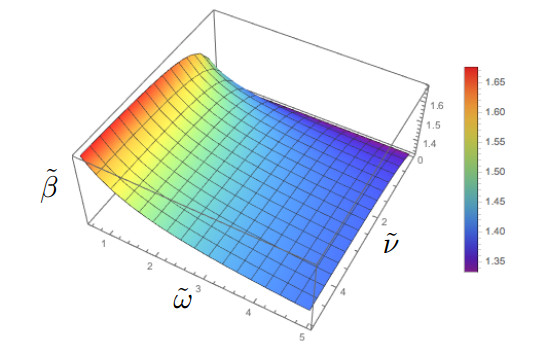
Citation: Kazunori Sato. Basic reproduction number of SEIRS model on regular lattice[J]. Mathematical Biosciences and Engineering, 2019, 16(6): 6708-6727. doi: 10.3934/mbe.2019335
[1] | Jingli Xie, Hongli Guo, Meiyang Zhang . Dynamics of an SEIR model with media coverage mediated nonlinear infectious force. Mathematical Biosciences and Engineering, 2023, 20(8): 14616-14633. doi: 10.3934/mbe.2023654 |
[2] | Jing’an Cui, Ya’nan Zhang, Zhilan Feng, Songbai Guo, Yan Zhang . Influence of asymptomatic infections for the effectiveness of facemasks during pandemic influenza. Mathematical Biosciences and Engineering, 2019, 16(5): 3936-3946. doi: 10.3934/mbe.2019194 |
[3] | Hai-Feng Huo, Qian Yang, Hong Xiang . Dynamics of an edge-based SEIR model for sexually transmitted diseases. Mathematical Biosciences and Engineering, 2020, 17(1): 669-699. doi: 10.3934/mbe.2020035 |
[4] | Abulajiang Aili, Zhidong Teng, Long Zhang . Dynamical behavior of a coupling SEIR epidemic model with transmission in body and vitro, incubation and environmental effects. Mathematical Biosciences and Engineering, 2023, 20(1): 505-533. doi: 10.3934/mbe.2023023 |
[5] | Chayu Yang, Jin Wang . Computation of the basic reproduction numbers for reaction-diffusion epidemic models. Mathematical Biosciences and Engineering, 2023, 20(8): 15201-15218. doi: 10.3934/mbe.2023680 |
[6] | Jianquan Li, Xiaoyu Huo, Yuming Chen . Threshold dynamics of a viral infection model with defectively infected cells. Mathematical Biosciences and Engineering, 2022, 19(7): 6489-6503. doi: 10.3934/mbe.2022305 |
[7] | Wenjuan Guo, Ming Ye, Xining Li, Anke Meyer-Baese, Qimin Zhang . A theta-scheme approximation of basic reproduction number for an age-structured epidemic system in a finite horizon. Mathematical Biosciences and Engineering, 2019, 16(5): 4107-4121. doi: 10.3934/mbe.2019204 |
[8] | Shanjing Ren . Global stability in a tuberculosis model of imperfect treatment with age-dependent latency and relapse. Mathematical Biosciences and Engineering, 2017, 14(5&6): 1337-1360. doi: 10.3934/mbe.2017069 |
[9] | Hamdy M. Youssef, Najat A. Alghamdi, Magdy A. Ezzat, Alaa A. El-Bary, Ahmed M. Shawky . A new dynamical modeling SEIR with global analysis applied to the real data of spreading COVID-19 in Saudi Arabia. Mathematical Biosciences and Engineering, 2020, 17(6): 7018-7044. doi: 10.3934/mbe.2020362 |
[10] | Gergely Röst, Jianhong Wu . SEIR epidemiological model with varying infectivity and infinite delay. Mathematical Biosciences and Engineering, 2008, 5(2): 389-402. doi: 10.3934/mbe.2008.5.389 |
The basic reproduction number, usually expressed by R0, is one of the most important indices of epidemic models. This quantity gives the criterion for determining whether the infectious disease can spread into the whole population or not: when R0>1 an epidemic will result from the introduction of infectious agent, whereas when R0<1 the number of infected is expected to decline (e.g., [1]). Therefore it is known from the threshold principle, that R0=1 gives its threshold.
This equation for theshold also coincides with the condition for the threshold between stability and instability of disease-free equilibrium, but we sometimes obtain different basic reproduction numbers even if the stability conditions for disease-free equilibrium are the same. When this happens, we can determine whether or not the infectious disease can expand, but it produces a possibility for us to make a wrong evaluation of its propagation speed.
In simplest cases, the basic reproduction number can be calculated as the ratio of newly reproduction rate of infectious individuals during the infected period of the focal infected individual. Unfortunately, however, for more complicated models, it requires some other contrivances to obtain the basic reproduction number in general.
In such situations, the most outstanding invention for obtaining R0 is to use next-generation matirix approach [2], in which R0 is defined as the spectral radius or dominant eigenvalue of the next-generation matrix. The computation of R0 by the next-generation matrix is formulated by [3] and [4] (these methods by next-generation matrix are reviewed by [5]). Since then, a tremendous number of studies have adopted this method to obtain R0, and now it has become a standard approach to finding R0. On the other hand, we should notice that we can only find a few examples of obtaining R0 in spatial epidemic models by using the next-generation model. In [6], R0 was first obtained for their infectious model on the lattice by using next-generation matrix.
The rest of this paper is organized as follows. Section 2 reviews the basic reproduction number of SIR model, which gives us the most understandable derivation and result. In Section 3, we verify the procedure for calculating the basic reproduction number by using the next-generation matrix shown in the seminal work [3], and we apply it to SEIRS model on a regular lattice. In Section 4, we compare our result with the previous studies so far.
First, we concentrate on the SIR model proposed by [7] as one of the most fundamental epidemic models. This SIR model can be written as follows:
dρSdt=−βρSρI, | (1) |
dρIdt=βρSρI−σρI, | (2) |
dρRdt=σρI. | (3) |
Here, ρS,ρI, and ρR indicate the fractions of susceptible, infected and recovered individual, respectively. The infection occurs by the right-hand side (RHS) in Eq (1) or the first term of the RHS in Eq (2), which are assumed by the product of both fractions ρS and ρI, i.e., the law of mass action. The recovery from infection randomly occurs by the second term of the RHS in Eq (2) or the RHS in Eq (3). The rates β>0 and σ>0 indicate the unit time of speed of infection and recovery, respectively.
To check the behavior after introduction of a small amount of infectious individuals into susceptible population, or a small disturbance around the disease-free equilibrium (1,0,0), which is an equilibrium of the system (1)–(3), one has to determine whether the disease can invade the population or not. Thus, the linearization of Eq (2) around (1,0,0) becomes
dρIdt=βρI−σρI=(β−σ)ρI |
and defining the ratio of infectious rate during the period of infected:
R0=βσ, | (4) |
then R0>1 if and only if β>σ.
Compared with the above result, limiting transmission of the disease only within the nearest neighboring individuals reduces the value of R0. Therefore, we review the derivation of R0 for SIR model by [8] (the notation in [8] slightly differs from the one presented below, but we can have an exact correspondence). First of all, Eq (2) corresponds to the following equation:
dρIdt=βρSI−σρI=(βqS/I−σ)ρI, | (5) |
where ρSI is the fraction of the nearest-neighboring pair S and I, and qS/I is the conditional probability that the focal site has state S under the condition of state I in the nearest-neighbor site: ρSI=ρIqS/I.
At the initial phase of an infection invading, we can write the rate of I in Eq (5) as
dρIdt|t=0=(βqS/I(0)−σ)ρI. | (6) |
Therefore, the basic reproduction number is defined as
R0=βσqS/I(0) | (7) |
and then if R0>1, then the disease can spread into population on a lattice. So we need to give the initial value of qS/I(0) at the neighborhood around the steady state without infectious individuals.
After the invasion of an infectious individual, the spatial structure with the dynamical steady state would be soon reached, and so it can be used as an initial condition for the variable qS/I to determine whether or not an infection will succeed to invade. Then we consider the dynamics of qS/I:
dqS/Idt=ddtρSIρI=dρSIdt1ρI−dρIdtρSIρ2I=(1−1z)βqS/SqS/I−βzqS/I−(1−1z)βqI/SqS/I−βq2S/I, | (8) |
where we use Eq (5) and the following relation:
dρSIdt=−β{(1−1z)ρISI+1zρSI}−σρSI+β(1−1z)ρSSI≈−β{(1−1z)ρ2SIρS+1zρSI}−σρSI+β(1−1z)ρSSρSIρS |
in which z is the number of nearest neighbors; e.g., z=4 for two-dimensional square lattice and approximated ρISI≈ρ2SI/ρS and ρSSI≈ρSSρSI/ρS come from pair approximation [9] in the second equality (we explain pair approximation in the next section).
Here, setting qS/S=1 and qI/S=0 as a disease-free equilibrium, then Eq (8) becomes
dqS/Idt=βqS/I[(1−2z)−qS/I], | (9) |
and so we obtain the steady state as qS/I=1−2/z. Substituting this as an initial state into Eq (7) results as
R0=(1−2z)βσ, | (10) |
which indicates that the basic reproduction number for SIR model on lattice or network by Eq (10) is less than the one for the corresponding non-spatial model by Eq (4).
Based on the above idea the basic reproduction numbers for basic epidemic models on regular lattice have been obtained by several studies [8,10,11]. In the next section we derive the basic reproduction number for SEIRS model, which is considered as more generalized model than SIR model, by using next-generation matrix introduced by [2] and studied extensively by [3].
We can calculate R0 through the next-generation matrix by [3] as follows.
Consider the vector
x=(x1,⋯,xm,xm+1,⋯,xn)T |
with xi≥0 (i=1,…,n). Here x1,⋯,xm corresponds to m population densities of infected classes. Then disease free state is defined as
Xs={x≥0|xi=0,i=1,…,m}. |
The epidemic model is rewritten as
dxidt=fi(x)=Fi(x)−Vi(x) |
where Vi=V−i−V+i. Fi,V+i,V−i are the terms corresponding to the appearance of new infections in compartment i, transfer of individuals into compartment i by all other means, and transfer of individuals out of compartment i, respectively. Also, these functions Fi,Vi,V−i,V+i satisfy the following conditions (A1)–(A5) with a disease free equilibrium x0:
(A1)x≥0⟹Fi,V+i,V−i≥0 (i=1,…,n).(A2)x=0⟹V−i=0.(A3)Fi=0 (i>m).(A4)x∈Xs⟹Fi=V+i=0 (i=1,…,m).(A5)All eigenvalues of −[∂Vi(x0)/∂xj]i=1,…,nj=1,…,n have negative real parts. |
Then R0 is defined as the spectrum radius or dominant eigenvalue of next-generation matrix FV−1:
R0=ρ(FV−1), |
where
F=[∂Fi∂xj(x0)],V=[∂Vi∂xj(x0)] |
for 1≤i,j≤m.
In the next subsections we use the above procedure to get R0's for SEIRS epidemic model in both cases of completely mixing and only the nearest neighboring infection on regular lattice.
In addition to the system (1)–(3) we use the notation ρE,ω,ν for the fraction of exposed individuals (who are in incubation period during which the individual has been infected but is not yet infectious), the rate of natural loss of immunity, transition rate to infectious, respectively. Then, we consider the following SEIRS model:
dρSdt=−βρSρI+ωρR, | (11) |
dρEdt=βρSρI−νρE, | (12) |
dρIdt=νρE−σρI, | (13) |
dρRdt=σρI−ωρR. | (14) |
Observe that dρS/dt+dρE/dt+dρI/dt+dρR/dt=0 and each variable indicates the fraction of each state, we can assume the total sum of ρS+ρE+ρI+ρR=1. Then we can choose only three variables, ρS,ρE,ρI as independent, and so ρR=1−(ρS+ρE+ρI). Therefore, we get the following system from Eqs (11)–(14):
dρSdt=−βρSρI+ω{1−(ρS+ρE+ρI)}, | (15) |
dρEdt=βρSρI−νρE, | (16) |
dρIdt=νρE−σρI. | (17) |
To express this system in non-dimensional terms, we choose new variables as follows:
˜β=βσ, ˜ν=νσ, ˜ω=ωσ, τ=σt. |
Then the above system (15)–(17) is transformed as
dρSdτ=−˜βρSρI+˜ω{1−(ρS+ρE+ρI)}, | (18) |
dρEdτ=˜βρSρI−˜νρE, | (19) |
dρIdτ=˜νρE−ρI. | (20) |
We consider {ρE,ρI} as population densities of infected class. Hence, when we check conditions (A1)–(A5) for this system (18)–(20), we find three cases of vectors F and V as follows:
F=(0˜βρSρI0) and V=(−˜ω{1−(ρS+ρE+ρI)}+˜βρSρI˜νρE−˜νρE+ρI),F=(00˜νρE) and V=(−˜ω{1−(ρS+ρE+ρI)}+˜βρSρI−˜βρSρI+˜νρEρI),F=(0˜βρSρI˜νρE) and V=(−˜ω{1−(ρS+ρE+ρI)}+˜βρSρI˜νρEρI). |
Also, observe that the disease-free equilibrium is (ρS,ρE,ρI)=(1,0,0); the matrices F and V are given as,
F=(0˜β00) and V=(˜ν0−˜ν1),F=(00˜ν0) and V=(˜ν−˜β01),F=(0˜β˜ν0) and V=(˜ν001), |
respectively. Then
FV−1=(˜β˜β00),FV−1=(001˜β),FV−1=(0˜β10), |
respectively. Therefore, basic reproduction numbers for these matrices become
R0=˜β,R0=˜β,R0=√˜β, | (21) |
respectively. As pointed out by [3], we should choose F and V by appropriate epidemiological interpretation rather than mathematically. The first and the second choices give the same basic reproduction numbers, but both the second and the third include the terms of progression of infectiousness in the vector F. Therefore, we should adopt the first choice which considers only newly infected individuals.
Using the same notatons in the previous sections and others, such as ρSSI, which indicates the fraction of the triplet SSI, the dynamics of SEIRS model on regular lattice is written as follows:
dρSSdτ=−2˜β(1−1z)ρSSI+2˜ωρSR, | (22) |
dρSEdτ=−˜β(1−1z)(ρISE−ρSSI)−˜νρSE+˜ωρER, | (23) |
dρSIdτ=−˜β{(1−1z)ρISI+1zρSI}+˜νρSE−ρSI+˜ωρIR, | (24) |
dρSRdτ=−˜β(1−1z)ρISR+ρSI−˜ω(ρSR−ρRR), | (25) |
dρEEdτ=2˜β(1−1z)ρESI−2˜νρEE, | (26) |
dρEIdτ=˜β{(1−1z)ρISI+1zρSI}+˜ν(ρEE−ρEI)−ρEI, | (27) |
dρERdτ=˜β(1−1z)ρISR−˜νρER+ρEI−˜ωρER, | (28) |
dρIIdτ=2˜νρEI−2ρII, | (29) |
dρIRdτ=ρII−ρIR+˜νρER−˜ωρIR, | (30) |
dρRRdτ=2ρIR−2˜ωρRR, |
where ρσσ′=ρσ′σ holds due to symmetry.
Observe that by using (22)–(30),
dρSdτ=dρSSdτ+dρSEdτ+dρSIdτ+dρSRdτ=−˜βρSI+˜ωρR=−˜βρSI+˜ω{1−(ρS+ρE+ρI)}, | (31) |
dρEdτ=dρSEdτ+dρEEdτ+dρEIdτ+dρERdτ=˜βρSI−˜νρE, | (32) |
dρIdτ=dρSIdτ+dρEIdτ+dρIIdτ+dρIRdτ=˜νρE−ρI, | (33) |
indicates that the system (31)–(33) including spatial structure corresponds to the non-spatial model (18)–(20) in the previous subsection. And so, the system of nine equations (22)–(30) with the following relation gives the dynamics of the nearest-neighboring pairs for spatial model: 1=ρS+ρE+ρI+ρR=(ρSS+ρSE+ρSI+ρSR)+(ρSE+ρEE+ρEI+ρER)+(ρSI+ρEI+ρII+ρIR)+(ρSR+ρER+ρIR+ρRR)=(ρSS+ρEE+ρII+ρRR)+2(ρSE+ρSI+ρSR+ρEI+ρER+ρIR), i.e., ρRR=1−(ρSS+ρEE+ρII)−2(ρSE+ρSI+ρSR+ρEI+ρER+ρIR).
Unfortunately, however, the system (22)–(30) is not closed since triplet densities such as ρSSI may change as time passes, whose dynamics also includes higher-order correlation functions (quartet densities) and these procedures continue forever. Therefore, we adopt an approximation called pair approximation [9] to this system. By pair approximation, conditional probabilities are defined:
qσ/σ′σ″=ρσσ′σ″ρσ′σ″, | (34) |
and the effect of the site with state σ from the site with state σ″ may be considered smaller compared with the site with state σ′ because the former is the next-nearest neighbor, but the latter is the nearest neighbor. Then we can approximate the conditional probability (34) as follows:
qσ/σ′σ″≈qσ/σ′=ρσσ′ρσ′. | (35) |
Using Eqs (34) and (35), we obtain
ρσσ′σ″≈ρσσ′ρσ′σ″ρσ′. | (36) |
Pair approximation (36) with ρS=ρSS+ρSE+ρSI+ρSR transforms Eqs (22)–(30) into the closed system as follows:
dρSSdτ=−2˜β(1−1z)ρSSρSIρSS+ρSE+ρSI+ρSR+2˜ωρSR, | (37) |
dρSEdτ=−˜β(1−1z)(ρSIρSEρSS+ρSE+ρSI+ρSR−ρSSρSIρSS+ρSE+ρSI+ρSR)−˜νρSE+˜ωρER, | (38) |
dρSIdτ=−˜β{(1−1z)ρ2SIρSS+ρSE+ρSI+ρSR+1zρSI}+˜νρSE−ρSI+˜ωρIR, | (39) |
dρSRdτ=−˜β(1−1z)ρSIρSRρSS+ρSE+ρSI+ρSR+ρSI−˜ω[ρSR−{1−(ρSS+ρEE+ρII)−2(ρSE+ρSI+ρSR+ρEI+ρER+ρIR)}], | (40) |
dρEEdτ=2˜β(1−1z)ρSEρSIρSS+ρSE+ρSI+ρSR−2˜νρEE, | (41) |
dρEIdτ=˜β{(1−1z)ρ2SIρSS+ρSE+ρSI+ρSR+1zρSI}+˜ν(ρEE−ρEI)−ρEI, | (42) |
dρERdτ=˜β(1−1z)ρSIρSRρSS+ρSE+ρSI+ρSR−˜νρER+ρEI−˜ωρER, | (43) |
dρIIdτ=2˜νρEI−2ρII, | (44) |
dρIRdτ=ρII−ρIR+˜νρER−˜ωρIR. | (45) |
We consider {ρSE,ρSI,ρEE,ρEI,ρER,ρII,ρIR} as population densities of infected class because these variables include E or I. When we check conditions (A1)–(A5) for the system (37)–(45) with the choice of F from the viewpoint of the reproduction of newly infected individuals, we can determine only one possible set of vectors F and V:
F=(0˜β(1−1z)ρSSρSIρSS+ρSE+ρSI+ρSR002˜β(1−1z)ρSEρSIρSS+ρSE+ρSI+ρSR˜β{(1−1z)ρ2SIρSS+ρSE+ρSI+ρSR+1zρSI}˜β(1−1z)ρSIρSRρSS+ρSE+ρSI+ρSR00) |
and
V=(2˜β(1−1z)ρSSρSIρSS+ρSE+ρSI+ρSR−2˜ωρSR˜β(1−1z)ρSIρSEρSS+ρSE+ρSI+ρSR+˜νρSE−˜ωρER˜β{(1−1z)ρ2SIρSS+ρSE+ρSI+ρSR+1zρSI}−˜νρSE+ρSI−˜ωρIR˜β(1−1z)ρSIρSRρSS+ρSE+ρSI+ρSR−ρSI+˜ω[ρSR−{1−(ρSS+ρEE+ρII)−2(ρSE+ρSI+ρSR+ρEI+ρER+ρIR)}]2˜νρEE−˜ν(ρEE−ρEI)+ρEI˜νρER−ρEI+˜ωρER−2˜νρEI+2ρII−ρII+ρIR−˜νρER+˜ωρIR). |
Observe that the first and the fourth elements correspond to the changes of variables ρSS and ρSR, respectively, and that the disease-free equilibrium is (ρSS,ρSE,ρSI,ρSR,ρEE,ρEI,ρER,ρII,ρIR)=(1,0,0,0,0,0,0,0,0),
F=(0(1−1/z)˜β00000000000000000000˜β/z00000000000000000000000000) |
and
V=(˜ν000−˜ω00−˜ν1+˜β/z0000−˜ω002˜ν000000−˜ν˜ν+1000000−1˜ν+˜ω00000−2˜ν0200000−˜ν−11+˜ω). |
Then, the next-generation matrix is
FV−1=(a11a12a13a14a15a16a1700000000000000a41a42a43a44a45a46a47000000000000000000000) | (46) |
where
1z−1a11=1z−1a12=a41=a42=˜βz+˜β,2z−1a13=1z−1a14=2a43=a44=˜β˜ω{1+(1+˜ν)(˜ν+˜ω)}(z+˜β)(1+˜ν)(1+˜ω)(˜ν+˜ω),1z−1a15=a45=˜β˜ω(1+˜ν+˜ω)(z+˜β)(1+˜ω)(˜ν+˜ω),2z−1a16=1z−1a17=2a46=a47=˜β˜ω(z+˜β)(1+˜ω). |
The characteristic equation of this next-generation matrix (46) becomes
λ6{λ−˜β[˜ν(1+˜ν+˜ω){(z−1)+z˜ω}+z˜ω(1+˜ω)](z+˜β)(1+˜ν)(1+˜ω)(˜ν+˜ω)}=0. |
Therefore the spectral radius of the next-generation matrix, or the basic reproduction number, for SEIRS model is
R0=ρ(FV−1)=˜β[˜ν(1+˜ν+˜ω){(z−1)+z˜ω}+z˜ω(1+˜ω)](z+˜β)(1+˜ν)(1+˜ω)(˜ν+˜ω). | (47) |
When we set z→∞ then we obtain
R0=˜βasz→∞ | (48) |
which corresponds to R0 for the standard SEIRS, SEIR, SIRS, SIR, and SIS models as Eq (4) and (21). Compared with Eq (48) and (47), epidemic breakout for the former only depends on the infectious rate ˜β, on the other hand, the latter depends not only on the infectious rate but also on other parameters, progression to infectiousness ˜ν and rate of the natural loss of immunity ˜ω.
In Figure 1, we show the dependence of these three parameters on the threshold value of R0=1. We can see that critical infectious rate ˜β increases as transition rate to infectious ˜ν but decreases as rate of the natural loss of immunity ˜ω. This is reasonable because the period during infected 1/˜ν is shorter as ˜ν increases, then the strength of infection should be larger to maintain the value of R0. On the other hand, if ˜ω increases, then the number of susceptibles, to some of which the focal infected individual has not yet transmitted disease, increases, and so ˜β can be reduced for the critical R0=1.
In Figure 2, we show R0 against ˜ν and ˜ω when ˜β→∞. If the infectious rate ˜β becomes very large, then the focal infectious individual can immediately transmit the disease to all susceptibles in the nearest neighbor. Therefore the maximum number of R0 should not exceed the number of the nearest neighbor z. Indeed, we can get this extremal value as
˜ν(1+˜ν+˜ω){(z−1)+z˜ω}+z˜ω(1+˜ω)(1+˜ν)(1+˜ω)(˜ν+˜ω) | (49) |
for ˜β→∞. When ˜ν→0 or ˜ω→0, Eq (49) converges to z or z−1, respectively. When ˜ν→∞ or ˜ω→∞, Eq (49) becomes z−11+˜ω or z. Furthermore we can check that Eq (49) is greater than z−1 and less than z by z−Eq (49)=˜ν(1+˜ν+˜ω)/(1+˜ν)(1+˜ω)(˜ν+˜ω)>0 and Eq (49)−(z−1)=˜ω{1+(1+˜ν)(˜ν+˜ω)}/(1+˜ν)(1+˜ω)(˜ν+˜ω)>0. From Figure 2 we can observe that R0 approaches to z=4 or z−1=3 for ˜ν→0 or ˜ω→0, respectively.
We consider simpler epidemic models with reducing variables and parameters.
When R never returns to S and ˜ω becomes zero, then we get SEIR model with pair approximation:
dρSSdτ=−2˜β(1−1z)ρSSρSIρSS+ρSE+ρSI+ρSR,dρSEdτ=−˜β(1−1z)(ρSIρSEρSS+ρSE+ρSI+ρSR−ρSSρSIρSS+ρSE+ρSI+ρSR)−˜νρSE,dρSIdτ=−˜β{(1−1z)ρ2SIρSS+ρSE+ρSI+ρSR+1zρSI}+˜νρSE−ρSI,dρSRdτ=−˜β(1−1z)ρSIρSRρSS+ρSE+ρSI+ρSR+ρSI,dρEEdτ=2˜β(1−1z)ρSEρSIρSS+ρSE+ρSI+ρSR−2˜νρEE,dρEIdτ=˜β{(1−1z)ρ2SIρSS+ρSE+ρSI+ρSR+1zρSI}+˜ν(ρEE−ρEI)−ρEI,dρERdτ=˜β(1−1z)ρSIρSRρSS+ρSE+ρSI+ρSR−˜νρER+ρEI,dρIIdτ=2˜νρEI−2ρII,dρIRdτ=ρII−ρIR+˜νρER. |
By similar calculation as SEIRS model for disease-free equilibrium, we obtain the next-generation matrix and its characteristic equation as
FV−1=((z−1)˜βz+˜β(z−1)˜βz+˜β0000000000000000000˜βz+˜β˜βz+˜β00000000000000000000000000) |
and
λ6{λ−(z−1)˜βz+˜β}=0. |
Therefore the basic reproduction number for SEIR model is
R0=(z−1)˜βz+˜β. | (50) |
When the exposed period can be neglected and we don't need consider the state E, then we get SIRS model with pair approximation:
dρSSdτ=−2˜β(1−1z)ρSSρSIρSS+ρSI+ρSR+2˜ωρSR,dρSIdτ=−˜β{(1−1z)(ρ2SIρSS+ρSI+ρSR−ρSSρSIρSS+ρSI+ρSR)+1zρSI}−ρSI+˜ωρIR,dρSRdτ=−˜β(1−1z)ρSIρSRρSS+ρSI+ρSR+ρSI−˜ω[ρSR−{1−(ρSS+ρII)−2(ρSI+ρSR+ρIR)}],dρIIdτ=2˜β{(1−1z)ρ2SIρSS+ρSI+ρSR+1zρSI}−2ρII,dρIRdτ=˜β(1−1z)ρSIρSRρSS+ρSI+ρSR+ρII−ρIR−˜ωρIR. |
We can consider {ρSI,ρII,ρIR} as population densities of infected class, and noticing that the first and the third elements correspond to the changes of variables ρSS and ρSR, respectively, then the next-generation matrix and its characteristic equation become
FV−1=((z−1)˜βz+˜β(z−1)˜β˜ω2(z+˜β)(1+˜ω)(z−1)˜β˜ω(z+˜β)(1+˜ω)2˜βz+˜β˜β˜ω(z+˜β)(1+˜ω)2˜β˜ω(z+˜β)(1+˜ω)000) |
and
λ2{λ−˜β[(z−1)+z˜ω](z+˜β)(1+˜ω)}=0. |
Therefore, the basic reproduction number for SIRS model is given as
R0=˜β[(z−1)+z˜ω](z+˜β)(1+˜ω). | (51) |
Next, we move to SIR model without the natural loss of immunity from R to S. SIR model with pair approximation is given as follows:
dρSSdτ=−2˜β(1−1z)ρSSρSIρSS+ρSI+ρSR,dρSIdτ=−˜β{(1−1z)(ρ2SIρSS+ρSI+ρSR−ρSSρSIρSS+ρSI+ρSR)+1zρSI}−ρSI,dρSRdτ=−˜β(1−1z)ρSIρSRρSS+ρSI+ρSR+ρSI,dρIIdτ=2˜β{(1−1z)ρ2SIρSS+ρSI+ρSR+1zρSI}−2ρII,dρIRdτ=˜β(1−1z)ρSIρSRρSS+ρSI+ρSR+ρII−ρIR. | (52) |
By similar calculation as SIRS model for disease-free equilibrium, we obtain the next-generation matrix and its characteristic equation as
FV−1=((z−1)˜βz+˜β002˜βz+˜β00000) |
and
λ2{λ−(z−1)˜βz+˜β}=0. |
Therefore, the basic reproduction number for SIR model is given by
R0=(z−1)˜βz+˜β. | (53) |
The last model SIS with pair approximation is given as follows:
dρSIdτ=−˜β{(1−1z)(ρ2SI1−ρSI−ρII−(1−2ρSI−ρII)ρSI1−ρSI−ρII)+1zρSI}−ρSI+ρIIdρIIdτ=˜β{(1−1z)ρ2SI1−ρSI−ρII+1zρSI}−ρII. |
We can consider all the variables {ρSI,ρII} as population densities of infected class, then the next-generation matrix and its characteristic equation become
FV−1=((z−1)˜βz+˜β(z−1)˜βz+˜β˜βz+˜β˜βz+˜β) |
and
λ(λ−z˜βz+˜β)=0. |
Therefore, the basic reproduction number for SIS model is
R0=z˜βz+˜β. | (54) |
Here, we consider the relationships of R0 among various models. If there is no natural loss of immunity, we set the model as ˜ω→0 for SEIRS model. By taking ˜ω→0 for Eq (47) R0 for SEIR model becomes
˜β[˜ν(1+˜ν+˜ω){(z−1)+z˜ω}+z˜ω(1+˜ω)](z+˜β)(1+˜ν)(1+˜ω)(˜ν+˜ω)˜ω→0⟶(z−1)˜βz+˜β, |
which coincides with Eq (50).
If the transition from E to I occurs instantaneously, i.e., the transition rate ˜ν is very large, then the model can be interpreted as SIRS model. By taking the limit ˜ν→∞ for Eq (47) R0 for SIRS model becomes
˜β[ν(1+˜ν+˜ω){(z−1)+z˜ω}+z˜ω(1+˜ω)](z+˜β)(1+˜ν)(1+˜ω)(˜ν+˜ω)˜ν→∞⟶˜β[(z−1)+z˜ω](z+˜β)(1+˜ω), |
which coincides with Eq (51).
SIR model can be obtained by taking the limit of ˜ω→0 for SIRS model (51). Therefore, R0 for SIR model is
˜β[(z−1)+z˜ω](z+˜β)(1+˜ω)˜ω→0⟶(z−1)˜βz+˜β, |
which coincides with Eq (53).
SIS model can be obtained by taking the limit of ˜ω→∞ for SIRS model (51) by regarding the transition from R to S as being instantaneous. So R0 for SIS model is obtained as
˜β[(z−1)+z˜ω](z+˜β)(1+˜ω)˜ω→∞⟶z˜βz+˜β, |
which coincides with Eq (54).
In this paper, we adopted an approximation to evaluate R0, so in order to check its accuracy, we would compare the result with the Monte Carlo simulation. To do this, we need an equation for some variable corresponding to R0, which increases for R0>1 but decreases for R0<1. Fortunately, we can find the variable for the SIR model, and so we explain it below.
SIR model gives the linearized equation of Eq (52) for ρSI around disease free equilibrium gives
dρSIdτ=[(1−1z)˜β−(1+1z˜β)]ρSI. | (55) |
The ratio of positive term to negative one (1−1/z)˜β/(1+˜β/z)=(z−1)˜β/(z+˜β) coincides with R0 by Eq (53).
We can expect the newly produced number of S-I pairs as this quantity in Monte Carlo simulation. Let us consider one infectious individual to invade into a susceptible population on two-dimensional square lattice space z=4 (Figure 3).
There are four S-I pairs, then we trace how many S-I pairs are newly produced by the direct disease transmission of this infectious to the neighboring susceptibles before changing I to R.
(ⅰ) If the transition from I to R occurs, its probability is 1/(1+˜β) and no newly S-I pairs are produced.
(ⅱ) If the transition from I to R does not occur and one of four Ss is infected, its probability is 1−1/(1+˜β). Then if the transition from I to R occurs, the probability becomes {1−1/(1+˜β)}⋅1/(1+34˜β) and three S-I pairs are produced.
(ⅲ) If the transition from I to R does not occur and one of the four Ss is infected. And then if the transition from I to R does not occur and one of the three Ss is infected. Then if the transition from I to R occurs, the probability becomes {1−1/(1+˜β)}⋅{1−1/(1+34˜β)}⋅1/(1+24˜β). 2×3 S-I pairs are produced.
(ⅳ) If the transition from I to R does not occur and one of the four Ss is infected. And if the transition from I to R does not occur and one of the three Ss is infected. And if the transition from I to R does not occur and one of two Ss is infected. Then if the transition from I to R occurs, the probability becomes {1−1/(1+˜β)}⋅{1−1/(1+34˜β)}⋅{1−1/(1+24˜β)}⋅1/(1+14˜β). 3×3 S-I pairs are produced.
(ⅴ) At the remaining probability {1−1/(1+˜β)}⋅{1−1/(1+34˜β)}⋅{1−1/(1+24˜β)}⋅{1−1/(1+14˜β)}, 4×3 S-I pairs are produced.
The total sum of (ⅰ)–(ⅴ) over 4 (averaged for one S-I pair) gives 3˜β/(4+˜β), which is the case of z=4 in Eq (53).
In the real simulation space there are more sites around these five sites and tertiary infections that can occur in these four Ss by the transmission from going around of secondary infections along the loop. So, the number of newly produced S-I pairs are expected to be somehow small. The results are shown in Figure 4.
Unfortunately we cannot succeed in finding a way of evaluating R0 by Monte Carlo simulation for other models.
We obtained the basic reproduction number for SEIRS model on the regular lattice as Eq (47) by using the next-generation matrix. Inaba [12] stated R0 is determined uniquely, but from the viewpoint of [13], basic reproduction number by one method may not always agree with the one by another. Here we compare it to the results of other previous studies.
Ringa and Bauch [11] gives a basic reproduction number for SEIRS model on the lattice as
R0=˜β(z−1)2z[(z−1)+˜β˜ν], | (56) |
in which [11] uses the different notation n instead of z on page 158. It has much contrast to our Eq (47), which is dependent on ˜ω but Eq (56) does not include it. In addition, observe that the effects of ˜ν are completely opposite between them. Therefore, they have no coincidence on the critical condition for disease outbreak. Ringa and Bauch [11] adopted several assumptions to derive R0. We cannot specify which assumption most crucially causes the differences, but it shows the need to pay attention to use additional kind of approximations.
Keeling [8] studied the SIR model on a network including regular lattice, and obtained the basic reproduction number as
R0=(1−2z)˜β, | (57) |
as shown in Section 2. Equation (57) says R0=0 for z=2 and then someone interprets it as disease cannot invade into the susceptible population for any infectious rate on one-dimensional linear lattice space. However, it is natural to consider that R0 is always positive and the threshold condition to invade into the susceptible population is R0=1. Equation (53) gives 0<R0=˜β/(2+˜β)<1 for z=2, then the basic reproduction number is positive but less than the threshold one, so infectious disease fails to invade on one dimensional lattice space.
When we carefully check the derivation of Eq (10) from Eqs (8) and (9), Eq (6) can be read as
dρIdτ|t=0=(˜βqS/I(0)−1)ρI={(1−1z)˜β−1z˜β−1}ρI. |
It indicates that
R0=(1−1z)˜β1z˜β+1, | (58) |
which coincides with Eq (53). Hence, there is no difference in the critical infectious rate for outbreak between Eqs (53) and (57). However, the former is bounded by z−1, where z is the number of nearest neighboring individuals, but the latter is unbounded when the infectious rate ˜β goes to infinity.
Kiss et al. [13] distinguished two kinds of basic reproductive ratio as R0, an average number of transmissions an individual causes, and ˉR0, growth-based basic reproduction ratio. They exemplified ˉR0 by mean-field approximation for both SIS and SIR models, i.e.,
ˉRMF0=˜β, |
and by pairwise approximation ( = pair approximation) for SIS
ˉRPW0=12[(1−2z)˜β−1+√{(1−2z)˜β−3}2+8{(1−1z)˜β−1}] | (59) |
and for SIR
ˉRPW0=(1−2z)˜β. | (60) |
For SIR they also give
R0=(1−1z)˜β1z˜β+1, |
which coincides with Eq (58) because transmission probability before recovery is (˜β/z)/{(˜β/z)+1} and a newly infected individual has z−1 susceptible neighbors. However, using Eq (55), we can interpret transition rate of S-I pair to another as 1z˜β+1 instead of 1, then growth-based basic reproduction ratio also becomes Eq (58) but not Eq (60). Therefore, there seems no difference between the two basic reproduction numbers by [13] for the SIR model. Here, we use z instead of their n.
Bauch [10] concentrates on SIS lattice model and gives the basic reproduction number as
R0=˜β(z−2)2z−12+12√(˜β(z−2)z+1)2+8˜βz | (61) |
in Eq (50) in [10], in which the author uses Q and β/ν instead of z and ˜β, respectively. Equation (61) is the same as Eq (59) by [13]. Observe that Eq (54) and (61) have the same critical infectious rate for an outbreak. Bauch [10] claimed that the basic reproduction number obtained by ordinary pair approximation as in Eq (61) is unbounded when ˜β goes to infinity. And then Bauch [10] proposed another new approximation called "invasory pair approximation" and succeeded in obtaining a bounded R0 for infinite large infectious rate. Alternatively we show another form of R0 by ordinary pair approximation using the next-generation matrix, which satisfies boundedness for infinite large infectious rate. Invasory pair approximation, in turn, gives the value −z−1+z2+√z4+2z3−5z2+2z+12(z−1) for ˜β→∞, which is easily seen to be greater than an integer z≥2. On the other hand, the basic reproduction number (54) is an increasing function of ˜β and gives z when ˜β goes to infinity. It seems reasonable that the possible number of newly infected individuals is less than or equal to z, so ordinary pair approximation is considered to give rather a good estimation than invasory pair approximation in this meaning.
In the end, we should refer to the seminal work [14]. Trapman [14] gives a formal definition of the asymptotic reproduction number and its alternative to be applicable by pair approximation. Trapman [14] shows an example of SIR model on a regular network whose reproduction number can be calculated exactly and can compare several R0's including [8] and correspondence to Eq (53), which is heuristically derived in [14]. This approach, i.e., comparison to an exact solution, clearly shows what a better solution or an approximation is. So, we would like to propose such models with exact basic reproduction number and evaluate the results obtained by th next-generation matrix.
In this paper, we derived basic reproduction number R0 for SEIRS, SEIR, SIRS, SIR, SIS models on regular lattice space using the next-generation matrix approach with pair approximation. We cannot find an appropriate quantity or variable to compare these results with Monte Carlo simulation except SIR model and then, we leave it as a future problem to estimate a basic reproduction number by Monte Carlo simulation for other basic epidemic models.
Here, we consider the basic reproduction number R0 for fundamental epidemic models by using next-generation matrix only on a regular lattice. However, realistic epidemics may usually occur on heterogeneous networks, then we will study the effects of these spatial structures on R0 by next-generation matrix approach in the future.
We sincerely appreciate Prof. Akira Sasaki and Dr. Koichi Saeki for telling me an idea to use next-generation matrix approach to get basic reproduction number for epidemic models on lattice. We also thank two anonymous reviewers whose comments are very much helpful to revise our manuscript. The authors would like to thank Enago (www.enago.jp) for the English language review.
The author declares there is no conflict of interest.
[1] | O. Diekmann, H. Heesterbeek and T. Britton, Mathematical Tools for Understanding Infectious Disease Dynamics, Princeton University Press, Princeton and Oxford, 2013. |
[2] | O. Diekmann, J. A. P. Heesterbeek and J. A. J. Metz, On the definition and the computation of the basic reproduction ratio R0 in models for infectious diseases in heterogeneous populations, J. Math. Biol., 28 (1990), 365–382. |
[3] | P. van den Driessche and J. Watmough, Reproduction numbers and sub-threshold endemic equilibria for compartmental models of disease transmission, Math. Biosci., 180 (2002), 29–48. |
[4] | C. Castillo-Chavez, Z. Feng and W. Huang, On the computation of R0 and its role on global stability, in Mathematical Approaches for Emerging and Reemerging Infectious Diseases: An Introduction, Springer, New York (2002), 229–250. |
[5] | M. Martcheva, An Introduction to Mathematical Epidemiology, Springer: New York, 2015, 16. |
[6] | K. Saeki and A. Sasaki, The role of spatial heterogeneity in the evolution of local and global infections of viruses, PLoS Comput. Biol., 14 (2018), e1005952. |
[7] | W. O. Kermack and A. G. Mc.Kendrick, A contributions to the mathematical theory of epidemics, Proc. R. Soc. A, 115 (1991), 700–721. |
[8] | M. J. Keeling, The effects of local spatial structure on epidemiological invasions, P. Roy. Soc. Lond. B Bio., 266 (1999), 859–867. |
[9] | H. Matsuda, N. Ogita, A. Sasaki, et al., Statistical mechanics of population: The lattice Lotoka-Volterra model, Prog. Theor. Phys., 88 (1992), 1035–1049. |
[10] | C. T. Bauch, The spread of infectious diseases in spatially structured populations: An invasory pair approximation, Math. Biosci., 198 (2005), 217–237. |
[11] | N. Ringa and C. T. Bauch, Dynamics and control of foot-and-mouth disease in endemic countries: A pair approximation model, J. Theor. Biol., 357 (2014), 150–159. |
[12] | H. Inaba, Age-Structured Population Dynamics in Demography and Epidemiology, Springer, New York, 2017. |
[13] | I. Z. Kiss, J. C. Miller and P. L. Simon, Mathematics of Epidemics on Networks, Cham: Springer (2017) 598. |
[14] | P. Trapman, Reproduction numbers for epidemics on networks using pair approximation, Math. Biosci., 210 (2007), 464–489. |
1. | Kazuhiro Bessho, Kenta Yashima, Toyomitsu Horii, Masakazu Hori, Spatially explicit modeling of metapopulation dynamics of broadcast spawners and stabilizing/destabilizing effects of heterogeneity of quality across local habitats, 2020, 492, 00225193, 110157, 10.1016/j.jtbi.2020.110157 | |
2. | F Zuhairoh, D Rosadi, A R Effendie, Determination of Basic Reproduction Numbers using Transition Intensities Multi-state SIRD Model for COVID-19 in Indonesia, 2021, 1821, 1742-6588, 012050, 10.1088/1742-6596/1821/1/012050 | |
3. | Long Zhang, Xiaolin Fan, Zhidong Teng, Global dynamics of a nonautonomous SEIRS epidemic model with vaccination and nonlinear incidence, 2021, 0170-4214, 10.1002/mma.7359 | |
4. | Honglv Xu, Yi Zhang, Min Yuan, Liya Ma, Meng Liu, Hong Gan, Wenwen Liu, Gillian Gianna Anne Lum, Fangbiao Tao, Basic Reproduction Number of the 2019 Novel Coronavirus Disease in the Major Endemic Areas of China: A Latent Profile Analysis, 2021, 9, 2296-2565, 10.3389/fpubh.2021.575315 | |
5. | Phongchai Jittamai, Natdanai Chanlawong, Wanyok Atisattapong, Wanwarat Anlamlert, Natthiya Buensanteai, Reproduction number and sensitivity analysis of cassava mosaic disease spread for policy design, 2021, 18, 1551-0018, 5069, 10.3934/mbe.2021258 | |
6. | A. Abdelkhalek, A.H. Abdel Kader, I.L. El-Kalla, A. Elsaid, Amr Elsonbaty, K.S. Nisar, Dynamical analysis of a complex competitive two-strain epidemic network model with vaccination, 2025, 13, 26668181, 101058, 10.1016/j.padiff.2024.101058 |