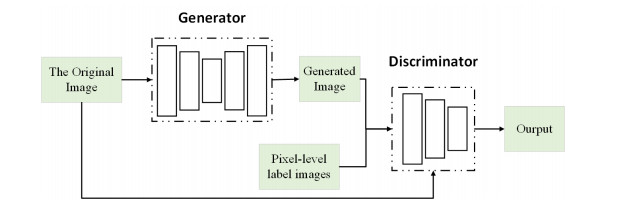
People's diversified tourism needs provide a broad development space and atmosphere for various tourism forms. The geographic resource information of the tourism unit can vividly highlight the unit's geographic spatial location and reflect the individual's spatial and attribute characteristics. It is not only the main goal of researching the information base of tourism resources, but it is also the difficulty that needs to be solved at present. This paper describes the use of image processing technology to realize the analysis and positioning of geographic tourism resources. Specifically, we propose a conditional generative adversarial network (CGAN) model, Ra-CGAN, with a multi-level channel attention mechanism. First, we built a generative model G with a multi-level channel attention mechanism. By fusing deep semantic and shallow detail information containing the attention mechanism, the network can extract rich contextual information. Second, we constructed a discriminative network D. We improved the segmentation results by correcting the difference between the ground-truth label map and the segmentation map generated by the generative model. Finally, through adversarial training between G and D with conditional constraints, we enabled high-order data distribution features learning to improve the boundary accuracy and smoothness of the segmentation results. In this study, the proposed method was validated on the large-scale remote sensing image object detection datasets DIOR and DOTA. Compared with the existing work, the method proposed in this paper achieves very good performance.
Citation: Xiuxia Li. Analysis and positioning of geographic tourism resources based on image processing method with Ra-CGAN modeling[J]. AIMS Geosciences, 2022, 8(4): 658-668. doi: 10.3934/geosci.2022036
[1] | Yaping Xu, Lei Wang, Chengliang Liu, Cuiling Liu . Identifying Unlawful Constructions in Cultural Relic Sites Based on Subpixel Mapping—a Case Study in Mangshan Tombs, China. AIMS Geosciences, 2017, 3(2): 268-283. doi: 10.3934/geosci.2017.2.268 |
[2] | Miyuru B Gunathilake, Thamashi Senerath, Upaka Rathnayake . Artificial neural network based PERSIANN data sets in evaluation of hydrologic utility of precipitation estimations in a tropical watershed of Sri Lanka. AIMS Geosciences, 2021, 7(3): 478-489. doi: 10.3934/geosci.2021027 |
[3] | Nudthawud Homtong, Wisaroot Pringproh, Kankanon Sakmongkoljit, Sattha Srikarom, Rungtiwa Yapun, Ben Wongsaijai . Remote sensing-based groundwater potential evaluation in a fractured-bedrock mountainous area. AIMS Geosciences, 2024, 10(2): 242-262. doi: 10.3934/geosci.2024014 |
[4] | Nora Tilly, Daniel Kelterbaum . Investigating the Surface and Subsurface in Karstic Regions – Terrestrial Laser Scanning versus Low-Altitude Airborne Imaging and the Combination with Geophysical Prospecting. AIMS Geosciences, 2017, 3(3): 352-374. doi: 10.3934/geosci.2017.3.352 |
[5] | Firoz Ahmad, Laxmi Goparaju, Abdul Qayum . Wild life habitat suitability and conservation hotspot mapping: Remote Sensing and GIS based decision support system. AIMS Geosciences, 2018, 4(1): 66-87. doi: 10.3934/geosci.2018.1.66 |
[6] | Sonia Malvica, Enrico Nicosia, Carmelo Maria Porto . Is the projected landscape also perceived? A proposed research plan on Etna Park's conflicting destination image. AIMS Geosciences, 2023, 9(4): 783-797. doi: 10.3934/geosci.2023042 |
[7] | Roger G. Barry . “Models in Meteorology and Climatology” Fifty Years on. AIMS Geosciences, 2017, 3(4): 552-560. doi: 10.3934/geosci.2017.4.552 |
[8] | Pingping Chen, Mingyang Qi, Long Chen . Distributed sensors and neural network driven building earthquake resistance mechanism. AIMS Geosciences, 2022, 8(4): 718-730. doi: 10.3934/geosci.2022040 |
[9] | Valentina Albanese . Global images vs cultural images: mixed methods to deepen territorial representations. AIMS Geosciences, 2022, 8(4): 593-608. doi: 10.3934/geosci.2022032 |
[10] | Brian E. Bunker, Jason A. Tullis, Jackson D. Cothren, Jesse Casana, Mohamed H. Aly . Object-based Dimensionality Reduction in Land Surface Phenology Classification. AIMS Geosciences, 2016, 2(4): 302-328. doi: 10.3934/geosci.2016.4.302 |
People's diversified tourism needs provide a broad development space and atmosphere for various tourism forms. The geographic resource information of the tourism unit can vividly highlight the unit's geographic spatial location and reflect the individual's spatial and attribute characteristics. It is not only the main goal of researching the information base of tourism resources, but it is also the difficulty that needs to be solved at present. This paper describes the use of image processing technology to realize the analysis and positioning of geographic tourism resources. Specifically, we propose a conditional generative adversarial network (CGAN) model, Ra-CGAN, with a multi-level channel attention mechanism. First, we built a generative model G with a multi-level channel attention mechanism. By fusing deep semantic and shallow detail information containing the attention mechanism, the network can extract rich contextual information. Second, we constructed a discriminative network D. We improved the segmentation results by correcting the difference between the ground-truth label map and the segmentation map generated by the generative model. Finally, through adversarial training between G and D with conditional constraints, we enabled high-order data distribution features learning to improve the boundary accuracy and smoothness of the segmentation results. In this study, the proposed method was validated on the large-scale remote sensing image object detection datasets DIOR and DOTA. Compared with the existing work, the method proposed in this paper achieves very good performance.
Tourism resources are the premise and foundation of tourism development. Most of the traditional tourism resource information is managed semi-manually. With the in-depth mining and orderly development of tourism resources, the number of tourism resources has grown rapidly. Traditional tourism resource analysis and positioning methods cannot easily meet the needs of modern management, and they have gradually exposed shortcomings such as large workloads, low efficiency and slow updating. Therefore, there is an urgent need to solve these problems. With the increasing role of tourism in the national economy, many institutions at home and abroad have developed tourism resource information databases suitable for their own needs, realizing the management of individual tourism resource information. However, most of the systems only simply collect and summarize information on individual tourism resources, and they lack geographic information related to individual tourism resources. The geographic information of the tourism unit can vividly highlight the geographic spatial location of the unit and reflect the spatial and attribute characteristics of the unit. It is not only the main goal of researching the information base of tourism resources, but it is also the difficulty that needs to be solved at present.
To develop tourism in a region, it is necessary to analyze the tourism geographic resources of the region, which is the basis for development and construction. Tourism development is a development activity based on objective conditions. Therefore, development and construction must be carried out on the basis of tourism resources. The resources of geographical tourism mainly involve two aspects: natural resources and humanities and social resources.
Natural resources refer to landscapes, surface landforms, climate environment, animal and plant resources, etc. The most important reason why certain places can be developed as tourist spots is that the area has a natural environment that is unique. At present, natural resources are still the most important elements in the development of tourism resources. An important factor for upgrading natural resources to tourism resources is that they can be admired and must be unique at the same time. Humanities and social resources are another form of tourism development resources, and they are new resources formed through long-term human practice. The so-called humanities and social resources aspect usually includes historical and cultural resources, landscape and ancient architectural resources, folk culture, social atmosphere resources and so on. Under normal circumstances, humanistic and social resources are extremely unique and irreproducible.
Remote-sensing image target detection aims to locate and identify objects of interest in remote sensing images, and it is a key technology for the intelligent interpretation of remote sensing images. As an important branch of visual target detection, remote-sensing image target detection has a wide range of uses in the fields of geographic tourism resource analysis, natural disaster detection, military reconnaissance and urban planning. The purpose of this paper was to use advanced image processing technology to realize automatic analysis and the positioning of geographic tourism resources.
In recent years, with the rapid development of deep neural network technology, the improvement of computer parallel computing capabilities and the emergence of large-scale labeled datasets, a series of advanced deep neural network-based remote-sensing image target detection algorithms have been developed [12]. However, due to the repeated convolution and pooling operations in the deep convolutional network model, upsampling cannot completely compensate for the resulting information loss problem, so the resulting prediction results are relatively rough, the small target information is lost and the target edge information is difficult to extract. At the same time, the robustness of the network needs to be further improved.
To address this challenge, generative adversarial networks (GANs) were introduced. GANs are a research hotspot in the field of computer vision. They contain two training models, one is a generative model (G), which is used to obtain the data distribution. The other is the discriminative model (D), which is used to estimate that the samples belong to the training data rather than the data generated by G. Through iterative adversarial training between G and D, the data generated by G can infinitely approximate the distribution of real sample data, and D cannot tell whether the input is real sample data or data generated by G to ensure that the model reaches the optimal state. Since GANs do not assume the distribution law of sample data, when the sample data dimension is high and the distribution is complex, the training of GANs becomes difficult to control. Thus, the conditional GAN (CGAN) was born.
Therefore, this paper proposes a CGAN remote-sensing image object detection algorithm (Ra-CGAN) with a multi-level channel attention mechanism to realize the analysis and positioning of geographic tourism resources. The model proposed in this paper includes a generative network G and a discriminative network D. The generation network G is a segmentation model with a multi-level channel attention mechanism. It builds a channel attention mechanism through self-learning, so that each layer feature highlights useful information for the task, suppresses useless information and fully integrates the corresponding layers in the encoder and decoder. It also contains shallow local information and deep semantic information of attention to enhance the informativeness of features at each scale. It provides more accurate modeling of remote sensing data distribution through adversarial training of G and D. Compared with other methods, the method in this paper pays more attention to the high-order spatial consistency of the data, and the edge details of the segmentation result map are smoother and more complete, which improves the performance of the model.
The rapid development of deep learning and the emergence of large-scale remote sensing datasets [12] have ushered in a leap forward in the development of remote-sensing image target detection. Inspired and influenced by the region-based convolutional neural network (R-CNN) target detection framework, deep learning has gradually begun to develop in the field of remote-sensing image target detection. A remote-sensing image target detection algorithm has been proposed. Cheng et al. [3] designed a rotation-invariant layer based on the R-CNN framework to achieve rotation-invariant detection of remote-sensing image targets. The authors of [4] designed a score-based unsupervised boundary regression based on an R-CNN to achieve more accurate target localization in remote sensing imaging. Cheng et al. [5] significantly improved the rotation invariance and discriminative performance of CNN features by designing rotation invariance and Fisher discriminant constraints on CNN features.
In 2015, the emergence of the Faster R-CNN provided the basis for faster and more accurate object detection in remote sensing imaging. Inspired by the Faster R-CNN and region proposal network (RPN), Deng et al. [6] designed a region generation network and a vehicle location and attribute prediction network for vehicle detection in remote sensing images. The authors of [7] solved the problem of target rotation and deformation in remote sensing images by designing a rotation-invariant RPN. Zhang et al. [8] designed a scale-adaptive remote-sensing image target detection region generation network according to the characteristics of remote-sensing image targets. Cheng et al. [9] introduced cross-scale connections and attention mechanisms on the Faster R-CNN framework with an FPN structure to improve the discriminability of features. The authors of [10] improved the performance of remote-sensing image object detection by designing a feature attention and adaptive multi-receptive field mechanism.
GANs are one of the most promising types of semi-supervised methods developed in recent years; they train models by generating adversarial methods. Jin et al. [11] used a deep convolutional GAN to extract residential areas in remote sensing images. Compared with traditional methods, the overall accuracy was high, and the outlines of residential areas were more regular. The Attention GAN [12] method applies the attention mechanism to a GAN for remote-sensing image scene classification, and it obtains good results on multiple datasets, but the generator lacks feature fusion, and the context information extraction is not sufficient. Using dilated convolution brings a large amount of computation. The above results show that the introduction of GANs to the semantic segmentation task can enhance the spatial long-distance continuity, resulting in more accurate and smoother results compared to non-adversarial training. However, generative models still use lower-level features to generate segmentation maps, and in the face of complex remote sensing targets, the ability to extract features needs to be further improved. Therefore, in the field of remote sensing, there are insufficient label samples, difficulty in manual labeling and difficulty in extracting features with strong discriminative power. This paper describes the use of a GAN to design a remote-sensing image target detection algorithm.
The Ra-CGAN model consists of two sub-networks, namely, the generative network and the discriminative network, and the model structure is shown in Figure 1. Generating network G inputs raw images and pixel-level label images. The input of the discriminative network D has two combinations, where one is the combination of the original image and the image generated by the generative network, and the other is the combination of the original image and the pixel-level label image. During training, the original and pixel-level labeled images are used as positive samples and the original and generated images are used as negative samples.
The generator is a segmentation model with a multi-level channel attention mechanism, which mainly includes three components, namely, the encoder, the multi-level channel attention module and the decoder. These three components are used for feature extraction, feature fusion and class prediction, respectively. The encoder includes five sets of convolutional blocks. Each group of convolutional blocks consists of two convolutional layers with a kernel size of 3, a batch normalization layer and a ReLU activation function. In order to enhance the network's acquisition of complex background target information, a channel attention layer has been added. The channel attention layer automatically obtains the importance of each feature channel through self-learning. Then, according to this importance, useful features are boosted and features that are not useful for the current task are suppressed. Finally, a max-pooling layer with a size of 2 and a stride of 2 is used for feature dimensionality reduction, and the size of the feature map is reduced in turn. The decoder restores the original size of the feature map and generates a predicted image with the same resolution as the input image. At the same time, the depth of the feature layer is reduced, and a deconvolution layer with a step size of 2 and a convolutional kernel size of 2 is set in turn. Finally, in order to further improve the network's acquisition of multi-scale target edge information, a feature map containing a channel attention mechanism is used to perform multi-level skip links. The information obtained by deconvolution is fused at each layer to enrich global semantic information and local detail information.
The channel attention module [13] is the attention layer. First, the feature map U obtained by the convolution operation is globally average pooled according to the spatial dimension. Each 2-dimensional feature channel becomes a real number. This real number has a global receptive field, and the dimension of the output matches the number of feature channels of the input. Second, in order to fully obtain the dynamic and nonlinear dependencies between channels, limit the complexity of the model and allow the network to update the channel weights spontaneously, two fully connected layers have been introduced. The role of the two fully connected layers is to fuse the feature information of each channel. Finally, the extracted channel weights are weighted to each channel of the previous feature map to obtain the feature map Ua with a channel attention mechanism.
The input of the discriminator is the mosaic of the original image and the segmentation image or the label image in the channel dimension. Doing so better preserves the original features of the samples. Maximum pooling layers are generally not used in GAN discriminators. Because the gradient provided after pooling is sparse, it is not conducive to guide the learning of the generator. A good discriminator not only has strong classification ability, but it also can provide more information to the generator. The activation function in this work uses a LeakyReLu to solve the problem of gradient disappearance that may be caused by a ReLu. The ReLu function will truncate the negative value to 0, and the LeakyReLu function will not be 0 when the input is a negative value, allowing a small negative value to pass. Since the gradient of the discriminator is particularly important for the generator, in the discriminator, a LeakyReLu is used instead of a ReLu, and strided convolution is used instead of a max pooling layer.
The loss function of the Ra-CGAN model is a hybrid loss function, which is defined as
l(θG,θD)=N∑n=1lcrossG(G(xn),yn)−λ[lcrossD(D(xn,yn),1)+lcrossD(D(xn,G(xn)),0)] | (1) |
In the formula, N represents the number of training images xn. yn represents the corresponding label image. θG and θD represent the parameters of the generative network and discriminative network, respectively. G(xn) represents the image generated by the generative network, that is, the pixel-level prediction image. D(xn,yn) and D represent the two input modes of the discriminative network. The training process requires two sub-networks to be trained alternately to optimize the entire model. The steps are as follows:
1) Optimize the discriminative network. Before training the network, first fix the parameters of the generative network and optimize the discriminative network. At this point, the loss function of the discriminative network is defined as
LD=N∑n=1lcrossD(D(xn,yn),1)+lcrossD(D(xn,G(xn)),0) | (2) |
The discriminant network has two input modes, where one is D(xn,yn), which is the mosaic of the original image and the pixel-level label map. At this time, the label of the discriminative network is true, that is, it is equal to l. The other is D, that is, the mosaic map of the original image and the prediction map generated by the generative network. At this time, the label of the discriminative network is false, that is, it is equal to 0. The above two combinations are input into the discriminator respectively, and back-propagation is performed to update the parameters of the discriminator.
2) Optimize the generative network. The parameters of the discriminative network are fixed, and the generative network is optimized. At this point, the discriminative network loss function is defined as
LG=N∑n=1lcrossG(G(xn),yn)−λlcrossD(D(xn,G(xn)),0) | (3) |
a) Input the original image xn into the generative network G, obtain the generated pixel-level prediction map G(xn) and calculate the cross-entropy loss value between G(xn) and the pixel-level label map yn, that is, lcrossG.
b) G(xn) and the original image xn are spliced through the channel dimension and input into the discriminative network D. Because the purpose of generating network G is to make the generated pixel-level prediction map G(xn) as close as possible to the real label map yn, the loss function of discriminative network D is marked as true at this time. The discriminative network D trained in Step a) has the ability to judge whether the input image comes from the real label image or the generated image, that is, the cross-entropy loss value of the discriminative network D at this moment reflects the difference between the input image G(xn) and the original image xn, i.e. lcrossD.
c) Take lcrossG and lcrossD as the loss function of the back-propagation of the generative network at the same time, namely, L=lcrossG+λlcrossD, where λ represents the weight coefficient of the loss function of the discriminative network, which is used to determine the degree of supervision feedback of the discriminative network to the generative network. When λ = 0, the whole network is equivalent to the traditional semantic segmentation network. After that, the parameters of the generative network G are updated once using the back-propagation algorithm.
Equation (3) minimizes the loss of the generated prediction map and the true label map by introducing the loss brought by the discriminative network. Here, −λlcrossD is replaced with λlcrossD. The reason is that the objective function can be maximized. The discriminative network predicts the probability of G(xn) as xn so that the generated image of the generative network is closer to the real label map. When the discriminator makes accurate predictions, a stronger gradient signal can be produced, which has a great effect on reducing training time. The cross-entropy function is used for all equations.
3) Repeat the first two steps of alternating training for all training samples until the training reaches the specified number of times, and the training is completed.
In the field of remote sensing imagery, public datasets are commonly used for experimental evaluation [14]. To verify the effectiveness of our method, we conducted experiments on two public remote-sensing image object detection datasets: DIOR [1] and DOTA [2]. The DIOR [1] dataset includes 20 object categories, namely, airplanes (No. 1), airports (No. 2), baseball fields (No. 3), basketball courts (No. 4), bridges across rivers (No. 5), chimneys (No. 6), dams (No. 7), highway service areas (No. 8), highway toll booths (No. 9), golf courses (No. 10), athletic fields (No. 11), docks (No. 12), overpasses (No. 13), ships (No. 14), gymnasiums (No. 15), oil storage tanks (No. 16), tennis courts (No. 17), railway stations (No. 18), vehicles (No. 19) and windmills (No. 20). There is a total of 23463 images and 192472 object instances. Among them, 5862 images were used for training, 5863 images were used for validation and 11738 images were used for testing. The size of the image was 800 × 800, and the spatial resolution of the image was 0.5~30 m. In the experiments, we merged the training and validation images together for training. The experiments used average precision (AP) and mean average precision (mAP) as detection evaluation indicators. The calculation method of AP and mAP adopts the PASCALVOC2007 [15] standard.
The DOTA [2] dataset includes 15 categories, namely, airplanes (No. 1), baseball fields (No. 2), bridges across rivers (No. 3), track and field (No. 4), small vehicles (No. 5), large vehicles (No. 6), ships (No. 7), tennis courts (No. 8), basketball courts (No. 9), oil storage tanks (No. 10), football fields (No. 11), traffic roundabouts (No. 12), docks (No. 13), swimming pools (No. 14) and helicopters (No. 15). The DOTA [2] dataset has a total of 2806 images, and the image size varies from 800 × 800 to 4000 × 4000, and the spatial resolution of the images is 0.1~4.5 m. The training set contained 1411 images, the validation set contained 458 images and the test set contained 937 images. In the experiment, the training set and the verification set were combined for training; the test results were submitted to the DOTA [2] test server for evaluation.
In order to verify the effectiveness of this algorithm, we compared our method with other remote-sensing image target detection methods on the DIOR and DOTA datasets. The comparison results are shown in Figure 2. On the DIOR [1] dataset, we compared three methods, including the Faster R-CNN [16], CornerNet [17], YOLO v3 [18] and YOLO v4 [19]. Among them, the basic backbone network of the Faster R-CNN was ResNet101, and the detection head adopted an FPN structure. The basic backbone network of the CornerNet was Hourglass-104. The basic backbone network of YOLOv3 was Darknet53. As shown in Figure 2, although the AP values of YOLOv3 were higher than those of the proposed method on some categories, the results of our proposed method were better than those of YOLOv3 on most categories. YOLOv4 is a newer generation of the YOLO algorithm. It can be seen that the experimental results of the YOLOv4 algorithm have improved AP values in each category compared to YOLOv3. Similarly, although the accuracy rate is better than our method in some categories. But, overall, the performance of our method is still better. The mAP values of YOLOv4, YOLOv3, Faster R-CNN, CornerNet and the method proposed in this paper were 66.37%, 57.10%, 65.15%, 64.95% and 73.59%, respectively. It can be seen that the method in this paper is significantly improved compared to other methods on the DIOR dataset.
On the DOTA [2] dataset, we mainly compared three methods, namely, those based on the R-FCN [20], Faster R-CNN [16], YOLOv2 [21] and YOLOv5 [22]. Among them, the backbone network used by the R-FCN and Faster R-CNN algorithms were both ResNet101. The YOLOv2 [21] backbone network was Darknet-19. The Faster R-CNN detection head adopted an FPN structure. The experimental results are shown in Figure 3. The mAP values of YOLOv2, YOLO v5, R-FCN, Faster R-CNN and our method were 39.20%, 72.19%, 52.52%, 71.29% and 74.14%, respectively. It can be seen that, as the latest generation of the YOLO algorithm, YOLOv5 had a very obvious performance improvement compared to YOLOv2. The mAP value increased by more than 30%. However, its overall performance was still slightly weaker than that of our method. It can be seen that our method shows very good performance on all categories of the DOTA dataset. Among the three methods compared, the Faster R-CNN method had the best performance. Compared with Faster R-CNN, the method proposed in this paper still improved performance by 2.8 percentage points.
Tourism resources are the foundation of tourism. Using information technology to analyze and locate tourism resources plays an important role in promoting tourism development. The Ra-CGAN proposed in this paper is an end-to-end CNN model based on CGANs for object detection in remote sensing imaging. This enables automatic analysis and the positioning of geographic tourism resources. For the generative model, a segmentation model with a multi-level channel attention mechanism is used. While improving the multi-scale target information, it also provides a more realistic generated image for the discriminative model. Adversarial training is used to further improve the performance of the model. Taking the adversarial training loss as a loss term of the objective function of the segmentation network is equivalent to adding a regularization term to optimize the higher-order consistency, making the continuity and smoothness of the target better. Finally, the effectiveness of the dynamic feature fusion network was verified on the large-scale remote-sensing image object detection datasets DIOR and DOTA. The Ra-CGAN method proposed in this paper has achieved good results for remote-sensing image target detection. However, since there are few public datasets with pixel-level labels, deep CNN training requires huge amounts of data. Subsequent work will consider transferring the semi-supervised idea to the training of segmentation models and using massive unlabeled remote sensing imaging information to assist in the target detection of remote sensing images.
All data used to support the findings of the study are included in this paper.
This study has not received any funding support yet.
The author declares that there is no conflict of interest.
[1] |
Li K, Wan G, Cheng G, et al. (2020) Object detection in optical remote sensing images: A survey and a new benchmark. ISPRS J Photogram Remote Sens 159: 296–307. https://doi.org/10.1016/j.isprsjprs.2019.11.023 doi: 10.1016/j.isprsjprs.2019.11.023
![]() |
[2] | Xia GS, Bai X, Ding J, et al. (2018) DOTA: A large-scale dataset for object detection in aerial images. In Proceedings of the IEEE conference on computer vision and pattern recognition, 3974–3983. https://doi.org/10.1109/CVPR.2018.00418 |
[3] |
Cheng G, Zhou P, Han J (2016) Learning rotation-invariant convolutional neural networks for object detection in VHR optical remote sensing images. IEEE Trans Geosci Remote Sens 54: 7405–7415. https://doi.org/10.1109/TGRS.2016.2601622 doi: 10.1109/TGRS.2016.2601622
![]() |
[4] |
Long Y, Gong Y, Xiao Z, et al. (2017) Accurate object localization in remote sensing images based on convolutional neural networks. IEEE Trans Geosci Remote Sens 55: 2486–2498. https://doi.org/10.1109/TGRS.2016.2645610 doi: 10.1109/TGRS.2016.2645610
![]() |
[5] |
Cheng G, Han J, Zhou P, et al. (2018) Learning rotation-invariant and fisher discriminative convolutional neural networks for object detection. IEEE Trans Image Process 28: 265–278. https://doi.org/10.1109/TIP.2018.2867198 doi: 10.1109/TIP.2018.2867198
![]() |
[6] |
Deng Z, Sun H, Zhou S, et al. (2017) Toward fast and accurate vehicle detection in aerial images using coupled region-based convolutional neural networks. IEEE J Sel Top Appl Earth Obs Remote Sens 10: 3652–3664. https://doi.org/10.1109/JSTARS.2017.2694890 doi: 10.1109/JSTARS.2017.2694890
![]() |
[7] |
Li K, Cheng G, Bu S, et al. (2017) Rotation-insensitive and context-augmented object detection in remote sensing images. IEEE Trans Geosci Remote Sens 56: 2337–2348. https://doi.org/10.1109/TGRS.2017.2778300 doi: 10.1109/TGRS.2017.2778300
![]() |
[8] |
Zhang S, He G, Chen HB, et al. (2019) Scale adaptive proposal network for object detection in remote sensing images. IEEE Geosci Remote Sens Lett 16: 864–868. https://doi.org/10.1109/LGRS.2018.2888887 doi: 10.1109/LGRS.2018.2888887
![]() |
[9] |
Cheng G, Si Y, Hong H, et al. (2020) Cross-scale feature fusion for object detection in optical remote sensing images. IEEE Geosci Remote Sens Lett 18: 431–435. https://doi.org/10.1109/LGRS.2020.2975541 doi: 10.1109/LGRS.2020.2975541
![]() |
[10] | Li C, Xu C, Cui Z, et al. (2019) Feature-attentioned object detection in remote sensing imagery. In 2019 IEEE International Conference on Image Processing (ICIP), 3886–3890. https://doi.org/10.1109/ICIP.2019.8803521 |
[11] | Jin F, Wang F, Rui J, et al. (2017) Residential area extraction based on conditional generative adversarial networks. In 2017 SAR in Big Data Era: Models, Methods and Applications (BIGSARDATA), IEEE, 1–5. https://doi.org/10.1109/BIGSARDATA.2017.8124931 |
[12] |
Yu Y, Li X, Liu F (2019) Attention GANs: Unsupervised deep feature learning for aerial scene classification. IEEE Trans Geosci Remote Sens 58: 519–531. https://doi.org/10.1109/TGRS.2019.2937830 doi: 10.1109/TGRS.2019.2937830
![]() |
[13] | Hu J, Shen L, Sun G (2018) Squeeze-and-excitation networks. In Proceedings of the IEEE conference on computer vision and pattern recognition, 7132–7141. https://doi.org/10.1109/CVPR.2018.00745 |
[14] | Fang Y, Li P, Zhang J, et al. (2021) Cohesion Intensive Hash Code Book Co-construction for Efficiently Localizing Sketch Depicted Scenes. IEEE Trans Geosci Remote Sens. https: //doi.org/10.1109/TGRS.2021.3132296 |
[15] |
Everingham M, Van Gool L, Williams CKI, et al. (2010) The pascal visual object classes (voc) challenge. Int J Comput Vis 88: 303–338. https://doi.org/10.1007/s11263-009-0275-4 doi: 10.1007/s11263-009-0275-4
![]() |
[16] | Ren S, He K, Girshick R, et al. (2015) Faster r-cnn: Towards real-time object detection with region proposal networks. Adv Neural Inf Process Syst 28. |
[17] | Law H, Deng J (2018) Cornernet: Detecting objects as paired keypoints. In Proceedings of the European conference on computer vision (ECCV), 734–750. https://doi.org/10.1007/978-3-030-01264-9_45 |
[18] | Redmon J, Farhadi A (2018) Yolov3: An incremental improvement. arXiv preprint arXiv: 1804.02767. |
[19] | Bochkovskiy A, Wang CY, Liao HYM (2020) Yolov4: Optimal speed and accuracy of object detection. arXiv preprint arXiv: 2004.10934. |
[20] | Dai J, Li Y, He K, et al. (2016) R-fcn: Object detection via region-based fully convolutional networks. Adv Neural Inf Process Syst 29. |
[21] | Redmon J, Farhadi A (2017) YOLO9000: better, faster, stronger. In Proceedings of the IEEE conference on computer vision and pattern recognition, 7263–7271. https://doi.org/10.1109/CVPR.2017.690 |
[22] | Thuan D (2021) Evolution of Yolo algorithm and Yolov5: The State-of-the-Art object detention algorithm. |