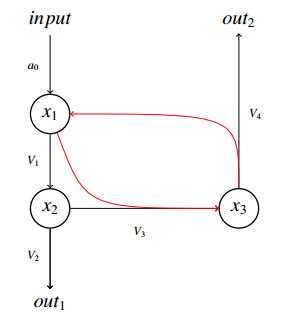
The Precursor Shutoff Valve (PSV) has been proposed as a motif in biochemical networks, active for example in prioritization of primary over secondary metabolism in plants in low-input conditions. Another branch prioritization mechanism in a biochemical network is a difference in thresholds for activation of the two pathways from the branch point. It has been shown by Adams and colleagues that both mechanisms can play a part in a model of plant metabolism involving Michaelis-Menten kinetics [
Citation: Roderick Edwards, Michelle Wood. Branch prioritization motifs in biochemical networks with sharp activation[J]. AIMS Mathematics, 2022, 7(1): 1115-1146. doi: 10.3934/math.2022066
[1] | Michal Fečkan, Július Pačuta, Michal Pospíśil, Pavol Vidlička . Averaging methods for piecewise-smooth ordinary differential equations. AIMS Mathematics, 2019, 4(5): 1466-1487. doi: 10.3934/math.2019.5.1466 |
[2] | Yike Wang, Gaoxia Wang, Ximei Hou, Fan Yang . Motif adjacency matrix and spectral clustering of directed weighted networks. AIMS Mathematics, 2023, 8(6): 13797-13814. doi: 10.3934/math.2023706 |
[3] | Marc R. Roussel, Moisés Santillán . Biochemical Problems, Mathematical Solutions. AIMS Mathematics, 2022, 7(4): 5662-5669. doi: 10.3934/math.2022313 |
[4] | Hany A. Hosham, Alaa A. Alzulaibani, Tarek Sellami, Khaled Sioud, Thoraya N. Alharthi . A class of discontinuous systems exhibit perturbed period doubling bifurcation. AIMS Mathematics, 2024, 9(9): 25098-25113. doi: 10.3934/math.20241223 |
[5] | Woocheol Choi, Young-Pil Choi . A sharp error analysis for the DG method of optimal control problems. AIMS Mathematics, 2022, 7(5): 9117-9155. doi: 10.3934/math.2022506 |
[6] | Jianwei Jiao, Keqin Su . A new Sigma-Pi-Sigma neural network based on L1 and L2 regularization and applications. AIMS Mathematics, 2024, 9(3): 5995-6012. doi: 10.3934/math.2024293 |
[7] | Tianqi Luo, Xiaohua Liu . Iteration changes discontinuity into smoothness (Ⅰ): Removable and jumping cases. AIMS Mathematics, 2023, 8(4): 8772-8792. doi: 10.3934/math.2023440 |
[8] | Xing Zhang, Lei Liu, Yan-Jun Liu . Adaptive NN control based on Butterworth low-pass filter for quarter active suspension systems with actuator failure. AIMS Mathematics, 2021, 6(1): 754-771. doi: 10.3934/math.2021046 |
[9] | Tianqi Luo, Xiaohua Liu . Iteration changes discontinuity into smoothness (Ⅱ): oscillating case. AIMS Mathematics, 2023, 8(4): 8793-8810. doi: 10.3934/math.2023441 |
[10] | Zhengqi Zhang, Huaiqin Wu . Cluster synchronization in finite/fixed time for semi-Markovian switching T-S fuzzy complex dynamical networks with discontinuous dynamic nodes. AIMS Mathematics, 2022, 7(7): 11942-11971. doi: 10.3934/math.2022666 |
The Precursor Shutoff Valve (PSV) has been proposed as a motif in biochemical networks, active for example in prioritization of primary over secondary metabolism in plants in low-input conditions. Another branch prioritization mechanism in a biochemical network is a difference in thresholds for activation of the two pathways from the branch point. It has been shown by Adams and colleagues that both mechanisms can play a part in a model of plant metabolism involving Michaelis-Menten kinetics [
Recently, Adams, Ehlting, and Edwards [1] developed a model of phenylalanine metabolism in plants that involved two mechanisms by which a plant may prioritize primary over secondary metabolism when input is low, while allowing secondary metabolism to operate at very high levels when there is sufficient input. The importance of these mechanisms lies in the fact that the input is the same for both primary and secondary metabolism, and there is a branch point in the sequences of biochemical reaction pathways, one of which leads to output (efflux) that drives primary metabolism, and the other, secondary. It is not obvious at first glance how a plant can shut down secondary metabolism but maintain primary metabolism when input is low, while allowing secondary output to be very large when input levels are high. The two mechanisms proposed by Adams and colleagues are
1. Threshold Separation, and
2. The Precursor Shutoff Valve (PSV).
While differences in thresholds are common in biochemical network models, the PSV was a new term and concept introduced in the paper by Adams and colleagues. The model for the phenylpropanoid network developed there was a reduced model from a more complex and more realistic network that tracks all the reactions in the relevant pathways, but a reduction that retains the essential structure and the gradual-but-saturated (Michaelis-Menten) nature of the reaction kinetics. Nevertheless, it consisted of a system of four nonlinear differential equations, analysis of which is not straightforward. It was shown that both mechanisms on their own contributed to the desired effect of prioritizing primary metabolism only at a low input level, but that the effect was enhanced when both mechanisms were present. In particular, thresholds for primary and secondary branches had to be widely (orders of magnitude) separated to be effective in the absence of the PSV, but much less so in the presence of the PSV, and even the PSV on its own was somewhat effective.
The simple structural nature of these mechanisms suggested that they might underlie similar behaviour in other systems with different reaction kinetics --- for example, steeper than Michaelis-Menten. On the other hand, the analysis of these highly nonlinear systems becomes difficult in high dimensions (when many variables are involved), and it would be advantageous to have a simpler class of equations that approximate the biochemical kinetics in a way that retains essential structures such as the two mechanisms involved in the phenylpropanoid network, but in a way that facilitates analysis. A first step in this direction was taken by Glass and Edwards [10], who showed that the Threshold Separation mechanism also functions in systems with infinitely steep reaction kinetics (Heaviside switches instead of Michaelis-Menten functions), related to and motivated by Glass networks, which are used as qualitative models of gene regulatory systems. The effect of the mechanism there was stronger than in Michaelis-Menten systems, such that thresholds do not have to be widely separated, but simply need to be unequal.
The idea to use step functions as approximations of steep sigmoidal reaction functions to produce qualitatively similar systems that are more tractable to analysis was first introduced by Glass and Kauffman [11,12]. The analysis of the resulting systems, called Glass networks since 2000 [7], has been extensively developed since, mainly to model gene regulatory networks (for references, see the recent review by Glass and Edwards [10]). A number of specific systems have been modelled by this approach, such as the gene networks for flower morphogenesis in Arabidopsis thaliana [17], for the initiation of sporulation in Bacillus subtilis [4], for carbon starvation response in Escherichia coli [6,8,14,21], for the yeast cell cycle [3], and for immune cell function [2]. Other examples are cited by Glass and Edwards [10]. Glass networks include a degradation term for each variable, which is appropriate in the context of gene regulation, where the molecules are large: proteins and mRNA. Glass and Edwards [10] discuss the possibility of using such analysis in models of biochemical networks that may not include degradation.
Here, we investigate the viability of the two mechanisms (Threshold Separation and the PSV) for branch prioritization in networks with steep reaction functions, but without degradation. Analysis in such systems is easier than in systems with Michaelis-Menten functions or Hill functions with higher exponents. We will show that while Threshold Separation is highly effective and sensitive in sharply-switching networks (even more so than in networks with Michaelis-Menten functions), the PSV is not at all effective in this context, adding nothing to the prioritization effect. Thus, the value of the PSV for prioritization depends on the switching being less steep, while the Threshold Separation is effective to some degree in a wider variety of systems, though the separation may have to be extreme if the switching function is not steep. It is the gradual nature of switching that is exploited by the PSV to enhance prioritization.
The work we present here is also a case study in the analysis of networks with steep activation functions that (unlike Glass networks) do not have degradation terms.
In Section 2, we present the structure we use for comparison of systems and mechanisms, the simplest structure in which both mechanisms can operate, slightly simpler than the one that occurs in the phenylpropanoid network model. This PSV motif, with or without Threshold Separation, can of course exist as a component of many larger systems. In Section 3, we extend the earlier analysis of step function systems to include the PSV, without many restrictions on parameters, and we compare the behaviour with and without each of the two mechanisms. The implications are discussed briefly in Section 4.
The simplest structure that includes the PSV motif is shown in Figure 1. This is a reduced version the phenylpropanoid network model of Adams and colleagues [1], but here we wish to consider it as a generic structure (motif) that might occur in many specific biochemical systems. The corresponding dynamical equations are
˙x1=a0−V1−V3+V4˙x2=V1−V3−V2˙x3=V3−V4 | (2.1) |
where V1 through V4 are the fluxes, which in that model were given by Michaelis-Menten functions, so that the full system could be written
˙x1=a0−a1x1K1+x1−a3x2x1(K23+x2)(K33+x1)+a4x3K4+x3˙x2=a1x1K1+x1−a3x2x1(K23+x2)(K33+x1)−a2x2K2+x2˙x3=a3x2x1(K23+x2)(K33+x1)−a4x3K4+x3 | (2.2) |
where the corresponding flux terms are written in the same positions in Equations (2.1) and (2.2), and each ai is what is usually called the maximum flux parameter for flux Vi. We assume that a0≥0 and all other parameters in (2.2) are positive. We say that x1 is the concentration of the precursor, which is upstream from the branching point at x2, but which is also required to activate the secondary pathway, by producing the compound whose concentration is x3. For brevity, we will sometimes loosely say that x1 is "the precursor", rather than "the concentration of the precursor", and say "producing xi", instead of "producing the molecule whose concentration is xi, " and the like.
In the phenylpropanoid network of Adams et al. [1], the precursor is shikimate, which produces phenylalanine via a sequence of reactions. Phenylalanine then serves as the branching point, since it is used both in producing proteins that are needed in primary metabolism, and in producing compounds that are used in the complex set of reactions for secondary metabolism. These compounds are shikimate esters, and shikimate (the precursor) is required to make this chain of reactions go, so the precursor has the ability to shut off the secondary metabolism when the shikimate ester loop is inactivated.
The model of Adams and colleagues had an additional variable that can be thought of as separating the precursor into two populations: one, x1, that participates in producing x2 and another, x4, that combines with x2 to produce x3. Shikimate exists in two cellular compartments: plastid and cytosol. The chain of reactions by which phenylalanine (x2) is produced from shikimate occurs in plastid, while the binding of shikimate to a downstream product of phenylalanine to produce shikimate esters occurs in cytosol. However, the division of shikimate molecules into two populations is not necessary in principle for the PSV to operate, so here our precursor is represented only by x1.
For comparison, the 4-dimensional model of Adams and colleagues [1] is as shown in Figure 2, where the concentrations of plastidial and cytosolic shikimate are represented by x1 and x4, respectively (we have changed the labelling from Adams and colleagues, reversing x3 and x4, V2 and V5 and corresponding parameters of those fluxes). The equations are given by
˙x1=a0−V1−V5˙x2=V1−V3−V2˙x3=V3−V4˙x4=V5−V3+V4, | (2.3) |
which are equivalent to
˙x1=a0−a1x1K1(1+bx2)+x1−a+5x1K+5+x1+a−5x4K−5+x4˙x2=a1x1K1(1+bx2)+x1−a3x2x4(K23+x2)(K33+x4)−a2x2K2+x2˙x3=a3x2x4(K23+x2)(K33+x4)−a4x3K4+x3˙x4=a+5x1K+5+x1−a−5x4K−5+x4−a3x2x4(K23+x2)(K33+x4)+a4x3K4+x3. | (2.4) |
This model had an additional parameter, b, which allowed for an inhibitory effect of phenylalanine concentration (x2) on the conversion of plastidial shikimate (x1) to phenylalanine. Adams and colleagues considered mainly the case in which b=0. They reported on simulations with b>0 to show that the essential behaviour is not changed for small positive values of b, so we will not include this in our reduced form of the PSV motif. System (2.2) is obtained from (2.4) by eliminating the fluxes between x1 and x4 and combining them into a single variable. To show that the results of Adams et al. hold in this case, one need only take K−5=K+5=K5 and a−5=a+5=a5, and for non-equilibrium situations take a5 arbitrarily large, so that shikimate moves arbitrarily rapidly between cytosolic and plasmidial pools, and acts effectively as a single pool (note that the index here was 2 and not 5 in the paper by Adams et al.).
When the PSV (the involvement of the precursor in the secondary pathway) is removed, system (2.2) becomes
˙x1=a0−V1˙x2=V1−V3−V2˙x3=V3−V4, | (2.5) |
as shown in Figure 3. With Michaelis-Menten control functions for the reactions, this becomes
˙x1=a0−a1x1K1+x1˙x2=a1x1K1+x1−a3x2K23+x2−a2x2K2+x2˙x3=a3x2K23+x2−a4x3K4+x3. | (2.6) |
Again, the biochemical context requires a0≥0 and all other parameters >0.
While this system allows for the most direct comparison to the system with the PSV, it is more complicated than necessary for the operation of the Threshold Separation motif, which depends only on the difference between thresholds for primary and secondary pathways at the branching point. Glass and Edwards [10] studied this motif in a two-dimensional context, that can be obtained from system (2.6) by removing x1 and feeding the input a0 directly into x2, since the only role of x1 now is to feed the input into x2. In fact, the Threshold Separation is really a one-variable phenomenon, acting at the branching point, so it could be reduced still further by removing x3 and considering the flux V3 as the secondary output flux (see Figure 4).
Glass and Edwards [10] show how the system
dxidt=Fi(X),i=1,2,…,N | (3.1) |
as a general framework for sharply-switching networks without degradation, relates to
dxidt=Fi(X)−γixi,i=1,2,…,N | (3.2) |
in the limit as the degradation rates γi→0, where the Fi is a scalar that depends on the Boolean State vector X (Xi=0 if xi<θi and Xi=1 if xi>θi), θi is a threshold concentration, and N is the number of chemical species. In the context of gene regulation, these species are protein products of genes, γi>0, and this is a Glass network. Each xi is bounded within the interval [0,maxX(Fi)/γi]. In more generality, if a gene regulates more than one other gene it can do so at different thresholds, so one has Xij=0 if xi<θij and Xij=1 if xi>θij. Note that Xij is not so far defined at xi=θij, but we will deal with these discontinuities by considering the sharp switching of Xij as a limit of steep but smooth sigmoidal functions, so that Xij will take values in [0,1] at xi=θij. The idea of Glass and Edwards was to consider systems without degradation, but with sharp switching, as occurs when there is high cooperativity in reactions. It is not necessary to take a limit as hypothetical degradation terms go to 0; one can simply start with Eq (3.1).
Glass and Edwards [10] use as an example a sharply-switching version of the phenylpropanoid system to explain the prioritization of primary over secondary metabolism in plants when input is low. They considered only the operation of the Threshold Separation mechanism in the sharply-switching context, showing that it was effective in prioritizing primary metabolism, as in the original Michaelis-Menten reaction system, but even more distinctly. Here, we consider the PSV mechanism in the sharply-switching context. One may anticipate that the PSV is a mechanism that could be present in a wide variety of biochemical systems, and it is desirable to determine in what types of system it is effective and in what types of system it is not.
When there is no degradation, it is possible for the system to blow up. The model of Adams, et al. is an open one: there is an input flux and there are two output fluxes. Conditions on the input in relation to other parameters in the system must be met to ensure that no variable goes to infinity (which would not be very plausible in biochemical systems). For example, the maximum output capacity must exceed the input. From the point of view of sharply-switching systems, like Glass networks, the phase space is divided into rectangular regions (boxes) bounded by the threshold values, and the vector field is piecewise linear within each box. In fact, when we have no degradation terms, the vector fields are piecewise constant within each box. Flow is unbounded if in a box where one or more variable is above its highest threshold the flow is constrained to that box and away from that highest threshold, or if the flow cycles between a set of boxes above the highest threshold of one variable such that the net movement is away from that highest threshold. Fi can be negative, though not when xi is below its lowest threshold (since it is not possible for any variable to go below 0.
The solution to system (3.1) in a given box with constant Fi is
xi(t)=xi(0)+tFi. |
If a trajectory exits the box at xi(t∗)=θik, then the time at which this occurs is given by
t∗=θik−xi(0)Fi |
and the other coordinates of the exit point are clearly
xj(t∗)=xj(0)+FjFi(θik−xi(0)). |
Trajectories in boxes are parallel, rather than converging towards a focal point, as they do in Glass networks. Maps from switching point to switching point can be calculated, but it is not possible to have a fixed point in the interior of a box. Fixed points can exist, but only in threshold domains.
Within a box, xi is increasing if Fi>0 and is decreasing if Fi<0. When Fi=0, strictly speaking xi remains fixed, but if we consider Xi or Xik to be a steep and strictly monotonic sigmoidal function, instead of a step function, then Fi will not usually be exactly zero, and the form of its dependence on Xi will determine whether it is slightly positive or slightly negative.
If there are N variables in a system, then the (N−1)-dimensional boundaries between the N-dimensional boxes in phase space are sometimes called walls, and a black wall occurs between two boxes in which the flow is directed in towards the wall from both sides [19]. It will be typical in these networks for flows to be constrained to threshold domains such as black walls, or more generally, threshold intersections. To handle flows in such domains rigorously, we must resort to Filippov solutions [13], or else singular perturbation analysis [19]. We generally prefer the latter, as it avoids possible non-uniqueness that can arise in the Filippov approach, though in most situations encountered (such as in black walls), the two methods give the same solutions. The singular perturbation approach considers the step functions as infinitely steep limits (q→0) of Hill functions:
X=H(x,θ,q)=x1/qθ1/q+x1/q. | (3.3) |
In a threshold domain in which a subset, S, of the variables are at their threshold value, and the variables in the complementary subset, ˉS, are not, we describe the fast flow of the switching variables by transforming from xs to Xs in Equation (3.1) for all s∈S, to get
dXsdτ=Xs(1−Xs)θs[Fs(XS,XˉS)], |
where XˉS∈{0,1}|ˉS| and τ=t/q is the fast time variable. When this has an asymptotically stable equilibrium X∗S∈(0,1)|S|, we can then use the fixed point to determine the dynamics of the slow variables (i∈ˉS) and obtain the sliding flow in the threshold domain:
dxidt=Fi(X∗S,XˉS). |
Full details may be found in [19], where a degradation term is always included, but this can simply be taken to be 0, as here. Note that Hill functions are natural in this context but any other class of strictly monotone sigmoidal functions with a step function limit would be expected to produce qualitatively the same results. More general sigmoids are considered in some of the literature on gene networks [11,18,20].
Figure 1 shows the system to be modelled. In the model of Adams et al., the variables x1,x2, and x3 represent concentrations of shikimate, phenylalanine, and shikimate esters, respectively. In general, x1 is the precursor, x2 feeds into both primary and secondary pathways, and x3 is a complex involving the precursor.
The switching system version of the model is:
˙x1=a0+a4X3−a1X12−a3X11X22˙x2=a1X12−a2X21−a3X11X22˙x3=a3X11X22−a4X3, | (3.4) |
where the parameter a0 represents the input flux, while a1,a2,a3, and a4 represent the maximum fluxes for each of the four fluxes in the system, V1,V2,V3, and V4. Each flux is now considered to turn on sharply when the relevant variables are above a threshold. Thus, the switching variables are X11,X12,X21,X22, and X3 where:
Xij={1if xi>θij0if xi<θij. |
We have chosen to index the thresholded variables in this way to enforce that θ11<θ12 and θ21≤θ22. That is, x1 becomes available to combine with x2 at a lower concentration than it becomes available for conversion into x2, and, as its name suggests, the primary output pathway cannot have a higher threshold than the secondary pathway (or at least the first step of the secondary pathway). The reason for the ordering of thresholds for x1 will be discussed further below, after the proof of Proposition 1.
In order for Equations (3.4) to represent a realistic biochemical system, it should not be possible for any variable to grow arbitrarily large. Since it is an open system, if the input were to exceed the maximum possible output, then unbounded growth would be inevitable. The following Proposition shows that the input must be bounded in various ways in relation to maximum flux parameters.
Proposition 1. Consider Equations (3.4) with θ11<θ12 and θ21≤θ22. If any of the following three conditions holds, then the system has unbounded growth:
● a0>a1,
● a0>a2+a3,
● a0>a2+a4.
Proof. First, note that all variables remain non-negative if initially non-negative: When x1=0, ˙x1=a0+a4X3≥0; when x2=0, ˙x2=a1X12≥0; when x3=0, ˙x3=a3X11X22≥0. Now, suppose a0>a1. Then
ddt(x1+x3)=a0−a1X12≥a0−a1>0, |
so (x1+x3)→∞ as t→∞. Since both x1 and x3 must remain non-negative, either x1→∞ or x3→∞ or both. Now suppose a0>a2+a3. Then
ddt(x1+x2+x3)=a0−a2X21−a3X11X22≥a0−(a2+a3)>0, |
so (x1+x2+x3)→∞. Finally, suppose a0>a2+a4. Then
ddt(x1+x2+2x3)=a0−a2X21−a4X3≥a0−(a2+a4)>0, |
so (x1+x2+2x3)→∞.
If a0<min{a1,a2+a3,a2+a4}, we will see below that the system is bounded when θ21<θ22, but if these are equal, another condition will be required. The situation is more complicated if θ12<θ11, where further conditions are needed to ensure boundedness. Adams and colleagues [1] found in their more complicated model that an ordering of thresholds similar to θ11<θ12 was needed to produce biologically sensible behaviour, so we do not consider the opposite ordering further here.
The following are assumptions on parameters made to reflect the functioning of realistic processes.
Assumption 1. The parameters of system (3.4) satisfy
● a0≥0 (System influx cannot be negative)
● a1,a2,a3,a4>0 (All rate parameters must be positive)
● a0<a1 (First step of the pathway must have capacity to handle maximum input)
● a0<a2+a3 (Total output from branch point must have capacity to handle maximum input)
● a0<a2+a4 (Total system output must have capacity to handle maximum input)
● θ11<θ12 (Precursor can be used to facilitate secondary pathway whenever that is active)
● θ21≤θ22 (Secondary pathway is not prioritized, or it would not be secondary)
As for Adams, et al. [1], we are interested in the ability of a system to prioritize the primary output when input is low by two mechanisms. Glass & Edwards [10] dealt with a model without the PSV, and considered the Threshold Separation mechanism by studying the system's behaviour when the thresholds for the two pathways were separated and when they were not. Here we study the system with the PSV and again consider separated thresholds and equal thresholds. The two thresholds are those for flux V2 into the primary pathway and flux V3 into the beginning of the secondary pathway. In each case, we consider low input (a0<a2) and high input (a0>a2). The goal is to locate the equilibrium in each case with each input level, and to show that it is globally stable, in order to determine which pathways are "switched on".
● The PSV model with Threshold Separation: θ21<θ22
● The PSV model without Threshold Separation: θ21=θ22=θ2
First, we deal with the separated threshold PSV structure.
The phase space is divided into regions (boxes) where each variable is above or below each of its thresholds. Let us label these boxes by a string of integers, 0, 1, or 2, given for each variable by Xi1+Xi2 for i=1 or 2, and Xi for i=3. Thus, 120 represents the box where θ11<x1<θ12, x2>θ22 and x3<θ3, or equivalently, where X11=1,X12=0,X21=1,X22=1, and X3=0. In general, abc represents the box where X11+X12=a,X21+X22=b, and X3=c.
We assume throughout that a0<min{a1,a2+a3,a2+a4} so that unbounded growth is not guaranteed by Proposition 1. The flow in each of the boxes, obtained directly from Equations (3.4), is given in Table 1. In some boxes the flow direction depends on the choice of the maximum flux rate parameters, ai for i=1,…,4, and/or the input level, a0. Note that in states 200,210 and 220 there is no ambiguity in the direction of x1 under our assumption that a0<a1.
State | Flow | ||
˙x1 | ˙x2 | ˙x3 | |
000 | a0 | 0 | 0 |
001 | a0+a4 | 0 | −a4 |
010 | a0 | −a2 | 0 |
011 | a0+a4 | −a2 | −a4 |
020 | a0 | −a2 | 0 |
021 | a0+a4 | −a2 | −a4 |
100 | a0 | 0 | 0 |
101 | a0+a4 | 0 | −a4 |
110 | a0 | −a2 | 0 |
State | Flow | ||
˙x1 | ˙x2 | ˙x3 | |
111 | a0+a4 | −a2 | −a4 |
120 | a0−a3 | −a2−a3 | a3 |
121 | a0+a4−a3 | −a2−a3 | a3−a4 |
200 | a0−a1 | a1 | 0 |
201 | a0+a4−a1 | a1 | −a4 |
210 | a0−a1 | a1−a2 | 0 |
211 | a0+a4−a1 | a1−a2 | −a4 |
220 | a0−a1−a3 | a1−a2−a3 | a3 |
221 | a0+a4−a1−a3 | a1−a2−a3 | a3−a4 |
It is clear from Table 1 that in some cases the flow in two adjacent boxes can be directed towards the boundary between them from both sides, so these are black walls. For example, in 200, x2 is increasing and some trajectories in this box will hit the wall where x2=θ21, while if a1<a2, then in 210 x2 is decreasing. Thus, if a trajectory enters the wall between these two boxes, it cannot leave the wall into either box and can only slide along the wall. If a1>a2, however, this wall is not black but transparent and the flow passes through from 200 to 210. We will use singular perturbation theory to determine the flow in black walls, assuming that the true switching is a steep Hill function, and considering the limit of infinite steepness.
In Table 2, we give the directions of flow in each box in terms of the possible "successor" states, i.e., states that the flow from a given box may enter next. Flow is directed towards one box or another, depending on where in the given box one starts, and in some cases depending on relative parameter values. The flow may enter these boxes, or may be stopped by a black wall, which is then the successor state. All of the possible successor states in each case are given in the second column, including boxes, if the flow goes through a transparent wall, or a black wall if one exists. Black walls between boxes are labelled with 12 or 32 instead of an integer for the variable that is at its threshold value, the former when xi=θi1 and the latter when xi=θi2. Thus, for example, the state 2120 is the wall between 200 and 210.
State | Successor states |
000 | 100 |
001 | 000,101 |
010 | 000,110 |
011 | 001,010,111 |
020 | 010, 1220 (if a0<a3), 120 (if a0>a3) |
021 | 011,020, 1221 (if a0<a3−a4), 121 (if a0>a3−a4) |
100 | 3200 |
101 | 100, 3201 (if a0<a1−a4), 201 (if a0>a1−a4) |
110 | 100, 3210 |
111 | 101,110, 3211 (if a0<a1−a4), 211 (if a0>a1−a4) |
120 | 110, |
1220 (if a0<a3) | |
3220 (if a0>a3) | |
1212 (if a3<a4), 121 (if a3>a4) | |
121 | 111 |
1221 (if a0<a3−a4) | |
3221 (if a3−a4<a0<a1+a3−a4), 221 (if a0>a1+a3−a4) | |
1212 (if a3<a4) | |
200 | 3200, 2120 (if a1<a2), 210 (if a1>a2) |
201 | 200, 2121 (if a1<a2), 211 (if a1>a2) |
3201 (if a0<a1−a4) | |
210 | 3210 |
2120 (if a1<a2) | |
2320 (if a2<a1<a2+a3), 220 (if a1>a2+a3) | |
211 | 210 |
3211 (if a0<a1−a4) | |
2121 (if a1<a2) | |
2321 (if a2<a1<a2+a3), 221 (if a1>a2+a3) | |
220 | 120 (if a0<a3), 3220 (if a0>a3), 2212 (if a3<a4), 221 (if a3>a4) |
2320 (if a2<a1<a2+a3), 210 (if a1<a2) | |
221 | 3221 (if a3−a4<a0<a1+a3−a4), 121 (if a0<a3−a4) |
2321 (if a2<a1<a2+a3), 211 (if a1<a2) | |
2212 (if a3<a4) |
To determine the flow within a black wall where xi=θij, we transform the equation for the variable xi into one for Xij by means of Eq (3.3), taking
Xij=x1/qiθ1/qij+x1/qi |
and considering the limit as q→0. This analysis is outlined above at the end of Section 3.1, and given more completely by Plahte and Kjøglum [19]. The equation of such a switching variable becomes
X′ij=Xij(1−Xij)θij[f(X)], |
where X is a vector of values Xkℓ, and where the derivative is with respect to the fast time variable, τ=t/q. As long as Xij converges to a fixed point in (0,1), Tikhonov's theorem applies, and we can use the equilibrium value of Xij to determine the flow of the slow variables, xk (k≠i).
As an example, consider the wall 1221, where x1=θ11, which is black in the case a0<a3−a4 (which is of course only possible if a3>a4). Then,
X′11=X11(1−X11)θ11[a0+a4X3−a1X12−a3X11X22]=X11(1−X11)θ11[a0+a4−a3X11] |
so X11→a0+a4a3∈(0,1) asymptotically (in the fast τ time). Then
˙x2=−a2−a3X11=−a2−(a0+a4),˙x3=a3X11−a4=(a0+a4)−a4=a0. | (3.5) |
Thus, x2 decreases while x3 increases and trajectories must exit the black wall at x2=θ22. At this point, both x1 and x2 are at threshold values, and we label this state 12321. A singular perturbation analysis of such threshold intersection states is then needed to determine where the flow goes from there. The newly reached threshold may simply be transparent, in which case trajectories pass through to the wall on the other side (where the first variable is still at threshold), but it may not be transparent and the first variable may no longer be constrained to its wall, so there are many possibilities, in general. The flow in all possible black walls is given in Table 3, and the successor states (threshold transition states) are given in Table 4.
State | Condition | Flow | ||
˙x1 | ˙x2 | ˙x3 | ||
1220 | a0<a3 | 0 | −a0−a2 | a0 |
1221 | a0<a3−a4 | 0 | −a0−a2−a4 | a0 |
3200 | (always) | 0 | a0 | 0 |
3201 | a0<a1−a4 | 0 | a0+a4 | −a4 |
3210 | (always) | 0 | a0−a2 | 0 |
3211 | a0<a1−a4 | 0 | a0+a4−a2 | −a4 |
3220 | a0>a3 | 0 | a0−a2−2a3 | a3 |
3221 | a3−a4<a0 | |||
<a1+a3−a4 | 0 | a0+a4−a2−2a3 | a3−a4 | |
2120 | a1<a2 | a0−a1 | 0 | 0 |
2121 | a1<a2 | a0+a4−a1 | 0 | −a4 |
2320 | a2<a1<a2+a3 | a0−2a1+a2 | 0 | a1−a2 |
2321 | a2<a1<a2+a3 | a0−2a1+a2+a4 | 0 | a1−a2−a4 |
1212 | a3<a4 | a0 | −a2−a3 | 0 |
2212 | a3<a4 | a0−a1 | a1−a2−a3 | 0 |
State | Successor states |
1220 | 12320, 12212 |
1221 | 12321 |
3200 | 32120 |
3201 | 32121, 3200 |
3210 | 32120 (if a0<a2), 32320 (if a0>a2) |
3211 | 3210, 32121 (if a0<a2−a4), 32321 (if a0>a2−a4) |
3220 | 32212 (if a3<a4), 3221 (if a3>a4), 32320 (if a0>a2), |
3210 (if a0<a2) | |
3221 | 32321 (if a0<a2+2a3−a4), 32212 (if a3<a4) |
2120 | 32120 |
2121 | 2120, 32121 (if a0<a1−a4) |
2320 | 32320, 23212 |
2321 | 32321 (if a0<2a1−a2−a4), 23212 (if a1<a2+a4) |
1212 | 32212, 13212 |
2212 | 32212, 23212 (if a1<a2+a3) |
To illustrate the analysis of the flow from the intersection of two thresholds, consider state 12321 as above. We transform both the x1 and x2 equations in terms of X11 and X22 to get
X′11=X11(1−X11)θ11[a0+a4−a3X11X22]X′22=X22(1−X22)θ22[−a2−a3X11X22] |
Clearly, X22 simply decreases here, no matter the value of X11X22, so X22→0. It is not difficult to see, then, that eventually X11 must increase, because a0+a4−a3X11X22→a0+a4>0 as X22→0. Now, X11=1 is a fixed point of the first equation above, but it can only be approached asymptotically, and similarly for X22→0, so the flow in the square (X11,X22)∈[0,1]2 asymptotically approches the corner (X11,X22)=(1,0). Thus, the trajectory emerges in the box 111 (since x1 goes up from θ11 and x2 goes down from θ22).
The situation is somewhat different in the state 32121. Tables 3 and 4 tell us that trajectories can only arrive at this threshold intersection from 3201, which is only black when a0<a1−a4 (and so a1>a4), from 3211 when a0<a2−a4, and again this wall is only black when a0<a1−a4, or from 2121 when a0<a1−a4, and this wall is only black when a1<a2. Thus, all three routes to this state require a0<a1−a4, and the third route also requires a1<a2, while the second route also requires a0<a2−a4. The fast flow in this state is given by
X′12=X12(1−X12)θ12[a0+a4−a1X12]X′21=X21(1−X21)θ21[a1X12−a2X21] |
Since a0<a1−a4, we have that X12→a0+a4a1∈(0,1). Then, (X12,X21)=(a0+a4a1,a0+a4a2) is a fixed point in (0,1)2 if a0<a2−a4 as well, and the stability of this point can be found from the Jacobian matrix:
[X12(1−X12)θ12[−a1]0X21(1−X21)θ21[a1]X21(1−X21)θ21[−a2]] | (3.6) |
which clearly has negative eigenvalues, being lower triangular with negatives on the diagonal, so the fixed point is stable, locally, but also globally, as can be seen from the flow directions in the phase portrait. See Figure 5(a).
Thus, when a0<a2−a4, the flow becomes constrained to the threshold intersection region x1=θ12,x2=θ21 and x3 evolves in slow time according to
˙x3=−a4 |
so we arrive eventually in the state 321212 where x3=θ3 also. Here, it can be shown that this state is transparent, in that X3→0, so the subsequent state is 32120. In fact, the fast flow in the triple threshold intersection is given by
X′12=X12(1−X12)θ12[a0+a4X3−a1X12]X′21=X21(1−X21)θ21[a1X12−a2X21]X′3=X3(1−X3)θ3[−a4X3] |
so X3→0, and then X12→a0a1 and X21→a0a2. Note that a0<a2−a4<a2, so X21 as well as X12 stay within the interval (0,1). In the region where x3<θ3, we will have ˙x3=0, so the trajectory does not actually drop below x3=θ3, but this is an artifact of the infinite steepness of the switching. If the switching were perturbed by any amount into a steep sigmoidal form (q>0), then x3 would continue to drop below θ3. This is indicated by the fact that X3→0, so we should consider the trajectory to enter the state 32120.
If, on the other hand, a0>a2−a4, then the flow in the domain (X12,X21)∈[0,1]2 can be determined from the nullclines and flow directions between them. The X12 nullcline is just the vertical line in the (X12,X21) plane, X12=a0+a4a1, and the X21 nullcline is the line X21=a1a2X12, but it is important to realize that X12=0 or 1 and X21=0 or 1 are nullclines, too. When a0>a2−a4, the two interior nullclines do not intersect in [0,1]2, but would intersect above it (where X21>1). An analysis of the phase portrait shows that the flow must approach the point (X12,X21)=(a0+a4a1,1) asymptotically. See Figure 5(b). Thus, the trajectory exits into the state 3211.
The possible successor states for all feasible double and triple threshold intersections are given in Table 5.
State | Successor states |
12320 | 110 |
12321 | 111 |
12212 | 1221 (if a0<a3−a4), 1212 (if a3<a4), 121 (if a0>a3−a4>0) |
13212 | 110 |
32120 | stable (if a0<a2), 3210 (if a0>a2) |
32121 | 32120 (if a0<a2−a4 and a0<a1−a4), 3211 (if a2−a4<a0<a1−a4) |
32320 | 3210 (if a0<a2), 323212 (if a0>a2) |
32321 | 323212 (if a2−a4<a0<2a3+a2−a4 and a0<2a1−a2−a4), |
3211 (if a0<a2−a4 and a0<a1−a4), | |
211 (if a0<a1−a4 and a1<a2), | |
2321 (if a2<a1<a2+a3 and a0>2a1−a2−a4), | |
221 (if a1>a2+a3 and a0>a1+a3−a4), | |
3221 (if a2+2a3−a4<a0<a1+a3−a4) | |
32212 | 323212 (if a3<a4), 3221 (if a0>a3−a4>0) |
23212 | 323212 (if a2<a1<a2+a3 and a1<a4), |
2321 (if a2+a4<a1<a2+a3), | |
210 (if a1<a2), | |
221 (if a2+a4<a2+a3<a1), | |
2212 (if a2+a3<a1 and a2+a3<a2+a4) | |
323212 | stable (if a0>a2), 3210 (if a0<a2) |
With complete information on the state transition diagram for any parameter values, we are now in a position to prove the following two results, giving first local and then global stability of a unique fixed point, a different point in the case a0<a2 than in the case a0>a2.
Proposition 2. Suppose a0<min{a1,a2+a3,a2+a4} in system (3.4). If a0<a2, the state 32120 (i.e., x1=θ12,x2=θ21,x3<θ3) is locally stable. If a0>a2, the state 323212 (i.e., x1=θ12,x2=θ22,x3=θ3) is locally stable.
Proof. The singular perturbation expansion for x1 and x2 in state 32120 is
X′12=X12(1−X12)θ12[a0−a1X12]X′21=X21(1−X21)θ21[a1X12−a2X21] |
Since a0<a1, X12→a0a1∈(0,1) and eventually, X21→a1a2X12=a0a2∈(0,1), when a0<a2. The Jacobian is again the one given in (3.6), and so the equilibrium is locally stable, and global stability within (0,1)2 is easy to ascertain from the phase portrait. Thus, once this threshold intersection is reached, it is stable in the x1 and x2 directions. Then ˙x3=0 so there is no movement in the x3 direction either. The fact that a trajectory from 32120 into any adjacent state would contradict the flow can be read in part from Table 4, where it is seen that from 3200, 3210, and 2120, the flow is into 32120. The state 1120 is a transparent wall:
X′21=X21(1−X21)θ21[−a2X21] |
so X21→0, and there is no sliding flow along this wall (either into or out of 32120). A trajectory from 32120 into any of the adjacent boxes, 100, 110, 200, and 210, contradicts the flow direction in one or both variables, from Table 2. So, from either the macroscopic or microscopic perspective, 32120 is stable. It must be noted that although Tikhonov's theorem only guarantees convergence on finite time intervals, the flow here is into the 32120 state from all adjacent regions, and this is still true for the perturbed system with q>0 sufficiently small, so no escape is possible for the perturbed system either* and the point x1=θ12,x2=θ22,x3=θ3 is genuinely locally stable.
*The finite time interval of convergence in Tikhonov's theorem becomes a problem if there is flow towards the boundary in some parts of the |S|-cube [0,1]|S|, the phase space of the fast variables, here (X12,X21). For example, there may be a saddle point as well as an asymptotically stable fixed point in the interior of this cube (or square if |S|=2), as occurs in Example 4 of [9].
The singular perturbation expansion in state 323212 is
X′12=X12(1−X12)θ12[a0+a4X3−a1X12−a3X22]X′22=X22(1−X22)θ22[a1X12−a2−a3X22]X′3=X3(1−X3)θ3[a3X22−a4X3] |
which has equilibrium (X∗12,X∗22,X∗3)=(a0a1,a0−a2a3,a0−a2a4)∈(0,1)3 when a0>a2 (note that a0<a2+a3, so a0−a2a3<1, and a0<a2+a4, so a0−a2a4<1). Letting u1=X∗12(1−X∗12)θ12, u2=X∗22(1−X∗22)θ22, and u3=X∗3(1−X∗3)θ3, the Jacobian evaluated at the equilibrium is
J∗=[−a1u1−a3u1a4u1a1u2−a3u200a3u3−a4u3] |
with characteristic equation
λ3+(a1u1+a3u2+a4u3)λ2+[a1a4u1u3+a3a4u2u3+2a1a3u1u2]λ+a1a3a4u1u2u3=0. |
Rewriting the equation as λ3+b1λ2+b2λ+b3=0, we have b1>0 and b3>0 because all ai>0 and ui>0. Furthermore, b1b2>b3 because b1b2 contains the term a1a3a4u1u2u3=b3 added to exclusively positive terms. Hence, by the Routh-Hurwitz Criterion, the fast-time system is stable at the equilibrium (X∗12,X∗22,X∗3). Again, Tikhonov's theorem only guarantees convergence on finite time intervals, but the flow is into state 323212 from all adjacent states, when a0>a2, and this is still true for q>0 sufficiently small, so no escape is possible and x1=θ12,x2=θ22,x3=θ3 is locally stable.
Note that if a0<a2, then the flow in the interior of the (X1,X2,X3) cube above is towards the point (a0a1,0,0), so the trajectory from 323212 exits into the region 3210.
Proposition 3. If a0<min{a1,a2+a3,a2+a4}, then solutions to system (3.4) are bounded. If a0<a2, the state 32120 (i.e., x1=θ12,x2=θ21,x3<θ3) is globally stable. If a0>a2, the state 323212 (i.e., x1=θ12,x2=θ22,x3=θ3) is globally stable.
Proof. First we show that, eventually, all trajectories lead to the region where x1≥θ12 and cannot then escape. From Equations (3.4), it is clear that ˙x2≤0 when x1<θ12, and the former inequality is strict when x2>θ21. Also, ˙x1>0 when x1<θ12, except possibly when x1≥θ11 and x2≥θ22. In fact, it can be deduced from Tables 4 and 5 that when x2=θ22 (i.e., when in a state ⋅32⋅), there is no possibility of a transition from a state 232⋅ or 3232⋅ to a state 1⋅⋅, so that if x2 is only equal to its second threshold and not above it, x1 cannot decrease below θ12. Thus, if x1<θ12, and x2≤θ22, then x2 cannot increase above θ22 but x1 must increase until it reaches x12. If x1<θ12 and x2>θ22 then either x2 decreases to θ22 first or x1 increases to θ12 first. In either case, x1≥θ12 will be achieved in finite time, but we must show that it cannot repeatedly drop below θ12 again, so further analysis is needed.
We can partition the system states into four subsets:
S1={x1≥θ12,x2≤θ22}={X12>0,X22<1}S2={x1≥θ12,x2>θ22}={X12>0,X22=1}S3={x1<θ12,x2>θ22}={X12=0,X22=1}S4={x1<θ12,x2≤θ22}={X12=0,X22<1}. |
We will show that although S1∪S2 is not an invariant set, it is eventually invariant in the sense that trajectories that leave must return and cannot leave again. (In fact, there is a subset of S1∪S2 that is strictly invariant, but it is easier to work with these sets.) The argument above shows that from S4 we must go to S1, but from S3 we can go to S4 or to S2.
Now, we show that to get from x1≥θ12 to x1<θ12 we must be in a state where x2>θ22. Equivalently, the only route from S1∪S2 to S3∪S4 is S2→S3. This transition is only possible if a0<a3 at least. The proof comes from inspection of Tables 2, 4 and 5, which show the only states in which x1 can decrease again below θ12 (from a state 32⋅⋅ or 2⋅⋅ to a state 1⋅⋅) is from 220 to 120 (if a0<a3) or 221 to 121 (if a0<a3−a4). In both of these cases x2>θ22 both before and after the transition, and the weakest parameter condition is a0<a3.
Next, we show that, possibly after a finite transient time, we can only get from x2≤θ22 to x2>θ22 in states where x1>θ12, or equivalently, the only way to get to S2∪S3 from S1∪S4 is from S1 to S2 and only when X12=1. This transition is only possible if a1>a2+a3 at least. This can be deduced again from the Tables, but it is clear anyway from the ˙x2 equation in (3.4) that x2 can only increase when X12>0. It is possible to go from state 32321 in S1 to either 221 or 3221 in S2 in some parameter regimes, but these all require a3<a4 (or else one of our basic conditions a0<a1 or a0<a2+a3 must be violated). If a3<a4 then whenever X3=1, x3 is decreasing, so after a finite time, x3≤θ3, and X3<1. So, after a transient, the transitions from 32321 to ⋅21 are not accessible. Thus, eventually, the only route to S2 from S1 is from states 2⋅⋅. All of these transitions require a1>a2+a3.
Going back one step further, we can only get to x2>θ12 from x2≤θ12 in S1 in states where x3>θ3, and we must have a0>a1−a4 at least. To see this, note in the first equation in (3.4) that if X22=0, and X3=0, then x1 cannot be increasing when x1>θ12, since a0<a1. However, to check threshold states, we refer again to the Tables, which show that the only transitions that lead to 2⋅⋅ from a state with x1 and x2 lower than state 2 are 101→201, 111→211, 32121 to 211, and 32321→221 or 3221, in all of which X3=1. Note that the last two were shown to be feasible only during a transient, and the others all require at least a0>a1−a4.
Let us summarize the above arguments. The flow is always from S4 to S1. From S3, the flow must also always take the system out of S3, usually to S4, though in some parameter ranges it is possible to go back to S2 as well. There can be transients involving an initial state in S1 with x1>θ12 going to S2 or initially in S2 and passing from there to S3, or if a3<a4 with x3>θ3 initially, involving the finite time it takes for x3 to reach θ3, so that X3=1 is no longer accessible. After these finite transients, the only way to transition from S2 to S3 is by means of a trajectory that passes through S1 with x1 going from θ12 or below to above θ12, then passing through S2 and then to S3. The minimum conditions for the three steps are a0>a1−a4, a1>a2+a3 and a0<a3, respectively. In fact, the last transition requires a0<a3−a4 if it goes from 221→121. But this last is inconsistent with the previous two conditions: a1>a2+a3⟹a1>a3 and then this and a0>a1−a4⟹a0>a3−a4. Under the conditions required to arrive at 221, the flow must go to 3221, and thence down to 32321. So, the only accessible route from S2 to S3 is from 220→120. Coming from states in S1 where x3>θ3 means that when we reach 220 we have x3=θ3 with X3=0 (which we can think of as being infinitesimally below the threshold x3=θ3 and thus inside the 220 box). This occurs because x3 can never decrease below θ3 once it has ever reached or exceeded it, since ˙x3≥0 when X3=0. Thus, if a trajectory subsequently reaches 220, where in principle x3 or x1 can switch next, x3 will always switch instantly, before x1 has a chance. So the subsequent state must then be 221, and the route 220→120 to S3 is closed.
Although it is possible to go from S2→S3→S2, it is not possible to go from S3→S2→S3. Thus, no cycling through S2 and S3 is possible. This can be seen by examining state transitions within S2∪S3 in all allowable cases of parameter values. See Figure 6. There are seven possible state transition diagrams, depending first on whether or not a3<a4, and secondly on the magnitute of a0 in relation to a3 and a1+a3−a4 when a3<a4, and in relation to a3 and a3−a4 when a3>a4. Note that when a3<a4, it is not possible to have a0<a3−a4, and when a3>a4, it is not possible to have a0>a1+a3−a4 (since a0<a1).
This establishes that even if initially a trajectory goes from S2 to S3, it cannot do so again. Thus, S1∪S2 is eventually invariant.
Next, we show that within S1, the set S0={x1=θ12,x2≤θ22,x3≤θ3}={0<X12<1,X22<1,X3<1} is invariant. When X22=0 and X3=0 it is clear from the first equation in (3.4) that x1 increases when X12=0 and decreases when X12=1, so the wall is black and it is not possible for x1 to leave the threshold. For threshold states in x2 and x3, inspection of Tables 4 and 5 shows that possible successor states to any state in S0, namely, 3200, 3210, 32120, 32320, or 323212, are all within S0.
All other states in S1∪S2 lead to S0. This can be seen from the Tables. If initially in S2, the flow can go immediately to S1 or to S3→S4→S1 or to S3→S2→S1. It is not possible to stay in S2. If initially in S1, then either one goes to S2 and follows one of the flows previously outlined, all of which end up at S0, or one goes directly to S0.
The final step is to see that within S0, if a0<a2, all trajectories go to the stable state 32120 and if a0>a2, all trajectories go to the stable state 323212.
Examples of the full state transition diagrams for representative choices of parameter relationships are given in Figures 7 and 8. There are many other possible choices of parameter values, of course, and for each set of parameter inequalities there is a different state transition diagram. The propositions above hold for any set of parameters that satisfy Assumption 1.
Now we consider the system with the PSV but with equal thresholds for primary and secondary branches. We will see that prioritization of primary output at low input levels cannot be achieved when relying on the PSV alone.
In Equations (3.4), we now have X21=X22, since θ21=θ22=θ2.
While Propostion 1 is, of course, still true, its converse is no longer true without including another condition.
Proposition 4. If Assumption 1 is satisfied, θ21=θ22=θ2 and a0>a4a3(a2+a3), then the flow in the state x1=θ12,x2=θ2,x3>θ3 (i.e., 32121) is unbounded.
Proof. The singular perturbation analysis of the fast time equations for the switching variables leads to
X′12=X12(1−X12)θ12[a0+a4−a1X12−a3X2]X′2=X2(1−X2)θ2[a1X12−(a2+a3)X2], |
and the nullclines in (0,1)2 intersect at
X12=(a0+a4)(a2+a3)a1(a2+2a3),X2=a0+a4a2+2a3 |
when this point is in (0,1)2. That is the case when a0+a4<a2+2a3 and (a0+a4)(a2+a3)<a1(a2+2a3), i.e., when
a0<a2+2a3−a4,anda0<a1(a2+2a3)a2+a3−a4. | (3.7) |
The state 32121 is stable if the switching variables are confined to remain at their thresholds, which is equivalent to the above condition for a stable fixed point of the fast variables in (0,1)2, and if x3 remains above its threshold, i.e., if ˙x3≥0. The flow is unbounded if ˙x3>0. This last condition is X2>a4a3, i.e., a0+a4a2+2a3>a4a3, or equivalently,
a0>a4a3(a2+a3). | (3.8) |
By Assumption 1, a0<a2+a3, so inequality (3.8) can only be achieved if a3>a4. Inequalities (3.7) and (3.8) can be simultaneously satisfied by some values of a0 if and only if a4a3(a2+a3)<a2+2a3−a4 and a4a3(a2+a3)<a1(a2+2a3)a2+a3−a4. The first of these is equivalent to (2a3+a2)(a3−a4)>0, which is true exactly when a3>a4, and the second is equivalent to a1>a4a3(a2+a3), which must be true under Assumption 1 (note that if a1<a4a3(a2+a3) but a0>a4a3(a2+a3), then a0>a1, which violates Assumption 1).
Thus, if Assumption 1 is satisfied, and a0>a4a3(a2+a3), then state 32121 is stable and x3 increases to infinity.
Thus, we now need an additional assumption to ensure bounded behaviour in the equal threshold case:
Assumption 2. a0<a4a3(a2+a3)
The analysis of the state transition diagram for all parameter conditions satisfying Assumptions 1 and 2 can be done as in the case with Threshold Separation. The calculations are done in the same manner as for the separated threshold case, but keeping in mind that in equations (3.4), we now have X21=X22, since θ21=θ22=θ2, so the state label for x2 can only be 0 (x2<θ2), 12 (x2=θ2) or 1 (x2>θ2) (or more accurately, X2=0,X2∈(0,1),X2=1).
Tables 6 and 7 show the flow and successor states for each regular domain in the case of equal thresholds.
State | Flow | ||
˙x1 | ˙x2 | ˙x3 | |
000 | a0 | 0 | 0 |
001 | a0+a4 | 0 | −a4 |
010 | a0 | −a2 | 0 |
011 | a0+a4 | −a2 | −a4 |
100 | a0 | 0 | 0 |
101 | a0+a4 | 0 | −a4 |
State | Flow | ||
˙x1 | ˙x2 | ˙x3 | |
110 | a0−a3 | −a2−a3 | a3 |
111 | a0+a4−a3 | −a2−a3 | a3−a4 |
200 | a0−a1 | a1 | 0 |
201 | a0+a4−a1 | a1 | −a4 |
210 | a0−a1−a3 | a1−a2−a3 | a3 |
211 | a0+a4−a1−a3 | a1−a2−a3 | a3−a4 |
State | Successor states |
000 | 100 |
001 | 000,101 |
010 | 000, 1210 (if a0<a3), 110 (if a0>a3) |
011 | 001,010, 1211 (if a0<a3−a4), 111 if (a0>a3−a4) |
100 | 3200 |
101 | 100, 3201 (if a0<a1−a4), 201 (if a0>a1−a4) |
110 | 100, 1112 (if a3<a4), 111 (if a3>a4) |
1210 (if a0<a3) | |
3210 (if a0>a3) | |
111 | 101 |
1211 (if a0<a3−a4) | |
1112 (if a3<a4) | |
3211 (if a3−a4<a0<a1+a3−a4), 211 (if a0>a1+a3−a4) | |
200 | 3200, 2120 (if a1<a2+a3), 210 (if a1>a2+a3) |
201 | 200, 2121 (if a1<a2+a3), 211 (if a1>a2+a3) |
3201 (if a0<a1−a4) | |
210 | 3210 (if a0>a3), 110 (if a0<a3), 2112 (if a3<a4), 211 (if a3>a4) |
2120 (if a1<a2+a3) | |
211 | 3211 (if a3−a4<a0<a1+a3−a4), 111 (if a0<a3−a4) |
2121 (if a1<a2+a3) | |
2112 (if a3<a4) |
Table 8 shows the flow for each of the potentially black walls in the equal threshold case, and the conditions under which those walls are black.
State | Condition | Flow | ||
˙x1 | ˙x2 | ˙x3 | ||
1210 | a0<a3 | 0 | −a0−a2 | a0 |
1211 | a0<a3−a4 | 0 | −a0−a2−a4 | a0 |
1112 | a3<a4 | a0 | −a2−a3 | 0 |
3200 | (always) | 0 | a0 | 0 |
3201 | a0<a1−a4 | 0 | a0+a4 | −a4 |
3210 | a0>a3 | 0 | a0−2a3−a2 | a3 |
3211 | a3−a4<a0 | |||
<a1+a3−a4 | 0 | a0+a4−a2−2a3 | a3−a4 | |
2120 | a1<a2+a3 | a0−a1−a1a3a2+a3 | 0 | a1a3a2+a3 |
2121 | a1<a2+a3 | a0+a4−a1−a1a3a2+a3 | 0 | a1a3a2+a3−a4 |
2112 | a3<a4 | a0−a1 | a1−a2−a3 | 0 |
Table 9 shows the possible successor states for each of the black walls above, under the condition that the wall is black, of course, in addition to any other conditions mentioned.
State | Successor states |
1210 | 12120 |
1211 (if a0<a3−a4), 12112 (if a0>a3−a4) | |
1211 | 12121 |
1112 | 32112, 11212 |
3200 | 32120 |
3201 | 32121, 3200 |
3210 | 32120, 32112 (if a3<a4), 3211 (if a3>a4) |
3211 | 32112 (if a3<a4), 32121 (if a0<a2+2a3−a4) |
2120 | 32120 (if a0<a1+a1a3a2+a3), |
21212 (if a4>a1a3a2+a3), 2121 (if a4<a1a3a2+a3) | |
2121 | 21212 (if a4>a1a3a2+a3), 32121 (if a0<a1−a4+a1a3a2+a3) |
2112 | 32112, 21212 (if a1<a2+a3) |
Finally, Table 10 shows the successor states to the double and triple threshold intersections that arise from the flow.
State | Successor states |
12120 | 100 |
12121 | 101 |
12112 | 1112 (if a3<a4), 1211 (if a0<a3−a4), 111 (if a0>a3−a4>0) |
11212 | 100 |
32120 | 321212 |
32121 | 321212 |
32112 | 3211 (if a3>a4), 321212 (if a3<a4) |
21212 | 321212, |
2121 (if a4a3<a1a2+a3<1) | |
321212 | stable |
The result of the analysis represented by the above Tables is that the state 321212 is globally stable under Assumptions 1 and 2. First we confirm that this state is locally stable, and then we prove that it is also globally attracting.
Proposition 5. Suppose a0<min{a1,a2+a3,a2+a4,a4a3(a2+a3)} in system (3.4). Then the state 321212 (i.e., x1=θ12,x2=θ2,x3=θ3) is locally stable.
Proof. The singular perturbation expansion in state 321212 is
X′12=X12(1−X12)θ12[a0+a4X3−a1X12−a3X2]X′2=X2(1−X2)θ2[a1X12−(a2+a3)X2]X′3=X3(1−X3)θ3[a3X2−a4X3] |
which has equilbrium (X∗12,X∗2,X∗3)=(a0a1,a0a2+a3,a0a3a4(a2+a3)), which is in (0,1)3 under Assumptions 1 and 2. Letting u1=X∗12(1−X∗12)θ12, u2=X∗2(1−X∗2)θ2, and u3=X∗3(1−X∗3)θ3, the Jacobian evaluated at the equilibrium is
J∗=[−a1u1−a3u1a4u1a1u2−(a2+a3)u200a3u3−a4u3], |
with characteristic equation
λ3+[a1u1+(a2+a3)u2]λ2+[a1a4u1u3+(a2+a3)a4u2u3+a1(a2+2a3)u1u2]λ+[a1(a2+a3)a4u1u2u3]=0, |
which is λ3+b1λ2+b2λ+b3=0 with b1,b3>0 and b1b2>b3 since b1b2 contains the term a1(a2+a3)a4u1u2u3=b3, plus other terms all positive. Thus, the Routh-Hurwitz Criterion implies that the fast-time system is stable at the equilibrium. The Tikhonov theorem allows us to conclude that solutions to the perturbed system (q>0 sufficiently small) converge to this solution on finite time intervals, but the fact that the flow is inwards to this state from all adjacent states, even for the perturbed system, guarantees that the point x1=θ12,x2=θ2,x3=θ3 is locally stable.
Proposition 6. If a0<min{a1,a2+a3,a2+a4,a4a3(a2+a3)}, then solutions to system (3.4) are bounded, and the state 321212 is globally stable.
Proof. The proof follows that of Proposition 3 very closely, except that X22 and θ22 must be replaced everywhere with X2 and θ2. We do not reproduce it all in detail again. Some parts of the proof are simpler, since there are fewer possibilities of successor states, and at the very end, it is clear that all states in S0={x1=θ12,x2≤θ2,x3≤θ3} go to state 321212, regardless of the input being low or high (but still satisfying Assumptions 1 and 2, of course).
Figures 9 and 10 show representative state transition diagrams for the equal threshold PSV system, in the low input (a0<a2) and high input (a0>a2) conditions, respectively. Note that the globally attracting steady state is the same for high and low input.
For the model in which thresholds for primary and secondary outputs are the same, the consequence of Propositions 5 and 6 is that for any feasible input level, a0 (satisfying the boundedness assumptions), X2 and X3 both go to a positive level depending linearly on a0: X2=a0a2+a3 and X3=a0a3a4(a2+a3). The primary and secondary output fluxes are therefore:
V2=a2X∗2=a0a2a2+a3,V4=a4X∗3=a0a3a2+a3 |
and thus, there is no prioritization of primary output at low input levels. If a3 is large in relation to a2, to allow large secondary output at high input levels, then secondary output flux is also large relative to primary output flux at low input levels. Note that V2+V4=a0 as it must be to balance input and output fluxes.
This is in contrast to the case with Threshold Separation, from Propositions 2 and 3, in which case the outputs depend on whether input is low (a0<a2) or high (a0>a2). In the low input case, X21 goes to a0a2, and X3 goes to 0, so primary and secondary output fluxes are
V2=a2X∗21=a0,V4=a4X∗3=0, |
while in the high input case, X21 goes to 1, and X3 goes to a0−a2a4, so the primary and secondary output fluxes are
V2=a2X∗21=a2,V4=a4X∗3=a0−a2. |
Again, note that V2+V4=a0 as necessary.
Figure 11 shows these primary and secondary fluxes in the two cases: without and with the Threshold Separation.
These fluxes can be compared to those of Glass and Edwards [10] for the sharply-switching system without the PSV. There, converting to our current notation, the fluxes were exactly as found here for the system with the PSV, both in the case of separated thresholds and equal thresholds. Thus, the flux plots in the absence of the PSV are essentially the same as in Figure 11. The final conclusion, therefore, is that for a sharply-switching system, the PSV does not help to prioritize primary output at low input levels, though the separation of thresholds for primary and secondary pathways is effective in achieving this. This is in contrast to the original Michaelis-Menten model, where the PSV was effective in prioritizing primary output at low input levels even when thresholds were equal, and improved the prioritization when thresholds were somewhat separated. In fact, here, in the sharply-switching model, the Threshold Separation mechanism can be seen to be completely effective, no matter how small the separation, since V4=0 at low input levels in that case. Including the PSV cannot improve on that. When the fluxes switch on more gradually, then threshold separation by itself must be quite extreme to effectively prioritize primary output at low input. Thus, it is only with more gradually increasing flux terms that the PSV provides an enhancement to the prioritization effect, so that thresholds need not be separated by much or at all.
Thus, one expects not to find a PSV mechanism in sharply-switching systems where branch prioritization is needed at low input. Rather, threshold separation should be expected in such cases. In systems with gradually increasing production terms, however, we may expect to find a PSV used to effect branch prioritization at low input. This is the case for the phenylpropanoid network in plants, the system that motivated consideration of the PSV, but it is likely to be a motif that appears in other contexts, although the authors are not aware yet of any other examples.
Finally, the above analysis shows how sharply-switching open systems without degradation terms can be analyzed by means of state transition diagrams and singular perturbation analysis, similar to the theory developed for Glass networks. The same method of analysis could be applied to any such biochemical network, as long as the activation functions are sharply-switching. This analysis could, in principle, be automated, so that tables of successor states and state transition diagrams could be computed for specific parameter ranges, much like is done in software used to determine the behaviour of gene networks [5,15,16]. For more gradual activating functions, like that of Michaelis-Menten kinetics, a different set of behaviours can arise, one example being the PSV motif, but analysis is more difficult. In future work, we propose to study ramp approximations of Michaelis-Menten functions as a way to simplify the analysis.
The first author is supported by grant RGPIN-2017-04042 from the Natural Sciences and Engineering Research Council (NSERC) of Canada.
All authors declare no conflicts of interest in this paper.
[1] |
Z. P. Adams, J. Ehlting, R. Edwards, The regulatory role of shikimate in plant phenylalanine metabolism, J. Theor. Biol., 462 (2019), 158–170. doi: 10.1016/j.jtbi.2018.11.005. doi: 10.1016/j.jtbi.2018.11.005
![]() |
[2] |
S. Collombet, C. van Oevelen, J. L. Sardina, W. Abou-Jaoudé, B. Di Stefano, M. Thomas-Chollier, et al., Logical modeling of lymphoid and myeloid cell specification and transdifferentiation, Proceedings of the National Academy of Sciences, 114 (2017), 5792–5799. doi: 10.1073/pnas.1610622114. doi: 10.1073/pnas.1610622114
![]() |
[3] |
M. I. Davidich, S. Bornholdt, Boolean network model predicts cell cycle sequence of fission yeast, PLoS ONE, 3 (2008), e1672. doi: 10.1371/journal.pone.0001672. doi: 10.1371/journal.pone.0001672
![]() |
[4] |
H. de Jong, J. Geiselmann, G. Batt, C. Hernandez, M. Page, Qualitative simulation of the initiation of sporulation in Bacillus subtilis, B. Math. Biol., 66 (2004), 261–299. doi: 10.1016/j.bulm.2003.08.009. doi: 10.1016/j.bulm.2003.08.009
![]() |
[5] |
H. de Jong, J. Geiselmann, C. Hernandez, M. Page, Genetic Network Analyzer: Qualitative simulation of genetic regulatory networks, Bioinformatics, 19 (2003), 336–344. doi: 10.1093/bioinformatics/btf851. doi: 10.1093/bioinformatics/btf851
![]() |
[6] |
S. Drulhe, G. Ferrari-Trecate, H. de Jong, The switching threshold reconstruction problem for piecewise-affine models of genetic regulatory networks, IEEE T. Automat. Contr., 53 (2008), 153–165. doi: 10.1109/TAC.2007.911326. doi: 10.1109/TAC.2007.911326
![]() |
[7] |
R. Edwards, Analysis of continuous-time switching networks, Physica D, 146 (2000), 165–199. doi: 10.1016/S0167-2789(00)00130-5. doi: 10.1016/S0167-2789(00)00130-5
![]() |
[8] |
R. Edwards, S. Kim, P. van den Driessche, Control design for sustained oscillation in a two gene regulatory network, J. Math. Biol., 62 (2011), 453–478. doi: 10.1007/s00285-010-0343-y. doi: 10.1007/s00285-010-0343-y
![]() |
[9] |
R. Edwards, A. Machina, G. McGregor, P. van den Driessche, A modelling framework for gene regulatory networks including transcription and translation, B. Math. Biol., 77 (2015), 953–983. doi: 10.1007/s11538-015-0073-9. doi: 10.1007/s11538-015-0073-9
![]() |
[10] |
L. Glass, R. Edwards, Hybrid models of genetic networks: Mathematical challenges and biological relevance, J. Theor. Biol., 458 (2018), 111–118. doi: 10.1016/j.jtbi.2018.09.014. doi: 10.1016/j.jtbi.2018.09.014
![]() |
[11] |
L. Glass, S.A. Kauffman, Co-operative components, spatial localization and oscillatory cellular dynamics, J. Theor. Biol., 34 (1972), 219–237. doi: 10.1016/0022-5193(72)90157-9. doi: 10.1016/0022-5193(72)90157-9
![]() |
[12] |
L. Glass, S. A. Kauffman, The logical analysis of continuous, non-linear biochemical control networks, J. Theor. Biol., 39 (1973), 103–129. doi: 10.1016/0022-5193(73)90208-7. doi: 10.1016/0022-5193(73)90208-7
![]() |
[13] |
J. L. Gouzé, T. Sari, A class of piecewise linear differential equations arising in biological models, Dynamical Systems, 17 (2002), 299–316. doi: 10.1080/1468936021000041681. doi: 10.1080/1468936021000041681
![]() |
[14] | F. Grognard, H. de Jong, J. L. Gouzé, Piecewise-linear models of genetic regulatory networks: Theory and example, in Biology and Control Theory: Current Challenges (eds. I. Queinnec, S. Tarbouriech, G. Garcia and S.I. Niculescu), Springer, 357 (2007), 137–159. doi: 10.1007/978-3-540-71988-5_7. |
[15] |
L. Ironi, L. Panzeri, A computational framework for qualitative simulation of nonlinear dynamical models of gene-regulatory networks, BMC Bioinformatics, 10 (2009), S14. doi: 10.1186/1471-2105-10-S12-S14. doi: 10.1186/1471-2105-10-S12-S14
![]() |
[16] |
L. Ironi, D. X. Tran, Nonlinear and temporal multiscale dynamics of gene regulatory networks: A qualitative simulator, Math. Comput. Simul., 125 (2016), 15–37. doi: 10.1016/j.matcom.2015.11.007. doi: 10.1016/j.matcom.2015.11.007
![]() |
[17] |
L. Mendoza, D. Thieffry, E. R. Alvarez-Buylla, Genetic control of flower morphogenesis in Arabidopsis thaliana: a logical analysis, Bioinformatics, 15 (1999), 593–606. doi: 10.1093/bioinformatics/15.7.593. doi: 10.1093/bioinformatics/15.7.593
![]() |
[18] |
T. Mestl, E. Plahte, S. W. Omholt, A mathematical framework for describing and analysing gene regulatory networks, J. Theor. Biol., 176 (1995), 291–300. doi: 10.1006/jtbi.1995.0199. doi: 10.1006/jtbi.1995.0199
![]() |
[19] |
E. Plahte, S. Kjøglum, Analysis and generic properties of gene regulatory networks with graded response functions, Physica D, 201 (2005), 150–176. doi: 10.1016/j.physd.2004.11.014. doi: 10.1016/j.physd.2004.11.014
![]() |
[20] |
E. Plahte, T. Mestl, S.W. Omholt, A methodological basis for description and analysis of systems with complex switch-like interactions, J. Math. Biol., 36 (1998), 321–348. doi: 10.1007/s002850050103. doi: 10.1007/s002850050103
![]() |
[21] |
D. Ropers, H. de Jong, M. Page, D. Schneider, J. Geiselmann, Qualitative simulation of the carbon starvation response in Escherichia coli, Biosystems, 84 (2006), 124–152. doi: 10.1016/j.biosystems.2005.10.005. doi: 10.1016/j.biosystems.2005.10.005
![]() |
1. | Skye Doré-Hall, Roderick Edwards, Ramp approximations of Michaelis–Menten functions in a model of plant metabolism, 2022, 442, 01672789, 133544, 10.1016/j.physd.2022.133544 | |
2. | Marc R. Roussel, Moisés Santillán, Biochemical Problems, Mathematical Solutions, 2022, 7, 2473-6988, 5662, 10.3934/math.2022313 | |
3. | Tamilvizhi Thanarajan, Youseef Alotaibi, Surendran Rajendran, Krishnaraj Nagappan, Improved wolf swarm optimization with deep-learning-based movement analysis and self-regulated human activity recognition, 2023, 8, 2473-6988, 12520, 10.3934/math.2023629 |
State | Flow | ||
˙x1 | ˙x2 | ˙x3 | |
000 | a0 | 0 | 0 |
001 | a0+a4 | 0 | −a4 |
010 | a0 | −a2 | 0 |
011 | a0+a4 | −a2 | −a4 |
020 | a0 | −a2 | 0 |
021 | a0+a4 | −a2 | −a4 |
100 | a0 | 0 | 0 |
101 | a0+a4 | 0 | −a4 |
110 | a0 | −a2 | 0 |
State | Flow | ||
˙x1 | ˙x2 | ˙x3 | |
111 | a0+a4 | −a2 | −a4 |
120 | a0−a3 | −a2−a3 | a3 |
121 | a0+a4−a3 | −a2−a3 | a3−a4 |
200 | a0−a1 | a1 | 0 |
201 | a0+a4−a1 | a1 | −a4 |
210 | a0−a1 | a1−a2 | 0 |
211 | a0+a4−a1 | a1−a2 | −a4 |
220 | a0−a1−a3 | a1−a2−a3 | a3 |
221 | a0+a4−a1−a3 | a1−a2−a3 | a3−a4 |
State | Successor states |
000 | 100 |
001 | 000,101 |
010 | 000,110 |
011 | 001,010,111 |
020 | 010, 1220 (if a0<a3), 120 (if a0>a3) |
021 | 011,020, 1221 (if a0<a3−a4), 121 (if a0>a3−a4) |
100 | 3200 |
101 | 100, 3201 (if a0<a1−a4), 201 (if a0>a1−a4) |
110 | 100, 3210 |
111 | 101,110, 3211 (if a0<a1−a4), 211 (if a0>a1−a4) |
120 | 110, |
1220 (if a0<a3) | |
3220 (if a0>a3) | |
1212 (if a3<a4), 121 (if a3>a4) | |
121 | 111 |
1221 (if a0<a3−a4) | |
3221 (if a3−a4<a0<a1+a3−a4), 221 (if a0>a1+a3−a4) | |
1212 (if a3<a4) | |
200 | 3200, 2120 (if a1<a2), 210 (if a1>a2) |
201 | 200, 2121 (if a1<a2), 211 (if a1>a2) |
3201 (if a0<a1−a4) | |
210 | 3210 |
2120 (if a1<a2) | |
2320 (if a2<a1<a2+a3), 220 (if a1>a2+a3) | |
211 | 210 |
3211 (if a0<a1−a4) | |
2121 (if a1<a2) | |
2321 (if a2<a1<a2+a3), 221 (if a1>a2+a3) | |
220 | 120 (if a0<a3), 3220 (if a0>a3), 2212 (if a3<a4), 221 (if a3>a4) |
2320 (if a2<a1<a2+a3), 210 (if a1<a2) | |
221 | 3221 (if a3−a4<a0<a1+a3−a4), 121 (if a0<a3−a4) |
2321 (if a2<a1<a2+a3), 211 (if a1<a2) | |
2212 (if a3<a4) |
State | Condition | Flow | ||
˙x1 | ˙x2 | ˙x3 | ||
1220 | a0<a3 | 0 | −a0−a2 | a0 |
1221 | a0<a3−a4 | 0 | −a0−a2−a4 | a0 |
3200 | (always) | 0 | a0 | 0 |
3201 | a0<a1−a4 | 0 | a0+a4 | −a4 |
3210 | (always) | 0 | a0−a2 | 0 |
3211 | a0<a1−a4 | 0 | a0+a4−a2 | −a4 |
3220 | a0>a3 | 0 | a0−a2−2a3 | a3 |
3221 | a3−a4<a0 | |||
<a1+a3−a4 | 0 | a0+a4−a2−2a3 | a3−a4 | |
2120 | a1<a2 | a0−a1 | 0 | 0 |
2121 | a1<a2 | a0+a4−a1 | 0 | −a4 |
2320 | a2<a1<a2+a3 | a0−2a1+a2 | 0 | a1−a2 |
2321 | a2<a1<a2+a3 | a0−2a1+a2+a4 | 0 | a1−a2−a4 |
1212 | a3<a4 | a0 | −a2−a3 | 0 |
2212 | a3<a4 | a0−a1 | a1−a2−a3 | 0 |
State | Successor states |
1220 | 12320, 12212 |
1221 | 12321 |
3200 | 32120 |
3201 | 32121, 3200 |
3210 | 32120 (if a0<a2), 32320 (if a0>a2) |
3211 | 3210, 32121 (if a0<a2−a4), 32321 (if a0>a2−a4) |
3220 | 32212 (if a3<a4), 3221 (if a3>a4), 32320 (if a0>a2), |
3210 (if a0<a2) | |
3221 | 32321 (if a0<a2+2a3−a4), 32212 (if a3<a4) |
2120 | 32120 |
2121 | 2120, 32121 (if a0<a1−a4) |
2320 | 32320, 23212 |
2321 | 32321 (if a0<2a1−a2−a4), 23212 (if a1<a2+a4) |
1212 | 32212, 13212 |
2212 | 32212, 23212 (if a1<a2+a3) |
State | Successor states |
12320 | 110 |
12321 | 111 |
12212 | 1221 (if a0<a3−a4), 1212 (if a3<a4), 121 (if a0>a3−a4>0) |
13212 | 110 |
32120 | stable (if a0<a2), 3210 (if a0>a2) |
32121 | 32120 (if a0<a2−a4 and a0<a1−a4), 3211 (if a2−a4<a0<a1−a4) |
32320 | 3210 (if a0<a2), 323212 (if a0>a2) |
32321 | 323212 (if a2−a4<a0<2a3+a2−a4 and a0<2a1−a2−a4), |
3211 (if a0<a2−a4 and a0<a1−a4), | |
211 (if a0<a1−a4 and a1<a2), | |
2321 (if a2<a1<a2+a3 and a0>2a1−a2−a4), | |
221 (if a1>a2+a3 and a0>a1+a3−a4), | |
3221 (if a2+2a3−a4<a0<a1+a3−a4) | |
32212 | 323212 (if a3<a4), 3221 (if a0>a3−a4>0) |
23212 | 323212 (if a2<a1<a2+a3 and a1<a4), |
2321 (if a2+a4<a1<a2+a3), | |
210 (if a1<a2), | |
221 (if a2+a4<a2+a3<a1), | |
2212 (if a2+a3<a1 and a2+a3<a2+a4) | |
323212 | stable (if a0>a2), 3210 (if a0<a2) |
State | Flow | ||
˙x1 | ˙x2 | ˙x3 | |
000 | a0 | 0 | 0 |
001 | a0+a4 | 0 | −a4 |
010 | a0 | −a2 | 0 |
011 | a0+a4 | −a2 | −a4 |
100 | a0 | 0 | 0 |
101 | a0+a4 | 0 | −a4 |
State | Flow | ||
˙x1 | ˙x2 | ˙x3 | |
110 | a0−a3 | −a2−a3 | a3 |
111 | a0+a4−a3 | −a2−a3 | a3−a4 |
200 | a0−a1 | a1 | 0 |
201 | a0+a4−a1 | a1 | −a4 |
210 | a0−a1−a3 | a1−a2−a3 | a3 |
211 | a0+a4−a1−a3 | a1−a2−a3 | a3−a4 |
State | Successor states |
000 | 100 |
001 | 000,101 |
010 | 000, 1210 (if a0<a3), 110 (if a0>a3) |
011 | 001,010, 1211 (if a0<a3−a4), 111 if (a0>a3−a4) |
100 | 3200 |
101 | 100, 3201 (if a0<a1−a4), 201 (if a0>a1−a4) |
110 | 100, 1112 (if a3<a4), 111 (if a3>a4) |
1210 (if a0<a3) | |
3210 (if a0>a3) | |
111 | 101 |
1211 (if a0<a3−a4) | |
1112 (if a3<a4) | |
3211 (if a3−a4<a0<a1+a3−a4), 211 (if a0>a1+a3−a4) | |
200 | 3200, 2120 (if a1<a2+a3), 210 (if a1>a2+a3) |
201 | 200, 2121 (if a1<a2+a3), 211 (if a1>a2+a3) |
3201 (if a0<a1−a4) | |
210 | 3210 (if a0>a3), 110 (if a0<a3), 2112 (if a3<a4), 211 (if a3>a4) |
2120 (if a1<a2+a3) | |
211 | 3211 (if a3−a4<a0<a1+a3−a4), 111 (if a0<a3−a4) |
2121 (if a1<a2+a3) | |
2112 (if a3<a4) |
State | Condition | Flow | ||
˙x1 | ˙x2 | ˙x3 | ||
1210 | a0<a3 | 0 | −a0−a2 | a0 |
1211 | a0<a3−a4 | 0 | −a0−a2−a4 | a0 |
1112 | a3<a4 | a0 | −a2−a3 | 0 |
3200 | (always) | 0 | a0 | 0 |
3201 | a0<a1−a4 | 0 | a0+a4 | −a4 |
3210 | a0>a3 | 0 | a0−2a3−a2 | a3 |
3211 | a3−a4<a0 | |||
<a1+a3−a4 | 0 | a0+a4−a2−2a3 | a3−a4 | |
2120 | a1<a2+a3 | a0−a1−a1a3a2+a3 | 0 | a1a3a2+a3 |
2121 | a1<a2+a3 | a0+a4−a1−a1a3a2+a3 | 0 | a1a3a2+a3−a4 |
2112 | a3<a4 | a0−a1 | a1−a2−a3 | 0 |
State | Successor states |
1210 | 12120 |
1211 (if a0<a3−a4), 12112 (if a0>a3−a4) | |
1211 | 12121 |
1112 | 32112, 11212 |
3200 | 32120 |
3201 | 32121, 3200 |
3210 | 32120, 32112 (if a3<a4), 3211 (if a3>a4) |
3211 | 32112 (if a3<a4), 32121 (if a0<a2+2a3−a4) |
2120 | 32120 (if a0<a1+a1a3a2+a3), |
21212 (if a4>a1a3a2+a3), 2121 (if a4<a1a3a2+a3) | |
2121 | 21212 (if a4>a1a3a2+a3), 32121 (if a0<a1−a4+a1a3a2+a3) |
2112 | 32112, 21212 (if a1<a2+a3) |
State | Successor states |
12120 | 100 |
12121 | 101 |
12112 | 1112 (if a3<a4), 1211 (if a0<a3−a4), 111 (if a0>a3−a4>0) |
11212 | 100 |
32120 | 321212 |
32121 | 321212 |
32112 | 3211 (if a3>a4), 321212 (if a3<a4) |
21212 | 321212, |
2121 (if a4a3<a1a2+a3<1) | |
321212 | stable |
State | Flow | ||
˙x1 | ˙x2 | ˙x3 | |
000 | a0 | 0 | 0 |
001 | a0+a4 | 0 | −a4 |
010 | a0 | −a2 | 0 |
011 | a0+a4 | −a2 | −a4 |
020 | a0 | −a2 | 0 |
021 | a0+a4 | −a2 | −a4 |
100 | a0 | 0 | 0 |
101 | a0+a4 | 0 | −a4 |
110 | a0 | −a2 | 0 |
State | Flow | ||
˙x1 | ˙x2 | ˙x3 | |
111 | a0+a4 | −a2 | −a4 |
120 | a0−a3 | −a2−a3 | a3 |
121 | a0+a4−a3 | −a2−a3 | a3−a4 |
200 | a0−a1 | a1 | 0 |
201 | a0+a4−a1 | a1 | −a4 |
210 | a0−a1 | a1−a2 | 0 |
211 | a0+a4−a1 | a1−a2 | −a4 |
220 | a0−a1−a3 | a1−a2−a3 | a3 |
221 | a0+a4−a1−a3 | a1−a2−a3 | a3−a4 |
State | Successor states |
000 | 100 |
001 | 000,101 |
010 | 000,110 |
011 | 001,010,111 |
020 | 010, 1220 (if a0<a3), 120 (if a0>a3) |
021 | 011,020, 1221 (if a0<a3−a4), 121 (if a0>a3−a4) |
100 | 3200 |
101 | 100, 3201 (if a0<a1−a4), 201 (if a0>a1−a4) |
110 | 100, 3210 |
111 | 101,110, 3211 (if a0<a1−a4), 211 (if a0>a1−a4) |
120 | 110, |
1220 (if a0<a3) | |
3220 (if a0>a3) | |
1212 (if a3<a4), 121 (if a3>a4) | |
121 | 111 |
1221 (if a0<a3−a4) | |
3221 (if a3−a4<a0<a1+a3−a4), 221 (if a0>a1+a3−a4) | |
1212 (if a3<a4) | |
200 | 3200, 2120 (if a1<a2), 210 (if a1>a2) |
201 | 200, 2121 (if a1<a2), 211 (if a1>a2) |
3201 (if a0<a1−a4) | |
210 | 3210 |
2120 (if a1<a2) | |
2320 (if a2<a1<a2+a3), 220 (if a1>a2+a3) | |
211 | 210 |
3211 (if a0<a1−a4) | |
2121 (if a1<a2) | |
2321 (if a2<a1<a2+a3), 221 (if a1>a2+a3) | |
220 | 120 (if a0<a3), 3220 (if a0>a3), 2212 (if a3<a4), 221 (if a3>a4) |
2320 (if a2<a1<a2+a3), 210 (if a1<a2) | |
221 | 3221 (if a3−a4<a0<a1+a3−a4), 121 (if a0<a3−a4) |
2321 (if a2<a1<a2+a3), 211 (if a1<a2) | |
2212 (if a3<a4) |
State | Condition | Flow | ||
˙x1 | ˙x2 | ˙x3 | ||
1220 | a0<a3 | 0 | −a0−a2 | a0 |
1221 | a0<a3−a4 | 0 | −a0−a2−a4 | a0 |
3200 | (always) | 0 | a0 | 0 |
3201 | a0<a1−a4 | 0 | a0+a4 | −a4 |
3210 | (always) | 0 | a0−a2 | 0 |
3211 | a0<a1−a4 | 0 | a0+a4−a2 | −a4 |
3220 | a0>a3 | 0 | a0−a2−2a3 | a3 |
3221 | a3−a4<a0 | |||
<a1+a3−a4 | 0 | a0+a4−a2−2a3 | a3−a4 | |
2120 | a1<a2 | a0−a1 | 0 | 0 |
2121 | a1<a2 | a0+a4−a1 | 0 | −a4 |
2320 | a2<a1<a2+a3 | a0−2a1+a2 | 0 | a1−a2 |
2321 | a2<a1<a2+a3 | a0−2a1+a2+a4 | 0 | a1−a2−a4 |
1212 | a3<a4 | a0 | −a2−a3 | 0 |
2212 | a3<a4 | a0−a1 | a1−a2−a3 | 0 |
State | Successor states |
1220 | 12320, 12212 |
1221 | 12321 |
3200 | 32120 |
3201 | 32121, 3200 |
3210 | 32120 (if a0<a2), 32320 (if a0>a2) |
3211 | 3210, 32121 (if a0<a2−a4), 32321 (if a0>a2−a4) |
3220 | 32212 (if a3<a4), 3221 (if a3>a4), 32320 (if a0>a2), |
3210 (if a0<a2) | |
3221 | 32321 (if a0<a2+2a3−a4), 32212 (if a3<a4) |
2120 | 32120 |
2121 | 2120, 32121 (if a0<a1−a4) |
2320 | 32320, 23212 |
2321 | 32321 (if a0<2a1−a2−a4), 23212 (if a1<a2+a4) |
1212 | 32212, 13212 |
2212 | 32212, 23212 (if a1<a2+a3) |
State | Successor states |
12320 | 110 |
12321 | 111 |
12212 | 1221 (if a0<a3−a4), 1212 (if a3<a4), 121 (if a0>a3−a4>0) |
13212 | 110 |
32120 | stable (if a0<a2), 3210 (if a0>a2) |
32121 | 32120 (if a0<a2−a4 and a0<a1−a4), 3211 (if a2−a4<a0<a1−a4) |
32320 | 3210 (if a0<a2), 323212 (if a0>a2) |
32321 | 323212 (if a2−a4<a0<2a3+a2−a4 and a0<2a1−a2−a4), |
3211 (if a0<a2−a4 and a0<a1−a4), | |
211 (if a0<a1−a4 and a1<a2), | |
2321 (if a2<a1<a2+a3 and a0>2a1−a2−a4), | |
221 (if a1>a2+a3 and a0>a1+a3−a4), | |
3221 (if a2+2a3−a4<a0<a1+a3−a4) | |
32212 | 323212 (if a3<a4), 3221 (if a0>a3−a4>0) |
23212 | 323212 (if a2<a1<a2+a3 and a1<a4), |
2321 (if a2+a4<a1<a2+a3), | |
210 (if a1<a2), | |
221 (if a2+a4<a2+a3<a1), | |
2212 (if a2+a3<a1 and a2+a3<a2+a4) | |
323212 | stable (if a0>a2), 3210 (if a0<a2) |
State | Flow | ||
˙x1 | ˙x2 | ˙x3 | |
000 | a0 | 0 | 0 |
001 | a0+a4 | 0 | −a4 |
010 | a0 | −a2 | 0 |
011 | a0+a4 | −a2 | −a4 |
100 | a0 | 0 | 0 |
101 | a0+a4 | 0 | −a4 |
State | Flow | ||
˙x1 | ˙x2 | ˙x3 | |
110 | a0−a3 | −a2−a3 | a3 |
111 | a0+a4−a3 | −a2−a3 | a3−a4 |
200 | a0−a1 | a1 | 0 |
201 | a0+a4−a1 | a1 | −a4 |
210 | a0−a1−a3 | a1−a2−a3 | a3 |
211 | a0+a4−a1−a3 | a1−a2−a3 | a3−a4 |
State | Successor states |
000 | 100 |
001 | 000,101 |
010 | 000, 1210 (if a0<a3), 110 (if a0>a3) |
011 | 001,010, 1211 (if a0<a3−a4), 111 if (a0>a3−a4) |
100 | 3200 |
101 | 100, 3201 (if a0<a1−a4), 201 (if a0>a1−a4) |
110 | 100, 1112 (if a3<a4), 111 (if a3>a4) |
1210 (if a0<a3) | |
3210 (if a0>a3) | |
111 | 101 |
1211 (if a0<a3−a4) | |
1112 (if a3<a4) | |
3211 (if a3−a4<a0<a1+a3−a4), 211 (if a0>a1+a3−a4) | |
200 | 3200, 2120 (if a1<a2+a3), 210 (if a1>a2+a3) |
201 | 200, 2121 (if a1<a2+a3), 211 (if a1>a2+a3) |
3201 (if a0<a1−a4) | |
210 | 3210 (if a0>a3), 110 (if a0<a3), 2112 (if a3<a4), 211 (if a3>a4) |
2120 (if a1<a2+a3) | |
211 | 3211 (if a3−a4<a0<a1+a3−a4), 111 (if a0<a3−a4) |
2121 (if a1<a2+a3) | |
2112 (if a3<a4) |
State | Condition | Flow | ||
˙x1 | ˙x2 | ˙x3 | ||
1210 | a0<a3 | 0 | −a0−a2 | a0 |
1211 | a0<a3−a4 | 0 | −a0−a2−a4 | a0 |
1112 | a3<a4 | a0 | −a2−a3 | 0 |
3200 | (always) | 0 | a0 | 0 |
3201 | a0<a1−a4 | 0 | a0+a4 | −a4 |
3210 | a0>a3 | 0 | a0−2a3−a2 | a3 |
3211 | a3−a4<a0 | |||
<a1+a3−a4 | 0 | a0+a4−a2−2a3 | a3−a4 | |
2120 | a1<a2+a3 | a0−a1−a1a3a2+a3 | 0 | a1a3a2+a3 |
2121 | a1<a2+a3 | a0+a4−a1−a1a3a2+a3 | 0 | a1a3a2+a3−a4 |
2112 | a3<a4 | a0−a1 | a1−a2−a3 | 0 |
State | Successor states |
1210 | 12120 |
1211 (if a0<a3−a4), 12112 (if a0>a3−a4) | |
1211 | 12121 |
1112 | 32112, 11212 |
3200 | 32120 |
3201 | 32121, 3200 |
3210 | 32120, 32112 (if a3<a4), 3211 (if a3>a4) |
3211 | 32112 (if a3<a4), 32121 (if a0<a2+2a3−a4) |
2120 | 32120 (if a0<a1+a1a3a2+a3), |
21212 (if a4>a1a3a2+a3), 2121 (if a4<a1a3a2+a3) | |
2121 | 21212 (if a4>a1a3a2+a3), 32121 (if a0<a1−a4+a1a3a2+a3) |
2112 | 32112, 21212 (if a1<a2+a3) |
State | Successor states |
12120 | 100 |
12121 | 101 |
12112 | 1112 (if a3<a4), 1211 (if a0<a3−a4), 111 (if a0>a3−a4>0) |
11212 | 100 |
32120 | 321212 |
32121 | 321212 |
32112 | 3211 (if a3>a4), 321212 (if a3<a4) |
21212 | 321212, |
2121 (if a4a3<a1a2+a3<1) | |
321212 | stable |