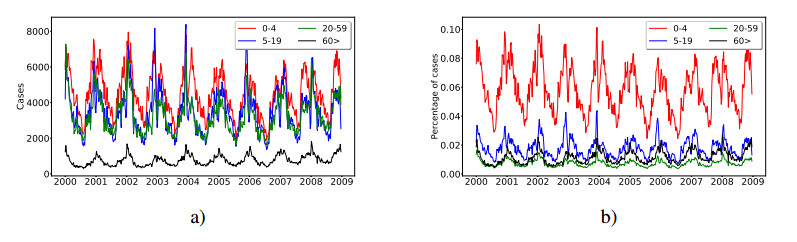
Citation: Yendry N. Arguedas, Mario Santana-Cibrian, Jorge X. Velasco-Hernández. Transmission dynamics of acute respiratory diseases in a population structured by age[J]. Mathematical Biosciences and Engineering, 2019, 16(6): 7477-7493. doi: 10.3934/mbe.2019375
[1] | Qiuyi Su, Jianhong Wu . Impact of variability of reproductive ageing and rate on childhood infectious disease prevention and control: insights from stage-structured population models. Mathematical Biosciences and Engineering, 2020, 17(6): 7671-7691. doi: 10.3934/mbe.2020390 |
[2] | Pengyan Liu, Hong-Xu Li . Global behavior of a multi-group SEIR epidemic model with age structure and spatial diffusion. Mathematical Biosciences and Engineering, 2020, 17(6): 7248-7273. doi: 10.3934/mbe.2020372 |
[3] | Yicang Zhou, Zhien Ma . Global stability of a class of discrete age-structured SIS models with immigration. Mathematical Biosciences and Engineering, 2009, 6(2): 409-425. doi: 10.3934/mbe.2009.6.409 |
[4] | Andrey V. Melnik, Andrei Korobeinikov . Lyapunov functions and global stability for SIR and SEIR models withage-dependent susceptibility. Mathematical Biosciences and Engineering, 2013, 10(2): 369-378. doi: 10.3934/mbe.2013.10.369 |
[5] | Juan Pablo Aparicio, Carlos Castillo-Chávez . Mathematical modelling of tuberculosis epidemics. Mathematical Biosciences and Engineering, 2009, 6(2): 209-237. doi: 10.3934/mbe.2009.6.209 |
[6] | Jianxin Yang, Zhipeng Qiu, Xue-Zhi Li . Global stability of an age-structured cholera model. Mathematical Biosciences and Engineering, 2014, 11(3): 641-665. doi: 10.3934/mbe.2014.11.641 |
[7] | Amira Bouhali, Walid Ben Aribi, Slimane Ben Miled, Amira Kebir . Impact of immunity loss on the optimal vaccination strategy for an age-structured epidemiological model. Mathematical Biosciences and Engineering, 2024, 21(6): 6372-6392. doi: 10.3934/mbe.2024278 |
[8] | Frédérique Clément, Béatrice Laroche, Frédérique Robin . Analysis and numerical simulation of an inverse problem for a structured cell population dynamics model. Mathematical Biosciences and Engineering, 2019, 16(4): 3018-3046. doi: 10.3934/mbe.2019150 |
[9] | Simone De Reggi, Francesca Scarabel, Rossana Vermiglio . Approximating reproduction numbers: a general numerical method for age-structured models. Mathematical Biosciences and Engineering, 2024, 21(4): 5360-5393. doi: 10.3934/mbe.2024236 |
[10] | Karl Peter Hadeler . Structured populations with diffusion in state space. Mathematical Biosciences and Engineering, 2010, 7(1): 37-49. doi: 10.3934/mbe.2010.7.37 |
Acute respiratory diseases afflict populations all over the world and represent a serious health burden in many countries [1]. Respiratory syncytial virus (RSV) and influenza are particularly important as the main viruses that cause mortality and morbidity worldwide on a yearly basis [2,3,4,5,6]. It is estimated that 8.1% of all deaths between 2003 and 2009 were caused by influenza and RSV. 7478 Moreover adults over 60 years old present the highest mortality rate caused by Acute Respiratory Infections (ARIs) [7]. Age and other diseases such as diabetes, hypertension and obesity, are factors that compromise the recovery of individuals affected by these class of diseases [8].
It is estimated that, worldwide, 5% of elders and 20% of children develop influenza type A or B each year [9]. Children are the main disseminators of influenza because of the high contact rate within their age class and 50% of influenza infections in elders are caused by direct contact with children [10], implying that social distancing between grandparents and grandchildren can be effective in reducing the risk of this population. In [11,12] it is shown that school closures cause a decrease in the transmission of respiratory diseases, and the return of children after vacations increases them. However, the nature of the interaction between different age groups is not completely clear. Quantifying the influence of one age group upon the others, as well as its impact on ARIs transmission dynamics, is an important issue that must be addressed.
There are several studies in the literature related to the estimation of the transmission rate in these diseases [13,14,15,16,17]. According to [18,19] measuring the transmission rate is one of the most important factors necessary to predict the impact caused by public health programs and establish measures to mitigate disease impact on a population.
Age structure is a main determinant of ARIs dynamics. Contact rate, the level of susceptibility as well as immunity, among other aspects, may vary depending upon age. Unfortunately, there is scarce information on the impact that different age groups have on the propagation of ARIs. Usually, different transmission rates are proposed for each group of the population, sometimes derived from a contact matrix that describes the interaction between all groups.
Our work develops an age-structured epidemiological model for the transmission of ARIs. We fit the model to a dataset of ARIs from San Luis Potosí City in Mexico and use a Bayesian inference method to estimate the main parameters in the model in order to understand the role of age in ARIs transmission. The work is organized as follows. Section 2 presents a detailed description and preliminary analysis of the data. Section 3 describes the SEIRS model for the ARIs transmission dynamics, then section 4 explains the Bayesian inference methodology used to estimate the parameters of interest. The results of the analysis are presented in Section 5. Finally, the discussion and conclusion about our findings appears in Section 6.
Our data consists of weekly records of confirmed ARIs cases from the General Hospital of the State of San Luis Potosi, Mexico, reported by the State Department of Epidemiology and Health Services. There are reports available from 2000 to 2010 for each of four age groups. Group 1 corresponds to children 0 to 4 years old, Group 2 consists of young children and teenagers from 5 to 19, Group 3 has adults from 20 to 59 and Group 4 includes older adults 60 years old or higher. This ARIs data complies with the standard of the International Classification of Disease, 10th Review of the World Health Organization. Our data includes respiratory infections classified with the ICD-10 codes J00- J06, J20, J2, except J02.0 and J03.0 [7]. The number of infected individuals per week is shown in Figure 1 a). We note that the number of cases is similar in the first three age groups. The age class with lower cases corresponds to old adults. However, if we present the data as a percentage of the total population in each age group, it can be seen that children's group (0–4) has considerably more cases while the other three groups are more similar to each other (see Figure 1 b).
To analyze the data, we partition the time series into eight periods of one year each (52 consecutive weeks) starting from late July (30th week of 2000). Therefore, the periods are July 2000 to July 2001, July 2001 to July 2002, and so on. We do not include in our analysis data from 2008-2010 since the influenza outbreak of 2009 presents atypical characteristics when compared to the previous years. For each period we define a peak week as the calendar week with the highest number of ARIs cases. Then we identify a pre-peak period (epidemics' ascent) to start with the flu season that usually falls in early October (week 40), and a post-peak (epidemics' descent) to end with the winter season usually in late March (week 12) of the following year. Figure 2 shows data from 2000 to 2001 for each age group. The peek of the infection is indicated with a purple continuous line while the purple dashed lines represent the beginning and the end of the outbreak. The orange band indicates the two-week Easter holiday, and the purple band indicates summer and winter vacations, as read from the Mexican Public Education System (PES) calendar.
We observe that, for the school age groups (0-4 and 5-19), the first epidemic outbreak begins around the first week of August. By the end of December 2000 approximately, the number of cases decreases, to increase again two or three weeks later. These weeks coincide with the PES winter vacations (last two weeks of 2000 and the first week of 2001). For the group of older people, the peak week is the one farthest from the beginning of the rise of the epidemic compared to the same trend in other age classes.
Our data suggests that the start of vacations diminishes the incidence in school age individuals (age groups from 0 to 19 years old) and that the time when the number of infections begins to rise again is around the return to school. The opposite happens with elders, for which the start of vacations seems to be the time when they are more susceptible to ARIs. This pattern occurs in 75 % of the periods studied.
The spread of viruses and bacteria that cause ARIs is directly related to the number of contacts between individuals. Consequently, as our data suggests, it is natural to expect a decrease in cases of infection during school vacations. Other studies on similar kind of diseases [10,12,20] have confirmed what we observed in our data: vacation and social isolation decrease the number of new infections.
We calculate here some statistical measures that provide information about the risk of infection of each age group. The Relative Risk (RR) evaluates the change in the proportion of infected individuals in the population before and after the epidemic peak week relative to the total number of reported cases during the year. Following [8], the relative risk for age group k is defined by
RRk=Bk/∑4i=1BiAk/∑4i=1Ai,k=1,2,3,4, | (2.1) |
where the total number of cases occurring before and after the outbreak peak are denoted by Bk and Ak, respectively. The RRs values informs about the group that has a higher risk of infection in the pre-peak time. Estimates of relative risk for each group along with their 95% confidence intervals are shown in Table 1. Technical details regarding the calculation of the confidence intervals can be found in [21]. We observed that the age group 5-19 tends to have greater risk before the peak while group 20-59 tends to have higher risk after the peak. The pattern is not clear for both the children and the senior adults as the pre-peak and post-peak relative risks change depending on the year.
2000-2001 | 2001-2002 | 2002-2003 | 2003-2004 | 2004-2005 | 2005-2006 | 2006-2007 | 2007-2008 | |
5 < | 0.87 | 1.22 | 1.07 | 0.95 | 1.81 | 1.03 | 0.98 | 1.22 |
[0.86, 0.87] | [1.21, 1.23] | [1.07, 1.08] | [0.94, 0.96] | [1.79, 1.83] | [1.02, 1.04] | [0.97, 0.99] | [1.21, 1.24] | |
5–19 | 1.11 | 1.07 | 1.06 | 1.18 | 1.25 | 0.96 | 1.35 | 1.17 |
[1.10, 1.12] | [1.05, 1.08] | [1.05, 1.07] | [1.17, 1.19] | [1.23, 12.6] | [0.95, 0.97] | [1.33, 1.37] | [1.16, 1.19] | |
20–59 | 0.97 | 0.78 | 0.80 | 0.95 | 0.48 | 0.77 | 1.04 | 0.71 |
[0.96, 0.98] | [0.77, 0.78] | [0.79, 0.81] | [0.94, 0.96] | [0.47, 0.49] | [0.76, 0.78] | [1.03, 1.05] | [0.71, 0.72] | |
≥ 60 | 1.78 | 0.76 | 1.16 | 0.83 | 0.50 | 2.39 | 0.37 | 0.78 |
[1.73, 1.83] | [0.74, 0.78] | [1.14, 1.19] | [0.81, 0.85] | [0.48, 0.51] | [2.33, 2.45] | [0.36, 0.38] | [0.76, 0.80] |
To evaluate the role of age in the transmission of ARIs, we use a multi-group SEIRS deterministic model with age structure. Multi-group models have been introduced in the literature to describe the transmission dynamics of infectious diseases in heterogeneous host populations.
A multigroup model is formulated by dividing the population of size N(t) into m distinct groups. For 1≤k≤m, the k-th group is further partitioned into four compartments: the susceptible, latent, infectious, and recovered, whose numbers of individuals at time t are denoted by Sk(t),Ek(t),Ik(t) and Rk(t), respectively. Individuals are born susceptible and if they are exposed and infected, then show a latency period before becoming infectious. After their recovery, they remain immune for a period of time.
The influx of individuals into the first susceptible group is given by a constant νi, called the fertility rate. The sex-ratio is assumed to be 1:1. Within the k-th group, it is assumed that death occurs due to cardiovascular diseases, respiratory infections, etc. The average time in the latency state is given by 1/ηk. We assume that individuals in state Ik recover with a constant rate γk, and once recovered they remain immune (recovered compartment) to the disease during a mean time 1/ωk. Let 1/αk be the average time that one individuals remain in age group k. Finally, λk(t) denotes the force of the infection of group k at time t and it is given as
λk(t)=βk4∑j=1cjkIj(t)Nj(t)h(t), | (3.1) |
where Nj(t)=Sj(t)+Ej(t)+Ij(t)+Rj(t). The function
h(t)=ρ[1+ϕcos(2π52t)] | (3.2) |
describes seasonality in the contact rate [22]. The parameter βk is the infection risk of group k, or the probability that one contact made by a group k individual generates a new infection. The m×m-square matrix C=(cjk) is the contact matrix and each element cjk represents the average number of contacts between age groups j and k.
Our model is the following system of differential equations:
˙S1(t)=Γ+ω1Rk−(λk(t)+μk)Sk−α1S1,˙Sk(t)=ωkRk−(λk(t)+μk+αk)Sk+αk−1Sk−1,k=2,3,4,˙Ek(t)=λk(t)Sk−(μk+ηk+αk)Ek+αk−1Ek−1,˙Ik(t)=ηkEk−(μk+γk+αk)Ik+αk−1Ik−1,˙Rk(t)=γkEk−(μk+ωk+αk)Rk+αk−1Rk−1, | (3.3) |
where Γ=ν2X2+ν3X3 is the recruitment rate with X2, X3 representing the number of fertile women at the beginning of the epidemic in age group 2 and 3, respectively. It is only defined for the first group of susceptible because individuals are assumed to be born healthy. Model (3.3) is represented by the diagram in Figure 3. We make the following assumptions for the parameters in model (3.3):
(ⅰ) Γ,μk,γk,ηk,ωk are positive for all k∈{1,2,3,4}.
(ⅱ) α4=0.
(ⅲ) Let ¯βkj=βk⋅ckj. Here ¯βkj is nonnegative for all k,j∈{1,2,...,m} and the m×m-square matrix (¯βkj),1≤k,j≤m is irreducible.
(ⅳ) ν1=ν4=0 since the fertile period of a woman is considered from 15 to 49 years old.
We now would like to see whether our model is able to describe our data. In order to do so, it is necessary to provide adequate values for all the parameters involved. We use a Bayesian inference approach to estimate the values of relevant parameters as described in Section 2.
From the mathematical point of view, estimating the parameters of a system of differential equations is considered a non-linear inverse problem. The standard way to solve this class of problems is to use an optimization approach. The idea is to find a set of parameters that provide a solution as closer as possible to the data. A better alternative, from our perspective, is to see it as an inference problem. We use a Bayesian approach where the solution of the inverse problem is the analysis of the posterior distribution of the parameters of interest. The Bayesian framework provides a formal way to quantify uncertainty about the unknown quantities. The idea is to find the parameter set that generate solutions closer (in a probabilistic sense) to the observed data. Instead of keeping only one solution, as an optimization approach would, we consider a set of solutions.
To briefly describe the Bayesian inference methodology, some notation is needed. Let Y be a vector of observations (data) and let θ be a vector of parameters representing the unknown quantities of interest. From the Bayesian perspective, all the unknown quantities θ are considered random variables and the data Y is fixed. All the available knowledge regarding the unknowns is codified into a probability distribution known as prior with joint density function π(θ). The observational model, also known as the likelihood function, π(Y|θ), relates the data with the unknown quantities by describing the probability of the data if the true values of the parameters were known. The prior can be combined with the likelihood through Bayes theorem as follows
π(θ|Y)=π(Y|θ)π(θ)π(Y). | (4.1) |
Equation (4.1) is known as the posterior distribution of θ given the data Y and represents the updated knowledge. Until further data is available, this posterior distribution contains the only relevant information. Now that we have established the basic notation used in Bayesian inference, we can describe in detail how we use this approach to infer the quantities of interest in the SEIRS model.
Our data consists of weekly reports of ARIs confirmed cases. To account for the discrete nature of these counts, it is reasonable to assume that the observations are realizations of a Poisson distribution with a time dependent mean parameter. However, prior knowledge regarding this type of diseases suggest that the variance of the data is larger than the mean, which indicates that there is over-dispersion. Therefore, a more appropriate model is the Negative Binomial distribution since it has an additional parameter that allows the variance to exceed the mean [21].
We have four age groups and 52 weeks for each time period. Let Yik be the number of reported cases for week i and age group k, i=1,2,3,…,52, k=1,2,3,4. Then, conditional to the parameters θ, we assume that Yik follows a Negative Binomial distribution with mean Aik(θ) and dispersion parameter κ, where Aik(θ) is the prevalence of the infection for week j obtained from solving the SEIRS model in (3.3). Here θ contains all the necessary parameters to solve the SEIRS model including, for example, demographic information such as the population size, birth and death rates, etc.
Furthermore, we assume that the observations Yik are conditionally independent given the parameters, that is equivalent to say that all the dependency in the data is codified into the SEIRS model. This last assumption allows to write the likelihood function as a product of Negative Binomial probability functions, i.e.
π(Y|θ)=4∏i=152∏j=1Γ(yij+1/κ)Γ(yij)Γ(1/κ)(1/κ1/κ+Aij(θ))1/κ(Aij(θ)1/κ+Aij(θ))yij. |
We use the same dispersion parameter for each age group to reduce the number of parameters to estimate.
For many diseases, the parameters involved in the SEIRS model can be fixed based upon previous studies or expert knowledge. However, since ARIs is really a set of diseases, it is unreasonable to estimate a single or unique value for parameters such as recovery rates or infection risk. Therefore, it is appropriate to consider these quantities as random variables. With this idea in mind, the parameters that will then be estimated are the infection probabilities βk, the recovery rates γk, the immunity rates ωk, the amplitude and phase shift of the seasonality function ρ and ϕ, and the dispersion parameter κ of the Negative Binomial distribution. These parameters were chosen because they are considered either the most relevant or the most controversial to be fixed a priori.
Independent informative truncated Gamma priors with shape aγk=4 and scale bγk=0.2 hyper-parameters are used for the recovery rates γk, k=1,2,3,4. We consider a priori that 0.25≤γk≤2, which implies that the mean recovery time 1/γk must be between 0.5 and 4 weeks, which is reasonable for this class of diseases. As the support of γk is bounded, we could simply use a uniform prior distribution. However, this choice would result in a very informative prior for 1/γk. By choosing the hyper-parameters aγk, bγk as above, we provide similar knowledge to both parameters. In fact, the hyper-parameters are used to guarantee that the expected value of the resulting probability distribution coincides with a reference value for the parameter of interest either taken from the literature or deduced from personal communication with experts in the field.
For the parameters ωk a similar prior scheme as the one described above was used. Independent truncated Gamma priors with shape aωk=3 and scale bωk=0.02 were chosen. We assume that the immunity rate must be lower than 0.25, which results in a mean immunity time of at least 4 weeks.
Uniform(0, 1) priors were used for βk and ρ while a Uniform(0,2π) was used for ϕ. Finally, for the dispersion parameter we use a Gamma prior with aκ=2 and scale bκ=0.5. Table 2 summarizes our prior distributions.
Parameter | Expected value | Support | Prior distribution |
γk | 0.8 | [0.25, 2] | Gamma, aγk=4, bγk=0.2 |
ωk | 0.06 | [0.25,∞) | Gamma, aωk=3, bωk=0.02 |
k | 1 | [0,∞) | Gamma, aκ=2, bκ=0.5 |
ρ | 0.5 | [0, 1] | Uniform |
ϕ | 0.5 | [0,2π] | Uniform |
βk | 0.5 | [0, 1] | Uniform |
A list of all the parameters involved in the SEIRS model is shown in Table 3. For those parameters that are fixed, the table shows the values that will be used to model the data described in Section 2. These values are obtained from previous results, official statistical sites and other various sources. More details are given in later sections.
Parameter | Description | Value |
N | Population size | 772604 |
μk | Death rate | 2.7×10−6,1.1×10−7,7.3×10−7,2.9×10−5 |
νk | Birth rate | 6.6×10−4,5.3×10−4 |
αk | Aging rates | 1/(52⋅4),1/(52⋅14),1.0/(52⋅39) |
ωk | Immunity rate | To be estimated |
ηk | Latency rate | 1 |
γk | Recovery rate | To be estimated |
Ckj | Number of contacts between groups | Estimated using POLYMOD, Table 4 |
βk | Probability of infection | To be estimated |
κ | Dispersion parameter | To be estimated |
λk(t) | Force of infection | Defined by (3.1) |
h(t) | Seasonality function | Defined by (3.2) |
ρ | Seasonality amplitude | To be estimated |
ϕ | Seasonality phase shift | To be estimated |
The posterior distribution for the parameters of interest θ=(β1,β2,β3,β4,γ1,γ2,γ3,γ4,ω1,ω2,ω3,ω4,ρ,ϕ,κ) satisfies
π(θ|y)∝π(y|θ)π(θ). |
It does not have an analytical form since the likelihood function depends on the solution of the SEIRS model, which also has no explicit solution and must be approximated numerically. In order to analyze the posterior distribution we use the t-walk, a general purpose Markov Chain Monte Carlo (MCMC) algorithm to sample from continuous probability distributions and does not require tuning [23]. This algorithm generates samples from the posterior distribution that can be used to estimate marginal posterior densities, mean, variance, quantiles, etc. We refer the reader to [24] for more details on MCMC methods.
In this section we fit our SEIRS model to the ARIs data described before. Instead of estimating all the parameters of interest using the data from 2000 to 2008, we analyze one year at a time. This allow us to identify important changes (if any) from one year to the other.
All the analysis is performed in Python 2.7 software. In order to solve the SEIRS model, we use the numerical ordinary differential equations solver odeint available in the scipy package. To explore the posterior distribution we use the implementation of the t-walk available in Python. The algorithm is run for two million iterations and the first 100, 000 are discarded as burn-in. We use 4000 samples to construct estimates of the marginal posterior distributions, probability bands for the evolution of the infection, etc. We ran the algorithm starting from different initial points to be certain that each time the MCMC converges to the same posterior distribution. These initial points are generated at random from the prior distributions.
To initialize the model for the first period (July 2000 to July 2001), we assume that the number of infected Ik(0) for each age group is given by the data records at week 30. This point is close to the lowest point of the infection for that year and we consider that here starts a new infection period. The number of susceptible individuals is set as (Nk−Ik)(0), k=1,2,3,4, where Nk denotes the population size for age group k. This data was obtained from the National Institute of Statistics and Geography (INEGI). The number of exposed Ek(0) and the number of recovered Rk(0) are set to 0. Although this is not ideal, the estimation algorithm corrects this initial approximation error. Once we obtain the estimates for the data of the first period, we use them to initialize the MCMC for the next period.
We fixed birth, death, aging, and latency rates as shown in table 3. Mortality rates for each age group were taken from the study conducted in [7]; this work analyzes the impact of pandemic influenza A(H1N1) on the mortality of a Mexican population, and obtains mortality rates for our age groups, attributed to RSV and FLU. Fertility rates were taken from a study carried out by the Mexican National Council of Population (CONAPO)*. It is considered that the fertile population is between 15 and 49 years old. In group 2 we have a portion of this fertile population (from 15 to 19 years old), which has a rate of 69.5 births per thousand women, per year. The third group refers to the other part of the fertile population, from 20 to 49 years old, which has an average rate of 55.14 births per thousand women, per year. The aging rates are estimated from the number of weeks that individuals remain in their corresponding age group. Finally, the estimates for the number of contacts between age groups can be found in Table 4 and are based on the POLYMOD study on contact data. [25].
* Approximations to the level of fertility in Mexico 1990-2014.
0-4 | 5-19 | 20-59 | ≥ 60 | |
0-4 | 2.21 | 1.13 | 2.86 | 0.53 |
5-19 | 1.13 | 10.12 | 3.87 | 0.71 |
20-59 | 2.86 | 3.87 | 9.69 | 3.08 |
≥ 60 | 0.53 | 0.71 | 3.08 | 2.56 |
In this section we present the analysis of the period 2006-2007. Figure 4 shows the posterior median of the infection with a solid line which represents correctly the tendency of the data. The dashed lines represent 95% pointwise probability bands for the expected value of the infection at each time. There is some lack of fit especially for age group 5-19. As can be seen from the figure, the number of infections substantially decreases at the end of the year, possibly caused by the vacation period as we already have discussed. The model is unable to recover this behavior since it assumes that the number of contacts among groups remains constant.
Figure 5 shows the empirical estimates of the marginal posterior distributions for all the unknown parameters and their corresponding prior distributions. It can be seen that, for most of the parameters, there is a considerable difference between their prior and their posterior distributions. For parameters β2, β3, β4, ρ, ϕ and κ the posterior distribution is clearly unimodal and with low variability. All gamma parameters (recovery rates) and β1 (probability of infection in the group from 0-5 years old) have different posterior distributions with respect to their corresponding priors but there is considerably more variability. It seems that there is not much information in the data for parameters ω3 and ω4 as their posterior distributions are very similar to the priors. Therefore, we could simplify the model by fixing those two parameters. However, this would be difficult since, as we have mentioned before, data corresponds to a group of diseases.
To have a better understanding of the posterior estimates and the role of age in the transmission process, Table 5 shows median posterior estimates and 95% posterior probability intervals for each parameter. It can be seen that the first group is reinfected more frequently since its mean immunity time 1/ω1 is the smallest among all age groups ranging from 4 to almost 10 weeks with a median of 5 weeks. Conversely, young adults have the longest immunity time as expected. In terms of the probability of infection βk, it is clear that children from 0 to 4 years old are the most likely to be infected (βk) and adults from 20 to 59 years old are those with less probability. Also, the recovery time is larger for children, potentially exceeding two weeks. The other three groups recover faster, typically in less than 1 week.
Low | Median | High | |
β1 | 0.25 | 0.66 | 0.97 |
β2 | 0.09 | 0.16 | 0.21 |
β3 | 0.02 | 0.04 | 0.07 |
β4 | 0.08 | 0.16 | 0.22 |
1/γ1 | 0.68 | 0.92 | 2.17 |
1/γ2 | 0.50 | 0.57 | 0.95 |
1/γ3 | 0.50 | 0.64 | 1.12 |
1/γ4 | 0.50 | 0.60 | 1.10 |
1/ω1 | 4.04 | 4.96 | 9.67 |
1/ω2 | 4.60 | 8.92 | 18.42 |
1/ω3 | 5.49 | 18.60 | 80.77 |
1/ω4 | 5.02 | 18.13 | 58.90 |
ρ | 0.07 | 0.10 | 0.16 |
ϕ | 2.72 | 3.28 | 3.99 |
κ | 0.02 | 0.03 | 0.07 |
From the posterior samples we can also estimate the median force of infection for each group, which is shown in Figure 6. It is clear that children less than 4 years have the highest force of infection followed by children between 5 and 19 years old. Also, adults have the lowest values as expected.
Despite the fact that older adults have the second highest probability of infection, they have a low force of infection. It is possible that this phenomenon is associated to their low participation in social activities (see contact matrix C in Table 4) and their high immunity time.
For each period defined in Section 2 we performed the previous analysis. Tables 6 and ?? contains posterior median estimates and 95% probability intervals for all the parameters and all the periods analyzed in this study and can be found as supplementary material. Although numerical results present small changes in magnitude from one year to another, the patterns we observed are the same for all periods.
The objective of this work is to understand the role of age groups in the propagation of ARIs by estimating key parameters related to the dynamics of the infections. In order to achieve this, we propose a SEIRS model and estimate parameters such as the mean recovery time, the mean immunity time and the probability of infection for each group. A Bayesian approach was chosen to perform the inference using ARIs data from San Luis Potosí, Mexico from 2000 to 2008.
For each age group we focused on four characteristics: the probability of infection (βk), the average time that one individual remains infectious (1/γk), the average time that one individual remains immune (1/ωk), and the force of infection (λk(t)), which can be seen as a function of the other parameters. From the estimation process, specifically Table 5 and Figure 6, we saw that children under five years old have the highest probability of infection (with a posterior median of 0.66), the lowest immunity time (around five weeks), the longest recovery time (up to two weeks) and, in general, the largest force of infection. In other words, the children's group (0-4) is more prone to get sick, it remains infectious for longer periods and it is reinfected more rapidly. All of this leads us to hypothesize that children less than 5 years old are the primary spreaders of acute respiratory infections in San Luis Potosí's population from 2000 to 2008.
On the other hand, the group of young adults (20-59) is the one that differs the most from the little children's group since the former has the lowest probability of infection (with a posterior median of 0.04), the longest immunity time (around 18 weeks), a low recovery time (up to one week) and the lowest force of infection. It means that the group of young adults does not get sick often, it remains infectious only a few days and it stays healthy for longer periods. Then, it is reasonable to conclude that the group of young adults is the one that less contributes to the spread of this class of infections. Although we are mentioning a few point estimates to compare the characteristics of these two age groups, the posterior distributions for each parameter support our claims regarding the differences among little children and young adults.
The model fits the data reasonably well, nonetheless, it is not able to predict the decrement of cases reported during the vacations period at the end of each year. A possible explanation for this behavior in the data is that the drop in the reported cases is not due to a biological aspect of the infectious process or due to a change in the circulating viruses and bacteria that cause respiratory diseases, but rather, a matter of decreasing the number of contacts among school age groups. We observed that it is a repetitive pattern each year that only seems to affect individuals that effectively reduce their activities during those three weeks of vacation. Furthermore, there is evidence that by the end of holidays ARIs records increase again. In order to estimate the effect of vacations, we need a more flexible model that could change the number of contacts between groups with time. This can be achieved, for example, by allowing matrix C to change with time or to use a more flexible seasonality function. We leave this as future research.
YNA thanks CONACyT for the PhD scholarship. YNA also thanks to the Kovalevskaia Fund and the Mexican Mathematical Society for partial financial support. MS-C thanks CONACYT for the support provided through the Cátedras CONACYT program to develop this work. JXV-H acknowledges support from UNAM PAPIIT grant IN110917. The authors thank Dr. Daniel Noyola and Dr. Andreu Comas from UASLP for providing access to the San Luis Potosí data. YNA would like to thank Dr. Andreu Comas for his valuable knowledge and feedback on epidemiology.
All authors declare no conflicts of interest in this paper.
2000-2001 | 2001-2002 | 2002-2003 | 2003-2004 | ||||||||||||
Lower | Median | Upper | Lower | Median | Upper | Lower | Median | Upper | Lower | Median | Upper | ||||
β1 | 0.13 | 0.37 | 0.94 | 0.11 | 0.45 | 0.96 | 0.27 | 0.65 | 0.98 | 0.23 | 0.53 | 0.94 | |||
β2 | 0.04 | 0.12 | 0.20 | 0.03 | 0.10 | 0.22 | 0.05 | 0.13 | 0.23 | 0.07 | 0.16 | 0.23 | |||
β3 | 0.01 | 0.03 | 0.07 | 0.02 | 0.05 | 0.09 | 0.01 | 0.03 | 0.07 | 0.01 | 0.04 | 0.07 | |||
β4 | 0.05 | 0.17 | 0.30 | 0.06 | 0.18 | 0.33 | 0.03 | 0.10 | 0.28 | 0.07 | 0.15 | 0.26 | |||
ρ | 0.01 | 0.08 | 0.20 | 0.08 | 0.13 | 0.22 | 0.05 | 0.11 | 0.17 | 0.08 | 0.11 | 0.16 | |||
ϕ | 1.72 | 2.99 | 5.09 | 2.61 | 3.62 | 4.47 | 2.67 | 3.65 | 4.49 | 3.18 | 3.86 | 4.62 | |||
γ1 | 0.27 | 0.68 | 1.42 | 0.29 | 0.72 | 1.31 | 0.33 | 0.91 | 1.39 | 0.40 | 0.88 | 1.40 | |||
γ2 | 0.49 | 1.32 | 1.94 | 0.39 | 1.06 | 1.94 | 0.44 | 1.34 | 1.94 | 0.88 | 1.64 | 1.99 | |||
γ3 | 0.29 | 1.08 | 1.93 | 0.61 | 1.56 | 1.98 | 0.30 | 1.07 | 1.92 | 0.52 | 1.50 | 1.97 | |||
γ4 | 0.50 | 1.32 | 1.98 | 0.53 | 1.26 | 1.94 | 0.30 | 0.89 | 1.90 | 0.63 | 1.31 | 1.93 | |||
ω1 | 0.02 | 0.14 | 0.24 | 0.04 | 0.13 | 0.24 | 0.03 | 0.14 | 0.24 | 0.05 | 0.18 | 0.25 | |||
ω2 | 0.02 | 0.08 | 0.19 | 0.01 | 0.07 | 0.22 | 0.01 | 0.08 | 0.20 | 0.04 | 0.08 | 0.21 | |||
ω3 | 0.00 | 0.03 | 0.16 | 0.01 | 0.03 | 0.16 | 0.01 | 0.03 | 0.20 | 0.01 | 0.04 | 0.21 | |||
ω4 | 0.01 | 0.03 | 0.16 | 0.01 | 0.03 | 0.19 | 0.01 | 0.04 | 0.22 | 0.01 | 0.05 | 0.20 | |||
κ | 0.03 | 0.04 | 0.14 | 0.03 | 0.04 | 0.11 | 0.02 | 0.03 | 0.11 | 0.02 | 0.03 | 0.08 |
2004-2005 | 2005-2006 | 2006-2007 | 2007-2008 | ||||||||||||
Lower | Median | Upper | Lower | Median | Upper | Lower | Median | Upper | Lower | Median | Upper | ||||
β1 | 0.18 | 0.67 | 0.99 | 0.18 | 0.54 | 0.95 | 0.25 | 0.66 | 0.97 | 0.37 | 0.73 | 0.99 | |||
β2 | 0.02 | 0.14 | 0.21 | 0.07 | 0.15 | 0.22 | 0.09 | 0.16 | 0.21 | 0.07 | 0.15 | 0.21 | |||
β3 | 0.02 | 0.05 | 0.07 | 0.01 | 0.05 | 0.07 | 0.02 | 0.04 | 0.07 | 0.01 | 0.05 | 0.07 | |||
β4 | 0.08 | 0.17 | 0.25 | 0.05 | 0.14 | 0.25 | 0.08 | 0.16 | 0.22 | 0.06 | 0.14 | 0.21 | |||
ρ | 0.07 | 0.11 | 0.16 | 0.08 | 0.11 | 0.14 | 0.07 | 0.10 | 0.16 | 0.06 | 0.10 | 0.14 | |||
ϕ | 2.75 | 3.33 | 4.20 | 3.32 | 3.98 | 4.60 | 2.72 | 3.28 | 3.99 | 2.99 | 3.49 | 4.11 | |||
γ1 | 0.38 | 1.01 | 1.48 | 0.37 | 0.96 | 1.48 | 0.46 | 1.09 | 1.47 | 0.61 | 1.06 | 1.45 | |||
γ2 | 0.34 | 1.56 | 1.99 | 0.96 | 1.69 | 1.98 | 1.06 | 1.76 | 1.99 | 0.90 | 1.68 | 1.99 | |||
γ3 | 0.75 | 1.65 | 1.99 | 0.54 | 1.55 | 1.97 | 0.89 | 1.55 | 1.99 | 0.58 | 1.55 | 1.99 | |||
γ4 | 0.84 | 1.53 | 1.97 | 0.54 | 1.29 | 1.97 | 0.91 | 1.68 | 1.99 | 0.65 | 1.53 | 1.97 | |||
ω1 | 0.05 | 0.16 | 0.24 | 0.07 | 0.18 | 0.25 | 0.10 | 0.20 | 0.25 | 0.09 | 0.17 | 0.25 | |||
ω2 | 0.05 | 0.11 | 0.24 | 0.05 | 0.11 | 0.23 | 0.05 | 0.11 | 0.22 | 0.05 | 0.10 | 0.23 | |||
ω3 | 0.01 | 0.04 | 0.21 | 0.02 | 0.05 | 0.19 | 0.01 | 0.05 | 0.18 | 0.02 | 0.07 | 0.21 | |||
ω4 | 0.01 | 0.03 | 0.19 | 0.01 | 0.04 | 0.18 | 0.02 | 0.06 | 0.20 | 0.01 | 0.04 | 0.17 | |||
κ | 0.02 | 0.02 | 0.07 | 0.02 | 0.03 | 0.09 | 0.02 | 0.03 | 0.07 | 0.02 | 0.03 | 0.08 |
[1] | C. Ruiz-Matus, P. Kuri-Morales and J.Narro-Robles, Comportamiento de las temporadas de in-fluenza en México de 2010 a 2016, análisis y prospectiva, Gaceta Médica de México, 153 (2017), 205–213. |
[2] | B. Cowling, G. Freeman, J. Wong, et al., Preliminary inferences on the age-specific seriousness of human disease caused by avian influenza a (H7N9) infections in China, March to April 2013, Eur. Surveil., 18 (2013), 20475. |
[3] | G. Emukule, P. Spreeuwenberg, S. Chaves, et al., Estimating influenza and respiratory syncytial virus-associated mortality in Western Kenya using health and demographic surveillance system data, 2007-2013, PloS One, 12 (2017), e0180890. |
[4] | S. Tempia, S. Walazaa, C. Viboud, et al., Mortality associated with seasonal and pandemic in-fluenza and respiratory syncytial virus among children < 5 years of age in a high HIV prevalence setting in South Africa, 1998–2009, Clin. Infect. Dis., 58 (2014), 1241–1249. |
[5] | L. White, M. Waris, P. Cane, et al., The transmission dynamics of groups a and b human respi-ratory syncytial virus (hRSV) in England & Wales and Finland: seasonality and cross-protection, Epidemiol. Infect., 133 (2005), 279–289. |
[6] | H. Zhou, W. Thompson, C. Viboud, et al., Hospitalizations associated with influenza and respira-tory syncytial virus in the United States, 1993–2008, Clin. Infect. Dis., 54 (2012), 1427–1436. |
[7] | A. Comas-García, C. García-Sepúlveda, J. Méndez-de Lira, et al., Mortality attributable to pan-demic influenza a (H1N1) 2009 in San Luis Potosí, México, Influenza Other Respir. Viruses, 5 (2011), 76–82. |
[8] | CJ. Worby, C. Kenyon, R. Lynfield, et al., Examining the role of different age groups, and of vaccination during the 2012 Minnesota pertussis outbreak, Sci. Rep., 5 (2015), 13182. |
[9] | C. Nj and K. Subbarao, Influenza, Lancet, 354 (1999), 1277–82. |
[10] | S. Towers and Z. Feng, Social contact patterns and control strategies for influenza in the elderly, Math. Biosci., 240 (2012), 241–249. |
[11] | S. Cauchemez, A. Valleron, P. Boelle, et al., Estimating the impact of school closure on influenza transmission from sentinel data, Nature, 452 (2008), 750. |
[12] | J. Tamerius, C. Viboud, J. Shaman, et al., Impact of school cycles and environmental forcing on the timing of pandemic influenza activity in Mexican states, May-December 2009, PLoS Comput. Biol., 11 (2015), e1004337. |
[13] | M. Angulo and J. Velasco-Hernandez, Robust qualitative estimation of time-varying contact rates in uncertain epidemics, Epidemics (2018). Available from: https://doi.org/10.1016/j. epidem.2018.03.001 |
[14] | A. Elkadry, Transmission rate in partial differential equation in epidemic models, Ph.D thesis, Marshall University, 2013. |
[15] | K. Hadeler, Parameter identification in epidemic models, Math. Biosci., 229 (2011), 185–189. |
[16] | A. Lange, Reconstruction of disease transmission rates: applications to measles, dengue, and influenza, J. Theor. Biol., 400 (2016), 138–153. |
[17] | M. Pollicott, H. Wang and H. Weiss, Extracting the time-dependent transmission rate from infec-tion data via solution of an inverse ODE problem, J. Biol. Dyn., 6 (2012), 509–523. |
[18] | R. Anderson and R. May, Infectious diseases of humans: dynamics and control, Oxford University Press, 1992. |
[19] | J. Velasco-Hernández, M. Nuñez-Lopez, A. Comas-García, et al., Superinfection between in-fluenza and RSV alternating patterns in San Luis Potosí State, México, PloS One, 10 (2015), e0115674. |
[20] | R. Schmidt-Ott, M. Schwehm and M. Eichner, Influence of social contact patterns and demo-graphic factors on influenza simulation results, BMC Infect. Dis., 16 (2016), 646. |
[21] | A. Agresti, Categorical data analysis, 2nd edition, John Wiley & Sons, 2002. |
[22] | J. Ponciano and M. Capistrán, First principles modeling of nonlinear incidence rates in sea-sonalepidemics, PLoS Comput. Biol., 7 (2011), e1001079. |
[23] | J. Christen and C. Fox., A general purpose sampling algorithm for continuous distributions (the t-walk), Bayesian Anal., 5 (2010), 263–282. |
[24] | C. Robert and G. Casella, Monte Carlo Statistical Methods, 2nd edition, Springer, New York, 2004. |
[25] | J. Mossong, N. Hens, M. Jit, et al., Social contacts and mixing patterns relevant to the spread of infectious diseases, PLoS Med., 5 (2008), e74. |
1. | Quentin Richard, Samuel Alizon, Marc Choisy, Mircea T. Sofonea, Ramsès Djidjou-Demasse, Gabor Balazsi, Age-structured non-pharmaceutical interventions for optimal control of COVID-19 epidemic, 2021, 17, 1553-7358, e1008776, 10.1371/journal.pcbi.1008776 | |
2. | Kernel Prieto, Jhoana P. Romero–Leiton, Current forecast of HIV/AIDS using Bayesian inference, 2021, 34, 0890-8575, 10.1111/nrm.12332 | |
3. | John C. Lang, Use of mathematical modelling to assess respiratory syncytial virus epidemiology and interventions: a literature review, 2022, 84, 0303-6812, 10.1007/s00285-021-01706-y | |
4. | Kernel Prieto, Simone Lolli, Current forecast of COVID-19 in Mexico: A Bayesian and machine learning approaches, 2022, 17, 1932-6203, e0259958, 10.1371/journal.pone.0259958 | |
5. | EDUARDO IBARGÜEN-MONDRAGÓN, KERNEL PRIETO, SANDRA PATRICIA HIDALGO-BONILLA, A MODEL ON BACTERIAL RESISTANCE CONSIDERING A GENERALIZED LAW OF MASS ACTION FOR PLASMID REPLICATION, 2021, 29, 0218-3390, 375, 10.1142/S0218339021400118 | |
6. | Kernel Prieto, M. Victoria Chávez–Hernández, Jhoana P. Romero–Leiton, Jeffrey Shaman, On mobility trends analysis of COVID–19 dissemination in Mexico City, 2022, 17, 1932-6203, e0263367, 10.1371/journal.pone.0263367 | |
7. | Albert Orwa Akuno, L. Leticia Ramírez-Ramírez, Jesús F. Espinoza, Inference on a Multi-Patch Epidemic Model with Partial Mobility, Residency, and Demography: Case of the 2020 COVID-19 Outbreak in Hermosillo, Mexico, 2023, 25, 1099-4300, 968, 10.3390/e25070968 |
2000-2001 | 2001-2002 | 2002-2003 | 2003-2004 | 2004-2005 | 2005-2006 | 2006-2007 | 2007-2008 | |
5 < | 0.87 | 1.22 | 1.07 | 0.95 | 1.81 | 1.03 | 0.98 | 1.22 |
[0.86, 0.87] | [1.21, 1.23] | [1.07, 1.08] | [0.94, 0.96] | [1.79, 1.83] | [1.02, 1.04] | [0.97, 0.99] | [1.21, 1.24] | |
5–19 | 1.11 | 1.07 | 1.06 | 1.18 | 1.25 | 0.96 | 1.35 | 1.17 |
[1.10, 1.12] | [1.05, 1.08] | [1.05, 1.07] | [1.17, 1.19] | [1.23, 12.6] | [0.95, 0.97] | [1.33, 1.37] | [1.16, 1.19] | |
20–59 | 0.97 | 0.78 | 0.80 | 0.95 | 0.48 | 0.77 | 1.04 | 0.71 |
[0.96, 0.98] | [0.77, 0.78] | [0.79, 0.81] | [0.94, 0.96] | [0.47, 0.49] | [0.76, 0.78] | [1.03, 1.05] | [0.71, 0.72] | |
≥ 60 | 1.78 | 0.76 | 1.16 | 0.83 | 0.50 | 2.39 | 0.37 | 0.78 |
[1.73, 1.83] | [0.74, 0.78] | [1.14, 1.19] | [0.81, 0.85] | [0.48, 0.51] | [2.33, 2.45] | [0.36, 0.38] | [0.76, 0.80] |
Parameter | Expected value | Support | Prior distribution |
γk | 0.8 | [0.25, 2] | Gamma, aγk=4, bγk=0.2 |
ωk | 0.06 | [0.25,∞) | Gamma, aωk=3, bωk=0.02 |
k | 1 | [0,∞) | Gamma, aκ=2, bκ=0.5 |
ρ | 0.5 | [0, 1] | Uniform |
ϕ | 0.5 | [0,2π] | Uniform |
βk | 0.5 | [0, 1] | Uniform |
Parameter | Description | Value |
N | Population size | 772604 |
μk | Death rate | 2.7×10−6,1.1×10−7,7.3×10−7,2.9×10−5 |
νk | Birth rate | 6.6×10−4,5.3×10−4 |
αk | Aging rates | 1/(52⋅4),1/(52⋅14),1.0/(52⋅39) |
ωk | Immunity rate | To be estimated |
ηk | Latency rate | 1 |
γk | Recovery rate | To be estimated |
Ckj | Number of contacts between groups | Estimated using POLYMOD, Table 4 |
βk | Probability of infection | To be estimated |
κ | Dispersion parameter | To be estimated |
λk(t) | Force of infection | Defined by (3.1) |
h(t) | Seasonality function | Defined by (3.2) |
ρ | Seasonality amplitude | To be estimated |
ϕ | Seasonality phase shift | To be estimated |
0-4 | 5-19 | 20-59 | ≥ 60 | |
0-4 | 2.21 | 1.13 | 2.86 | 0.53 |
5-19 | 1.13 | 10.12 | 3.87 | 0.71 |
20-59 | 2.86 | 3.87 | 9.69 | 3.08 |
≥ 60 | 0.53 | 0.71 | 3.08 | 2.56 |
Low | Median | High | |
β1 | 0.25 | 0.66 | 0.97 |
β2 | 0.09 | 0.16 | 0.21 |
β3 | 0.02 | 0.04 | 0.07 |
β4 | 0.08 | 0.16 | 0.22 |
1/γ1 | 0.68 | 0.92 | 2.17 |
1/γ2 | 0.50 | 0.57 | 0.95 |
1/γ3 | 0.50 | 0.64 | 1.12 |
1/γ4 | 0.50 | 0.60 | 1.10 |
1/ω1 | 4.04 | 4.96 | 9.67 |
1/ω2 | 4.60 | 8.92 | 18.42 |
1/ω3 | 5.49 | 18.60 | 80.77 |
1/ω4 | 5.02 | 18.13 | 58.90 |
ρ | 0.07 | 0.10 | 0.16 |
ϕ | 2.72 | 3.28 | 3.99 |
κ | 0.02 | 0.03 | 0.07 |
2000-2001 | 2001-2002 | 2002-2003 | 2003-2004 | ||||||||||||
Lower | Median | Upper | Lower | Median | Upper | Lower | Median | Upper | Lower | Median | Upper | ||||
β1 | 0.13 | 0.37 | 0.94 | 0.11 | 0.45 | 0.96 | 0.27 | 0.65 | 0.98 | 0.23 | 0.53 | 0.94 | |||
β2 | 0.04 | 0.12 | 0.20 | 0.03 | 0.10 | 0.22 | 0.05 | 0.13 | 0.23 | 0.07 | 0.16 | 0.23 | |||
β3 | 0.01 | 0.03 | 0.07 | 0.02 | 0.05 | 0.09 | 0.01 | 0.03 | 0.07 | 0.01 | 0.04 | 0.07 | |||
β4 | 0.05 | 0.17 | 0.30 | 0.06 | 0.18 | 0.33 | 0.03 | 0.10 | 0.28 | 0.07 | 0.15 | 0.26 | |||
ρ | 0.01 | 0.08 | 0.20 | 0.08 | 0.13 | 0.22 | 0.05 | 0.11 | 0.17 | 0.08 | 0.11 | 0.16 | |||
ϕ | 1.72 | 2.99 | 5.09 | 2.61 | 3.62 | 4.47 | 2.67 | 3.65 | 4.49 | 3.18 | 3.86 | 4.62 | |||
γ1 | 0.27 | 0.68 | 1.42 | 0.29 | 0.72 | 1.31 | 0.33 | 0.91 | 1.39 | 0.40 | 0.88 | 1.40 | |||
γ2 | 0.49 | 1.32 | 1.94 | 0.39 | 1.06 | 1.94 | 0.44 | 1.34 | 1.94 | 0.88 | 1.64 | 1.99 | |||
γ3 | 0.29 | 1.08 | 1.93 | 0.61 | 1.56 | 1.98 | 0.30 | 1.07 | 1.92 | 0.52 | 1.50 | 1.97 | |||
γ4 | 0.50 | 1.32 | 1.98 | 0.53 | 1.26 | 1.94 | 0.30 | 0.89 | 1.90 | 0.63 | 1.31 | 1.93 | |||
ω1 | 0.02 | 0.14 | 0.24 | 0.04 | 0.13 | 0.24 | 0.03 | 0.14 | 0.24 | 0.05 | 0.18 | 0.25 | |||
ω2 | 0.02 | 0.08 | 0.19 | 0.01 | 0.07 | 0.22 | 0.01 | 0.08 | 0.20 | 0.04 | 0.08 | 0.21 | |||
ω3 | 0.00 | 0.03 | 0.16 | 0.01 | 0.03 | 0.16 | 0.01 | 0.03 | 0.20 | 0.01 | 0.04 | 0.21 | |||
ω4 | 0.01 | 0.03 | 0.16 | 0.01 | 0.03 | 0.19 | 0.01 | 0.04 | 0.22 | 0.01 | 0.05 | 0.20 | |||
κ | 0.03 | 0.04 | 0.14 | 0.03 | 0.04 | 0.11 | 0.02 | 0.03 | 0.11 | 0.02 | 0.03 | 0.08 |
2004-2005 | 2005-2006 | 2006-2007 | 2007-2008 | ||||||||||||
Lower | Median | Upper | Lower | Median | Upper | Lower | Median | Upper | Lower | Median | Upper | ||||
β1 | 0.18 | 0.67 | 0.99 | 0.18 | 0.54 | 0.95 | 0.25 | 0.66 | 0.97 | 0.37 | 0.73 | 0.99 | |||
β2 | 0.02 | 0.14 | 0.21 | 0.07 | 0.15 | 0.22 | 0.09 | 0.16 | 0.21 | 0.07 | 0.15 | 0.21 | |||
β3 | 0.02 | 0.05 | 0.07 | 0.01 | 0.05 | 0.07 | 0.02 | 0.04 | 0.07 | 0.01 | 0.05 | 0.07 | |||
β4 | 0.08 | 0.17 | 0.25 | 0.05 | 0.14 | 0.25 | 0.08 | 0.16 | 0.22 | 0.06 | 0.14 | 0.21 | |||
ρ | 0.07 | 0.11 | 0.16 | 0.08 | 0.11 | 0.14 | 0.07 | 0.10 | 0.16 | 0.06 | 0.10 | 0.14 | |||
ϕ | 2.75 | 3.33 | 4.20 | 3.32 | 3.98 | 4.60 | 2.72 | 3.28 | 3.99 | 2.99 | 3.49 | 4.11 | |||
γ1 | 0.38 | 1.01 | 1.48 | 0.37 | 0.96 | 1.48 | 0.46 | 1.09 | 1.47 | 0.61 | 1.06 | 1.45 | |||
γ2 | 0.34 | 1.56 | 1.99 | 0.96 | 1.69 | 1.98 | 1.06 | 1.76 | 1.99 | 0.90 | 1.68 | 1.99 | |||
γ3 | 0.75 | 1.65 | 1.99 | 0.54 | 1.55 | 1.97 | 0.89 | 1.55 | 1.99 | 0.58 | 1.55 | 1.99 | |||
γ4 | 0.84 | 1.53 | 1.97 | 0.54 | 1.29 | 1.97 | 0.91 | 1.68 | 1.99 | 0.65 | 1.53 | 1.97 | |||
ω1 | 0.05 | 0.16 | 0.24 | 0.07 | 0.18 | 0.25 | 0.10 | 0.20 | 0.25 | 0.09 | 0.17 | 0.25 | |||
ω2 | 0.05 | 0.11 | 0.24 | 0.05 | 0.11 | 0.23 | 0.05 | 0.11 | 0.22 | 0.05 | 0.10 | 0.23 | |||
ω3 | 0.01 | 0.04 | 0.21 | 0.02 | 0.05 | 0.19 | 0.01 | 0.05 | 0.18 | 0.02 | 0.07 | 0.21 | |||
ω4 | 0.01 | 0.03 | 0.19 | 0.01 | 0.04 | 0.18 | 0.02 | 0.06 | 0.20 | 0.01 | 0.04 | 0.17 | |||
κ | 0.02 | 0.02 | 0.07 | 0.02 | 0.03 | 0.09 | 0.02 | 0.03 | 0.07 | 0.02 | 0.03 | 0.08 |
2000-2001 | 2001-2002 | 2002-2003 | 2003-2004 | 2004-2005 | 2005-2006 | 2006-2007 | 2007-2008 | |
5 < | 0.87 | 1.22 | 1.07 | 0.95 | 1.81 | 1.03 | 0.98 | 1.22 |
[0.86, 0.87] | [1.21, 1.23] | [1.07, 1.08] | [0.94, 0.96] | [1.79, 1.83] | [1.02, 1.04] | [0.97, 0.99] | [1.21, 1.24] | |
5–19 | 1.11 | 1.07 | 1.06 | 1.18 | 1.25 | 0.96 | 1.35 | 1.17 |
[1.10, 1.12] | [1.05, 1.08] | [1.05, 1.07] | [1.17, 1.19] | [1.23, 12.6] | [0.95, 0.97] | [1.33, 1.37] | [1.16, 1.19] | |
20–59 | 0.97 | 0.78 | 0.80 | 0.95 | 0.48 | 0.77 | 1.04 | 0.71 |
[0.96, 0.98] | [0.77, 0.78] | [0.79, 0.81] | [0.94, 0.96] | [0.47, 0.49] | [0.76, 0.78] | [1.03, 1.05] | [0.71, 0.72] | |
≥ 60 | 1.78 | 0.76 | 1.16 | 0.83 | 0.50 | 2.39 | 0.37 | 0.78 |
[1.73, 1.83] | [0.74, 0.78] | [1.14, 1.19] | [0.81, 0.85] | [0.48, 0.51] | [2.33, 2.45] | [0.36, 0.38] | [0.76, 0.80] |
Parameter | Expected value | Support | Prior distribution |
γk | 0.8 | [0.25, 2] | Gamma, aγk=4, bγk=0.2 |
ωk | 0.06 | [0.25,∞) | Gamma, aωk=3, bωk=0.02 |
k | 1 | [0,∞) | Gamma, aκ=2, bκ=0.5 |
ρ | 0.5 | [0, 1] | Uniform |
ϕ | 0.5 | [0,2π] | Uniform |
βk | 0.5 | [0, 1] | Uniform |
Parameter | Description | Value |
N | Population size | 772604 |
μk | Death rate | 2.7×10−6,1.1×10−7,7.3×10−7,2.9×10−5 |
νk | Birth rate | 6.6×10−4,5.3×10−4 |
αk | Aging rates | 1/(52⋅4),1/(52⋅14),1.0/(52⋅39) |
ωk | Immunity rate | To be estimated |
ηk | Latency rate | 1 |
γk | Recovery rate | To be estimated |
Ckj | Number of contacts between groups | Estimated using POLYMOD, Table 4 |
βk | Probability of infection | To be estimated |
κ | Dispersion parameter | To be estimated |
λk(t) | Force of infection | Defined by (3.1) |
h(t) | Seasonality function | Defined by (3.2) |
ρ | Seasonality amplitude | To be estimated |
ϕ | Seasonality phase shift | To be estimated |
0-4 | 5-19 | 20-59 | ≥ 60 | |
0-4 | 2.21 | 1.13 | 2.86 | 0.53 |
5-19 | 1.13 | 10.12 | 3.87 | 0.71 |
20-59 | 2.86 | 3.87 | 9.69 | 3.08 |
≥ 60 | 0.53 | 0.71 | 3.08 | 2.56 |
Low | Median | High | |
β1 | 0.25 | 0.66 | 0.97 |
β2 | 0.09 | 0.16 | 0.21 |
β3 | 0.02 | 0.04 | 0.07 |
β4 | 0.08 | 0.16 | 0.22 |
1/γ1 | 0.68 | 0.92 | 2.17 |
1/γ2 | 0.50 | 0.57 | 0.95 |
1/γ3 | 0.50 | 0.64 | 1.12 |
1/γ4 | 0.50 | 0.60 | 1.10 |
1/ω1 | 4.04 | 4.96 | 9.67 |
1/ω2 | 4.60 | 8.92 | 18.42 |
1/ω3 | 5.49 | 18.60 | 80.77 |
1/ω4 | 5.02 | 18.13 | 58.90 |
ρ | 0.07 | 0.10 | 0.16 |
ϕ | 2.72 | 3.28 | 3.99 |
κ | 0.02 | 0.03 | 0.07 |
2000-2001 | 2001-2002 | 2002-2003 | 2003-2004 | ||||||||||||
Lower | Median | Upper | Lower | Median | Upper | Lower | Median | Upper | Lower | Median | Upper | ||||
β1 | 0.13 | 0.37 | 0.94 | 0.11 | 0.45 | 0.96 | 0.27 | 0.65 | 0.98 | 0.23 | 0.53 | 0.94 | |||
β2 | 0.04 | 0.12 | 0.20 | 0.03 | 0.10 | 0.22 | 0.05 | 0.13 | 0.23 | 0.07 | 0.16 | 0.23 | |||
β3 | 0.01 | 0.03 | 0.07 | 0.02 | 0.05 | 0.09 | 0.01 | 0.03 | 0.07 | 0.01 | 0.04 | 0.07 | |||
β4 | 0.05 | 0.17 | 0.30 | 0.06 | 0.18 | 0.33 | 0.03 | 0.10 | 0.28 | 0.07 | 0.15 | 0.26 | |||
ρ | 0.01 | 0.08 | 0.20 | 0.08 | 0.13 | 0.22 | 0.05 | 0.11 | 0.17 | 0.08 | 0.11 | 0.16 | |||
ϕ | 1.72 | 2.99 | 5.09 | 2.61 | 3.62 | 4.47 | 2.67 | 3.65 | 4.49 | 3.18 | 3.86 | 4.62 | |||
γ1 | 0.27 | 0.68 | 1.42 | 0.29 | 0.72 | 1.31 | 0.33 | 0.91 | 1.39 | 0.40 | 0.88 | 1.40 | |||
γ2 | 0.49 | 1.32 | 1.94 | 0.39 | 1.06 | 1.94 | 0.44 | 1.34 | 1.94 | 0.88 | 1.64 | 1.99 | |||
γ3 | 0.29 | 1.08 | 1.93 | 0.61 | 1.56 | 1.98 | 0.30 | 1.07 | 1.92 | 0.52 | 1.50 | 1.97 | |||
γ4 | 0.50 | 1.32 | 1.98 | 0.53 | 1.26 | 1.94 | 0.30 | 0.89 | 1.90 | 0.63 | 1.31 | 1.93 | |||
ω1 | 0.02 | 0.14 | 0.24 | 0.04 | 0.13 | 0.24 | 0.03 | 0.14 | 0.24 | 0.05 | 0.18 | 0.25 | |||
ω2 | 0.02 | 0.08 | 0.19 | 0.01 | 0.07 | 0.22 | 0.01 | 0.08 | 0.20 | 0.04 | 0.08 | 0.21 | |||
ω3 | 0.00 | 0.03 | 0.16 | 0.01 | 0.03 | 0.16 | 0.01 | 0.03 | 0.20 | 0.01 | 0.04 | 0.21 | |||
ω4 | 0.01 | 0.03 | 0.16 | 0.01 | 0.03 | 0.19 | 0.01 | 0.04 | 0.22 | 0.01 | 0.05 | 0.20 | |||
κ | 0.03 | 0.04 | 0.14 | 0.03 | 0.04 | 0.11 | 0.02 | 0.03 | 0.11 | 0.02 | 0.03 | 0.08 |
2004-2005 | 2005-2006 | 2006-2007 | 2007-2008 | ||||||||||||
Lower | Median | Upper | Lower | Median | Upper | Lower | Median | Upper | Lower | Median | Upper | ||||
β1 | 0.18 | 0.67 | 0.99 | 0.18 | 0.54 | 0.95 | 0.25 | 0.66 | 0.97 | 0.37 | 0.73 | 0.99 | |||
β2 | 0.02 | 0.14 | 0.21 | 0.07 | 0.15 | 0.22 | 0.09 | 0.16 | 0.21 | 0.07 | 0.15 | 0.21 | |||
β3 | 0.02 | 0.05 | 0.07 | 0.01 | 0.05 | 0.07 | 0.02 | 0.04 | 0.07 | 0.01 | 0.05 | 0.07 | |||
β4 | 0.08 | 0.17 | 0.25 | 0.05 | 0.14 | 0.25 | 0.08 | 0.16 | 0.22 | 0.06 | 0.14 | 0.21 | |||
ρ | 0.07 | 0.11 | 0.16 | 0.08 | 0.11 | 0.14 | 0.07 | 0.10 | 0.16 | 0.06 | 0.10 | 0.14 | |||
ϕ | 2.75 | 3.33 | 4.20 | 3.32 | 3.98 | 4.60 | 2.72 | 3.28 | 3.99 | 2.99 | 3.49 | 4.11 | |||
γ1 | 0.38 | 1.01 | 1.48 | 0.37 | 0.96 | 1.48 | 0.46 | 1.09 | 1.47 | 0.61 | 1.06 | 1.45 | |||
γ2 | 0.34 | 1.56 | 1.99 | 0.96 | 1.69 | 1.98 | 1.06 | 1.76 | 1.99 | 0.90 | 1.68 | 1.99 | |||
γ3 | 0.75 | 1.65 | 1.99 | 0.54 | 1.55 | 1.97 | 0.89 | 1.55 | 1.99 | 0.58 | 1.55 | 1.99 | |||
γ4 | 0.84 | 1.53 | 1.97 | 0.54 | 1.29 | 1.97 | 0.91 | 1.68 | 1.99 | 0.65 | 1.53 | 1.97 | |||
ω1 | 0.05 | 0.16 | 0.24 | 0.07 | 0.18 | 0.25 | 0.10 | 0.20 | 0.25 | 0.09 | 0.17 | 0.25 | |||
ω2 | 0.05 | 0.11 | 0.24 | 0.05 | 0.11 | 0.23 | 0.05 | 0.11 | 0.22 | 0.05 | 0.10 | 0.23 | |||
ω3 | 0.01 | 0.04 | 0.21 | 0.02 | 0.05 | 0.19 | 0.01 | 0.05 | 0.18 | 0.02 | 0.07 | 0.21 | |||
ω4 | 0.01 | 0.03 | 0.19 | 0.01 | 0.04 | 0.18 | 0.02 | 0.06 | 0.20 | 0.01 | 0.04 | 0.17 | |||
κ | 0.02 | 0.02 | 0.07 | 0.02 | 0.03 | 0.09 | 0.02 | 0.03 | 0.07 | 0.02 | 0.03 | 0.08 |