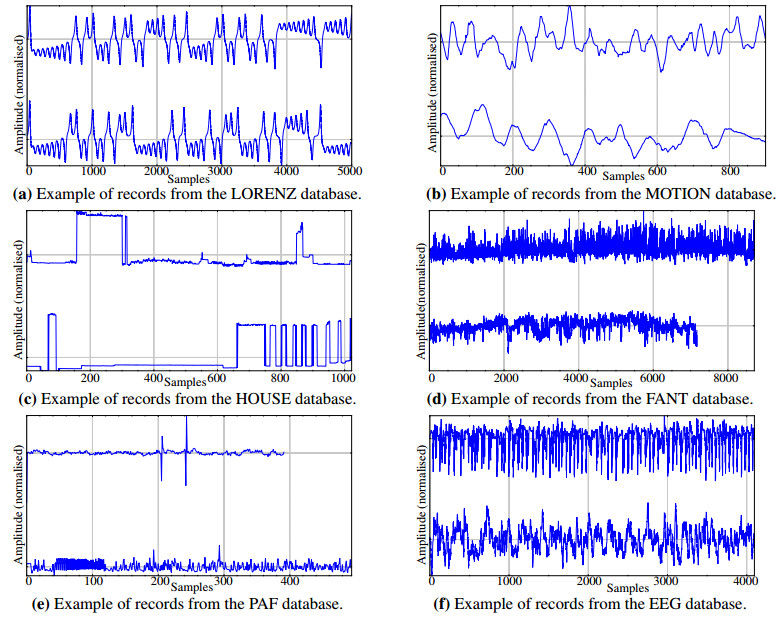
Citation: David Cuesta–Frau. Permutation entropy: Influence of amplitude information on time series classification performance[J]. Mathematical Biosciences and Engineering, 2019, 16(6): 6842-6857. doi: 10.3934/mbe.2019342
[1] | Bei Liu, Wenbin Tan, Xian Zhang, Ziqi Peng, Jing Cao . Recognition study of denatured biological tissues based on multi-scale rescaled range permutation entropy. Mathematical Biosciences and Engineering, 2022, 19(1): 102-114. doi: 10.3934/mbe.2022005 |
[2] | David Cuesta-Frau, Borja Vargas . Permutation Entropy and Bubble Entropy: Possible interactions and synergies between order and sorting relations. Mathematical Biosciences and Engineering, 2020, 17(2): 1637-1658. doi: 10.3934/mbe.2020086 |
[3] | Enas Abdulhay, Maha Alafeef, Hikmat Hadoush, V. Venkataraman, N. Arunkumar . EMD-based analysis of complexity with dissociated EEG amplitude and frequency information: a data-driven robust tool -for Autism diagnosis- compared to multi-scale entropy approach. Mathematical Biosciences and Engineering, 2022, 19(5): 5031-5054. doi: 10.3934/mbe.2022235 |
[4] | Liangyu Yang, Tianyu Shi, Jidong Lv, Yan Liu, Yakang Dai, Ling Zou . A multi-feature fusion decoding study for unilateral upper-limb fine motor imagery. Mathematical Biosciences and Engineering, 2023, 20(2): 2482-2500. doi: 10.3934/mbe.2023116 |
[5] | Erik M. Bollt, Joseph D. Skufca, Stephen J . McGregor . Control entropy: A complexity measure for nonstationary signals. Mathematical Biosciences and Engineering, 2009, 6(1): 1-25. doi: 10.3934/mbe.2009.6.1 |
[6] | Xuyang Xie, Zichun Yang, Lei Zhang, Guoqing Zeng, Xuefeng Wang, Peng Zhang, Guobing Chen . An improved Autogram and MOMEDA method to detect weak compound fault in rolling bearings. Mathematical Biosciences and Engineering, 2022, 19(10): 10424-10444. doi: 10.3934/mbe.2022488 |
[7] | J. Murillo-Escobar, Y. E. Jaramillo-Munera, D. A. Orrego-Metaute, E. Delgado-Trejos, D. Cuesta-Frau . Muscle fatigue analysis during dynamic contractions based on biomechanical features and Permutation Entropy. Mathematical Biosciences and Engineering, 2020, 17(3): 2592-2615. doi: 10.3934/mbe.2020142 |
[8] | Dae Hyeon Kim, Jin-Oh Park, Dae-Young Lee, Young-Seok Choi . Multiscale distribution entropy analysis of short epileptic EEG signals. Mathematical Biosciences and Engineering, 2024, 21(4): 5556-5576. doi: 10.3934/mbe.2024245 |
[9] | Bei Liu, Hongzi Bai, Wei Chen, Huaquan Chen, Zhen Zhang . Automatic detection method of epileptic seizures based on IRCMDE and PSO-SVM. Mathematical Biosciences and Engineering, 2023, 20(5): 9349-9363. doi: 10.3934/mbe.2023410 |
[10] | Fu Tan, Bing Wang, Daijun Wei . A new structural entropy measurement of networks based on the nonextensive statistical mechanics and hub repulsion. Mathematical Biosciences and Engineering, 2021, 18(6): 9253-9263. doi: 10.3934/mbe.2021455 |
Permutation Entropy (PE) [6] is probably becoming one of the most successful complexity estimators in the recent years. The number of works based on this measure is sky-rocketing [38], arguably due its simplicity, robustness, and ability to capture the underlying dynamics of the time series under analysis.
PE has already been applied in a diversity of fields, and other new ones will surely emerge due its versatility. In medicine, PE has been used in practically all its specialities. For example, in cardiology, it has been applied to heart rate variability data series for sleep breathing pause detection [29], to classify emotional changes [33], to assess a possible cardiac autonomic neuropathy [9], or to find out if atrial fibrillation is stochastic or deterministic [3]. Neurology is also a medical field where PE has been extensively exploited. For example, the paper [28] describes a method based on PE to track anaesthetic induced changes using electroencephalograms (EEG). In the same context, other works have used PE to segment sleep stages [27]. PE and the EEG can even be used to detect epileptic seizures [35]. Other medical specialities have not been so widely exploited yet, but there are significant studies already. In internal medicine, PE has been used to classify temperature records as healthy or febrile–prone patients [13], or to analyse blood glucose time series to anticipate the possible development of a diabetes. In physical medicine and rehabilitation, there are many studies based on PE analysis of gait data, as in [37], where authors successfully classified normal and pathological gait using PE. There are other scientific and technical fields that have benefited from the capabilities of PE. In mechanics, PE is being used as a fault diagnosis tool [32,18]. PE is also becoming a popular tool in econometrics applications [42,39,21]. Finally, PE has had its place in time series analysis related to climate data [19].
Since its conception, there are two limitations that are frequently considered when working with PE: the ordinal ambiguity of equal values in subsequences [15,41], and the lack of information related to the sample differences in amplitude [17]. In the first case, consider, for example, two subsequences drawn from a time series, xi={1,3,2} and xj={1,3,3}. If each sample in a subsequence is assigned a value corresponding to its position, starting at 0, xi and xj could be re-written as xi={10,31,22} and xj={10,31,32}, where the sample sub–indices account for the position within the subsequence. If those subsequences are ordered in ascending order, as it is one of the first steps in PE calculation, xi results in yi={10,22,31} (order {0,2,1}), but xj can result both in yj={10,31,32} (order {0,1,2}) or yj={10,32,31} (order {0,2,1}). As a consequence, the ordinal patterns can be incorrectly assigned and PE computation be skewed. Some methods have been proposed in the literature to account for these ambiguities [7,4], and their influence has been heuristically characterised in [15].
As for the second case of differences in amplitude, let's consider again two subsequences and their orders: xi={10,31,22} and xj={10,301,29.92}. If sorted, the resulting order in both cases is {0,2,1}, namely, they provide the same information to PE, despite having very different amplitude values that could be also related to the dynamics of the time series. Other methods have also been proposed recently to address this possible weakness of PE, such as Weighted–Permutation Entropy (WPE) [17], Fine–Grained Permutation Entropy (FGPE) [34], and Amplitude–Aware Permutation Entropy (AAPE) [4], among others. In this case, there is no comparative study to assess the real effectiveness of these approaches, and the practical influence of amplitude differences has not been characterized yet, the goal of the present paper.
This characterization study will specifically test the performance of the following methods: standard PE, WPE, FGPE, and AAPE. The experimental dataset was chosen carefully to ensure a representative variation of time series properties and features. This data set was drawn from publicly available databases for replication and comparison purposes. The experiments will consist on assessing the classification performance of all the methods under analysis, and then compare the classification performance achieved. The results confirmed that a combination of ordinal and amplitude information, the whole, could achieve a higher classification accuracy than each type of information considered independently, its parts, PE (ordinal information) and Sample Entropy (SampEn, amplitude information) [23].
PE was proposed in the well known seminal paper by Bandt and Pompe [6]. This method was devised to estimate the complexity of a time series based on the relative frequency of the ordinal patterns found. PE inputs are a time series x of length N, x={x0,x1,…,xN−1}, and an embedded dimension m>2. All the possible N−(m−1) subsequences, starting at index j, with 0≤j<N−m+1, of length m are then extracted from vector x, termed xmj={xj,xj+1,…,xj+m−1}. Initially, each xmj has a default sample order given by the indices of the samples taken from initial index j, that is, πm={0,1,…,m−1}. When xmj is sorted in ascending order, a new order vector emerges πmj={π0,π1,…,πm−1}, such that xj+π0≤xj+π1≤xj+π2…≤xj+πm−1. There are potentially m! different ordinal patterns of length m, termed Πmi, with 0≤i<m!. All these patterns can be computed in advance, or added dynamically to a list when they are first found. Each time a πmj matches a Πmi, a motif counter ci is incremented, being all these counters members of a vector c of length m!. Finally, the relative frequencies of each possible pattern can be computed and stored in another vector p, whose non–zero members provide the final PE result as:
PE=−m!−1∑k=0pklog2pk,∀pk>0 | (2.1) |
The steps to compute PE are described in detail in Algorithm 1.
![]() |
For example, let x be the sequence {−0.45, 1.9, 0.87, -0.91, 2.3, 1.1, 0.75, 1.3, -1.6, 0.47, -0.15, 0.65, 0.55, -1.1, 0.3} (a random sequence with mean 0 and standard deviation 1.0). In order to compute PE(x,m,N) with N=15, and taking m=3, the following m!=6 ordinal patterns of length 3 have to be considered first: Π30={0,1,2}, Π31={0,2,1}, Π32={1,0,2}, Π33={2,0,1}, Π34={1,2,0}, Π35={2,1,0}.
Then, the N−m+1=13 subsequences that can be drawn from x are x30={−0.45,1.9,0.87}, x31={1.9,0.87,−0.91}, x32={0.87,−0.91,2.3}, x33={−0.91,2.3,1.1}, x34={2.3,1.1,0.75}, x35={1.1,0.75,1.3}, x36={0.75,1.3,−1.6}, x37={1.3,−1.6,0.47}, x38={−1.6,0.47,−0.15}, x39={0.47,−0.15,0.65}, x310={−0.15,0.65,0.55}, x311={0.65,0.55,−1.1}, x312={0.55,−1.1,0.3}. All these subsequences have an associated ordinal pattern π3={0,1,2} initially.
When the previous subsequences are ordered, the results are: y30={−0.45,0.87,1.9}, with π30={0,2,1}; y31={−0.91,0.87,1.9}, with π31={2,1,0}; y32={−0.91,0.87,2.3}, with π32={1,0,2}; y33={−0.91,1.1,2.3}, with π33={0,2,1}; y34={0.75,1.1,2.3}, with π34={2,1,0}; y35={0.75,1.1,1.3}, with π35={1,0,2}; y36={−1.6,0.75,1.3}, with π36={2,0,1}; y37={−1.6,0.47,1.3}, with π37={1,2,0}; y38={−1.6,−0.15,0.47}, with π38={0,2,1}; y39={−0.15,0.47,0.65}, with π39={1,0,2}; y310={−0.15,0.55,0.65}, with π310={0,2,1}; y311={−1.1,0.55,0.65}, with π311={2,1,0} and y312={−1.1,0.3,0.55}, with π312={1,2,0}.
All the π3j ordinal patterns have to be compared with the pattern templates in Π3. Thus, Π30={0,1,2} is not found in π3j. Π31={0,2,1} is found 4 times. Π32={1,0,2} is found 3 times. Π33={2,0,1} is found once. Π34={1,2,0} is found twice, and Π35={2,1,0} appears three times. Therefore, c={0,4,3,1,2,3}, and p={0,0.31,0.23,0.08,0.15,0.23}, from which PE(x,3,15)=−0.31log20.31−0.23log20.23−0.08log20.08−0.15log20.15−0.23log20.23=2.20.
WPE was introduced in [17]. It was devised as an improvement over PE to account for the variability of amplitudes that result in the same motif. For example, given three subsequences x3i={1,2,3}, x3j={1,2,3000}, and x3k={1,2000,2001}, they result in the same ordinal pattern π3ijk={0,1,2}, despite their clear amplitude and even shape differences. The basic idea is to apply a correcting factor or weight to the relative frequencies that takes into account sample variability, not only order. These weights wj are added prior to computing such relative frequencies, and are given by wj=1mm−1∑k=0(xj+k−¯Xmj)2, where ¯Xmj is the arithmetic mean of xmj, and W will be the new denominator instead of N−m−1, with W=N−m∑j=0wj. The steps to compute WPE are described in detail in Algorithm 2.
![]() |
Using the same example as for PE, the weight for each subsequence (the variance of each xmj) would be as follows: w0=0.925, w1=0.227, w2=1.724, w3=1.754, w4=0.441, w5=0.052, w6=1.582, w7=1.487, w8=0.752, w9=0.117, w10=0.127, w11=0.644, w12=0.527, with W=10.359. The ordinal patterns found are the same as for PE, and therefore each weight has to be added to the corresponding relative frequency, p={0,3.55810.359,1.89310.359,1.58210.359,2.01410.359,1.31210.359}, from which WPE(x,m,N)=−0.3435log20.3435−0.1827log20.1827−0.1527log20.1527−0.1944log20.1944−0.1267log20.1267=2.23.
FGPE was first described in [34]. It is another approach to the problem of not considering amplitude information in the standard PE algorithm. This method proposes to include an additional parameter q to quantify the differences dj between consecutive values in each pattern xmj as:
q=⌊max(dj)std(dj)α⌋ |
where α is another user–defined parameter, dj={|xj+1−xj|,…,|xj+m−1−xj+m−2|}, and ⌊⌋ is the floor function, it keeps the integer part of the operand. For simplicity, we used α=1, as in [34]. The resulting value of q is added as an additional symbol at the end of each πmj. As a consequence, Πm is not known in advance, it has to be updated dynamically when a new pattern is found. The algorithm for FGPE is shown in Algorithm 3 (# is the sizeof operator).
![]() |
Probably, the most ambitious method to address PE weaknesses is the Amplitude Aware Permutation Entropy (AAPE) method [4]. This method feeds the amplitude into the PE basic method by quantifying the contribution of the subsequence mean and differences, but it also quantifies the possible effect of ties [15]. In this study, this later contribution will be removed from the AAPE method since it is not related to the amplitude influence, using a simplified version as in [12].
Only an additional parameter is required in this method. This parameter is termed A and lies in the interval [0,1]. The histogram of relative frequencies, instead of being updated by adding 1 each time a match is found, it is updated using a more elaborated expression:
ci=ci+Am|xj|+m−1∑k=1(Am|xj+k|+1−Am−1|xj+k−xj+k−1|) | (2.2) |
where the term m−1∑k=0(Am|xj+k|) accounts for the mean influence, and the term m−1∑k=1(1−Am−1|xj+k−xj+k−1|) for the amplitude differences. The resulting modification of the PE method is shown in Algorithm 4.
![]() |
SampEn is a sample amplitude–based entropy statistic that has been included in the present study for comparative purposes, but it is not the main focus of the assessment. It was first defined in [23], and since then it has become one of the most successful non–linear tool used for time series classification, with an endless list of applications [14,1,10,30,11,31,24].
In this case, xmj is compared with all the other possible subsequences in the time series xmi, with i≠j, in terms of amplitude differences. This difference is given by dji=max(|xj+k−xi+k|),0≤k≤m−1. An additional pre–defined input parameter, r, is the difference threshold beyond which the subsequences are taken as dissimilar.
If the subsequences under comparison are considered similar, a specific counter Bmj(r) is increased. This process is repeated for all xmi, and the final average number of similar subsequences is computed as:
Bm(r)=1N−mN−m−1∑j=0Bmj(r) |
Another counter Am(r) is obtained in the same way making m=m+1 and repeating the previous process. From these two counters, SampEn can finally be calculated:
SampEn(m,r,N)=−log[Am(r)Bm(r)] | (2.3) |
The experimental dataset is composed of 6 representative datasets of real and synthetic time series specially devised for classification purposes. The specific datasets are described next:
● LORENZ. A Lorenz attractor was used to generate synthetic signals for classification. A total of 100 time series were created with random initialisation. One of the sets had the parameter values σ=10,ρ=28, and β=8/3, with a time step of 0.0001. The other dataset had σ=9, being the other parameters equal. These values were chosen just to ensure any classification method would be capable of finding differences between the two resulting classes. This type of time series have been used in a number of non–linear signal processing studies [40,16]. Examples of records in this dataset are shown in Figure 1a.
● MOTION. This database is included in the UEA and UCR public time series classification repository (www.timeseriesclassification.com) [5]. The time series were obtained from the movement of worms [36]. The objective was to compare phenotypes by assessing differences in movement patterns. The system employed to record the movement of the worms is described in detail in [8]. The specific time series included in this study correspond to the 77 records of length 900 in the test subset, first dimension only, with 2 classes, wild–type or one of four mutant types. Examples of these records are shown in Figure 1b.
● HOUSE. This database is also included in the same repository as the MOTION dataset. It contains two classes of 20 records each one. Class 1 is household agammaegate use of electricity, and Class 2 is agammaegate electricity load of tumble dryer and washing machine [26]. The length of the records is 1022 samples. Figure 1c depicts two examples drawn from this database.
● FANT. This dataset was drawn from the Fantasia database, available at [20]. It contains 40 RR–interval records from 20 young and 20 elderly healthy subjects monitored during 120 minutes. The length of the records is not uniform, but greater than 5000 samples in all cases. This database is described in [22]. Examples of records in this database can be found in Figure 1d.
● PAF. This is one of the many databases publicly available at Physionet [20]. The Paroxysmal Atrial Fibrillation (PAF) records of this database are described in detail in [25]. Specifically, only the short duration records of 5 minutes were included in the experiments, with a total of 50 records, 25 with PAF episodes, and 25 without. Examples of records in this database can be found in Figure 1e.
● EEG. This database contains 4097 samples–long electroencephalograph records recorded by the Department of Epileptology, University of Bonn [2]. This database has been used in many classification studies and has been included for comparison purposes. It is publicly available at http://epileptologie-bonn.de. Two classes out of the five originally available are included in the experiments, specifically records that correspond to the 100 seizure–free EEGs of this database from epilepsy patients, and 100 EEGs that do include seizures. Examples of records from the two classes are shown in Figure 1f.
The first experiment was devised to assess the classification performance in terms of accuracy of all the individual methods tested. This accuracy corresponds to the proportion of correctly classified time series (all the experimental datasets contain two classes) over the total number of records in the dataset. These results are shown in Tables 1, 2, 3, 4, 5, for PE–based measures, and m=3,4,6,8,9 respectively, using the datasets described above. Parameters α for FPGE, and A for AAPE were also varied.
PE | WPE | FGPE(α=0.5) | FGPE(α=1.0) | AAPE(A=0.5) | AAPE(A=1.0) | |
LORENZ | 0.571±0.022 | 0.640±0.029 | 0.555±0.019 | 0.577±0.019 | 0.947±0.012 | 0.966±0.006 |
MOTION | 0.651 | 0.646 | 0.546 | 0.569 | 0.602 | 0.602 |
HOUSE | 0.650 | 0.600 | 0.875 | 0.600 | 0.600 | 0.600 |
FANT | 0.650 | 0.600 | 0.700 | 0.775 | 0.700 | 0.625 |
PAF | 0.820 | 0.740 | 0.780 | 0.700 | 0.800 | 0.820 |
EEG | 0.915 | 0.800 | 0.635 | 0.610 | 0.835 | 0.770 |
PE | WPE | FGPE(α=0.5) | FGPE(α=1.0) | AAPE(A=0.5) | AAPE(A=1.0) | |
LORENZ | 0.587±0.038 | 0.877±0.022 | 0.640±0.046 | 0.539±0.019 | 0.915±0,017 | 0.924±0.016 |
MOTION | 0.668 | 0.635 | 0.618 | 0.657 | 0.613 | 0.629 |
HOUSE | 0.650 | 0.575 | 0.700 | 0.600 | 0.600 | 0.600 |
FANT | 0.675 | 0.575 | 0.600 | 0.600 | 0.675 | 0.650 |
PAF | 0.820 | 0.800 | 0.800 | 0.780 | 0.880 | 0.840 |
EEG | 0.910 | 0.650 | 0.595 | 0.545 | 0.835 | 0.820 |
PE | WPE | FGPE(α=0.5) | FGPE(α=1.0) | AAPE(A=0.5) | AAPE(A=1.0) | |
LORENZ | 0.575±0.035 | 0.983±0.005 | 0.664±0.027 | 0.568±0.029 | 0.874±0.038 | 0.888±0.019 |
MOTION | 0.679 | 0.651 | 0.629 | 0.718 | 0.629 | 0.635 |
HOUSE | 0.650 | 0.675 | 0.750 | 0.625 | 0.625 | 0.600 |
FANT | 0.625 | 0.700 | 0.700 | 0.725 | 0.650 | 0.650 |
PAF | 0.820 | 0.740 | 0.700 | 0.700 | 0.840 | 0.820 |
EEG | 0.900 | 0.575 | 0.790 | 0.645 | 0.840 | 0.855 |
PE | WPE | FGPE(α=0.5) | FGPE(α=1.0) | AAPE(A=0.5) | AAPE(A=1.0) | |
LORENZ | 0.558±0.030 | 0.982±0.011 | 0.691±0.037 | 0.622±0.022 | 0.806±0.019 | 0.817±0.030 |
MOTION | 0.685 | 0.640 | 0.624 | 0.553 | 0.635 | 0.635 |
HOUSE | 0.615 | 0.725 | 0.700 | 0.650 | 0.625 | 0.675 |
FANT | 0.600 | 0.825 | 0.575 | 0.575 | 0.625 | 0.575 |
PAF | 0.800 | 0.760 | 0.620 | 0.620 | 0.780 | 0.780 |
EEG | 0.875 | 0.545 | 0.900 | 0.910 | 0.800 | 0.810 |
PE | WPE | FGPE(α=0.5) | FGPE(α=1.0) | AAPE(A=0.5) | AAPE(A=1.0) | |
LORENZ | 0.560±0.028 | 0.979±0.010 | 0.698±0.026 | 0.625±0.047 | 0.777±0.035 | 0.780±0.008 |
MOTION | 0.685 | 0.635 | 0.659 | 0.636 | 0.640 | 0.640 |
HOUSE | 0.675 | 0.775 | 0.700 | 0.650 | 0.625 | 0.600 |
FANT | 0.625 | 0.875 | 0.575 | 0.600 | 0.750 | 0.700 |
PAF | 0.760 | 0.700 | 0.640 | 0.600 | 0.750 | 0.740 |
EEG | 0.865 | 0.560 | 0.900 | 0.915 | 0.770 | 0.770 |
For a better visualisation of the capabilities of each method, Table 6 includes the highest classification accuracy achieved with each method, regardless of the parameter values. In general, the metrics that include amplitude information in their computation outperform the standard PE method.
PE | WPE | FGPE | AAPE | |
LORENZ | 0.587±0.038 | 0.983±0.005 | 0.698±0.026 | 0.966±0.006 |
MOTION | 0.685 | 0.651 | 0.718 | 0.640 |
HOUSE | 0.675 | 0.775 | 0.875 | 0.625 |
FANT | 0.675 | 0.875 | 0.775 | 0.750 |
PAF | 0.820 | 0.800 | 0.800 | 0.880 |
EEG | 0.915 | 0.800 | 0.915 | 0.855 |
Table 7 shows the results obtained using SampEn, with r=0.15,0.20,0.25, and m=1,2,3. For comparison purposes, Table 8 summarizes the best results achieved using all the methods assessed.
r=0.25 | r=0.20 | r=0.15 | |||||||
m=1 | m=2 | m=3 | m=1 | m=2 | m=3 | m=1 | m=2 | m=3 | |
LORENZ | 0.765±0.028 | 0.757±0.031 | 0.642±0.038 | 0.740±0.031 | 0.695±0.025 | 0.640±0.026 | 0.718±0.031 | 0.562±0.035 | 0.752±0.017 |
MOTION | 0.566 | 0.595 | 0.604 | 0.549 | 0.581 | 0.602 | 0.541 | 0.573 | 0.578 |
HOUSE | 0.850 | 0.850 | 0.825 | 0.925 | 0.925 | 0.950 | 0.925 | 0.950 | 0.925 |
FANT | 0.750 | 0.725 | 0.750 | 0.750 | 0.700 | 0.700 | 0.800 | 0.750 | 0.750 |
PAF | 0.660 | 0.680 | 0.600 | 0.640 | 0.640 | 0.540 | 0.660 | 0.540 | 0.640 |
EEG | 0.565 | 0.650 | 0.675 | 0.550 | 0.690 | 0.685 | 0.515 | 0.720 | 0.720 |
PE–Based | SampEn | |
LORENZ | WPE(m=6)=0.983 | SampEn(m=1,r=0.25)=0.765 |
MOTION | FGPE(m=6,α=1)=0.718 | SampEn(m=3,r=0.25)=0.604 |
HOUSE | FPGE(m=3,α=0.5)=0.875 | SampEn(m=3,r=0.20)=0.950 |
FANT | WPE(m=9)=0.875 | SampEn(m=1,r=0.15)=0.800 |
PAF | AAPE(m=4,A=0.5)=0.880 | SampEn(m=2,r=0.25)=0.680 |
EEG | PE(m=3)/FPGE(m=9,α=1)=0.915 | SampEn(m=2,r=0.15)=0.720 |
There is not a clear winner in terms of classification performance, but there is arguably a clear loser, the standard PE method. This method yields in general the lowest performance of all the methods tested. It was only able to provide equal classification results for the EEG database (Table 6). Although PE classification was significant in most cases (except for the LORENZ database), there was always another amplitude–included metric that yielded a higher or at least comparable performance. In any case, there is a great dependence on the input parameters, mainly m.
WPE seems to perform best for high m values. For m=8,9 (Tables 4 and 5), WPE was capable of finding differences in at least 4 datasets (the same in both cases, LORENZ, HOUSE, FANT, and PAF), whereas for lower m values, only 2 or 3 datasets were correctly segmented. However, it is important to note that for m=3, WPE was also able to segment the EEG database.
FGPE probably achieved the overall highest performance, with a classification accuracy above 0.7 in almost all cases (Table 6), the only one. It can be reasonably assumed that this performance could even be improved with a more exhaustive parameter search, mainly for α. However, this performance is more scattered with regard to parameter space, and that entails a more cumbersome algorithm customisation for the problem at hand. In addition, the FPGE algorithm is more complex and slower since the number of motifs is not known in advance.
The AAPE algorithm is similar to that of WPE, and in contrast to FGPE, it is based on weights, not on additional symbols for the ordinal sequences. Of the three amplitude–included methods, this one yields the lowest performance, but it is still slightly better than that of the standard PE method. As for FGPE, other A parameter values would probably improve such performance. In other words, there is room for improvement, whereas PE is already optimized by means of m. SampEn is an entropy metric based solely on amplitude. It was included in the experiments as a reference for the possible influence of amplitude, with no interference from ordinal patterns. The performance described in Table 7 confirmed the suitability of this analysis, since SampEn was also able to classify significantly all the datasets except MOTION. The value of the parameters were in the usually recommended region of r=0.20 and m=2, although as in any multiparameter metric, other values could even yield a higher accuracy.
The best SampEn results were achieved for the HOUSE database classification (0.95 accuracy). This can be due to the fact that these signals resemble a discrete Markov process where differences lie mainly on signal level. In this case, results were very significant regardless of the m or r value employed. This was also the only case in which SampEn outperformed the PE–based metrics. Of all the parameters tested, only for m=3 and r=0.15 was SampEn able to distinguish between classes in a maximum of 4 datasets. In such case, LORENZ, HOUSE, FANT, and EEG classes were detected significantly, with a classification accuracy higher than 0.700 (Table 7).
Other possibility we explored was to use a model that combined more than a single measure. This approach has yielded very good results in other similar works [13]. However, in this case the results achieved using a logistic model with PE and SampEn together, did not improve the results of WPE, FGPE, or AAPE. The models reached the same performance as that achieved by either PE or SampEn, but no synergy was observed. That is why this kind of solution has not been included in the present study.
Obviously, there will surely be datasets where the behaviour and performance of all these metrics will be different from that observed and reported in this paper. However, from the results, it can be stated that the general trend is that amplitude does have an influence on classification performance. If a generic metric is needed, with no time for customisation, WPE is probably the best choice, since it does not need to configure any parameter other than m, as the standard PE, and the algorithm is quite simple. If maximum performance is required, both FPGE and AAPE have the possibility of further customisation by means of an additional parameter, being FPGE in principle more accurate but also more complex. SampEn confirmed the significance of the information carried by the amplitude, but since the performance was not as high as with WPE, FGPE, or AAPE, it becomes apparent that the optimal solution is to combine ordinal and amplitude information, together as in this study, or maybe separately, as in [13].
PE is a very efficient non-linear metric, in the sense of measuring tool, to assess the dynamics of a time series. The algorithm is very simple to implement, robust against noise, and only requires a weak stationarity [6]. However, since the computations are based on ordinal patterns only, the lack of amplitude consideration is often seen as a possible weakness.
In order to address such weakness, some approaches have been proposed in the last years [17,34,4]. These approaches have been reported to improve the results of the standard PE algorithm, but no comparative and general analysis had been developed so far. The study in this a paper used three of these methods: WPE, FGPE, and AAPE, with a varied and diverse experimental dataset and under the same conditions for all the methods, in order to assess the real influence of amplitude variations on PE performance.
When amplitude information was included in the calculations, the classification performance achieved was usually higher than with the standard PE method. Moreover, the three methods that combined ordinal and amplitude information, WPE, FGPE, and AAPE, also outperformed a method based only on amplitude, such as SampEn. Arguably, it can be concluded that a combination of both approaches is the best solution, and amplitude does have a significant influence on PE.
A generic recommendation would be to use WPE instead of PE and expect a better performance, without additional parameters, and with a little additional algorithm complexity. If classification accuracy is key, a proper customisation of FGPE will probably yield the best results, at the cost of a higher computational burden. AAPE is less complex, unless the complete version is used (including ties effect). If only amplitude is considered, AAPE can also yield good results with less algorithm changes than FGPE. The performance when ties are also included, has been studied in another paper [15], and the complexity of the algorithm raises substantially.
The author declares that he has no conflict of interest.
[1] | R. Alcaraz, D. Abásolo, R. Hornero, et al., Study of Sample Entropy ideal computational pa-rameters in the estimation of atrial fibrillation organization from the ECG, in 2010 Computing in Cardiology, 2010, 1027–1030. |
[2] | R. G. Andrzejak, K. Lehnertz, F. Mormann, et al., Indications of nonlinear deterministic and finite-dimensional structures in time series of brain electrical activity: Dependence on recording region and brain state, Phys. Rev. E, 64 (2001), 061907. |
[3] | K. N. Aronis, R. D. Berger, H. Calkins, et al., Is human atrial fibrillation stochastic or determinis-tic? Insights from missing ordinal patterns and causal entropy–complexity plane analysis, Chaos, 28 (2018), 063130. |
[4] | H. Azami and J. Escudero, Amplitude-aware permutation entropy: Illustration in spike detection and signal segmentation, Comput. Meth. Prog. Bio., 128 (2016), 40–51. |
[5] | A. Bagnall, J. Lines, A. Bostrom, et al., The great time series classification bake off: A review and experimental evaluation of recent algorithmic advances, Data Min. Knowl. Disc., 31 (2017), 606–660. |
[6] | C. Bandt and B. Pompe, Permutation entropy: A natural complexity measure for time series, Phys. Rev. Lett., 88 (2002), 174102. |
[7] | C. Bian, C. Qin, Q. D. Y. Ma, et al., Modified Permutation-entropy analysis of heartbeat dynamics, Phys. Rev. E, 85 (2012), 021906. |
[8] | A. E. X. Brown, E. I. Yemini, L. J. Grundy, et al., A dictionary of behavioral motifs reveals clusters of genes affecting caenorhabditis elegans locomotion, Proceed. Nat. Aca. Sci., 110 (2013), 791–796. |
[9] | C. Carricarte-Naranjo, D. J. Cornforth, L. M. Sánchez-Rodríguez, et al., Rényi and permutation entropy analysis for assessment of cardiac autonomic neuropathy, in EMBEC & NBC 2017 (eds. H. Eskola, O. Väisänen, J. Viik and J. Hyttinen), Springer Singapore, Singapore, 2018, 755–758. |
[10] | E. Cirugeda-Roldán, D. Cuesta-Frau, P. Miró-Martínez, et al., A new algorithm for quadratic sample entropy optimization for very short biomedical signals: Application to blood pressure records, Comput. Meth. Prog. Bio., 114 (2014), 231–239. |
[11] | E. Cirugeda-Roldán, D. Novák, V. Kremen, et al., Characterization of complex fractionated atrial electrograms by Sample Entropy: An international multi–center study, Entropy, 17 (2015), 7493–7509. |
[12] | D. Cuesta-Frau, P. Miró-Martínez, S. Oltra-Crespo, et al., Classification of glucose records from patients at diabetes risk using a combined permutation entropy algorithm, Comput. Meth. Prog. Bio., 165 (2018), 197–204. |
[13] | D. Cuesta-Frau, P. Miró-Martínez, S. Oltra-Crespo, et al., Model selection for body temperature signal classification using both amplitude and ordinality-based entropy measures, Entropy, 20. |
[14] | D. Cuesta-Frau, D. Novák, V. Burda, et al., Characterization of artifact influence on the classifi-cation of glucose time series using sample entropy statistics, Entropy, 20. |
[15] | D. Cuesta-Frau, M. Varela-Entrecanales, A. Molina-Picó, et al., Patterns with equal values in permutation entropy: Do they really matter for biosignal classification?, Complexity, 2018 (2018), 1–15. |
[16] | B. Deng, L. Cai, S. Li, et al., Multivariate multi-scale weighted Permutation Entropy analysis of EEG complexity for Alzheimer's disease, Cogn. Neurodynamics, 11 (2017), 217–231. |
[17] | B. Fadlallah, B. Chen, A. Keil, et al., Weighted-permutation entropy: A complexity measure for time series incorporating amplitude information, Phys. Rev. E, 87 (2013), 022911. |
[18] | Y. Gao, F. Villecco, M. Li, et al., Multi-scale permutation entropy based on improved LMD and HMM for rolling bearing diagnosis, Entropy, 19. |
[19] | J. Garland, T. R. Jones, M. Neuder, et al., Anomaly detection in paleoclimate records using permutation entropy, Entropy, 20. |
[20] | A. L. Goldberger, L. A. N. Amaral, L. Glass, et al., PhysioBank, PhysioToolkit, and PhysioNet: Components of a new research resource for complex physiologic signals, Circulation, 101 (2000), 215–220. |
[21] | M. Henry and G. Judge, Permutation entropy and information recovery in nonlinear dynamic economic time series, Econometrics, 7. |
[22] | N. Iyengar, C. K. Peng, R. Morin, et al., Age-related alterations in the fractal scaling of cardiac interbeat interval dynamics, Am. J. Physiol. Integrat. Comparat. Physiol., 271 (1996), R1078–R1084, PMID: 8898003. |
[23] | D. E. Lake, J. S. Richman, M. P. Griffin, et al., Sample entropy analysis of neonatal heart rate variability, Am. J. Physiol. Integrat. Comparat. Physiol., 283 (2002), R789–R797. |
[24] | H. Li, C. Peng and D. Ye, A study of sleep staging based on a sample entropy analysis of electroencephalogram, Bio-Med. Mater. Eng., 26 (2015), S1149–S1156. |
[25] | G. B. Moody, A. L. Goldberger, S. McClennenm, et al., Predicting the onset of Paroxysmal Atrial Fibrillation: The Computers in Cardiology Challenge 2001, Comput. Cardiol., 28 (2011), 113–116. |
[26] | D. Murray, J. Liao, L. Stankovic, et al., A data management platform for personalised real-time energy feedback, in Procededings of the 8th International Conference on Energy Efficiency in Domestic Appliances and Lighting, 2015. |
[27] | N. Nicolaou and J. Georgiou, The use of permutation entropy to characterize sleep electroen-cephalograms, Clin. EEG Neurosci., 42 (2011), 24–28, PMID: 21309439. |
[28] | E. Olofsen, J. Sleigh and A. Dahan, Permutation entropy of the electroencephalogram: A measure of anaesthetic drug effect, Br. J. Anaesth., 101 (2008), 810–821. |
[29] | A. G. Ravelo-García, J. L. Navarro-Mesa, Casanova-Blancas, et al., Application of the permuta-tion entropy over the heart rate variability for the improvement of electrocardiogram-based sleep breathing pause detection, Entropy, 17 (2015), 914–927. |
[30] | J. S. Richman, Sample entropy statistics and testing for order in complex physiological signals, Commun. Stat. Theor. M., 36 (2007), 1005–1019. |
[31] | M. O. Sokunbi, Sample entropy reveals high discriminative power between young and elderly adults in short fMRI data sets, Front. Neuroinform., 8 (2014), 69. |
[32] | X. Wang, S. Si, Y. Wei, et al., The optimized multi-scale permutation entropy and its application in compound fault diagnosis of rotating machinery, Entropy, 21. |
[33] | Y. Xia, L. Yang, L. Zunino, et al., Application of permutation entropy and permutation min-entropy in multiple emotional states analysis of RRI time series, Entropy, 20 (2018), 148. |
[34] | X. F. Liu and Y. Wang, Fine-grained permutation entropy as a measure of natural complexity for time series, Chinese Phys. B, 18 (2009), 2690. |
[35] | Y. Yang, M. Zhou, Y. Niu, et al., Epileptic seizure prediction based on permutation entropy, in Front. Comput. Neurosci., 2018. |
[36] | E. Yemini, T. Jucikas, L. J. Grundy, et al., A database of caenorhabditis elegans behavioral phenotypes, Nat. Meth., 10 (2013), 877–879. |
[37] | M. Zanin, D. Gómez-Andrés, I. Pulido-Valdeolivas, et al., Characterizing normal and pathological gait through permutation entropy, Entropy, 20. |
[38] | M. Zanin, L. Zunino, O. A. Rosso, et al., Permutation entropy and its main biomedical and econophysics applications: A review, Entropy, 14 (2012), 1553–1577. |
[39] | Y. Zhang and P. Shang, Permutation entropy analysis of financial time series based on hills diversity number, Commun. Nonlinear Sci. Numer. Simul., 53 (2017), 288–298. |
[40] | L. Zunino and C. W. Kulp, Detecting nonlinearity in short and noisy time series using the permu-tation entropy, Phys. Lett. A, 381 (2017), 3627–3635. |
[41] | L. Zunino, F. Olivares, F. Scholkmann, et al., Permutation entropy based time series analysis: Equalities in the input signal can lead to false conclusions, Phys. Lett. A, 381 (2017), 1883–1892. |
[42] | L. Zunino, M. Zanin, B. M. Tabak, et al., Forbidden patterns, permutation entropy and stock market inefficiency, Physica A, 388 (2009), 2854–2864. |
1. | David Cuesta-Frau, Jakub Schneider, Eduard Bakštein, Pavel Vostatek, Filip Spaniel, Daniel Novák, Classification of Actigraphy Records from Bipolar Disorder Patients Using Slope Entropy: A Feasibility Study, 2020, 22, 1099-4300, 1243, 10.3390/e22111243 | |
2. | David Cuesta-Frau, Antonio Molina-Picó, Borja Vargas, Paula González, Permutation Entropy: Enhancing Discriminating Power by Using Relative Frequencies Vector of Ordinal Patterns Instead of Their Shannon Entropy, 2019, 21, 1099-4300, 1013, 10.3390/e21101013 | |
3. | David Cuesta-Frau, Slope Entropy: A New Time Series Complexity Estimator Based on Both Symbolic Patterns and Amplitude Information, 2019, 21, 1099-4300, 1167, 10.3390/e21121167 | |
4. | David Cuesta-Frau, Using the Information Provided by Forbidden Ordinal Patterns in Permutation Entropy to Reinforce Time Series Discrimination Capabilities, 2020, 22, 1099-4300, 494, 10.3390/e22050494 | |
5. | Matthew W. Flood, Bernd Grimm, Mashallah Rezakazemi, EntropyHub: An open-source toolkit for entropic time series analysis, 2021, 16, 1932-6203, e0259448, 10.1371/journal.pone.0259448 | |
6. | Fabian Hirsch, Afra Wohlschlaeger, Graph analysis of nonlinear fMRI connectivity dynamics reveals distinct brain network configurations for integrative and segregated information processing, 2022, 108, 0924-090X, 4287, 10.1007/s11071-022-07413-7 | |
7. | Lukáš Nalos, Dagmar Jarkovská, Jitka Švíglerová, Annabell Süß, Jakub Záleský, Daniel Rajdl, Milada Krejčová, Jitka Kuncová, Josef Rosenberg, Milan Štengl, TdP Incidence in Methoxamine-Sensitized Rabbit Model Is Reduced With Age but Not Influenced by Hypercholesterolemia, 2021, 12, 1664-042X, 10.3389/fphys.2021.692921 | |
8. | Ikaro Daniel de Carvalho Barreto, Tatijana Stosic, Rômulo Simões Cezar Menezes, Antonio Samuel Alves da Silva, Osvaldo A. Rosso, Borko Stosic, Hydrological changes caused by the construction of dams and reservoirs: The CECP analysis, 2023, 33, 1054-1500, 023115, 10.1063/5.0135352 | |
9. | Yaoxin Zheng, Shiyan Li, Kang Xing, Xiaojuan Zhang, A Novel Noise Reduction Method of UAV Magnetic Survey Data Based on CEEMDAN, Permutation Entropy, Correlation Coefficient and Wavelet Threshold Denoising, 2021, 23, 1099-4300, 1309, 10.3390/e23101309 | |
10. | Bei Liu, Wenbin Tan, Xian Zhang, Ziqi Peng, Jing Cao, Recognition study of denatured biological tissues based on multi-scale rescaled range permutation entropy, 2022, 19, 1551-0018, 102, 10.3934/mbe.2022005 | |
11. | Mahdy Kouka, David Cuesta-Frau, Slope Entropy Characterisation: The Role of the δ Parameter, 2022, 24, 1099-4300, 1456, 10.3390/e24101456 | |
12. | Andrei Velichko, Hanif Heidari, A Method for Estimating the Entropy of Time Series Using Artificial Neural Networks, 2021, 23, 1099-4300, 1432, 10.3390/e23111432 | |
13. | Huangjing Ni, Zijie Song, Lei Liang, Qiaowen Xing, Jiaolong Qin, Xiaochuan Wu, Decreased Resting-State Functional Complexity in Elderly with Subjective Cognitive Decline, 2021, 23, 1099-4300, 1591, 10.3390/e23121591 | |
14. | Yanwei Zhai, Zheng Lv, Jun Zhao, Wei Wang, Knowledge discovery and variable scale evaluation for long series data, 2022, 0269-2821, 10.1007/s10462-022-10250-0 | |
15. | Chiara Barà, Laura Sparacino, Riccardo Pernice, Yuri Antonacci, Alberto Porta, Dimitris Kugiumtzis, Luca Faes, Comparison of discretization strategies for the model-free information-theoretic assessment of short-term physiological interactions, 2023, 33, 1054-1500, 033127, 10.1063/5.0140641 | |
16. | Mahdy Kouka, David Cuesta-Frau, Vicent Moltó-Gallego, Slope Entropy Characterisation: An Asymmetric Approach to Threshold Parameters Role Analysis, 2024, 26, 1099-4300, 82, 10.3390/e26010082 | |
17. | Peng Guan, Tianrui Zhang, Lianhong Zhou, RUL Prediction of Rolling Bearings Based on Multi-Information Fusion and Autoencoder Modeling, 2024, 12, 2227-9717, 1831, 10.3390/pr12091831 | |
18. | Jinde Zheng, Junfeng Wang, Haiyang Pan, Jinyu Tong, Qingyun Liu, Refined time-shift multiscale slope entropy: a new nonlinear dynamic analysis tool for rotating machinery fault feature extraction, 2024, 112, 0924-090X, 19887, 10.1007/s11071-024-10106-y | |
19. | Ozan Nadirgil, Carbon price prediction using multiple hybrid machine learning models optimized by genetic algorithm, 2023, 342, 03014797, 118061, 10.1016/j.jenvman.2023.118061 | |
20. | Junfeng Wang, Jinde Zheng, Haiyang Pan, Jinyu Tong, Qingyun Liu, Refined composite multiscale slope entropy and its application in rolling bearing fault diagnosis, 2024, 152, 00190578, 371, 10.1016/j.isatra.2024.07.008 | |
21. | Isadora Cardoso-Pereira, João B. Borges, Aline C. Viana, Antonio A. F. Loureiro, Heitor S. Ramos, POPAyI: Muscling Ordinal Patterns for Low-Complex and Usability-Aware Transportation Mode Detection, 2024, 11, 2327-4662, 17170, 10.1109/JIOT.2024.3357632 | |
22. | Massimiliano Zanin, David Papo, Algorithmic Approaches for Assessing Multiscale Irreversibility in Time Series: Review and Comparison, 2025, 27, 1099-4300, 126, 10.3390/e27020126 | |
23. | Xin Xu, Jiangnan Xu, Ruoyu Du, Tingting Xu, Research on Depression Recognition Model and Its Temporal Characteristics Based on Multiscale Entropy of EEG Signals, 2025, 27, 1099-4300, 142, 10.3390/e27020142 | |
24. | Felipe Olivares, Massimiliano Zanin, Quantifying Deviations from Gaussianity with Application to Flight Delay Distributions, 2025, 27, 1099-4300, 354, 10.3390/e27040354 | |
25. | Felipe Olivares, F. Javier Marín-Rodríguez, Kishor Acharya, Massimiliano Zanin, Evaluating Methods for Detrending Time Series Using Ordinal Patterns, with an Application to Air Transport Delays, 2025, 27, 1099-4300, 230, 10.3390/e27030230 |
PE | WPE | FGPE(α=0.5) | FGPE(α=1.0) | AAPE(A=0.5) | AAPE(A=1.0) | |
LORENZ | 0.571±0.022 | 0.640±0.029 | 0.555±0.019 | 0.577±0.019 | 0.947±0.012 | 0.966±0.006 |
MOTION | 0.651 | 0.646 | 0.546 | 0.569 | 0.602 | 0.602 |
HOUSE | 0.650 | 0.600 | 0.875 | 0.600 | 0.600 | 0.600 |
FANT | 0.650 | 0.600 | 0.700 | 0.775 | 0.700 | 0.625 |
PAF | 0.820 | 0.740 | 0.780 | 0.700 | 0.800 | 0.820 |
EEG | 0.915 | 0.800 | 0.635 | 0.610 | 0.835 | 0.770 |
PE | WPE | FGPE(α=0.5) | FGPE(α=1.0) | AAPE(A=0.5) | AAPE(A=1.0) | |
LORENZ | 0.587±0.038 | 0.877±0.022 | 0.640±0.046 | 0.539±0.019 | 0.915±0,017 | 0.924±0.016 |
MOTION | 0.668 | 0.635 | 0.618 | 0.657 | 0.613 | 0.629 |
HOUSE | 0.650 | 0.575 | 0.700 | 0.600 | 0.600 | 0.600 |
FANT | 0.675 | 0.575 | 0.600 | 0.600 | 0.675 | 0.650 |
PAF | 0.820 | 0.800 | 0.800 | 0.780 | 0.880 | 0.840 |
EEG | 0.910 | 0.650 | 0.595 | 0.545 | 0.835 | 0.820 |
PE | WPE | FGPE(α=0.5) | FGPE(α=1.0) | AAPE(A=0.5) | AAPE(A=1.0) | |
LORENZ | 0.575±0.035 | 0.983±0.005 | 0.664±0.027 | 0.568±0.029 | 0.874±0.038 | 0.888±0.019 |
MOTION | 0.679 | 0.651 | 0.629 | 0.718 | 0.629 | 0.635 |
HOUSE | 0.650 | 0.675 | 0.750 | 0.625 | 0.625 | 0.600 |
FANT | 0.625 | 0.700 | 0.700 | 0.725 | 0.650 | 0.650 |
PAF | 0.820 | 0.740 | 0.700 | 0.700 | 0.840 | 0.820 |
EEG | 0.900 | 0.575 | 0.790 | 0.645 | 0.840 | 0.855 |
PE | WPE | FGPE(α=0.5) | FGPE(α=1.0) | AAPE(A=0.5) | AAPE(A=1.0) | |
LORENZ | 0.558±0.030 | 0.982±0.011 | 0.691±0.037 | 0.622±0.022 | 0.806±0.019 | 0.817±0.030 |
MOTION | 0.685 | 0.640 | 0.624 | 0.553 | 0.635 | 0.635 |
HOUSE | 0.615 | 0.725 | 0.700 | 0.650 | 0.625 | 0.675 |
FANT | 0.600 | 0.825 | 0.575 | 0.575 | 0.625 | 0.575 |
PAF | 0.800 | 0.760 | 0.620 | 0.620 | 0.780 | 0.780 |
EEG | 0.875 | 0.545 | 0.900 | 0.910 | 0.800 | 0.810 |
PE | WPE | FGPE(α=0.5) | FGPE(α=1.0) | AAPE(A=0.5) | AAPE(A=1.0) | |
LORENZ | 0.560±0.028 | 0.979±0.010 | 0.698±0.026 | 0.625±0.047 | 0.777±0.035 | 0.780±0.008 |
MOTION | 0.685 | 0.635 | 0.659 | 0.636 | 0.640 | 0.640 |
HOUSE | 0.675 | 0.775 | 0.700 | 0.650 | 0.625 | 0.600 |
FANT | 0.625 | 0.875 | 0.575 | 0.600 | 0.750 | 0.700 |
PAF | 0.760 | 0.700 | 0.640 | 0.600 | 0.750 | 0.740 |
EEG | 0.865 | 0.560 | 0.900 | 0.915 | 0.770 | 0.770 |
PE | WPE | FGPE | AAPE | |
LORENZ | 0.587±0.038 | 0.983±0.005 | 0.698±0.026 | 0.966±0.006 |
MOTION | 0.685 | 0.651 | 0.718 | 0.640 |
HOUSE | 0.675 | 0.775 | 0.875 | 0.625 |
FANT | 0.675 | 0.875 | 0.775 | 0.750 |
PAF | 0.820 | 0.800 | 0.800 | 0.880 |
EEG | 0.915 | 0.800 | 0.915 | 0.855 |
r=0.25 | r=0.20 | r=0.15 | |||||||
m=1 | m=2 | m=3 | m=1 | m=2 | m=3 | m=1 | m=2 | m=3 | |
LORENZ | 0.765±0.028 | 0.757±0.031 | 0.642±0.038 | 0.740±0.031 | 0.695±0.025 | 0.640±0.026 | 0.718±0.031 | 0.562±0.035 | 0.752±0.017 |
MOTION | 0.566 | 0.595 | 0.604 | 0.549 | 0.581 | 0.602 | 0.541 | 0.573 | 0.578 |
HOUSE | 0.850 | 0.850 | 0.825 | 0.925 | 0.925 | 0.950 | 0.925 | 0.950 | 0.925 |
FANT | 0.750 | 0.725 | 0.750 | 0.750 | 0.700 | 0.700 | 0.800 | 0.750 | 0.750 |
PAF | 0.660 | 0.680 | 0.600 | 0.640 | 0.640 | 0.540 | 0.660 | 0.540 | 0.640 |
EEG | 0.565 | 0.650 | 0.675 | 0.550 | 0.690 | 0.685 | 0.515 | 0.720 | 0.720 |
PE–Based | SampEn | |
LORENZ | WPE(m=6)=0.983 | SampEn(m=1,r=0.25)=0.765 |
MOTION | FGPE(m=6,α=1)=0.718 | SampEn(m=3,r=0.25)=0.604 |
HOUSE | FPGE(m=3,α=0.5)=0.875 | SampEn(m=3,r=0.20)=0.950 |
FANT | WPE(m=9)=0.875 | SampEn(m=1,r=0.15)=0.800 |
PAF | AAPE(m=4,A=0.5)=0.880 | SampEn(m=2,r=0.25)=0.680 |
EEG | PE(m=3)/FPGE(m=9,α=1)=0.915 | SampEn(m=2,r=0.15)=0.720 |
PE | WPE | FGPE(α=0.5) | FGPE(α=1.0) | AAPE(A=0.5) | AAPE(A=1.0) | |
LORENZ | 0.571±0.022 | 0.640±0.029 | 0.555±0.019 | 0.577±0.019 | 0.947±0.012 | 0.966±0.006 |
MOTION | 0.651 | 0.646 | 0.546 | 0.569 | 0.602 | 0.602 |
HOUSE | 0.650 | 0.600 | 0.875 | 0.600 | 0.600 | 0.600 |
FANT | 0.650 | 0.600 | 0.700 | 0.775 | 0.700 | 0.625 |
PAF | 0.820 | 0.740 | 0.780 | 0.700 | 0.800 | 0.820 |
EEG | 0.915 | 0.800 | 0.635 | 0.610 | 0.835 | 0.770 |
PE | WPE | FGPE(α=0.5) | FGPE(α=1.0) | AAPE(A=0.5) | AAPE(A=1.0) | |
LORENZ | 0.587±0.038 | 0.877±0.022 | 0.640±0.046 | 0.539±0.019 | 0.915±0,017 | 0.924±0.016 |
MOTION | 0.668 | 0.635 | 0.618 | 0.657 | 0.613 | 0.629 |
HOUSE | 0.650 | 0.575 | 0.700 | 0.600 | 0.600 | 0.600 |
FANT | 0.675 | 0.575 | 0.600 | 0.600 | 0.675 | 0.650 |
PAF | 0.820 | 0.800 | 0.800 | 0.780 | 0.880 | 0.840 |
EEG | 0.910 | 0.650 | 0.595 | 0.545 | 0.835 | 0.820 |
PE | WPE | FGPE(α=0.5) | FGPE(α=1.0) | AAPE(A=0.5) | AAPE(A=1.0) | |
LORENZ | 0.575±0.035 | 0.983±0.005 | 0.664±0.027 | 0.568±0.029 | 0.874±0.038 | 0.888±0.019 |
MOTION | 0.679 | 0.651 | 0.629 | 0.718 | 0.629 | 0.635 |
HOUSE | 0.650 | 0.675 | 0.750 | 0.625 | 0.625 | 0.600 |
FANT | 0.625 | 0.700 | 0.700 | 0.725 | 0.650 | 0.650 |
PAF | 0.820 | 0.740 | 0.700 | 0.700 | 0.840 | 0.820 |
EEG | 0.900 | 0.575 | 0.790 | 0.645 | 0.840 | 0.855 |
PE | WPE | FGPE(α=0.5) | FGPE(α=1.0) | AAPE(A=0.5) | AAPE(A=1.0) | |
LORENZ | 0.558±0.030 | 0.982±0.011 | 0.691±0.037 | 0.622±0.022 | 0.806±0.019 | 0.817±0.030 |
MOTION | 0.685 | 0.640 | 0.624 | 0.553 | 0.635 | 0.635 |
HOUSE | 0.615 | 0.725 | 0.700 | 0.650 | 0.625 | 0.675 |
FANT | 0.600 | 0.825 | 0.575 | 0.575 | 0.625 | 0.575 |
PAF | 0.800 | 0.760 | 0.620 | 0.620 | 0.780 | 0.780 |
EEG | 0.875 | 0.545 | 0.900 | 0.910 | 0.800 | 0.810 |
PE | WPE | FGPE(α=0.5) | FGPE(α=1.0) | AAPE(A=0.5) | AAPE(A=1.0) | |
LORENZ | 0.560±0.028 | 0.979±0.010 | 0.698±0.026 | 0.625±0.047 | 0.777±0.035 | 0.780±0.008 |
MOTION | 0.685 | 0.635 | 0.659 | 0.636 | 0.640 | 0.640 |
HOUSE | 0.675 | 0.775 | 0.700 | 0.650 | 0.625 | 0.600 |
FANT | 0.625 | 0.875 | 0.575 | 0.600 | 0.750 | 0.700 |
PAF | 0.760 | 0.700 | 0.640 | 0.600 | 0.750 | 0.740 |
EEG | 0.865 | 0.560 | 0.900 | 0.915 | 0.770 | 0.770 |
PE | WPE | FGPE | AAPE | |
LORENZ | 0.587±0.038 | 0.983±0.005 | 0.698±0.026 | 0.966±0.006 |
MOTION | 0.685 | 0.651 | 0.718 | 0.640 |
HOUSE | 0.675 | 0.775 | 0.875 | 0.625 |
FANT | 0.675 | 0.875 | 0.775 | 0.750 |
PAF | 0.820 | 0.800 | 0.800 | 0.880 |
EEG | 0.915 | 0.800 | 0.915 | 0.855 |
r=0.25 | r=0.20 | r=0.15 | |||||||
m=1 | m=2 | m=3 | m=1 | m=2 | m=3 | m=1 | m=2 | m=3 | |
LORENZ | 0.765±0.028 | 0.757±0.031 | 0.642±0.038 | 0.740±0.031 | 0.695±0.025 | 0.640±0.026 | 0.718±0.031 | 0.562±0.035 | 0.752±0.017 |
MOTION | 0.566 | 0.595 | 0.604 | 0.549 | 0.581 | 0.602 | 0.541 | 0.573 | 0.578 |
HOUSE | 0.850 | 0.850 | 0.825 | 0.925 | 0.925 | 0.950 | 0.925 | 0.950 | 0.925 |
FANT | 0.750 | 0.725 | 0.750 | 0.750 | 0.700 | 0.700 | 0.800 | 0.750 | 0.750 |
PAF | 0.660 | 0.680 | 0.600 | 0.640 | 0.640 | 0.540 | 0.660 | 0.540 | 0.640 |
EEG | 0.565 | 0.650 | 0.675 | 0.550 | 0.690 | 0.685 | 0.515 | 0.720 | 0.720 |
PE–Based | SampEn | |
LORENZ | WPE(m=6)=0.983 | SampEn(m=1,r=0.25)=0.765 |
MOTION | FGPE(m=6,α=1)=0.718 | SampEn(m=3,r=0.25)=0.604 |
HOUSE | FPGE(m=3,α=0.5)=0.875 | SampEn(m=3,r=0.20)=0.950 |
FANT | WPE(m=9)=0.875 | SampEn(m=1,r=0.15)=0.800 |
PAF | AAPE(m=4,A=0.5)=0.880 | SampEn(m=2,r=0.25)=0.680 |
EEG | PE(m=3)/FPGE(m=9,α=1)=0.915 | SampEn(m=2,r=0.15)=0.720 |