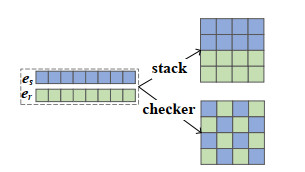
Citation: Hui-Yu Huang, Wei-Chang Tsai. An effcient motion deblurring based on FPSF and clustering[J]. Mathematical Biosciences and Engineering, 2019, 16(5): 4036-4052. doi: 10.3934/mbe.2019199
[1] | Yang Liu, Tianran Tao, Xuemei Liu, Jiayun Tian, Zehong Ren, Yize Wang, Xingzhi Wang, Ying Gao . Knowledge graph completion method for hydraulic engineering coupled with spatial transformation and an attention mechanism. Mathematical Biosciences and Engineering, 2024, 21(1): 1394-1412. doi: 10.3934/mbe.2024060 |
[2] | Hongqiang Zhu . A graph neural network-enhanced knowledge graph framework for intelligent analysis of policing cases. Mathematical Biosciences and Engineering, 2023, 20(7): 11585-11604. doi: 10.3934/mbe.2023514 |
[3] | Shi Liu, Kaiyang Li, Yaoying Wang, Tianyou Zhu, Jiwei Li, Zhenyu Chen . Knowledge graph embedding by fusing multimodal content via cross-modal learning. Mathematical Biosciences and Engineering, 2023, 20(8): 14180-14200. doi: 10.3934/mbe.2023634 |
[4] | Xianfang Wang, Qimeng Li, Yifeng Liu, Zhiyong Du, Ruixia Jin . Drug repositioning of COVID-19 based on mixed graph network and ion channel. Mathematical Biosciences and Engineering, 2022, 19(4): 3269-3284. doi: 10.3934/mbe.2022151 |
[5] | Huiqing Wang, Sen Zhao, Jing Zhao, Zhipeng Feng . A model for predicting drug-disease associations based on dense convolutional attention network. Mathematical Biosciences and Engineering, 2021, 18(6): 7419-7439. doi: 10.3934/mbe.2021367 |
[6] | Lifang Wang, Xinyu Lu, Zejun Jiang, Zhikai Zhang, Ronghan Li, Meng Zhao, Daqing Chen . FRS: A simple knowledge graph embedding model for entity prediction. Mathematical Biosciences and Engineering, 2019, 16(6): 7789-7807. doi: 10.3934/mbe.2019391 |
[7] | Hanming Zhai, Xiaojun Lv, Zhiwen Hou, Xin Tong, Fanliang Bu . MLSFF: Multi-level structural features fusion for multi-modal knowledge graph completion. Mathematical Biosciences and Engineering, 2023, 20(8): 14096-14116. doi: 10.3934/mbe.2023630 |
[8] | Ying Xu, Jinyong Cheng . Secondary structure prediction of protein based on multi scale convolutional attention neural networks. Mathematical Biosciences and Engineering, 2021, 18(4): 3404-3422. doi: 10.3934/mbe.2021170 |
[9] | Zhangjie Wu, Minming Gu . A novel attention-guided ECA-CNN architecture for sEMG-based gait classification. Mathematical Biosciences and Engineering, 2023, 20(4): 7140-7153. doi: 10.3934/mbe.2023308 |
[10] | Peng Wang, Shiyi Zou, Jiajun Liu, Wenjun Ke . Matching biomedical ontologies with GCN-based feature propagation. Mathematical Biosciences and Engineering, 2022, 19(8): 8479-8504. doi: 10.3934/mbe.2022394 |
In recent years, large-scale knowledge bases such as FreeBase [1], DBpedia [2], YAGO [3], and WordNet [4] have been widely used in various domains, including information retrieval [5], question answering [6], and recommender systems [7]. Their powerful abilities for semantic processing and knowledge generalization have paved a new way for data management and mining. A knowledge graph (KG) is a graph representation of a knowledge base in the form of a collection of fact triples (s,r,o), each of which denotes a relation edge r between a head entity node s and a tail entity node o. The task of knowledge graph completion (KGC) is performed to improve the integrity of the KG which suffers from incompleteness by predicting the missing triples according to the facts. KGC can be performed by either extracting new facts from external sources, such as online encyclopedias and news wires [8], or by inferring them from those already in the KG. The latter approach, typically called Link Prediction (LP), is the focus of our paper.
Knowledge graph embedding (KGE) models have been shown to achieve the best performance for the task of link prediction in KGs among all the existing methods [9]. To learn low-dimensional vector or matrix representations of entities and relations in KGs, a lot of knowledge graph embedding models are proposed. Specifically, the classic triple-based embedding models are mainly divided into translation-based models (e.g., TransE [10], TransH [11], TransAH [12], TransR [13], TransD [14]), bilinear and tensor models (e.g., DistMult [15], SimplE [16], TuckER [17]), neural network models (e.g., NTN [18], ER-MLP [19], ConvE [20], ConvKB [21], Conv-TransE [22], InteractE [23], DMACM [24], KMAE [25], JointE [26], CTKGC [27]), and complex vector models (e.g., ComplEx [28], RotatE [29], QuatE [30]).
Among all of the above models, convolutional neural network (CNN) based KGE models are attracting increasing research interest because they benefit from the explosion of deep learning techniques and exhibit strong expression and generalization abilities. The performance of CNN-based KGE models depends on the interactions between entities and relations. Considerable research efforts have been devoted to increasing the interactions between entities and relations. InteractE [23] increases interactions by embedding permutations. The directional multi-dimensional attention mechanism is designed to explore the deep expressive characteristic of the triple in DMACM [24]. Gaussian kernel and multi-attention techniques are adopted to expand the entities and relation embedding in KMAE [25]. JointE [26] utilizes 1D and 2D convolution operations jointly to extract the surface and explicit knowledge and increase the interactions between entities and relations. However, in order to improve performance, these models are complex with a large number of parameters. How to balance the efficiency and effectiveness of the CNN-based KGE model is still an open problem.
In this paper, we propose a lightweight CNN-based KGE model named IntSE with channel attention to improve the prediction accuracy for LP in KGs. Inspired by InteractE, checkered feature reshaping and circular convolution are adopted to increase the interactions between the components of entity and relation embeddings in IntSE. A channel attention module of IntSE is designed to enhance useful interactions while suppressing useless ones. IntSE is light and flexible, greatly reducing the number of parameters, but still has a competitive prediction accuracy for LP. The main contributions of this paper are summarized as follows:
1) We propose a lightweight CNN-based KGE model named IntSE to improve the prediction accuracy for LP in KGs. IntSE is efficient and effective for LP through three key components: checkered feature reshaping, circular convolution, and channel attention mechanism. Checkered feature reshaping and circular convolution operations are very effective for increasing interactions between entities and relations. Moreover, the channel attention mechanism further enhances the beneficial interactions.
2) In order to further improve the performance of link prediction, we propose a channel attention block called LPSENet, which improves the gate mechanism of the SENet prototype. LPSENet improves the expression ability while reducing the model overfitting of IntSE.
3) We conduct extensive experiments on publicly available datasets to evaluate the performance of IntSE. The results show that IntSE achieves significantly higher accuracy for LP in KGs than the mainstream KGE models.
Paper organization: The rest of this paper is organized as follows. The existing literature related to this work is discussed in Section 2. Our proposed IntSE models for LP are presented in Section 3. The experimental setup and results are described in Section 4. The ablation studies which investigate how the checkered feature reshaping, circular convolution, and channel attention mechanism affect the performance of IntSE are discussed in Section 5. Finally, the whole paper is concluded in Section 6.
KGE learns an embedding representation of entities and relations of a KG in a continuous low-dimensional vector space. Wang et al. [31], Nguyen [9], and Liu et al. [32] systematically reviewed KGE models for knowledge graph completion (KGC). Moreover, Rossi et al. [33] and Akrami et al. [34] conducted experimental studies to assess the effectiveness of different link prediction (LP) methods on real-world data. According to the different ways of expressing the semantics between entities and relations, there are four families of KGE models: (a) TransE [10] and its families (such as TransH [11], TransAH [12], TransR [13], and TransD [14]) used the translation invariance to express the association between entities and relations; (b) DistMult [15] and its improved versions [16,17] utilized a diagonal matrix to express the relations for model simplification; (c) ComplEx [28] and its variants [29,30] further extended DistMult to the complex field, which could better model the asymmetric relations in KGs; and (d) Neural network (NN) based models (such as ER-MLP [19], NTN [13], ConvE [20], Conv-TransE [22], ConvKB [21], InteractE [23], DMACM [24], KMAE [25], JointE [26]) used neural network to model semantics between entity embeddings and relation embeddings. Among all the above methods, NN-based models demonstrated outstanding performance in prediction accuracy due to their excellent feature extraction ability. NN-based models for KGE, especially CNN-based models, have received more attention in recent years due to the advantages of convolution operation, such as parameter sharing, generalization, overfitting reduction, and robustness.
ConvE [20] is the first work on the CNN-based KGE model. It reshaped the head entity embedding and the relation embedding separately and stacked them into a 2D convolution layer to extract semantic features of entities and relations. The generated feature mapping was vectorized and projected into the embedding space of tail entities. Then, all candidate tail entity embedding vectors were matched through their inner products. Conv-TransE [22] considered that the reshaping of entity and relation embeddings in ConvE destroyed the translation invariance of the embedding vector. Thus, it removed the embedding vector reshape operation in ConvE and directly put the known entity and relation embeddings into the convolutional layer. The remaining procedure of Conv-TransE was the same as that of ConvE. Thus, Conv-TransE could be regarded as a variant of ConvE. ConvKB [21] was another CNN-based KGE model, which stacked the head entity embedding, relation embedding, and tail entity embedding into a matrix M, and used a convolution operation with filters ω∈R1×3 to act on each dimensional vector after M transposition. ConvKB was controversial since it obtained a competitive result on the FB15k-237 [35] dataset, but a disappointing result on the WN18RR [20]. As its performance varies with datasets and implementations, we do not compare it in our experiments. InteractE [23] found that the stacked feature reshaping of ConvE (see Figure 1) limited the feature interaction between entities and relations. Thus, it increased the feature interaction between entity and relation embeddings to improve the performance for LP. Specifically, it reshaped the entity and relation embeddings into a checkered structure (see Figure 1), while using circular convolution to enhance the edge feature interaction. However, feature permutation in InteractE is costly. DMACM [24] found that the existing CNN-based KGE model ignored the directional relation characteristic and implicit fine-grained feature in the triple. Thus, it was proposed to explore the directional information and an inherent deep expressive characteristic of the triple by the directional multi-dimensional attention. Then, the output of directional multi-dimensional attention could be put in the convolution neural network with filters ω∈R1×3. KMAE [25] first expanded the entity embedding and relation embedding into entity kernel and relation kernel using the Gaussian kernel function. Entity kernel or relation kernel combined relation embedding or entity embedding input into a 2D convolutional layer, then two groups of channel attention and spatial attention captured high-quality feature vector information. JointE [26] consisted of path 1 and path 2 in two feed-forward paths. Path 1 used 1D convolution filters over input the entity and relation embedding to extract the surface and explicit knowledge. To reduce the number of parameters, path 2 employed different 2D convolution filters to extract the deep features from the reshaped entity and relation embedding. The final features of the entity and relation were obtained by element-wise addition between the output of path 1 and the output of path 2.
In conclusion, CNN-based KGE models should expand feature extraction capability and increase the interactions between the entities and relations to improve the performance of LP. The key components of existing methods include the initial embedding of the entities and relation, the reshaping plan of the entities and relation embeddings, the convolution operations to extract features, and the attention-based feature calibration. Although many complex models can improve the performance for LP, they introduce too many parameters that hinder the model's efficiency. Thus, our goal is to design a CNN-based KGE model that can strike a better balance between performance and cost.
In this section, we introduce the proposed model IntSE. We first introduce the related notations and problem definition and then describe the architecture of IntSE in detail, as shown in Figure 2.
Let E and R denote the set of entities and relation types. A Knowledge Graph (KG) G consists of a set of fact triples (s,r,o), which is formally expressed as follows:
G={(s,r,o)∣s,o∈E,r∈R}⊆E×R×E |
Link Prediction (LP) is to predict the missing entity in a triple (s,r,o), such as predicting o for a given (s,r,?) or s for a given (?,r,o). LP can be formalized as a single sample learning-to-rank problem. A KGE-based LP method has two key components, namely encoding and scoring. The encoding component maps the head entity s, relation r, and tail entity o to the d-dimensional vector representations es, er, eo∈Rd. Then, the scoring component measures the authenticity of triples. The goal of LP is to learn a scoring function ψ about entity and relation embeddings so that the score ψ(s,r,o) of fact triples (s,r,o) is higher than that ψ(s′,r,o′) of non-fact triples (s′,r,o′).
The architecture of our IntSE model is shown in Figure 2. The key components of IntSE are checkered feature reshaping, circular convolution operation, and channel attention mechanism. Given a d-dimensional entity embedding vector es and a d-dimensional relation embedding vector er, IntSE first reshapes es and er into a checkered matrix ϕchk(es,er)∈Rm×n, where m×n=2d, and then sends it to a 2D circular convolutional layer. Then, the convolutional layer outputs a feature map tensor X=[x1, x2, …, xC]∈RH×W×C, which is sent to the channel attention block for feature calibration to generate the calibrated feature map tensor ¯X∈RH×W×C. Next, ¯X is vectorized into vec(¯X)∈RHWC, projected to the d-dimensional vector space using the linear transformation of the parameter matrix as W∈RHWC×d, and finally matched with the tail entity eo via the inner product operation.
The checkered feature reshaping increases the feature interaction between the components of entity and relation embeddings, and thus improves the expression ability of CNNs. Different from the stacked feature reshaping (see Figure 1), a checkered structure (see Figure 1) arranges the entity and relation embeddings such that no two adjacent cells are occupied by components of the same embedding to increase the feature interaction between entity and relation embeddings. The components of entity and relation embedding are randomly assigned to cells of a checkered structure in IntSE. Then, a 2D circular convolutional layer with filters ω∈R3×3 is adopted to extract the deep and latent knowledge from the checkered features. Circular convolution induces more interactions than standard convolution and thus further expands the expression ability of IntSE.
In this paper, we are inspired by InteractE and borrow the checkered feature reshaping and circular convolution components to IntSE. Unlike the feature enumerations of InteractE, which take up a large number of parameters, IntSE only uses one checkered feature reshaping and one circular convolutional layer to improve the efficiency of the model.
SENet [36] explicitly defines the association between feature channels and filters the feature channel information by self-learning the weight of each channel, so as to enhance useful features and suppress useless features. We are inspired by SENet and related works, SENet is added to IntSE to enhance useful interactions and improve the performance of LP. However, the improvement is still quite limited. Therefore, we further propose a new SENet variant called LPSENet as shown in Figure 3 to enhance the useful features for LP.
For a feature map tensor X=[x1,x2,…,xC]∈RH×W×C, where xi represents the feature map of i-th channel of size H×W, and C denotes the total number of channels. The operation flow of LPSENet is as follows:
1) Squeeze: LPSENet performs the Global Average Pooling (GAP) on each feature channel xc to obtain statistical information as lc in the following:
lc=p(X)=1WHW,H∑i=1,j=1Xij. | (3.1) |
The total C statistical values are aggregated into a compressed feature tensor L=[l1,l2,…,lC]∈R1×1×C, which contains the global information of all features.
2) Excitation: To capture the dependence between channels, the gating mechanism is used to generate the weight matrix A=[a1,a2,…,aC]∈R1×1×C corresponding to each feature channel. Unlike the gating mechanism of SENet, we design a lighter and more efficient gating mechanism. SENet uses a gating mechanism consisting of a fully connected dimension-reduction layer, a ReLU [37] activation layer, and a fully connected dimension-increasing layer. The fully connected layer has a huge number of parameters and is prone to overfitting. Thus, in the LPSENet, the fully connected layer is changed to the convolutional layer with filters ω∈R1×1, and Dropout is added to avoid overfitting. Equations (3.2) and (3.3) formalize the gate mechanism of SENet and LPSENet, respectively.
A=σ(W2f(W1L)), | (3.2) |
A=σ(f(f(L⋆ω1)⋆ω2)), | (3.3) |
where f is the ReLU function and σ is the Sigmoid function, W1∈RCq×C and W2∈RC×Cq are learned to explicitly model the correlation between feature channels, and q is the reduction ratio, ω1 and ω2 are the convolution filters.
Compared with the SENet, LPSENet introduces more non-linearity. Thus, it has a higher expressive ability and is more robust to overfitting. The convolutional layer has the same output effect as the fully connected layer, but reduces the number of parameters and improves the computational efficiency. The experimental results in Section 5 will show that the LPSENet is more suitable for LP than SENet.
3) Scale: The output weight A of the excitation operation represents the importance of each feature channel after feature selection, and the final output of the LPSENet block ¯X=[¯x1,¯x2,…,¯xC]∈RH×W×C is obtained by rescaling the input feature map X with the activations:
¯xc=ac⋅xc | (3.4) |
where ¯xc refers to channel-wise multiplication between the scalar ac and the feature map xc.
The goal of LP is to learn a scoring function ψ about entity and relation embeddings so that the score ψ(s,r,o) of fact triples (s,r,o) is higher than that ψ(s′,r,o′) of non-fact triples (s′,r,o′). Table 1 summarizes the scoring functions of CNN-based KGE models, where ¯es and ¯er denote the 2D stack reshapings of the entity embedding es and relation embedding er, [;] represents the concatenation operation, ⋆ denotes the standard convolution operation, ω denotes the set of convolutional filters, W and w are the learned weight matrix, ϕchk(Pk(es,er)) denotes k different checkered reshaping of es and er, ♣ denotes the circular convolution, vec(⋅) represents the vectorization function, f and g are non-linear functions, DM(⋅) represents the directional multi-dimensional attention functions of DMACM [24], kernel(⋅) and Mul(⋅) represent the Gaussian kernel functions and multi-attention in KMAE [25], ca(⋅) represents the channel attention functions of IntSE.
Model | fScoring Function ψ(es,er,eo) |
ConvE [20] | f(vec(f([¯es;¯er]⋆ω))W)eo |
Conv-TransE [22] | g(vec(f([es;er]⋆ω))W)eo |
ConvKB [21] | concat(g([es;er;eo]⋆ω)w) |
InteractE [23] | g(vec(f(ϕchk(Pk(es,er))♣ω))W)eo |
DMACM [24] | concat(f(DM(es,er,eo))⋆ω)W |
KMAE [25] | g(Mul([kernel(¯es);¯er]||[¯es;kernel(¯er)]))eo |
JointE [26] | f([es;er]⋆ω11D⋆ω21D+vec([¯es⋆ωr;¯er⋆ωs])W)eo |
IntSE | g(vec(ca(f(ϕchk(es,er)♣ω)))W)eo |
In order to train the model parameters, we use the binary cross entropy with label smoothing as the loss function [20], as shown in Eq (3.5):
Γ(p,t)=−1N∑i(ti⋅log2(pi)+(1−ti)⋅log2(1−pi)), | (3.5) |
where p=σ(ψ(s,r,o)) indicates the probability of correct link prediction through applying the logistic sigmoid function σ(⋅) to the scores ψ(s,r,o) and t is the smoothing label. In this paper, we use Adam [38] as the optimizer and label smoothing to reduce the overfitting due to the saturation of output non-linearity at the labels [39].
In this section, we first describe the setup of our experiments. Then, we present the detailed experimental results and provide thorough analyses.
Dataset: We use the two most widely used knowledge graph datasets for link prediction, namely FB15k-237 [35] and WN18RR [20], in our experiments. Specifically, FB15k-237 is an improved version of the FB15k [10] dataset derived from FreeBase [1], where all reversal relations in FB15k are deleted to prevent test triples from being directly deduced by reverse training triples. WN18RR is a subset of the WN18 [10] dataset derived from WordNet [4], and the inverse relationships are also deleted similar to FB15k-237. The characteristics of the two datasets are shown in Table 2.
Dataset | #entities | #relations | #train | #valid | #test |
FB15k-237 | 14,541 | 237 | 272,115 | 17,535 | 20,466 |
WN18RR | 40,943 | 11 | 86,835 | 3034 | 3134 |
Evaluation metrics: In this paper, we use the four commonly used evaluation criteria for link prediction, namely, MR, MRR, Hits@1, and Hits@10, to measure the performance of different models. The mean rank (MR) represents the mean of the test triples' ranks, i.e.,
MR=12|T|∑(s,r,o)∈T(ranks+ranko), |
where |T| is the size of the test set. The mean reciprocal rank (MRR) is the average inverse of the harmonic mean of the test triples' ranks, i.e.,
MRR=12|T|∑(s,r,o)∈T(1ranks+1ranko). |
And Hits@k is the percentage of top-k results that are correct. Among the four indicators, lower MR and higher MRR, Hits@1, and Hits@10 indicate better performance.
Hyperparameter setting and implementation: The ranges of hyperparameters are set as follows: the learning rate γ∈{0.01,0.001,0.005,0.0001}, the entity and relation embedding dimension d∈{100,200}, the kernel size in the 2D convolutional layer k∈{5,7,9,11}, the reduction ratio of LPSENet 1/q, where q∈{2,4,8,16}. In addition, the experiment also uses batch normalization and Dropout to avoid overfitting. To decide the values of hyperparameters, each model is trained for 500 epochs via the grid search method, and the hyperparameters with the best performance are selected according to the MRR on the validation set [20,23]. For the FB15k-237 dataset, the parameter settings are γ=0.0001, d=200, k=9, and q=4, and the batch size is 128. For the WN18RR dataset, the parameter settings are γ=0.001, d=200, k=11, and q=8, and the batch size is 256. We implemented our models with PyTorch in Python 3. All experiments were conducted on a server with 4 Intel® Xeon® w-2104 CPUs @ 3.20GHz, 32GB DDR4 RAM, and 1 NVIDIA® GeForce® RTXTM 2060 GPU with 8GB GDDR6 RAM.
In the experiments, we compare IntSE with the six baseline models, namely ConvE [20], Conv-TransE [22], InteractE [23], DMACM [24], KMAE [25], JointE [26].
The prediction accuracy of different models on the FB15k-237 and WN18RR datasets is shown in Table 3. The scores of all the baselines are taken directly from the values reported in the original papers. The best and second-best results are highlighted by bold and underlined texts, respectively. We observe that IntSE achieves high accuracy in both datasets: it achieves competitive performance in all evaluation metrics and outperforms all the baselines in terms of MRR and Hits@1 on FB15k-237. Specifically, we draw the following conclusions from the experimental results:
Model | FB15k-237 | WN18RR | ||||||
MRR | MR | Hits@10 | Hits@1 | MRR | MR | Hits@10 | Hits@1 | |
ConvE [20] | 0.325 | 244 | 0.501 | 0.237 | 0.430 | 4187 | 0.520 | 0.400 |
Conv-TransE [22] | 0.330 | - | 0.510 | 0.240 | 0.460 | - | 0.520 | 0.430 |
InteractE [23] | 0.354 | 172 | 0.535 | 0.263 | 0.463 | 5202 | 0.528 | 0.430 |
DMACM [24] | 0.270 | 244 | 0.440 | - | 0.230 | 552 | 0.540 | - |
KMAE [25] | 0.326 | 235 | 0.502 | 0.240 | 0.448 | 4441 | 0.524 | 0.415 |
JointE [26] | 0.356 | 177 | 0.543 | 0.262 | 0.471 | 4655 | 0.537 | 0.438 |
IntSE | 0.359 | 179 | 0.540 | 0.267 | 0.469 | 5007 | 0.532 | 0.439 |
1) IntSE outperforms InteractE in terms of MRR, Hits@10, and Hits@1 on both datasets, which indicates that channel attention effectively improves the performance of the CNN-based KGE model for LP. Furthermore, IntSE obtains improvements of 2.5 and 3% over InteractE in terms of MRR and Hits@1 on the FB15k-237 dataset. Then, on the WN18RR dataset, the improvement rates of IntSE over InteractE on MRR and Hits@1 are 1.7 and 2.3%. These results confirm that IntSE not only retains the advantages of InteractE but also further enhances the useful features of InteractE.
2) Both IntSE and InteractE outperform KMAE in terms of MRR, Hits@10, and Hits@1 on both datasets, which indicates that checkered feature reshaping and circular convolution operations increase feature interactions between the entities and relations are effective. The ablation studies of IntSE in Section 5 further demonstrate the effectiveness of checkered feature reshaping and circular convolution operations.
3) It is unexpected that DMACM outperforms all the models in the MR and Hits@10 on the WN18RR dataset. However, DMACM is inferior to others models in the MRR metrics on both datasets. The reason may be the distinguishing feature of WN18RR, which has fewer relations but more entities.
4) Compared to JointE, the state-of-the-art CNN-based KGE model, IntSE performs better in terms of Hits@1 on both datasets. Moreover, they are well-matched in other metrics.
To further verify the effectiveness of IntSE, we also evaluate the performance of the models for LP in different categories of relations on the FB15k-237 dataset. We use FB15k-237 in this set of experiments because its relation is more diverse. Based on the average number of tail entities per head entity and the average number of head entities per tail entity, the relation is divided into four categories: 1-to-1, 1-to-n, n-to-1, and n-to-m. Here, an average number of less than 1.5 is marked as "1" and "n" otherwise. Among the 224 distinct relations in the test set of FB15k-237, 5.8% are 1-to-1, 11.6% are 1-to-n, 33.9% are n-to-1, and 48.7% are n-to-m relations. But on the WN18RR dataset, the 11 distinct relations in the test set are distributed as 2, 4, 3, and 2 in these four classes [34]. Because all the other baselines don't report the performance of LP in different relation categories in their papers, ConvE [20] and InteractE [23], two classical CNN-based KGE models, are used for comparison in the experiments. MRR and Hits@10 are used as evaluation criteria [14]. Table 4 presents the experimental results of different models for LP on the FB15k-237 dataset. From Table 4, we find that IntSE achieves better performance than ConvE and InteractE in all four relation categories, whether it deals with simple relation categories (e.g., 1-to-1) or more complex relation categories (e.g., 1-to-n and n-to-m). It is verified again that IntSE has good robustness and is suitable for link prediction tasks with various relation categories.
ConvE [20] | InteractE [23] | IntSE | |||||
MRR | Hits@10 | MRR | Hits@10 | MRR | Hits@10 | ||
Head Pred | 1-to-1 | 0.374 | 0.505 | 0.386 | 0.547 | 0.391 | 0.561 |
1-to-n | 0.091 | 0.170 | 0.106 | 0.192 | 0.112 | 0.198 | |
n-to-1 | 0.444 | 0.644 | 0.466 | 0.647 | 0.472 | 0.652 | |
n-to-m | 0.261 | 0.459 | 0.276 | 0.476 | 0.285 | 0.479 | |
Tail Pred | 1-to-1 | 0.366 | 0.510 | 0.368 | 0.547 | 0.372 | 0.550 |
1-to-n | 0.762 | 0.878 | 0.777 | 0.881 | 0.780 | 0.888 | |
n-to-1 | 0.069 | 0.150 | 0.074 | 0.141 | 0.079 | 0.152 | |
n-to-m | 0.375 | 0.603 | 0.395 | 0.617 | 0.400 | 0.621 |
IntSE only uses one checkered feature reshaping and one circular convolutional layer; that is, the number of feature permutation perm in IntSE is one. We conduct experiments in the same setting to further evaluate the impact of feature permutation on the prediction accuracy of InteractE and IntSE. Table 5 presents the experimental results. We can see that the prediction accuracy of InteractE and IntSE drops slowly with the increase of perm on the FB15k-237 dataset. IntSE and InteractE work best when perm is one. This result is consistent with that reported in the paper InteractE [23]. Nevertheless, IntSE is still superior to InteractE in all cases under all the metrics. From Table 5, we can further conclude that increasing the beneficial feature interactions is crucial to improve the performance of CNN-based KGE models.
InteractE [23] | IntSE | |||||
perm = 1 | perm = 2 | perm = 3 | perm = 1 | perm = 2 | perm = 3 | |
MRR | 0.353 | 0.353 | 0.349 | 0.354 | 0.353 | 0.350 |
Hits@10 | 0.537 | 0.534 | 0.533 | 0.539 | 0.537 | 0.535 |
Hits@20 | 0.619 | 0.616 | 0.613 | 0.620 | 0.615 | 0.614 |
Hits@40 | 0.694 | 0.692 | 0.688 | 0.695 | 0.691 | 0.688 |
Hits@80 | 0.764 | 0.761 | 0.759 | 0.766 | 0.763 | 0.758 |
Hits@160 | 0.829 | 0.824 | 0.822 | 0.830 | 0.827 | 0.822 |
We also evaluate the parameter efficiency of different models on the FB15k-237 dataset. Table 6 presents the experimental results. The number of parameters in IntSE is larger than ConvE because IntSE adopts 96 convolution filters of size 9×9 while ConvE adopts 32 convolution filters of size 3×3. The fully-connected layer of IntSE has much more parameters than ConvE. The number of parameters in IntSE is nearly equal to that of InteractE when the feature permutation perm is one. The channel attention mechanism of IntSE is lightweight with only a few thousand additional parameters. The number of parameters in InteractE increases significantly with the feature permutation perm because the number of parameters in the convolution and fully-connected layers of InteractE is positively correlated with perm.
Model | ConvE [20] | InteractE [23] | IntSE | |||
perm = 1 | perm = 2 | perm = 3 | perm = 4 | |||
Parameters | 4.96M | 10.7M | 18.38M | 26.07M | 33.75M | 10.7M |
There are three key components in IntSE: checkered feature reshaping, circular convolution operation, and channel attention mechanism. Based on the public FB15k-237 dataset, ablation studies are conducted to evaluate the effects of these key components on the performance of IntSE. Specially, we evaluate the effects of the three different channel attention mechanisms on the performance of IntSE.
The effects of key components on the performance of IntSE are shown in Table 7. The sr, cr, sc, cc, and ca denote the stacked feature reshaping, checkered feature reshaping, standard convolution, circular convolution, and channel attention components, respectively. From Table 7, we can see that checkered feature reshaping, circular convolution operation, and channel attention are three components that significantly contribute to the performance of IntSE. The channel attention mechanism significantly boosts performance in all cases. Although cr+cc outperforms most variants of IntSE with the help of checkered feature reshaping and circular convolution, it is inferior to IntSE. IntSE obtains improvements of 4 and 3.5% over cr+cc in terms of MRR and Hits@1 with the help of the channel attention mechanism. These findings further demonstrate the effectiveness of IntSE and the importance of the channel attention mechanism.
Model | MRR | MR | Hits@10 | Hits@1 |
sr+sc (ConvE) | 0.325 | 244 | 0.501 | 0.237 |
sr+sc+ca | 0.342 | 181 | 0.525 | 0.251 |
cr+sc | 0.338 | 185 | 0.519 | 0.249 |
cr+sc+ca | 0.350 | 193 | 0.536 | 0.262 |
cr+cc | 0.346 | 175 | 0.532 | 0.258 |
cr+cc+ca (IntSE) | 0.359 | 179 | 0.540 | 0.267 |
The channel attention mechanism is the key component of IntSE. To further confirm the necessity of the channel attention mechanism, we evaluate the effects of the three different channel attention mechanisms on the performance of IntSE. Specifically, SENet [36] is the first work to boost the representation power of a CNN by enhancing channel relationship; SKNet [40] is the first to explicitly focus on the adaptive receptive field size of neurons by introducing the attention mechanism; ECA-Net [41] is the most effective channel attention module of deep CNNs in computer vision applications. Table 8 presents the detailed results of IntSE variants with different channel attention mechanisms under the four evaluation indicators: MR, MRR, Hits@1, and Hits@10.
Model | MRR | MR | Hits@10 | Hits@1 |
IntSE-SENet | 0.358 | 183 | 0.538 | 0.266 |
IntSE-SKNet | 0.350 | 193 | 0.527 | 0.262 |
IntSE-ECANet | 0.346 | 223 | 0.529 | 0.250 |
IntSE-ECANet' | 0.355 | 184 | 0.536 | 0.263 |
IntSE-SENet performs the best among all models compared. To our surprise, ECA-Net brings side effects on IntSE. Especially the IntSE-ECANet model, which integrates checkered feature reshaping, circular convolution operation, and ECA-Net, has the lowest performance. The main reason is that a KG has different data characteristics from images and video. The IntSE model is so simple that there is only one convolution layer with a small input size, while deep CNNs in computer vision applications often have very large input sizes. Detailed analyses of the experimental results lead to the following views in the CNN-based KGE models for LP:
1) The channel attention is more important than the spatial attention. SKnet uses soft attention to fuse the features of multiple convolution branches with different kernel sizes. The fact that IntSE outperforms IntSE-SKNet for LP indicates that the convolution kernel size of IntSE is more appropriate. Moreover, since IntSE-SENet outperforms IntSE-SKNet, we again confirm that channel attention is more important than spatial attention. The experimental results in [42] also show that the channel-first order is slightly better than the spatial-first order. Therefore, we should pay more attention to explicitly defining the association between feature channels so as to improve the performance for LP.
2) The global cross-channel interaction is more important than local cross-channel interaction. Although ECA-Net is an efficient and effective channel attention mechanism for deep CNNs, the performance of IntSE-ECANet is lower than IntSE-SENet. The possible reason is that a fast 1D convolution with kernel size k in ECA-Net only captures the local cross-channel interaction rather than the global cross-channel interaction. To verify the hypothesis, we conduct additional experiments on a model named IntSE-ECANet' which adopts a variant of ECANet with only a single fully connected layer. Since IntSE-ECANet' exhibits better performance than IntSE-ECANet, we further confirm that the global cross-channel interaction is more important than the local cross-channel interaction in CNN-based KGE models for LP. We observe that IntSE-SENet performs the best among all models compared. This indicates that the two fully connected layers designed in SENet to capture the non-linear global cross-channel interactions are effective, and we can improve the performance of the CNN-based KGE model on LP by introducing SENet.
The channel attention module of IntSE is LPSENet which changes the gating mechanism of SENet. In the LPSENet, the fully connected layer is changed to the convolutional layer with filters ω∈R1×1, and Dropout is added to avoid overfitting. We also evaluate the performance of IntSE with different gating mechanisms. The results are shown in Table 9. We can see that IntSE achieves better performance with the gating mechanism of LPSENet. The reason is that it has a higher expressive ability and more robustness to overfitting.
Model | MRR | MR | Hits@10 | Hits@1 |
IntSE-SENet | 0.466 | 5123 | 0.530 | 0.434 |
IntSE | 0.469 | 5007 | 0.532 | 0.439 |
A lightweight CNN-based knowledge graph embedding (KGE) model with channel attention called IntSE is proposed in this paper. Although CNN-based KGE models attract more attention from the research and achieve higher LP accuracy than other KGE models, they often contain too many parameters and have very low efficiency. We do our utmost to explore the balance between the efficiency and effectiveness of the CNN-based KGE model and propose IntSE through extensive experiments. The key idea of IntSE is to increase the favorable feature interactions between the entities and relations in a triple of KG. Checkered feature reshaping and circular convolution operations increase the feature interactions, and the channel attention further enhances useful feature interactions in IntSE. The ablation studies are carried out to explore the effectiveness of each component on the IntSE for LP. Especially the impacts of different channel attention mechanisms (i.e., SENet, SKNet, and ECA-Net) on IntSE are investigated. Compared with the state-of-the-art CNN-based KGE models, IntSE mostly achieved the best performance under various evaluation criteria on public datasets. These extensive experimental results substantiate the efficiency and effectiveness of our model.
In future work, we would like to explore whether other improved versions of SENet can further improve the performance of InteractE and investigate whether incorporating the spatial attention module into IntSE can boost its performance. In addition, it would also be interesting to consider how to improve the performance of KGE models for other downstream tasks, e.g., question answering [6], recommendation [7], and entity resolution [31].
This work was supported by the National Natural Science Foundation of China under Grant (No. 61976032 and No. 62002039), the Fundamental Research Funds for the Central Universities (No. 3132022261 and No. 3132022634).
The authors declare that they have no known competing financial interests or personal relationships that could have appeared to influence the work reported in this paper.
[1] | M. Egmont-Petersen, D. De Ridder and H. Handels, Image processing with neural networks a review, Pattern Recogn., 35 (2002), 2279–2301. |
[2] | D. De Didder, R. P. Duin, M. Egmont-Petersen, et al., Nonlinear image processing using artificial neural networks, . Imag. Elect. Phys., 126 (2003), 352–450. |
[3] | C. J. Schuler, M. Hirsch, S. Harmeling, et al., IEEE T. Pattern Anal., 38 (2016), 1439–1451. |
[4] | A. Levin, Blind motion deblurring using image statistics, Proc. Adv. Neural Infor. Process. Sys., (2006), 841–848. |
[5] | A. Goldstein and R. Fattal, Blur-kernel estimation from spectral irregularities, European Conference on Computer Vision, (2012), 622–635. |
[6] | W. Hu, J. Xue and N. Zheng, PSF estimation via gradient domain correlation, IEEE T. Image Process., 21 (2012), 386–392. |
[7] | H. Y. Huang and W. C. Tsai, Blurred image restoration using fast blur-kernel estimation, 10th International Conference on Intelligent Information Hiding and Multimedia Signal Processing, (2014), 435–438. |
[8] | Y. Liao, W. Li, J. Cui, et al., Blur kernel estimation model with combined constraints for blind image deblurring, Digital Image Computing: Techniques and Applications, (2018), 1–8. |
[9] | W. H. Richardson, Bayesian-based iterative method of image restoration, J. Opt. Soc. Am., 62 (1972), 55–59. |
[10] | Y. W. Tai, P. Tan and M. S. Brown, Richardson-Lucy deblurring for scenes under a projective motion path, IEEE T. Pattern Anal., 33 (2011), 1603–1618. |
[11] | H. L. Yang, P. H. Huang and S. H. Lai, A novel gradient attenuation Richardson-lucy algorithm for image motion deblurring, Signal Process., 103 (2014), 399–414. |
[12] | M. Welk, P. Raudaschl, T. Schwarzbauer, et al., Fast and robust linear motion deblurring, Signal Image Video P., 9 (2015), 1221–1234. |
[13] | M. Dobeˇs, L. Machala and T. F¨urst, Blurred image restoration:A fast method of finding the motion length and angle, Digit. Signal Process., 20 (2010), 1677–1686. |
[14] | G. Liu, S. Chang and Y. Ma, Blind image deblurring using spectralproperties of convolution operators, IEEE T. Image Process., 23 (2014), 5047–5056. |
[15] | T. Yue, S. Cho, J. Wang, et al., Hybrid image deblurring by fusing edge and power spectrum information, Process of European Conference on Computer Vision, (2014). |
[16] | A. M. Deshpande and S. Patnaik, Single image motion deblurring: An accurate PSF estimation and ringing reduction, Optik, 125 (2014), 3612–3618. |
[17] | W. A. Shao, Q. Ge, H. S. Deng, et al., Motion deblurring using non-stationary image modeling, J. Math. Imaging Vis., 52 (2015), 234–248. |
[18] | N. He, K. Lu, B. K. Bao, et al., Single-image motion deblurring using an adaptive image prior, Inform. Science, 281 (2015), 736–749. |
[19] | J. Jia, Single image motion deblurring using transparency, Process of IEEE Conference on Computer Vision and Pattern Recognition, (2007), 1–7. |
[20] | A. Levin, Y. Weiss, F. Durand, et al., Understanding and evaluating blind deconvolution algorithms, Process of IEEE Conference on Computer Vision and Pattern Recognition, (2009), 1964– 1971. |
[21] | A. Levin, Y. Weiss, F. Durand, et al., Understanding blind deconvolution algorithms, IEEE T. Pattern Anal., 33 (2011), 2354–2367. |
[22] | L. Xu, S. Zheng and J. Jia, Unnatural L0 sparse representation for natural image deblurring, Process of IEEE Conference on Computer Vision and Pattern Recognition, (2013). |
[23] | T. S. Cho, C. Paris, B. K. P. Horn, et al., Blur kernel estimation using the Radon transform, Process of IEEE Conference on Computer Vision and Pattern Recognition, (2011), 241–248. |
[24] | D. Krishnan, T. Tay and R. Fergus, Blind deconvolution using a normalized sparsity measure, Process of IEEE Conference on Computer Vision and Pattern Recognition, (2011), 223–240. |
[25] | M. Ljubenovi´c and M. A. T. Figueiredo, Blind image deblurring using class-adapted image priors, Process of IEEE Conference on Image Processing, (2017), 490–494. |
[26] | J. Pan, D. Sun, H. Pfister, et al., Deblurring images via dark channel prior, IEEE T. Pattern Anal., 10 (2018), 2315–2328. |
[27] | D. J. Field, Relations between the statistics of natural images and the response properties of cortical cells, J. Opt. Soc. Am. A, 4 (1987), 2379–2394. |
[28] | G. J. Burton and I. R. Moorhead, Color and spatial structure in natural scenes, Appl. Optics, 26 (1987), 157–170. |
[29] | L. Cohen, The generalizaton of the Wiener-Khinchin theorem, IEEE Signal Proc. Let., 5 (1998), 292–294. |
[30] | J. R. Fienup, Phase retreival algorithms: A comparsion, Appl. Opt., 21 (1982), 2758–2769. |
[31] | S. Watanabe, H. Shioya and K. Gohara, Phase retrieval based on an evolution multicriterion optimisation method, Process of IEEE Conference on Evolutionary Computation, (2012), 1–8. |
[32] | Z. Wang, A. C. Bovik, H. R. Sheikh, et al., Image quality assessment: from error visibility to structural similarity, Process of IEEE Conference on Image Processing, 13 (2004), 600–612. |
[33] | M. Sonka, V. Hlavac and Roger Boyle, Image processing, Analysis, and Machine Vision, 3rd Ed., Thomson Learning Pub., 2008. |
[34] | D. Krishnan and R. Fergus, Fast image deconvolution using hyper-Laplacian priors, Proc. Conf. NIPS, (2009), 1033–1041. |
1. | Panfei Yin, Erping Zhao, , A knowledge graph completion model based on weighted fusion description information and transform of the dimension and the scale, 2025, 55, 0924-669X, 10.1007/s10489-025-06230-w | |
2. | Qiang Yu, Liang Bao, Peng Nie, Lei Zuo, Positionally Restricted Masked Knowledge Graph Completion via Multi-Head Mutual Attention, 2025, 29497159, 10.1016/j.jiixd.2025.02.006 |
Model | fScoring Function ψ(es,er,eo) |
ConvE [20] | f(vec(f([¯es;¯er]⋆ω))W)eo |
Conv-TransE [22] | g(vec(f([es;er]⋆ω))W)eo |
ConvKB [21] | concat(g([es;er;eo]⋆ω)w) |
InteractE [23] | g(vec(f(ϕchk(Pk(es,er))♣ω))W)eo |
DMACM [24] | concat(f(DM(es,er,eo))⋆ω)W |
KMAE [25] | g(Mul([kernel(¯es);¯er]||[¯es;kernel(¯er)]))eo |
JointE [26] | f([es;er]⋆ω11D⋆ω21D+vec([¯es⋆ωr;¯er⋆ωs])W)eo |
IntSE | g(vec(ca(f(ϕchk(es,er)♣ω)))W)eo |
Dataset | #entities | #relations | #train | #valid | #test |
FB15k-237 | 14,541 | 237 | 272,115 | 17,535 | 20,466 |
WN18RR | 40,943 | 11 | 86,835 | 3034 | 3134 |
Model | FB15k-237 | WN18RR | ||||||
MRR | MR | Hits@10 | Hits@1 | MRR | MR | Hits@10 | Hits@1 | |
ConvE [20] | 0.325 | 244 | 0.501 | 0.237 | 0.430 | 4187 | 0.520 | 0.400 |
Conv-TransE [22] | 0.330 | - | 0.510 | 0.240 | 0.460 | - | 0.520 | 0.430 |
InteractE [23] | 0.354 | 172 | 0.535 | 0.263 | 0.463 | 5202 | 0.528 | 0.430 |
DMACM [24] | 0.270 | 244 | 0.440 | - | 0.230 | 552 | 0.540 | - |
KMAE [25] | 0.326 | 235 | 0.502 | 0.240 | 0.448 | 4441 | 0.524 | 0.415 |
JointE [26] | 0.356 | 177 | 0.543 | 0.262 | 0.471 | 4655 | 0.537 | 0.438 |
IntSE | 0.359 | 179 | 0.540 | 0.267 | 0.469 | 5007 | 0.532 | 0.439 |
ConvE [20] | InteractE [23] | IntSE | |||||
MRR | Hits@10 | MRR | Hits@10 | MRR | Hits@10 | ||
Head Pred | 1-to-1 | 0.374 | 0.505 | 0.386 | 0.547 | 0.391 | 0.561 |
1-to-n | 0.091 | 0.170 | 0.106 | 0.192 | 0.112 | 0.198 | |
n-to-1 | 0.444 | 0.644 | 0.466 | 0.647 | 0.472 | 0.652 | |
n-to-m | 0.261 | 0.459 | 0.276 | 0.476 | 0.285 | 0.479 | |
Tail Pred | 1-to-1 | 0.366 | 0.510 | 0.368 | 0.547 | 0.372 | 0.550 |
1-to-n | 0.762 | 0.878 | 0.777 | 0.881 | 0.780 | 0.888 | |
n-to-1 | 0.069 | 0.150 | 0.074 | 0.141 | 0.079 | 0.152 | |
n-to-m | 0.375 | 0.603 | 0.395 | 0.617 | 0.400 | 0.621 |
InteractE [23] | IntSE | |||||
perm = 1 | perm = 2 | perm = 3 | perm = 1 | perm = 2 | perm = 3 | |
MRR | 0.353 | 0.353 | 0.349 | 0.354 | 0.353 | 0.350 |
Hits@10 | 0.537 | 0.534 | 0.533 | 0.539 | 0.537 | 0.535 |
Hits@20 | 0.619 | 0.616 | 0.613 | 0.620 | 0.615 | 0.614 |
Hits@40 | 0.694 | 0.692 | 0.688 | 0.695 | 0.691 | 0.688 |
Hits@80 | 0.764 | 0.761 | 0.759 | 0.766 | 0.763 | 0.758 |
Hits@160 | 0.829 | 0.824 | 0.822 | 0.830 | 0.827 | 0.822 |
Model | MRR | MR | Hits@10 | Hits@1 |
sr+sc (ConvE) | 0.325 | 244 | 0.501 | 0.237 |
sr+sc+ca | 0.342 | 181 | 0.525 | 0.251 |
cr+sc | 0.338 | 185 | 0.519 | 0.249 |
cr+sc+ca | 0.350 | 193 | 0.536 | 0.262 |
cr+cc | 0.346 | 175 | 0.532 | 0.258 |
cr+cc+ca (IntSE) | 0.359 | 179 | 0.540 | 0.267 |
Model | MRR | MR | Hits@10 | Hits@1 |
IntSE-SENet | 0.358 | 183 | 0.538 | 0.266 |
IntSE-SKNet | 0.350 | 193 | 0.527 | 0.262 |
IntSE-ECANet | 0.346 | 223 | 0.529 | 0.250 |
IntSE-ECANet' | 0.355 | 184 | 0.536 | 0.263 |
Model | MRR | MR | Hits@10 | Hits@1 |
IntSE-SENet | 0.466 | 5123 | 0.530 | 0.434 |
IntSE | 0.469 | 5007 | 0.532 | 0.439 |
Model | fScoring Function ψ(es,er,eo) |
ConvE [20] | f(vec(f([¯es;¯er]⋆ω))W)eo |
Conv-TransE [22] | g(vec(f([es;er]⋆ω))W)eo |
ConvKB [21] | concat(g([es;er;eo]⋆ω)w) |
InteractE [23] | g(vec(f(ϕchk(Pk(es,er))♣ω))W)eo |
DMACM [24] | concat(f(DM(es,er,eo))⋆ω)W |
KMAE [25] | g(Mul([kernel(¯es);¯er]||[¯es;kernel(¯er)]))eo |
JointE [26] | f([es;er]⋆ω11D⋆ω21D+vec([¯es⋆ωr;¯er⋆ωs])W)eo |
IntSE | g(vec(ca(f(ϕchk(es,er)♣ω)))W)eo |
Dataset | #entities | #relations | #train | #valid | #test |
FB15k-237 | 14,541 | 237 | 272,115 | 17,535 | 20,466 |
WN18RR | 40,943 | 11 | 86,835 | 3034 | 3134 |
Model | FB15k-237 | WN18RR | ||||||
MRR | MR | Hits@10 | Hits@1 | MRR | MR | Hits@10 | Hits@1 | |
ConvE [20] | 0.325 | 244 | 0.501 | 0.237 | 0.430 | 4187 | 0.520 | 0.400 |
Conv-TransE [22] | 0.330 | - | 0.510 | 0.240 | 0.460 | - | 0.520 | 0.430 |
InteractE [23] | 0.354 | 172 | 0.535 | 0.263 | 0.463 | 5202 | 0.528 | 0.430 |
DMACM [24] | 0.270 | 244 | 0.440 | - | 0.230 | 552 | 0.540 | - |
KMAE [25] | 0.326 | 235 | 0.502 | 0.240 | 0.448 | 4441 | 0.524 | 0.415 |
JointE [26] | 0.356 | 177 | 0.543 | 0.262 | 0.471 | 4655 | 0.537 | 0.438 |
IntSE | 0.359 | 179 | 0.540 | 0.267 | 0.469 | 5007 | 0.532 | 0.439 |
ConvE [20] | InteractE [23] | IntSE | |||||
MRR | Hits@10 | MRR | Hits@10 | MRR | Hits@10 | ||
Head Pred | 1-to-1 | 0.374 | 0.505 | 0.386 | 0.547 | 0.391 | 0.561 |
1-to-n | 0.091 | 0.170 | 0.106 | 0.192 | 0.112 | 0.198 | |
n-to-1 | 0.444 | 0.644 | 0.466 | 0.647 | 0.472 | 0.652 | |
n-to-m | 0.261 | 0.459 | 0.276 | 0.476 | 0.285 | 0.479 | |
Tail Pred | 1-to-1 | 0.366 | 0.510 | 0.368 | 0.547 | 0.372 | 0.550 |
1-to-n | 0.762 | 0.878 | 0.777 | 0.881 | 0.780 | 0.888 | |
n-to-1 | 0.069 | 0.150 | 0.074 | 0.141 | 0.079 | 0.152 | |
n-to-m | 0.375 | 0.603 | 0.395 | 0.617 | 0.400 | 0.621 |
InteractE [23] | IntSE | |||||
perm = 1 | perm = 2 | perm = 3 | perm = 1 | perm = 2 | perm = 3 | |
MRR | 0.353 | 0.353 | 0.349 | 0.354 | 0.353 | 0.350 |
Hits@10 | 0.537 | 0.534 | 0.533 | 0.539 | 0.537 | 0.535 |
Hits@20 | 0.619 | 0.616 | 0.613 | 0.620 | 0.615 | 0.614 |
Hits@40 | 0.694 | 0.692 | 0.688 | 0.695 | 0.691 | 0.688 |
Hits@80 | 0.764 | 0.761 | 0.759 | 0.766 | 0.763 | 0.758 |
Hits@160 | 0.829 | 0.824 | 0.822 | 0.830 | 0.827 | 0.822 |
Model | ConvE [20] | InteractE [23] | IntSE | |||
perm = 1 | perm = 2 | perm = 3 | perm = 4 | |||
Parameters | 4.96M | 10.7M | 18.38M | 26.07M | 33.75M | 10.7M |
Model | MRR | MR | Hits@10 | Hits@1 |
sr+sc (ConvE) | 0.325 | 244 | 0.501 | 0.237 |
sr+sc+ca | 0.342 | 181 | 0.525 | 0.251 |
cr+sc | 0.338 | 185 | 0.519 | 0.249 |
cr+sc+ca | 0.350 | 193 | 0.536 | 0.262 |
cr+cc | 0.346 | 175 | 0.532 | 0.258 |
cr+cc+ca (IntSE) | 0.359 | 179 | 0.540 | 0.267 |
Model | MRR | MR | Hits@10 | Hits@1 |
IntSE-SENet | 0.358 | 183 | 0.538 | 0.266 |
IntSE-SKNet | 0.350 | 193 | 0.527 | 0.262 |
IntSE-ECANet | 0.346 | 223 | 0.529 | 0.250 |
IntSE-ECANet' | 0.355 | 184 | 0.536 | 0.263 |
Model | MRR | MR | Hits@10 | Hits@1 |
IntSE-SENet | 0.466 | 5123 | 0.530 | 0.434 |
IntSE | 0.469 | 5007 | 0.532 | 0.439 |