)(δ+μ)(δ+d+μ)+β2θ(1−ε)(δ+d+μ)2,ΓδRc=−δ(δ+μ)(δ+d+μ)[β1(1−θ)(δ+μ)2+β2θ(1−ε)(δ+d+μ)2β1(1−θ)(δ+μ)+β2θ(1−ε)(δ+d+μ)],ΓμRc=−(γ+2μ)(γ+μ)−μ(δ+μ)(δ+d+μ)[β1(1−θ)(δ+μ)2+β2θ(1−ε)(δ+d+μ)2β1(1−θ)(δ+μ)+β2θ(1−ε)(δ+d+μ)]. |
Table 3 exhibits the elasticity of Rc with respect to the model parameters. The parameter values were taken from Table 1, in addition to ρ=0.80, SD=0.60 and ε=0.40. Any positive value for the elasticity in Table 3 means that the contribution of the parameter to Rc is to increase its value. For instance, Γβ2Rc=0.9587 means that an increase in β2 by 1% will increase Rc by 0.9587%. On the contrary, any negative value for the elasticity means that the contribution of the parameter to Rc is to decrease its value. For example, ΓεRc=−0.6391 means that an increase in ε by 1% will decrease Rc by 0.6391%. We notice that the most sensitive parameters are β2, ρ, SD and ε. Therefore, the increase in imposing social distancing and lockdown measures and detecting asymptomatic individuals through random diagnostic testing reduces the spread of the disease.
Parameter P | Value | Sensitivity index ΓPRc |
η | 1250 | 1 |
β1 | 0.0554×10−7 | 0.0413 |
β2 | 1.0596×10−7 | 0.9587 |
γ | 0.167 | 0.0002 |
θ | 0.54 | 0.9103 |
δ | 3.2772×10−1 | −0.9826 |
d | 2.3724×10−1 | −0.0173 |
μ | 3.653×10−5 | −1.0003 |
ρ | 0.80 | 1 |
ε | 0.40 | −0.6391 |
SD | 0.10 | −0.1111 |
0.30 | −0.4286 | |
0.50 | −1 | |
0.70 | −2.3333 | |
0.90 | −9 | |
0.99 | −99 |
Model (2.1) examines some of the policies that have been applied in Saudi Arabia to control COVID-19, namely, the effectiveness of random diagnostic testing on the asymptomatic class (ε), lockdown (ρ) and social distancing (SD). We executed several scenarios to investigate the impact of these policies by simulating Model (2.1) for infected cases. Figure 2 shows the actual data in Saudi Arabia and the fitting curve for Model (2.1) for infected cases from March 12, 2020 to September 23, 2020. We solve Model (2.1) numerically with the following initial values: S(0)=34813577, E(0)=150, Ia(0)=95, Is(0)=44, R(0)=1 and the parameter values given in Table 1. The parameters ε, ρ and SD took different values for each scenario. We describe different scenarios in Table 4. For the fitting values of ρ and SD, refer to Table 2.
Scenario | ε | ρ | SD |
1st | Different values | Fitting values | Fitting values |
2nd | Different values | No lockdown (ρ=1) | No social distancing (SD=0) |
3rd | Different values | Different values | Fitting values |
4th | Different values | Fitting values | Different values |
5th | Different values | 0.85 | Different values |
6th | Different values | 0.75 | Different values |
7th | Different values | Fitting values | Fitting values |
for three time periods |
The results of applying the scenarios (1st−7th) are summarized in Tables 5–11 and illustrated in Figures 6–12. We compared the scenarios with the peak value of the infected cases in the fitting of Model (2.1), that is, 59,830, which occured on Day 123.
ρ | SD | ε | Peak (day) | Cases | Percentage Change |
Fitting values | Fitting values | 0.01 | 122 | 52,010 | −13.07% |
0.02 | 122 | 45,140 | −24.55% | ||
0.03 | 122 | 39,120 | −34.61% | ||
0.04 | 122 | 33,830 | −43.45% | ||
0.10 | 121 | 13,770 | −76.98% | ||
0.20 | 68 | 4,159 | −93.04% | ||
0.30 | 58 | 1,556 | −97.39% | ||
0.40 | 37 | 705 | −98.82% |
ρ | SD | ε | Peak (day) | Cases | Percentage Change |
1 | 0 | 0.10 | 53 | 4,728,000 | +7802.39% |
0.20 | 57 | 4,420,000 | +7287.60% | ||
0.30 | 62 | 4,057,000 | +6680.88% | ||
0.40 | 69 | 3,607,000 | +5928.75% | ||
0.50 | 79 | 3,042,000 | +4984.41% | ||
0.60 | 97 | 2,332,000 | +3797.71% | ||
0.70 | 133 | 1,431,000 | +2291.78% | ||
0.80 | 266 | 391,700 | +554.68% |
ρ | SD | ε | Peak (day) | Cases | Percentage Change |
1 | Fitting values | 0.10 | 84 | 1,163,000 | +1843.84% |
0.20 | 112 | 630,200 | +953.31% | ||
0.30 | 115 | 195,700 | +227.09% | ||
0.40 | 113 | 27,360 | −54.27% | ||
0.85 | Fitting values | 0.10 | 113 | 454,000 | +658.81% |
0.20 | 115 | 127,900 | +113.77% | ||
0.30 | 113 | 22,410 | −62.54% | ||
0.40 | 77-83 | 4,208 | −92.96% | ||
0.75 | Fitting values | 0.10 | 115 | 110,600 | +84.85% |
0.20 | 113 | 23,550 | −60.63% | ||
0.30 | 83 | 5,273 | −91.18% | ||
0.40 | 68 | 1,514 | −97.46% | ||
0.55 | Fitting values | 0.10 | 70 | 2,882 | −95.18% |
0.20 | 68 | 1,175 | −98.03% | ||
0.30 | 58 | 474 | −99.20% | ||
0.40 | 30 | 244 | −99.59% |
ρ | SD | ε | Peak (day) | Cases | Percentage Change |
Fitting values | 0.40 | 0.10 | 124 | 2,314,000 | +3767.62% |
0.20 | 138,139 | 2,035,000 | +3301.30% | ||
0.30 | 160 | 1,764,000 | +2848.35% | ||
0.40 | 194 | 1,441,000 | +2308.49% | ||
Fitting values | 0.50 | 0.10 | 150 | 1,874,000 | +3032.21% |
0.20 | 171 | 1,629,000 | +2622.71% | ||
0.30 | 203 | 1,434,000 | +2296.79% | ||
0.40 | 247 | 1,121,000 | +1773.64% | ||
Fitting values | 0.60 | 0.10 | 198 | 1,439,000 | +2305.15% |
0.20 | 229 | 1,271,000 | +2024.35% | ||
0.30 | 279 | 902,300 | +1408.11% | ||
0.40 | 377 | 506,400 | +746.39% | ||
Fitting values | 0.70 | 0.10 | 302 | 776,200 | +1197.34% |
0.20 | 387 | 480,900 | +703.77% | ||
0.30 | 572 | 213,800 | +257.34% | ||
0.40 | 1402, 1408 | 28,830 | −51.81% |
ρ | SD | ε | Peak (day) | Cases | Percentage Change |
0.85 | 0.40 | 0.10 | 88 | 2,674,000 | +4369.33% |
0.20 | 98 | 2,284,000 | +3717.48% | ||
0.30 | 114 | 1,847,000 | +2987.08% | ||
0.40 | 138 | 1,357,000 | +2168.09% | ||
0.85 | 0.50 | 0.10 | 106 | 2,052,000 | +3329.72% |
0.20 | 121,122 | 1,666,000 | +2684.56% | ||
0.30 | 145 | 1,247,000 | +1984.24% | ||
0.40 | 185,186 | 801,700 | +1239.96% | ||
0.85 | 0.60 | 0.10 | 141 | 1,310,000 | +2089.54% |
0.20 | 168,169 | 957,200 | +1499.87% | ||
0.30 | 216 | 599,300 | +901.67% | ||
0.40 | 320 | 265,700 | +344.09% | ||
0.85 | 0.70 | 0.10 | 240 | 488,600 | +716.64% |
0.20 | 331,332 | 246,000 | +311.16% | ||
0.30 | 600 | 60,790 | +1.60% | ||
0.40 | 12 | 139 | −99.76% |
ρ | SD | ε | Peak (day) | Cases | Percentage Change |
0.75 | 0.40 | 0.10 | 100 | 2,246,000 | +3653.97% |
0.20 | 113 | 1,859,000 | +3007.14% | ||
0.30 | 133,134 | 1,430,000 | +2290.11% | ||
0.40 | 167 | 967,500 | +1517.08% | ||
0.75 | 0.50 | 0.10 | 123 | 1,630,000 | +2624.39% |
0.20 | 144 | 1,259,000 | +2004.30% | ||
0.30 | 178 | 867,000 | +1349.11% | ||
0.40 | 242 | 475,400 | +694.58% | ||
0.75 | 0.60 | 0.10 | 172 | 924,900 | +1445.88% |
0.20 | 214,215 | 608,800 | +917.55% | ||
0.30 | 297 | 310,600 | +419.13% | ||
0.40 | 550 | 75,830 | +26.74% | ||
0.75 | 0.70 | 0.10 | 344 | 226,300 | +278.23% |
0.20 | 585-588 | 64,690 | +8.12% | ||
0.30 | 12 | 139 | −99.76% | ||
0.40 | 12 | 139 | −99.76% |
Event | ε1 | ε2 | ε3 | Peak (day) | Cases | Percentage Change |
Ⅰ | 0.10 | 0 | 0 | 123 | 38,480 | −35.68% |
Ⅱ | 0.10 | 0.10 | 0 | 124 | 20,890 | −65.08% |
Ⅲ | 0 | 0.10 | 0.10 | 121 | 21,920 | −63.36% |
Ⅳ | 0.20 | 0 | 0 | 124 | 23,650 | −60.47% |
Ⅴ | 0.20 | 0.20 | 0 | 125 | 6,539 | −89.07% |
Ⅵ | 0 | 0.20 | 0.20 | 68 | 11,470 | −80.82% |
Ⅶ | 0.10 | 0.20 | 0 | 125 | 10,870 | −81.83% |
Ⅷ | 0.10 | 0.20 | 0.10 | 121 | 7,132 | −88.07% |
Ⅸ | 0.20 | 0.10 | 0 | 124,125 | 12,680 | −78.80% |
Ⅹ | 0.20 | 0.10 | 0.10 | 121 | 8,326 | −86.08% |
Ⅺ | 0 | 0.20 | 0.10 | 121 | 11,480 | −80.81% |
The 1st scenario examines the impact of detecting asymptomatic individuals by applying random diagnostic testing in Saudi Arabia. At the same time, the lockdown and social distancing are kept as the fitting values. Figure 6 illustrates that the peak of infected cases decreases with increasing ε. For instance, when detecting only 2% of the asymptomatic individuals (ε=0.02) by random diagnostic testing, the cases will reach 45,140 on Day 122. Compared with actual data, the number of cases decreased by 24.55% (see Table 5). This indicates the importance of applying the random diagnostic testing to find asymptomatic individuals and reduce the cases.
The 2nd scenario was applied to determine whether it is possible to rely only on detecting asymptomatic individuals through random diagnostic testing without imposing social distancing and a lockdown. Figure 7 shows that the infected cases will far surpass the actual data. For example, if the detection of asymptomatic cases reaches 60% (ε=0.60), the peak of cases will be 2,332,000 on Day 97 (see Table 6). That is an increase of 3797.71% relative to the peak value of the actual data. We concluded that detecting asymptomatic individuals is insufficient without imposing social distancing and lockdown.
The 3rd scenario analyzes four different values for the level of lockdown (ρ=1, 0.85, 0.75, 0.55) the values for social distancing were kept as the fitting values. When ρ=1, there is no lockdown implementation. The remaining values of ρ correspond to the lockdown level undertaken by Saudi Arabia in Phase 1, Phase 2 and Phase 4, respectively [37].
Figure 8 shows that increasing the lockdown level leads to a decrease in the infected cases. Note that increasing the lockdown level means decreasing the value of ρ. Moreover, increasing the lockdown level requires a smaller value for detecting asymptomatic individuals through random diagnostic testing to reach case numbers lower than the actual data. To explain further, when the lockdown level is equal to 1, 0.85, 0.75 or 0.55, we need to apply a 40, 30, 20 or 10% level of random diagnostic testing, respectively, to detect the asymptomatic individuals (see Table 7).
The 4th scenario investigates the impact of social distancing while keeping the value of ρ equal to the fitting values. Figure 9 illustrates that increasing the application of social distancing delays peak days; however, only when SD=0.70 and ε=0.40 does the cases reach a value that is lower than the actual data by 51.81%, i.e., 28,830 (see Table 8).
The 5th and 6th scenarios examine the impact of imposing a lockdown at low levels and relying on social distancing and random diagnostic testing. We let ρ=0.85 and 0.75 for the 5th and 6th scenarios, respectively. Figures 10 and 11 show that it is possible to reach a lower number of infected cases than in the actual data if SD=0.70, where ε=0.40 for the case where ρ=0.85 (see Table 9), and ε=0.30 for the case where ρ=0.75 (see Table 10). This indicates that enforcing low lockdown levels is effective with a high level of social distancing implementation and 30% or more of the detection of asymptomatic individuals through random diagnostic testing.
The 7th scenario was designed to determine the effect of different values of ε through the phases. We assume that the value of ε is ε1 in Phase 1 to Phase 2, ε2 in Phase 3 to Phase 5 and ε3 in Phase 6 to Phase 7 (see Table 11). Figure 12(a) and (b) show that performing random diagnostic tests in the middle phases (Phase 3–5) and the beginning phases (Phase 1 and 2), rather than the last phases (Phase 6 and 7), gives better results. Furthermore, applying the tests in all phases reduces the number of cases, as seen in Figure 12(c).
We conclude from the analysis of the 1st to the 6th scenarios that applying random diagnostic testing alone is inadequate without imposing social distancing and lockdown. The importance of using random diagnostic testing to find asymptomatic individuals is apparent when lockdown and social distancing measures are enforced; only then does the number of cases decline. An increase in the level of lockdown decreases the required percentage of random diagnostic tests to lower the number of cases. However, an increase in social distancing does not have the same effect as a lockdown; a high percentage of random diagnostic tests is needed to reduce the number of cases. On the other hand, implementation of lower levels of lockdown is possible only when a high level of social distancing and a high percentage of random diagnostic tests are imposed.
As for the 7th scenario, we deduce that performing the random diagnostic tests in all phases is effective in lowering the number of cases. However, applying the random diagnostic tests in the early and middle phases is sufficient to reduce the cost of conducting these tests.
This study was purposed to develop a mathematical model to examine the impact of random diagnostic testing on COVID-19 patients in the presence of a lockdown and social distancing in Saudi Arabia. Qualitative and numerical analyses were applied to the model. The model was well posed and had two equilibrium points. The COVID-19 free equilibrium existed and was locally and globally asymptotically stable if Rc<1. Conversely, the COVID-19 endemic equilibrium existed and was locally and globally asymptotically stable if Rc>1.
The model was validated by using the data from the COVID-19 dashboard of the Saudi Ministry of Health spanning March 12, 2020 to September 23, 2020. In addition, the numerical experiments performed using the model showed the consistency of the numerical solutions with the qualitative analysis.
Furthermore, the sensitivity analysis for Rc revealed that the most influential parameter in terms of increasing Rc was the transmission rate due to contact with asymptomatic individuals (β2). However, the control parameters, i.e., the lockdown (ρ), social distancing (SD) and the effectiveness of random diagnostic testing for asymptomatic individuals (ε), played a significant role in decreasing Rc. Finally, we analyzed different scenarios numerically for the control strategies applied in Saudi Arabia.
We concluded that applying random diagnostic testing to detect asymptomatic individuals in the presence of a lockdown and social distancing significantly reduced the cases. On the other hand, it was impossible to rely on random diagnostic testing without imposing a lockdown and social distancing. Moreover, implementing a lockdown at low levels required increased social distancing levels and a high percentage of random diagnostic testing.
The authors declare that there is no conflict of interest.
[1] | E. O. Wilson, Sociobiology: The New Synthesis, Belknap Press, 1975. |
[2] | R. A. Hinde, Ethology: Its Nature and Relation with Other Sciences, Oxford University Press, 1982. |
[3] | H. Whitehead, Analyzing Animal Societies, University of Chicago Press, 2008. |
[4] | A. F. Fraser, D. M. Broom, Farm Animal Behaviour and Welfare, CAB International, 1997. |
[5] | E. O. Price, Animal Domestication and Behaviour, CABI Publishing, 2002. |
[6] | R. A. Hinde, Primate Social Relationships, Blackwell Scientific Publications, 1983. |
[7] | T. T. Strusaker, Correlates of ecology and social organization among African cercopithecines, Folia Primatol., 11 (1969), 80-118. |
[8] | S. R. Sundaresan, I. R. Fishhoff, J. Dushoff, D. I. Rubenstein, Network metrics reveal differences in social organization between two fission-fusion species, Grevy's zebra and onager, Oecologia, 151 (2007), 140-149. |
[9] | D. P. Croft, R. James, J. Krause, Exploring Animal Social Networks, Princeton University Press, 2008. |
[10] | T. Wey, D. T. Blumstein, W. Shen, F. Jordan, Social network analysis of animal behaviour: A promising tool for the study of sociality, Anim. Behav., 75 (2008), 333-344. |
[11] | C. Kasper, B. Voelkl, A social network analysis of primate groups, Primates, 50 (2009), 343-356. |
[12] | J. Moreno, Who Shall Survive?, Beacon, 1934. |
[13] | J. Scott, P. J. Carrington, The SAGE Handbook of Social Network Analysis, SAGE Publications, 2011. |
[14] | G. A. Lundberg, M. Steel, Social attraction-patterns in a village, Sociometry, 1 (1938), 375-419. |
[15] | J. A. Barnes, Class and committee in a Norwegian island parish, Hum. Relat., 7 (1954), 39-58. |
[16] | J. H. Levine, The sphere of influence, Am. Sociol. Rev., 37 (1972), 14-27. |
[17] | M. Granowetter, The strength of weak ties, Am. J. Sociol., 78 (1973), 1360-1380. |
[18] | B. Ryan, N. C. Gross, The diffusion of hybrid seed corn in two Iowa communities, Rural Sociol., 8 (1943), 15-24. |
[19] | E. Katz, H. Levine, M. L. Hamilton, Traditions of research on the diffusion of innovation, Am. Sociol. Rev., 28 (1963), 237-253. |
[20] | S. Milgram, The small-world problem, Psychol. Today, 2 (1964), 60-67. |
[21] | E. M. Rogers, Diffusion of Innovations, Free Press, 2003. |
[22] | M. Bond, Social influences on corporate political donations in Britain, Brit. J. Sociol., 55 (2004), 55-77. |
[23] | D. Knoke, Policy Networks, in The SAGE Handbook of Social Network Analysis (eds. J. Scott, P. J. Carrington), SAGE Publications, 2011, 210-222. |
[24] | M. Diani, Social Movements and collective actions, in The SAGE Handbook of Social Network Analysis (eds. J. Scott, P. J. Carrington), SAGE Publications, 2011, 223-235. |
[25] | M. O. Jackson, Social and Economic Networks, Princeton University Press, 2008. |
[26] | A. L. Barabâsi, J. Hawoong, N. Zoltan, R. Erzsebet, A. Schubert, T. Vicsek, Evolution of the social network of scientific collaborations, Phys. A, 311 (2002), 590-614. |
[27] | M. E. Newman, Coauthorship networks and patterns of scientific collaboration, Proc. Natl. Acad. Sci. USA, 101 (2004), 5200-5205. |
[28] | D. J. Watts, S. H. Strogatz, Collective dynamics of "small-world" networks, Nature, 393 (1998), 440-442. |
[29] | M. E. Newman, Networks: An Introduction, Oxford University Press, 2010. |
[30] | H. Kummer, Soziales Verhalten einer Mantelpavianen-Gruppe, Schweizerische Zeitschr. Psychol., 33 (1957), 1-91. |
[31] | D. S. Sade, Some aspects of parent-off spring and sibling relations in a group of rhesus monkeys, with a discussion of grooming, Am. J. Phys. Anthropol., 23 (1965), 1-18. |
[32] | R. A. Hinde, Interactions, relationships and social structure, Man, 11 (1976), 1-17. |
[33] | R. W. Byrne, A. Whiten, S. P. Henzi, Social relationships of mountain baboons: Leadership and affiliation in a non-female-bonded monkey, Am. J. Primatol., 207 (1989), 191-207. |
[34] | B. D. Chepko-sade, K. P. Reitz, D. S. Sade, Sociometrics of Macaca mulatta IV: Network analysis of social structure of a pre-fission group, Soc. Netw., 11 (1989), 293-314. |
[35] | C. A. Chapman, Association patterns of spider monkeys: The influence of ecology and sex on social organization, Behav. Ecol. Sociobiol., 26 (1990), 409-414. |
[36] | C. P. Yeager, Proboscis monkey (Nasalis larvatus) social organization: Group structure, Am. J. Primatol., 106 (1990), 95-106. |
[37] | D. S. Sade, Sociometrics of Macaca mulatta I. Linkage, cliques in grooming matrices, Fol. Primatol., 18 (1972), 196-223. |
[38] | D. S. Sade, Sociometrics of Macaca mulatta Ⅲ. n-path centrality in grooming networks, Soc. Netw., 11 (1989), 273-292. |
[39] | D. S. Sade, M. Altmann, J. Loy, G. Hausfater, J. A. Breuggeman, Sociometrics of Macaca mulatta: Ⅱ. Decoupling centrality and dominance in rhesus monkey social networks, Am. J. Phys. Anthropol., 77 (1988), 409-425. |
[40] | S. Wasserman, K. Faust, Social Network Analysis: Methods and Applications, Cambridge University Press, 1994. |
[41] | M. Barthelemy, B. Gondran, E. Guichard, Spatial structure of the internet traffic, Phys. A, 319 (2003), 633-642. |
[42] | P. Bonacich, Factoring and weighting approaches to status scores and clique identification, J. Math. Sociol., 2 (1972), 113-120. |
[43] | M. E. J. Newman, A measure of betweenness centrality based on random walks, Soc. Netw., 27 (2005), 39-54. |
[44] | M. E. J. Newman, M. Girvan, Finding and evaluating community structure in networks, Phys. Rev. E, 69 (2004), 026113. |
[45] | A. Clauset, Finding local community structure in networks, Phys. Rev. E, 72 (2005), 026132. |
[46] | M. E. J. Newman, Modularity and community structure in networks, Proc. Natl. Acad. Sci. USA, 103 (2006), 8577-8582. |
[47] | D. Knoke, S. Yang, Social network analysis, Sage Publications, 2019. |
[48] | D. Lusseau, The emergent properties of a dolphin social network, Biol. Lett., 270 (2003), 186-188. |
[49] | D. P. Croft, J. Krause, R. James, Social networks in the guppy (Poecilia reticulata), Biol. Lett., 271 (2004), 516-519. |
[50] | B. Voelkl, Does group structure influence the social transmission of information?, Fol. Primatol., 75 (2004), 423. |
[51] | D. P. Croft, R. James, A. J. W. Ward, M. S. Botham, D. Mawdsley, J. Krause, Assortative interactions and social networks in fish, Oecologia, 143 (2005), 211-219. |
[52] | J. C. Flack, M. Girvan, F. B. M. de Waal, D. C. Krakauer, Policing stabilizes construction of social niches in primates, Nature, 439 (2006), 426-429. |
[53] | C. Sueur, O. Petit, Organization of group members at departure Is driven by social structure in Macaca, Int. J. Primatol., 29 (2008), 1085-1089. |
[54] | S. P. Henzi, D. Lusseau, T. Weingrill, Cyclicity in the structure of female baboon social networks, Behav. Ecol. Sociobiol., 63 (2009), 1015-1021. |
[55] |
J. Lehmann, C. Boesch, Sociality of the dispersing sex: The nature of social bonds in West African female chimpanzees, Pan troglodytes, Anim. Behav., 77 (2009), 377-387. doi: 10.1016/j.anbehav.2008.09.038
![]() |
[56] | J. Lehmann, R. I. M. Dunbar, Network cohesion, group size and neocortex size in female-bonded Old World primates, Proc. R. Soc. B, 276 (2009), 4417-4422. |
[57] | G. Ramos-Fernández, D. Boyer, F. Aureli, L. G. Vick, Association networks in spider monkeys (Ateles geoffroyi), Behav. Ecol. Sociobiol., 63 (2009), 999-1013. |
[58] | N. J. B. Boogert, S. M. Reader, W. Hoppitt, K. N. Laland, The origin and spread of innovations in starlings, Anim. Behav., 75 (2008), 1509-1518. |
[59] | B. Voelkl, R. Noë, The influence of social structure on the propagation of social information in artificial primate groups: A graph-based simulation approach, J. Theoret. Biol., 252 (2008), 77-86. |
[60] | M. Franz, C. L. Nunn, Network-based diffusion analysis: A new method for detecting social learning, Proc. R. Soc. B, 276 (2009), 1829-1836. |
[61] | C. Vital, P. Martins, Using graph theory metrics to infer information flow through animal social groups: A computer simulation analysis, Ethology, 115 (2009), 347-355. |
[62] | W. Hoppitt, A. Kandler, J. R. Kendal, K. N. Laland, The effect of task structure on diffusion dynamics: Implications for diffusion curve and network-based analyses, Learn. Behav., 38 (2010), 243-251. |
[63] | L. M. Aplin, D. R. Farine, J. Morand-Ferron, A. Cockburn, A. Thornton, B. C. Sheldon, Experimentally induced innovations lead to persistent culture via conformity in wild birds, Nature, 7540 (2015), 538. |
[64] | B. Voelkl, C. Kasper, Social structure of primate interaction networks facilitates the emergence of cooperation, Biol. Lett., 5 (2009), 462-464. |
[65] | B. Voelkl, The "Hawk-Dove" game and the spread of the evolutionary process in small heterogeneous populations, Games, 1 (2010), 103-116. |
[66] | B. Voelkl, The evolution of generalized reciprocity in social interaction networks, Theoret. Popul. Biol., 104 (2015), 17-25. |
[67] | B. Mccowan, K. Anderson, A. Heagarty, A. Cameron, Utility of social network analysis for primate behavioral management and well-being, Appl. Anim. Behav. Sci., 109 (2008), 396-405. |
[68] | B. A. Beisner, M. E. Jackson, A. Cameron, B. Mccowan, Effects of natal male alliances on aggression and power dynamics in rhesus macaques, Am. J. Primatol., 801 (2011), 790-801. |
[69] | V. Dufour, C. Sueur, A. Whiten, The impact of moving to a novel environment on social networks, activity and wellbeing in two new world primates, Am. J. Primatol., 811 (2011), 802-811. |
[70] | M. C. Crofoot, D. I. Rubenstein, A. S. Maiya, T. Y. Berger-wolf, Aggression, grooming and group-level cooperation in white-faced capuchins (Cebus capucinus): Insights from social networks, Am. J. Primatol., 833 (2011), 821-833. |
[71] | B. Tiddi, F. Aureli, G. Schino, B. Voelkl, Social relationships between adult females and the alpha male in wild tufted capuchin monkeys, Am. J. Primatol., 73 (2011), 812-820. |
[72] | A. J. J. MacIntosh, A. Jacobs, C. Garcia, K. Shimizu, K. Mouri, M. A. Huffman, et al., Monkeys in the middle: Parasite transmission through the social network of a wild primate, PLoS One, 7 (2012), e51144. |
[73] | L. J. N. Brent, S. Semple, Social capital and physiological stress levels in free-ranging adult female rhesus macaques, Behaviour, 102 (2011), 76-83. |
[74] | P. C. Lopes, P. Block, B. König, Infection-induced behavioural changes reduce connectivity and the potential for disease spread in wild mice contact networks, Sci. Rep., 6 (2016), 31790. |
[75] | M. Dow, F. B. M. de Waal, Assignment methods for the analysis of network subgroup interactions, Soc. Netw., 11 (1989), 237-255. |
[76] | I. Matsuda, P. Zhang, L. Swedell, U. Mori, A. Tuuga, A., H. Bernard, et al., Comparisons of intraunit relationships in nonhuman primates living in multilevel social systems, Int. J. Primatol., 33 (2012), 1038-1053. |
[77] | R. Milo, S. Shen-Orr, S. Itzkovitz, N. Kashtan, D. Chklovskii, U. Alon, Network motifs: Simple building blocks of complex networks, Science, 298 (2002), 824-827. |
[78] | N. Snyder-Mackler, J. C. Beehner, T. J. Bergman, Defining higher levels in the multilevel societies of geladas (Theropithecus gelada), Int. J. Primatol., 33 (2012), 1054-1068. |
[79] | C. Sueur, O. Petit, A. de Marco, A. T. Jacobs, K. Watanabe, B. Thierry, A comparative network analysis of social style in macaques, Anim. Behav., 82 (2011), 845-852. |
[80] | B. Voelkl, R. Noë, Simulation of information propagation in real-life primate networks: Longevity, fecundity, fidelity, Behav. Ecol. Sociobiol., 64 (2010), 1449-1459. |
[81] | D. I. Rubenstein, Networks of terrestrial ungulates: linking form and function, in Animal Social Networks (eds. J. Krause, R. James, D. W. Franks, D. P. Croft), Oxford University Press, 2015, 184-196. |
[82] | E. A. Foster, D. W. Franks, L. J. Morrell, K. C. Balcomb, K. M. Parsons, A. van Ginneken, et al., Social network correlates of food availability in an endangered population of killer whales, Orcinus orca. Anim. Behav., 83 (2012), 731-736. |
[83] | R. Albert, A. L. Barabasi, Statistical mechanics of complex networks, Rev. Mod. Phys., 74 (2002), 47-97. |
[84] | M. E. J Newman, The structure and function of complex networks, SIAM Rev., 45 (2003), 167-256. |
[85] | S. Macdonald, B. Voelkl, Primate social networks, in Animal Social Networks (eds. J. Krause, R. James, D. W. Franks, D. P. Croft), Oxford University Press, 2015,123-136. |
[86] | R. R. Kao, L. Danon, D. M. Green, I. Z. Kiss, Demographic structure and pathogen dynamics on the network of livestock movements in Great Britain, Proc. R. Soc. B, 273 (2007), 1999-2007. |
[87] | R. R. Kao, D. M. Green, J. Johnson, I. Z. Kiss, Disease dynamics over very different time-scales: Foot-and-mouth disease and scrapie on the network of livestock movements in the UK, J. R. Soc. Interface, 4 (2007), 907-916. |
[88] | L. Danon, A. P. Ford, T. House, C. P. Jewell, M. J. Keeling, G. O. Roberts, et al., Networks and the epidemiology of infectious disease, Interdiscipl. Persp. Infect. Dis., 2011 (2011), 284909. |
[89] | R. M. Anderson, R. M. May, Population biology of infectious diseases: Part 1, Nature, 280 (1979), 361-367. |
[90] | M. J. E. Newman, The spread of epidemic disease on networks, Phys. Rev. E, 66 (2003), 016128. |
[91] | R. M. May, Network structure and the biology of populations', Trends Ecol. Evol., 21 (2006), 394-399. |
[92] | L. Hufnagel, D. Brockmann, T. Geisel, Forecast and control of epidemics in a globalized world, Proc. Natl. Acad. Sci. USA, 101 (2004), 7794-7799. |
[93] | L. A. Meyers, Contact network epidemiology: Bond percolation applied to infectious disease prediction and control, Bull. Am. Math. Soc., 44 (2007), 63-86. |
[94] | R. M. May, R. M Anderson, Transmission dynamics of HIV infection, Nature, 326 (1987), 137-142. |
[95] | A. S. Klovdahl, J. J. Potterat, D. E. Woodhouse, J. B. Muth, S. Q. Muth, W. W. Darrow, Social networks and infectious disease: The Colorado Springs study, Soc. Sci. Med., 38 (1994), 79-88. |
[96] | F. Liljeros, C. R. Edling, L. A. Nunes Amaral, E. Stanley, Y. Åberg, The web of human sexual contacts, Nature, 411 (2001), 907-908. |
[97] | M. A. Nowak, Evolutionary Dynamics, Harvard University Press, 2006. |
[98] | M. W. Schein, M. H. Fohrman, Social dominance relationships in a herd of dairy cattle, Brit. J. Anim. Behav., 3 (1955), 45-55. |
[99] | M. Bigras-Poulin, R. A. Thompson, M. Chriel, S. Mortensen, M. Greiner, Network analysis of Danish cattle industry trade patterns as an evaluation of risk potential for disease spread, Prevent. Vet. Med., 76 (2006), 11-39. |
[100] | L. Fiebig, T. Smieszek, J. Saurina, J. Hattendorf, J. Zinsstag, Contacts between poultry farms, their spatial dimension and their relevance for avian influenza preparedness, Geospat. Health, 4 (2009), 79-95. |
[101] | B. Martinez-Lopez, A. M. Perez, J. M. Sanchez-Vizcaino, Social network analysis. Review of general concepts and use in preventive veterinary medicine, Transb. Emerg. Dis., 56 (2009), 109-120. |
[102] | V. V. Volkova, R. Howey, N. J. Savill, M. E. J. Woolhouse, Sheep movement networks and the transmission of infectious diseases, PLoS One, 5 (2010), e11185. |
[103] | R. P. Smith, A. J. C. Cook, R. M. Christley, Descriptive and social network analysis of pig transport data recorded by quality assured pig farms in the UK, Prevent. Vet. Med., 108 (2013), 167-177. |
[104] | J. Ribeiro-Lima, E. A. Enns, B. Thompson, M. E. Craft, S. J. Wells, From network analysis to risk analysis-An approach to risk-based surveillance for bovine tuberculosis in Minnesota, US, Prevent. Vet. Med., 118 (2015), 328-340. |
[105] | H. H. Lentz, A. Koher, P. Hövel, J. Gethmann, C. Sauter-Louis, T. Selhorst, et al., Disease spread through animal movements: a static and temporal network analysis of pig trade in Germany, PLoS One, 11 (2016), 0155195. |
[106] | P. Bajardi, A. Barrat, L. Savini, V. Colizza, Optimizing surveillance for livestock disease spreading through animal movements, J. R. Soc. Interface, 9 (2012), 2814-2825. |
[107] | M. M. Mweu, G. Fournié, T. Halasa, N. Toft, S. S. Nielsen, Temporal characterisation of the network of Danish cattle movements and its implication for disease control: 2000-2009, Prevent. Vet. Med., 110 (2013), 379-387. |
[108] | S. Nickbakhsh, L. Matthews, J. E. Dent, G. T. Innocent, M. E. Arnold, S. W. Reid, et al., Implications of within-farm transmission for network dynamics: Consequences for the spread of avian influenza, Epidemics, 5 (2013), 67-76. |
[109] | B. Vidondo, B. Voelkl, Dynamic network measures reveal the impact of cattle markets and alpine summering on the risk of epidemic outbreaks in the Swiss cattle population, BMC Vet. Res. 14 (2018), 88. |
[110] | J. Krause, D. Lusseau, R. James, Animal social networks: An introduction, Behav. Ecol. Sociobiol., 63 (2009), 967-973. |
[111] | M. J. Silk, D. P. Croft, R. J. Delahay, D. J. Hodgson, M. Boots, N. Weber, et al., Using social network measures in wildlife disease ecology, epidemiology, and management, BioScience, 67 (2017), 245-257. |
[112] | M. E. Craft, Infectious disease transmission and contact networks in wildlife and livestock, Phil. Trans. R. Soc. B, 370 (2015), 20140107. |
[113] | R. H. Griffin, C. L. Nunn, Community structure and the spread of infectious disease in primate social networks, Evol. Ecol., 26 (2012), 779-800. |
[114] | C. L. Nunn, F. Jordan, C. M. McCabe, J. L. Verdolin, J. H. Fewell, Infectious disease and group size: More than just a numbers game, Phil. Trans. R. Soc. B, 370 (2015), 20140111. |
[115] | S. S. Godfrey, C. M. Bull, R. James, K. Murray, Network structure and parasite transmission in a group living lizard, the gidgee skink, Egernia stokesii, Behav. Ecol. Sociobiol., 63 (2009), 1045-1056. |
[116] | K. L. VanderWaal, E. R. Atwill, S. Hooper, K. Buckle, B. McCowan, Network structure and prevalence of Cryptosporidium in Belding's ground squirrels, Behav. Ecol. Sociobiol., 67 (2013), 1951-1959. |
[117] | T. Porphyre, M. Stevenson, R. Jackson, J. McKenzie, Original article Influence of contact heterogeneity on TB reproduction ratio R0 in a free-living brushtail possum Trichosurus vulpecula population, Vet. Res., 39 (2008), 31. |
[118] | J. Rushmore, D. Caillaud, R. J. Hall, R. M. Stumpf, L. A. Meyers, S. Altizer, Network-based vaccination improves prospects for disease control in wild chimpanzees, J. R. Soc. Interface, 11 (2014), 20140349. |
[119] | J. A. Drewe, K. T. D. Eames, J. R. Madden, G. P. Pearce, Integrating contact network structure into tuberculosis epidemiology in meerkats in South Africa: Implications for control, Prevent. Vet. Med., 101 (2011), 113-120. |
[120] | M. D. J. Blyton, S. C. Banks, R. Peakall, D. B. Lindenmayer, D. M. Gordon, Not all types of host contacts are equal when it comes to E. coli transmission, Ecol. Lett., 17 (2014), 970-978. |
[121] | C. R. Webb, Farm animal networks: Unraveling the contact structure of the British sheep population, Prevent. Vet. Med., 68 (2005), 3-17. |
[122] | F. Natale, A. Giovannini, L. Savini, D. Palma, L. Possenti, G. Fiore, et al., Network analysis of Italian cattle trade patterns and evaluation of risks for potential disease spread, Prevent. Vet. Med., 92 (2009), 341-350. |
[123] | C. Dubé, C. Ribble, D. Kelton, B. Mcnab, A Review of network analysis terminology and its application to foot-and-mouth disease modelling and policy development, Transbound. Emerg. Dis., 56 (2009), 73-85. |
[124] | H. Chen, G. Smith, S. Zhang, K. Qin, J. Wang, S. Li, et al., H5N1 virus outbreak in migratory waterfowl, Nature, 436 (2005), 191-192. |
[125] | B. J. Hoye, V. J. Munster, H. Nishiura, R. A. M. Fouchier, J. Madsen, M. Klaassen, Reconstructing an annual cycle of interaction: Natural infection and antibody dynamics to avian influenca along a migratory flyway, Oikos, 120 (2011), 748-755. |
[126] | K. R. Finn, M. J. Silk, M. A. Porter, N. Pinter-Wollman, The use of multilayer network analysis in animal behaviour, Anim. Behav., 149 (2019), 7-22. |
[127] | K. Robert, D. Garant, F. Pelletier, Keep in touch: Does spatial overlap correlate with contact rate frequency?, J. Wildl. Manag., 76 (2012), 1670-1675. |
[128] | M. L. Gilbertson, L. A. White, M. E. Craft, Trade‐offs with telemetry‐derived contact networks for infectious disease studies in wildlife, Meth. Ecol. Evol., 2020. |
[129] | S. E. Perkins, F. Cagnacci, A. Stradiotto, D. Arnoldi, P. J. Hudson, Comparison of social networks derived from ecological data: implications for inferring infectious disease dynamics, J. Anim. Ecol., 78 (2009), 1015-1022. |
[130] | J. Krause, A. D. M. Wilson, D. P. Croft, New technology facilitates the study of social networks, Trends Ecol. Evol., 26 (2011), 5-6. |
[131] | C. Rutz, Z. T. Burns, R. James, S. M. H. Ismar, J. Burt, B. Otis, et al., Automated mapping of social networks in wild birds, Curr. Biol., 22 (2012), R669-R671. |
[132] | I. Psorakis, B. Voelkl, C. J. Garroway, R. Radersma, L. M. Aplin, R. A. Crates, et al., Inferring social structure from temporal data, Behav. Ecol. Sociobiol., 69 (2015), 857-866. |
[133] | J. R. Ginsberg, T. P. Young, Measuring associations between individuals or groups in behavioural studies, Anim. Behav., 44 (1992), 377-379. |
[134] | L. Beijder, D. Fletcher, S. Brager, A method for testing association patterns of social animals, Anim. Behav., 56 (1998), 719-725. |
[135] | L. A. White, J. D. Forester, M. E. Craft, Using contact networks to explore mechanisms of parasite transmission in wildlife, Biol. Rev., 92 (2017), 389-409. |
[136] | R. K. Hamede, J. Bashford, H. McCallum, M. Jones, Contact networks in a wild Tasmanian devil (Sarcophilus harrisii) population: Using social network analysis to reveal seasonal variability in social behaviour and its implications for transmission of devil facial tumour disease, Ecol. Lett., 12 (2009), 1147-1157. |
[137] | T. C. Germann, K. Kadau, I. M. Longini, C. A. Macken, Mitigation strategies for pandemic influenza in the United States, Proc. Natl. Acad. Sci. USA, 103 (2006), 5935-5940. |
[138] | S. E. Robinson, M. G. Everett, R. M. Christley, Recent network evolution increases the potential for large epidemics in the British cattle population, J. R. Soc. Interface, 4 (2007), 669-674. |
[139] | J. C. Gibbens, C. E. Sharpe, J. W. Wilesmith, L. M. Mansley, E. Michalopoulou, J. B., et al., Descriptive epidemiology of the 2001 foot-and-mouth disease epidemic in Great Britain: The first five months, Vet. Rec., 149 (2001), 729-743. |
[140] | I. Z. Kiss, D. M. Green, R. R. Kao, The network of sheep movements within Great Britain: Network properties and their implications for infectious disease spread, J. R. Soc. Interface, 3 (2006), 669-677. |
[141] | D. M. Green, I. Z. Kiss, R. R. Kao, Modelling the initial spread of foot-and-mouth disease through animal movements, Proc. R. Soc. B, 273 (2006), 2729-2735. |
[142] | M. C.Vernon, M. J. Keeling, Representing the UK's cattle herd as static and dynamic networks, Proc. R. Soc. B, 276 (2009), 469-476. |
[143] | P. Sah, S. T. Leu, P. C. Cross, P. J. Hudson, S. Bansal, Unravelling the disease consequences and mechanisms of modular structure in animal social networks, Proc. Natl. Acad. Sci. USA, 114 (2017), 4165-4170. |
[144] | R. Pastor-Satorras, A. Vespignani, Epidemic spreading in scale-free networks, Phys. Rev. Lett., 86 (2001), 3200-3203. |
[145] | A. L. Lloyd, R. M. May, How viruses spread among computers and people, Science, 292 (2001), 1316-1317. |
[146] | D. C. Bell, J. S. Atkinson, J. W. Carlson, Centrality measures for disease transmission networks, Soc. Netw., 21 (1999), 1-21. |
[147] | M. J. Keeling, The effects of local spatial structure on epidemiological invasions, Proc. R. Soc. B, 266 (1999), 859-867. |
[148] | K. T. D. Eames, M. J. Keeling, Modeling dynamic and network heterogeneities in the spread of sexually transmitted diseases, Proc. Natl. Acad. Sci. USA, 99 (2002), 13330-13335. |
[149] | C. Buckee, L. Danon, S. Gupta, Host community structure and the maintenance of pathogen diversity, Proc. R. Soc. B, 274 (2007), 1715-1721. |
[150] | M. Salathé, J. H. Jones, Dynamics and control of diseases in networks with community structure, PLoS Comp. Biol., 6 (2010), e1000736. |
[151] | S. M. Firestone, M. P. Ward, R. M. Christley, N. K. Dhand, The importance of location in contact networks: Describing early epidemic spread using spatial social network analysis, Prevent. Vet. Med., 102 (2011), 185-195. |
[152] | J. Frössling, A. Ohlson, C. Björkman, N. Hakansson, M. Nöremark, Application of network analysis parameters in risk-based surveillance-Examples based on cattle trade data and bovine infections in Sweden, Prevent. Vet. Med., 105 (2012), 202-208. |
[153] | L. García Álvarez, C. R. Webb, M. A. Holmes, A novel field-based approach to validate the use of network models for disease spread between dairy herds, Epidemiol. Infect., 139 (2011), 1863-1874. |
[154] | R. Biek, A. G. Rodrigo, D. Holley, A. Drummond, C. R. Anderson, H. A. Ross, et al., Epidemiology, genetic diversity, and evolution of endemic feline immunodeficiency virus in a population of wild cougars, J. Virol., 77 (2003), 9578-9589. |
[155] | B. T. Grenfell, O. G. Pybus, J. R. Gog, J. L. N. Wood, J. M. Daly, J. A. Mumford, et al., Unifying the epidemiological and evolutionary dynamics of pathogens, Science, 303 (2004), 327-333. |
[156] | R. Biek, A. Drummond, M. Poss, A virus reveals population structure and recent demographic history of its carnivore host, Science, 311 (2006), 538-542. |
[157] | E. A. Archie, G. Luikart, V. O. Ezenwa, Infecting epidemiology with genetics: A new frontier in disease ecology, Trends Ecol. Evol., 24 (2008), 21-30. |
[158] | C. M. Bull, S. S. Godfrey, D. M. Gordon, Social networks and the spread of Salmonella in a sleepy lizard population, Mol. Ecol., 21 (2012), 4386-4392. |
[159] | K. L. VanderWaal, E. R. Atwill, L. A. Isbell, B. McCowan, Linking social and pathogen transmission networks using microbial genetics in giraffe (Giraffa camelopardalis), J. Anim. Ecol., 83 (2014), 406-414. |
[160] | K. L. VanderWaal, E. R. Atwill, L. A. Isbell, B. McCowan, B. Quantifying microbe transmission networks for wild and domestic ungulates in Kenya, Biol. Conserv., 169 (2014), 136-146. |
[161] |
J. S. Lee, E. W. Ruell, E. E. Boydston, L. M. Lyren, R. S. Alonso, J. L. Troyer, et al., Gene flow and pathogen transmission among bobcats (Lynx rufus) in a fragmented urban landscape, Mol. Ecol., 21 (2012), 1617-1631. doi: 10.1111/j.1365-294X.2012.05493.x
![]() |
[162] | B. Y. Reis, I. S. Kohane, K. D. Mandl, An epidemiological network model for disease outbreak detection, PLoS Med, 4 (2007), e210. |
[163] | F. Schirdewahn, V. Colizza, H. H. Lentz, A. Koher, V. Belik, P. Hövel, Surveillance for outbreak detection in livestock-trade networks, in Temporal Network Epidemiology (eds. M. Naoki, P. Holme), Springer, 2017, 215-240. |
[164] | P. Skums, A. Kirpich, P. I. Baykal, A. Zelikovsky, G. Chowell, Global transmission network of SARS-CoV-2: From outbreak to pandemic, medRxiv, 2020. |
[165] | D. Lusseau, H. Whitehead, S. Gero, Incorporating uncertainty into the study of animal social networks, Anim. Behav., 75 (2008), 1809-1815. |
[166] | R. James, D. P. Croft, J. Krause, Potential banana skins in animal social network analysis, Behav. Ecol. Sociobiol., 63 (2009), 989-997. |
[167] | B. Voelkl, C. Kasper, C. Schwab, Network measures for dyadic interactions: Stability and reliability, Am. J. Primatol., 73 (2011), 731-740. |
[168] | J. Krause, S. Krause, R. Arlinghaus, I. Psorakis, S. Roberts, C. Rutz, Reality mining of animal social systems, Trends Ecol. Evol., 28 (2013), 541-551. |
[169] | M. Berdoy, J. P. Webster, D. W. Macdonald, Fatal attraction in rats infected with Toxoplasma gondii, Proc. R. Soc. B, 267 (2000), 1591-1594. |
[170] | A. Vyas, S. Kim, N. Giacomini, J. C. Boothroyd, R. M. Sapolsky, Behavioral changes induced by Toxoplasma infection of rodents are highly specific to aversion of cat odors, Proc. Natl. Acad. Sci. USA, 104 (2007), 6442-6447. |
[171] | D. P. Croft, M. Edenbrow, S. K. Darden, I. W. Ramnarine, C. van Oosterhout, J. Cable, Effect of gyrodactylid ectoparasites on host behaviour and social network structure in guppies Poecilia reticulata, Behav. Ecol. Sociobiol., 65 (2011), 2219-2227. |
[172] | F. J. Theis, L. V. Ugelvig, C. Marr, S. Cremer, Opposing effects of allogrooming on disease transmission in ant societies, Phil Trans. R. Soc. B, 370 (2015), 20140108. |
[173] | V. O. Ezenwa, E. A. Archie, M. E. Craft, D. M. Hawley, L. B. Martin, J. Moore, et al., Host behaviour-parasite feedback: an essential link between animal behaviour and disease ecology, Proc. R. Soc. B, 283 (2016), 20153078. |
[174] | L. A. White, J. D. Forester, M. E. Craft, Dynamic, spatial models of parasite transmission in wildlife: Their structure, applications and remaining challenges, J. Anim. Ecol., 87 (2018), 559-580. |
[175] | K. Büttner, J. Salau, J., Krieter, Quality assessment of static aggregation compared to the temporal approach based on a pig trade network in Northern Germany, Prevent. Vet. Med., 129 (2016), 1-8. |
[176] | M. J. Silk, D. J., Hodgson, C. Rozins, D. P. Croft, R. J. Delahay, M. Boots, et al., Integrating social behaviour, demography and disease dynamics in network models: applications to disease management in declining wildlife populations, Phil. Trans. R. Soc. B, 374 (2019), 20180211. |
[177] | M. E. Craft, E. Volz, C. Packer, L. A. Meyers, Disease transmission in territorial populations: The small-world network of Serengeti lions, J. R. Soc. Interface, 8 (2011), 776-786. |
[178] | N. Weber, S. P. Carter, S. R. X. Dall, R. J. L. Delahay, J. L. McDonald, S. Bearhop, et al., Badger social networks correlate with tuberculosis infection, Curr. Biol., 23 (2013), R915-R916. |
[179] | K. P. Huyvaert, R. E. Russell, K. A. Patyk, M. E. Craft, P. C. Cross, M. G. Garner, et al., Challenges and opportunities developing mathematical models of shared pathogens of domestic and wild animals, Vet. Sci., 5 (2018), 92. |
[180] | S. Kraberger, N. M. Fountain-Jones, R. B. Gagne, J. Malmberg, N.G. Dannemiller, K. Logan, et al., Frequent cross-species transmissions of foamy virus between domestic and wild felids, Virus Evol., 6 (2020), vez058. |
[181] | R. K. Plowright, C. R. Parrish, H. McCallum, P. J. Hudson, A. I. Ko, A. L. Graham, et al., Pathways to zoonotic spillover, Nat. Rev. Microbiol., 15 (2017), 502. |
[182] | B. J. Coburn, B. G. Wagner, S. Blower, Modeling influenza epidemics and pandemics: insights into the future of swine flu (H1N1). BMC Med., 7 (2009), 30. |
[183] | C. M. Scoglio, C. Bosca, M. H. Riad, F. D. Sahneh, S. C. Britch, L. W. Cohnstaedt, et al., Biologically informed individual-based network model for Rift Valley fever in the US and evaluation of mitigation strategies, PloS One, 11 (2016), e0162759. |
[184] | S. K. Lau, P. C. Woo, K. S. Li, Y. Huang, H. W. Tsoi, B. H. Wong, et al., Severe acute respiratory syndrome coronavirus-like virus in Chinese horseshoe bats, Proc. Natl. Acad. Sci. USA, 102 (2005), 14040-14045. |
[185] | C. M. Luo, N. Wang, X. L. Yang, H. Z. Liu, W. Zhang, B. Li, et al., Discovery of novel bat coronaviruses in south China that use the same receptor as Middle East respiratory syndrome coronavirus, J. Virol., 92 (2018), e00116-18. |
[186] | N. Wang, S. Y. Li, X. L. Yang, H. M. Huang, Y. J. Zhang, H. Guo, et al., Serological evidence of bat SARS-related coronavirus infection in humans, China, Virol. Sin., 33 (2018),104-107. |
[187] | L. E. Escobar, R. Moen, M. E. Craft, K. L. VanderWaal, Mapping parasite transmission risk from white-tailed deer to a declining moose population, Eur. J. Wildl. Res., 65 (2019), 60. |
[188] | P. Sah, J. Mann, S. Bansal, Disease implications of animal social network structure: A synthesis across social systems, J. Anim. Ecol., 87 (2018), 546-558. |
Parameter | Description | Value | Unit | Source |
N | Population of Saudi Arabia | 34218169 | Individual | [44] |
η | Birth rate | 1250 | Individual×Day−1 | [37] |
μ | Natural death rate | 3.6529×10−5 | Day−1 | [37] |
β1 | Transmission rate by Is | 0.0554×10−7 | (Individual×Day)−1 | Estimated |
β2 | Transmission rate by Ia | 1.0596×10−7 | (Individual×Day)−1 | Estimated |
γ | Incubation rate | 1/6 | Day−1 | [9] |
θ | Probability of becoming Ia | 0.54 | − | Estimated |
ε | Effectiveness of random diagnostic testing for Ia | 0 | − | Estimated |
δ | Recovery rate | 3.2772×10−1 | Day−1 | [37] |
d | Death rate due to COVID-19 | 2.3724×10−1 | Day−1 | [37] |
Phase | Time | ρ | SD | Rc |
Phase 1 | t1=[12,22] | 0.85 | 0.15 | 4.4267 |
Phase 2 | t2=[22,25] | 0.75 | 0.30 | 3.2166 |
t3=[25,28] | 0.65 | 0.45 | 2.1904 | |
t4=[28,36] | 0.60 | 0.55 | 1.6543 | |
Phase 3 | t5=[36,56] | 0.55 | 0.55 | 1.5164 |
Phase 4 | t6=[56,67] | 0.55 | 0.60 | 1.3479 |
t7=[67,83] | 0.55 | 0.69 | 1.0446 | |
Phase 5 | t8=[83,88] | 0.40 | 0.80 | 0.4902 |
Phase 6 | t9=[88,92] | 0.65 | 0.70 | 1.1947 |
t10=[92,112] | 0.75 | 0.70 | 1.3785 | |
Phase 7 | t11=[112,120] | 0.80 | 0.76 | 1.1764 |
t12=[120,130] | 0.80 | 0.80 | 0.9803 | |
t13=[130,144] | 0.80 | 0.82 | 0.8823 | |
t14=[144,169] | 0.85 | 0.83 | 0.8853 | |
t15=[169,179] | 0.85 | 0.82 | 0.9374 | |
t16=[179,193] | 0.90 | 0.82 | 0.9926 | |
t17=[193,204] | 0.95 | 0.85 | 0.8731 | |
t18=[204,207] | 0.95 | 0.88 | 0.6985 |
Parameter P | Value | Sensitivity index ΓPRc |
η | 1250 | 1 |
β1 | 0.0554×10−7 | 0.0413 |
β2 | 1.0596×10−7 | 0.9587 |
γ | 0.167 | 0.0002 |
θ | 0.54 | 0.9103 |
δ | 3.2772×10−1 | −0.9826 |
d | 2.3724×10−1 | −0.0173 |
μ | 3.653×10−5 | −1.0003 |
ρ | 0.80 | 1 |
ε | 0.40 | −0.6391 |
SD | 0.10 | −0.1111 |
0.30 | −0.4286 | |
0.50 | −1 | |
0.70 | −2.3333 | |
0.90 | −9 | |
0.99 | −99 |
Scenario | ε | ρ | SD |
1st | Different values | Fitting values | Fitting values |
2nd | Different values | No lockdown (ρ=1) | No social distancing (SD=0) |
3rd | Different values | Different values | Fitting values |
4th | Different values | Fitting values | Different values |
5th | Different values | 0.85 | Different values |
6th | Different values | 0.75 | Different values |
7th | Different values | Fitting values | Fitting values |
for three time periods |
ρ | SD | ε | Peak (day) | Cases | Percentage Change |
Fitting values | Fitting values | 0.01 | 122 | 52,010 | −13.07% |
0.02 | 122 | 45,140 | −24.55% | ||
0.03 | 122 | 39,120 | −34.61% | ||
0.04 | 122 | 33,830 | −43.45% | ||
0.10 | 121 | 13,770 | −76.98% | ||
0.20 | 68 | 4,159 | −93.04% | ||
0.30 | 58 | 1,556 | −97.39% | ||
0.40 | 37 | 705 | −98.82% |
ρ | SD | ε | Peak (day) | Cases | Percentage Change |
1 | 0 | 0.10 | 53 | 4,728,000 | +7802.39% |
0.20 | 57 | 4,420,000 | +7287.60% | ||
0.30 | 62 | 4,057,000 | +6680.88% | ||
0.40 | 69 | 3,607,000 | +5928.75% | ||
0.50 | 79 | 3,042,000 | +4984.41% | ||
0.60 | 97 | 2,332,000 | +3797.71% | ||
0.70 | 133 | 1,431,000 | +2291.78% | ||
0.80 | 266 | 391,700 | +554.68% |
ρ | SD | ε | Peak (day) | Cases | Percentage Change |
1 | Fitting values | 0.10 | 84 | 1,163,000 | +1843.84% |
0.20 | 112 | 630,200 | +953.31% | ||
0.30 | 115 | 195,700 | +227.09% | ||
0.40 | 113 | 27,360 | −54.27% | ||
0.85 | Fitting values | 0.10 | 113 | 454,000 | +658.81% |
0.20 | 115 | 127,900 | +113.77% | ||
0.30 | 113 | 22,410 | −62.54% | ||
0.40 | 77-83 | 4,208 | −92.96% | ||
0.75 | Fitting values | 0.10 | 115 | 110,600 | +84.85% |
0.20 | 113 | 23,550 | −60.63% | ||
0.30 | 83 | 5,273 | −91.18% | ||
0.40 | 68 | 1,514 | −97.46% | ||
0.55 | Fitting values | 0.10 | 70 | 2,882 | −95.18% |
0.20 | 68 | 1,175 | −98.03% | ||
0.30 | 58 | 474 | −99.20% | ||
0.40 | 30 | 244 | −99.59% |
ρ | SD | ε | Peak (day) | Cases | Percentage Change |
Fitting values | 0.40 | 0.10 | 124 | 2,314,000 | +3767.62% |
0.20 | 138,139 | 2,035,000 | +3301.30% | ||
0.30 | 160 | 1,764,000 | +2848.35% | ||
0.40 | 194 | 1,441,000 | +2308.49% | ||
Fitting values | 0.50 | 0.10 | 150 | 1,874,000 | +3032.21% |
0.20 | 171 | 1,629,000 | +2622.71% | ||
0.30 | 203 | 1,434,000 | +2296.79% | ||
0.40 | 247 | 1,121,000 | +1773.64% | ||
Fitting values | 0.60 | 0.10 | 198 | 1,439,000 | +2305.15% |
0.20 | 229 | 1,271,000 | +2024.35% | ||
0.30 | 279 | 902,300 | +1408.11% | ||
0.40 | 377 | 506,400 | +746.39% | ||
Fitting values | 0.70 | 0.10 | 302 | 776,200 | +1197.34% |
0.20 | 387 | 480,900 | +703.77% | ||
0.30 | 572 | 213,800 | +257.34% | ||
0.40 | 1402, 1408 | 28,830 | −51.81% |
ρ | SD | ε | Peak (day) | Cases | Percentage Change |
0.85 | 0.40 | 0.10 | 88 | 2,674,000 | +4369.33% |
0.20 | 98 | 2,284,000 | +3717.48% | ||
0.30 | 114 | 1,847,000 | +2987.08% | ||
0.40 | 138 | 1,357,000 | +2168.09% | ||
0.85 | 0.50 | 0.10 | 106 | 2,052,000 | +3329.72% |
0.20 | 121,122 | 1,666,000 | +2684.56% | ||
0.30 | 145 | 1,247,000 | +1984.24% | ||
0.40 | 185,186 | 801,700 | +1239.96% | ||
0.85 | 0.60 | 0.10 | 141 | 1,310,000 | +2089.54% |
0.20 | 168,169 | 957,200 | +1499.87% | ||
0.30 | 216 | 599,300 | +901.67% | ||
0.40 | 320 | 265,700 | +344.09% | ||
0.85 | 0.70 | 0.10 | 240 | 488,600 | +716.64% |
0.20 | 331,332 | 246,000 | +311.16% | ||
0.30 | 600 | 60,790 | +1.60% | ||
0.40 | 12 | 139 | −99.76% |
ρ | SD | ε | Peak (day) | Cases | Percentage Change |
0.75 | 0.40 | 0.10 | 100 | 2,246,000 | +3653.97% |
0.20 | 113 | 1,859,000 | +3007.14% | ||
0.30 | 133,134 | 1,430,000 | +2290.11% | ||
0.40 | 167 | 967,500 | +1517.08% | ||
0.75 | 0.50 | 0.10 | 123 | 1,630,000 | +2624.39% |
0.20 | 144 | 1,259,000 | +2004.30% | ||
0.30 | 178 | 867,000 | +1349.11% | ||
0.40 | 242 | 475,400 | +694.58% | ||
0.75 | 0.60 | 0.10 | 172 | 924,900 | +1445.88% |
0.20 | 214,215 | 608,800 | +917.55% | ||
0.30 | 297 | 310,600 | +419.13% | ||
0.40 | 550 | 75,830 | +26.74% | ||
0.75 | 0.70 | 0.10 | 344 | 226,300 | +278.23% |
0.20 | 585-588 | 64,690 | +8.12% | ||
0.30 | 12 | 139 | −99.76% | ||
0.40 | 12 | 139 | −99.76% |
Event | ε1 | ε2 | ε3 | Peak (day) | Cases | Percentage Change |
Ⅰ | 0.10 | 0 | 0 | 123 | 38,480 | −35.68% |
Ⅱ | 0.10 | 0.10 | 0 | 124 | 20,890 | −65.08% |
Ⅲ | 0 | 0.10 | 0.10 | 121 | 21,920 | −63.36% |
Ⅳ | 0.20 | 0 | 0 | 124 | 23,650 | −60.47% |
Ⅴ | 0.20 | 0.20 | 0 | 125 | 6,539 | −89.07% |
Ⅵ | 0 | 0.20 | 0.20 | 68 | 11,470 | −80.82% |
Ⅶ | 0.10 | 0.20 | 0 | 125 | 10,870 | −81.83% |
Ⅷ | 0.10 | 0.20 | 0.10 | 121 | 7,132 | −88.07% |
Ⅸ | 0.20 | 0.10 | 0 | 124,125 | 12,680 | −78.80% |
Ⅹ | 0.20 | 0.10 | 0.10 | 121 | 8,326 | −86.08% |
Ⅺ | 0 | 0.20 | 0.10 | 121 | 11,480 | −80.81% |
Parameter | Description | Value | Unit | Source |
N | Population of Saudi Arabia | 34218169 | Individual | [44] |
η | Birth rate | 1250 | Individual×Day−1 | [37] |
μ | Natural death rate | 3.6529×10−5 | Day−1 | [37] |
β1 | Transmission rate by Is | 0.0554×10−7 | (Individual×Day)−1 | Estimated |
β2 | Transmission rate by Ia | 1.0596×10−7 | (Individual×Day)−1 | Estimated |
γ | Incubation rate | 1/6 | Day−1 | [9] |
θ | Probability of becoming Ia | 0.54 | − | Estimated |
ε | Effectiveness of random diagnostic testing for Ia | 0 | − | Estimated |
δ | Recovery rate | 3.2772×10−1 | Day−1 | [37] |
d | Death rate due to COVID-19 | 2.3724×10−1 | Day−1 | [37] |
Phase | Time | ρ | SD | Rc |
Phase 1 | t1=[12,22] | 0.85 | 0.15 | 4.4267 |
Phase 2 | t2=[22,25] | 0.75 | 0.30 | 3.2166 |
t3=[25,28] | 0.65 | 0.45 | 2.1904 | |
t4=[28,36] | 0.60 | 0.55 | 1.6543 | |
Phase 3 | t5=[36,56] | 0.55 | 0.55 | 1.5164 |
Phase 4 | t6=[56,67] | 0.55 | 0.60 | 1.3479 |
t7=[67,83] | 0.55 | 0.69 | 1.0446 | |
Phase 5 | t8=[83,88] | 0.40 | 0.80 | 0.4902 |
Phase 6 | t9=[88,92] | 0.65 | 0.70 | 1.1947 |
t10=[92,112] | 0.75 | 0.70 | 1.3785 | |
Phase 7 | t11=[112,120] | 0.80 | 0.76 | 1.1764 |
t12=[120,130] | 0.80 | 0.80 | 0.9803 | |
t13=[130,144] | 0.80 | 0.82 | 0.8823 | |
t14=[144,169] | 0.85 | 0.83 | 0.8853 | |
t15=[169,179] | 0.85 | 0.82 | 0.9374 | |
t16=[179,193] | 0.90 | 0.82 | 0.9926 | |
t17=[193,204] | 0.95 | 0.85 | 0.8731 | |
t18=[204,207] | 0.95 | 0.88 | 0.6985 |
Parameter P | Value | Sensitivity index ΓPRc |
η | 1250 | 1 |
β1 | 0.0554×10−7 | 0.0413 |
β2 | 1.0596×10−7 | 0.9587 |
γ | 0.167 | 0.0002 |
θ | 0.54 | 0.9103 |
δ | 3.2772×10−1 | −0.9826 |
d | 2.3724×10−1 | −0.0173 |
μ | 3.653×10−5 | −1.0003 |
ρ | 0.80 | 1 |
ε | 0.40 | −0.6391 |
SD | 0.10 | −0.1111 |
0.30 | −0.4286 | |
0.50 | −1 | |
0.70 | −2.3333 | |
0.90 | −9 | |
0.99 | −99 |
Scenario | ε | ρ | SD |
1st | Different values | Fitting values | Fitting values |
2nd | Different values | No lockdown (ρ=1) | No social distancing (SD=0) |
3rd | Different values | Different values | Fitting values |
4th | Different values | Fitting values | Different values |
5th | Different values | 0.85 | Different values |
6th | Different values | 0.75 | Different values |
7th | Different values | Fitting values | Fitting values |
for three time periods |
ρ | SD | ε | Peak (day) | Cases | Percentage Change |
Fitting values | Fitting values | 0.01 | 122 | 52,010 | −13.07% |
0.02 | 122 | 45,140 | −24.55% | ||
0.03 | 122 | 39,120 | −34.61% | ||
0.04 | 122 | 33,830 | −43.45% | ||
0.10 | 121 | 13,770 | −76.98% | ||
0.20 | 68 | 4,159 | −93.04% | ||
0.30 | 58 | 1,556 | −97.39% | ||
0.40 | 37 | 705 | −98.82% |
ρ | SD | ε | Peak (day) | Cases | Percentage Change |
1 | 0 | 0.10 | 53 | 4,728,000 | +7802.39% |
0.20 | 57 | 4,420,000 | +7287.60% | ||
0.30 | 62 | 4,057,000 | +6680.88% | ||
0.40 | 69 | 3,607,000 | +5928.75% | ||
0.50 | 79 | 3,042,000 | +4984.41% | ||
0.60 | 97 | 2,332,000 | +3797.71% | ||
0.70 | 133 | 1,431,000 | +2291.78% | ||
0.80 | 266 | 391,700 | +554.68% |
ρ | SD | ε | Peak (day) | Cases | Percentage Change |
1 | Fitting values | 0.10 | 84 | 1,163,000 | +1843.84% |
0.20 | 112 | 630,200 | +953.31% | ||
0.30 | 115 | 195,700 | +227.09% | ||
0.40 | 113 | 27,360 | −54.27% | ||
0.85 | Fitting values | 0.10 | 113 | 454,000 | +658.81% |
0.20 | 115 | 127,900 | +113.77% | ||
0.30 | 113 | 22,410 | −62.54% | ||
0.40 | 77-83 | 4,208 | −92.96% | ||
0.75 | Fitting values | 0.10 | 115 | 110,600 | +84.85% |
0.20 | 113 | 23,550 | −60.63% | ||
0.30 | 83 | 5,273 | −91.18% | ||
0.40 | 68 | 1,514 | −97.46% | ||
0.55 | Fitting values | 0.10 | 70 | 2,882 | −95.18% |
0.20 | 68 | 1,175 | −98.03% | ||
0.30 | 58 | 474 | −99.20% | ||
0.40 | 30 | 244 | −99.59% |
ρ | SD | ε | Peak (day) | Cases | Percentage Change |
Fitting values | 0.40 | 0.10 | 124 | 2,314,000 | +3767.62% |
0.20 | 138,139 | 2,035,000 | +3301.30% | ||
0.30 | 160 | 1,764,000 | +2848.35% | ||
0.40 | 194 | 1,441,000 | +2308.49% | ||
Fitting values | 0.50 | 0.10 | 150 | 1,874,000 | +3032.21% |
0.20 | 171 | 1,629,000 | +2622.71% | ||
0.30 | 203 | 1,434,000 | +2296.79% | ||
0.40 | 247 | 1,121,000 | +1773.64% | ||
Fitting values | 0.60 | 0.10 | 198 | 1,439,000 | +2305.15% |
0.20 | 229 | 1,271,000 | +2024.35% | ||
0.30 | 279 | 902,300 | +1408.11% | ||
0.40 | 377 | 506,400 | +746.39% | ||
Fitting values | 0.70 | 0.10 | 302 | 776,200 | +1197.34% |
0.20 | 387 | 480,900 | +703.77% | ||
0.30 | 572 | 213,800 | +257.34% | ||
0.40 | 1402, 1408 | 28,830 | −51.81% |
ρ | SD | ε | Peak (day) | Cases | Percentage Change |
0.85 | 0.40 | 0.10 | 88 | 2,674,000 | +4369.33% |
0.20 | 98 | 2,284,000 | +3717.48% | ||
0.30 | 114 | 1,847,000 | +2987.08% | ||
0.40 | 138 | 1,357,000 | +2168.09% | ||
0.85 | 0.50 | 0.10 | 106 | 2,052,000 | +3329.72% |
0.20 | 121,122 | 1,666,000 | +2684.56% | ||
0.30 | 145 | 1,247,000 | +1984.24% | ||
0.40 | 185,186 | 801,700 | +1239.96% | ||
0.85 | 0.60 | 0.10 | 141 | 1,310,000 | +2089.54% |
0.20 | 168,169 | 957,200 | +1499.87% | ||
0.30 | 216 | 599,300 | +901.67% | ||
0.40 | 320 | 265,700 | +344.09% | ||
0.85 | 0.70 | 0.10 | 240 | 488,600 | +716.64% |
0.20 | 331,332 | 246,000 | +311.16% | ||
0.30 | 600 | 60,790 | +1.60% | ||
0.40 | 12 | 139 | −99.76% |
ρ | SD | ε | Peak (day) | Cases | Percentage Change |
0.75 | 0.40 | 0.10 | 100 | 2,246,000 | +3653.97% |
0.20 | 113 | 1,859,000 | +3007.14% | ||
0.30 | 133,134 | 1,430,000 | +2290.11% | ||
0.40 | 167 | 967,500 | +1517.08% | ||
0.75 | 0.50 | 0.10 | 123 | 1,630,000 | +2624.39% |
0.20 | 144 | 1,259,000 | +2004.30% | ||
0.30 | 178 | 867,000 | +1349.11% | ||
0.40 | 242 | 475,400 | +694.58% | ||
0.75 | 0.60 | 0.10 | 172 | 924,900 | +1445.88% |
0.20 | 214,215 | 608,800 | +917.55% | ||
0.30 | 297 | 310,600 | +419.13% | ||
0.40 | 550 | 75,830 | +26.74% | ||
0.75 | 0.70 | 0.10 | 344 | 226,300 | +278.23% |
0.20 | 585-588 | 64,690 | +8.12% | ||
0.30 | 12 | 139 | −99.76% | ||
0.40 | 12 | 139 | −99.76% |
Event | ε1 | ε2 | ε3 | Peak (day) | Cases | Percentage Change |
Ⅰ | 0.10 | 0 | 0 | 123 | 38,480 | −35.68% |
Ⅱ | 0.10 | 0.10 | 0 | 124 | 20,890 | −65.08% |
Ⅲ | 0 | 0.10 | 0.10 | 121 | 21,920 | −63.36% |
Ⅳ | 0.20 | 0 | 0 | 124 | 23,650 | −60.47% |
Ⅴ | 0.20 | 0.20 | 0 | 125 | 6,539 | −89.07% |
Ⅵ | 0 | 0.20 | 0.20 | 68 | 11,470 | −80.82% |
Ⅶ | 0.10 | 0.20 | 0 | 125 | 10,870 | −81.83% |
Ⅷ | 0.10 | 0.20 | 0.10 | 121 | 7,132 | −88.07% |
Ⅸ | 0.20 | 0.10 | 0 | 124,125 | 12,680 | −78.80% |
Ⅹ | 0.20 | 0.10 | 0.10 | 121 | 8,326 | −86.08% |
Ⅺ | 0 | 0.20 | 0.10 | 121 | 11,480 | −80.81% |