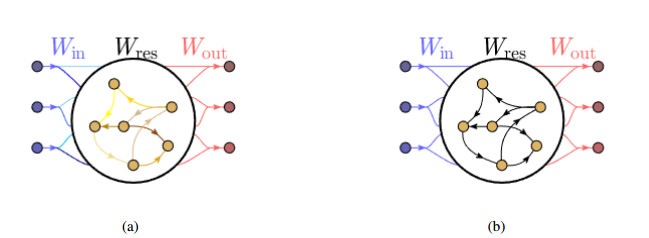
Reservoir computing (RC) is a promising approach for model-free prediction of complex nonlinear dynamical systems. Here, we reveal that the randomness in the parameter configurations of the RC has little influence on its short-term prediction accuracy of chaotic systems. This thus motivates us to articulate a new reservoir structure, called homogeneous reservoir computing (HRC). To further gain the optimal input scaling and spectral radius, we investigate the forecasting ability of the HRC with different parameters and find that there is an ellipse-like optimal region in the parameter space, which is completely beyond the area where the spectral radius is smaller than unity. Surprisingly, we find that this optimal region with better long-term forecasting ability can be accurately reflected by the contours of the l2-norm of the output matrix, which enables us to judge the quality of the parameter selection more directly and efficiently.
Citation: Bolin Zhao. Seeking optimal parameters for achieving a lightweight reservoir computing: A computational endeavor[J]. Electronic Research Archive, 2022, 30(8): 3004-3018. doi: 10.3934/era.2022152
[1] | Bin Ren, Huanfei Ma . Global optimization of hyper-parameters in reservoir computing. Electronic Research Archive, 2022, 30(7): 2719-2729. doi: 10.3934/era.2022139 |
[2] | Zhiming Cai, Liping Zhuang, Jin Chen, Jinhua Jiang . Lightweight high-performance pose recognition network: HR-LiteNet. Electronic Research Archive, 2024, 32(2): 1145-1159. doi: 10.3934/era.2024055 |
[3] | Xinzheng Xu, Yanyan Ding, Zhenhu Lv, Zhongnian Li, Renke Sun . Optimized pointwise convolution operation by Ghost blocks. Electronic Research Archive, 2023, 31(6): 3187-3199. doi: 10.3934/era.2023161 |
[4] | Jianjun Huang, Xuhong Huang, Ronghao Kang, Zhihong Chen, Junhan Peng . Improved insulator location and defect detection method based on GhostNet and YOLOv5s networks. Electronic Research Archive, 2024, 32(9): 5249-5267. doi: 10.3934/era.2024242 |
[5] | Chengtian Ouyang, Huichuang Wu, Jiaying Shen, Yangyang Zheng, Rui Li, Yilin Yao, Lin Zhang . IEDO-net: Optimized Resnet50 for the classification of COVID-19. Electronic Research Archive, 2023, 31(12): 7578-7601. doi: 10.3934/era.2023383 |
[6] | Dewang Li, Meilan Qiu, Jianming Jiang, Shuiping Yang . The application of an optimized fractional order accumulated grey model with variable parameters in the total energy consumption of Jiangsu Province and the consumption level of Chinese residents. Electronic Research Archive, 2022, 30(3): 798-812. doi: 10.3934/era.2022042 |
[7] | Yiming Zhang, Zhiwei Pan, Shuyou Zhang, Na Qiu . Probabilistic invertible neural network for inverse design space exploration and reasoning. Electronic Research Archive, 2023, 31(2): 860-881. doi: 10.3934/era.2023043 |
[8] | Xinyi Xu, Shaojuan Ma, Cheng Huang . Uncertainty prediction of wind speed based on improved multi-strategy hybrid models. Electronic Research Archive, 2025, 33(1): 294-326. doi: 10.3934/era.2025016 |
[9] | Ruyang Yin, Jiping Xing, Pengli Mo, Nan Zheng, Zhiyuan Liu . BO-B&B: A hybrid algorithm based on Bayesian optimization and branch-and-bound for discrete network design problems. Electronic Research Archive, 2022, 30(11): 3993-4014. doi: 10.3934/era.2022203 |
[10] | Hyung-Chun Lee . Efficient computations for linear feedback control problems for target velocity matching of Navier-Stokes flows via POD and LSTM-ROM. Electronic Research Archive, 2021, 29(3): 2533-2552. doi: 10.3934/era.2020128 |
Reservoir computing (RC) is a promising approach for model-free prediction of complex nonlinear dynamical systems. Here, we reveal that the randomness in the parameter configurations of the RC has little influence on its short-term prediction accuracy of chaotic systems. This thus motivates us to articulate a new reservoir structure, called homogeneous reservoir computing (HRC). To further gain the optimal input scaling and spectral radius, we investigate the forecasting ability of the HRC with different parameters and find that there is an ellipse-like optimal region in the parameter space, which is completely beyond the area where the spectral radius is smaller than unity. Surprisingly, we find that this optimal region with better long-term forecasting ability can be accurately reflected by the contours of the l2-norm of the output matrix, which enables us to judge the quality of the parameter selection more directly and efficiently.
In the early 2000s, the echo state networks (ESNs) attributed to Jaeger [1] and the liquid state machines (LSMs) attributed to Maass [2] were consecutively and independently proposed, which could be seen as the birth of the seminal reservoir computing (RC) framework. Although the RC is often regarded as a typical class of simple three-layered recurrent neural networks (RNNs), it is different from the conventional RNNs in that the input weight matrix and the intermediate reservoir weight matrix are randomly generated and fixed at the initial time and only the output layer needs to be trained using the training data. Surprisingly, this randomly generated structure exhibits excellent performance on the reconstruction and model-free prediction of chaotic dynamical systems [3,4,5,6].
Many investigations have been devoted to the design of appropriate structures to achieve a lightweight RC. Appeltant et al. proposed a framework of RC, in which the spatial reservoir structure is folded into a temporal space so that the function of RC can be realized by using only one nonlinear neuron with delayed feedback [7]. Note that this single-neuron reservoir structure is spatially expanded with only one connection weight and a very simple ring topology in the reservoir. Inspired by this framework, Rodan explored the three simplest RC structures, which showed that these structures are sufficient for predicting some typical dynamical systems accurately [8]. Griffith et al. also experimentally demonstrated that the utilization of the very simple reservoir topological structures does not negatively impact the performance of the RC [9]. However, there have been few studies considering whether the number of the parameters can be further reduced in a manner where the reservoir structure is a randomly generated network and how many parameters we need at least for achieving the prediction tasks.
Additionally, a series of studies have investigated the effect of the parameters on the RC's performance theoretically and experimentally. Particularly taken into account is the optimal spectral radius of the reservoir weight matrix for some specific prediction tasks [10,11,12,13,14,15,16]. The theoretical analysis of the spectral radius is devoted to giving a sufficient and necessary condition for the echo state property (ESP). Analyses indicate that the ESP is violated if the spectral radius of the reservoir weight matrix exceeds unity [1,10,13]. The subsequent study shows that, when we relate the network response to the temporal or statistical properties of the input-driven signal, a spectral radius above unity may not destroy the ESP [13,14]. Computationally, it was found that the performance of the RC gets better when the reservoirs are scaled toward the "edge of chaos" [17,18,19] and much effort has been devoted to finding the optimal spectral radius for specific dynamical systems [16]. However, there have been few studies taking both the input scaling and the spectral radius into consideration and depicting the optimal region in the parameter space of the RC.
Although many advances summarized above have been presented, a series of questions naturally and further arise. For instance, "Can we further reduce the number of the parameters in both the input matrix and the reservoir matrix without harming the prediction ability?", "Where is the optimal region located in the parameter space for achieving a lightweight RC?", and "How to judge the parameter selection without testing data?" To fully address these questions, the main purpose of this article is to find a more lightweight RC structure and provide a parameter selection method for it.
Specifically, we first show that the randomness of the RC parameters has little influence on the short-term prediction accuracy of chaotic systems. Thus, we present a new reservoir structure, called homogeneous reservoir computing (HRC). The HRC's input edges share the same weight and the reservoir edges share another single weight. We demonstrate that, compared with the conventional RC, the performance of the HRC attains at almost the same accuracy in the short-term prediction tasks of chaotic dynamical systems. In addition, we verify that in the case where the input weights are non-homogeneous and the edges of the reservoir are homogeneous, the HRC is comparable to the conventional RC in terms of the accurate prediction over a specific time duration. We also find that the optimal parameter region of the HRC presents a regular ellipse-like region lying entirely outside the area where the spectral radius is smaller than unity. Surprisingly, the bound of this optimal region can be accurately reflected by the contours of the l2-norm of the output matrix. As such, we propose a direct method to judge the quality of the parameter selection for the HRC.
The main contributions of this work can be summarized into four aspects. First, we provide a lightweight RC structure, named as "homogeneous reservoir computing", which only requires two adjustable parameters to be comparable to the conventional RC in the short-term prediction accuracy of the chaotic systems. The required number of the parameters is less than all the existing RC structures. Second, we find an optimal parameter region for achieving long-term forecasting tasks, which always consists of a negative correlation area and an ellipse-like area. We indeed provide an intuitive enlightenment on how the parameters of the HRC should be selected using the found optimal region. Third, we find surprisingly that the size of the l2-norm of the output matrix can accurately measure the long-term forecasting capability of the HRC. Inspired by this finding, we propose a metric to judge the quality of the parameter selection of the HRC. Last, we provide a computationally friendly algorithm for accurately selecting the optimal parameters for achieving prediction tasks without performing the prediction process in the testing data.
To begin with, we briefly introduce the basic framework of the RC. A conventional RC network structure is shown in Figure 1(a), which consists of three layers, an input layer consisting of Din input neurons, a randomly recurrently connected reservoir layer with Dres intermediate reservoir neurons, and an output layer composed of Dout output neurons. The input neurons are sparsely connected to the intermediate neurons of the reservoir layer, whose weight matrix is denoted by Win, the neurons inside the reservoir are sparsely connected with each other, whose weight matrix is denoted by Wres, the reservoir and the output layer are fully connected, whose weight matrix is denoted by Wout. The RC paradigm randomly assigns the input weights in Win and the reservoir weights in Wres, only the output matrix Wout need to be trained via a regularized linear least-squares optimization procedure [20].
During the training phase, the RC receives the input data sequence {x(t)}Tt=0 and recursively maps the sequence to the high-dimensional reservoir state space written by
r(t+1)=tanh[Wresr(t)+Winx(t)], | (2.1) |
where r(t)=[r1(t),r2(t),…,rDres(t)]⊤ is a Dres-dimensional vector with the component ri(t) representing the state of the i-th reservoir neuron at time t. After that, we apply a linear output layer and get the Dout-dimensional output y(t) as
y(t)=Woutr(t). |
We intend to match y(t) to the target output ytarget(t) by minimizing the loss function
L=T∑t=0‖y(t)−ytarget(t)‖22+γ‖Wout‖22, | (2.2) |
where γ is the regularization parameter to prevent overfitting and y(t) takes as x(t+1) in the task of predicting dynamical systems. Using Tikhonov's regularization, Wout can be given by
Wout=YtargetR⊤(RR⊤+γI)−1, |
where Ytarget=[ytarget(0) ytarget(1) … ytarget(T)] represents the target output matrix and R=[r(1) r(2) … r(T+1)] is the reservoir state matrix.
During the predicting phase, x(t) is replaced by Woutr(t) in Eq (2.1) and the reservoir state turns into a high-dimensional autonomous system as
r(t+1)=tanh[(Wres+WinWout)r(t)], |
which can produce the predicted time series continuously.
Now, we propose our framework of the HRC as sketchily depicted in Figure 1(b). Compared with the conventional RC, the HRC owns the homogeneous edges connecting the input layer with the reservoir layer, which means that the weights of these edges are all the same. Besides, the edges in the reservoir layer are homogeneous as well. In our framework, we construct the adjacency matrix of the reservoir as a sparse Erd¨os-Renyi random network, whose average node degree is denoted by d. Then, in order to get the homogeneous reservoir weight matrix, we multiply the adjacency matrix by the feedback state scaling factor α, one of the parameters of our particular interest. As for the input edges, we assign each intermediate node to be connected with only one input node and each input node to be connected with the same number of intermediate reservoir nodes. All the input edges share the same connection strength β, another parameter of our interest. Thus, the recursion of the reservoir state is written as
rH(t+1)=tanh[αWadjresrH(t)+βWadjinx(t)], |
where Wadjres and Wadjin are the 0-1 matrices representing the connection structure of the HRC. We verify that the prediction ability of the HRC is comparable to that of the conventional RC through testing different chaotic dynamical systems. Homogenization of these edges is more conducive to reducing the number of the parameters of the RC and is potentially conducive to the hardware implementation of the RC as well.
In this section, the short-term forecasting accuracy between the conventional RC and the HRC is compared computationally using different but representative chaotic dynamical systems. We choose the low-dimensional Lorenz system and large spatiotemporally chaotic solutions of the Kuramoto-Sivashinsky equation to show their performance on conducting the short-term prediction tasks.
The Lorenz system is described by
˙x=σ(y−x),˙y=x(r−z)−y,˙z=xy−bz, |
where the parameters are standardly set as σ=10, r=28, and b=83. Our goal is to train the RC and the HRC on the same segment of the Lorenz dynamics, and then to test their prediction accuracy in the following 50 time steps.
The prediction results of the RC and the HRC for the Lorenz system are shown in Figure 2. Here, we use the same connection structure and the same spectral radius ρ for the RC and the HRC, while only their connection strengths are selected, respectively, as listed in Table 1, to ensure the achievement of their best performance. For the input data, we select the sampling time step as Δt=0.005. After the training phase with different training data lengths, we use the RC and the HRC to predict the next 50 steps of the system, respectively, and display the prediction error. Obviously, in the prediction of the subsequent 50 steps, the accuracy of the HRC is comparable with that of the conventional RC. Surprisingly, when the number of training steps is 500, the prediction accuracy of the HRC is even significantly better than that of the RC. Through the observation of the state function of the intermediate nodes, we find that this phenomenon is possibly due to the randomness of the input parameter selection. Some input edges with large connection strength can cause the reservoir nodes related with them to receive large input intensities which push them to the saturated regime of the activation function. As a result, these saturated nodes, which are almost invalid, reduce the richness of the reservoir state dynamics and negatively impact the ability of the RC in capturing the nonlinear dynamics of the target chaotic dynamical system.
Dres | α | β | ρ | |
RC | 900 | [0,0.3] | [−0.1,0.1] | 0.6 |
HRC | 900 | 0.142 | 0.01 | 0.6 |
For a more complex spatiotemporally chaotic system, we test the short-term prediction of the chaotic solutions of the KSE which is a 1-dimensional partial differential equation given by
∂y∂t=−y∂y∂x−∂2y∂x2−∂4y∂x4, |
where y(x,t) is a scalar field. We set the spatial domain as x∈[0,22] and divide it evenly using 64 grid points and numerically solve the KSE with the time step Δt=0.25. These settings are the same as those used in [16]. When the other parameters are selected as those displayed in Table 2, both the HRC and the RC realize accurate prediction in the first 5 Lyapunov time, as shown in Figure 3.
Dres | α | β | ρ | |
RC | 2944 | [0,1.0] | [−0.5,0.5] | 0.1 |
HRC | 2944 | 0.014 | 0.2 | 0.1 |
In this section, we select a tolerance bound ϵ of the prediction error according to the statistical properties of each chaotic system, and measure the long-term forecasting ability of the RC and the HRC according to the time steps Nϵ when the norm of error between the predicted output and the target output exceeds ϵ for the first time
Nϵ=inf{N:‖y(T+N)−ytarget(T+N)‖2≥ϵ}. |
We find that the long-term forecasting ability of the HRC is not as good as that of the conventional RC. However, if we recover the randomness of the input weight matrix and keep the reservoir matrix homogeneous as before, the HRC can forecast as many time steps as the conventional RC. For the reason given above, we just consider the case that only the edges in the reservoir layer of the HRC are homogeneous.
After displaying the accurate prediction steps with different input scaling and spectral radius, we find that for a variety of chaotic systems, there always exists an ellipse-like optimal region in the parameter space. The shape and the location of this ellipse are associated with the average degree d of the adjacency matrix of the reservoir as Figure 4(a). Most of the studies associated with the RC scale the reservoir weight matrix to a spectral radius below or around unity to obtain the ESP. However, we note that our ellipse-like optimal region lies almost entirely outside this widely accepted optimal region within which only a small area can achieve a high number of accurate prediction steps. By calculating the maximum Lyapunov exponent of the dynamical system reconstructed by the HRC, we verify experimentally that in this ellipse-like optimal region where the spectral radius can be far beyond unity, the HRC can not only accurately predict short-term "weather" of the target system, but capture its long-term "climate" as well. This shows that the parameters chosen from the ellipse-like optimal region do not destroy the ESP of the HRC. We further find that a larger spectral radius will make the convergence of the intermediate states of the HRC greatly slow down, thus requiring longer driven data to push the reservoir state to the correct dynamics.
Taking the Lorenz system as an example, we set the tolerance bound ϵ=0.5 and randomly generate 100 Erd¨os-Renyi network structures as adjacency matrices of the HRC. We train all 100 HRC and get their average accurate prediction steps ¯Nϵ which is treated as the long-term forecasting ability corresponding to different parameters. It can be seen that the parameter area with the largest number of accurate prediction steps is mostly concentrated in an oblique "ellipse-like" area, which is completely outside the bound of the spectral radius equal to 1 as shown in Figure 4(a) (The solid black line represents the spectral radius equal to 1). The existence of the elliptical region is independent of other structural parameters of HRC, but its shape and location are associated with the average node degree in the reservoir layer. Within the area where the spectral radius is smaller than unity, there is a small optimal region where the input scale in this region has a negative correlation with the spectral radius.
Additionally, we test the broad applicability of our result on the weather data collected in the city of Delhi during the period of 4 years (from 2013 to 2017) as the training data and predict the daily average temperature for the ensuing period. We set the tolerance bound ϵ=4 and randomly generate 50 Erd¨os-Renyi network structures to get their average accurate prediction steps ¯Nϵ. We find that HRC can accurately predict the daily temperature for the next 30 days when the parameters are appropriately selected. There is also an obvious optimal parameter region where the input scaling is negatively correlated with the spectral radius within the area where the spectral radius is smaller than unity, as shown in the right panel of Figure 5(a). Likewise, there is also an ellipse-like region in the area where the spectral radius is greater than unity. The optimal input scaling interval increases monotonically with the spectral radius in this ellipse-like region, as shown in the left panel of Figure 5(a).
Finally, it is noted that the spectral radius of unity can be regarded as an exact "inflection point". The optimal parameter region exhibits completely different behaviors when the spectral radius exceeds one in the prediction tasks of various chaotic dynamical systems and actual scenarios. The cause of this phenomenon awaits further and systematic exploration.
In this section, we show that the bound of the optimal region obtained above corresponds accurately to the l2-norm of the output matrix. We still consider the prediction task of the Lorenz system and calculate the l2-norm of the output matrices of the HRC associated with different input scaling and spectral radius as Figure 4(b). Surprisingly, the contours of the l2-norm of the output matrix in the parameter space are consistent with the trend of the lower bound of the optimal region in Figure 4(a), including the ellipse-like region and the negative correlation region as well. Furthermore, if we choose appropriate accurate prediction steps and l2-norm size, the contours of the long-term prediction ability with certain accurate prediction steps almost exactly coincide with the contours of l2-norm of the output matrix, as shown in Figure 6. For the Delhi Weather Data, the contours of the l2-norm of the output matrix also accurately coincide with the contours of the optimal region despite the decrease of the boundary smoothness, as shown in Figure 5(b), (c).
Thus, without testing data, we provide a method to judge the long-term forecasting ability of the HRC by calculating the l2-norm of its output matrix. Empirically, it can be treated as an accurate metric of the quality of the parameter selection. In numerical experiments, we can calculate the l2-norm of Wout under different parameters selected from a coarse grid and seek better parameters corresponding to a smaller the l2-norm of Wout. Specifically, we provide a method to select the optimal parameters for an actual scenario using this metric without performing the prediction process in the testing data. We find that outside the lower bound of the optimal parameter region, the l2-norm of the output matrix with the same spectral radius rapidly declines with the increase of the input scaling, while once it gets into the optimal region, the change slows down considerably, as shown in Figure 7. As the input scaling crosses the upper bound of the optimal region, the norm of the output matrix increases slightly. Inspired by this phenomenon, we first choose an optional input scaling interval based on the scale of the training data and divide the interval into incremental grid points. In general, the interval we choose should be such that the product of its upper bound and the training data lies in the unsaturated region of the activation function. After that, for a determined spectral radius, we calculate the corresponding l2-norm of Wout sequentially. Once the trend of the l2-norm of Wout obtained from two adjacent calculations changes from decreasing to increasing, we can determine that the current parameters are already inside the optimal region.
In addition, this phenomenon also inspires us to optimize the performance of the RC by reducing the norm of the output matrix. It can be realized by appropriately increasing the coefficient of the regularization term in Eq (2.2). Besides, existing studies have shown that removing reservoir neurons and their respective edges corresponding to the largest weights in the output matrix can effectively improve the performance [21], which is essentially a way to reduce the norm of the output matrix as well.
In this article, we provide a lightweight framework of the RC called homogeneous reservoir computing. Compared with the conventional RC, there are only two parameters in the HRC, the input scaling and the feedback state scaling. We experimentally verify that the homogenization of the input edges and the reservoir edges in the HRC has no negative influence on the short-term prediction accuracy of chaotic dynamical systems. Additionally, the HRC with homogeneous reservoir edges only is comparable to the conventional RC in the long-term forecasting ability.
For the long-term forecasting ability, an optimal region of the parameter space is given. We provide an intuitive enlightenment on how the parameters of the HRC should be selected according to the properties of this optimal region. The optimal region with the spectral radius below unity shows a negative correlation between the input scaling and the feedback state scaling. This phenomenon can be interpreted intuitively in a sense that the signals received by the reservoir neurons, which are in the form of weighted summation of the input scaling and the feedback state scaling, need to be in the nonlinear region of the activation function. However, most of the optimal parameters are found to be in an ellipse-like region beyond the area where the spectral radius is smaller than unity. It is counterintuitive as reservoir computing with a spectral radius much larger than unity is easier to destroy the ESP. The ellipse-like region reflects a proportional relationship between the input scaling and the feedback state scaling. When we choose a larger spectral radius, the feedback state signal received by a reservoir neuron becomes larger as well. As a result, we need to choose a larger input scaling to provide a sufficiently strong driven signal. Further investigation is anticipated to unveil the optimal proportion between the input-driven signal and the feedback state signal for the reservoir neurons.
We also find surprisingly that the optimal parameter region is consistent with the region where the l2-norm of the trained output matrix is small. The lower boundary of the optimal region can be accurately fitted by the contour line of Wout's norm. Therefore, we give a direct method to judge the quality of the parameter selection by calculating the norm of the output matrix. In addition, we also capture the abrupt changes in the rate of the l2-norm change of the output matrix within and outside the optimal region. Inspired by this finding, we articulate a computationally friendly algorithm to select a set of optimal parameters for a real-world scenario.
We acknowledge that the HRC still has some limitations because of the homogenization of its connecting edges. We can only use a sparse adjacency matrix as the network structure of the homogeneous reservoir layer because the fully-connected structures can cause the rank of the adjacency matrix to degenerate to one, which thus likely reduces the representation capability of the HRC. We find that sparse Erd¨os-Renyi random networks all perform well in the prediction tasks, but the optimal network structure of the HRC for any specific chaotic system remains unclear. Additionally, we need to provide much better and more precise parameter settings to achieve the same short-term or long-term forecasting ability as the conventional RC. In this article, we provide a metric for judging the parameters which can help us get such a precise parameter setting, but the fundamental mechanism rendering this method effective still needs further exploration.
There are still several directions awaiting in-depth study in the near future: (1) Elucidating the reason why the parameters with a better forecasting ability mostly are located in the region corresponding to the spectral radius larger than unity, and why the parameters region presents in the elliptical form, (2) deciphering the mechanism that induces the l2-norm of the output matrix to have the capability in accurate reflection of the long-term forecasting ability for dynamical systems, (3) designing the method to select the optimal parameters according to the statistical information of the data, (4) investigating the relation between the optimal region and the connection structure, such as the average degree of reservoir neurons, and (5) applying the developed and developing optimal frameworks to the paramount problems, such as the hidden structures detection, tipping-point detection, and the causality detection [22,23,24], in time series analytics fusing the representative methods of dynamical systems and machine learning.
The author, as the member of the Zhuobo Program of Fudan University, gratefully acknowledges the useful discussions and comments from Professor Wei Lin. This work is supported by the National Natural Science Foundation of China (Grant Nos. 11925103 & 61773125) and by the Shanghai Municipal Science and Technology Major Project (Grant No. 2021SHZDZX0103).
The authors declare there is no conflicts of interest.
[1] | H. Jaeger, The "echo state" approach to analysing and training recurrent neural networks-with an erratum note, German National Research Center for Information Technology, Bonn, Germany, 148 (2001), 13. |
[2] |
W. Maass, T. Natschläger, H. Markram, Real-time computing without stable states: A new framework for neural computation based on perturbations, Neural Comput., 14 (2002), 2531–2560. https://doi.org/10.1162/089976602760407955 doi: 10.1162/089976602760407955
![]() |
[3] |
H. Jaeger, H. Haas, Harnessing nonlinearity: Predicting chaotic systems and saving energy in wireless communication, Science, 304 (2004), 78–80. https://doi.org/10.1126/science.1091277 doi: 10.1126/science.1091277
![]() |
[4] |
Z. Lu, J. Pathak, B. Hunt, M. Girvan, R. Brockett, E. Ott, Reservoir observers: Model-free inference of unmeasured variables in chaotic systems, Chaos, 27 (2017), 041102. https://doi.org/10.1063/1.4979665 doi: 10.1063/1.4979665
![]() |
[5] |
J. Pathak, Z. Lu, B. R. Hunt, M. Girvan, E. Ott, Using machine learning to replicate chaotic attractors and calculate lyapunov exponents from data, Chaos, 27 (2017), 121102. https://doi.org/10.1063/1.5010300 doi: 10.1063/1.5010300
![]() |
[6] |
J. Pathak, B. Hunt, M. Girvan, Z. Lu, E. Ott, Model-free prediction of large spatiotemporally chaotic systems from data: A reservoir computing approach, Phys. Rev. Lett., 120 (2018), 024102. https://doi.org/10.1103/PhysRevLett.120.024102 doi: 10.1103/PhysRevLett.120.024102
![]() |
[7] |
L. Appeltant, M. C. Soriano, G. Van der Sande, J. Danckaert, S. Massar, J. Dambre, et al., Information processing using a single dynamical node as complex system, Nat. Commun., 2 (2011), 1–6. https://doi.org/10.1038/ncomms1476 doi: 10.1038/ncomms1476
![]() |
[8] |
A. Rodan, P. Tino, Minimum complexity echo state network, IEEE Trans. Neural Networks, 22 (2010), 131–144. https://doi.org/10.1109/TNN.2010.2089641 doi: 10.1109/TNN.2010.2089641
![]() |
[9] |
A. Griffith, A. Pomerance, D. J. Gauthier, Forecasting chaotic systems with very low connectivity reservoir computers, Chaos, 29 (2019), 123108. https://doi.org/10.1063/1.5120710 doi: 10.1063/1.5120710
![]() |
[10] |
M. Buehner, P. Young, A tighter bound for the echo state property, IEEE Trans. Neural Networks, 17 (2006), 820–824. https://doi.org/10.1109/TNN.2006.872357 doi: 10.1109/TNN.2006.872357
![]() |
[11] | M. Lukosevicius, H. Jaeger, Overview of reservoir recipes, Technical Report, Jacobs University Bremen, 2007. |
[12] | D. Verstraeten, Reservoir Computing: Computation with Dynamical Systems, Ph.D thesis, Ghent University, 2009. |
[13] |
I. B. Yildiz, H. Jaeger, S. Kiebel, Re-visiting the echo state property, Neural Networks, 35 (2012), 1–9. https://doi.org/10.1016/j.neunet.2012.07.005 doi: 10.1016/j.neunet.2012.07.005
![]() |
[14] |
G. Manjunath, H. Jaeger, Echo state property linked to an input: Exploring a fundamental characteristic of recurrent neural networks, Neural Comput., 25 (2013), 671–696. https://doi.org/10.1162/neco_a_00411 doi: 10.1162/neco_a_00411
![]() |
[15] | S. Basterrech, Empirical analysis of the necessary and sufficient conditions of the echo state property, in 2017 International Joint Conference on Neural Networks, IEEE, (2017), 888–896. https://doi.org/10.1109/IJCNN.2017.7965946 |
[16] |
J. Jiang, Y. C. Lai, Model-free prediction of spatiotemporal dynamical systems with recurrent neural networks: Role of network spectral radius, Phys. Rev. Res., 1 (2019), 033056. https://doi.org/10.1103/PhysRevResearch.1.033056 doi: 10.1103/PhysRevResearch.1.033056
![]() |
[17] |
C. G. Langton, Computation at the edge of chaos: Phase transitions and emergent computation, Phys. D, 42 (1990), 12–37. https://doi.org/10.1016/0167-2789(90)90064-V doi: 10.1016/0167-2789(90)90064-V
![]() |
[18] |
N. Bertschinger, T. Natschläger, Real-time computation at the edge of chaos in recurrent neural networks, Neural Comput., 16 (2004), 1413–1436. https://doi.org/10.1162/089976604323057443 doi: 10.1162/089976604323057443
![]() |
[19] | N. Bertschinger, T. Natschläger, R. Legenstein, At the edge of chaos: Real-time computations and self-organized criticality in recurrent neural networks, Adv. Neural Inf. Process. Syst., 17 (2004). |
[20] | B. Schrauwen, D. Verstraeten, J. Van Campenhout, An overview of reservoir computing: theory, applications and implementations, in Proceedings of the 15th European Symposium on Artificial Neural Networks, (2007), 471–482. |
[21] |
A. Haluszczynski, J. Aumeier, J. Herteux, C. Räth, Reducing network size and improving prediction stability of reservoir computing, Chaos, 30 (2020), 063136. https://doi.org/10.1063/5.0006869 doi: 10.1063/5.0006869
![]() |
[22] |
Q. Zhu, H. F. Ma, W. Lin, Detecting unstable periodic orbits based only on time series: When adaptive delayed feedback control meets reservoir computing, Chaos, 29 (2019), 093125. https://doi.org/10.1063/1.5120867 doi: 10.1063/1.5120867
![]() |
[23] |
J. W. Hou, H. F. Ma, D. He, J. Sun, Q. Nie, W. Lin, Harvesting random embedding for high-frequency change-point detection in temporal complex, Natl. Sci. Rev., 2022. https://doi.org/10.1093/nsr/nwab228 doi: 10.1093/nsr/nwab228
![]() |
[24] |
X. Ying, S. Y. Leng, H. F. Ma, Q. Nie, Y. C. Lai, W. Lin, Continuity scaling: A rigorous framework for detecting and quantifying causality accurately, Research, 2022 (2022), 9870149. https://doi.org/10.34133/2022/9870149 doi: 10.34133/2022/9870149
![]() |
1. | Zuo Wei Ye, Du Qu Wei, Predicting chaos in permanent magnet synchronous motor using dual reservoir interaction with high-order state reservoir computing, 2025, 100, 0031-8949, 036010, 10.1088/1402-4896/adb4af |
Dres | α | β | ρ | |
RC | 900 | [0,0.3] | [−0.1,0.1] | 0.6 |
HRC | 900 | 0.142 | 0.01 | 0.6 |
Dres | α | β | ρ | |
RC | 2944 | [0,1.0] | [−0.5,0.5] | 0.1 |
HRC | 2944 | 0.014 | 0.2 | 0.1 |