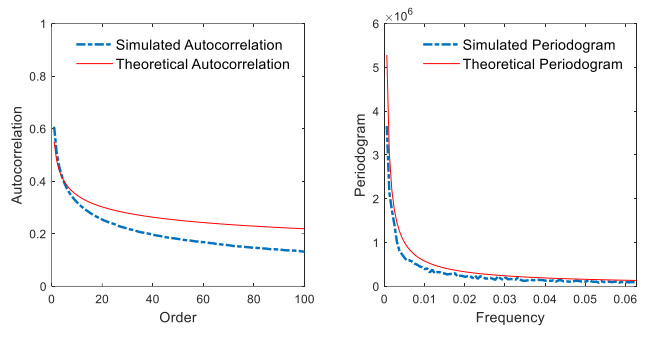
Long memory in test statistics can either originate from fractional integration or be spuriously induced by a short memory process with a structural break. This research estimated and detected long memory from the two causes by simulations and empirical analysis. The simulation results showed that fractional integration and structural break processes could demonstrate long memory properties. The 2ELW estimator was stable for fractional integration but not stable for time series with structural breaks. The modified W statistic based on 2ELW was efficient in discriminating fractional integration and structural breaks. Moreover, we found that six volatility time series of stock indexes and individual stocks in the Chinese market experience significant long memory and structural breaks, and the fractional differencing parameter is overestimated without controlling structural breaks.
Citation: Yirong Huang, Liang Ding, Yan Lin, Yi Luo. A new approach to detect long memory by fractional integration or short memory by structural break[J]. AIMS Mathematics, 2024, 9(6): 16468-16485. doi: 10.3934/math.2024798
[1] | Rubén V. Arévalo, J. Alberto Conejero, Òscar Garibo-i-Orts, Alfred Peris . Stock volatility as an anomalous diffusion process. AIMS Mathematics, 2024, 9(12): 34947-34965. doi: 10.3934/math.20241663 |
[2] | Abdulaziz Khalid Alsharidi, Saima Rashid, S. K. Elagan . Short-memory discrete fractional difference equation wind turbine model and its inferential control of a chaotic permanent magnet synchronous transformer in time-scale analysis. AIMS Mathematics, 2023, 8(8): 19097-19120. doi: 10.3934/math.2023975 |
[3] | Liushuang Meng, Bin Wang . Risk spillovers and extreme risk between e-commerce and logistics markets in China. AIMS Mathematics, 2024, 9(10): 29076-29106. doi: 10.3934/math.20241411 |
[4] | Nichaphat Patanarapeelert, Jiraporn Reunsumrit, Thanin Sitthiwirattham . On nonlinear fractional Hahn integrodifference equations via nonlocal fractional Hahn integral boundary conditions. AIMS Mathematics, 2024, 9(12): 35016-35037. doi: 10.3934/math.20241667 |
[5] | Junseok Kim . A normalized Caputo–Fabrizio fractional diffusion equation. AIMS Mathematics, 2025, 10(3): 6195-6208. doi: 10.3934/math.2025282 |
[6] | Abdon Atangana . Fractional derivatives, dimensions, and geometric interpretation: An answer to your worries. AIMS Mathematics, 2025, 10(2): 2562-2588. doi: 10.3934/math.2025119 |
[7] | Mohammed Alharbey, Turki Mohammed Alfahaid, Ousama Ben-Salha . Asymmetric volatility spillover between oil prices and regional renewable energy stock markets: A time-varying parameter vector autoregressive-based connectedness approach. AIMS Mathematics, 2023, 8(12): 30639-30667. doi: 10.3934/math.20231566 |
[8] | Zhanshou Chen, Muci Peng, Li Xi . A new procedure for unit root to long-memory process change-point monitoring. AIMS Mathematics, 2022, 7(4): 6467-6477. doi: 10.3934/math.2022360 |
[9] | Thavavel Vaiyapuri, Prasanalakshmi Balaji, S. Shridevi, Santhi Muttipoll Dharmarajlu, Nourah Ali AlAseem . An attention-based bidirectional long short-term memory based optimal deep learning technique for bone cancer detection and classifications. AIMS Mathematics, 2024, 9(6): 16704-16720. doi: 10.3934/math.2024810 |
[10] | Xiao Qi, Tianyao Duan, Lihua Wang, Huan Guo . CATL's stock price forecasting and its derived option pricing: a novel extended fNSDE-net method. AIMS Mathematics, 2025, 10(2): 2444-2465. doi: 10.3934/math.2025114 |
Long memory in test statistics can either originate from fractional integration or be spuriously induced by a short memory process with a structural break. This research estimated and detected long memory from the two causes by simulations and empirical analysis. The simulation results showed that fractional integration and structural break processes could demonstrate long memory properties. The 2ELW estimator was stable for fractional integration but not stable for time series with structural breaks. The modified W statistic based on 2ELW was efficient in discriminating fractional integration and structural breaks. Moreover, we found that six volatility time series of stock indexes and individual stocks in the Chinese market experience significant long memory and structural breaks, and the fractional differencing parameter is overestimated without controlling structural breaks.
The long memory of financial markets is still a controversial topic. If a time series has the property of long memory, its autocorrelation function (ACF) slowly decreases as the lag order increases, and its spectrum density function (SDF) is infinite at zero frequency. The Hurst index (H) is one of the most important parameters for measuring the degree of long memory, being crucial to the research and application of long memory. Many empirical results have shown that time series in some fields demonstrate the property of long memory, such as in macroeconomics [1,2], exchange markets [3,4], and equity markets [5,6]. In the weak form of capital efficiency, market prices should reflect historical information. The appearance of long memory would violate the efficient market hypothesis (EMH) that past returns cannot predict future returns. Previous studies have tested the hypothesis of long memory for mature and emerging markets with mixed results. Floros et al. [7] tested the long memory of the Portuguese equity market, using ARFIMA and GARCH models, showing an evident long memory of stock returns. Duppati et al. [8] analyzed five Asian equity indices to test long memory by using the GARCH framework. Luo and Huang [9] used the proposed combined estimator of Hurst exponent based on the conventional R/S-type method and showed that three Chinese stock volatility series experience significant long memory. Luo and Huang [10] employed the AFIGARCH model to demonstrate that the Chinese and American stock volatility series are simultaneously characterized by structural break and long memory. These contradictory results indicate that the model used in the studies can impact the efficacy of long memory tests.
The observed long memory in real data can either be induced by fractional processes [11] or spuriously caused by a structural break (hereafter named as structural break process) [12], which is simply a short memory process. For example, standard estimators such as local Whittle (LW) [13] and Geweke, Porter-Hudak (GPH) [14] always overestimate long memory parameters when there are structural breaks in the time series. Although some scholars [4,15] have tried to modify the standard estimator using a tapered technique, it is still challenging to apply a standard estimator to efficiently detect long memory properties caused either by fractional integration or a structural break process. Therefore, some empirical results that conclude that the data generating process is a fractional process according to long memory parameters may be spuriously caused by a short memory process with a structural break. Although fractional integration and structural break process may both show long memory parameters, their generating regimes and models are different. Therefore, the selection of a model is important to detect the generating process of the long memory process.
Some scholars proposed and developed formal testing procedures to detect fractional integration and short memory processes contaminated by structural breaks. Two groups can be distinguished for the testing procedures. The null hypothesis of the first group is that the time series used to test is a fractional integration process with constant long memory parameters (e.g., [16,17] among others). The other group presents the null hypothesis of a short memory process with a structural break (e.g., [18,19]). According to [20], the simulating results indicate that the W statistics proposed by [17] are more efficient compared with other statistics, which use LW as the estimator of the long memory parameter. Shimotsu and Phillips [21] developed exact local Whittle (ELW) estimation, which does not depend on data differencing and tapering based on LW. Therefore, it is necessary to introduce an ELW estimator to modify the W test statistic and verify its efficiency.
To improve the discriminating performance between real and spurious long memory, this research introduces the 2ELW estimator to modify the W test statistic and evaluate its efficiency by simulation. We further investigate the applications of the modified test statistic and appropriate estimator for the daily volatility series of the stock market in China to indicate whether the long memory observed in the volatility series is genuine or spurious.
This paper is arranged in five sections. In the second section, we investigate the theoretical and empirical long memory behavior of fractional integration or structural break process. Section 3 introduces the 2ELW method used to estimate the long memory parameter and assesses its efficiency by simulation. Section 4 proposes the method to detect fractional integration and structural break and assesses its efficiency by simulation. Section 5 provides the empirical results of the daily realized volatility of China's equity market. The final section gives conclusions.
The property of long memory is always characterized by autocorrelation and spectral functions. The two methods are equivalent. The process shows the property of long memory if the k-th order autocorrelation function satisfies the relationship as k→∞:
ρy(k)∼C⋅k2d−1, | (1) |
where C > 0 is the constant, denotes the same convergence speed, and d denotes the parameter of long memory with the value between 0 and 0.5.
Equation (1) shows that the ACF slowly decays at a rate of hyperbolicity, which decreases as the lag order k increases. Therefore, the observed time series with long distances show a strong correlation.
The process shows the property of long memory if it has the spectral function satisfying the following relationship as ω→0+, d≠0:
f(ω)∼G(ω)⋅|ω|−2d, | (2) |
where the spectral function of the short memory process is denoted as G(ω), which always has the assumption of positive function around zero frequency, and 0 < G(0) < ∞, d denotes the parameter of long memory property with the value between 0 and 0.5. Equation (2) shows that the spectral function of a long memory process would reach a peak value, which may be infinity, when G(ω) is at zero.
As shown in [11], the fractional integration process {yt}Tt=1 is defined as:
(1−L)dyt=εt, | (3) |
where d denotes real value, t=1,2,⋯, L denotes the lag operator, εt∼I(0) or i.i.d., E(εt)=0, 0<σ2ε<∞, E(εtεs)=0 (t≠s).
The parameter d in Eq (3) plays a key role in characterizing long memory. If d is between 0 and 0.5, the autocorrelation function of the process with the property of long memory has the forms shown in Eq (1) and spectral function shown in Eq (2). The fractional differencing operator could be expanded as:
(1−L)d=1−dL+d(d−1)2!L2−d(d−1)(d−2)3!L3−⋯=∑∞k=0(dk)(−1)kLk=∑∞k=0Γ(k−d)Γ(k+1)Γ(−d)Lk, | (4) |
where Γ(α)=∫∞0tα−1e−tdt denotes the Gamma function.
To demonstrate the property of the fractional integration process, we simulate 100 fractional integration time series with a length of 10,000 for d = 0.4. The dashed lines in Figure 1 show the averaging autocorrelation and averaging spectral density curve of the simulated series, and the solid lines show the theoretical autocorrelation and the spectral density curves of the long memory process shown by Eq (2). The results indicate that the autocorrelation and spectral density of fractional integration and long memory process are very close. Therefore, the fractional integration process has the property of long memory.
The property of long memory may be linked with several structural break processes [22]. The Markov switching (MS) process is one of the most typical structural break processes with the following representation:
yt=μst+εt, | (5) |
where t = 1, 2, ⋯, T, {st}Tt=1 is stationary Markov chain, in which st=0 for the first state and st=1 for the second state, and the transition probability pij=P{st=i|st−1=j}, i, j = 0, 1, εt∼i.i.d.(0,σ2ε), μ0≠μ1. If pjj=1−cjTδj, 0<c0,c1<1, 0<δ0,δ1<1, as T→∞, it is shown [22] that:
Var(ST)∼C⋅T2d+1, | (6) |
where ST=XT1+⋯+XTT is the sum of series {XTt}Tt=1, and d=(min{δ0,δ1}−|δ0−δ1|)/2∈(0,1/2).
We simulate 100 Markov chain series with a length of 1000 and the parameters T = 10000, μ0=0, μ1=1, c0=c1=0.9, δ0=δ1=0.8, p00=p11=0.9994, εt∼N(0,1). The dashed lines in Figure 2 describe the curves of averaging autocorrelation and averaging spectral density of the Markov chain series, and the solid line shows the theoretical autocorrelation and the spectral density curves shown by Eqs (1) and (2) for long memory process, respectively. The results show that the autocorrelation and spectral density of the structural break process are close to those of the long memory process. Therefore, the structural break process shows a long memory property. In the literature (e.g., [22]), the fractional integration process is considered as real long memory, while the structural break process is spurious long memory.
Traditional estimators such as GPH and LW are the most popular types of standard estimators for long memory parameters. GPH is simpler, and LW is more robust and efficient. Although they can provide good estimates for the long memory parameter of stationary long memory processes [13], they are not appropriate estimators for nonstationary long memory processes (d≥0.5) [23]. To expand the suitable range of GPH and LW and avoid the non-consistency and non-stable bias, some scholars suggest to first deal with data by using differencing and tapering operators, and then use standard methods to estimate long memory parameters for differenced or tapered data. Although these methods are easily applied and current algorithms are available, they still have some limitations.
Based on the standard LW estimator, a modified LW estimator [21], the exact local Whittle (ELW), avoids using differencing and tapering operators. The ELW estimator is appropriate for stationary and nonstationary situations. Therefore, we can use the ELW estimator to estimate long memory parameters for several cases. Further, it can provide a robust foundation for constructing efficient appropriate confident intervals for unknown long memory parameters.
The objective function of the ELW method can be written as:
QELWm(G,d)=1m∑mj=1[log(G⋅ω−2dj)+1GIΔdy(ωj)], | (7) |
where j=1,2,⋯,m, ωj=2πj/T, the bandwidth m is the parameter controlling the frequency number in the likelihood function, and the periodogram for Δd(y)=(1−L)d(y) is expressed as IΔdy(ωj) = 12πT|∑Ti=1Δd(y)exp(iωt)|. The ELW method minimizes the objective function to estimate d.
Given the value of G, the ELW estimator based on the Eq (7) is equivalent to the following formula:
ˆdELW=argmind∈[Δ1,Δ2][log(ˆG(d))−2d1m∑mj=1log(ωj)], | (8) |
where Δ1 and Δ2 are the down limit and up limit of d, respectively, ˆG(d)=1m∑mj=1IΔdy(ωj).
Shimotsu [24] considers the case with unknown mean and polynomial trend and develops the conventional ELW estimator into a two-steps ELW estimator (hereafter, 2ELW). First, we get the estimate of ˆdTLW by the TLW estimator proposed by [15] and further estimate the differencing parameter by the following formula:
ˆd2ELW=ˆdTLW−R'F(ˆdTLW)/R''F(ˆdTLW), | (9) |
where RF(d)=log(ˆG(d))-2d1m∑mj = 1log(ωj). We use ˆd2ELW to substitute ˆdTLW in Eq (9), and repeat the calculation until ˆd2ELW converges to a stable value. According to [24], the 2ELW estimator has the asymptotic normal distribution, that is, √m(ˆd2ELW−d0)→pN(0,1/4), for the process with -0.5 < d < 2 but no polynomial trend, and for the process with -0.5 < d < 1.75 and polynomial trend. The 2ELW estimator inherits the property of ELW.
We simulate 1000 fractional integration series with the length of N = 5000 and d = 0.2, 0.4, 0.6, and 0.8, and estimate d for each simulated series by the above 2ELW estimator. The Bartlett taper hBt = 1-|2t-TT| is used, and the bandwidth parameter is set as m = T0.6, T0.7, and T0.8. The mean and 95% confidence interval of the estimated d is presented in Table 1. The results show that the 2ELW estimator can provide an efficient estimate for the fractional integration process, and the results of the estimate keep stable with different parameters of bandwidth.
Frequency | d=0.20 | d=0.40 | d=0.60 | d=0.80 |
T0.6 | 0.2004 [0.1240, 0.2784] |
0.4004 [0.3193, 0.4760] |
0.6121 [0.5247, 0.6854] |
0.7982 [0.7106, 0.8744] |
T0.7 | 0.2001 [0.1464, 0.2520] |
0.4002 [0.3484, 0.4491] |
0.6100 [0.5552, 0.6617] |
0.7988 [0.7425, 0.8498] |
T0.8 | 0.2110 [0.1772, 0.2434] |
0.4106 [0.3739, 0.4454] |
0.6152 [0.5806, 0.6478] |
0.8082 [0.7734, 0.8410] |
We simulate 1000 series with a length of 5000 for the Markov process yt = μst+εt, where εt∼N(0,1), t = 1, 2, ⋯, T, {st}Tt=1 is the Markov chain, which is stationary with the values of 0 and 1, and the transition probability is p01=P{st=0|st−1=1}=0.9995, μ0=0, μ1=1. We use the 2ELW estimator with the same parameter setting as above to estimate d for each simulated series. The mean and 95% confidence interval of the estimated d is shown in Table 2. The results show that most estimates of d are between 0 and 0.5, with different results for different simulated series and with different bandwidth parameters. Therefore, the property of structural break can induce the spurious long memory.
Frequency | δ1=δ2=0.2 d=0.10 |
δ1=δ2=0.4 d=0.20 |
δ1=δ2=0.6 d=0.30 |
δ1=δ2=0.8 d=0.40 |
T0.6 | 0.0160 [-0.0738, 0.0969] |
0.2888 [0.2048, 0.3649] |
0.4975 [0.4311, 0.5686] |
0.4314 [0.2726, 0.5158] |
T0.7 | 0.0582 [0.0076, 0.1123] |
0.3266 [0.2846, 0.3728] |
0.3651 [0.3280, 0.4022] |
0.3082 [0.1963, 0.3573] |
T0.8 | 0.1187 [0.0850, 0.1517] |
0.2636 [0.2382, 0.2899] |
0.2605 [0.2369, 0.2828] |
0.2217 [0.1261, 0.2560] |
Qu [17] proposed a test statistic (hereafter denoted as Qu test) that is constructed based on the null hypothesis of a stationary long memory process with spectral density function f(λ)≃Gλ−2d, where 1/2 < d < 1/2, G∈(0, ∞), and the alternative hypothesis of a short memory process with mean shift. The specification of Qu test is written as:
W=supr∈[ε,1](∑mj=1v2j)−1/2[∑[mr]j=1vj(IjG(dLW)λ−2dLWj−1)], | (10) |
where dLW is the estimate of fractional differencing parameter with LW estimator, ε is the truncated parameter, vj=logλj−(1/m)∑mj=1logλj, G(d)=m−1∑mj=1λ2djIj, Ij=Ix(λj)=(2πn)−1|∑nt=1xtexp(iλjt)|2. We can use the Qu test to detect long memory and structural break processes.
As we know, the 2ELW estimator performs better than LW and has better properties than LW, and it can be used for stationary and nonstationary process. Therefore, we estimate d2ELW of d to substitute dLW in Eq (10), and construct the test statistic as follows:
W=supr∈[ε,1](∑mj=1v2j)−1/2[∑[mr]j=1vj(IjG(d2ELW)λ−2d2ELWj−1)]. | (11) |
The size of a statistic test is defined as the probability of incorrectly rejecting the null hypothesis. The power of a statistical test is defined as the probability of correctly rejecting the null hypothesis. For a given hypothesis and test statistic, the size should be made as small as possible, and the power should be made as large as possible. For the test in our paper, the null hypothesis of the test is the fractional integration process, and the corresponding alternative hypothesis is the short memory process with the structural break.
To evaluate the empirical size of the test, we design the following simulating procedure.
Step 1: We specify the fractional integration process with long memory parameter and ARMA term and generate fractional integration series with different lengths. The values of the long memory parameter and ARMA term are set as the ones in [17]. According to [17], the long memory parameter d is typically between 0.30 and 0.45, especially for financial applications; therefore, we also set d = 0.4. In detail, the models considered in this paper include the following four specifications. ARFIMA(0, d, 0): (1−L)0.4yt=εt, ARFIMA(1, d, 0): (1−0.4L)(1−L)0.4yt=εt, ARFIMA(0, d, 1): (1−L)0.4yt=(1+0.4L)εt, ARFIMA(2, d, 0): (1−0.5L)(1−0.3L)(1−L)0.4yt=εt.
Step 2: We apply the new test and Qu test to calculate the test statistic for each generated series. The null hypothesis is rejected if the value of the test statistic is bigger than the critical value at a significant level. Repeating the data generation and the testing steps will produce several test results. The empirical size is calculated as the proportion of times in which the null hypothesis is rejected.
We simulate 1000 fractional integration series with d = 0.4 and the different lengths, including 500, 1000, 2000, 3000, 5000, and 10000. The ratio of rejecting the null hypothesis at a significance level of 5% and 1% is calculated. Table 3 provides the calculated results of empirical size. We can see that all size level values are less than 5% and 1%, and as the sample length becomes bigger, the test size is closer to 5% and 1%. Therefore, the probability of incorrectly rejecting the null hypothesis based on ˜W is less than 5%. Moreover, the empirical size of the new test ˜W is almost lower than that of Qu test W.
Sample length | ARFIMA(0, d, 0) | ARFIMA(1, d, 0) | ARFIMA(0, d, 1) | ARFIMA(2, d, 0) | |||||
ε=0.02 | ε=0.05 | ε=0.02 | ε=0.05 | ε=0.02 | ε=0.05 | ε=0.02 | ε=0.05 | ||
5% nominal level | |||||||||
500 | W | 0.0330 | 0.0640 | 0.0170 | 0.0330 | 0.0180 | 0.0500 | 0.0760 | 0.0690 |
˜W | 0.0160 | 0.0250 | 0.0140 | 0.0250 | 0.0220 | 0.0250 | 0.0740 | 0.0660 | |
1000 | W | 0.0370 | 0.0690 | 0.0240 | 0.0480 | 0.0200 | 0.0470 | 0.0240 | 0.0350 |
˜W | 0.0230 | 0.0270 | 0.0210 | 0.0300 | 0.0260 | 0.0320 | 0.0220 | 0.0360 | |
2000 | W | 0.0260 | 0.0630 | 0.0230 | 0.0500 | 0.0180 | 0.0530 | 0.0400 | 0.0460 |
˜W | 0.0180 | 0.0260 | 0.0290 | 0.0320 | 0.0310 | 0.0280 | 0.0440 | 0.0450 | |
3000 | W | 0.0380 | 0.0730 | 0.0220 | 0.0520 | 0.0260 | 0.0590 | 0.0360 | 0.0430 |
˜W | 0.0340 | 0.0430 | 0.0310 | 0.0330 | 0.0330 | 0.0330 | 0.0340 | 0.0370 | |
5000 | W | 0.0340 | 0.0750 | 0.0300 | 0.0700 | 0.0250 | 0.0570 | 0.0500 | 0.0570 |
˜W | 0.0410 | 0.0410 | 0.0360 | 0.0370 | 0.0340 | 0.0420 | 0.0500 | 0.0570 | |
10000 | W | 0.0210 | 0.0660 | 0.0310 | 0.0720 | 0.0280 | 0.0770 | 0.0760 | 0.0690 |
˜W | 0.0300 | 0.0250 | 0.0350 | 0.0430 | 0.0390 | 0.0500 | 0.0740 | 0.0660 | |
1% nominal level | |||||||||
500 | W | 0.0060 | 0.0160 | 0.0020 | 0.0020 | 0.0010 | 0.0100 | 0.0180 | 0.0150 |
˜W | 0.0030 | 0.0040 | 0.0040 | 0.0030 | 0.0020 | 0.0010 | 0.0170 | 0.0140 | |
1000 | W | 0.0040 | 0.0190 | 0.0050 | 0.0080 | 0.0010 | 0.0120 | 0.0020 | 0.0040 |
˜W | 0.0070 | 0.0070 | 0.0030 | 0.0050 | 0.0040 | 0.0050 | 0.0030 | 0.0020 | |
2000 | W | 0.0020 | 0.0110 | 0.0020 | 0.0050 | 0.0030 | 0.0140 | 0.0110 | 0.0060 |
˜W | 0.0030 | 0.0050 | 0.0050 | 0.0040 | 0.0050 | 0.0050 | 0.0080 | 0.0050 | |
3000 | W | 0.0100 | 0.0270 | 0.0010 | 0.0080 | 0.0080 | 0.0170 | 0.0050 | 0.0060 |
˜W | 0.0100 | 0.0090 | 0.0020 | 0.0040 | 0.0100 | 0.0090 | 0.0050 | 0.0060 | |
5000 | W | 0.0030 | 0.0210 | 0.0040 | 0.0110 | 0.0050 | 0.0150 | 0.0110 | 0.0120 |
˜W | 0.0040 | 0.0040 | 0.0050 | 0.0070 | 0.0070 | 0.0070 | 0.0110 | 0.0120 | |
10000 | W | 0.0040 | 0.0160 | 0.0060 | 0.0090 | 0.0040 | 0.0180 | 0.0180 | 0.0150 |
˜W | 0.0050 | 0.0020 | 0.0050 | 0.0060 | 0.0060 | 0.0050 | 0.0170 | 0.0140 | |
Note: ε is the truncated parameter and d is the long memory parameter with a value of 0.40. |
To evaluate the power of the test, we apply a similar simulating procedure. First, we specify several short memory processes with structural break and generate spurious long memory series. The models considered include: (1) Markov switching (MS), which is defined as Eq (5); (2) White noise with a monotonic deterministic trend (WNMT): yt=3t−0.1+εt, εt ∼ iid N(0, 1); (3) White noise with a nonmonotonic deterministic trend (WNNMT): yt=sin(4πt/n)+εt, εt ∼ iid N(0, 3); and (4) Stationary random level shift (SRLS): yt=μt+εt, μt=(1−γt)μt−1+γtzt, γt ∼ iidB(1, 0.003), εt and zt ∼ iidN(0, 1). Second, we apply the test statistic to each series and repeat the data generation and testing steps. The empirical power of the test is calculated as the proportion of times where the null hypothesis is rejected.
We simulate 1000 series for a short memory process with a structural break with different lengths, including 500, 1000, 2000, 3000, 5000, and 10000. The ratio of rejecting the null hypothesis that the simulated series is a fractional integration process is calculated at a significance level of 5% and 1%. Table 4 provides the results of the empirical power for the new test ˜W and Qu test W. The results indicate that most values of test power are higher than 95%, and as the sample length becomes bigger, the test power is higher. Therefore, the probability of correctly rejecting the null hypothesis based on ˜W is higher than 95%. Moreover, the empirical powers of the new test ˜W are almost higher than that of Qu test W.
Sample length | Test statistic | MS | WNMT | WNNMT | SRLS | ||||
ε=0.02 | ε=0.05 | ε=0.02 | ε=0.05 | ε=0.02 | ε=0.05 | ε=0.02 | ε=0.05 | ||
5% nominal level | |||||||||
500 | W | 0.7030 | 0.7690 | 0.1820 | 0.2940 | 0.0970 | 0.1770 | 0.7350 | 0.8150 |
˜W | 0.9840 | 0.9850 | 0.9650 | 0.9740 | 1.0000 | 1.0000 | 0.8820 | 0.9300 | |
1000 | W | 0.8450 | 0.8990 | 0.3370 | 0.5370 | 0.4570 | 0.7160 | 0.8780 | 0.9290 |
˜W | 0.9900 | 0.9930 | 0.9860 | 0.9880 | 1.0000 | 1.0000 | 0.9260 | 0.9620 | |
2000 | W | 0.9080 | 0.9520 | 0.6260 | 0.8590 | 0.8210 | 0.9780 | 0.9250 | 0.9620 |
˜W | 0.9970 | 0.9980 | 0.9940 | 0.9950 | 1.0000 | 1.0000 | 0.9680 | 0.9750 | |
3000 | W | 0.9230 | 0.9570 | 0.8200 | 0.9500 | 1.0000 | 1.0000 | 0.9680 | 0.9750 |
˜W | 0.9990 | 0.9990 | 0.9980 | 1.0000 | 1.0000 | 1.0000 | 0.9920 | 0.9920 | |
5000 | W | 0.9060 | 0.9390 | 0.9550 | 0.9970 | 1.0000 | 1.0000 | 0.9920 | 0.9920 |
˜W | 0.9990 | 1.0000 | 1.0000 | 1.0000 | 1.0000 | 1.0000 | 1.0000 | 1.0000 | |
10000 | W | 0.8540 | 0.9260 | 1.0000 | 1.0000 | 1.0000 | 1.0000 | 1.0000 | 1.0000 |
˜W | 1.0000 | 1.0000 | 1.0000 | 1.0000 | 1.0000 | 1.0000 | 1.0000 | 1.0000 | |
1% nominal level | |||||||||
ε=0.02 | ε=0.05 | ε=0.02 | ε=0.05 | ε=0.02 | ε=0.05 | ε=0.02 | ε=0.05 | ||
500 | W | 0.5180 | 0.5930 | 0.0550 | 0.1020 | 0.0300 | 0.0660 | 0.3010 | 0.4220 |
˜W | 0.9760 | 0.9760 | 0.9310 | 0.9450 | 1.0000 | 1.0000 | 0.4640 | 0.6620 | |
1000 | W | 0.6870 | 0.7640 | 0.1420 | 0.2960 | 0.2420 | 0.5060 | 0.4600 | 0.6600 |
˜W | 0.9840 | 0.9840 | 0.9600 | 0.9720 | 1.0000 | 1.0000 | 0.4650 | 0.6590 | |
2000 | W | 0.7530 | 0.8290 | 0.3700 | 0.6390 | 0.6050 | 0.9150 | 0.4610 | 0.6590 |
˜W | 0.9910 | 0.9940 | 0.9830 | 0.9870 | 1.0000 | 1.0000 | 0.5900 | 0.7350 | |
3000 | W | 0.7750 | 0.8380 | 0.6030 | 0.8440 | 1.0000 | 1.0000 | 0.5890 | 0.7350 |
˜W | 0.9960 | 0.9990 | 0.9920 | 0.9910 | 1.0000 | 1.0000 | 0.8040 | 0.8340 | |
5000 | W | 0.7550 | 0.8190 | 0.8720 | 0.9830 | 1.0000 | 1.0000 | 0.8040 | 0.8330 |
˜W | 0.9980 | 0.9990 | 0.9980 | 0.9950 | 1.0000 | 1.0000 | 0.9770 | 0.9530 | |
10000 | W | 0.6190 | 0.7210 | 0.9980 | 1.0000 | 1.0000 | 1.0000 | 1.0000 | 0.9950 |
˜W | 0.9960 | 0.9970 | 1.0000 | 1.0000 | 1.0000 | 1.0000 | 1.0000 | 0.9980 |
In this section, we use the 2ELW estimator shown in Eq (9) to substitute the ELW estimator in Eq (10) to build the new test statistic called ˜W. From the results of the size and power of rejecting the null hypothesis of the fractional integration process, which are adequately demonstrated by the simulation, the test statistic can discriminate between fractional integration process and structural break process. In detail, when the time series is derived from the fractional integration process, the test statistic does not reject the null hypothesis; moreover, when the time series is derived from a short memory process with a structural break, the null hypothesis could be rejected. Overall, the modified ˜W test has decent size and power and performs better than the Qu test W. In the next section, we will apply the new test statistic to detect fractional integration and structural break and further use the appropriate estimator to estimate long memory parameters for six volatility series of market indexes and individual stocks.
The data sample includes the 5-min close price of four Chinese stock indexes—the Shanghai stock exchange composite index (hereafter, SZZS), the Shenzhen stock exchange component index (hereafter, SZCZ), the Shanghai-Shenzhen 300 index (hereafter, HS300), and the CSI Smallcap 500 index (hereafter, ZZ500)—and two individual stocks: Ping An Bank Co., Ltd. (PAYH) and Kweichow Moutai Co., Ltd. (GZMT). In detail, SZZS and SZCZ reflect the A-share stocks traded on the Shanghai and Shenzhen stock exchanges, respectively. HS300 index includes the 300 A-share stocks traded on the Shanghai and Shenzhen stock exchanges and is seen as indicative of trends in both markets. ZZ500 index includes the top 500 stocks in total market value after excluding the constituent stocks of the HS300 index. PAYH and GZMT are individual A-stocks traded in Shenzhen and Shanghai stock exchanges. All sample periods range from January 1, 2005, to December 31, 2023.
The 5-min log return series is calculated as follows:
rt=100×[log(Pt)−log(Pt−1)], | (12) |
where Pt represents closing price of 5 min at time t.
The daily realized volatility (RV) [25] as a proxy of daily volatility is measured by the following formula:
RV(m)t=∑mi=1[r(m)i]2, | (13) |
where r(m)i denotes the i-th 5-min logarithmic close return at day t, and m denotes the number of 5-min returns at day t.
Figure 3 presents the daily volatility series for SZZS, SZCZ, HS300, ZZ500, PAYH, and GZMT. Table 5 presents the results of statistical analysis. The results indicate that there are some common features among the three volatility series, for example, short dependence, volatility clustering, and heavy tail. Moreover, they all are not unit root processes and show distinct stationary long memory.
SZZS | SZCZ | HS300 | ZZ500 | PAYH | GZMT | |
Number | 4615 | 4615 | 4554 | 4125 | 4615 | 4615 |
Minimum | 7.4977×10-6 | 1.2063×10-5 | 6.6163×10-6 | 1.1616×10-5 | 0.0000 | 0.0000 |
Maximum | 0.0072 | 0.0084 | 0.0075 | 0.0084 | 0.0347 | 0.0148 |
Mean | 2.0127×10-4 | 2.6410×10-4 | 2.2985×10-4 | 2.6998×10-4 | 6.7144×10-4 | 5.0438×10-4 |
Standard Deviation | 3.9586×10-4 | 4.3842×10-4 | 4.2136×10-4 | 5.0142×10-4 | 0.0011 | 8.0451×10-4 |
Skewness | 7.8574 | 6.8873 | 7.5240 | 6.1557 | 10.2729 | 6.6943 |
Kurtosis | 95.9140 | 77.5222 | 88.2748 | 59.0801 | 227.68 | 71.3534 |
JB | 1.7075×107*** | 1.1044×107*** | 1.4228×107*** | 5.6660×106*** | 9.7883×107*** | 9.3289×106*** |
KS | 0.5000*** | 0.5000*** | 0.5000*** | 0.5000*** | 0.5000*** | 0.5000*** |
Q(5) | 5.3073×104*** | 4.8928×104*** | 5.2906×104*** | 5.0545×104*** | 2.7802×104*** | 2.9610×104*** |
Q(10) | 7.8717×104*** | 7.3454×104*** | 7.6993×104*** | 7.8002×104*** | 3.9683×104*** | 3.9131×104*** |
ADF | -29.8203*** | -29.2595*** | -29.4255*** | -26.8728*** | -35.0303*** | -33.7009*** |
d | 0.3864 | 0.3866 | 0.3842 | 0.3967 | 0.3160 | 0.3133 |
Number of structural break | 3 | 3 | 3 | 3 | 4 | 4 |
Notes: SD, JB, ADF, and Q denote standard deviation, Jarque Bera, Augmented Dickey-Fuller, and Ljung Box statistics, respectively. d denotes the estimate of differencing parameters based on 2ELW method at bandwidth m = T0.7. *, **, *** indicate significance at significance nominal level of 10%, 5%, and 1%, respectively. The sequential procedure [26] based on supFT(k+1|k) statistics is used to detect the number of structural breaks. |
As shown in Section 5.1, six volatility series experience the property of long memory. It is necessary to further apply the test statistic shown in Eq (11) to detect whether this long memory is originated from a fractional integration process or induced by structural break in the series. Almost all test statistics of the new test ˜W are significant at level 5% but not significant for the Qu test W. The detecting results by the new test ˜W shown in Table 6 for six volatility series indicate that all daily volatility of stock indexes and individual stocks experience spurious long memory, which is consistent with the results of estimating structural breaks in the next section. The detecting results of the Qu test W show the spurious long memory of individual stocks but barely for stock indexes. From the results of Table 6, it is shown that the proposed new statistic has a higher ability to detect long memory and structural breaks in volatility series than the original Qu test.
Volatility series | W | ˜W | ||
ε=0.02 | ε=0.05 | ε=0.02 | ε=0.05 | |
m=T0.60 | ||||
SZZS | 0.9986 | 0.9986 | 1.2002* | 1.2002** |
SZCZ | 0.9674 | 0.9674 | 1.1180* | 1.1180* |
HS300 | 1.0138 | 1.0138 | 1.1853* | 1.1853** |
ZZ500 | 0.8654 | 0.8654 | 1.4260** | 1.4260** |
PAYH | 1.1739* | 1.1050* | 2.0732*** | 2.0732*** |
GZMT | 1.2910** | 1.2234** | 1.9436*** | 1.9436*** |
m=T0.70 | ||||
SZZS | 1.0552 | 1.0552* | 1.0849 | 1.0426* |
SZCZ | 1.0631 | 0.9826 | 1.1092 | 1.0122 |
HS300 | 1.0734 | 1.0734* | 0.9440 | 0.9440 |
ZZ500 | 1.0417 | 1.0417* | 1.4607** | 1.4607*** |
PAYH | 1.1098 | 0.6656 | 1.7682*** | 1.4232** |
GZMT | 1.0826 | 1.0826* | 1.0361 | 0.6801 |
m=T0.80 | ||||
SZZS | 0.6679 | 0.6679 | 1.1206* | 1.1206* |
SZCZ | 0.6834 | 0.6834 | 1.1995* | 1.1995** |
HS300 | 1.1420* | 1.1420* | 1.5649*** | 1.5649*** |
ZZ500 | 0.9489 | 0.9348 | 0.8272 | 0.7989 |
PAYH | 1.3974** | 1.3974** | 1.5155** | 1.5155*** |
GZMT | 1.3050** | 1.3050** | 1.5261*** | 1.5261*** |
Notes: At ε=0.02, test statistic ˜W has the critical values of 1.118, 1.252, and 1.517 at significance level of 10%, 5%, and 1%, respectively. At ε=0.05, test statistic ˜W has the critical values of 1.022, 1.155, and 1.426 at significance level of 10%, 5%, and 1%, respectively. *, **, and *** show significance at 10%, 5%, and 1%, respectively. |
We apply the test statistic proposed by [27] to detect the number of structural breaks in three daily volatility series, which include supFT(k)1, DmaxFT2, and supFT(k+1|k)3. The forms of these statistics are introduced in detail in [27]. The steps for estimating structural breaks are as follows. The first step is to test whether there exists a structural break, and the second one is to estimate the number of structural breaks. Although the methods based on LWZ [28] and BIC [29] perform well, they may underestimate the number of structural breaks when there is heteroscedasticity in the volatility series. Therefore, the sequential procedure proposed by [26] is selected to identify the number of structural breaks. The third step is to further identify the location date and estimate the mean of each period divided according to the detected points of structural breaks. The model considered in this research is the mean shift model, that is, yt=δi+ut, where yt is time series at time t, δi is the mean of the i-th period, ut is the random term at time t, t=1,2,⋯,T, i =1,2,⋯,m, and m is the number of structural breaks. We use OLS to estimate the parameters of the model.
1This test statistic is used for the case in which the number of structural breaks is known, for which the null and alternative hypothesis are set as no structural break and k structural breaks, respectively.
2This test statistic is used for the case in which the number of structural breaks is unknown, for which the null and alternative hypothesis are set as no structural break and unknown k structural breaks, respectively. This test statistic includes two types according to its weight: UDmaxFT and WDmaxFT.
3This test statistic is used for the case in which the number of structural breaks is known, for which the null and alternative hypothesis are set as no structural break and k structural breaks, respectively.
Table 7 provides the results of testing and estimating structural breaks. For daily volatility of SZZS, the supFT(k) (k = 1, 2, 3, 4, 5), UDmax, WDmax, SupF(2|1), and SupF(3|2) are significant at 1%, but SupF(4|3) and SupF(5|4) are not significant at 10%. Therefore, there may be at least one structural break for the daily volatility series of SZZS index. Moreover, the sequential procedure gives three structural breaks. The corresponding location date is given in the fourth column of Table 7. Three break dates are October 9, 2009, June 20, 2013, and April 21, 2016, and the means of the four periods are 3.7182 × 10-4, 1.2774 × 10-4, 3.1906 × 10-4, and 0.8776 × 10-4. The results for the other five volatility series are also shown in Table 7. The detecting results show that all the volatility series, especially the four stock indexes, have almost the same number of breaks and break dates. Moreover, their volatility level in the first period is the highest.
Volatility series | Test statistics | Number of breaks | Date of break | Volatility mean (×10−4) |
SZZS | SupF(1|0)= 62.6673*** SupF(2|1)=30.8391*** SupF(3|2)=17.6714*** SupF(4|3)=5.3531 SupF(5|4)=5.0070 |
Seq.:3 BIC:3 LWZ:3 |
T1='2009-10-09' T2='2013-06-20' T3='2016-04-21' |
σF=2.0127 σ1=3.7182 σ2=1.2774 σ3=3.1906 σ4=0.8776 |
SZCZ | SupF(1|0)=108.8058*** SupF(2|1)=17.7389*** SupF(3|2)=15.4759*** SupF(4|3)=10.4038* SupF(5|4)=4.3863 |
Seq.:3 BIC:3 LWZ:4 |
T1='2009-10-09' T2='2013-05-28' T3='2016-03-30' |
σF=2.6410 σ1=4.3962 σ2=2.0142 σ3=3.8999 σ4=1.3998 |
HS300 | SupF(1|0)=59.9358*** SupF(2|1)=30.5248*** SupF(3|2)=13.4609** SupF(4|3)=11.2809* SupF(5|4)=0.0000 |
Seq.:3 BIC:2 LWZ:3 |
T1='2009-10-09' T2='2013-05-23' T3='2016-03-14' |
σF=2.2985 σ1=4.1579 σ2=1.6544 σ3=3.4221 σ4=1.1228 |
ZZ500 | SupF(1|0)=81.6174*** SupF(2|1)=37.9784*** SupF(3|2)=12.2999** SupF(4|3)=8.1152 SupF(5|4)=0.0000 |
Seq.:3 BIC:2 LWZ:3 |
T1='2009-09-01' T2='2013-12-11' T3='2016-06-24' |
σF=2.6998 σ1=6.7159 σ2=1.9922 σ3=4.1633 σ4=1.1999 |
PAYH | SupF(1|0)=163.5319*** SupF(2|1)=24.4941*** SupF(3|2)=23.9975*** SupF(4|3)=26.6674*** SupF(5|4)=0.0000 |
Seq.:4 BIC:4 LWZ:3 |
T1='2009-09-03' T2='2012-11-12' T3='2015-09-17' T4='2018-08-23' |
σF=6.7144 σ1=13.1276 σ2=3.7233 σ3=8.5094 σ4=2.6979 σ5=4.1498 |
GZMT | SupF(1|0)=98.7432*** SupF(2|1)=10.8309** SupF(3|2)=15.8123*** SupF(4|3)=14.3869** SupF(5|4)=0.0000 |
Seq.:4 BIC:1 LWZ:3 |
T1='2009-03-06' T2='2012-11-20' T3='2015-10-08' T4='2018-08-03' |
σF=5.0438 σ1=9.6366 σ2=3.3326 σ3=5.6520 σ4=2.6985 σ5=3.6271 |
Notes: The parameter setting, test statistic, number of structural breaks, and the estimate of location and mean for each period are shown in Column 1, 2, 3, and 4, respectively. *, **, and *** show significance at 10%, 5%, and 1%, respectively. |
To reflect the impact of structural breaks on the estimating results of long memory, the full sample is divided into several subsamples based on the estimate of structural breaks, and the 2ELW method is used to estimate d for each subsample. From the estimating results given in Table 8, there is a big difference between the estimating results of d in the subsample and full sample. Some estimates of subsamples are smaller than one in the full sample, but others are not. Therefore, the volatility series in different samples experience different long memory.
Volatility series | Tm | Subsample 1 | Subsample 2 | Subsample 3 | Subsample 4 | Subsample 5 | Full sample |
T0.6 | 0.3030 | 0.3016 | 0.3878 | 0.1999 | 0.3975 | ||
SZZS | T0.7 | 0.3122 | 0.2866 | 0.6688 | 0.2065 | - | 0.3864 |
T0.8 | 0.3661 | 0.3950 | 0.5012 | 0.1898 | - | 0.4144 | |
T0.6 | 0.3041 | 0.3147 | 0.3823 | 0.2831 | - | 0.4061 | |
SZCZ | T0.7 | 0.3304 | 0.3011 | 0.4671 | 0.2599 | - | 0.3866 |
T0.8 | 0.3710 | 0.3701 | 0.4527 | 0.2560 | - | 0.4071 | |
T0.6 | 0.3252 | 0.3178 | 0.3936 | 0.2339 | - | 0.3768 | |
HS300 | T0.7 | 0.3359 | 0.3024 | 0.6616 | 0.2396 | - | 0.3842 |
T0.8 | 0.3835 | 0.3783 | 0.5240 | 0.2140 | - | 0.4350 | |
T0.6 | 0.3190 | 0.2672 | 0.4713 | 0.2729 | - | 0.4132 | |
ZZ500 | T0.7 | 0.3185 | 0.2904 | 0.4916 | 0.2404 | - | 0.3967 |
T0.8 | 0.3888 | 0.4049 | 0.4553 | 0.2350 | - | 0.4083 | |
T0.6 | 0.2289 | 0.3806 | 0.2808 | 0.3748 | 0.3463 | 0.3138 | |
PAYH | T0.7 | 0.3053 | 0.3502 | 0.4393 | 0.3932 | 0.2852 | 0.3160 |
T0.8 | 0.2302 | 0.2995 | 0.4453 | 0.4991 | 0.2760 | 0.3181 | |
T0.6 | 0.0823 | 0.5318 | 0.3038 | 0.2064 | 0.2172 | 0.2845 | |
GZMT | T0.7 | 0.2461 | 0.4197 | 0.3872 | 0.2434 | 0.2977 | 0.3133 |
T0.8 | 0.3587 | 0.3767 | 0.4558 | 0.2856 | 0.2890 | 0.3547 | |
Note: d is estimated by the 2ELW method. *, **, and *** show significance at 10%, 5%, and 1%, respectively. |
The estimated results of d for the daily volatility series of SZZS index in the full sample for m=T0.6, T0.7and T0.8 are 0.3975, 0.3864, and 0.4144, respectively. The estimation in four subsamples is between 0.3 and 0.4 and between 0.1 and 0.2. Moreover, the estimates of some subsamples for different bandwidth parameters are close. Therefore, there is a distinct impact of a structural break on the estimate of long memory. The estimation becomes more robust when the structural break is eliminated from the volatility series. Moreover, all the subsamples significantly experience the property of long memory, even though there is nonstationary long memory for some subsamples. The estimate of the long memory parameter in the third subsample for all the indexes and stocks is the biggest among all the subsamples.
The property of long memory observed in empirical research may either originate from the fractional integration process or be spuriously induced by the structural break process. Our research modifies the detecting statistic proposed by [17], evaluates its performance based on simulation, and empirically estimates the long memory parameter of the daily volatility series for the stock market in China. The conclusions are as follows. First, the fractional integration process and the short memory process contaminated by structural breaks both show the characteristics of long memory, while the structural break process generates spurious long memory. Second, the 2ELW method could give robust estimating results for the long memory parameter but is significantly impacted by the bandwidth parameter of the 2ELW estimator for the structural break process. Third, the ˜W test statistic based on the 2ELW estimator can efficiently discriminate between the fractional integration process and the structural break process. Fourth, there exists the property of real long memory and structural break for the daily volatility in China's stock market, and the long memory parameter estimate is affected by the property of structural break.
The simulation and empirical tests of this research demonstrate a new approach with the modified ˜W test statistic based on the introduction of the 2ELW estimator to the current W test statistic to distinguish between real long memory process (i.e., fractional integration) and spurious long memory (i.e., a short memory process contaminated by a structural break). This approach may provide a potential answer for previous research showing market long memory that may be overestimated under the structural break process. A true long memory in the market could be used by portfolio managers when building investment strategies and forecasting financial risk.
We believe that future research should focus on further differentiation between fractional integration and structural break; the differentiation between fractional integration and other important properties such as trend, nonlinearity, fat-tail, heteroscedasticity, etc.; the data generating process for temporal aggregation or cross-sectional aggregation, and efficient estimation of the models for the long memory parameter with other properties at the same time.
Yirong Huang: Conceptualization, Methodology, Software, Formal analysis, Writing – original draft, review & editing, Validation; Liang Ding: Writing – original draft, review & editing, Validation; Yan Lin: Data curation, Validation, Writing – review & editing; Yi Luo: Writing – original draft, review & editing, Software, Data curation, Validation, Visualization. All authors have read and approved the final version of the manuscript for publication.
The authors declare they have not used Artificial Intelligence (AI) tools in the creation of this article.
This work was supported by the research grants from the Humanities and Social Science Foundation of Ministry of Education of China (Research on the hybrid forecast model of financial volatility based on big data and deep learning: Theory and application, No. 22YJAZH032), and the Natural Science Foundation of Guangdong Province (No. 2022A1515011478).
The authors declared no potential conflicts of interest with respect to the research, authorship, and/or publication of this article.
[1] |
U. Hassler, J. Wolters, Long memory in inflation rates: International evidence, J. Bus. Econ. Stat., 13 (1995), 37–45. https://doi.org/10.2307/1392519 doi: 10.2307/1392519
![]() |
[2] |
K. Choi, E. Zivot, Long memory and structural changes in the forward discount: An empirical investigation, J. Int. Money Finan., 26 (2007), 342–363. https://doi.org/10.1016/j.jimonfin.2007.01.002 doi: 10.1016/j.jimonfin.2007.01.002
![]() |
[3] |
Y. W. Cheung, Long memory in foreign-exchange rates, J. Bus. Econ. Stat., 11 (1993), 93–101. https://doi.org/10.2307/1391309 doi: 10.2307/1391309
![]() |
[4] |
C. Velasco, Gaussian semiparametric estimation of non-stationary time series, J. Time Ser. Anal., 20 (1999), 87–127. https://doi.org/10.1111/1467-9892.00127 doi: 10.1111/1467-9892.00127
![]() |
[5] |
K. V. Chow, K. C. Denning, S. Ferris, G. Noronha, Long-term and short-term price memory in the stock market, Econ. Lett., 49 (1995), 287–293. https://doi.org/10.1016/0165-1765(95)00690-H doi: 10.1016/0165-1765(95)00690-H
![]() |
[6] |
G. Bhardwaj, N. R. Swanson, An empirical investigation of the usefulness of ARFIMA models for predicting macroeconomic and financial time series, J. Econom., 131 (2006), 539–578. https://doi.org/10.1016/j.jeconom.2005.01.016 doi: 10.1016/j.jeconom.2005.01.016
![]() |
[7] |
C. Floros, S. Jaffry, G. V. Lima, Long memory in the Portuguese stock market, Stud. Econ. Financ., 24 (2007), 220–232. https://doi.org/10.1108/10867370710817400 doi: 10.1108/10867370710817400
![]() |
[8] |
G. Duppati, A. S. Kumar, F. Scrimgeour, L. Li, Long memory volatility in Asian stock markets, Pac. Account. Rev., 29 (2017), 423–442. https://doi.org/10.1108/PAR-02-2016-0009 doi: 10.1108/PAR-02-2016-0009
![]() |
[9] |
Y. Luo, Y. Huang, A new combined approach on Hurst exponent estimate and its applications in realized volatility, Physica A, 492 (2018), 1364-1372. https://doi.org/10.1016/j.physa.2017.11.063 doi: 10.1016/j.physa.2017.11.063
![]() |
[10] |
Y. Luo, Y. Huang, Long memory or structural break? Empirical evidences from index volatility in stock market, China Financ. Rev. Int., 9 (2019), 324-337. https://doi.org/10.1108/CFRI-11-2017-0222 doi: 10.1108/CFRI-11-2017-0222
![]() |
[11] |
J. R. M. Hosking, Fractional differencing, Biometrika, 68 (1981), 165–176. https://doi.org/10.2307/2335817 doi: 10.2307/2335817
![]() |
[12] |
P. Perron, Z. Qu, Long-memory and level shifts in the volatility of stock market return indices, J. Bus. Econ. Stat., 28 (2010), 275–290. https://doi.org/10.1198/jbes.2009.06171 doi: 10.1198/jbes.2009.06171
![]() |
[13] |
P. M. Robinson, Gaussian semiparametric estimation of long range dependence, Ann. Statist., 23 (1995), 1630–1661. https://doi.org/10.1214/aos/1176324317 doi: 10.1214/aos/1176324317
![]() |
[14] |
J. Geweke, S. Porter-Hudak, The estimation and application of long memory time series models, J. Time Ser. Anal., 4 (1983), 221–238. https://doi.org/10.1111/j.1467-9892.1983.tb00371.x doi: 10.1111/j.1467-9892.1983.tb00371.x
![]() |
[15] |
C. M. Hurvich, B. K. Ray, Estimation of the memory parameter for nonstationary or noninvertible fractionally integrated processes, J. Time Ser. Anal., 16 (1995), 17–41. https://doi.org/10.1111/j.1467-9892.1995.tb00221.x doi: 10.1111/j.1467-9892.1995.tb00221.x
![]() |
[16] |
A. Ohanissian, J. R. Russell, R. S. Tsay, True or spurious long memory? A new test, J. Bus. Econ. Stat., 26 (2008), 161–175. https://doi.org/10.1198/073500107000000340 doi: 10.1198/073500107000000340
![]() |
[17] |
Z. Qu, A test against spurious long memory, J. Bus. Econ. Stat., 29 (2011), 423–438. https://doi.org/10.1198/jbes.2010.09153 doi: 10.1198/jbes.2010.09153
![]() |
[18] |
A. Jach, P. Kokoszka, Wavelet-domain test for long-range dependence in the presence of a trend, Statistics, 42 (2008), 101–113. https://doi.org/10.1080/02331880701597222 doi: 10.1080/02331880701597222
![]() |
[19] |
C. Baek, V. Pipiras, Statistical tests for a single change in mean against long-range dependence, J. Time Ser. Anal., 33 (2012), 131–151. https://doi.org/10.1111/j.1467-9892.2011.00747.x doi: 10.1111/j.1467-9892.2011.00747.x
![]() |
[20] |
A. Leccadito, O. Rachedi, G. Urga, True versus spurious long memory: Some theoretical results and a Monte Carlo comparison, Econom. Rev., 34 (2015), 452–479. https://doi.org/10.1080/07474938.2013.808462 doi: 10.1080/07474938.2013.808462
![]() |
[21] |
K. Shimotsu, P. C. B. Phillips, Exact local Whittle estimation of fractional integration, Ann. Stat., 33 (2005), 1890–1933. https://doi.org/10.1214/009053605000000309 doi: 10.1214/009053605000000309
![]() |
[22] |
F. X. Diebold, A. Inoue, Long memory and regime switching, J. Econom., 105 (2001), 131–159. https://doi.org/10.1016/S0304-4076(01)00073-2 doi: 10.1016/S0304-4076(01)00073-2
![]() |
[23] | P. C. B. Phillips, K. Shimotsu, Local Whittle estimation in nonstationary and unit root cases, Ann. Stat., 32 (2004), 656–692. Available from: http://www.jstor.org/stable/3448481. |
[24] |
K. Shimotsu, Exact local Whittle estimation of fractional integration with unknown mean and time trend, Economet. Theor., 26 (2010), 501–540. https://doi.org/10.1017/S0266466609100075 doi: 10.1017/S0266466609100075
![]() |
[25] |
T. G. Andersen, T. Bollerslev, Answering the skeptics: Yes, standard volatility models do provide accurate forecasts, Int. Econ. Rev., 39 (1998), 885–905. https://doi.org/10.2307/2527343 doi: 10.2307/2527343
![]() |
[26] |
J. Bai, P. Perron, Computation and analysis of multiple structural change models, J. Appl. Econ., 18 (2003), 1–22. https://doi.org/10.1002/jae.659 doi: 10.1002/jae.659
![]() |
[27] |
J. Bai, P. Perron, Estimating and testing linear models with multiple structural changes, Econometrica, 66 (1998), 47–78. https://doi.org/10.2307/2998540 doi: 10.2307/2998540
![]() |
[28] | J. Liu, S. Wu, J. V. Zidek, On segmented multivariate regression, Stat. Sin., 7 (1997), 497–525. Available from: https://www.jstor.org/stable/24306090. |
[29] |
Y. C. Yao, Estimating the number of change-points via Schwarz' criterion, Stat. Probab. Lett., 6 (1988), 181–189. https://doi.org/10.1016/0167-7152(88)90118-6 doi: 10.1016/0167-7152(88)90118-6
![]() |
Frequency | d=0.20 | d=0.40 | d=0.60 | d=0.80 |
T0.6 | 0.2004 [0.1240, 0.2784] |
0.4004 [0.3193, 0.4760] |
0.6121 [0.5247, 0.6854] |
0.7982 [0.7106, 0.8744] |
T0.7 | 0.2001 [0.1464, 0.2520] |
0.4002 [0.3484, 0.4491] |
0.6100 [0.5552, 0.6617] |
0.7988 [0.7425, 0.8498] |
T0.8 | 0.2110 [0.1772, 0.2434] |
0.4106 [0.3739, 0.4454] |
0.6152 [0.5806, 0.6478] |
0.8082 [0.7734, 0.8410] |
Frequency | δ1=δ2=0.2 d=0.10 |
δ1=δ2=0.4 d=0.20 |
δ1=δ2=0.6 d=0.30 |
δ1=δ2=0.8 d=0.40 |
T0.6 | 0.0160 [-0.0738, 0.0969] |
0.2888 [0.2048, 0.3649] |
0.4975 [0.4311, 0.5686] |
0.4314 [0.2726, 0.5158] |
T0.7 | 0.0582 [0.0076, 0.1123] |
0.3266 [0.2846, 0.3728] |
0.3651 [0.3280, 0.4022] |
0.3082 [0.1963, 0.3573] |
T0.8 | 0.1187 [0.0850, 0.1517] |
0.2636 [0.2382, 0.2899] |
0.2605 [0.2369, 0.2828] |
0.2217 [0.1261, 0.2560] |
Sample length | ARFIMA(0, d, 0) | ARFIMA(1, d, 0) | ARFIMA(0, d, 1) | ARFIMA(2, d, 0) | |||||
ε=0.02 | ε=0.05 | ε=0.02 | ε=0.05 | ε=0.02 | ε=0.05 | ε=0.02 | ε=0.05 | ||
5% nominal level | |||||||||
500 | W | 0.0330 | 0.0640 | 0.0170 | 0.0330 | 0.0180 | 0.0500 | 0.0760 | 0.0690 |
˜W | 0.0160 | 0.0250 | 0.0140 | 0.0250 | 0.0220 | 0.0250 | 0.0740 | 0.0660 | |
1000 | W | 0.0370 | 0.0690 | 0.0240 | 0.0480 | 0.0200 | 0.0470 | 0.0240 | 0.0350 |
˜W | 0.0230 | 0.0270 | 0.0210 | 0.0300 | 0.0260 | 0.0320 | 0.0220 | 0.0360 | |
2000 | W | 0.0260 | 0.0630 | 0.0230 | 0.0500 | 0.0180 | 0.0530 | 0.0400 | 0.0460 |
˜W | 0.0180 | 0.0260 | 0.0290 | 0.0320 | 0.0310 | 0.0280 | 0.0440 | 0.0450 | |
3000 | W | 0.0380 | 0.0730 | 0.0220 | 0.0520 | 0.0260 | 0.0590 | 0.0360 | 0.0430 |
˜W | 0.0340 | 0.0430 | 0.0310 | 0.0330 | 0.0330 | 0.0330 | 0.0340 | 0.0370 | |
5000 | W | 0.0340 | 0.0750 | 0.0300 | 0.0700 | 0.0250 | 0.0570 | 0.0500 | 0.0570 |
˜W | 0.0410 | 0.0410 | 0.0360 | 0.0370 | 0.0340 | 0.0420 | 0.0500 | 0.0570 | |
10000 | W | 0.0210 | 0.0660 | 0.0310 | 0.0720 | 0.0280 | 0.0770 | 0.0760 | 0.0690 |
˜W | 0.0300 | 0.0250 | 0.0350 | 0.0430 | 0.0390 | 0.0500 | 0.0740 | 0.0660 | |
1% nominal level | |||||||||
500 | W | 0.0060 | 0.0160 | 0.0020 | 0.0020 | 0.0010 | 0.0100 | 0.0180 | 0.0150 |
˜W | 0.0030 | 0.0040 | 0.0040 | 0.0030 | 0.0020 | 0.0010 | 0.0170 | 0.0140 | |
1000 | W | 0.0040 | 0.0190 | 0.0050 | 0.0080 | 0.0010 | 0.0120 | 0.0020 | 0.0040 |
˜W | 0.0070 | 0.0070 | 0.0030 | 0.0050 | 0.0040 | 0.0050 | 0.0030 | 0.0020 | |
2000 | W | 0.0020 | 0.0110 | 0.0020 | 0.0050 | 0.0030 | 0.0140 | 0.0110 | 0.0060 |
˜W | 0.0030 | 0.0050 | 0.0050 | 0.0040 | 0.0050 | 0.0050 | 0.0080 | 0.0050 | |
3000 | W | 0.0100 | 0.0270 | 0.0010 | 0.0080 | 0.0080 | 0.0170 | 0.0050 | 0.0060 |
˜W | 0.0100 | 0.0090 | 0.0020 | 0.0040 | 0.0100 | 0.0090 | 0.0050 | 0.0060 | |
5000 | W | 0.0030 | 0.0210 | 0.0040 | 0.0110 | 0.0050 | 0.0150 | 0.0110 | 0.0120 |
˜W | 0.0040 | 0.0040 | 0.0050 | 0.0070 | 0.0070 | 0.0070 | 0.0110 | 0.0120 | |
10000 | W | 0.0040 | 0.0160 | 0.0060 | 0.0090 | 0.0040 | 0.0180 | 0.0180 | 0.0150 |
˜W | 0.0050 | 0.0020 | 0.0050 | 0.0060 | 0.0060 | 0.0050 | 0.0170 | 0.0140 | |
Note: ε is the truncated parameter and d is the long memory parameter with a value of 0.40. |
Sample length | Test statistic | MS | WNMT | WNNMT | SRLS | ||||
ε=0.02 | ε=0.05 | ε=0.02 | ε=0.05 | ε=0.02 | ε=0.05 | ε=0.02 | ε=0.05 | ||
5% nominal level | |||||||||
500 | W | 0.7030 | 0.7690 | 0.1820 | 0.2940 | 0.0970 | 0.1770 | 0.7350 | 0.8150 |
˜W | 0.9840 | 0.9850 | 0.9650 | 0.9740 | 1.0000 | 1.0000 | 0.8820 | 0.9300 | |
1000 | W | 0.8450 | 0.8990 | 0.3370 | 0.5370 | 0.4570 | 0.7160 | 0.8780 | 0.9290 |
˜W | 0.9900 | 0.9930 | 0.9860 | 0.9880 | 1.0000 | 1.0000 | 0.9260 | 0.9620 | |
2000 | W | 0.9080 | 0.9520 | 0.6260 | 0.8590 | 0.8210 | 0.9780 | 0.9250 | 0.9620 |
˜W | 0.9970 | 0.9980 | 0.9940 | 0.9950 | 1.0000 | 1.0000 | 0.9680 | 0.9750 | |
3000 | W | 0.9230 | 0.9570 | 0.8200 | 0.9500 | 1.0000 | 1.0000 | 0.9680 | 0.9750 |
˜W | 0.9990 | 0.9990 | 0.9980 | 1.0000 | 1.0000 | 1.0000 | 0.9920 | 0.9920 | |
5000 | W | 0.9060 | 0.9390 | 0.9550 | 0.9970 | 1.0000 | 1.0000 | 0.9920 | 0.9920 |
˜W | 0.9990 | 1.0000 | 1.0000 | 1.0000 | 1.0000 | 1.0000 | 1.0000 | 1.0000 | |
10000 | W | 0.8540 | 0.9260 | 1.0000 | 1.0000 | 1.0000 | 1.0000 | 1.0000 | 1.0000 |
˜W | 1.0000 | 1.0000 | 1.0000 | 1.0000 | 1.0000 | 1.0000 | 1.0000 | 1.0000 | |
1% nominal level | |||||||||
ε=0.02 | ε=0.05 | ε=0.02 | ε=0.05 | ε=0.02 | ε=0.05 | ε=0.02 | ε=0.05 | ||
500 | W | 0.5180 | 0.5930 | 0.0550 | 0.1020 | 0.0300 | 0.0660 | 0.3010 | 0.4220 |
˜W | 0.9760 | 0.9760 | 0.9310 | 0.9450 | 1.0000 | 1.0000 | 0.4640 | 0.6620 | |
1000 | W | 0.6870 | 0.7640 | 0.1420 | 0.2960 | 0.2420 | 0.5060 | 0.4600 | 0.6600 |
˜W | 0.9840 | 0.9840 | 0.9600 | 0.9720 | 1.0000 | 1.0000 | 0.4650 | 0.6590 | |
2000 | W | 0.7530 | 0.8290 | 0.3700 | 0.6390 | 0.6050 | 0.9150 | 0.4610 | 0.6590 |
˜W | 0.9910 | 0.9940 | 0.9830 | 0.9870 | 1.0000 | 1.0000 | 0.5900 | 0.7350 | |
3000 | W | 0.7750 | 0.8380 | 0.6030 | 0.8440 | 1.0000 | 1.0000 | 0.5890 | 0.7350 |
˜W | 0.9960 | 0.9990 | 0.9920 | 0.9910 | 1.0000 | 1.0000 | 0.8040 | 0.8340 | |
5000 | W | 0.7550 | 0.8190 | 0.8720 | 0.9830 | 1.0000 | 1.0000 | 0.8040 | 0.8330 |
˜W | 0.9980 | 0.9990 | 0.9980 | 0.9950 | 1.0000 | 1.0000 | 0.9770 | 0.9530 | |
10000 | W | 0.6190 | 0.7210 | 0.9980 | 1.0000 | 1.0000 | 1.0000 | 1.0000 | 0.9950 |
˜W | 0.9960 | 0.9970 | 1.0000 | 1.0000 | 1.0000 | 1.0000 | 1.0000 | 0.9980 |
SZZS | SZCZ | HS300 | ZZ500 | PAYH | GZMT | |
Number | 4615 | 4615 | 4554 | 4125 | 4615 | 4615 |
Minimum | 7.4977×10-6 | 1.2063×10-5 | 6.6163×10-6 | 1.1616×10-5 | 0.0000 | 0.0000 |
Maximum | 0.0072 | 0.0084 | 0.0075 | 0.0084 | 0.0347 | 0.0148 |
Mean | 2.0127×10-4 | 2.6410×10-4 | 2.2985×10-4 | 2.6998×10-4 | 6.7144×10-4 | 5.0438×10-4 |
Standard Deviation | 3.9586×10-4 | 4.3842×10-4 | 4.2136×10-4 | 5.0142×10-4 | 0.0011 | 8.0451×10-4 |
Skewness | 7.8574 | 6.8873 | 7.5240 | 6.1557 | 10.2729 | 6.6943 |
Kurtosis | 95.9140 | 77.5222 | 88.2748 | 59.0801 | 227.68 | 71.3534 |
JB | 1.7075×107*** | 1.1044×107*** | 1.4228×107*** | 5.6660×106*** | 9.7883×107*** | 9.3289×106*** |
KS | 0.5000*** | 0.5000*** | 0.5000*** | 0.5000*** | 0.5000*** | 0.5000*** |
Q(5) | 5.3073×104*** | 4.8928×104*** | 5.2906×104*** | 5.0545×104*** | 2.7802×104*** | 2.9610×104*** |
Q(10) | 7.8717×104*** | 7.3454×104*** | 7.6993×104*** | 7.8002×104*** | 3.9683×104*** | 3.9131×104*** |
ADF | -29.8203*** | -29.2595*** | -29.4255*** | -26.8728*** | -35.0303*** | -33.7009*** |
d | 0.3864 | 0.3866 | 0.3842 | 0.3967 | 0.3160 | 0.3133 |
Number of structural break | 3 | 3 | 3 | 3 | 4 | 4 |
Notes: SD, JB, ADF, and Q denote standard deviation, Jarque Bera, Augmented Dickey-Fuller, and Ljung Box statistics, respectively. d denotes the estimate of differencing parameters based on 2ELW method at bandwidth m = T0.7. *, **, *** indicate significance at significance nominal level of 10%, 5%, and 1%, respectively. The sequential procedure [26] based on supFT(k+1|k) statistics is used to detect the number of structural breaks. |
Volatility series | W | ˜W | ||
ε=0.02 | ε=0.05 | ε=0.02 | ε=0.05 | |
m=T0.60 | ||||
SZZS | 0.9986 | 0.9986 | 1.2002* | 1.2002** |
SZCZ | 0.9674 | 0.9674 | 1.1180* | 1.1180* |
HS300 | 1.0138 | 1.0138 | 1.1853* | 1.1853** |
ZZ500 | 0.8654 | 0.8654 | 1.4260** | 1.4260** |
PAYH | 1.1739* | 1.1050* | 2.0732*** | 2.0732*** |
GZMT | 1.2910** | 1.2234** | 1.9436*** | 1.9436*** |
m=T0.70 | ||||
SZZS | 1.0552 | 1.0552* | 1.0849 | 1.0426* |
SZCZ | 1.0631 | 0.9826 | 1.1092 | 1.0122 |
HS300 | 1.0734 | 1.0734* | 0.9440 | 0.9440 |
ZZ500 | 1.0417 | 1.0417* | 1.4607** | 1.4607*** |
PAYH | 1.1098 | 0.6656 | 1.7682*** | 1.4232** |
GZMT | 1.0826 | 1.0826* | 1.0361 | 0.6801 |
m=T0.80 | ||||
SZZS | 0.6679 | 0.6679 | 1.1206* | 1.1206* |
SZCZ | 0.6834 | 0.6834 | 1.1995* | 1.1995** |
HS300 | 1.1420* | 1.1420* | 1.5649*** | 1.5649*** |
ZZ500 | 0.9489 | 0.9348 | 0.8272 | 0.7989 |
PAYH | 1.3974** | 1.3974** | 1.5155** | 1.5155*** |
GZMT | 1.3050** | 1.3050** | 1.5261*** | 1.5261*** |
Notes: At ε=0.02, test statistic ˜W has the critical values of 1.118, 1.252, and 1.517 at significance level of 10%, 5%, and 1%, respectively. At ε=0.05, test statistic ˜W has the critical values of 1.022, 1.155, and 1.426 at significance level of 10%, 5%, and 1%, respectively. *, **, and *** show significance at 10%, 5%, and 1%, respectively. |
Volatility series | Test statistics | Number of breaks | Date of break | Volatility mean (×10−4) |
SZZS | SupF(1|0)= 62.6673*** SupF(2|1)=30.8391*** SupF(3|2)=17.6714*** SupF(4|3)=5.3531 SupF(5|4)=5.0070 |
Seq.:3 BIC:3 LWZ:3 |
T1='2009-10-09' T2='2013-06-20' T3='2016-04-21' |
σF=2.0127 σ1=3.7182 σ2=1.2774 σ3=3.1906 σ4=0.8776 |
SZCZ | SupF(1|0)=108.8058*** SupF(2|1)=17.7389*** SupF(3|2)=15.4759*** SupF(4|3)=10.4038* SupF(5|4)=4.3863 |
Seq.:3 BIC:3 LWZ:4 |
T1='2009-10-09' T2='2013-05-28' T3='2016-03-30' |
σF=2.6410 σ1=4.3962 σ2=2.0142 σ3=3.8999 σ4=1.3998 |
HS300 | SupF(1|0)=59.9358*** SupF(2|1)=30.5248*** SupF(3|2)=13.4609** SupF(4|3)=11.2809* SupF(5|4)=0.0000 |
Seq.:3 BIC:2 LWZ:3 |
T1='2009-10-09' T2='2013-05-23' T3='2016-03-14' |
σF=2.2985 σ1=4.1579 σ2=1.6544 σ3=3.4221 σ4=1.1228 |
ZZ500 | SupF(1|0)=81.6174*** SupF(2|1)=37.9784*** SupF(3|2)=12.2999** SupF(4|3)=8.1152 SupF(5|4)=0.0000 |
Seq.:3 BIC:2 LWZ:3 |
T1='2009-09-01' T2='2013-12-11' T3='2016-06-24' |
σF=2.6998 σ1=6.7159 σ2=1.9922 σ3=4.1633 σ4=1.1999 |
PAYH | SupF(1|0)=163.5319*** SupF(2|1)=24.4941*** SupF(3|2)=23.9975*** SupF(4|3)=26.6674*** SupF(5|4)=0.0000 |
Seq.:4 BIC:4 LWZ:3 |
T1='2009-09-03' T2='2012-11-12' T3='2015-09-17' T4='2018-08-23' |
σF=6.7144 σ1=13.1276 σ2=3.7233 σ3=8.5094 σ4=2.6979 σ5=4.1498 |
GZMT | SupF(1|0)=98.7432*** SupF(2|1)=10.8309** SupF(3|2)=15.8123*** SupF(4|3)=14.3869** SupF(5|4)=0.0000 |
Seq.:4 BIC:1 LWZ:3 |
T1='2009-03-06' T2='2012-11-20' T3='2015-10-08' T4='2018-08-03' |
σF=5.0438 σ1=9.6366 σ2=3.3326 σ3=5.6520 σ4=2.6985 σ5=3.6271 |
Notes: The parameter setting, test statistic, number of structural breaks, and the estimate of location and mean for each period are shown in Column 1, 2, 3, and 4, respectively. *, **, and *** show significance at 10%, 5%, and 1%, respectively. |
Volatility series | Tm | Subsample 1 | Subsample 2 | Subsample 3 | Subsample 4 | Subsample 5 | Full sample |
T0.6 | 0.3030 | 0.3016 | 0.3878 | 0.1999 | 0.3975 | ||
SZZS | T0.7 | 0.3122 | 0.2866 | 0.6688 | 0.2065 | - | 0.3864 |
T0.8 | 0.3661 | 0.3950 | 0.5012 | 0.1898 | - | 0.4144 | |
T0.6 | 0.3041 | 0.3147 | 0.3823 | 0.2831 | - | 0.4061 | |
SZCZ | T0.7 | 0.3304 | 0.3011 | 0.4671 | 0.2599 | - | 0.3866 |
T0.8 | 0.3710 | 0.3701 | 0.4527 | 0.2560 | - | 0.4071 | |
T0.6 | 0.3252 | 0.3178 | 0.3936 | 0.2339 | - | 0.3768 | |
HS300 | T0.7 | 0.3359 | 0.3024 | 0.6616 | 0.2396 | - | 0.3842 |
T0.8 | 0.3835 | 0.3783 | 0.5240 | 0.2140 | - | 0.4350 | |
T0.6 | 0.3190 | 0.2672 | 0.4713 | 0.2729 | - | 0.4132 | |
ZZ500 | T0.7 | 0.3185 | 0.2904 | 0.4916 | 0.2404 | - | 0.3967 |
T0.8 | 0.3888 | 0.4049 | 0.4553 | 0.2350 | - | 0.4083 | |
T0.6 | 0.2289 | 0.3806 | 0.2808 | 0.3748 | 0.3463 | 0.3138 | |
PAYH | T0.7 | 0.3053 | 0.3502 | 0.4393 | 0.3932 | 0.2852 | 0.3160 |
T0.8 | 0.2302 | 0.2995 | 0.4453 | 0.4991 | 0.2760 | 0.3181 | |
T0.6 | 0.0823 | 0.5318 | 0.3038 | 0.2064 | 0.2172 | 0.2845 | |
GZMT | T0.7 | 0.2461 | 0.4197 | 0.3872 | 0.2434 | 0.2977 | 0.3133 |
T0.8 | 0.3587 | 0.3767 | 0.4558 | 0.2856 | 0.2890 | 0.3547 | |
Note: d is estimated by the 2ELW method. *, **, and *** show significance at 10%, 5%, and 1%, respectively. |
Frequency | d=0.20 | d=0.40 | d=0.60 | d=0.80 |
T0.6 | 0.2004 [0.1240, 0.2784] |
0.4004 [0.3193, 0.4760] |
0.6121 [0.5247, 0.6854] |
0.7982 [0.7106, 0.8744] |
T0.7 | 0.2001 [0.1464, 0.2520] |
0.4002 [0.3484, 0.4491] |
0.6100 [0.5552, 0.6617] |
0.7988 [0.7425, 0.8498] |
T0.8 | 0.2110 [0.1772, 0.2434] |
0.4106 [0.3739, 0.4454] |
0.6152 [0.5806, 0.6478] |
0.8082 [0.7734, 0.8410] |
Frequency | δ1=δ2=0.2 d=0.10 |
δ1=δ2=0.4 d=0.20 |
δ1=δ2=0.6 d=0.30 |
δ1=δ2=0.8 d=0.40 |
T0.6 | 0.0160 [-0.0738, 0.0969] |
0.2888 [0.2048, 0.3649] |
0.4975 [0.4311, 0.5686] |
0.4314 [0.2726, 0.5158] |
T0.7 | 0.0582 [0.0076, 0.1123] |
0.3266 [0.2846, 0.3728] |
0.3651 [0.3280, 0.4022] |
0.3082 [0.1963, 0.3573] |
T0.8 | 0.1187 [0.0850, 0.1517] |
0.2636 [0.2382, 0.2899] |
0.2605 [0.2369, 0.2828] |
0.2217 [0.1261, 0.2560] |
Sample length | ARFIMA(0, d, 0) | ARFIMA(1, d, 0) | ARFIMA(0, d, 1) | ARFIMA(2, d, 0) | |||||
ε=0.02 | ε=0.05 | ε=0.02 | ε=0.05 | ε=0.02 | ε=0.05 | ε=0.02 | ε=0.05 | ||
5% nominal level | |||||||||
500 | W | 0.0330 | 0.0640 | 0.0170 | 0.0330 | 0.0180 | 0.0500 | 0.0760 | 0.0690 |
˜W | 0.0160 | 0.0250 | 0.0140 | 0.0250 | 0.0220 | 0.0250 | 0.0740 | 0.0660 | |
1000 | W | 0.0370 | 0.0690 | 0.0240 | 0.0480 | 0.0200 | 0.0470 | 0.0240 | 0.0350 |
˜W | 0.0230 | 0.0270 | 0.0210 | 0.0300 | 0.0260 | 0.0320 | 0.0220 | 0.0360 | |
2000 | W | 0.0260 | 0.0630 | 0.0230 | 0.0500 | 0.0180 | 0.0530 | 0.0400 | 0.0460 |
˜W | 0.0180 | 0.0260 | 0.0290 | 0.0320 | 0.0310 | 0.0280 | 0.0440 | 0.0450 | |
3000 | W | 0.0380 | 0.0730 | 0.0220 | 0.0520 | 0.0260 | 0.0590 | 0.0360 | 0.0430 |
˜W | 0.0340 | 0.0430 | 0.0310 | 0.0330 | 0.0330 | 0.0330 | 0.0340 | 0.0370 | |
5000 | W | 0.0340 | 0.0750 | 0.0300 | 0.0700 | 0.0250 | 0.0570 | 0.0500 | 0.0570 |
˜W | 0.0410 | 0.0410 | 0.0360 | 0.0370 | 0.0340 | 0.0420 | 0.0500 | 0.0570 | |
10000 | W | 0.0210 | 0.0660 | 0.0310 | 0.0720 | 0.0280 | 0.0770 | 0.0760 | 0.0690 |
˜W | 0.0300 | 0.0250 | 0.0350 | 0.0430 | 0.0390 | 0.0500 | 0.0740 | 0.0660 | |
1% nominal level | |||||||||
500 | W | 0.0060 | 0.0160 | 0.0020 | 0.0020 | 0.0010 | 0.0100 | 0.0180 | 0.0150 |
˜W | 0.0030 | 0.0040 | 0.0040 | 0.0030 | 0.0020 | 0.0010 | 0.0170 | 0.0140 | |
1000 | W | 0.0040 | 0.0190 | 0.0050 | 0.0080 | 0.0010 | 0.0120 | 0.0020 | 0.0040 |
˜W | 0.0070 | 0.0070 | 0.0030 | 0.0050 | 0.0040 | 0.0050 | 0.0030 | 0.0020 | |
2000 | W | 0.0020 | 0.0110 | 0.0020 | 0.0050 | 0.0030 | 0.0140 | 0.0110 | 0.0060 |
˜W | 0.0030 | 0.0050 | 0.0050 | 0.0040 | 0.0050 | 0.0050 | 0.0080 | 0.0050 | |
3000 | W | 0.0100 | 0.0270 | 0.0010 | 0.0080 | 0.0080 | 0.0170 | 0.0050 | 0.0060 |
˜W | 0.0100 | 0.0090 | 0.0020 | 0.0040 | 0.0100 | 0.0090 | 0.0050 | 0.0060 | |
5000 | W | 0.0030 | 0.0210 | 0.0040 | 0.0110 | 0.0050 | 0.0150 | 0.0110 | 0.0120 |
˜W | 0.0040 | 0.0040 | 0.0050 | 0.0070 | 0.0070 | 0.0070 | 0.0110 | 0.0120 | |
10000 | W | 0.0040 | 0.0160 | 0.0060 | 0.0090 | 0.0040 | 0.0180 | 0.0180 | 0.0150 |
˜W | 0.0050 | 0.0020 | 0.0050 | 0.0060 | 0.0060 | 0.0050 | 0.0170 | 0.0140 | |
Note: ε is the truncated parameter and d is the long memory parameter with a value of 0.40. |
Sample length | Test statistic | MS | WNMT | WNNMT | SRLS | ||||
ε=0.02 | ε=0.05 | ε=0.02 | ε=0.05 | ε=0.02 | ε=0.05 | ε=0.02 | ε=0.05 | ||
5% nominal level | |||||||||
500 | W | 0.7030 | 0.7690 | 0.1820 | 0.2940 | 0.0970 | 0.1770 | 0.7350 | 0.8150 |
˜W | 0.9840 | 0.9850 | 0.9650 | 0.9740 | 1.0000 | 1.0000 | 0.8820 | 0.9300 | |
1000 | W | 0.8450 | 0.8990 | 0.3370 | 0.5370 | 0.4570 | 0.7160 | 0.8780 | 0.9290 |
˜W | 0.9900 | 0.9930 | 0.9860 | 0.9880 | 1.0000 | 1.0000 | 0.9260 | 0.9620 | |
2000 | W | 0.9080 | 0.9520 | 0.6260 | 0.8590 | 0.8210 | 0.9780 | 0.9250 | 0.9620 |
˜W | 0.9970 | 0.9980 | 0.9940 | 0.9950 | 1.0000 | 1.0000 | 0.9680 | 0.9750 | |
3000 | W | 0.9230 | 0.9570 | 0.8200 | 0.9500 | 1.0000 | 1.0000 | 0.9680 | 0.9750 |
˜W | 0.9990 | 0.9990 | 0.9980 | 1.0000 | 1.0000 | 1.0000 | 0.9920 | 0.9920 | |
5000 | W | 0.9060 | 0.9390 | 0.9550 | 0.9970 | 1.0000 | 1.0000 | 0.9920 | 0.9920 |
˜W | 0.9990 | 1.0000 | 1.0000 | 1.0000 | 1.0000 | 1.0000 | 1.0000 | 1.0000 | |
10000 | W | 0.8540 | 0.9260 | 1.0000 | 1.0000 | 1.0000 | 1.0000 | 1.0000 | 1.0000 |
˜W | 1.0000 | 1.0000 | 1.0000 | 1.0000 | 1.0000 | 1.0000 | 1.0000 | 1.0000 | |
1% nominal level | |||||||||
ε=0.02 | ε=0.05 | ε=0.02 | ε=0.05 | ε=0.02 | ε=0.05 | ε=0.02 | ε=0.05 | ||
500 | W | 0.5180 | 0.5930 | 0.0550 | 0.1020 | 0.0300 | 0.0660 | 0.3010 | 0.4220 |
˜W | 0.9760 | 0.9760 | 0.9310 | 0.9450 | 1.0000 | 1.0000 | 0.4640 | 0.6620 | |
1000 | W | 0.6870 | 0.7640 | 0.1420 | 0.2960 | 0.2420 | 0.5060 | 0.4600 | 0.6600 |
˜W | 0.9840 | 0.9840 | 0.9600 | 0.9720 | 1.0000 | 1.0000 | 0.4650 | 0.6590 | |
2000 | W | 0.7530 | 0.8290 | 0.3700 | 0.6390 | 0.6050 | 0.9150 | 0.4610 | 0.6590 |
˜W | 0.9910 | 0.9940 | 0.9830 | 0.9870 | 1.0000 | 1.0000 | 0.5900 | 0.7350 | |
3000 | W | 0.7750 | 0.8380 | 0.6030 | 0.8440 | 1.0000 | 1.0000 | 0.5890 | 0.7350 |
˜W | 0.9960 | 0.9990 | 0.9920 | 0.9910 | 1.0000 | 1.0000 | 0.8040 | 0.8340 | |
5000 | W | 0.7550 | 0.8190 | 0.8720 | 0.9830 | 1.0000 | 1.0000 | 0.8040 | 0.8330 |
˜W | 0.9980 | 0.9990 | 0.9980 | 0.9950 | 1.0000 | 1.0000 | 0.9770 | 0.9530 | |
10000 | W | 0.6190 | 0.7210 | 0.9980 | 1.0000 | 1.0000 | 1.0000 | 1.0000 | 0.9950 |
˜W | 0.9960 | 0.9970 | 1.0000 | 1.0000 | 1.0000 | 1.0000 | 1.0000 | 0.9980 |
SZZS | SZCZ | HS300 | ZZ500 | PAYH | GZMT | |
Number | 4615 | 4615 | 4554 | 4125 | 4615 | 4615 |
Minimum | 7.4977×10-6 | 1.2063×10-5 | 6.6163×10-6 | 1.1616×10-5 | 0.0000 | 0.0000 |
Maximum | 0.0072 | 0.0084 | 0.0075 | 0.0084 | 0.0347 | 0.0148 |
Mean | 2.0127×10-4 | 2.6410×10-4 | 2.2985×10-4 | 2.6998×10-4 | 6.7144×10-4 | 5.0438×10-4 |
Standard Deviation | 3.9586×10-4 | 4.3842×10-4 | 4.2136×10-4 | 5.0142×10-4 | 0.0011 | 8.0451×10-4 |
Skewness | 7.8574 | 6.8873 | 7.5240 | 6.1557 | 10.2729 | 6.6943 |
Kurtosis | 95.9140 | 77.5222 | 88.2748 | 59.0801 | 227.68 | 71.3534 |
JB | 1.7075×107*** | 1.1044×107*** | 1.4228×107*** | 5.6660×106*** | 9.7883×107*** | 9.3289×106*** |
KS | 0.5000*** | 0.5000*** | 0.5000*** | 0.5000*** | 0.5000*** | 0.5000*** |
Q(5) | 5.3073×104*** | 4.8928×104*** | 5.2906×104*** | 5.0545×104*** | 2.7802×104*** | 2.9610×104*** |
Q(10) | 7.8717×104*** | 7.3454×104*** | 7.6993×104*** | 7.8002×104*** | 3.9683×104*** | 3.9131×104*** |
ADF | -29.8203*** | -29.2595*** | -29.4255*** | -26.8728*** | -35.0303*** | -33.7009*** |
d | 0.3864 | 0.3866 | 0.3842 | 0.3967 | 0.3160 | 0.3133 |
Number of structural break | 3 | 3 | 3 | 3 | 4 | 4 |
Notes: SD, JB, ADF, and Q denote standard deviation, Jarque Bera, Augmented Dickey-Fuller, and Ljung Box statistics, respectively. d denotes the estimate of differencing parameters based on 2ELW method at bandwidth m = T0.7. *, **, *** indicate significance at significance nominal level of 10%, 5%, and 1%, respectively. The sequential procedure [26] based on supFT(k+1|k) statistics is used to detect the number of structural breaks. |
Volatility series | W | ˜W | ||
ε=0.02 | ε=0.05 | ε=0.02 | ε=0.05 | |
m=T0.60 | ||||
SZZS | 0.9986 | 0.9986 | 1.2002* | 1.2002** |
SZCZ | 0.9674 | 0.9674 | 1.1180* | 1.1180* |
HS300 | 1.0138 | 1.0138 | 1.1853* | 1.1853** |
ZZ500 | 0.8654 | 0.8654 | 1.4260** | 1.4260** |
PAYH | 1.1739* | 1.1050* | 2.0732*** | 2.0732*** |
GZMT | 1.2910** | 1.2234** | 1.9436*** | 1.9436*** |
m=T0.70 | ||||
SZZS | 1.0552 | 1.0552* | 1.0849 | 1.0426* |
SZCZ | 1.0631 | 0.9826 | 1.1092 | 1.0122 |
HS300 | 1.0734 | 1.0734* | 0.9440 | 0.9440 |
ZZ500 | 1.0417 | 1.0417* | 1.4607** | 1.4607*** |
PAYH | 1.1098 | 0.6656 | 1.7682*** | 1.4232** |
GZMT | 1.0826 | 1.0826* | 1.0361 | 0.6801 |
m=T0.80 | ||||
SZZS | 0.6679 | 0.6679 | 1.1206* | 1.1206* |
SZCZ | 0.6834 | 0.6834 | 1.1995* | 1.1995** |
HS300 | 1.1420* | 1.1420* | 1.5649*** | 1.5649*** |
ZZ500 | 0.9489 | 0.9348 | 0.8272 | 0.7989 |
PAYH | 1.3974** | 1.3974** | 1.5155** | 1.5155*** |
GZMT | 1.3050** | 1.3050** | 1.5261*** | 1.5261*** |
Notes: At ε=0.02, test statistic ˜W has the critical values of 1.118, 1.252, and 1.517 at significance level of 10%, 5%, and 1%, respectively. At ε=0.05, test statistic ˜W has the critical values of 1.022, 1.155, and 1.426 at significance level of 10%, 5%, and 1%, respectively. *, **, and *** show significance at 10%, 5%, and 1%, respectively. |
Volatility series | Test statistics | Number of breaks | Date of break | Volatility mean (×10−4) |
SZZS | SupF(1|0)= 62.6673*** SupF(2|1)=30.8391*** SupF(3|2)=17.6714*** SupF(4|3)=5.3531 SupF(5|4)=5.0070 |
Seq.:3 BIC:3 LWZ:3 |
T1='2009-10-09' T2='2013-06-20' T3='2016-04-21' |
σF=2.0127 σ1=3.7182 σ2=1.2774 σ3=3.1906 σ4=0.8776 |
SZCZ | SupF(1|0)=108.8058*** SupF(2|1)=17.7389*** SupF(3|2)=15.4759*** SupF(4|3)=10.4038* SupF(5|4)=4.3863 |
Seq.:3 BIC:3 LWZ:4 |
T1='2009-10-09' T2='2013-05-28' T3='2016-03-30' |
σF=2.6410 σ1=4.3962 σ2=2.0142 σ3=3.8999 σ4=1.3998 |
HS300 | SupF(1|0)=59.9358*** SupF(2|1)=30.5248*** SupF(3|2)=13.4609** SupF(4|3)=11.2809* SupF(5|4)=0.0000 |
Seq.:3 BIC:2 LWZ:3 |
T1='2009-10-09' T2='2013-05-23' T3='2016-03-14' |
σF=2.2985 σ1=4.1579 σ2=1.6544 σ3=3.4221 σ4=1.1228 |
ZZ500 | SupF(1|0)=81.6174*** SupF(2|1)=37.9784*** SupF(3|2)=12.2999** SupF(4|3)=8.1152 SupF(5|4)=0.0000 |
Seq.:3 BIC:2 LWZ:3 |
T1='2009-09-01' T2='2013-12-11' T3='2016-06-24' |
σF=2.6998 σ1=6.7159 σ2=1.9922 σ3=4.1633 σ4=1.1999 |
PAYH | SupF(1|0)=163.5319*** SupF(2|1)=24.4941*** SupF(3|2)=23.9975*** SupF(4|3)=26.6674*** SupF(5|4)=0.0000 |
Seq.:4 BIC:4 LWZ:3 |
T1='2009-09-03' T2='2012-11-12' T3='2015-09-17' T4='2018-08-23' |
σF=6.7144 σ1=13.1276 σ2=3.7233 σ3=8.5094 σ4=2.6979 σ5=4.1498 |
GZMT | SupF(1|0)=98.7432*** SupF(2|1)=10.8309** SupF(3|2)=15.8123*** SupF(4|3)=14.3869** SupF(5|4)=0.0000 |
Seq.:4 BIC:1 LWZ:3 |
T1='2009-03-06' T2='2012-11-20' T3='2015-10-08' T4='2018-08-03' |
σF=5.0438 σ1=9.6366 σ2=3.3326 σ3=5.6520 σ4=2.6985 σ5=3.6271 |
Notes: The parameter setting, test statistic, number of structural breaks, and the estimate of location and mean for each period are shown in Column 1, 2, 3, and 4, respectively. *, **, and *** show significance at 10%, 5%, and 1%, respectively. |
Volatility series | Tm | Subsample 1 | Subsample 2 | Subsample 3 | Subsample 4 | Subsample 5 | Full sample |
T0.6 | 0.3030 | 0.3016 | 0.3878 | 0.1999 | 0.3975 | ||
SZZS | T0.7 | 0.3122 | 0.2866 | 0.6688 | 0.2065 | - | 0.3864 |
T0.8 | 0.3661 | 0.3950 | 0.5012 | 0.1898 | - | 0.4144 | |
T0.6 | 0.3041 | 0.3147 | 0.3823 | 0.2831 | - | 0.4061 | |
SZCZ | T0.7 | 0.3304 | 0.3011 | 0.4671 | 0.2599 | - | 0.3866 |
T0.8 | 0.3710 | 0.3701 | 0.4527 | 0.2560 | - | 0.4071 | |
T0.6 | 0.3252 | 0.3178 | 0.3936 | 0.2339 | - | 0.3768 | |
HS300 | T0.7 | 0.3359 | 0.3024 | 0.6616 | 0.2396 | - | 0.3842 |
T0.8 | 0.3835 | 0.3783 | 0.5240 | 0.2140 | - | 0.4350 | |
T0.6 | 0.3190 | 0.2672 | 0.4713 | 0.2729 | - | 0.4132 | |
ZZ500 | T0.7 | 0.3185 | 0.2904 | 0.4916 | 0.2404 | - | 0.3967 |
T0.8 | 0.3888 | 0.4049 | 0.4553 | 0.2350 | - | 0.4083 | |
T0.6 | 0.2289 | 0.3806 | 0.2808 | 0.3748 | 0.3463 | 0.3138 | |
PAYH | T0.7 | 0.3053 | 0.3502 | 0.4393 | 0.3932 | 0.2852 | 0.3160 |
T0.8 | 0.2302 | 0.2995 | 0.4453 | 0.4991 | 0.2760 | 0.3181 | |
T0.6 | 0.0823 | 0.5318 | 0.3038 | 0.2064 | 0.2172 | 0.2845 | |
GZMT | T0.7 | 0.2461 | 0.4197 | 0.3872 | 0.2434 | 0.2977 | 0.3133 |
T0.8 | 0.3587 | 0.3767 | 0.4558 | 0.2856 | 0.2890 | 0.3547 | |
Note: d is estimated by the 2ELW method. *, **, and *** show significance at 10%, 5%, and 1%, respectively. |