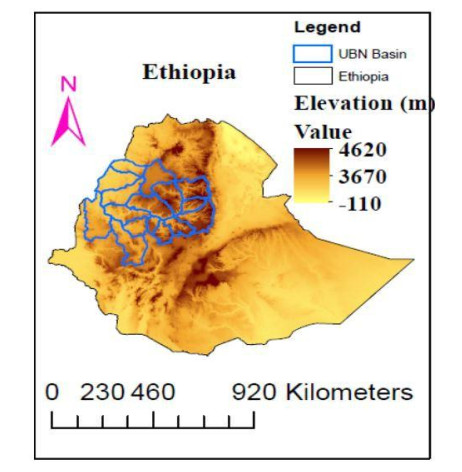
A composite analysis is used to evaluate the teleconnections between the long term (June- August) rainfall anomalies with east and west phases of quasi-biennial oscillation (QBO) in the stratospheric zonal winds from 1979–2017. Applying the lower equatorial stratospheric zonal wind index in JJA rainfall prediction is based on its tendency to persist for several months after the phase change from easterly to westerly and vice versa. Below normal condition rainfall used to coin drought. This study is important because, the Upper Blue Nile region is one of the most inviting areas for different activities like agriculture and hydroelectric power; therefore, timely prediction of June-August rainfall serves farmers and other concerned sectors. The aim of this analysis is to establish the global signal quasi-biennial oscillation contribution alone at different time lags and its association among Southern Oscillation Index (SOI) for estimation June-August rainfall of the Upper Blue Nile. The rainfall used as predictand while quasi-biennial oscillation & SOI datasets are used as predictors in regression model after testing collinearity of these two independent variables. Performance of regression model and actual value is tested by using statistical techniques: Root Mean Square Error, Mean Absolute Error and bias. The performance is seen reasonably high between actual and estimated values show strong agreement.
Citation: Abebe Kebede Habtegebreal, Abebaw Bizuneh Alemu, U. Jaya Prakash Raju. Examining the Role of Quasi-biennial Oscillation on Rainfall patterns over Upper Blue Nile Basin of Ethiopia[J]. AIMS Environmental Science, 2021, 8(3): 190-203. doi: 10.3934/environsci.2021013
[1] | Tewodros Woldemariam Tesfaye, C.T. Dhanya, A.K. Gosain . Evaluation of ERA-Interim, MERRA, NCEP-DOE R2 and CFSR Reanalysis precipitation Data using Gauge Observation over Ethiopia for a period of 33 years. AIMS Environmental Science, 2017, 4(4): 596-620. doi: 10.3934/environsci.2017.4.596 |
[2] | Oltion Marko, Joana Gjipalaj, Dritan Profka, Neritan Shkodrani . Soil erosion estimation using Erosion Potential Method in the Vjosa River Basin, Albania. AIMS Environmental Science, 2023, 10(1): 191-205. doi: 10.3934/environsci.2023011 |
[3] | Moven Manjowe, Terence Darlington Mushore, Juliet Gwenzi, Collen Mutasa, Electdom Matandirotya, Emmanuel Mashonjowa . Circulation mechanisms responsible for wet or dry summers over Zimbabwe. AIMS Environmental Science, 2018, 5(3): 154-172. doi: 10.3934/environsci.2018.3.154 |
[4] | Nurtiti Sunusi, Giarno . Bias of automatic weather parameter measurement in monsoon area, a case study in Makassar Coast. AIMS Environmental Science, 2023, 10(1): 1-15. doi: 10.3934/environsci.2023001 |
[5] | John R. Christy . Examination of Extreme Rainfall Events in Two Regions of the United States since the 19th Century. AIMS Environmental Science, 2019, 6(2): 109-126. doi: 10.3934/environsci.2019.2.109 |
[6] | Zinabu A. Alemu, Emmanuel C. Dioha, Michael O. Dioha . Hydro-meteorological drought in Addis Ababa: A characterization study. AIMS Environmental Science, 2021, 8(2): 148-168. doi: 10.3934/environsci.2021011 |
[7] | Kitipol Nualtong, Ronnason Chinram, Piyawan Khwanmuang, Sukrit Kirtsaeng, Thammarat Panityakul . An efficiency dynamic seasonal regression forecasting technique for high variation of water level in Yom River Basin of Thailand. AIMS Environmental Science, 2021, 8(4): 283-303. doi: 10.3934/environsci.2021019 |
[8] | Eric Ariel L. Salas, Sakthi Kumaran Subburayalu . Implications of climate change on nutrient pollution: a look into the nitrogen and phosphorus loadings in the Great Miami and Little Miami watersheds in Ohio. AIMS Environmental Science, 2019, 6(3): 186-221. doi: 10.3934/environsci.2019.3.186 |
[9] | Finu Shrestha, Kabir Uddin, Sudan Bikash Maharjan, Samjwal Ratna Bajracharya . Application of remote sensing and GIS in environmental monitoring in the Hindu Kush Himalayan region. AIMS Environmental Science, 2016, 3(4): 646-662. doi: 10.3934/environsci.2016.4.646 |
[10] | Goshu Desalegn, Anita Tangl, Maria Fekete-Farkas . Greening through taxation: assessing the potential opportunities and challenges of plastic products in Ethiopia. AIMS Environmental Science, 2022, 9(4): 432-443. doi: 10.3934/environsci.2022026 |
A composite analysis is used to evaluate the teleconnections between the long term (June- August) rainfall anomalies with east and west phases of quasi-biennial oscillation (QBO) in the stratospheric zonal winds from 1979–2017. Applying the lower equatorial stratospheric zonal wind index in JJA rainfall prediction is based on its tendency to persist for several months after the phase change from easterly to westerly and vice versa. Below normal condition rainfall used to coin drought. This study is important because, the Upper Blue Nile region is one of the most inviting areas for different activities like agriculture and hydroelectric power; therefore, timely prediction of June-August rainfall serves farmers and other concerned sectors. The aim of this analysis is to establish the global signal quasi-biennial oscillation contribution alone at different time lags and its association among Southern Oscillation Index (SOI) for estimation June-August rainfall of the Upper Blue Nile. The rainfall used as predictand while quasi-biennial oscillation & SOI datasets are used as predictors in regression model after testing collinearity of these two independent variables. Performance of regression model and actual value is tested by using statistical techniques: Root Mean Square Error, Mean Absolute Error and bias. The performance is seen reasonably high between actual and estimated values show strong agreement.
The wave means flow interactions in the upper troposphere lower stratosphere region so that a quasi-biennial oscillation (QBO) with proper downward extension can be generated [1]. The downward propagation of the QBO phases occurs evidently, but it does not penetrate to sufficiently low altitudes. This is due to the strength of easterly Jets seldom extend downward. This quasi-biennial oscillation driven by equatorially trapped gravity waves propagate from the troposphere to the stratosphere [2]. It propagates downward alternatively westerly and easterly zonal wind shears in the tropical stratosphere with a period of ~ 28 months [2]. Due to the QBO there is a modulation water vapor mixing ratios in the air entering the stratosphere through the cold, tropical tropopause region [3,4], and tropical deep convection [5,6,7]. By using five decades of radiosonde data near equatorial stations indicated that the ENSO influence on the QBO is zonally symmetric [8].
The warmer temperature during QBO westerly shear conditions and colder temperatures during QBO easterly shear conditions are larger during La-Ninã than during El-Ninõ for all stations for the entire period [9]. This strengthens earlier findings that the greatest dehydration of air entering the stratosphere from the troposphere occurs during the winter under La Niña and easterly QBO conditions [8]. The seasonal rainfall anomalies: short and long rain over East Africa was estimated using QBO by considering a number of stations [10,11]. According to the study of Seleshi Y and Zanke U [12] determine June-September rainfall in the Ethiopian highlands is positively correlated to the Southern Oscillation Index (SOI) and negatively correlated equatorial Eastern Pacific sea surface temperature (SST).
The central and northern parts of Ethiopia experiences two main rainy seasons: short rain (February-May) and long rains (June-September) [13]. Giving an emphasis for the long season rainfall is potentially important for agriculture, hydropower and other socio-economic activities in countries like Ethiopia [14] the mass of peoples' economy relay up on agricultural activities. Recent studies over the Upper Blue Nile have largely focused on the ENSO [15], so that other important long-term source of climate variability overlook is an essential issue for climate prediction.
The shortage of rainfall over the Upper Blue Nile affects the socio-economic life of Ethiopia and the territory countries [16]. Such natural extreme is associated with significant anomalies in the Pacific (SST) [17]. Both El-Niño and La-Niña are the phenomenon in the equatorial Pacific Ocean characterized by anomaly of SST [18]. Negative values indicate El Niño conditions as lower pressure in the eastern Pacific and associated with warmer water and weakened easterly trade winds. On the other condition the positive Southern Oscillation index (SOI) corresponds to negative SST index leads to La-Niña [16].
The natural oscillations in regions of the Pacific Ocean have a significant impact on the patterns of weather and climate around the globe including the Upper Blue Nile basin of Ethiopia [19]; however, among these oscillations the dominant one is El Niño Southern Oscillation (ENSO) with return period of about four years, varying from two to seven years [20]. Abtew W et al. [21] used monthly rainfall a number of rain gauge monitoring network in the Upper Blue Nile Basin found that high/low rainfall is likely to occur during La Niña/ El Niño years and extremely dry wet are highly likely to occur during El Niño/ La Niña year's respectively.
Rainfall is a crucial variable to climate and issues related to weather. Lack or heavy rainfall leads to climate extremes like drought and flood which have negative impacts on socio-economic and environment. These climatic extremes are due to global signals which deviate from the average value. Based on this commonly known climate signal, SOI plays a key role in detecting climate change and forecasting the rainfall variable in different regions of the world. The QBO for detecting climate change variables is important for seeing how the middle atmosphere interacts with the lower atmosphere mechanically and chemically [22]. Therefore, using like Oceans oscillation studying further about QBO and its influence on climate change currently an emerging climate science.
This study relies upon temporally correlation patterns statistically to evaluate the climate condition relationships among the equatorial stratospheric zonal wind at 30hpa and rainfall over the Upper Blue Nile. The main objective of this study is to get a physical sound as a predictor variable in the long-range forecast rainfall from the QBO of Upper Blue Nile of Ethiopia.
The Upper Blue Nile is found in the northwestern region of the Ethiopia (Figure 1). The elevation varies from 480 m near the Sudanese/Ethiopian border to over 4200 m near the central part of the basin [23].
The spatial and temporal variation of climate in the basin is affected by the movement of air masses associated with Inter Tropical Convergence Zone (ITCZ) [24]. During the winter dry season (known in Ethiopia as Bega) the ITCZ lies south of Ethiopia and the Blue Nile region is affected by a dry northeast continental air-mass [24]. From March, the ITCZ returns bringing small rains (Belg) particularly to the southern and south western parts of the Basin [24]. In May, the northward movement of the ITCZ produces a short intermission before the main wet season (known in Ethiopia as the Kremt). Around June, the ITCZ moves further north and the southwest air-stream extends over the entire Ethiopian highlands to produce the main rainy season [24].
The average annual rainfall varies between 1400 and 1800 mm/year, ranging from an average of about 1000 mm/year near the Ethiopia Sudan border to 1400 mm/year in the upper part of the basin, and in excess of 2000 mm/year in the parts Didessa and Beles sub basins [14]. The rainfall trend was studied in the Upper Blue Nile basin, most of the results showed that there was no significant trend in the seasonal and annual basin-wide average rainfall [25,26,27]. The annual mean potential evapotranspiration decreases with increasing elevation from 1845 mm to 924 mm [23]. In the Sudan, potential evaporation increases, this produces a significant loss of Blue Nile water. For instance, the Sennar region has a potential evaporation rate of 2,500 mm/year, but receives only 500 mm/year of rain [15]. The temperature in the basin varies with elevation. The climate is generally temperate at higher elevations and tropical at lower elevations. According to the study of Conway D [23] depending on elevation and mean annual temperature he classified the climate of the Upper Blue Nile basin.
The QBO index is a type of oscillation in the atmosphere high above the equator, in the stratosphere, strong zonal winds blow in a continuous circuit around the Earth. Over an altitude, the winds begin westerlies, however, after sometime the westerlies propagation weaken and eventually reverse direction and become strong easterlies. The whole cycle progresses at a fairly uniform rate, taking on average 26 months to return to the initial state. The downward propagation easterlies amplitude is approximately twice as strong as westelies phase and strong at the top of the vertical QBO region, but westerlies domination is found at the bottom region of QBO.
Rainfall measurement through observation, model and satellite-based techniques are important to assess and rainfall spatially and temporally. The conventional rain gauge observations are found sparsely and unevenly distributed in Ethiopia and across Upper Blue Nile basin. Therefore, applying high resolution satellite data is important to capture rainfall in any region of the world after it validated. The Climate Hazards Group Infrared Precipitation with Stations (CHIRPS) rain fall product was evaluated in comparison to rain gauge data, Tropical Applications of Meteorology using Satellite and ground-based observations (TAMSAT 3) and the African Rainfall Climatology(ARC 2) products [28].
The Southern Oscillation Index (SOI) data from https://www.crudata.uea.ac.uk/cru/data/soi/ and globally averaged equatorial stratospheric at 30-hpa zonal wind index (QBO) from http://www.geo.fu-berlin.de/en/met/ag/strat/produkte/qbo/index.htmlare selected as potentially predictors rainfall of Upper Blue Nile basin. The data used as the predictant variable summer (JJA) rainfall which is supplied by CHIRPS: http://www.chg.geog.ucsb.edu/data. Before implementing this research, the CHIRPS data were validated by different locations over the study area. After validation of CHIRPS data then we implemented from the period 1981–2017. The correlation between QBO with monthly and seasonal rainfall are evaluated.
The composite analysis was used to examine the teleconnections between the seasonal rainfall anomalies of June-August (summer season) over Upper Blue Nile and the west and east phases of QBO in the stratospheric zonal winds, and also explore the predictive potential of the summer season using information about the phases of the QBO for the period 1981–2017. We used CHIRPS grid data with a resolution of 0.05 degrees instead of using a large amount of station data that is used by PCA to reduce predictive dimension.
The Pearson correlation analysis is made between summer season (JJA) with different seasons of QBO which has westerly and easterly phases. In this study CHIRPS grid data is used with high resolution in order to reduce dimensionality. The zero and non-zero lag is also implemented with different time steps to determine the relationships of rainfall and QBO signals. We also visualized monthly and seasonal linear correlation among SOI and QBO with rainfall over Upper Blue Nile as previously studied by [29]. In Eq 1 below, the value of R is between -1 and 1. These positive and negative values are indicative of the positive or negative relationships, respectively.
(1) |
Where N is the total number of records, X and Y are a long term mean of observed and predicted, respectively.
Regression method Multiple regression models implemented to determine how dependent variable Y (rainfall) responses among linearly on a number of predictor variables [30]. This situation leads to the multiple regressions for models with two or more predictors with one response. There are also regression models with two or more response variables. The independent value Xi is associated with dependent variable Y. Considering a multiple linear regression model with predictor variables X1, X2, ..., Xk and relationship with Y, can be defined as follows:
(2) |
Where, β0, β1, β2, …, βK regression coefficients Y is the dependent (response) variable, Xi is independent (predictors) variable and ε is the error term.
X1 = S OIS ON, X2 = QBOJJA are the two predictors of SOI and QBO indices with time lags of data which are categorized into four seasons. The coefficients are a linear trend β0, SOISON season with amplitude β1 and QBOJJA with amplitudes β2 respectively. By selecting predictors (QBOSON and SOISON) for the Upper Blue Nile region of Ethiopia there is detection of the colliarity issue among these two independent variables. In the multicollinear analysis, the variance inflation factor (VIF) is used. Multicollinearity diagnosis is the VIF [31] defined as: (3)
(3) |
Where, R2 is the coefficient of determination when variable j is regressed on j-1 remaining independent variables. A variable is considered to be problematic if its VIF is larger than 10.0 [31].
The independent variable QBO is evaluated by using different methods to visualize its accuracy with the observational JJA rain fall for different year intervals. The root mean squared error (RMSE), bias and the mean absolute error (MAE) are implemented statistics for evaluating the overall quality of the rainfall estimation by using QBO phases and its contribution with SOI during regression model. The MAE is the average of the absolute value of the residuals (error) which is very similar to RMSE but it is less sensitive to large errors. The RMSE is the square root of the average squared distance of a data point from the fitted line.
(4) |
(5) |
(6) |
Where, P refers predicted value, O is observed rain fall and N is the number of observed values
The dryness and wetness are analyzed coinciding with the time lag phases of QBO from 1979–2017. Using composite analysis, we used the year intervals with above normal (AN) rainfall and coinciding with west phases of the QBO and with below normal (BN) rainfall coinciding with the east phase of the QBO. During composite analysis we categorize wetness and dryness from the normal condition which are visualized positive and negative values respectively.
Applying the lower equatorial stratospheric zonal wind index in seasonal prediction based on its tendency to persist for several months after the phase change from easterly to westerly and vice versa. Figure 2 shows westerly and easterly QBO phases (color shades) for the period 1979–2017 or 468 months. The disruption of the western phase of the QBO started at 15 hPa in 2015 and was fully observed at 30 hPa pressure levels. This disruption continued at 40 hpa throughout 2015, there was also a drought on the Upper Blue Nile during this year [32]. Under normal conditions, rainfall is used to determine the state of drought, but the duration, severity and intensity vary depending on the time series. The drought of 1983, 2002 and 2009 is associated with the eastern phase, but the 2015 drought associated with the western drought may be due to disruption of the QBO is the main cause.
The table below (two-year lags) indicates that the total study period is divided into equal intervals. We evaluate months of QBO phases which indicate relationships between JJA rainfall. In our data set, there are no outliers and every variable is continuous, so we used the Pearson correlation factor. The months of January and February are highly correlated with JJA precipitation for different time intervals. The maximum association was observed in the years 1989–1993, 1994–1988, 1999–2003 and 2004–2008 across each month of the QBO. The maximum correlation between QBO phases and JJA rainfall was investigated before five to six months as shown in Table 1. However, in the years 1984–1988 and 2009–2015 we observed a low association of QBO phases and JJA rainfall. This might be others oscillation like SOI more influential for JJA rainfall than QBO during these years
Years | Jan | Feb | Mar | Apr | May |
1979–1983 | 0.53 | 0.56 | 0.21 | 0.03 | −0.19 |
1984–1988 | 0.05 | 0.09 | −0.04 | −0.11 | −0.45 |
1989–1993 | −0.81 | −0.80 | −0.90 | −0.85 | −0.94 |
1994–1998 | −0.99 | −0.91 | −0.85 | −0.76 | −0.80 |
1999–2003 | −0.87 | −0.87 | −0.90 | −0.87 | −0.68 |
2004–2008 | −0.78 | −0.78 | 0.28 | 0.40 | 0.48 |
2009–2015 | −0.02 | −0.15 | −0.23 | −0.20 | −0.06 |
We do the evaluation by using the time lag QBO index at 30hPa to predict JJA rainfall over Upper Blue Nile. The correlation is done between SOI and QBO at different time lags with JJA rainfall of Upper Blue Nile. The evaluation of the QBO-index phases and SOI are done for different period over the study area. As shown in Figure 3, we have standardized the data by subtracting the mean and dividing by the standard deviation for each value the data. we have observed that annual mean time series for rainfall versus QBO-index with westerly and easterly phases of the QBO index and standardized SOI. The west phases of the QBO-indices were observed during 1982, 1985, 1987, 1990, 1992, 1995, 1997, 1999, 2001, 2004, 2006, 2008, 2010, 2013, 2015 and 2017 while east phases of QBO-index occurred in 1981, 1983, 1984, 1986, 1988, 1989, 1991, 1993, 1994, 1996, 1998, 2000, 2002, 2003, 2005, 2007, 2009, 2011, 2012 and 2014 and 2016. These two phases of QBO since 1981–2017 the west and east phases with probability occurrence 0.43 and 0.57 respectively.
During analysis the frequency of east and west phases of QBO index almost similar close positive and negative SOI for the analysis period. During the years: 1983, 1986, 1991, 1994, 1997, 2002, 2003, 2005 and 20014, the east phases of the QBO-index coincided with the negative phases of the SOI. The results' of these years associated with ENSO [19]. This observation is consisted with ENSO tends to be associated directly with east phases of the QBO.
Table 2 depicts a significant correlation with the QBO-index with time lag and JJA rainfall of Upper Blue Nile from the year 1981–2003. The June, July and August (JJA) rainfall which is evaluated depending on reference mean value zero and near to zero values were taken to be normal (NN), but results the phases of QBO clearly indicates each phase taken to be easterly /westerly above normal (AN) and below normal (BN) consequently. Over the region of Upper Blue Nile rainfall, stratospheric westerly wind phases corresponding to above normal rainfall, were observed 9 out of 12 and easterly wind phases BN 8 out of 11 with conditional probabilities of about 0.75 and 0.72 for the associations of above and below normal during the long term of rainfall over Upper Blue Nile.
The results clearly show above/below normal rainfall is associated with the stratospheric west- erly/easterly zonal wind phases. Such association between phases of QBO and seasonal (JJA) rainfall indicate encouraging potential for rainfall predictability using the information about the QBO phases over Upper Blue Nile.
QBO phases | Above normal (AN) | Near normal (NN) | Blow normal (BN) | Total |
West | 9 | 0 | 3 | 12 |
East | 1 | 2 | 8 | 11 |
Total | 10 | 2 | 11 | 23 |
The predicted time series showed small variability than observed and it reflected low RMSE, bias and MAE for each season. Such statistical measures were used to determine the error between ob- served and estimated (predicted) values therefore, these errors tends to zero otherwise less than the standard deviation of the observed data for a capability performance of the June - August QBO results. The QBO index performs well if the MAE and RMSE are found less than or equal to the standard deviation of the observed data. Depending on these statistical techniques QBO indices have a potential for estimating (predicting) rainfall over the region of Upper Blue Nile.
Four seasons (December-February (DJF), March-April (MAM), June-August (JJA) and September- November (SON)) of QBO phases relationship were evaluated with zero and nonzero time lag.
From four seasons the JJA and MAM QBO phases have potential for prediction JJA rainfall, Figure 4 indicates great values for predicting the rainfall, the most useful index appears to be the trend for QBO-index before the JJA rainfall season as depicted upper right panel of Figure 4. We visualized lag of QBO for different seasons in order to evaluate the relationships among annual, seasonal and monthly QBO and rainfall over the study area. We found that two years lags have higher correlation of 0.7 in MAM season of QBO from years 1989–2000 as shown in Figure 4. However, top left, bottom right and left panels shows a lag time and JJA QBO phases indicates good association than other seasons.
The lagged relationships between the two variables indicate high prospects for using them in the development of prediction methodology. It was observed that one-year time lag QBO seasonal indices (QBOJJA) shows a correlation of 0.56 from the year 1981–2003. In Figure 5, from the QBO indices which were hypothesized from four seasons two seasonal time lags indicate a high significant corre- lation with the summer (JJA) seasonal rainfall over Upper Blue Nile of Ethiopia. In this study about 91.3 % of the positive/negative anomalies and 8.7% normal condition was observed during the period anomaly of QBO-index. This highly significant correlation indicates some robust associations between the seasonal rainfall (long term rainfall) and the QBO. The relationship among the long-rains and the QBO-index shows predictive potential.
The regression coefficients along with the respective predictors (SONSOI and JJAQBO) of the regression model are shown in Table 3. These predictors are derived from QBO indices at 30 hpa from the lower parts of stratosphere layers of atmosphere and southern oscillation index (SOI). Both of the coefficients indicate that as the values of SONSOI and JJAQBO increases, the mean of JJARF also tends to increase. The regression output also shows that SONSOI and JJAQBO are statistically significant because their P-values are not greater than the usual significance level of 0.05.
Predictors | Months | Coefficients | P-value | Lower 95% | Upper 95% |
Constant | - | −0.0354 | 0.001 | −0.04 | −0.03 |
SOISON | SON (Sep, Oct & Nov) | 0.3755 | 0.034 | 0.23 | 0.52 |
QBOJJA | JJA (Jun, Jul & Aug) | 0.4686 | 0.0014 | 0.22 | 0.71 |
The computed values of model performance measures: Mean Absolute Error (MAE), Root Mean Square Error (RMSE) and bias confirm the models forecast capability. The confidence interval, outliers and normality of this model was evaluated in order to increase the capability of handling the actual value. This estimated value derived from QBO 30hPa and SOI was validated with the values of their respective observational as depicted on table 4 the results of the validation for the regression models.
The predicted period showed small variability than observed, which is reflected in the low RMSE and MAE. Such statistical measures were used to determine the error between observed and estimated values therefore, these errors tend to zero otherwise less than the standard deviation of the observed data for a capability performance of the models results. The model performs well because the MAE and RMSE are found less than or equal to the standard deviation of the observed data. Depending on these statistical techniques, the regression model well estimated the June-August rainfall over Upper Blue Nile.
Statistics | SD | RMSE | Bias | MAV |
Values | 0.6043 | 2.09 × 10−5 | 4.3 × 10−6 | 0.0152 |
The multiple linear regression model was implemented to test long term rainfall over the study area for evaluating the efficiency of the model. We obtained the estimated rainfall amount which is closer to the actual JJA rainfall. Before applying the regression model, we evaluated the two independent variables (QBO and SOI) by using collinearity methods. When these independent variables in the same regression model are correlated, they cannot independently predict the value of the dependent variable because some of the same variance in the dependent variable (JJA rainfall), which in turn reduces their statistical significance. For this reason, high correlation between QBO and SOI, as this period there is a dramatic increase in the P-value and reduction of significance level of one predictor variable when another predictor is included in the regression model, or when a high variance inflation factor is determined. For selected predictors (QBOJJA and SOISON) for Upper Blue Nile region of Ethiopia there is not collinearity problems.
Therefore, selected predictors QBO at 30hPa and SOI were used to build up the multiple linear regression model, according to the collinearity analysis for September-November and June-August months to estimate the rainfall Upper Blue Nile of Ethiopia. Multiple correlation coefficients indi- cated a strong correlation between estimated and actual long-term rainfall amount for June-August (JJA) which means that the predictors selected for estimation of rainfall amount capture strongly the mechanisms that control the rainfall dynamics.
Using atmospheric variable prediction of summer rainfall is crucial for agricultural, hydropower and other sectors for the management of water. The annual rainfall in 1983, 1984, 2002, 2009 and 2015 showed negative deviation from normal condition consequently drought years over Upper Blue Nile. The drought 1983, 2002 and 2009 are associated with easterly phase but the 2015 drought associated with westerly. In the region of Upper Blue Nile basin, stratospheric westerly wind phases corresponding to above normal (AN) rainfall and easterly wind phases below normal (BN) with conditional probabilities of about 0.75 and 0.72 for the associations of above and below normal during the long term of rainfall (JJA). This study shows about 91.3 % of the positive and negative anomalies and 8.7 % normal condition are observed during the period anomaly of QBO-index for 23 years.
By using statistical value of collinearity method, we evaluated the independent variable (QBO and SOI) in order to investigate the significance level of predictors and capability of JJA rainfall estimation. For the selected predictors (QBOJJA with time lags and SOISON), the relationships among predicted and actual rainfall was calculated by averaging value of the two. From this model, we determined that there is strong correlation that exists prior to six months of June-August (JJA) rainfall from SOI. We also investigated that JJA and SON seasons have strong potential to estimate the precipitation over Upper Blue Nile.
The Southern Oscillation Index (SOI), globally averaged equatorial stratospheric at 30-hpa zonal wind index (QBO), and rainfall data are obtained from https://www.crudata.uea.ac.uk/cru/data/soi/, https://www.geo.fu-berlin.de/en/met/ag/strat/produkte/qbo/index.html, and https://www.chg.geog.ucsb.edu/datarespectively. The authors would like to thank all of the data sources for providing the data sets.
The authors declare no conflict of interest.
[1] |
Geller MA, Zhou T, Shindell D, et al. (2016) Modeling the qboimprovements resulting from higher-model vertical resolution. J Adv model earth syst 8: 1092-1105. doi: 10.1002/2016MS000699
![]() |
[2] |
Dunkerton TJ (1997). The role of gravity waves in the quasi-biennial oscillation. J Geophys Res-Atmos 102: 26053-26076. doi: 10.1029/96JD02999
![]() |
[3] | Liang C, Eldering A, Gettelman A, et al. (2011) Record of tropical interannual variability of temperature and water vapor from a combined airs-mls data set. J Geophys Res-Atmos 116. |
[4] |
Randel WJ, Wu F, Oltmans SJ, et al. (2004) Interannual changes of stratospheric water vapor and correlations with tropical tropopause temperatures. J Atmos Sci 61: 2133-2148. doi: 10.1175/1520-0469(2004)061<2133:ICOSWV>2.0.CO;2
![]() |
[5] |
Collimore CC, Martin DW, Hitchman MH, et al. (2003) On the relationship between the qbo and tropical deep convection. J climate 16: 2552-2568. doi: 10.1175/1520-0442(2003)016<2552:OTRBTQ>2.0.CO;2
![]() |
[6] | Ho CH, Kim HS, Jeong JH, et al. (2009) Influence of stratospheric quasi-biennial oscillation on tropical cyclone tracks in the western north pacific. Geophys Res Lett 36. |
[7] | Liess S, Geller MA (2012) On the relationship between qbo and distribution of tropical deep convection. J Geophys Res-Atmos 117. |
[8] |
Yuan W, Geller MA, Love PT (2014) Enso influence on qbo modulations of the tropical tropopause. Q J Roy Meteor Soc 140: 1670-1676. doi: 10.1002/qj.2247
![]() |
[9] |
Gray WM, Sheaffer JD, Knaff JA. (1992) Influence of the stratospheric qbo on enso variability. J Meteorol Soc Jpn 70: 975-995. doi: 10.2151/jmsj1965.70.5_975
![]() |
[10] |
Camberlin P, Philippon N (2002) The east african march-may rainy season: Associated atmospheric dynamics and predictability over the 1968-97 period. J Climate 15: 1002-1019. doi: 10.1175/1520-0442(2002)015<1002:TEAMMR>2.0.CO;2
![]() |
[11] | Ngongolo HK, Smyshlyaev SP (2010) The statistical prediction of east african rainfalls using quasi-biennial oscillation phases information. Nat Sci 2: 1407. |
[12] | Seleshi Y, Zanke U. (2004) Recent changes in rainfall and rainy days in ethiopia. International Journal of Climatology: A J Royal Meteorol Soc 24: 973-983. |
[13] |
Korecha D, Barnston AG (2007) Predictability of june-september rainfall in ethiopia. Mon Weather Rev 135: 628-650. doi: 10.1175/MWR3304.1
![]() |
[14] | Awulachew SB, Yilma AD, Loulseged M, et al. (2007) Water resources and irrigation development in Ethiopia 123. Iwmi. |
[15] |
Block P, Rajagopalan B (2007) Interannual variability and ensemble forecast of upper blue nile basin kiremt season precipitation. J Hydrometeorol 8: 327-343. doi: 10.1175/JHM580.1
![]() |
[16] |
Cane MA (2005) The evolution of el niño, past and future. Earth Planet Sc Lett 230: 227-240. doi: 10.1016/j.epsl.2004.12.003
![]() |
[17] | Wolde-Georgis T (1997) El nino and drought early warning in ethiopia. Int J Afr Stud 2. |
[18] |
Trenberth K E (1997) The definition of el nino. B Am Meteorol Soc 78: 2771-2778. doi: 10.1175/1520-0477(1997)078<2771:TDOENO>2.0.CO;2
![]() |
[19] |
Zaroug MAH, Eltahir EAB, Giorgi F (2014) Droughts and floods over the upper catchment of the Blue Nile and their connections to the timing of El Niño and La Niña events. Hydrol Earth Syst Sci 18: 1239-1249. doi: 10.5194/hess-18-1239-2014
![]() |
[20] |
Zebiak SE, Cane MA (1987) A model el nino-southern oscillation. Mon Weather Rev 115: 2262-2278. doi: 10.1175/1520-0493(1987)115<2262:AMENO>2.0.CO;2
![]() |
[21] |
Abtew W, Melesse AM, Dessalegne T (2009) El niño southern oscillation link to the blue nile river basin hydrology. Hydrol Process 23: 3653-3660. doi: 10.1002/hyp.7419
![]() |
[22] | Charney JG, Drazin PG (1990) Propagation of planetary-scale disturbances from the lower into the upper atmosphere. The Atmosphere—A Challenge. American Meteorological Society, Boston, MA, 1990: 295-321. |
[23] |
Conway D (1997) A water balance model of the upper blue nile in ethiopia. Hydrolog Sci J 42:2 65-286. doi: 10.1080/02626669709492024
![]() |
[24] | Steenhuis TS, Collick AS, Easton ZM, et al. (2009) Predicting discharge and sediment for the abay (blue nile) with a simple model. Hydrol Process 23: 3728-3737. |
[25] |
Conway D (2000) The climate and hydrology of the upper blue nile river. Geogr J 166: 49-62. doi: 10.1111/j.1475-4959.2000.tb00006.x
![]() |
[26] |
Tesemma ZK, Mohamed YA, Steenhuis TS (2010) Trends in rainfall and runoff in the blue nile basin: 1964-2003. Hydrol Process 24: 3747-3758. doi: 10.1002/hyp.7893
![]() |
[27] |
Habtegebreal AK, Alemu AB (2019) Identification of rainfall intensity by using bahir dar c-band weather radar products. Int J Comput Sci Appl Math 5: 35-40. doi: 10.12962/j24775401.v5i2.4935
![]() |
[28] |
Ayehu G, Tadesse T, Gessesse B, et al. (2018) Validation of new satellite rainfall products over the upper blue nile basin, ethiopia. Atmo Meas Tech 11: 1921-1936. doi: 10.5194/amt-11-1921-2018
![]() |
[29] | Hall G (2015) Pearsons correlation coefficient. other words 1. |
[30] | Cohen J, Cohen P (1983) Applied multiple regression/correlation analysis for the behavioral sciences (2td eu.). Hillsdale, N]: Lawrence Erlbaum Associates. |
[31] | Rogerson PA (2001) A statistical method for the detection of geographic clustering. Geogr Anal 33: 215-227. |
[32] | Bayissa YA, Moges SA, Xuan Y, et al. (2015) Spatio-temporal assessment of meteorological drought under the in- fluence of varying record length: The case of upper blue nile basin, ethiopia. Hydrolog Sci J 60: 1927-1942. |
Years | Jan | Feb | Mar | Apr | May |
1979–1983 | 0.53 | 0.56 | 0.21 | 0.03 | −0.19 |
1984–1988 | 0.05 | 0.09 | −0.04 | −0.11 | −0.45 |
1989–1993 | −0.81 | −0.80 | −0.90 | −0.85 | −0.94 |
1994–1998 | −0.99 | −0.91 | −0.85 | −0.76 | −0.80 |
1999–2003 | −0.87 | −0.87 | −0.90 | −0.87 | −0.68 |
2004–2008 | −0.78 | −0.78 | 0.28 | 0.40 | 0.48 |
2009–2015 | −0.02 | −0.15 | −0.23 | −0.20 | −0.06 |
QBO phases | Above normal (AN) | Near normal (NN) | Blow normal (BN) | Total |
West | 9 | 0 | 3 | 12 |
East | 1 | 2 | 8 | 11 |
Total | 10 | 2 | 11 | 23 |
Predictors | Months | Coefficients | P-value | Lower 95% | Upper 95% |
Constant | - | −0.0354 | 0.001 | −0.04 | −0.03 |
SOISON | SON (Sep, Oct & Nov) | 0.3755 | 0.034 | 0.23 | 0.52 |
QBOJJA | JJA (Jun, Jul & Aug) | 0.4686 | 0.0014 | 0.22 | 0.71 |
Statistics | SD | RMSE | Bias | MAV |
Values | 0.6043 | 2.09 × 10−5 | 4.3 × 10−6 | 0.0152 |
Years | Jan | Feb | Mar | Apr | May |
1979–1983 | 0.53 | 0.56 | 0.21 | 0.03 | −0.19 |
1984–1988 | 0.05 | 0.09 | −0.04 | −0.11 | −0.45 |
1989–1993 | −0.81 | −0.80 | −0.90 | −0.85 | −0.94 |
1994–1998 | −0.99 | −0.91 | −0.85 | −0.76 | −0.80 |
1999–2003 | −0.87 | −0.87 | −0.90 | −0.87 | −0.68 |
2004–2008 | −0.78 | −0.78 | 0.28 | 0.40 | 0.48 |
2009–2015 | −0.02 | −0.15 | −0.23 | −0.20 | −0.06 |
QBO phases | Above normal (AN) | Near normal (NN) | Blow normal (BN) | Total |
West | 9 | 0 | 3 | 12 |
East | 1 | 2 | 8 | 11 |
Total | 10 | 2 | 11 | 23 |
Predictors | Months | Coefficients | P-value | Lower 95% | Upper 95% |
Constant | - | −0.0354 | 0.001 | −0.04 | −0.03 |
SOISON | SON (Sep, Oct & Nov) | 0.3755 | 0.034 | 0.23 | 0.52 |
QBOJJA | JJA (Jun, Jul & Aug) | 0.4686 | 0.0014 | 0.22 | 0.71 |
Statistics | SD | RMSE | Bias | MAV |
Values | 0.6043 | 2.09 × 10−5 | 4.3 × 10−6 | 0.0152 |