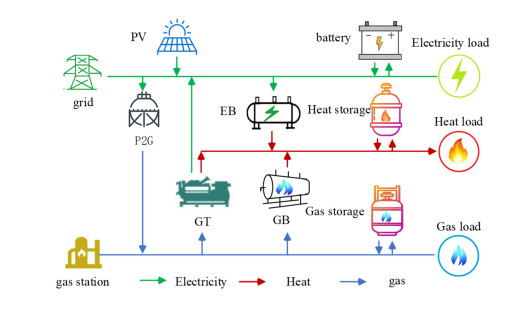
Considering the problem of time scale differences among subsystems in the integrated energy system of a park, as well as the increasing complexity of the system structure and number of control variables, there may be a deep reinforcement learning (DRL) "curse of dimensionality" problem, which hinders the further improvement of economic benefits and energy utilization efficiency of park-level integrated energy systems (PIES). This article proposes a reinforcement learning optimization algorithm for comprehensive energy PPO (Proximal Policy Optimization) in industrial parks considering multiple time scales for energy management. First, PIES are divided into upper and lower layers, the first containing power and thermal systems, and the second containing gas systems. The upper and lower layers of energy management models are built based on the PPO; then, both layers formulate the energy management schemes of the power, thermal, and gas systems in a long (30 min) and short time scale (6 min). Through confirmatory and comparative experiments, it is shown that the proposed method can not only effectively overcome the curse of dimensionality in DRL algorithms during training but can also develop different energy system management plans for PIES on a differentiated time scale, improving the overall economic benefits of the system and reducing carbon emissions.
Citation: Linrong Wang, Xiang Feng, Ruifen Zhang, Zhengran Hou, Guilan Wang, Haixiao Zhang. Energy management of integrated energy system in the park under multiple time scales[J]. AIMS Energy, 2024, 12(3): 639-663. doi: 10.3934/energy.2024030
[1] | Badr Ouhammou, Fatima Zohra Gargab, Samir El idrissi kaitouni, Slimane Smouh, Rachid El mrabet, Mohammed Aggour, Abdelmajid Jamil, Tarik Kousksou . Energy saving potential diagnosis for Moroccan university campuses. AIMS Energy, 2023, 11(3): 576-611. doi: 10.3934/energy.2023030 |
[2] | Mohamed G Moh Almihat, MTE Kahn . Centralized control system for islanded minigrid. AIMS Energy, 2023, 11(4): 663-682. doi: 10.3934/energy.2023033 |
[3] | Panagiotis Fragkos . Analysing the systemic implications of energy efficiency and circular economy strategies in the decarbonisation context. AIMS Energy, 2022, 10(2): 191-218. doi: 10.3934/energy.2022011 |
[4] | Jin H. Jo, Jamie Cross, Zachary Rose, Evan Daebel, Andrew Verderber, David G. Loomis . Financing options and economic impact: distributed generation using solar photovoltaic systems in Normal, Illinois. AIMS Energy, 2016, 4(3): 504-516. doi: 10.3934/energy.2016.3.504 |
[5] | Tiansong Cui, Yanzhi Wang, Shahin Nazarian, Massoud Pedram . Profit maximization algorithms for utility companies in an oligopolistic energy market with dynamic prices and intelligent users. AIMS Energy, 2016, 4(1): 119-135. doi: 10.3934/energy.2016.1.119 |
[6] | Gustavo Henrique Romeu da Silva, Andreas Nascimento, Christoph Daniel Baum, Nazem Nascimento, Mauro Hugo Mathias, Mohd Amro . Renewable energy perspectives: Brazilian case study on green hydrogen production. AIMS Energy, 2025, 13(2): 449-470. doi: 10.3934/energy.2025017 |
[7] | Akash Talwariya, Pushpendra Singh, Mohan Lal Kolhe, Jalpa H. Jobanputra . Fuzzy logic controller and game theory based distributed energy resources allocation. AIMS Energy, 2020, 8(3): 474-492. doi: 10.3934/energy.2020.3.474 |
[8] | Jiashen Teh, Ching-Ming Lai, Yu-Huei Cheng . Composite reliability evaluation for transmission network planning. AIMS Energy, 2018, 6(1): 170-186. doi: 10.3934/energy.2018.1.170 |
[9] | Bei Li, Siddharth Gangadhar, Pramode Verma, Samuel Cheng . Maximize Producer Rewards in Distributed Windmill Environments: A Q-Learning Approach. AIMS Energy, 2015, 3(1): 162-172. doi: 10.3934/energy.2015.1.162 |
[10] | Nadwan Majeed Ali, Handri Ammari . Design of a hybrid wind-solar street lighting system to power LED lights on highway poles. AIMS Energy, 2022, 10(2): 177-190. doi: 10.3934/energy.2022010 |
Considering the problem of time scale differences among subsystems in the integrated energy system of a park, as well as the increasing complexity of the system structure and number of control variables, there may be a deep reinforcement learning (DRL) "curse of dimensionality" problem, which hinders the further improvement of economic benefits and energy utilization efficiency of park-level integrated energy systems (PIES). This article proposes a reinforcement learning optimization algorithm for comprehensive energy PPO (Proximal Policy Optimization) in industrial parks considering multiple time scales for energy management. First, PIES are divided into upper and lower layers, the first containing power and thermal systems, and the second containing gas systems. The upper and lower layers of energy management models are built based on the PPO; then, both layers formulate the energy management schemes of the power, thermal, and gas systems in a long (30 min) and short time scale (6 min). Through confirmatory and comparative experiments, it is shown that the proposed method can not only effectively overcome the curse of dimensionality in DRL algorithms during training but can also develop different energy system management plans for PIES on a differentiated time scale, improving the overall economic benefits of the system and reducing carbon emissions.
With the continuous growth of China's energy demand and carbon emissions, environmental issues are becoming increasingly prominent, constraining the development of China's economy and society [1]. Economy and low carbon have become the trends of future energy development [2]. Park-integrated energy systems (PIES) have the characteristics of multi-energy coupling and joint scheduling [3], becoming an important lever for efficient and clean energy utilization and achieving the "dual carbon" goal [4]. PIES couple multiple types of energy, and through complementary operation, the efficient utilization and flexible conversion of various types of energy can be greatly improved [5].
Compared with traditional energy systems, PIES have three types of energy: Electrical, thermal, and gas. These systems have more complex structures and include multiple energy sources and energy conversion equipment [6]. There are multiple uncertainties, as both photovoltaic and wind power generation included in the system have inherent uncertainty [7]. PIES also show flexibility, allowing them to adjust energy supply to meet the system's supply and demand [8]. Additionally, PIES have complex profit-seeking characteristics; the system can use gas turbines and other equipment to convert gas into electricity when the electricity price is high, reducing the consumption of electricity and lowering costs [9]. In order to improve the economic benefits and energy coupling ability of PIES, many scholars have conducted research based on multi-time scale models. In [10], authors developed a multi-time scale hierarchical rolling optimization scheduling model based on the time difference of energy consumption and load of different equipment during the intraday scheduling stage, and adjusted the unit output by perceived load changes. In [11], the system was divided into three time scales, day ahead long-time scale, day ahead predictive control, and real-time scheduling, to perform rolling optimization and reduce operating costs. In [12], an intraday procurement plan was developed based on the bilateral game mechanism of operators, as well as a dynamic scheduling model during the intraday management phase based on the differences in time scales of electricity, heat, and gas energy equipment. In [13], the problem of scheduling time scale differentiation in heterogeneous energy subsystems was solved using a double-layer scheduling time scale. In [14], authors utilized a multi-time scale coordinated optimization method to establish PIES scheduling strategies for three-time scales—day ahead, day in, and real-time—and analyzed the impact of multiple energy storage devices on the economic benefits of the system. From the above, it can be seen that multi-time scale models are beneficial for solving the problem of time scale differences in PIES, but the scheduling decision of the system still mainly relies on an accurate prediction of source load storage. With the increasing variety of energy equipment in PIES, the difficulty of prediction has increased, affecting the optimization of energy management in the system.
In recent years, some scholars have attempted to use deep reinforcement learning (DRL) methods of artificial intelligence to solve the energy management of PIES. In [15], the energy management of generator sets and gas turbines in PIES was optimized based on a deep deterministic strategy gradient algorithm. In [16], the differential evolution deep Q-network (DQN) algorithm was used to improve the overall economic benefits of PIES and the utilization rate of energy storage equipment. Authors in [17] proposed a load scheduling and energy management strategy based on Q-learning algorithm for distributed energy management in microgrids. In [18], a real-time energy management system for microgrids was designed based on the DQN algorithm, achieving the goal of minimizing operating costs. The energy management method based on DRL effectively reduces the dependence on accurate prediction of new energy output and source load storage. However, the increasingly complex structure and increasing variety of energy and equipment in PIES may lead to a "dimensionality disaster" problem. At the same time, reinforcement learning requires agents to execute actions with the same dimensionality [19].
In order to address the time scale differences in PIES and the "curse of dimensionality" of DRL methods, this paper proposes a multi-time scale PPO reinforcement learning optimization algorithm for comprehensive energy management in PIES, which includes three types of energy sources: Electric, heat, and gas. The main contributions of this article are as follows:
(1) To solve the problem of time scale differences among energy subsystems in the PIES, this article divides the PIES into two layers: The upper layer, which includes the power system and the thermal system, and the lower layer, which includes the gas system. The upper and lower layers are coupled and cooperate with each other to meet the energy supply and demand balance in the PIES.
(2) Compared with traditional strategy gradient optimization algorithms, the PPO algorithm in reinforcement learning has the advantages of being insensitive to update step size and not requiring resampling during updates, making it suitable for PIES containing continuous data such as photovoltaic and load. Therefore, this article applies the PPO algorithm to train the upper and lower layers of PIES separately, reducing the difficulty of model training, to set corresponding management time scales for different energy systems in the upper and lower layers, and developing management plans for different energy systems within PIES. Simulation examples show that this method can effectively reduce the operating cost of PIES while meeting the differences in time scales for managing different energy systems.
PIES mainly include three types of energy—electrical, heat, and gas—and manage the energy transmission, conversion, and storage to meet energy loads demands, integrating different components: energy supply side, energy conversion side, energy storage side, and energy load side. The system structure is shown in Figure 1.
The energy supply side provides energy to PIES. The energy supply side mainly includes electricity purchased from the power grid, natural gas purchased from natural gas stations, and new energy equipment for photovoltaic power generation.
(1) Electricity grid
The electricity grid is responsible for providing electricity to PIES, and the constraints for purchasing power from the grid are shown in Eq (1).
0⩽PElet⩽PElemax | (1) |
In the equation above, PElet is the power purchased from the external power grid at time t; PElemax is the maximum transmission power of the external power grid interconnection line.
(2) Natural gas station
The natural gas station is responsible for providing natural gas to PIES, and the constraint conditions for purchasing gas power are shown in Eq (2).
0⩽GGast⩽GGasmax | (2) |
GGast is the gas purchasing power of the natural gas station at time t; GGasmax is the maximum power output of the natural gas station.
The energy conversion side includes GT (gas turbine), GB (gas boiler), EB (electric boiler), and P2G (electric to gas) equipment, which are used to convert energy between electricity, natural gas, and heat energy.
(1) Gas turbine
The GT equipment is a device that converts natural gas into electrical and heat energy. The relationship between the consumption of natural gas and the generation of heat and electrical energy by GT equipment at time slot t is shown in Eqs (3) and (4), respectively.
PGTt=GGTtηGT - E | (3) |
HGTt=GGTt(1−ηGT - E−μGT−loss) | (4) |
PGTt is the power output of the GT equipment; HGTt is the heat generation power of the GT equipment; GGTt is the gas consumption power of the GT equipment, and ηGT - E is the power generation efficiency of the GT equipment; μGT−loss is the gas loss rate of GT equipment.
The constraint conditions for GT operating power and climbing power are shown in Eqs (5) and (6), respectively.
GGTmin⩽GGTt⩽GGTmax | (5) |
0⩽|GGTt−GGTt−1|⩽ΔGGTmax | (6) |
GGTmin is the minimum operating power of GT; GGTmax is the maximum operating power of GT; ΔGGTmax is the upper limit of GT climbing power.
(2) Gas boiler
The GB is a device that converts natural gas into heat energy. Under time slot t, the relationship between the consumption of natural gas and the generation of heat energy by GB devices is shown in Eq (7).
HGBt=GGBtηGB(1−μGB−loss) | (7) |
HGBt is the heat generation power of the GB device; GGBt is the gas consumption power of GB equipment; ηGB is the gas-to-heat conversion efficiency of GB equipment; μGB−loss is the gas loss rate of GB equipment.
The constraint conditions for GB operating power and climbing power are shown in Eqs (8) and (9), respectively.
GGBmin⩽GGBt⩽GGBmax | (8) |
0⩽|GGBt−GGBt−1|⩽ΔGGBmax | (9) |
GGBmin is the minimum operating power of GB; GGBmax is the maximum operating power of GB; ΔGGBmax is the upper limit of GB climbing power.
(3) Electric boiler
The electric boiler is a device that converts electrical energy into heat energy. The relationship between the electrical power consumed by the EB device and the generated heat energy at time slot t is shown in Eq (10).
HEBt=PEBtηEB(1−μEB - loss) | (10) |
HEBt is the heat production work of the EB equipment; PEBt is the power consumption of the EB device; ηEB is the electric heating conversion efficiency of the EB equipment; μEB - loss is the electrical energy loss rate of the EB device.
The constraints on EB operating power and climbing power are shown in Eqs (11) and (12), respectively.
PEBmin⩽PEBt⩽PEBmax | (11) |
0⩽|PEBt−PEBt−1|⩽ΔPEBmax | (12) |
PEBmin is the minimum operating power of EB; PEBmax is the maximum operating power of EB; ΔPEBmax is the upper limit of EB climbing power.
(4) P2G equipment
The P2G is a device that converts electrical energy into natural gas [20]. The P2G device first decomposes water into oxygen and hydrogen through electrolysis, and then reacts to synthesize methane from carbon dioxide and hydrogen [21]. The relationship between the electrical power consumed by the P2G device and the amount of gas generated at time slot t is shown in Eq (13).
GP2Gt=PP2GtηP2G(1−μP2G - loss) | (13) |
GP2Gt is the gas production power of the P2G equipment; PP2Gt is the power consumption of the P2G device; ηP2G is the electrical conversion efficiency of the P2G equipment; μP2G - loss is the electrical energy loss rate of the P2G device.
The P2G operating power and climbing power constraints are shown in Eqs (14) and (15), respectively.
PP2Gmin⩽PP2Gt⩽PP2Gmax | (14) |
0⩽|PP2Gt−PP2Gt−1|⩽ΔPP2Gmax | (15) |
PP2Gmin is the minimum operating power of P2G; PP2Gmax is the maximum operating power of P2G; ΔPP2Gmax is the upper limit of P2G climbing power.
A battery is an efficient energy storage element that primarily stores and releases electrical energy through the conversion of electrical and chemical energy. Lithium, sodium sulfur, and lead-acid batteries are currently the most widely used. Although lead-acid batteries have the advantages of low cost and large storage capacity, they have short life cycles and low energy density and result in significant environmental pollution, not being suitable for application in PIES. The technology of sodium sulfur batteries is not yet mature and is not suitable for widespread application at present. Lithium batteries have the characteristics of low self-discharge rate, low energy density, high charging and discharging efficiency, and long battery life cycle [22]. This article will use lithium batteries as storage components for PIES.
Thermal storage devices are divided into sensible thermal energy storage and latent thermal energy storage. Latent thermal energy storage has the advantages of high energy storage density and temperature stability but has the drawbacks of high cost and complex energy storage. Explicit heat storage has the advantages of simplicity, low cost, and long lifespan but low energy storage density, large equipment volume, and unstable temperature [23]. This article considers that PIES have lower requirements for high energy storage density and temperature stability. Therefore, explicit energy storage devices are selected as the thermal storage components of PIES to further reduce the operating cost of PIES.
Natural gas storage technology includes gas tank, underground gas, liquefied natural gas, pipeline gas, and hydrate gas storage, as well as other related storage technologies. In this article, the storage of natural gas is carried out by the widely used gas storage tanks.
The energy storage side includes three types of equipment: batteries, gas storage tanks, and heat storage tanks, which are responsible for storing or releasing electrical energy, gas, and heat, respectively. The mathematical model of energy storage equipment is shown in Eq (16).
SXt+1=(1−μX - loss)SXt+[PX, chtηX, chδX,cht−(1−δX,cht)PX, distηX, dis]Δt | (16) |
X represents the energy category; ES, HS, and GS represent the battery, heat storage tank, and air storage tank, respectively; SXt andSXt+1 represent the energy storage at time slot t and at time slot t + 1; μX - loss is the loss coefficient of energy storage device X . PX, cht and PX, dist are the energy storage power and discharge power of energy storage deviceX at time slot t; ηX, ch and ηX, dis are the energy storage efficiency and release efficiency of energy storage device X ; δX,cht is a 0–1 variable that represents the energy storage status of the energy storage device X at time slot t; Δt is the unit time slot length.
The state constraints, capacity constraints, and energy storage and discharge power constraints of energy storage device X are shown in Eqs (17–19), respectively.
δX,cht+(1−δX,cht)=1 | (17) |
SXmin⩽SXt⩽SXmax | (18) |
0≤PX,ch/dist≤PX,ch/dismaxPX,ch/dist={PX,cht,δX,cht=1PX,dist,δX,cht=0 | (19) |
SXmin andSXmax are the lower and upper capacity limits of the energy storage device X ; PX, ch/dist is the maximum energy storage or discharge power of the energy storage device X .
The energy load in PIES mainly includes electricity, gas, and heat loads, all of which have the characteristics of temporal uncertainty.
The goal of PIES energy management is to adjust the output of each unit in the energy system while ensuring the safe operation of the system, so as to minimize the operating cost of the system. The system operating cost includes the cost of purchasing electricity from the power grid CEle , the cost of purchasing gas from natural gas stations CGas , the cost of operating energy storage equipment CRC , and the cost of carbon emissions from the system CC . The objective function is shown in Eq (20).
F=min(CEle+CGas+CRC+Cc) | (20) |
The calculation methods for electricity purchase cost and gas purchase cost are shown in Eqs (21) and (22), respectively.
CEle=T∑t=1cEletPEletΔt | (21) |
CGas=T∑t=1cGastGPHGHVGGastΔt | (22) |
CEle andCGas are the electricity and gas prices at time slot t; PElet and GGast are the electricity and gas purchasing power at time slot t; GPH is the equivalent power and heat energy conversion coefficient of gas; GHV is the high calorific value of gas combustion.
The operating cost CRC of energy storage equipment includes the operating cost CES of the battery, the operating cost CHS of the heat storage tank, and the operating cost CGS of the air storage tank. The representation of each cost is shown in Eqs (23–26).
CRC=CES+CHS+CGS | (23) |
CES=T∑t=1PESRΔtCEScapeDESRSESmax(m(ΔEESt)−ne−qΔEESt) | (24) |
CHS=T∑t=1cHSΔt(1−δHS,cht)PHS, ch/dist | (25) |
CGS=T∑t=1cGSΔt(1−δGS,cht)PGS, ch/dist | (26) |
PESR is the rated charging and discharging power of the battery; CEScape is the investment cost of building batteries; DESR is the rated discharge depth of the battery; m, n, and q are the fitting curve parameters for converting the irregular charging and discharging process of the battery into the standard cycle usage times [24]; EESt is the state of charge of the battery; CHS andCGS are the unit operating costs for the service life of heat and gas storage tanks.
The carbon emission cost of the system is shown in Eq (27).
Cc=T∑t=1cco2(σElePElet+σGTPGTt−σP2GPP2Gt)Δt | (27) |
σEle is the carbon emission coefficient of power grid purchasing; σGT is the carbon emission coefficient of GT equipment; σP2G is the carbon absorption coefficient of P2G equipment operation; cco2 is the carbon price.
The Markov decision process is the mathematical foundation for reinforcement learning. The Markov decision process (MDP) consists of (S,A,R,γ) elements, where S represents the set of states of the environment, A represents the set of actions of the agent, R represents the return function, γ is the discount factor, andγ∈(0,1] . The state transition process is at time t. The intelligent agent selects actionat to interact with the environment based on the current environmental statest , obtains a rewardrt , and enters the next statest+1 . The agent receives a reward for interacting with the environment at each time step until the end of the state. Gt represents the long-term benefits of the intelligent agent, as shown in Eq (28).
Gt=rt+γrt+1+γ2rt+2+⋯+γT−trT=T−t∑i=0γirt+i | (28) |
T is the length of the decision sequence.
Using the action value function Q to evaluate the quality of action a in state s and using the state value function V to evaluate the quality of the state, the value of the Q value function can be used to calculate the V value function, as defined in Eqs (29) and (30).
Qπ(s,a)=Eπ{Gt|St=s,At=a} | (29) |
Vπ(s)=∑a∈Aπ(a|s)Qπ(s,a) | (30) |
π(a|s) represents the probability of executing action a in the current state s , representing the agent's strategy.
Compared with traditional strategy gradient optimization algorithms, the PPO algorithm has the advantages of being insensitive to update step size and not requiring resampling during updates. It is suitable for PIES containing continuous data such as photovoltaic and load and can effectively avoid the curse of dimensionality.
PPO is a benchmark algorithm for reinforcement learning based on the actor critic (AC) framework proposed by OpenAI in 2017 [25]. The AC method includes both value-based and strategy-based learning methods. The AC framework consists of two networks, namely the actor network and the critical network. The actor network, also known as the policy network, is mainly used to generate policy functions. The critical network, also known as value network, is mainly used to evaluate the actions taken by actors in order to improve the strategy function of the actor network. The training flowchart of the PPO algorithm is shown in Figure 2.
(1) Actor network training
The actor network updates network parameter θ by optimizing the loss function JCLIP(θ) . The determination of JCLIP(θ) is shown in Eq (31).
JCLIP(θ)=E(st,at)−π(θold)[min(rt(θ)A(st,at),clip(rt(θ),1−ε,1+ε)A(st,at))] | (31) |
A(st,at) is the dominance function; rt(θ) is the importance sampling ratio; θ is the parameter of the actor network; ε is the pruning factor, which is a hyperparameter used to measure the degree of deviation between the new and old strategies. Due to the large update distance between the new and old strategies, the algorithm may become unstable. To avoid this situation, the importance sampling weight is limited to [1−ε,1+ε] .
The definition of the advantage function in Eq (5) is shown in Eq (32).
A(st,at)=yt−Vω(st)yt=Rt+γVω(st+1) | (32) |
Vω(st) is the output value of the critical network at time t; Rt is the reward at time t; ω is the network parameter of critical; yt is the Vω(st) estimated value for time t + 1.
The importance sampling ratio is the ratio of the new strategy distribution function to the old strategy distribution function, as shown in Eq (33).
rt(θ)=πθ(st|at)πθold(st|at) | (33) |
Using the gradient ascent method to update actor network parameters θ , the size of the update equation is shown in Eq (34).
θ←θ+σA∇θJ(θ) | (34) |
In the equation, σA is the learning rate of the actor network.
(2) Critical network training
The critical network updates the network parameter ω of critical by optimizing the loss function L(ω) . The definition of L(ω) is shown in Eq (35).
L(ω)=E[yt−Vω(st)]2 | (35) |
Use the gradient descent method to update the critical network parameter ω , as shown in Eq (36).
ω←ω−σC∇ωL(ω) | (36) |
In the equation, σC is the learning rate of the critical network.
The PPO algorithm is used to solve the PIES energy management model, as shown in Figure 3.
The initial input states of both the critical and the actor network are randomly sampled from the experience pool to obtain state st . The advantage of randomly sampling the initial states of each training round of the model from the experience pool is that it can reduce the randomness of the trained model in obtaining PIES energy management schemes. At the same time, the output of the critical network is the Vt value, while the output of the actor network is the action at . The agent interacts with the PIES environment according to the time slot and makes action at based on the current environment state st . The PIES environment returns the reward value Rt to the agent, and the experience pool is used to save the state st , action at , and reward Rt for each time period. The samples used for updating network weights in intelligent agents are randomly extracted from the experience pool. After offline training of the DRL model based on the PPO algorithm using training data, the model is saved and applied to the energy management of PIES.
The core idea of using the PPO reinforcement learning optimization algorithm for PIES considering multiple time scales is to first construct upper and lower energy management models based on different energy management time scales. The upper energy management model includes the power and heat systems, and the lower energy management model includes the gas system. Then, based on the PPO algorithm, long-term energy management of power and heat systems is achieved at a time scale of 30 min, while short-term energy management of gas systems is achieved at a time scale of 6 min, achieving differentiated energy management.
The upper and lower PPO scheduling models are mutually coupled, and the scheduling plan for the power and heat systems by the upper PPO serves as the environmental state of the lower PPO system. Due to the relatively long update time, the upper PPO scheduling model may experience a shortage of heat or power supply. At this time, the lower PPO scheduling model can be fully utilized to provide electricity and heat supply to the upper heat and power system by utilizing the GT and GB equipment in the lower PPO scheduling model. At the same time, in order to avoid the situation where the upper PPO scheduling excessively relies on the energy supply of the lower PPO scheduling and the system energy state is unstable, the cumulative adjustment of GT and GB operating power in the lower PPO scheduling is used as the reward function penalty term for the upper PPO scheduling. The specific energy management model is shown in Figure 4.
The state space sUPt of the upper PPO consists of the observed states of the upper power and heat systems (including photovoltaic power generation), as shown in Eq (37).
sUPt={cElet, GGBt,GGTt,Ploadt,Hloadt,PPVt,5∑i=1ΔGGBt+i,5∑i=1ΔGGTt+i,t} | (37) |
cElet is the electricity purchase price of the power grid; GGBt is the gas consumption power in GB; GGTt is the GT gas consumption power; Ploadt is the electrical load; Hloadt is the heat load; PPVt is the photovoltaic power generation; 5∑i=1ΔGGBt+i is the gas consumption power of the GB device at a long time scale; 5∑i=1ΔGGTt+i is the gas consumption power of the GT equipment; t is the time slot.
The action space of the upper PPO intelligent agent is shown in Eq (38).
aUPt={PElet, PP2Gt,PEBt,PES, ch/dist,PHS, ch/dist} | (38) |
PElet represents the power purchased from the external power grid; PP2Gt is the power consumption of the P2G device; PEBt is the power consumption of the EB device; PES, ch/dist and PHS, ch/dist are the storage/discharge power of the battery and the storage/discharge power of the heat storage tank, respectively.
By adding random disturbances in the upper action space to enhance the perception of the environment, the improved upper PPO action space is shown in Eq (39).
aUP′t=τaUPt+(1−τ)mt | (39) |
aUP′t represents the actual action space; τ represents the proportion of each component in the initial action space; mt represents an increased random disturbance and the upper limit of mt∈[−1,1] , and τ is 0.9, ensuring that the action space in the later stage of training still has perceptual ability.
The upper PPO reward function is used to guide the intelligent agent to select actions based on the current state and obtain the maximum cumulative return. The upper PPO reward function consists of three penalty terms. The first penalty term mainly includes the cost of power grid purchase, the operating cost of upper equipment, and the cost of operating power regulation. The significance of considering the cost of equipment operating power regulation is to prevent external power purchase or the fluctuation range of P2G and EB equipment operating power from being too large, causing sharp changes in system load and affecting system stability. The definition of CU1t is shown in Eq (40).
CU1t=CEleRct+CP2GRct+CEBRct+5∑i=1CElet+i+CESt+CHSt | (40) |
5∑i=1CElet+i represents the cost of purchasing electricity from the external power grid over a long period of time; CESt is the operating cost of the battery; CHSt is the operating cost of the heat storage tank; CEleRct is the cost of external power purchase and regulation; CP2GRct and CEBRct are the operating power regulation costs of P2G and EB devices, where CEleRct , CP2GRct , and CEBRct are defined as Eqs (41–43).
CEleRct=cEleRC|PElet−PElet−5| | (41) |
CP2GRct=cP2GRC|PP2Gt−PP2Gt−5| | (42) |
CEBRct=cEBRC|PEBt−PEBt−5| | (43) |
cEleRC , cP2GRC , and cEBRC are the prices for purchasing power from the external power grid, P2G equipment, and EB equipment.
The penalty term of the second part of the reward function includes the unbalanced supply and demand costs of electricity and heat energy, as defined in Eq (44).
CU2t=cEnbPEnbt+cHnbHHnbt | (44) |
cEnb and cHnb are the penalty prices for the imbalance between supply and demand of electricity and heat energy; PEnbt and HHnbt refer to the power with imbalanced supply and demand of electrical and heat energy, where PEnbt and HHnbt are defined as Eqs (45) and (46).
PEnbt=|(Ploadt+PP2Gt+PEBt+δES,chtPES, ch/dist)−(PElet+PPVt+(1−δES,cht)PES, ch/dist+PGTt)| | (45) |
HEnbt=|(HEBt+HGTt+HGBt+(1−δHS,cht)PHS, ch/dist)−(Hloadt+δHS,chtPHS, ch/dist)| | (46) |
The penalty term CU3t of the third part of the reward function includes the cumulative operating power regulation cost of GT equipment and GB equipment. The purpose of considering this regulation cost is to prevent the upper power and heat system from excessively relying on the electricity and heat energy supply of GT and GB equipment in the lower gas system. The definition of CU3t is shown in Eq (47).
CU3t=ccpa(5∑i=1ΔGGBt+i+5∑i=1ΔGGTt+i) | (47) |
ccpa is the penalty price for the cumulative power adjustment of GT and GB devices.
In summary, the reward function obtained by the upper PPO after executing action aUPt based on state sUPt is shown in Eq (48).
RUPt=−[(CU1t+CU2t+CU3t)+I[(PEnbt+HHnbt)<α]+r0]×0.001 | (48) |
I[(PEnbt+HHnbt)<α]+r0] is the indicator function; α is the maximum cumulative electricity and heat energy supply and demand imbalance value; r0 is a constant (which can change the cumulative return from negative to positive, improving the stability and convergence speed of the model).
The state space of the lower PPO includes the observed states of the lower gas system and the energy parameters generated by the upper power and heat system, as shown in Eq (49).
sDownt={cGast,Ploadt,Hloadt,Gloadt,t,PElet,PP2Gt,PEBt,PES, ch/dist,PHS, ch/dist} | (49) |
cGast is the gas purchase price of the natural gas station; Gloadt is the gas load.
The action space of the lower PPO intelligent agent is shown in Eq (50).
aDownt={GGast, GGTt,GGBt,PGS, ch/dist} | (50) |
GGast represents the power of natural gas station to purchase gas; GGTt is the gas consumption power of the GT equipment; GGBt is the gas consumption power of the GB device; PGS, ch/dist is the storage/discharge power of the air storage tank.
Adding random disturbances to the lower action space to enhance the perception of the environment, the improved lower PPO action space aDown′t is shown in Eq (51).
aDown′t=τaDown t+(1−τ)mt | (51) |
The lower PPO reward function includes the first part penalty term CD1t and the second part penalty term CD2t , which are used to guide the intelligent agent to select actions based on the current state.
The first part of the reward function penalty term is shown in Eq (52).
CD1t=CGasRct+CGTt+CGBt+CGast+CGSt | (52) |
CGasRct represents the cost of external gas purchase power regulation; CGTt and CGBt are the operating power regulation costs of GT equipment and GB equipment, where CGasRct , CGTt , and CGBt are defined as Eqs (53–55).
CGasRct=cGasRc|Gpurt−Gpurt−1| | (53) |
CGTt=cGTRc|GGTt−GGTt−1| | (54) |
CGBt=cGBRc|GGBt−GGBt−1| | (55) |
The second part of the reward function penalty term is shown in Eq (56):
CD2t=cGnbGGnbt | (56) |
cGnb is the penalty price for the imbalance between supply and demand in the gas system; GGnbt is the power of the imbalance between gas energy supply and demand, where GGnbt is defined as shown in Eq (57).
GGnbt=|(GGast+GP2Gt+(1−δGS,cht)PGS, ch/dist)−(Gloadt+GGBt+GGTt+δGS,chtPGS, ch/dist)| | (57) |
In summary, the reward function obtained by the lower PPO after executing action aDownt based on state sDownt is shown in Eq (58).
RDownt=−[(CD1t+CD2t)+I[GGnbt<α0]+r0]×0.001 | (58) |
In the equation, α0 is the maximum cumulative gas energy supply and demand imbalance value.
The electricity, heat, and gas loads, and photovoltaic power generation data in this article are sourced from a small domestic park. The structure is shown in Figure 1, and the system equipment parameters and other simulation parameters are shown in Tables 1 and 2 [26,27]. The electricity price of the power system is the time-of-use electricity price as shown in Table 3 [28], and the natural gas unit price is a fixed price of 4.2 yuan/Nm3 (Normal cubic meter). Most of the formulas in the text are based on references [29]. To verify the superiority of the double-layer PPO algorithm proposed in this article compared to the single-layer PPO algorithm in terms of runtime, it is necessary to control variables and ensure that the CPU and memory settings of the double-layer PPO algorithm and the single-layer PPO algorithm are consistent, both completed by a computer equipped with an I7-7700 CPU and 16 GB RAM.
Equipment | Lower power limit/MW | Upper power limit/MW |
Gas turbine | 0 | 1 |
Gas boiler | 0 | 0.55 |
Electric boiler | 0 | 0.4 |
P2G | 0 | 0.5 |
Battery | 0.06 | 0.24 |
Heat storage tank | 0.04 | 0.2 |
Gas storage tank | 0.07 | 0.35 |
Parameter | Numeric value | Parameter | Numeric value | Parameter | Numeric value |
ΔGGTmax | 0.1 MW | ΔGGBmax | 0.05 MW | ΔPEBmax | 0.1 MW |
ΔPP2Gmax | 0.04 MW | ηGT−E | 43% | ηGB | 97% |
ηEB | 93% | ηP2G | 85% | ηGT−loss | 15% |
μGB−loss | 5% | μEB−loss | 3% | ηP2G−loss | 20% |
PES,ch/dismax | 0.08 MW | PGS,ch/dismax | 0.08 MW | PHS,ch/dismax | 0.03 MW |
ηES, ch | 90% | ηHS, ch | 80% | ηGS, ch | 90% |
ηES, dis | 110% | ηHS, dis | 115% | ηGS, dis | 110% |
PElemax | 2 MW | GGasmax | 1.5 MW | cEleRC | 1 yuan |
cP2GRC | 1 yuan | cEBRC | 1 yuan | cGasRC | 1.5 yuan |
cGTRC | 1.5 yuan | cGBRC | 15000 yuan | cHS | 6 yuan |
cGS | 6 | cEScape | 45 | m | 694 |
n | 1.98 | q | 0.016 | DESR | 0.8 |
σA | 0.001 | σC | 0.002 | γ | 0.9 |
Time span | Time | Electrovalence/(yuan/kwh) |
Valley period | 23:00–morrow 07:00 | 0.2 |
Peacetime period | morrow 07:00–12:00 | 0.6 |
19:00–23:00 | ||
Peak period | 12:00–19:00 | 1.1 |
This experiment was implemented on the TensorFlow platform, with five upper PPO action space control objects and four lower PPO action space control objects. The same actor and critical networks, hidden layer, and activation function are used in the upper and lower levels. The number of hidden layers for both the actor and the critical network is 3, each layer containing 256 neurons; the activation function is ReLU. The network weights are updated using the Adam optimizer.
First, under the experimental environment and simulation parameters provided above, the upper and lower PPO models were trained separately, and the convergence characteristics of the upper and lower PPO were obtained, as shown in Figure 5.
From Figure 5, it can be seen that in the initial stage of training, the decision reward values of the upper and lower agents are relatively small due to their unfamiliarity with the environment. With the continuous interaction between agents and the environment, upper and lower agents continuously accumulate experience to update network weights, and the reward values obtained gradually increase until convergence. The upper PPO converges after approximately 400 rounds of training, while the lower PPO converges after approximately 500 rounds of training. The upper PPO converges faster because the reward function of the upper PPO includes the cumulative adjustment of the operating power of GT and GB devices in the lower PPO. The reward functions of the upper PPO and lower PPO both converge quickly, effectively adjusting the energy purchase, conversion, and storage behavior of the power, heat, and gas systems.
Then, PIES enters normal operation mode, and the upper PPO updates the energy management status of the power and heat systems at a long-term scale of 30 min, while the lower PPO updates the energy management status of the gas system at a short-term scale of 6 min, as shown in Figure 6: The upper and lower parts display the total energy supply and the total energy demand power, respectively.
During the valley electricity price period of the power grid, the lower electricity price drives the increase in external power purchase, causing the GT equipment to operate at lower power, while the operating power of EB and P2G equipment rebounds and energy storage increases. The power system balance is mainly maintained by external power purchase on the grid side, as shown in Figure 6a. On the heating network side, due to the GT equipment adopting the "electricity and heating" mode, the operating power is relatively low, and the supply and demand balance of the heat system is mainly maintained by the GB and EB equipment, as shown in Figure 6b. On the gas network side, the balance of the gas system is mainly maintained by external gas purchasing power and P2G equipment, as shown in Figure 6c.
From Figure 6a, it can be seen that the grid side maintains a balance between supply and demand of the power system by flexibly adjusting external purchasing power, batteries, GT, P2G, and EB equipment during the normal and peak periods of electricity prices. Due to the increase in electricity prices, external power purchases have decreased, resulting in a corresponding decrease in the operating power of P2G and EB equipment, a decrease in energy storage, and a corresponding increase in the operating power of GT equipment to maintain a balance between supply and demand in the power system. At this point, the power system mainly applies photovoltaic power generation and GT equipment to make up for the supply gap of external purchased power. The photovoltaic output becomes higher during the 10:00–13:00 period, and the output of GT equipment is higher during the 17:00–20:00 period. The decrease in operating power of the P2G and EB equipment reduces the energy supply of the gas system and heat system. The gas network side compensates for the supply gap of P2G equipment by increasing external gas purchasing power, maintaining the balance of the gas system, as shown in Figure 6b. The heating network side mainly uses the GB and GT equipment to fill the supply gap of the EB equipment and maintain the balance of the heat system, as shown in Figure 6c.
Comparative experiments were conducted on the proposed PPO reinforcement learning optimization algorithm for PIES considering multiple time scales, PPO algorithm, and traditional methods. The experimental data of the three methods were randomly selected from the test set, with a total scheduling period of 24 h and a time scale of 30 min. The traditional method uses the solving software CPLEX (a mathematical modeling tool that can help solve the optimal or feasible solution in the model). The operating costs of the three methods are shown in Table 4.
Operating cost/yuan | Proposed method | PPO | Traditional method | Effect 1 | Effect 2 |
Maximum value | 28735 | 33594 | 35386 | 5.06% | 14.46% |
Minimum value | 22378 | 30391 | 32462 | 6.37% | 26.3% |
Average value | 25576 | 32358 | 33490 | 3.38% | 20.9% |
Carbon emission | 1169 | 1552 | 1625 | 4.50% | 24.6% |
Training time | 5973 s | 13685 s | ̶ | ̶ | 56.35% |
From Table 4, it can be seen that using the algorithm proposed in this article for energy management has the lowest daily average operating cost and carbon emission cost. The former is 73.5% for the PPO algorithm and 69.1% for the traditional method; the carbon emission cost is 75.3% for the PPO algorithm method and 71.9% for the traditional method.
The traditional method relies on an accurate prediction of renewable energy and loads. To solve this problem, this paper adopts the PPO algorithm in reinforcement learning. Reinforcement learning is a model-free method that does not rely on accurate prediction and modeling of source loads and can effectively deal with uncertain energy supply problems such as photovoltaics. Meanwhile, compared to traditional methods, this article divides PIES into upper and lower parts, which can meet the differences in time scales of various energy subsystems. The PPO algorithm reduces operating costs by 3.38% and carbon emissions by 4.50%, compared with traditional methods.
Using a dual-layer PPO management model to partition and manage the same number of control variables can effectively improve the training success rate and convergence speed of the PPO management model, thereby reducing its effective training time. This is because the double-layer PPO overcomes the curse of dimensionality in model training by partitioning control variables. In addition, the single-layer PPO is limited by the system management time scale of 30 min, making it difficult to quickly adjust the three energy sources' supply and demand situations, resulting in the overall economic benefits of PIES being lower than the double-layer PPO algorithm proposed in this article. The simulation results show that the double-layer PPO algorithm reduces operating costs by 20.9% and carbon emissions by 2.5% compared with the single-layer PPO algorithm.
To verify the adaptive ability of the proposed solution to energy loss, the thermal load in the PIES system was incrementally increased, and the dynamic scheduling solution analysis of PIES was conducted again to see if it meets the energy demand of the thermal load in PIES.
(1) The power variation of a gas turbine considering thermal energy loss is shown in Figure 7.
(2) The power variation of a gas boiler considering thermal energy loss is shown in Figure 8.
(3) The power variation of an electric boiler considering thermal energy loss is shown in Figure 9.
As shown in Figures 7–9, during the valley period of the electricity price, the thermal power output of the electric boiler changes more significantly. During the normal and peak periods of the electricity price, the thermal power output of the gas turbine and gas boiler changes more significantly. This indicates that the gas turbine, gas boiler, and electric boiler proposed in this paper can all adapt to dynamic scheduling decisions and maintain the supply-demand balance of thermal energy in PIES.
This article proposes an integrated-energy PPO reinforcement learning optimization algorithm for a park-integrated energy management system that considers multiple time scales to address the uncertainty of photovoltaic output and load changes, as well as the differences in time scales of heterogeneous energy subsystem management. The method divides PIES into two layers, upper and lower, with the upper layer containing power and heat systems (including photovoltaic power generation), and the lower layer containing gas systems. The main conclusions are as follows:
This article uses the PPO algorithm in deep reinforcement learning to establish a PIES energy management model, which can make real-time decisions and effectively respond to the uncertainty of photovoltaic output and load changes.
The different time scales of the upper and lower layers in PIES not only meet the needs of heterogeneous energy subsystems for energy management time scale differences but also timely adjust the output of equipment in each subsystem to meet the energy supply and demand balance in PIES.
Compared with single-layer PPO and traditional energy management methods, the method proposed in this article has advantages in reducing carbon emissions and improving the economic benefits of PIES. The PPO algorithm reduces operating costs by 3.38% and carbon emissions by 4.50% compared with traditional methods. The simulation results show that the double-layer PPO algorithm reduces operating costs by 20.9% and carbon emissions by 2.5% compared with the single-layer PPO algorithm.
The authors declare that they have not used Artificial Intelligence (AI) tools in the creation of this article.
The work is supported by Inner Mongolia Electric Power (Group) Co., Ltd Technology Project [Grant No. LX2023-5-11].
The authors declare no conflict of interest.
Conceptualization, Linrong Wang; methodology, Haixiao Zhang and Xiang Feng; validation, Ruifen Zhang and Guilan Wang; formal analysis, Haixiao Zhang, Guilan Wang and Zhengran Hou; writing—original draft preparation, Haixiao Zhang; writing—review and editing, Guilan Wang; supervision, Linrong Wang and Guilan Wang. All authors have read and agreed to the published version of the manuscript.
[1] |
Feng J, Nan J, Wang C, et al. (2022) Source-load coordinated low-carbon economic dispatch of electric-gas integrated energy system based on carbon emission flow theory. Energies 15: 3641–3652. https://doi.org/10.3390/en15103641 doi: 10.3390/en15103641
![]() |
[2] |
Bhowmik C, Bhowmik S, Ray A, et al. (2017) Optimal green energy planning for sustainable development: A review. Renewable Sustainable Energy Rev 71: 796–813. https://doi.org/10.1016/j.rser.2016.12.105 doi: 10.1016/j.rser.2016.12.105
![]() |
[3] |
Li P, Wu D, Li Y, et al. (2020) A multi-objective union optimal configuration strategy for multi-microgrid integrated energy system considering bargaining game. Power Syst Tech 44: 3680–3690. https://doi.org/10.1016/p.st.20203680 doi: 10.1016/p.st.20203680
![]() |
[4] |
Lv J, Zhang S, Cheng H, et al. (2021) Review on district-level integrated energy system planning considering interconnection and interaction. Pro CSEE 41: 4001–4021. https://doi.org/10.3390/en20214001 doi: 10.3390/en20214001
![]() |
[5] |
Yu X, Xu X, Chen S, et al. (2016) A brief review to integrated energy system and energy internet. Trans China Electro Society 31: 1–13. https://doi.org/10.1016/eprint/104480 doi: 10.1016/eprint/104480
![]() |
[6] |
Ding T, Jia W, Shahidehpour M, et al. (2022) Review of optimization methods for energy hub planning, operation, trading, and control. IEEE Trans Sustainable Energy 13: 1802–1818. https://doi.org/10.1109/TSTE.2022.3172004 doi: 10.1109/TSTE.2022.3172004
![]() |
[7] |
Khodadadi A, Abedinzadeh T, Alipour H, et al. (2023) Optimal operation of energy hub systems under resiliency response options. J Electr Comput Eng 20: 23–36. https://doi.org/10.1155/2023/2590362 doi: 10.1155/2023/2590362
![]() |
[8] |
Song D, Meng W, Dong M, et al. (2022) A critical survey of integrated energy system: Summaries, methodologies and analysis. Energy Convers Manage 266: 58–63. https://doi.org/10.1016/j.enconman.2022.115863 doi: 10.1016/j.enconman.2022.115863
![]() |
[9] |
Jiang X, Sun C, Cao L, et al. (2022) Semi-decentralized energy routing algorithm for minimum-loss transmission in community energy internet. Int J Electrical Power Energy Syst 135: 35–47. https://doi.org/10.1016/j.ijepes.2021.107547 doi: 10.1016/j.ijepes.2021.107547
![]() |
[10] |
Yang M, Cui Y, Huang D, et al. (2022) Multi-time-scale coordinated optimal scheduling of integrated energy system considering frequency out-of-limit interval. Inter J Elect Power Energy Syst 141: 68–81. https://doi.org/10.1016/j.ijepes.2022.108268 doi: 10.1016/j.ijepes.2022.108268
![]() |
[11] |
Hu K, Wang B, Cao S, et al. (2022) A novel model predictive control strategy for multi-time scale optimal scheduling of integrated energy system. Energy Rep 8: 7420–7433. https://doi.org/10.1016/j.egyr.2022.05.184 doi: 10.1016/j.egyr.2022.05.184
![]() |
[12] |
Li X, Wang W, Wang H (2021) Hybrid time-scale energy optimal scheduling strategy for integrated energy system with bilateral interaction with supply and demand. Appl Energy 285: 458–463. https://doi.org/10.1016/j.apenergy.2021.116458 doi: 10.1016/j.apenergy.2021.116458
![]() |
[13] |
Li P, Guo T, Abeysekera M, et al. (2021) Intraday multi-objective hierarchical coordinated operation of a multi-energy system. Energy 228: 5–28. https://doi.org/10.1016/j.energy.2021.120528 doi: 10.1016/j.energy.2021.120528
![]() |
[14] |
Cheng S, Wang R, Xu J, et al. (2021) Multi-time scale coordinated optimization of an energy hub in the integrated energy system with multi-type energy storage systems. Sustainable Energy Technol Assess 47: 327–335. https://doi.org/10.1016/j.seta.2021.101327 doi: 10.1016/j.seta.2021.101327
![]() |
[15] |
Zhang B, Hu W, Li J, et al. (2020) Dynamic energy conversion and management strategy for an integrated electricity and natural gas system with renewable energy: Deep reinforcement learning approach. Energy Convers Manage 220: 63–75. https://doi.org/10.1016/j.enconman.2020.113063 doi: 10.1016/j.enconman.2020.113063
![]() |
[16] |
Xu Z, Han G, Liu L, et al. (2021) Multi-energy scheduling of an industrial integrated energy system by reinforcement learning-based differential evolution. IEEE Trans Green Commun Netw 5: 1077–1090. https://doi.org/10.1109/TGCN.2021.3061789 doi: 10.1109/TGCN.2021.3061789
![]() |
[17] |
Foruzan E, Soh LK, Asgarpoor S (2018) Reinforcement learning approach for optimal distributed energy management in a microgrid. IEEE Trans Power Syst 33: 5749–5758. https://doi.org/10.1109/TPWRS.2018.2823641 doi: 10.1109/TPWRS.2018.2823641
![]() |
[18] |
Gorostiza FS, Gonzalez-Longatt FM (2020) Deep reinforcement learning-based controller for SOC management of multi-electrical energy storage system. IEEE Trans Smart Grid 11: 5039–5050. https://doi.org/10.1109/TSG.2020.2996274 doi: 10.1109/TSG.2020.2996274
![]() |
[19] |
Zhang X, Liu Y, Duan J, et al. (2021) DDPG-based multi-agent framework for SVC tuning in urban power grid with renewable energy resources. IEEE Trans Power Syst 36: 5465–5475. https://doi.org/10.1109/TPWRS.2021.3081159 doi: 10.1109/TPWRS.2021.3081159
![]() |
[20] |
Zhu X, Yang J, Liu Y, et al. (2019) Optimal scheduling method for a regional integrated energy system considering joint virtual energy storage. IEEE Access 7: 138260–138272. https://doi.org/10.1109/ACCESS.2020.3046743 doi: 10.1109/ACCESS.2020.3046743
![]() |
[21] |
Li Y, Zhang F, Li Y, et al. (2021) An improved two-stage robust optimization model for CCHP-P2G microgrid system considering multi-energy operation under wind power outputs uncertainties. Energy 223: 48–60. https://doi.org/10.1016/j.energy.2021.120048 doi: 10.1016/j.energy.2021.120048
![]() |
[22] |
Fotopoulou M, Pediaditis P, Skopetou N, et al. (2024) A Review of the Energy Storage Systems of Non-Interconnected European Islands. Sustainability 16: 1572. https://doi.org/10.3390/su16041572 doi: 10.3390/su16041572
![]() |
[23] |
Rious V, Perez Y (2014) Review of supporting scheme for island power system storage. Renewable Sustainable Energy Rev 29: 754–765. https://doi.org/10.1016/j.rser.2013.08.015 doi: 10.1016/j.rser.2013.08.015
![]() |
[24] |
Guo M, Mu Y, Jia H, et al. (2021) Electric/thermal hybrid energy storage planning for park-level integrated energy systems with second-life battery utilization. Adva Appl Energy 4: 64–75. https://doi.org/10.1016/j.adapen.2021.100064 doi: 10.1016/j.adapen.2021.100064
![]() |
[25] |
Li Z, Zhang F, Liang J, et al. (2015) Optimization on microgrid with combined heat and power system. Proc CSEE 35: 3569–3576. https://doi.org/10.13334/j.0258-8013.pcsee.2015.14.011 doi: 10.13334/j.0258-8013.pcsee.2015.14.011
![]() |
[26] |
Zhou S, Hu Z, Gu W, et al. (2020) Combined heat and power system intelligent economic dispatch: A deep reinforcement learning approach. Inter J Electrical Power Energy Syst 120: 106016. https://doi.org/10.1016/j.ijepes.2020.106016 doi: 10.1016/j.ijepes.2020.106016
![]() |
[27] |
Yang HZ, Li ML, Jiang ZY, et al. (2020) Multi-time scale optimal scheduling of regional integrated energy systems considering integrated demand response. IEEE Access 8: 5080–5090. https://doi.org/10.1109/ACCESS.2019.2963463 doi: 10.1109/ACCESS.2019.2963463
![]() |
[28] |
Yang T, Zhao L, Liu Y, et al. (2021) Dynamic economic scheduling of integrated energy systems based on deep reinforcement learning. Power Syst Autom 45: 39–47. https://doi.org/10.7500/AEPS20200405004 doi: 10.7500/AEPS20200405004
![]() |
[29] |
Dong J, Wang HX, Zhou XR, et al. (2023) Low carbon economic dispatch of electricity gas heat integrated energy system considering comprehensive demand response. J North China Electr Power Univ, Nat Sci Ed 50: 81–90. https://doi.org/10.3969/j.ISSN.1007-2691.2023.03.08 doi: 10.3969/j.ISSN.1007-2691.2023.03.08
![]() |
1. | Menglu Lu, Tianqi Yang, Wenkui Zhang, Yang Xia, Xinping He, Xinhui Xia, Yongping Gan, Hui Huang, Jun Zhang, Hydrogen-bond enhanced urea-glycerol eutectic electrolyte to boost low-cost and long-lifespan aqueous sodium-ion batteries, 2025, 20954956, 10.1016/j.jechem.2025.01.004 |
Equipment | Lower power limit/MW | Upper power limit/MW |
Gas turbine | 0 | 1 |
Gas boiler | 0 | 0.55 |
Electric boiler | 0 | 0.4 |
P2G | 0 | 0.5 |
Battery | 0.06 | 0.24 |
Heat storage tank | 0.04 | 0.2 |
Gas storage tank | 0.07 | 0.35 |
Parameter | Numeric value | Parameter | Numeric value | Parameter | Numeric value |
ΔGGTmax | 0.1 MW | ΔGGBmax | 0.05 MW | ΔPEBmax | 0.1 MW |
ΔPP2Gmax | 0.04 MW | ηGT−E | 43% | ηGB | 97% |
ηEB | 93% | ηP2G | 85% | ηGT−loss | 15% |
μGB−loss | 5% | μEB−loss | 3% | ηP2G−loss | 20% |
PES,ch/dismax | 0.08 MW | PGS,ch/dismax | 0.08 MW | PHS,ch/dismax | 0.03 MW |
ηES, ch | 90% | ηHS, ch | 80% | ηGS, ch | 90% |
ηES, dis | 110% | ηHS, dis | 115% | ηGS, dis | 110% |
PElemax | 2 MW | GGasmax | 1.5 MW | cEleRC | 1 yuan |
cP2GRC | 1 yuan | cEBRC | 1 yuan | cGasRC | 1.5 yuan |
cGTRC | 1.5 yuan | cGBRC | 15000 yuan | cHS | 6 yuan |
cGS | 6 | cEScape | 45 | m | 694 |
n | 1.98 | q | 0.016 | DESR | 0.8 |
σA | 0.001 | σC | 0.002 | γ | 0.9 |
Time span | Time | Electrovalence/(yuan/kwh) |
Valley period | 23:00–morrow 07:00 | 0.2 |
Peacetime period | morrow 07:00–12:00 | 0.6 |
19:00–23:00 | ||
Peak period | 12:00–19:00 | 1.1 |
Operating cost/yuan | Proposed method | PPO | Traditional method | Effect 1 | Effect 2 |
Maximum value | 28735 | 33594 | 35386 | 5.06% | 14.46% |
Minimum value | 22378 | 30391 | 32462 | 6.37% | 26.3% |
Average value | 25576 | 32358 | 33490 | 3.38% | 20.9% |
Carbon emission | 1169 | 1552 | 1625 | 4.50% | 24.6% |
Training time | 5973 s | 13685 s | ̶ | ̶ | 56.35% |
Equipment | Lower power limit/MW | Upper power limit/MW |
Gas turbine | 0 | 1 |
Gas boiler | 0 | 0.55 |
Electric boiler | 0 | 0.4 |
P2G | 0 | 0.5 |
Battery | 0.06 | 0.24 |
Heat storage tank | 0.04 | 0.2 |
Gas storage tank | 0.07 | 0.35 |
Parameter | Numeric value | Parameter | Numeric value | Parameter | Numeric value |
ΔGGTmax | 0.1 MW | ΔGGBmax | 0.05 MW | ΔPEBmax | 0.1 MW |
ΔPP2Gmax | 0.04 MW | ηGT−E | 43% | ηGB | 97% |
ηEB | 93% | ηP2G | 85% | ηGT−loss | 15% |
μGB−loss | 5% | μEB−loss | 3% | ηP2G−loss | 20% |
PES,ch/dismax | 0.08 MW | PGS,ch/dismax | 0.08 MW | PHS,ch/dismax | 0.03 MW |
ηES, ch | 90% | ηHS, ch | 80% | ηGS, ch | 90% |
ηES, dis | 110% | ηHS, dis | 115% | ηGS, dis | 110% |
PElemax | 2 MW | GGasmax | 1.5 MW | cEleRC | 1 yuan |
cP2GRC | 1 yuan | cEBRC | 1 yuan | cGasRC | 1.5 yuan |
cGTRC | 1.5 yuan | cGBRC | 15000 yuan | cHS | 6 yuan |
cGS | 6 | cEScape | 45 | m | 694 |
n | 1.98 | q | 0.016 | DESR | 0.8 |
σA | 0.001 | σC | 0.002 | γ | 0.9 |
Time span | Time | Electrovalence/(yuan/kwh) |
Valley period | 23:00–morrow 07:00 | 0.2 |
Peacetime period | morrow 07:00–12:00 | 0.6 |
19:00–23:00 | ||
Peak period | 12:00–19:00 | 1.1 |
Operating cost/yuan | Proposed method | PPO | Traditional method | Effect 1 | Effect 2 |
Maximum value | 28735 | 33594 | 35386 | 5.06% | 14.46% |
Minimum value | 22378 | 30391 | 32462 | 6.37% | 26.3% |
Average value | 25576 | 32358 | 33490 | 3.38% | 20.9% |
Carbon emission | 1169 | 1552 | 1625 | 4.50% | 24.6% |
Training time | 5973 s | 13685 s | ̶ | ̶ | 56.35% |