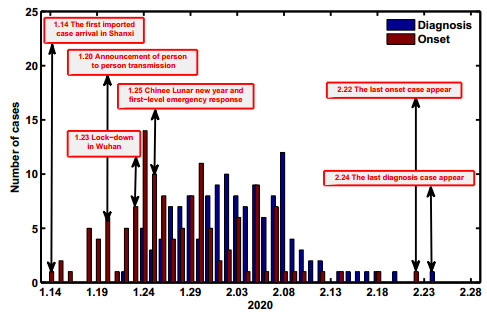
Since December 2019, an outbreak of a novel coronavirus pneumonia (WHO named COVID-19) swept across China. In Shanxi Province, the cumulative confirmed cases finally reached 133 since the first confirmed case appeared on January 22 2020, and most of which were imported cases from Hubei Province. Reasons for this ongoing surge in Shanxi province, both imported and autochthonous infected cases, are currently unclear and demand urgent investigation. In this paper, we developed a SEIQR difference-equation model of COVID-19 that took into account the transmission with discrete time imported cases, to perform assessment and risk analysis. Our findings suggest that if the lock-down date in Wuhan is earlier, the infectious cases are fewer. Moreover, we reveal the effects of city lock-down date on the final scale of cases: if the date is advanced two days, the cases may decrease one half (67, 95% CI: 66–68); if the date is delayed for two days, the cases may reach about 196 (95% CI: 193–199). Our investigation model could be potentially helpful to study the transmission of COVID-19, in other provinces of China except Hubei. Especially, the method may also be used in countries with the first confirmed case is imported.
Citation: Ming-Tao Li, Gui-Quan Sun, Juan Zhang, Yu Zhao, Xin Pei, Li Li, Yong Wang, Wen-Yi Zhang, Zi-Ke Zhang, Zhen Jin. Analysis of COVID-19 transmission in Shanxi Province with discrete time imported cases[J]. Mathematical Biosciences and Engineering, 2020, 17(4): 3710-3720. doi: 10.3934/mbe.2020208
[1] | Avinash Shankaranarayanan, Hsiu-Chuan Wei . Mathematical modeling of SARS-nCoV-2 virus in Tamil Nadu, South India. Mathematical Biosciences and Engineering, 2022, 19(11): 11324-11344. doi: 10.3934/mbe.2022527 |
[2] | Xianghong Zhang, Yunna Song, Sanyi Tang, Haifeng Xue, Wanchun Chen, Lingling Qin, Shoushi Jia, Ying Shen, Shusen Zhao, Huaiping Zhu . Models to assess imported cases on the rebound of COVID-19 and design a long-term border control strategy in Heilongjiang Province, China. Mathematical Biosciences and Engineering, 2022, 19(1): 1-33. doi: 10.3934/mbe.2022001 |
[3] | Jin Guo, Aili Wang, Weike Zhou, Yinjiao Gong, Stacey R. Smith? . Discrete epidemic modelling of COVID-19 transmission in Shaanxi Province with media reporting and imported cases. Mathematical Biosciences and Engineering, 2022, 19(2): 1388-1410. doi: 10.3934/mbe.2022064 |
[4] | Chenxi Dai, Jing Yang, Kaifa Wang . Evaluation of prevention and control interventions and its impact on the epidemic of coronavirus disease 2019 in Chongqing and Guizhou Provinces. Mathematical Biosciences and Engineering, 2020, 17(4): 2781-2791. doi: 10.3934/mbe.2020152 |
[5] | Haonan Zhong, Wendi Wang . Mathematical analysis for COVID-19 resurgence in the contaminated environment. Mathematical Biosciences and Engineering, 2020, 17(6): 6909-6927. doi: 10.3934/mbe.2020357 |
[6] | Haiyan Wang, Nao Yamamoto . Using a partial differential equation with Google Mobility data to predict COVID-19 in Arizona. Mathematical Biosciences and Engineering, 2020, 17(5): 4891-4904. doi: 10.3934/mbe.2020266 |
[7] | Xinmiao Rong, Liu Yang, Huidi Chu, Meng Fan . Effect of delay in diagnosis on transmission of COVID-19. Mathematical Biosciences and Engineering, 2020, 17(3): 2725-2740. doi: 10.3934/mbe.2020149 |
[8] | Juan Li, Wendi Bao, Xianghong Zhang, Yongzhong Song, Zhigui Lin, Huaiping Zhu . Modelling the transmission and control of COVID-19 in Yangzhou city with the implementation of Zero-COVID policy. Mathematical Biosciences and Engineering, 2023, 20(9): 15781-15808. doi: 10.3934/mbe.2023703 |
[9] | Sarita Bugalia, Vijay Pal Bajiya, Jai Prakash Tripathi, Ming-Tao Li, Gui-Quan Sun . Mathematical modeling of COVID-19 transmission: the roles of intervention strategies and lockdown. Mathematical Biosciences and Engineering, 2020, 17(5): 5961-5986. doi: 10.3934/mbe.2020318 |
[10] | Hyeonjeong Ahn, Hyojung Lee . Predicting the transmission trends of COVID-19: an interpretable machine learning approach based on daily, death, and imported cases. Mathematical Biosciences and Engineering, 2024, 21(5): 6150-6166. doi: 10.3934/mbe.2024270 |
Since December 2019, an outbreak of a novel coronavirus pneumonia (WHO named COVID-19) swept across China. In Shanxi Province, the cumulative confirmed cases finally reached 133 since the first confirmed case appeared on January 22 2020, and most of which were imported cases from Hubei Province. Reasons for this ongoing surge in Shanxi province, both imported and autochthonous infected cases, are currently unclear and demand urgent investigation. In this paper, we developed a SEIQR difference-equation model of COVID-19 that took into account the transmission with discrete time imported cases, to perform assessment and risk analysis. Our findings suggest that if the lock-down date in Wuhan is earlier, the infectious cases are fewer. Moreover, we reveal the effects of city lock-down date on the final scale of cases: if the date is advanced two days, the cases may decrease one half (67, 95% CI: 66–68); if the date is delayed for two days, the cases may reach about 196 (95% CI: 193–199). Our investigation model could be potentially helpful to study the transmission of COVID-19, in other provinces of China except Hubei. Especially, the method may also be used in countries with the first confirmed case is imported.
Since December 2019, an outbreak of a novel coronavirus pneumonia (WHO named COVID-19) swept across China [1]. Subsequently, on January 20, 2020, the Chinese government has revised the law provisions concerning infectious diseases to add the COVID-19 as a notifiable infectious disease (Category B infectious diseases). Considering the seriousness of the disease, public health officials have announced COVID-19 are managed according to Category A infectious diseases, the same as SARS in 2003. COVID-19 is mainly transmitted through respiratory droplets and can also be transmitted through intimate contact, etc. The average incubation period is 5.2 days (95% CI, 4.1 to 7.0) before clinical symptoms [1]. In addition, related researches found that there exists the infectivity in the incubation period, and most infection are mild or asymptomatic, only partly infection clinical manifestations are pneumonia. The primary symptoms are fever, cough, and weakness, and pulmonary imaging CT showed significantly changes in the lung [2].
With the arrival of Spring Festival of 2020, passengers travelled from Hubei Province go to all the rest parts of mainland China, which may increase the risk of imported case in other provinces. On January 20, the National Health Commission of China announced that there existed person-to-person transmission, which caused the panicked people to hasten away from Wuhan. From January 13, confirmed cases started to appear in other provinces of mainland China. In order to control the transmission of COVID-19, the Chinese authorities implemented the lock-down strategy in Wuhan to restrict the movement on January 23. As of January 25, a total of 30 provincial governments (except Tibet) initiated a first-level emergency response. Meanwhile, some non-pharmaceutical interventions include strict travel restriction [3], extensive monitoring of suspected cases, mandatory registration of all people arriving from Hubei Province, and the registered individuals with home quarantine for at least 14 days [4]. The surveillance for new cases, and the tracing and management of contacts have been strictly performed. People are suggested to stay at home as much as possible. All of these measures are designed to early detection, early reporting and early quarantine for infectious cases.
Using information published by the Health Commission of Shanxi Province [5], we constructed a dataset of COVID-19 patients that are laboratory-confirmed in Shanxi Province as the end of February 28, 2020. The detailed information includes the cumulative and daily laboratory-confirmed cases, and life track of these laboratory-confirmed cases. By means of statistical analysis the life track of all laboratory-confirmed cases, we obtain the data of symptom onset (the self-reported date of symptoms such as fever, cough, or other respiratory symptoms) for all laboratory-confirmed cases, and arrival date of the confirmed COVID-19 cases in Shanxi Province. The first confirmed case was reported on January 22 (notice that the arrival date of the first confirmed imported case and the first symptom onset case in Shanxi province was January 14), then confirmed cases continued to emerge and the cumulative confirmed cases reached 133 at the end of February, most of which were imported case from Hubei Province and their local family members in Shanxi province (89 cases). The time series of differences between disease symptom onset and confirmed diagnosis COVID-19 cases in Shanxi Province is shown in Figure 1. Cumulative infected COVID-19 cases and the actual time of arrival in Shanxi province with confirmed COVID-19 cases are shown in Figure 2a, b, respectively. To our knowledge, some works have done on transmission dynamics of COVID-19 in mainland China or Wuhan city [6,7,8,9,10,11,12,13], but few study has focused on Shanxi Province. Therefore, understanding the factors influencing COVID-19 outbreaks has become a major provincial public health priority in Shanxi Province.
In this paper, to make predictions and perform assessment and risk analysis for COVID-19 outbreak of Shanxi Province, we developed a SEIQR difference-equation model of COVID-19 that takes into account the transmission with discrete time imported cases. Then the model parameters were estimated by the extensive MCMC method, and numerical simulations support the data reasonably well. Finally, sensitivity analyses are conducted to inform the earlier with Wuhan city lock-down, the fewer cases in Shanxi and other imported provinces.
In this study, we developed a SEIQR difference-equation model to describe the transmission of COVID-19 in Shanxi Province. The model classifies the human population (N(t)) into susceptible cases S(t), exposed cases E(t), infectious cases I(t), confirmed cases Q(t) and recover cases R(t) compartments. Human population birth and death rates are ignored, and the human host incubation period is 1/δ days. A(t) and B(t) are the imported COVID-19 cases at discrete time t, which are considered entering the exposed compartment and infected compartment. Infectious cases at the rate m revert to confirmed cases and the recover rate is γ. Susceptible humans acquire COVID-19 through direct contact with exposed cases and infected cases at rates βS(t)E(t)N(t) and β1S(t)I(t)N(t), respectively. The transmission dynamic of COVID-19 diagram in Shanxi Province is shown in Figure 3. Hence, the following difference equations are considered to describe the COVID-19 transmission model:
{S(t+1)=S(t)−βS(t)E(t)+β1S(t)I(t)N(t),E(t+1)=E(t)+A(t)+βS(t)E(t)+β1S(t)I(t)N(t)−δE(t),I(t+1)=I(t)+B(t)+δE(t)−mI(t),Q(t+1)=Q(t)+mI(t)−γQ(t),R(t+1)=R(t)+γQ(t),N(t)=S(t)+E(t)+I(t)+Q(t)+R(t). | (2.1) |
All parameters of system are assumed to be nonnegative. If there does not exist imported cases A(t) and B(t), system (2.1) will become the following difference equations:
{S(t+1)=S(t)−βS(t)E(t)+β1S(t)I(t)N(t),E(t+1)=E(t)+βS(t)E(t)+β1S(t)I(t)N(t)−δE(t),I(t+1)=I(t)+δE(t)−mI(t),Q(t+1)=Q(t)+mI(t)−γQ(t),R(t+1)=R(t)+γQ(t),N(t)=S(t)+E(t)+I(t)+Q(t)+R(t). | (2.2) |
It is easy to obtain that the reproduction number of system (2.2) by using the method of paper [14,15], and which is
R0=βδ+β1m. |
By means of statistical analysis the life track of all laboratory-confirmed cases, we found the last infected case appeared at February 10 (the other four cases after this time were asymptomatic patients transferred to diagnosed patients). One can conclude that the last infected case was infected on February 05, when the incubation period is about 5.2 days (95% confidence interval [CI], 4.1 to 7.0) [1]. Hence, the transmission of COVID-19 in Shanxi Province can be divided into two stages. The first stage is used to give parameter estimation from January 14 to February 05, and the second stage is used to validate the accuracy of proposed model from February 05 to February 28 without any transmissions.
The human population is assumed to be fully susceptible to the virus. Hence, suppose that the initial value of the susceptible population S equals to 3.718×107, which is Year-end population of 2018 for Shanxi province [17], and while all others (including E,I,Q,R) are 0. In previous paper [1], they obtained that the mean incubation period is 5.2 days (95% confidence interval [CI], 4.1 to 7.0), so we choose the value of δ is 15.2. By means of statistical analysis of all laboratory-confirmed cases, we obtain the period between symptom onset and laboratory confirmation of the first stage is about 9 days, and the value of m is 0.11. For parameter γ, it depends on the actual situation of infected cases, and also does not influence the model simulation. Therefore, we assume γ=1/14. In Shanxi province, Shanxi provincial government initiated a first-level emergency response on January 25, after that all people from Hubei Province, whose family members, and related contacts were registered and complied with home quarantine for at least 14 days. In this situation, if someone has a fever, he or she will avoid contact with others. Hence, the transmission rate β1 will be less than β, and we assume that the proportion between β1 and β is 1/14 (which also means β1=1/14∗β). In order to estimate the value of parameter β, we use extensive Markov-chain Monte-Carlo (MCMC) simulations based on the adaptive combination Delayed rejection and Adaptive Metropolis (DRAM) algorithm [18,19] for system (2.1). Using 100000 sample realizations, we can acquire the parameter value for β with 1D parameter MCMC chain in Figure 4. Then we further get the mean value, the standard deviation, MCMC error and Geweke for parameter β, which are shown in Table 1. From Figure 4 and the value of Geweke, it is easy to see that the Markov-chain of parameter β is convergent. If there does not exist the imported cases for this model, we can obtain the mean value and standard deviation of R0 are 0.4494 and 0.0165, respectively. And which also means the epidemic will disappear in the future with current control measures.
Notation | Mean Value | Standard Deviation | MCMC error | Geweke |
β | 0.0768 | 0.0028 | 1.6453e-05 | 0.9998 |
For the first stage, the time evolution of both theoretical infectious cases and comparison with the infected of COVID-19 cases in Shanxi Province is shown in Figure 5, which also shows the 95% percent interval for all 100000 passing simulation trajectories and the median of these 100000 simulation outputs. It is clear that the theoretical prediction is nearly full agreement with real data, which also well validates the accuracy of proposed model. Then, system (2.2) was used to fit the infectious cases without any transmissions from February 05 to February 28. The adaptive Metropolis-Hastings algorithm was also used to carry out extensive Markov-chain Monte-Carlo (MCMC) simulations, and the mean, standard deviation, MCMC error and Geweke value of δ are shown in Table 2.
Notation | Mean Value | Standard Deviation | MCMC error | Geweke |
δ | 0.2860 | 0.0215 | 1.4622e-05 | 0.9970 |
With the uncertainty for estimated parameters values, Monte Carlo simulation runs are then conducted to assess the performance of the model by using the available model parameter in Tables 1 and 2. Figure 6 unveils the prospect of the epidemic in Shanxi province with cumulative number of infected COVID-19 cases in Shanxi Province, shows that the 95% percent interval for all 100000 passing simulation trajectories and the median of these 100000 simulation outputs. The disease will disappear in late February and the mean final scale of cases will reach 133 (95% CI: 131–135).
Due to the assumed model parameter proportion between β1 and β with substantial uncertainty in their values, we gave another six different proportions between β1 and β to estimate parameter β by using same actual data and same method of MCMC, and the results were shown in Table 3. Table 3 show that the value of β decreases huge as the proportion between β1 and β increasing from 0.01 to 1. From previous assumption we know that the proportion value between β1 and β is also meaning the control intensity for infected cases, which are inverse proportional relationship. The more control intensity, the less value of R0 by using same data for parameter estimation. Figure 7 shows the prospect of the epidemic for different proportions between β1 and β in Shanxi Province without any other control measures from February 05. From the figure we can obtain that the more control intensity for infectious people, the less final size of epidemic.
Parameter | β1=0.01β | β1=0.05β | β1=0.1β | |||||
Notation | Mean | Std | Mean | Std | Mean | Std | ||
β | 0.0815 | 0.0030 | 0.0784 | 0.0029 | 0.0749 | 0.0028 | ||
R0 | 0.4311 | 0.0159 | 0.4435 | 0.0162 | 0.4573 | 0.0169 | ||
Parameter | β1=0.2β | β1=0.5β | β1=β | |||||
Notation | Mean | Std | Mean | Std | Mean | Std | ||
β | 0.0687 | 0.0025 | 0.0549 | 0.0021 | 0.0412 | 0.0016 | ||
R0 | 0.4819 | 0.0178 | 0.5354 | 0.0201 | 0.5885 | 0.0229 |
As we know, the outbreak of COVID-19 was reported in Wuhan, then the confirmed cases from Wuhan started to appear in other Chinese provinces until January 13, 2020. In order to control the transmission of COVID-19, the Chinese authorities introduced the implementation of the city lock-down strategy in Wuhan to shut down the movement on January 23, 2020. And the time of lock-down strategy in Wuhan affects the number of imported cases in other provinces. In this section, we will explore that the impact of different Wuhan city lock-down dates for the cumulative infected COVID-19 cases in Shanxi Province. On January 20, the leader of the high-level expert group of the National Health Commission announced that there existed person-to-person transmission, then more and more people left Wuhan City as far as possible. Hence, we will give simulation results with three different Wuhan city lock-down dates, which is shown in Figure 8. The dates of Wuhan city lock-down were crucial in producing the outbreak pattern in Shanxi Province. It is obtained that the earlier with city lock-down, the fewer cases, and the later with city lock-down, the more cases. If the city lock-down date ahead two days, the final scale of cases will decrease one half (67, 95% CI: 66–68). And if the city lock-down date delay for two days, the mean final scale of cases will reach about 196 (95% CI: 193–199).
In 2020, an outbreak of COVID-19 epidemic was reported in mainland China. Since January 23, 2020, government has been seeking various prevention and control measures to drastically reduce within-population contact rates and transmission. These measures included travel restriction and quarantine of both suspected individuals and subjects who have had close contacts with suspected cases, mandatory registration of all people arriving from Hubei Province, the registered individuals with home quarantine for at least 14 days, and extending the Spring Festival holiday. By the end of February 2020, the COVID-19 epidemic of China has been basically controlled in all provinces and cities except Hubei province. In Shanxi Province, by organizing the detailed information of confirmed cases, the cumulative confirmed cases arrived 133 at the end of February, and most of which were imported case from Hubei Province (89 cases). To the best of our knowledge, few study has focused on Shanxi Province to study the transmission of COVID-19. To investigate the underlying dynamics of COVID-19 transmission in Shanxi province, we developed a SEIQR difference-equation model of COVID-19 that took into account the transmission with discrete time imported cases, to make predictions and perform assessment and risk analysis.
Statistical analysis of the detailed cases demonstrates that the time from symptom to diagnosis in Shanxi Province is about 9 days. The detailed infectious cases from January 14 to February 05 were used to give parameter estimation for model (2.1). If there does not exist the imported cases for this model, we can obtain the mean value and standard deviation of R0 are 0.4494 and 0.0165, respectively. In our simulation, the disease disappeared in late February and the mean final scale of cases reached 133 (95% CI: 131–135). Our findings suggested that the earlier with Wuhan city lock-down, the fewer cases, and the later with Wuhan city lock-down, the more cases. If the city lock-down date ahead two days, the final scale of cases will decrease one half (67, 95% CI: 66–68). And if the city lock-down date delay for two days, the mean final scale of cases will reach about 196 (95% CI: 193–199). Hence, the travel restrictions could be instrumental to national and international agencies for public health response planning, and the earlier the better for other provinces except Hubei in mainland China.
Compared to previous ordinary differential equation models, our model is a difference equation model. The advantage of our model is that it is not necessary to estimate the initial value of the model, and the Markov-chain of model parameter β is convergent (See Figure 4). It should be noted that the dynamical model developed in this study could increase the understanding of the spread and control of COVID-19, which could be potentially helpful to study the transmission of COVID-19, in other provinces of China except Hubei. We hope our work is a good studying point for the countries with the first confirmed case is imported.
The project is funded by the National Natural Science Foundation of China under Grants (11801398, 11671241, 61873154, 11601292, 61673151), Natural Science Foundation of Shan'Xi Province Grant No. 201801D221024, Research Project Supported by key research and development projects projects in Shanxi Province (202003D31011/GZ), and National Key Research and Development Project of China (2019YFC1200500; 2019YFC1200501), Zhejiang Provincial Natrual Science Foundation of China (Grant Nos. LR18A050001 and LR18A050004), and the Major Project of The Natinoal Social Science Fund of China (Grant No. 19ZDA324).
All authors declare no conflicts of interest in this paper.
[1] |
Q. Li, X. Guan, P. Wu, X. Wang, L. Zhou, Y. Tong, et al., Early Transmission Dynamics in Wuhan, China, of Novel Coronavirus-Infected Pneumonia, N. Engl. J. Med., 382 (2020), 1199-1207. doi: 10.1056/NEJMoa2001316
![]() |
[2] | A. Pan, L. Liu, C. Wang, H. Guo, X. Hao, Q. Wang, et al., Association of Public Health Interventions With the Epidemiology of the COVID-19 Outbreak inWuhan, China, JAMA-J Am. Med. Assoc., (2020), e206130. |
[3] |
M. Chinazzi, J. T. Davis, M. Ajelli, C. Gioannini, M. Litvinova, S. Merler, et al., The effect of travel restrictions on the spread of the 2019 novel coronavirus (2019-nCoV) outbreak, Science, 368 (2020), 395-400. doi: 10.1126/science.aba9757
![]() |
[4] | J. Zhang, G. Q. Sun, M. T. Li, W. Zhang, L. Chang, Z. Jia, et al., 2019 coronavirus disease (COVID-19) propagation prediction and assessment method with imported cases and generations: Shanxi province as a case, (2020), Submitted. |
[5] | Health Commission of Shanxi province. Available from: http://wjw.shanxi.gov.cn/ |
[6] | B. Tang, X. Wang, Q. Li, N. L. Bragazzi, S. Y. Tang, Y. N. Xiao, et al., Estimation of the transmission risk of the 2019-nCoV and its implication for public health interventions, J. Clin. Med., 9 (2020), 462. |
[7] | B. Tang, F. Xia, S. Tang, N. L. Bragazzi, Q. Li, X. Sun, et al., The Evolution of Quarantined and Suspected Cases Determines the Final Trend of the 2019-nCoV Epidemics Based on Multi-Source Data Analyses, Available at SSRN 3537099, 2020. |
[8] | L. Danon, E. Brooks-Pollock, M. Bailey, M. J. Keeling, A spatial model of CoVID-19 transmission in England and Wales: early spread and peak timing, medRxiv, (2020). |
[9] |
J. T. Wu, K. Leung, G. M. Leung, Nowcasting and forecasting the potential domestic and international spread of the 2019-nCoV outbreak originating in Wuhan, China: a modelling study, Lancet, 395 (2020), 689-697. doi: 10.1016/S0140-6736(20)30260-9
![]() |
[10] |
Z. Du, L. Wang, S. Cauchemez, X. Xu, X. Wang, B. J. Cowling, et al., Risk for transportation of 2019 novel coronavirus disease from Wuhan to other cities in China, Emerg. Infect. Dis., 26 (2020), 1049-1052. doi: 10.3201/eid2605.200146
![]() |
[11] | Z. F. Yang, Z. Q. Zeng, K. Wang, S. S. Wong, W. Liang, M. Zanin, et al., Modified SEIR and AI prediction of the epidemics trend of COVID-19 in China under public health interventions, J. Thorac. Dis., 12 (2020), 165-174. |
[12] |
T. M. Chen, J. Rui, Q. P. Wang, Z. Y. Zhao, J. A. Cui, L. Yin, A mathematical model for simulating the phase-based transmissibility of a novel coronavirus, Infect. Dis. Poverty., 9 (2020), 1-8. doi: 10.1186/s40249-019-0617-6
![]() |
[13] | S. Zhao, S. Musa, Q. Lin, J. Ran, G. Yang, W. Wang, et al., Estimating the unreported number of novel coronavirus (2019-nCoV) vases in China in the first half of January 2020: a data-driven modelling analysis of the early outbreak, J. Clin. Med., 9 (2020), 388. |
[14] | M. T. Li, G. Q. Sun, J. Zhang, Z. Jin, Transmission dynamics of a multi-group brucellosis model with mixed cross infection in public farm, Appl. Math. Comput., 237 (2014), 582-594. |
[15] |
M. T. Li, Z. Jin, G. Q. Sun, J. Zhang, Modeling direct and indirect disease transmission using multi-group model, J. Math. Anal. Appl., 446 (2017), 1292-1309. doi: 10.1016/j.jmaa.2016.09.043
![]() |
[16] | J. Geweke, Evaluating the accuracy of sampling-based approaches to the calculation of posterior moments. In: J.M. Bernardo, J. Berger, A.P. Dawid, A.F.M. Smith (Eds.), Bayesian Statistics, Oxford University Press, Oxford, 4 (1992), 169-193. |
[17] | China population & employment statistics yearbook. Beijing: China Statistics Press, (2019). |
[18] | H. Haario, M. Laine, A. Mira, E. Saksman, Dram: Efficient adaptive mcmc, Stat. Comput., 16 (2006), 339-354. |
[19] | D. Gamerman, H. F. Lopes, Markov chain monto carlo: stochastic simulation for bayesian inference, 2nd ed. Taylor and Francis Group, London New York, 2006. |
1. | Guo-Rong Xing, Ming-Tao Li, Li Li, Gui-Quan Sun, The Impact of Population Migration on the Spread of COVID-19: A Case Study of Guangdong Province and Hunan Province in China, 2020, 8, 2296-424X, 10.3389/fphy.2020.587483 | |
2. | Rong Yuan, Yangjun Ma, Congcong Shen, Jinqing Zhao, Xiaofeng Luo, Maoxing Liu, Global dynamics of COVID-19 epidemic model with recessive infection and isolation, 2021, 18, 1551-0018, 1833, 10.3934/mbe.2021095 | |
3. | Meng Liu, Raphael Thomadsen, Song Yao, Forecasting the Spread of COVID-19 under Different Reopening Strategies, 2020, 1556-5068, 10.2139/ssrn.3607977 | |
4. | Nao Yamamoto, Bohan Jiang, Haiyan Wang, Quantifying compliance with COVID-19 mitigation policies in the US: A mathematical modeling study, 2021, 6, 24680427, 503, 10.1016/j.idm.2021.02.004 | |
5. | Cody Carroll, Satarupa Bhattacharjee, Yaqing Chen, Paromita Dubey, Jianing Fan, Álvaro Gajardo, Xiner Zhou, Hans-Georg Müller, Jane-Ling Wang, Time dynamics of COVID-19, 2020, 10, 2045-2322, 10.1038/s41598-020-77709-4 | |
6. | Qinxia Wang, Shanghong Xie, Yuanjia Wang, Donglin Zeng, Survival-Convolution Models for Predicting COVID-19 Cases and Assessing Effects of Mitigation Strategies, 2020, 8, 2296-2565, 10.3389/fpubh.2020.00325 | |
7. | Meng Liu, Raphael Thomadsen, Song Yao, Forecasting the spread of COVID-19 under different reopening strategies, 2020, 10, 2045-2322, 10.1038/s41598-020-77292-8 | |
8. | Francesco Capuano, Modeling Growth, Containment and Decay of the COVID-19 Epidemic in Italy, 2020, 8, 2296-424X, 10.3389/fphy.2020.586180 | |
9. | Gui-Quan Sun, Shi-Fu Wang, Ming-Tao Li, Li Li, Juan Zhang, Wei Zhang, Zhen Jin, Guo-Lin Feng, Transmission dynamics of COVID-19 in Wuhan, China: effects of lockdown and medical resources, 2020, 101, 0924-090X, 1981, 10.1007/s11071-020-05770-9 | |
10. | Ming-Tao Li, Jin Cui, Juan Zhang, Gui-Quan Sun, Transmission analysis of COVID-19 with discrete time imported cases: Tianjin and Chongqing as cases, 2021, 24680427, 10.1016/j.idm.2021.03.007 | |
11. | A. Q. Khan, M. Tasneem, M. B. Almatrafi, Discrete-time COVID-19 epidemic model with bifurcation and control, 2021, 19, 1551-0018, 1944, 10.3934/mbe.2022092 | |
12. | S.M.E.K. Chowdhury, Mohammad Forkan, Shams Forruque Ahmed, Praveen Agarwal, A.B.M. Shawkat Ali, S.M. Muyeen, Modeling the SARS-CoV-2 parallel transmission dynamics: Asymptomatic and symptomatic pathways, 2022, 143, 00104825, 105264, 10.1016/j.compbiomed.2022.105264 | |
13. | Leishi Wang, Mingtao Li, Xin Pei, Juan Zhang, Optimal Breeding Strategy for Livestock with a Dynamic Price, 2022, 10, 2227-7390, 1732, 10.3390/math10101732 | |
14. | Ayman Mourad, Fatima Mroue, Zahraa Taha, Stochastic mathematical models for the spread of COVID-19: a novel epidemiological approach, 2022, 39, 1477-8599, 49, 10.1093/imammb/dqab019 | |
15. | Keisuke Ejima, Kwang Su Kim, Ana I. Bento, Shoya Iwanami, Yasuhisa Fujita, Kazuyuki Aihara, Kenji Shibuya, Shingo Iwami, Estimation of timing of infection from longitudinal SARS-CoV-2 viral load data: mathematical modelling study, 2022, 22, 1471-2334, 10.1186/s12879-022-07646-2 | |
16. | Min Peng, Jianing Zhang, Jingrui Gong, Xingqi Ran, Jvlu Liu, Lin Zhang, Epidemic modeling for the resurgence of COVID-19 in Chinese local communities, 2022, 3, 26664496, 229, 10.1016/j.jnlssr.2022.03.005 | |
17. | Jin Guo, Aili Wang, Weike Zhou, Yinjiao Gong, Stacey R. Smith?, Discrete epidemic modelling of COVID-19 transmission in Shaanxi Province with media reporting and imported cases, 2021, 19, 1551-0018, 1388, 10.3934/mbe.2022064 | |
18. | Chung-Han Hsieh, On Robust Economic Control of Epidemics With Application to COVID-19, 2021, 9, 2169-3536, 167948, 10.1109/ACCESS.2021.3136191 | |
19. | Satarupa Bhattacharjee, Shuting Liao, Debashis Paul, Sanjay Chaudhuri, Inference on the dynamics of COVID-19 in the United States, 2022, 12, 2045-2322, 10.1038/s41598-021-04494-z | |
20. | S. A. A. Biabani, N. A. Tayyib, A Review on the Use of Machine Learning Against the Covid-19 Pandemic, 2022, 12, 1792-8036, 8039, 10.48084/etasr.4628 | |
21. | Jingli Ren, Haiyan Wang, 2023, 9780443186790, 173, 10.1016/B978-0-44-318679-0.00012-0 | |
22. | Erik A. Vázquez Jiménez, Jesús Martínez Martínez, Leonardo D. Herrera Zuniga, J. Guadalupe Reyes Victoria, Discrete models for analyzing the behavior of COVID-19 pandemic in the State of Mexico, Mexico, 2022, 20, 1551-0018, 296, 10.3934/mbe.2023014 | |
23. | Mingtao Li, Jin Cui, Juan Zhang, Xin Pei, Guiquan Sun, Transmission characteristic and dynamic analysis of COVID-19 on contact network with Tianjin city in China, 2022, 608, 03784371, 128246, 10.1016/j.physa.2022.128246 | |
24. | Jiang Long, Chenxi Dai, Shanshan Kuang, Han Zhao, Dan Liu, Qing Luo, Kaifa Wang, A switching dynamic model based on phased COVID-19 data in Chongqing and its evaluation, 2022, 100, 15671348, 105270, 10.1016/j.meegid.2022.105270 | |
25. | Sacrifice Nana-Kyere, Francis Agyei Boateng, Paddy Jonathan, Anthony Donkor, Glory Kofi Hoggar, Banon Desmond Titus, Daniel Kwarteng, Isaac Kwasi Adu, Andrei Korobeinikov, Global Analysis and Optimal Control Model of COVID-19, 2022, 2022, 1748-6718, 1, 10.1155/2022/9491847 | |
26. | Abdullah Ali H. Ahmadini, Muhammad Naeem, Muhammad Aamir, Raimi Dewan, Shokrya Saleh A. Alshqaq, Wali Khan Mashwani, Analysis and Forecast of the Number of Deaths, Recovered Cases, and Confirmed Cases From COVID-19 for the Top Four Affected Countries Using Kalman Filter, 2021, 9, 2296-424X, 10.3389/fphy.2021.629320 | |
27. | Michael J. Tildesley, Anna Vassall, Steven Riley, Mark Jit, Frank Sandmann, Edward M. Hill, Robin N. Thompson, Benjamin D. Atkins, John Edmunds, Louise Dyson, Matt J. Keeling, Optimal health and economic impact of non-pharmaceutical intervention measures prior and post vaccination in England: a mathematical modelling study, 2022, 9, 2054-5703, 10.1098/rsos.211746 | |
28. | Buddhi Pantha, Subas Acharya, Hem Raj Joshi, Naveen K. Vaidya, Inter-provincial disparity of COVID-19 transmission and control in Nepal, 2021, 11, 2045-2322, 10.1038/s41598-021-92253-5 | |
29. | Ajay Kumar, Tsan-Ming Choi, Samuel Fosso Wamba, Shivam Gupta, Kim Hua Tan, Infection vulnerability stratification risk modelling of COVID-19 data: a deterministic SEIR epidemic model analysis, 2021, 0254-5330, 10.1007/s10479-021-04091-3 | |
30. | Carlos I. Mendoza, Inhomogeneous Transmission and Asynchronic Mixing in the Spread of COVID-19 Epidemics, 2021, 9, 2296-424X, 10.3389/fphy.2021.683364 | |
31. | Sarita Bugalia, Jai Prakash Tripathi, Hao Wang, Mathematical modeling of intervention and low medical resource availability with delays: Applications to COVID-19 outbreaks in Spain and Italy, 2021, 18, 1551-0018, 5865, 10.3934/mbe.2021295 | |
32. | Rahul Mishra, Hari Prabhat Gupta, Tanima Dutta, Analysis, Modeling, and Representation of COVID-19 Spread: A Case Study on India, 2021, 8, 2329-924X, 964, 10.1109/TCSS.2021.3077701 | |
33. | Harendra Pal Singh, Sumit Kaur Bhatia, Yashika Bahri, Riya Jain, Optimal control strategies to combat COVID-19 transmission: A mathematical model with incubation time delay, 2022, 9, 26667207, 100176, 10.1016/j.rico.2022.100176 | |
34. | M. El Sayed, M. A. El Safty, M. K. El-Bably, Topological approach for decision-making of COVID-19 infection via a nano-topology model, 2021, 6, 2473-6988, 7872, 10.3934/math.2021457 | |
35. | Xiang Wei, Junchan Zhao, Shuai Liu, Yisi Wang, Identifying influential spreaders in complex networks for disease spread and control, 2022, 12, 2045-2322, 10.1038/s41598-022-09341-3 | |
36. | David Jaurès Fotsa-Mbogne, Stéphane Yanick Tchoumi, Yannick Kouakep-Tchaptchie, Vivient Corneille Kamla, Jean-Claude Kamgang, Duplex Elvis Houpa-Danga, Samuel Bowong-Tsakou, David Bekolle, Estimation and optimal control of the multiscale dynamics of Covid-19: a case study from Cameroon, 2021, 106, 0924-090X, 2703, 10.1007/s11071-021-06920-3 | |
37. | Alessio Notari, Giorgio Torrieri, COVID-19 transmission risk factors, 2022, 116, 2047-7724, 146, 10.1080/20477724.2021.1993676 | |
38. | Wenjun Jing, Juping Zhang, Xiaoqin Zhang, Siew Ann Cheong, Epidemic Dynamics in Temporal Clustered Networks with Local-World Structure, 2023, 2023, 1099-0526, 1, 10.1155/2023/4591403 | |
39. | Hyojung Lee, Geunsoo Jang, Giphil Cho, Forecasting COVID-19 cases by assessing control-intervention effects in Republic of Korea: A statistical modeling approach, 2022, 61, 11100168, 9203, 10.1016/j.aej.2022.02.037 | |
40. | Vanshika Aggarwal, Geeta Arora, Homan Emadifar, Faraidun K. Hamasalh, Masoumeh Khademi, Sania Qureshi, Numerical Simulation to Predict COVID-19 Cases in Punjab, 2022, 2022, 1748-6718, 1, 10.1155/2022/7546393 | |
41. | Yas Al-Hadeethi, Intesar F El Ramley, M. I. Sayyed, Convolution model for COVID-19 rate predictions and health effort levels computation for Saudi Arabia, France, and Canada, 2021, 11, 2045-2322, 10.1038/s41598-021-00687-8 | |
42. | 2023, 9780443186790, 229, 10.1016/B978-0-44-318679-0.00013-2 | |
43. | Muhammad Naeem, Wali Khan Mashwani, Mohammad ABIAD, Habib Shah, Zardad Khan, Muhammad Aamir, Soft computing techniques for forecasting of COVID-19 in Pakistan, 2023, 63, 11100168, 45, 10.1016/j.aej.2022.07.029 | |
44. | R. Prem Kumar, Sanjoy Basu, P.K. Santra, D. Ghosh, G.S. Mahapatra, Optimal control design incorporating vaccination and treatment on six compartment pandemic dynamical system, 2022, 7, 26667207, 100115, 10.1016/j.rico.2022.100115 | |
45. | Daohan Huang, Fenghua Wen, Shunru Li, Addressing External Shock in Urban Agglomeration: Implications From the Transmission Pattern of COVID-19 in the Beijing-Tianjin-Hebei Area, 2022, 10, 2296-2565, 10.3389/fpubh.2022.870214 | |
46. | Eduarda T. C. Chagas, Pedro H. Barros, Isadora Cardoso-Pereira, Igor V. Ponte, Pablo Ximenes, Flávio Figueiredo, Fabricio Murai, Ana Paula Couto da Silva, Jussara M. Almeida, Antonio A. F. Loureiro, Heitor S. Ramos, Sergio Gómez, Effects of population mobility on the COVID-19 spread in Brazil, 2021, 16, 1932-6203, e0260610, 10.1371/journal.pone.0260610 | |
47. | Jia-Tong Li, Li Li, The Impacts of Reducing the Infection Rate and Infection Source on the Transmission of Emerging Infectious Diseases, 2022, 2022, 1607-887X, 1, 10.1155/2022/3473538 | |
48. | Nora El-Rashidy, Samir Abdelrazik, Tamer Abuhmed, Eslam Amer, Farman Ali, Jong-Wan Hu, Shaker El-Sappagh, Comprehensive Survey of Using Machine Learning in the COVID-19 Pandemic, 2021, 11, 2075-4418, 1155, 10.3390/diagnostics11071155 | |
49. | Gianmario Rinaldi, Prathyush P. Menon, Antonella Ferrara, W. David Strain, Christopher Edwards, Epidemiological model based periodic intervention policies for COVID-19 mitigation in the United Kingdom, 2022, 12, 2045-2322, 10.1038/s41598-022-19630-6 | |
50. | Nitesh Kumawat, Mubasher Rashid, Akriti Srivastava, Jai Prakash Tripathi, Hysteresis and Hopf bifurcation: Deciphering the dynamics of an in-host model of SARS-CoV-2 with logistic target cell growth and sigmoidal immune response, 2023, 176, 09600779, 114151, 10.1016/j.chaos.2023.114151 | |
51. | Sanjay Bhatter, Sangeeta Kumawat, Bhamini Bhatia, Sunil Dutt Purohit, Analysis of COVID-19 epidemic with intervention impacts by a fractional operator, 2024, 14, 2146-5703, 261, 10.11121/ijocta.1515 | |
52. | Ibad Ullah, Nigar Ali, Ihtisham Ul Haq, Imtiaz Ahmad, Mohammed Daher Albalwi, Md. Haider Ali Biswas, Kareem T. Elgindy, Analysis of COVID-19 Disease Model: Backward Bifurcation and Impact of Pharmaceutical and Nonpharmaceutical Interventions, 2024, 2024, 1687-0425, 1, 10.1155/2024/6069996 | |
53. | Jia-Lin Wang, Xin-Long Xiao, Fen-Fen Zhang, Xin Pei, Ming-Tao Li, Ju-Ping Zhang, Juan Zhang, Gui-Quan Sun, Forecast of peak infection and estimate of excess deaths in COVID-19 transmission and prevalence in Taiyuan City, 2022 to 2023, 2024, 9, 24680427, 56, 10.1016/j.idm.2023.11.005 | |
54. | Jiraporn Lamwong, Napasool Wongvanich, I-Ming Tang, Puntani Pongsumpun, Optimal Control Strategy of a Mathematical Model for the Fifth Wave of COVID-19 Outbreak (Omicron) in Thailand, 2023, 12, 2227-7390, 14, 10.3390/math12010014 | |
55. | 思凡 王, Analysis of Equilibrium Points of a Class of Infectious Disease Models with Environmental Viral Load and Vaccine Effects, 2024, 14, 2160-7583, 447, 10.12677/pm.2024.145199 | |
56. | JAI PRAKASH TRIPATHI, NITESH KUMAWAT, KOMAL TANWAR, DHANUMJAYA PALLA, MAIA MARTCHEVA, TRANSMISSION DYNAMICS OF COVID-19 WITH DIABETES IN INDIA: A COST-EFFECTIVE AND OPTIMAL CONTROL ANALYSIS, 2024, 32, 0218-3390, 643, 10.1142/S0218339024500232 | |
57. | Shiqian Nie, Xiaochun Lei, A time-dependent model of the transmission of COVID-19 variants dynamics using Hausdorff fractal derivative, 2023, 629, 03784371, 129196, 10.1016/j.physa.2023.129196 |
Notation | Mean Value | Standard Deviation | MCMC error | Geweke |
β | 0.0768 | 0.0028 | 1.6453e-05 | 0.9998 |
Notation | Mean Value | Standard Deviation | MCMC error | Geweke |
δ | 0.2860 | 0.0215 | 1.4622e-05 | 0.9970 |
Parameter | β1=0.01β | β1=0.05β | β1=0.1β | |||||
Notation | Mean | Std | Mean | Std | Mean | Std | ||
β | 0.0815 | 0.0030 | 0.0784 | 0.0029 | 0.0749 | 0.0028 | ||
R0 | 0.4311 | 0.0159 | 0.4435 | 0.0162 | 0.4573 | 0.0169 | ||
Parameter | β1=0.2β | β1=0.5β | β1=β | |||||
Notation | Mean | Std | Mean | Std | Mean | Std | ||
β | 0.0687 | 0.0025 | 0.0549 | 0.0021 | 0.0412 | 0.0016 | ||
R0 | 0.4819 | 0.0178 | 0.5354 | 0.0201 | 0.5885 | 0.0229 |
Notation | Mean Value | Standard Deviation | MCMC error | Geweke |
β | 0.0768 | 0.0028 | 1.6453e-05 | 0.9998 |
Notation | Mean Value | Standard Deviation | MCMC error | Geweke |
δ | 0.2860 | 0.0215 | 1.4622e-05 | 0.9970 |
Parameter | β1=0.01β | β1=0.05β | β1=0.1β | |||||
Notation | Mean | Std | Mean | Std | Mean | Std | ||
β | 0.0815 | 0.0030 | 0.0784 | 0.0029 | 0.0749 | 0.0028 | ||
R0 | 0.4311 | 0.0159 | 0.4435 | 0.0162 | 0.4573 | 0.0169 | ||
Parameter | β1=0.2β | β1=0.5β | β1=β | |||||
Notation | Mean | Std | Mean | Std | Mean | Std | ||
β | 0.0687 | 0.0025 | 0.0549 | 0.0021 | 0.0412 | 0.0016 | ||
R0 | 0.4819 | 0.0178 | 0.5354 | 0.0201 | 0.5885 | 0.0229 |