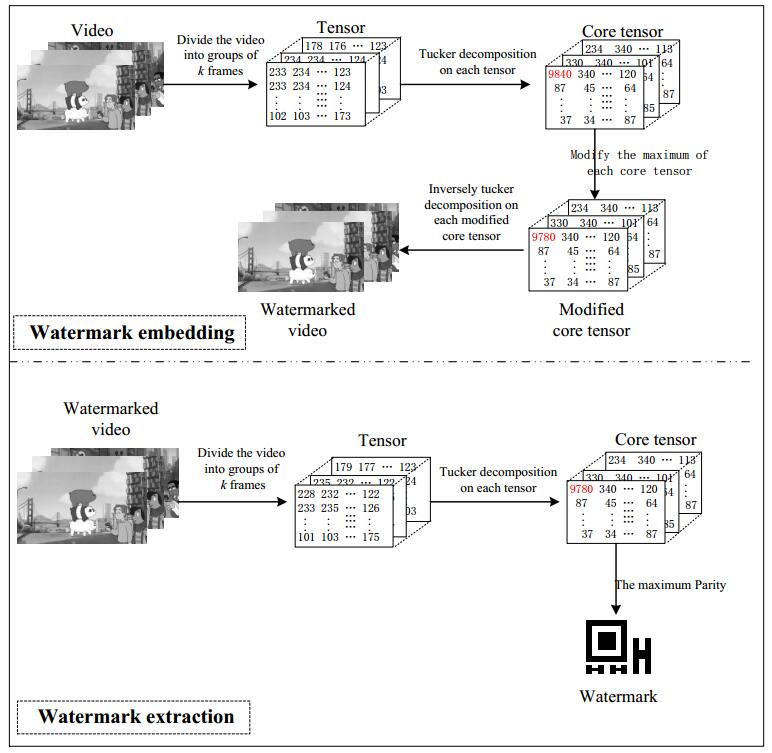
Citation: Shanqing Zhang, Xiaoyun Guo, Xianghua Xu, Li Li, Chin-Chen Chang. A video watermark algorithm based on tensor decomposition[J]. Mathematical Biosciences and Engineering, 2019, 16(5): 3435-3449. doi: 10.3934/mbe.2019172
[1] | Iman Mousavian, Mohammad Bagher Shamsollahi, Emad Fatemizadeh . Noninvasive fetal ECG extraction using doubly constrained block-term decomposition. Mathematical Biosciences and Engineering, 2020, 17(1): 144-159. doi: 10.3934/mbe.2020008 |
[2] | Kaiyin Zhou, YuxingWang, Sheng Zhang, Mina Gachloo, Jin-Dong Kim, Qi Luo, Kevin Bretonnel Cohen, Jingbo Xia . GOF/LOF knowledge inference with tensor decomposition in support of high order link discovery for gene, mutation and disease. Mathematical Biosciences and Engineering, 2019, 16(3): 1376-1391. doi: 10.3934/mbe.2019067 |
[3] | Qiuling Wu, Dandan Huang, Jiangchun Wei, Wenhui Chen . Adaptive and blind audio watermarking algorithm based on dither modulation and butterfly optimization algorithm. Mathematical Biosciences and Engineering, 2023, 20(6): 11482-11501. doi: 10.3934/mbe.2023509 |
[4] | Hongyan Xu . Digital media zero watermark copyright protection algorithm based on embedded intelligent edge computing detection. Mathematical Biosciences and Engineering, 2021, 18(5): 6771-6789. doi: 10.3934/mbe.2021336 |
[5] | Wenfa Qi, Wei Guo, Tong Zhang, Yuxin Liu, Zongming Guo, Xifeng Fang . Robust authentication for paper-based text documents based on text watermarking technology. Mathematical Biosciences and Engineering, 2019, 16(4): 2233-2249. doi: 10.3934/mbe.2019110 |
[6] | Chuanda Cai, Changgen Peng, Jin Niu, Weijie Tan, Hanlin Tang . Low distortion reversible database watermarking based on hybrid intelligent algorithm. Mathematical Biosciences and Engineering, 2023, 20(12): 21315-21336. doi: 10.3934/mbe.2023943 |
[7] | Shaozhang Xiao, Xingyuan Zuo, Zhengwei Zhang, Fenfen Li . Large-capacity reversible image watermarking based on improved DE. Mathematical Biosciences and Engineering, 2022, 19(2): 1108-1127. doi: 10.3934/mbe.2022051 |
[8] | Qichao Ying, Jingzhi Lin, Zhenxing Qian, Haisheng Xu, Xinpeng Zhang . Robust digital watermarking for color images in combined DFT and DT-CWT domains. Mathematical Biosciences and Engineering, 2019, 16(5): 4788-4801. doi: 10.3934/mbe.2019241 |
[9] | Yan Li, Junwei Wang, Shuangkui Ge, Xiangyang Luo, Bo Wang . A reversible database watermarking method with low distortion. Mathematical Biosciences and Engineering, 2019, 16(5): 4053-4068. doi: 10.3934/mbe.2019200 |
[10] | Kun Zheng, Junjie Shen, Guangmin Sun, Hui Li, Yu Li . Shielding facial physiological information in video. Mathematical Biosciences and Engineering, 2022, 19(5): 5153-5168. doi: 10.3934/mbe.2022241 |
Copyright protection is more and more important as digital videos become popular. Video watermark technology is the digital watermark technology with video being the carrier. It embeds confidential information into carrier based on the video's redundancy. Video watermark technology is invisible, able to resist malicious attacks, and has achieved video copyright protection or video content authentication [1].
Video watermark technology is classified into spatial domain watermark technology and transform domain watermark technology. Kalker [2] introduced spread spectrum into video watermark and proposed a classic video watermark algorithm for video broadcast monitoring. The algorithm regards a video as a sequence of images and embeds the watermark into the video frames in the spatial domain. The algorithm works well with broadcast transmission signal processing and produces a detection process with low complexity. But it is not robust against common attacks. The spatial video watermark algorithm proposed by Hartung [3] converts the original video image into a one-dimensional signal and modulates the watermark into a pseudo-random sequence. This watermark is embedded into the one-dimensional signal. This classical spatial domain algorithm has disadvantages in robustness against attacks such as video compression and filtering. Karybali [4] proposed an effective spatial watermark algorithm to improve robustness by perceptual masking and watermark blind extraction.
A spatial watermark algorithm directly modifies pixels of image in spatial domain. The advantages are good transparency and low complexity, whereas one main disadvantage is watermark loss after image compression or geometric attack [5,6,7]. A transform domain watermark algorithm transforms an image into a domain by Discrete Wavelet Transform (DWT), Discrete Fourier Transform (DFT), or Discrete Cosine Transform (DCT), and so forth. Watermark embedding is performed after the transform. The transform domain watermark technology is robust against attacks such as filtering and image compression. In 1995, Koch proposed a watermark algorithm in DCT domain, which is robust against compression and filtering attacks [8]. In 1997, Cox [9] summarized and analyzed the existing transform domain watermark algorithms and proposed to embed watermark into the low frequency coefficients of an image. The robustness of the watermark was effectively improved. In order to further improve anti-attack capability, Chandra [10] first used Singular Value Decomposition (SVD) for digital watermark in 2001, and embedded watermark image into singular value of the carrier image. The digital watermark algorithms with SVD can improve anti-attach capability, especially against geometric attacks.
Most video watermark algorithms so far regard a video as a series of consecutive images and embed watermark into the images. However, the correlation and redundancy among the frames of a video are not considered. They are not robust against frame attacks such as frame addition, frame deletion, or frame averaging.
We introduce tensor into video watermark in order to solve the above problems. A tensor is a multi-dimensional array and has advantages in representing multi-dimensional data. Tensor computation has been successfully used in face recognition [11], visual tracking [12] and action classification [13]. Tensor decomposition [14,15,16] has become a landmark in video-related research. However, published studies on tensor-based video watermark are rare [17,18]. In [17], Abdallah proposed a tensor-based video watermarking algorithm, but it is non-blind. Recently, Xu [18] represented a color image as a third-order tensor, and proposed a robust watermark algorithm, but that is only suitable for color image. In this study, we represented a grayscale video as a 3-order tensor and proposed a blind video watermark algorithm based on tensor decomposition. The flow chart of our algorithm is shown in Figure 1. The core tensor is obtained by Tucker decomposition of the 3-order tensor. The watermark is embedded by parity quantization [19] of the maximum value of core tensor. The modified core tensor is uniformly distributed across frames of a video by inverse Tucker decomposition. The watermark extraction is simply the inverse process of embedding. Experiments show that our algorithm is robust against common video attacks and imperceptible.
The main contributions of this study are as follows:
(1) We introduce tensor into video watermark. The correlation and redundancy among the frames of a video are considered to enhance the robustness against frame attacks.
(2) The stability of the algorithm is guaranteed because Tucker decomposition is reversible, and the core tensor represents the main energy of the original video and is relatively invariant.
(3) The modified core tensor is uniformly distributed among the frames of a video by inverse Tucker decomposition, so that the video quality and the imperceptibility of watermark are guaranteed.
This paper is organized as follows. The basics of tensor are introduced in Section 2, our watermark embedding and extraction algorithm is described in Section 3, and the experiment results are shown in Section 4.
The next notations are used.
Scalar: (a, b, etc.); Vector: (a, b, etc.); Matrix: (A, B, etc.); High-order tensor: (A,B,etc.).
A tensor is a high-order matrix, an extended form of a matrix toward the higher dimension. Vector and matrix are first-order tensor and second-order tensor, respectively. A N-order tensor A is defined as A∈RI1×⋅⋅⋅×IN. A video sequence can be regarded as a 3-order tensor, and its three dimensions are the width, height and length of the video.
Tensor has many advantages in representing multi-dimension data. For example, if a video is considered as a tensor, the properties of the original video can be preserved to the maximum extent. However, high-order tensor results in higher-level computation. So, tensor is usually unfolded to matrices for easy computation [20].
A is N-order tensor A∈RI1×⋅⋅⋅×IN, tensor A is unfolded into matrices A1,⋅⋅⋅,AN, Ak∈RIk×(I1×⋅⋅⋅×Ik−1×Ik+1×⋅⋅⋅IN). The unfolding of a 3-order tensor is shown in Figure 2.
The mode-n product of a N-order tensor A∈RI1×⋅⋅⋅×IN and a matrix U∈RJ×In is noted as A×nU, where ×nU∈RI1×⋅⋅⋅×In−1×J×In+1×⋅⋅⋅×IN, the entries are given by:
[(A×nU)i1×⋅⋅⋅in−1×j×in+1×⋅⋅⋅×iN=∑Inin=1ai1⋅⋅⋅iNujin] | (1) |
The mode-1 product of a 3-order tensor and a matrix is shown in Figure 3.
Tensor decomposition is generalization of matrix singular value decomposition in high dimensions [21]. An image F with size I1×I2 can be decomposed by SVD as follows:
[FI1×I2=UI1×I1⋅SI1×I2⋅VTI2×I2] | (2) |
where U and V are the left and right singular matrices of F, respectively. S is the diagonal matrix composed of the singular values of F.
A matrix is regarded as a 2-order tensor. According to the definition of mode product of tensor and matrix, the singular value decomposition SVD of a matrix can be represented as a 2-order tensor S mode product of a matrix U1 and a matrix U(2) sequentially:
[F=S×1U(1)×2U(2)] | (3) |
with
[F=U(1)⋅D1⋅VT1] | (4) |
[FT=U(2)⋅D2⋅VT2] | (5) |
For a tensor of high-order (k > 2), CP(CANDECOMP/PARAFAC)decomposition [22] and Tucker decomposition [23] are often used. CP decomposes a tensor into a finite sum of rank-1 tensors. CP guarantees the uniqueness of the decomposition result, but its rank solution is an NP problem. Tensor is decomposed into the mode product of the core tensor and factor matrices by Tucker decomposition. The core tensor contains the main information of the original tensor. Tucker decomposition is used in our study. High-Order Singular Value Decomposition (HOSVD) is the classic algorithm for Tucker decomposition. Given a tensor A with size M×N×K, Tucker decomposition with HOSVD [24] is as follows:
[A=S×1U×2V×3W] | (6) |
with
[A1=U⋅D1⋅VT1] | (7) |
[A2=V⋅D2⋅VT2] | (8) |
[A3=W⋅D3⋅VT3] | (9) |
where A1,A2,A3 are the unfolding matrices of A in three directions, respectively. And the core tensor S writes:
[S=A×1UT×2VT×3WT] | (10) |
Tucker decomposition of a 3-order tensor is shown in Figure 4, where 1≤R1≤M, 1≤R2≤N, 1≤R3≤K.
In our watermark algorithm, a video is initially represented as a tensor, which contains the relevance and redundancy among the frames of a video. Then, a core tensor is obtained by Tucker decomposition. Finally, a watermark is embedded into the core tensor by parity quantization.
The resolution of a video V is M×N, and the size of a watermark B is m×m. To make full use of the relevance and redundancy among the frames of a video, K frames of a grayscale video are grouped as a 3-order tensor. The size of tensor Ai(1≤i≤m2) is M×N×K. The core tensor Si(1≤i≤m2) and 3 factor matrices Ui,Vi,Wi are obtained through Tucker decomposition with HOSVD. The process of watermark embedding is as follows.
(1) Arnold Scrambling. In order to eliminate spatial correlation among the binary watermark pixels, B becomes B' through Arnold transformation. It can be defined by the following equation:
[(x′y′)=[(1abab+1)⋅(xy)]mod(m)] | (11) |
where (x, y) is the coordinate of the original watermark pixel, (x', y') is the transformed coordinate of (x, y) with Arnold, and m is the width of the matrix. In the experiment, a = 1 and b = 1. The Arnold transformation is performed for t times on the original watermark, and t is saved as a key for watermark extraction.
(2) Tucker decomposition with HOSVD. Tucker decomposition is performed for each Ai(1≤i≤m2) to obtain the core tensor Si.
[Si=Ai×1UTi×2VTi×3WTi] | (12) |
where Ai∈RM×N×K is the original video tensor, Ui∈RM×M, Vi∈RN×N, Wi∈RK×K are factor matrices.
(3) Quantification and modification of the core tensor. Parity quantization is used to embed watermark into the core tensor. For each tensor Ai, Si(1,1,1) is the maximum value of the core tensor Si, and is donated as σi.
a. Quantify the maximum value σi of each core tensor, denoted as λi=round(σiQ), where Q is the quantization intensity, and the value of Q is discussed below.
b. The maximum value of each core tensor Si is modified to embed watermark.
[σ′i={(λi+0.5)Qif(λi+B′i)isodd(λi−0.5)Qif(λi+B′i)iseven] | (13) |
(4) Reconstruction of the watermarked video. The watermarked video A′i is reconstructed by inverse Tucker decomposition with the modified core tensor S′i.
[A′i=S′i×1Ui×2Vi×3Wi] | (14) |
Watermark extraction is the inverse process of watermark embedding. The specific steps of watermark extraction are as follows.
(1) Tucker decomposition is performed on each watermarked video tensor A′i to obtain the core tensor S′i.
[S′i=A′i×1U′Ti×2V′Ti×3W′Ti] | (15) |
(2) The extracted watermark is determined according to the maximum value of the core tensor S′i.
a. Quantify the maximum value σ′i of each core tensor S′i, denoted as λ′i=floor(σ′iQ).
b. Determine the extracted information according to the parity of λ′i. B′i is 1 when λ′i is even; B′i is 0 when λ′i is odd.
(3) Perform inverse Arnold transformation on B' to obtain the original watermark B.
The follow metrics are used to measure the robustness and imperceptibility of our video watermark algorithm based on tensor decomposition. The imperceptibility of the watermark is evaluated with Peak Signal to Noise Ration (PSNR) and Mean Square Error (MSE).
[MSE=1MN∑Mi=1∑Nj=1|I(i,j)−I′(i,j)|2] | (16) |
where M and N are the height and width of a single-frame image, I and I' are the original video frame and watermarked video frame. The smaller the MSE value, the smaller the difference between the single-frame watermark image and the original image is. PSNR is calculated by MSE as follow:
[PSNR=10log10(2552MSE)] | (17) |
A smaller PSNR means that the distortion of the watermarked frame is more serious. In addition, the bit error rate (BER) and normalized correlation coefficient (NC) are used to evaluate the robustness of the watermark. The equations are as follows:
[BER=∑mi=1∑mj=1|B′(i,j)−B(i,j)|m×m] | (18) |
[NC=∑mi=1∑mj=1(B′(i,j)⋅B(i,j))√∑mi=1∑mj=1(B(i,j))2√∑mi=1∑mj=1(B′(i,j))2] | (19) |
where m is the size of the watermark, B and B' are the original watermark and extracted watermark, respectively. The robustness of watermark increases as NC increases.
The size of the test video is 352 × 640 and there are 2268 frames in total. The size of the watermark is 18 × 18. A bit of watermark is embedded into a group of K frames. The size of the tensor is 352 × 640 × K, K being 7 in our experiment. The number of scrambling t is 15. The relationship between quantization strength Q and watermark BER is shown in Figure 5. BER decreases as Q increases. The watermark is correctly extracted when Q≥1000. Q is set to 2000 in order to ensure the robustness of the algorithm and video quality. The PSNR of the first 100 frames of a video when Q = 2000 is shown in Figure 6. The PSNR of the watermarked video is over 40dB. The examples of our algorithm are shown in Figure 7.
Different attacks are used to testify the robustness of our watermark algorithm, including frame swapping, zooming, cropping, filtering, noising, and black-border filling. Our tensor-based watermark algorithm is robust against frame attacks, zooming, rotation, cropping in the experiments. The NC of the extracted watermark through the frames swapping in a group is shown in Table 1. The NC of the extracted watermark remains high even if about 50% frames in a group are swapped. The modified maximum value of the core tensor has uniform effect on each frame, because every element in the core tensor takes part in the mode product of the factor matrices by inverse Tucker decomposition.
Number of frames replaced | Extracted watermark | NC |
1 | ![]() |
1 |
2 | ![]() |
1 |
3 | ![]() |
1 |
4 | ![]() |
0.8055 |
The relation between the video's zooming and NC of the extracted watermark is shown in Figure 8. Watermark is extracted irrespective of a video being zoomed in or out as long as the video is restored at the same resolution. The NC of the extracted watermark is as high as 0.8098 even when the video is zoom out to 0.1 of its original size.
Watermark is extracted correctly when black-border is filled around a borderless video because the core tensor represents the main energy of a video and the energy from these zero-valued pixels in a video is tiny. The result is shown in Figure 9.
The watermark is also extracted correctly as long as the direction of the video remains unchanged. The relationship between the rotation angle and the maximum value of the core tensor is shown in Figure 10. The maximum value of the core tensor changes periodically as the rotation angle changes. The watermark is extracted correctly from the watermarked video after rotation correction [25,26]. Some experiment results for rotation attack are shown in Table 2.
Rotation angle | Rotated video | Corrected video | Extracted watermark | NC |
100 | ![]() |
![]() |
![]() |
1 |
450 | ![]() |
![]() |
![]() |
1 |
600 | ![]() |
![]() |
![]() |
1 |
The experiment results of black-border video by our algorithm are shown in Table 3. Watermark is extracted correctly irrespective of the cropping being in the up or down, left or right side when the cropped part is included in a black-boarder because the contribution on the maximum value of the core tensor from black-border (0-value pixels) is very small. The watermark extraction of filtering and noise attacks is shown in Table 4.
Cropping attack | Attacked video | Extracted watermark | NC |
No attack | ![]() |
![]() |
1 |
Cut 45 rows at the top | ![]() |
![]() |
1 |
Cut 45 rows on the left | ![]() |
![]() |
1 |
Attack type | Extracted watermark | NC |
Median filtering (3 × 3) | ![]() |
0.9587 |
Mean filtering (3 × 3) | ![]() |
0.9973 |
Gaussian noise (0, 0.01) | ![]() |
0.8675 |
Salt and pepper noise (0.01) | ![]() |
0.9973 |
Poisson noise | ![]() |
0.9783 |
A grayscale video is represented as a 3-order tensor in order to make full use of the relevance and redundancy among the frames of a video. The core tensor is obtained by Tucker decomposition, and the embedding and extraction of the video watermark is achieved using parity quantization of the maximum value of the core tensor. Watermark information is uniformly distributed across the frames of a video because of the reversibility and stability of Tucker decomposition, so that the video quality and the imperceptibility of watermark are guaranteed. It is robust against various video attacks, especially frame attacks. In our algorithm, only grayscale videos are used, color video watermarking method based on the tensor domain will be studied in the future.
This research was funded by the Public Welfare Technology and Industry Project of Zhejiang Provincial Science Technology Department (Grant No. LGG19F020016 and No. LGG18F020013) and the Key Research and Development Project of Zhejiang Province (Grant No. 2017C01022).
The authors declare no conflicts of interest.
[1] | A. H. Tewfik, Digital Watermarking, IEEE Signal Processing Magazine, 17 (2000), 17–18. |
[2] | T. Kalker, G. Depovere and J. Haitsma, Video watermarking system for broadcast monitoring, Secur. Watermark. Multim. Contents, 3657 (1999), 103–112. |
[3] | F. Hartung and B. Girod, Watermarking of uncompressed and compressed video, Elsevier North-Holland, 66 (1998), 283–301. |
[4] | I. G. Karybali and K. Berberidis, Efficient spatial image watermarking via new perceptual masking and blind detection schemes, IEEE Transact. Inform. Foren. Secur., 1 (2006), 256–274. |
[5] | M. J. Lee, K. S. Kim and H. K. Lee, Digital cinema watermarking for estimating the position of the pirate, IEEE Transact. Multim., 12 (2010), 605–621. |
[6] | J. Han and X. Zhao, An adaptive grayscale watermarking method in spatial domain, J. Inform. Comput. Sci., 12 (2015), 4759–4769. |
[7] | S. Wang, D. Zheng and J. Zhao, Adaptive watermarking and tree structure based image quality estimation, IEEE Transact. Multim., 16 (2014), 311–325. |
[8] | Z. Zhou, S. Chen and G. Wang, A robust digital image watermarking algorithm based on dct domain for copyright protection, Int. Symp. Smart Graph., 9317 (2012), 132–142. |
[9] | I. J. Cox and M. L. Miller, A Review of watermarking and the importance of perceptual modeling, Human Vision Electron. Imag. Confer., 3016 (1997), 92–99. |
[10] | D.V. S. Chandra, Digital image watermarking using singular value decomposition, Symposium on Circuits & Systems, USA, 2002. |
[11] | S. Wang, J. Yang and M. Sun, Sparse tensor discriminant color space for face verification, IEEE Transact. Neural Networks Learn. Systems, 23 (2012), 876–888. |
[12] | B. Ma, L. Huang and J. Shen, Discriminative tracking using tensor pooling, IEEE Transact. Cybernet., 46 (2017), 2411–2422. |
[13] | J. Li, X. Mao and X. Wu, Human action recognition based on tensor shape descriptor, IET Computer Vision, 10 (2016), 905–911. |
[14] | A. H. Phan, P. Tichavsky and A. Cichocki, CANDECOMP/PARAFAC decomposition of high-order tensors through tensor reshaping, IEEE Transact. Signal Process., 61 (2013), 4847–4860. |
[15] | A. S. Jermyn, Efficient tree decomposition of high-rank tensors, J. Comput. Phys., 377 (2018), 142–154. |
[16] | N. Sidiropoulos, L. L. De and X. Fu, Tensor Decomposition for Signal Processing and Machine Learning, IEEE Transact. Signal Process., 65 (2017), 3551–3582. |
[17] | E. E. Abdallah, A. B. Hamza and P. Bhattacharya, MPEG Video Watermarking Using Tensor Singular Value Decomposition, Image Analysis & Recognition, Canada, 2007. |
[18] | H. Xu, G. Jiang and M. Yu, A Color Image Watermarking Based on Tensor Analysis, IEEE Access, 6 (2018), 51500–51514. |
[19] | P. Li and W. K. Leung, Decoding low density parity check codes with finite quantization bits, Commu. Lett. IEEE, 4 (2000), 62–64. |
[20] | K. K. Sharma and A. Upadhyay, A novel image fusion technique for gray scale images using tensor unfolding in transform domains, Recent Advances & Innovations in Engineering, India, 2014. |
[21] | R. Costantini, L. Sbaiz and S. Susstrunk, Higher Order SVD Analysis for Dynamic Texture Synthesis, IEEE Transact. Image Process., 17 (2008), 42–52. |
[22] | Y. Wu, H. Tan and Y. Li, A fused CP factorization method for incomplete tensors, IEEE Transact. Neural Networks Learn. Systems, 30 (2018), 751–764. |
[23] | G. Zhou, A. Cichocki and Q. Zhao, Efficient Nonnegative Tucker Decompositions: Algorithms and Uniqueness, IEEE Transact. Image Process., 24 (2015), 4990–5003. |
[24] | A. Rajwade, A. Rangarajan and A. Banerjee, Image Denoising Using the Higher Order Singular Value Decomposition, IEEE Transact. Pattern Anal. Mac. Intell., 35 (2013), 849–862. |
[25] | K. Wang, G. Zhou and G. Fu, An Approach to Fast Eye Location and Face Plane Rotation Correction, J. Computer-Aided Design Comput. Graph., 25 (2013), 865–879. |
[26] | P. F. Checcacci and P. Spalla, Analysis and correction of errors in a polarimeter for Faraday rotation measurements, IEEE Transact. Antennas Propagat., 24 (1976), 253–255. |
1. | Shanqing Zhang, Xiaoyun Guo, Xianghua Xu, Li Li, A video watermark algorithm based on tensor feature map, 2023, 1380-7501, 10.1007/s11042-022-14299-5 | |
2. | Shanqing Zhang, Hui Li, Li Li, Jianfeng Lu, Ching-Chun Chang, A video watermarking algorithm based on time factor matrix, 2023, 82, 1380-7501, 7509, 10.1007/s11042-022-13609-1 | |
3. | Di Fan, Huiyuan Zhao, Changying Zhang, Hongyun Liu, Xiaoming Wang, Anti-Recompression Video Watermarking Algorithm Based on H.264/AVC, 2023, 11, 2227-7390, 2913, 10.3390/math11132913 | |
4. | Jelena Stojanov, Vladimir Balan, Eigenproblem of tensors - a geometrical viewpoint, 2023, 37, 0354-5180, 8603, 10.2298/FIL2325603S | |
5. | Xuru Li, Kun Wang, Xiaoqin Xue, Fuzhong Li, Sparse-View Spectral CT Reconstruction Based on Tensor Decomposition and Total Generalized Variation, 2024, 13, 2079-9292, 1868, 10.3390/electronics13101868 | |
6. | Hayato Itoh, Atsushi Imiya, Subspace Discrimination for Multiway Data, 2024, 66, 0924-9907, 657, 10.1007/s10851-024-01188-9 |
Number of frames replaced | Extracted watermark | NC |
1 | ![]() |
1 |
2 | ![]() |
1 |
3 | ![]() |
1 |
4 | ![]() |
0.8055 |
Rotation angle | Rotated video | Corrected video | Extracted watermark | NC |
100 | ![]() |
![]() |
![]() |
1 |
450 | ![]() |
![]() |
![]() |
1 |
600 | ![]() |
![]() |
![]() |
1 |
Cropping attack | Attacked video | Extracted watermark | NC |
No attack | ![]() |
![]() |
1 |
Cut 45 rows at the top | ![]() |
![]() |
1 |
Cut 45 rows on the left | ![]() |
![]() |
1 |
Attack type | Extracted watermark | NC |
Median filtering (3 × 3) | ![]() |
0.9587 |
Mean filtering (3 × 3) | ![]() |
0.9973 |
Gaussian noise (0, 0.01) | ![]() |
0.8675 |
Salt and pepper noise (0.01) | ![]() |
0.9973 |
Poisson noise | ![]() |
0.9783 |
Number of frames replaced | Extracted watermark | NC |
1 | ![]() |
1 |
2 | ![]() |
1 |
3 | ![]() |
1 |
4 | ![]() |
0.8055 |
Rotation angle | Rotated video | Corrected video | Extracted watermark | NC |
100 | ![]() |
![]() |
![]() |
1 |
450 | ![]() |
![]() |
![]() |
1 |
600 | ![]() |
![]() |
![]() |
1 |
Cropping attack | Attacked video | Extracted watermark | NC |
No attack | ![]() |
![]() |
1 |
Cut 45 rows at the top | ![]() |
![]() |
1 |
Cut 45 rows on the left | ![]() |
![]() |
1 |
Attack type | Extracted watermark | NC |
Median filtering (3 × 3) | ![]() |
0.9587 |
Mean filtering (3 × 3) | ![]() |
0.9973 |
Gaussian noise (0, 0.01) | ![]() |
0.8675 |
Salt and pepper noise (0.01) | ![]() |
0.9973 |
Poisson noise | ![]() |
0.9783 |