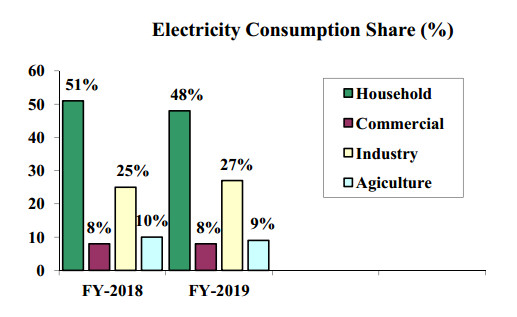
Citation: Kashif Raza Abbasi, Khadim Hussain, Jaffar Abbas, Festus Fatai Adedoyin, Pervez Ahmed Shaikh, Hazrat Yousaf, Faqeer Muhammad. Analyzing the role of industrial sector's electricity consumption, prices, and GDP: A modified empirical evidence from Pakistan[J]. AIMS Energy, 2021, 9(1): 29-49. doi: 10.3934/energy.2021003
[1] | Mburamatare Daniel, William K. Gboney, Hakizimana Jean de Dieu, Akumuntu Joseph, Fidele Mutemberezi . Empirical assessment of drivers of electricity prices in East Africa: Panel data experience of Rwanda, Uganda, Tanzania, Burundi, and Kenya. AIMS Energy, 2023, 11(1): 1-30. doi: 10.3934/energy.2023001 |
[2] | Sameer Thakare, Neeraj Dhanraj Bokde, Andrés E. Feijóo-Lorenzo . Forecasting different dimensions of liquidity in the intraday electricity markets: A review. AIMS Energy, 2023, 11(5): 918-959. doi: 10.3934/energy.2023044 |
[3] | Norman Maswanganyi, Edmore Ranganai, Caston Sigauke . Long-term peak electricity demand forecasting in South Africa: A quantile regression averaging approach. AIMS Energy, 2019, 7(6): 857-882. doi: 10.3934/energy.2019.6.857 |
[4] | Yan Li, Yaheng Su, Qixin Zhao, Bala Wuda, Kaibo Qu, Lei Tang . An electricity price optimization model considering time-of-use and active distribution network efficiency improvements. AIMS Energy, 2025, 13(1): 13-34. doi: 10.3934/energy.2025002 |
[5] | Hamza El Hafdaoui, Ahmed Khallaayoun, Kamar Ouazzani . Activity and efficiency of the building sector in Morocco: A review of status and measures in Ifrane. AIMS Energy, 2023, 11(3): 454-485. doi: 10.3934/energy.2023024 |
[6] | Mousa Tawfeeq, Alan R. Collins, Adam Nowak . The dynamic response of coal consumption to energy prices and GDP: An ARDL approach to the U.S.. AIMS Energy, 2020, 8(6): 1156-1172. doi: 10.3934/energy.2020.6.1156 |
[7] | Gunnar Lima, Andreas Nascimento, Marcelo P. Oliveira, Fagner L. G. Dias . Energy efficiency analysis: A household digital transformation. AIMS Energy, 2024, 12(4): 774-808. doi: 10.3934/energy.2024037 |
[8] | Emrah Gulay . Forecasting the total electricity production in South Africa: Comparative analysis to improve the predictive modelling accuracy. AIMS Energy, 2019, 7(1): 88-110. doi: 10.3934/energy.2019.1.88 |
[9] | Mojgan Pouralizadeh . A DEA model to sustainability improvement of the electricity supply chain in presence dual-role factors and undesirable outputs: A case on the power industry. AIMS Energy, 2020, 8(4): 580-614. doi: 10.3934/energy.2020.4.580 |
[10] | Fiona Bénard-Sora, Jean-Philippe Praene, Yatina Calixte . Assess the local electricity consumption: the case of Reunion island through a GIS based method. AIMS Energy, 2018, 6(3): 436-452. doi: 10.3934/energy.2018.3.436 |
Energy is an integral part of every country's economic development. Electricity is an incredibly flexible energy source that fuels the efficiency of almost any sector of the economy. Pakistan's disastrous energy failure policies have left the nation with a severe power catastrophe, suffering economic damages throughout the last twenty years. Hence, understanding the energy consumption and real GDP is very important to improve its financial growth and development process from a policy perspective.
Over three decades after [1], seminal work reaffirming the causal link between energy consumption and real production, the causal path between these factors remains uncertain [2,3]. Several earlier empirical studies discovered that electricity consumption Granger-causes real GDP, such as [4,5,6], although other studies claimed that electricity consumption does not Granger-causes real GDP [7,8]. On the other hand, some studies (e.g., [9,10,11,12] specify the number of explanations why recent research on the link between electricity and economic growth has contradictory findings. According to these researches, the existing electricity demand model focused only on observed variables, economic development, and energy price, and neglects the non-observable elements. Electricity consumption often depends on non-observable variables such as energy conservation, technical advances, and customer preference. Because electricity demand is considered a derived output, energy consumption relies on electrical appliances usage and capital stock. Appropriately, such findings indicate other exogenous factors aside from economic variables such as income and price (e.g., energy consumption factors, technological change, and customer preference) should be incorporated for modeling electricity demand. argued that capturing the impact of un-observable factors through a simple timing pattern is impractical [13]. Dimitropoulos et al. (2004), Dilaver and Hunt (2011), Hunt and Ninomiya (2003) and Hunt (2003) stated that the method Structural Time Series Modeling (STSM) was established by [15,16] is an adequate approach to capture the results of non-observable variables (trends in energy usage, technical changes, and customer fondness) effectively [9,12,13,14]. Furthermore, Harvey et al. (2004) claimed that time series is broken down into non-observed patterns and other abnormal elements in the STSM technique and that these are not identified [17]. The stochastic process, also regarded as the Underlying Energy Demand Trend (UEDT), incorporates not just exogenous technological advancement but other significant social and economic implications as for customers’ expectations, resource-saving scientific changes.
The uninterrupted provision of electricity is the critical factor to flourish in the industrial sector. It plays an indispensable role, as this sector is the engine of economic growth in Pakistan. However, Pakistan has faced a severe electricity crisis, directly and indirectly, involving all economic sectors, particularly concerning the changing energy structure. In the past, energy shortage and inefficiencies’ posed damages to the economy. The government launched various plans during 2013–2018 to fix the energy crisis for ensuring the smooth distribution of energy supply. These schemes added a total power of 12,230 MW to the national energy system [18]. Electricity consumption patterns evidenced that there was no substantial change in electricity demand. The household’s electricity consumption pattern indicated a slight decrease from 51% in 2018 to 48% in 2019. The industrial sector also revealed an increasing electricity consumption trend of 25% in 2018 and 27% in 2019; however, commercial consumption remained unchanged from 2018 to 2019. The agriculture sector consumption also declined by 1% from 10% in 2018 to 9% in 2019. The surge in industrial sector electricity consumption shows a positive sign that market was recovering from the earlier economic damages but still needs improvement in other sectors. Figure 1 shows a comparison of electricity consumption patterns from July 2018 to March 2019 fiscal year.
Several past studies evaluated the indispensable role of electricity consumption in Pakistan and applied linear and nonlinear conceptual models to report agammaegate demand for electricity consumption and quantify it [3,6,19,20,21]. The past literature findings specified that real wages, real substitute and actual electricity rates, temperature, and stochastic patterns appear to be critical determinants of Pakistan's agammaegate and sectoral electricity demand. The factors mentioned above evidenced that Pakistan has to develop an appropriate and well-designed economic model linked to electricity rate, consumption, and economic growth indicators. Figure 2 indicates the rising trends in electricity consumption and GDP while prices fluctuate from 1998 to 2018 due to government subsidy for the industrial boost in Pakistan.
The study's inspiration and contribution consist of a review of Pakistan's energy demand and supply and its effects on Pakistan's economy. Comparison to prior research, the present study provides fascinating and real contributions. First, we find limited studies that address all the variables related to the industrial sector in Pakistan. Second, the current research explores the relationship between electricity consumption, price, and real GDP of Pakistan's industrial sector using data from the 1960–2018 time series. Third, the study employs the unit root test, Johansen co-integration, Vector Error Correction Model (VECM), impulse response function, and variance decomposition tests, and offers more fruitful inferences. By comparison, within a VECM system, we research the variables' relationships. It helps us determine whether there is a long-term (or co-integrating) link between the variables and investigate their existence. Fourth, the study results would help formulate energy generation and consumption policies while also articulating an ambitious power rationing strategy to minimize economic loss due to energy shortages. Finally, similar studies are based on flawed data, but specific data from Pakistan's industrial sector is considered in this study. This novelty has created a gap between earlier research.
The remainder of the study organized as follows: the next section 2 presents the literature review, and section 3 parades econometric material and methods. Section 4 shows the results and discussion, while section 5 provides conclusions and policy implications.
Several studies have been done over the last few years, examining the relationship between an economy's electricity demand and economic progress. As of now, the relationship between energy use and financial growth is recorded significantly by [4,22,23,24]. Thus, our analysis estimates electricity consumption (EC) prices and gross domestic product (GDP) from the literature context. Followed Chen and Fang (2018) investigated the co-integrating and Granger causal relationships between economic development, industrial energy use, and human resources in China using a panel of 210 prefectural cities for the 2003–2012 period [25], also Cialani and Mortazavi (2018) examined the energy demand and its determinants after the liberalization of the power markets in 29 European countries [26]. Based on panel data from 1995–2015 for these countries and using a dynamic partial adjustment model, market elasticity is calculated for both residential and industrial demand for electricity. They discovered short-run and long-run causation of EC to GDP and inferred that a lack of energy could harm economic growth (EG). The causality evidence found from energy use to EG confirms the result of [28,29,30,31], whereas the causality findings from economic progress to electricity use are aligned with [27,28,29,30,31]. Moreover, another critical study by [32] enquired about the effect of power consumption, using yearly data for the period 1971–2012, on GDP, labor, and capital stocks. The Structural Vector Self-Regression (SVAR) method analysis shows that labor demand rises with economic growth. Conversely, Nazlioglu et al. (2014) inspected the causal association between Turkey's energy and financial development between 1967 and 2007 [33]. They use three investigative econometrics methods to achieve this objective: the bounds testing method to co-integration, the linear and the nonlinear Granger causality test. The analysis of co-integration indicates that in the long run, co-integrated relationship. Further, Al-bajjali and Yacoub (2018) inspected determinants of electricity consumption from 1986–2015, focused on six independent variables by employed VECM for analysis; the results confirmed that GDP, urbanization, and agammaegate water consumption, are essential and positively related electricity consumption [34].
Similarly, Ghafoor et al. (2016) studied that energy shortages have caused economic losses of between 2% and 3% of Pakistan's GDP [35]. Further, Sidique (2014) used the Granger causality test of Hsiao and reported that the effect of EC on EG is strong and highly meaningful [36]. However, Shahbaz and Hooi (2012) utilized the Autoregressive Distributed Lag (ARDL) model and found the bidirectional causality between EC and EG [37]. Additionally, Javid and Qayyum (2014) utilized the structural time series and found a growing EC pattern in the rural, industrial, and housing sectors [38]. Jamil and Ahmad (2010) presented Johansen cointegration and VECM Granger causality tests and noticed one-way causation flowing from EG to EC [39]; Khan and Abbas (2016) implemented a panel cointegration study, and calculated electricity demand in agricultural and industrial sectors are more resilient to reform [40]. The Autoregressive Integrated Moving Average (ARIMA) models were applied by [41] and observed that EC has a clear interaction with EG. They stated that the ARIMA model was the best in the forecast and indicated that schemes' productivity would be improved by increasing energy usage.
Lin and Yousaf (2021) measured the electricity consumption in Pakistan between 1989 and 2018 using the index decomposition. The findings indicate that the economic structure's impact was the key driving force in Pakistan's increasing overall electricity usage [42]. Recently Lin and Yousaf (2020) implemented the MARKAL model and measured the supply, demand, and diversity of primary energy supplies [43]. The findings suggested that the availability of primary energy decline slightly. These analyses investigated the correlation between EG and EC, the short-term causal relationship, long-run, and regression-related tests among the parameters. The result showed that the scarcity of electricity could damage the economy of Pakistan. The outcomes are varied, according to early findings. In particular, all research on Pakistan's electricity use and economic growth relationships indicates no consent on the direction of causality among these variables. The EC, therefore, plays a vital role in Pakistan's cultural identity of economic and financial growth. Structural transition and with up-gradation might be a little more specific in this case. Therefore, the characteristics and distinctions in energy use within the multiple segments need to be addressed. Lin and Yousaf (2020) indicated that it is possible to achieve optimum production by rising capital and energy technologies [44]. The different studies' findings are inconsistent, or even substantially contradictory, partially due to variations in methods or data length. They reviewed previous studies and their findings from the causal relationship between energy consumption and economic growth reported in Table 1. Whereas we find various studies evaluating the causal association between energy and economic development in Pakistan, no research explicitly examined the relationship between energy consumption, price, and GDP growth in the industrial sector. However, these variables are used in different aspects, methodology, and duration. This research extends the relevant literature on electricity by defining the industrial sector causality path between electricity consumption, prices, and real GDP.
Author | Country (Period) | Variables | Methodology | Direction of Causality | |||
→ | ← | ↔ | ≠ | ||||
[45] | China (1971–2001) | EC, GDP | Granger Causality |
✔ | ✘ | ✘ | ✘ |
[37] | Pakistan (1972–2009) | EC, RGDP | ARDL, VECM | ✘ | ✔ | ✘ | ✘ |
[46] | Switzerland (1950–2010) | EC, RGDP | ARDL, UECM | ✘ | ✘ | ✔ | ✘ |
[46] | United Kingdom(1975–2010) | EC, RGDP | Panel bootstrap causality | ✔ | ✘ | ✔ | ✘ |
[46] | OECD(1990–2008) | EC, GDP | Panel Granger causality, | ✔ | ✘ | ✔ | ✘ |
[46] | Lebanon (1983–2014) | EC, GDP | Toda-Yamamoto | ✘ | ✘ | ✔ | ✘ |
[46] | Algeria (1971–2010) | EC, GDP | Cointegration, VECM | ✘ | ✘ | ✔ | ✘ |
[3] | Pakistan (1972–2010) | EC, RGDP | Granger Causality | ✔ | ✘ | ✘ | ✘ |
[47] | Poland (2000–2012) | EC, GDP | Granger causality | ✘ | ✘ | ✔ | ✘ |
[48] | Turkey (1970–2011) | EC, GDP | Granger causality | ✘ | ✘ | ✔ | ✘ |
[19] | Malaysia (2005–2010) | EC, RGDP | Multiplier Approach | ✔ | ✘ | ✘ | ✘ |
[24] | Algeria(1980–2012) | RE, NRE | Granger causality | ✔ | ✘ | ✔ | ✘ |
[49] | Taiwan (1998–2014) | EC, RGDP | Granger causality | ✘ | ✘ | ✔ | ✘ |
[50] | U.S.(2005–2015) | EC, RGDP | Wavelet | ✔ | ✘ | ✘ | ✘ |
[51] | Ghana (1971–2014) | EC, Industrial Growth | ARDL, ECM | ✘ | ✔ | ✘ | ✘ |
[52] | Pakistan(1981–2017) | RE, NRE | ARDL, VECM | ✘ | ✘ | ✔ | ✘ |
[53] | Pakistan (1970–2018) | EC, EP, GDP | Granger causality VECM | ✔ | ✘ | ✘ | ✘ |
Note: →, ←, ↔, and ≠ denotes unidirectional bidirectional and no causality, respectively. |
Therefore, this study aims to capture a comprehensive view, based on the most up-to-date database, of the differentiation indices of electricity consumption (EC), price, and GDP of the industrial sector in Pakistan from 1970 to 2018. Nevertheless, no consideration was given to studying the modeling techniques of electricity consumption of Pakistan in general. We may examine the efficacy of the past policies by observing the EC, price, and GDP link. In formulating future policies, a greater understanding of how the EC is evolving in Pakistan is also crucial. This analysis allows us to use the VECM in combination with the variance decomposition method to determine the influence of selected variables that affect EC in Pakistan over the time defined.
The research investigates the relationship between electricity consumption, electricity prices, and the GDP of Pakistan's industrial sector. The study used time series secondary data based on yearly observation, covering five decades approximately from 1970–2018, exhibits in Table 2. The electricity will measure in Gigawatt hour (GWh). Because electricity has been a public enterprise in Pakistan, instead of being regulated by the market, the electricity price is cross-subsidizing in all sectors. We use the average price of electricity, while real GDP uses a proxy of industrial value-added taken by the world bank's most authentic source, National Transmission & Despatch Company (NTDC) and Government of Pakistan (GOP) official websites. Figure 3 depicts the diagram of the variables.
Variables | Data Source | Scale Unit |
Industrial electricity consumption | [54] | Gigawatt hours (GWh) |
Electricity prices | [55] | Millions |
Real gross domestic product | [56] | $Millions |
The cointegration test allows the series under examination to be non-stationary in the same integration order to evaluate the long-term relationship between variables. The model forecasts that price is a significant factor in demanding electricity. Our empirical research implements the extensively employed method to maximum likelihood by Johansen for the analysis. The first step is to check all the variables for their stationarity., and two following tests are widely prominent [57,58], to detect the integration in all series, which stated in Eq 1:
Δyt=β0+δYt−1+γ1Δyt−1+γ2yt−2+.........γpΔyt−p+μt | (1) |
where, yt denotes a series and ut expresses error terms. Appropriate lags of Δyt are incorporated the whiten the errors. According to the Schwarz Bayesian criterion (SBC), the lag length is chosen, later checking for the serial correlation of first and higher-order in residuals. For the H0 null hypothesis test in Eq 1 is ‘d,’ which = 0, against the one-tailed, and alternative, which is negative. The stationarity of yt would not be rejected If δ result results significantly negative. Modeling associations among non-stationary features essentially prerequisite their differencing to make stationarity. For several years, most of the long-run economic relationship lost due to differences; therefore, extensive data needed at level variable for the conservation of the long-run. Meanwhile prevents chosen variables from being spuriously regressed. A long-run equilibrium relationship among non-stationary time series data exists as indicated by economic theories. If variables are I(1), so the co-integration approach will be applied in the long-run. Augmented Dickey-Fuller (ADF) and Phillips–Perron (PP) unit root tests are the primary step to move into the co-integration model.
Following [59] and [60], a demand-side model was used to investigate the industrial sector's reaction to unanticipated electricity consumption shock electricity prices and GDP. Impulse response function assessment ensures a systematic long-run relationship to determine the underlying predictor and does not wander much farther away from one another. The Johansen-Juselius cointegration technique is favored over the Engle and Granger two-step cointegration process for estimating long-term relationships in multivariate condition. Since if we regress Y on X in the former model, the outcomes would vary from regressing X on Y. In comparison, the Engle-Granger technique is a two-step procedure and the second step would therefore be influenced by an error happening in the first step. The technique proposed by [61] for maximum likelihood is reflected in the following VAR model:
Xt=A1Xt−1+A2Xt−2+...+ApXt−p+εt | (2) |
where Xt is an (n × 1) economic time series vector. A1, A2, ..., the (n × n) coefficient matrices are represented by Ap and εt is an (n × 1) vector with zero mean and constant variance error terms. We notice two test statistics for the estimation of cointegrating vectors, i.e., likelihood ratio or trace statistics, under the Johansen-Juselius methodology, and maximal eigenvalue statistics, as follows:
λtrace(r)=−Tn∑i=r+1ln(1−∧λr)λmax(r,r+1)=−Tln(1−∧λr+1) | (3) |
trace statistics are used to test the null hypothesis that the number of cointegrating vectors is less or equal to r, whereas max statistics evaluate the null hypothesis that cointegrating vectors are equal to r. These measures are focused on drawing parallels, and both λtrace and λmax statistics would be weak if characteristic roots are near to 0, and there will be less support for long-term series relationships.
One of the Granger interpretation theorem's ramifications is that Granger causality occurs at least in one direction if the series has a cointegrating relationship, which indicates if a conditional variable strengthens the predictor variables prediction when shown in the model [61,62]. The popular meaning of lagging independent terms is investigated under the Vector Autoregression (VAR) concept of Granger causality. The causal relationship is calculated under a VECM that differs between short-and long-term casualties while the sequence is co-integrated. Important terminology for error correction leads to long-term Granger causality, while the general sense of lagged independent variables is an example of short-term Granger causality. In addition, the corrections to the long-run equilibrium suggested in Eqs 2–4 are summarized:
ΔEC=α1+l∑i−1β1iΔECt−i+m∑i=1γ1iΔEPt−1+n∑i=1δ1iΔGDPt−1+φ1ECTr,t−1+μ1t | (4) |
ΔEP=α2+l∑i−1β2iΔECt−i+m∑i=1γ2iΔEPt−1+n∑i=1δ2iΔGDPt−1+φ2ECTr,t−1+μ2t | (5) |
ΔGDP=α3+l∑i−1β3iΔECt−i+m∑i=1γ3iΔEPt−1+n∑i=1δ3iΔGDPt−1+φ3ECTr,t−1+μ3t | (6) |
where EC,EP,andGDP represents electricity consumption, electricity price, and real GDP. Correspondingly, ‘α’ is the intercept ‘n’ is the number of lags, ‘Δ’ is the 1st difference, the joint consequence of lags β1i,γ1i,δ1i, β2i,γ2i,δ2i and β3i,γ3i,δ3i in Eqs 2–4, respectively, while ui,t For (i=1,2,3) are residuals, and the Error Correction Terms (ECT) specified by ECTr,t−1 and 'ϕ' is the adjustment of model speed towards equilibrium. For example, scale and the statistical significance of the one-period lag ECTr,t−1 coefficient determines how quick the disequilibrium in EC,EPandGDP are corrected to return to the equilibrium. All the variables are used in the natural logarithmic form. A series of short-run changes slowly rectify the divergence from the long-run equilibrium. The size and statistical significance of the ECT is a measure of the degree to which the left side variable returns to its long-run equilibrium in reaction to the random shocks of each equation for each short-run cycle, while the error correction model via the ECT provides another method for the detection of Granger causality that can be avoided in standard Granger and Sim study. The actual regression coefficients are generally hard to read in a VAR system. These are then represented by techniques connected with VAR models, i.e., impulse response functions and decomposition methods of variance. Impulse response function in earlier researches has been utilized widely for policy evolution. Energy sector reform appraisal analyses include [63,64,65,66]. Causation from EPtoEC and GDPtoEP can be tested similarly from Eqs 4–6, respectively. As explained above, whereas the existence of causality indicated by co-integration, VECM confirms the direction of causality among the variables. In addition, in [67], the error correction model makes it possible to differentiate between long-term and short-term causality in contrast to returning the missing information to the system due to the terminology of error correction. Figure 4 demonstrates the graphical representation of the study framework.
Firstly, the stationarity check of all variables to be conducted, which is necessary to prevent the spurious regression. The H0 considered as non-stationary series (has unit root). All variables should be stationery earlier containing in the model. Across all variables, the ADF and PP tests suggest the presence of unit root at a 5% significance level, indicating that any statistical inferences in levels from the series would be invalid [68]. Though the non-stationarity hypothesis was discarded in its first difference for all the series, electricity consumption, electricity prices, and real GDP were integrated with order one or I(1) as a whole shown in Table 3. All variables are integrated with order one, a necessary condition of the process variables co-integration relationship.
Industrial Variables | Philips-Perron (PP) | Augmented Dickey-Fuller (ADF) | Order of Integration | ||
Levels | First difference | Levels | First difference | ||
EC | (0.32) | (0.00)* | (0.35) | (0.00)* | I(1) |
EP | (0.49) | (0.00)* | (0.51) | (0.00)* | I(1) |
GDP | (0.31) | (0.00)* | (0.29) | (0.00)* | I(1) |
Notes: *, ** asterisk specifies significance at 1% and 5% level, respectively. p-value denotes in () parentheses. |
After the first condition has been met, we step into the next process to determine whether there is a long-run relationship among the variables. Therefore, Johansen and Juselius (1990), Johansen (1988) maximum likelihood method applied to find the co-integration, which consists of the two following estimations: trace(λ−trace)andmaximumeigenvalue(λ−max)statistics [61,62]. The H0 is rejected against the H1 at a 5% level. Hence, it is confirmed that there is a long-run relationship exists in two variables in line with [34,53]. The illustration of unrestricted co-integration outcomes shown in Table 4.
Hypothesized | r = 0 | r ≤ 1 | r ≤ 2 |
Trace Statistics | 35.40 | 12.83 | 3.29 |
P-Value | (0.01)* | (0.003)* | (0.12) |
Max-Eigen Statistics | 22.57 | 9.54 | 3.33 |
P-Value | (0.03)* | (0.002)* | (0.07) |
Notes: The numbers in the parentheses are p-values, r: indicates the number of co-integration hypothesis relationships, *, ** asterisk implies significance at 5% and 10% level, correspondingly. |
We proceed to the VECM estimate, as defined by Eqs 4–6, drawing inferences for each variable within the sample on the direction of causality and the exogenity or endogeneity. The result of the VECM is exhibited in Table 5. All variables are projected at a one-period lag. The term error-correction depends on the previous period deviation from long-run equilibrium (the error) affects the explanatory variables short-run dynamics. Hence, the coefficient of ECT, ϕ, is the speed of adjustment; it measures the speed at which explained variables return towards equilibrium after a change in the other variables [69].
Industrial Sector | VECM (Long-run relationship effects) | ECT(t-Stats) | |||||
Coefficient(p-value) | Standard Error | Coefficient(p-value) | Standard error | Coefficient (p-value) | Standard error | ||
ΔEC | 1 | - | −0.19 | −0.39 | 0.15 | −0.21 | (−2.66)* |
(0.03)* | (0.01)* | ||||||
ΔEP | −0.13 | −0.12 | 1 | - | 0.05 | −0.12 | (−3.97)** |
(0.04)* | (0.12) | ||||||
ΔGDP | 0.09 | −0.14 | −0.16 | −0.24 | 1 | - | (−3.36)* |
(0.001)* | (0.00)** | ||||||
Notes: The number in the parentheses is the p-value. *, ** asterisks indicate significance at 5% and 1% level, respectively. |
ECT's coefficient is negative in all variables and found significant at the 5% level, where a 1% increase in electricity price decreases electricity consumption by 0.13%, as well a 1% increase in GDP increase EC by 0.09%. While a 1% upsurge in electricity consumption decreases electricity price by 0.19%, if GDP increase by 1%, then electricity price decreases by 0.16%. However, a 1% increase in electricity consumption increases GDP by 0.15%, and electricity prices have an insignificant effect on GDP. The study in line with [37,70,71,72] reported similar results in Pakistan's case between energy use and economic development and energy consumers, except exogenous energy prices. Additionally, our results are similar to those of [73] for China and [74] for Turkey. These findings indicate that the key factors for Pakistan's economic progress are energy use and price. The overall vector error-correction models confirm that the industrial sector energy management could be viable. Since our study is limited to the use of electricity instead of the total use of electricity, Our findings do not merely contradict with the results in the [39] and [75] that shows energy shortages in Pakistan could affect economic growth, as causality varies from short- and long-term energy consumption to GDP. Our findings also vary from those reported in [76], which also considers the existence of unidirectional causality at the agammaegate level from electricity consumption to GDP. There could be several factors for the discrepancy in their outcomes, such as various data periods included in the research and econometric methods Granger causality form of Hsiao. Our findings show that as real economic growth rises, the electricity demand increases and distributors need to boost their capacity to maintain an adequate supply. While the increase in price decreases electricity consumption, that can decrease the productivity of the industrial sector. However, electricity consumption reflects positively in economic growth that could be considered for the policymakers.
In the vector error correction model, the IRF tracks the predictor variables' response to an unexpected shock or novelty. In this study, we calculated IRFs for sector-specific EC, EP, and GDP. IRF outcomes for the industrial sector is seen in Figure 5.
To determine how a shock affects alternative variables and how long the effect lasts, we use the generalized variable impulse responses proposed by [77] and [78] for ten years, the impulse responses from the variables given. Illustration Figure 5 shows shock in EC, EP, and GDP separately. If one standard deviation is positively shocked at electricity price, then electricity consumption is continually rising, and the GDP decline declined about two years afterward, it is continuously increasing and shows a positive long-run relationship. However, in response to electricity price positive shock increasing electricity consumption two years, then even continuously, electricity price positive shock increases GDP for three years then stable, which shows the long-run relationship. If one standard deviation shock in electricity consumption, then GDP upwards about five years after that constantly. However, the shock in electricity price declined GDP about seven years later; it seems steady.
Until now, the research has been limited to in-samples. Furthermore, to assess the comparative importance of the causality check's findings, we are now carrying out variance decomposition analyses. Because it is well renowned, the variance decomposition effects rely on ordering the variables necessary to recognize the structural VAR from the projected modified form VAR approach. In literature, the normal method is to propose the most credible order driven by economic concept and confirm the outcomes' robustness by inverting the ordering see, for example, [39,79]. For short, we are considering three years and for the long-run eight years in all models.
Table 6 depicts the findings of variance decompositions for the model (EC, EP, and GDP). In the short-run, electricity consumption analysis causes a 96.07% variation in electricity consumption shock fluctuation. Further shock to electricity price influences 3.66% in electricity consumption fluctuation, whereas GDP affects 0.27% in response to electricity consumption change. To check the long-run shock to electricity consumption causes 87.97% variation of the fluctuation is self-explained—however, electricity prices and GDP effects by 11.78% and 0.25%, respectively.
Period | Variance Decomposition of EC | |||
S.E. | EC | EP | GDP | |
1 | 0.026154 | 100 | 0 | 0 |
2 | 0.040085 | 96.92866 | 2.657988 | 0.41335 |
3 | 0.055235 | 96.07497 | 3.657699 | 0.267331 |
4 | 0.066524 | 95.30433 | 4.487052 | 0.208615 |
5 | 0.076296 | 93.71978 | 6.059193 | 0.221029 |
6 | 0.084754 | 91.77491 | 7.982465 | 0.242629 |
7 | 0.092929 | 89.84477 | 9.908605 | 0.246629 |
8 | 0.100791 | 87.9735 | 11.77734 | 0.249163 |
9 | 0.108377 | 86.54688 | 13.1834 | 0.269722 |
10 | 0.11552 | 85.69038 | 13.99968 | 0.309941 |
Table 7 illustrates the outcomes of variance decompositions for the model (EP, EC, and GDP). The short-run shock to electricity price causes a 44.97% variation of the electricity price fluctuation is generally self-explained. Further shock to electricity consumption influences 54.86% in the fluctuation of electricity prices. At the same time, GDP affects 0.17% to electricity prices. The long-run shock to electricity price causes a 24.15% variation of the fluctuation is self-explained—however, electricity consumption and GDP effects by 71.39% and 4.46%, respectively.
Period | Variance Decomposition of EP: | |||
S.E. | EC | EP | GDP | |
1 | 0.048996 | 56.37411 | 43.62589 | 0 |
2 | 0.080503 | 47.46051 | 52.45839 | 0.081095 |
3 | 0.111427 | 54.85629 | 44.96984 | 0.173865 |
4 | 0.136671 | 58.00428 | 41.5015 | 0.49422 |
5 | 0.158198 | 61.49309 | 36.99908 | 1.507833 |
6 | 0.17542 | 65.03976 | 32.20885 | 2.751394 |
7 | 0.190837 | 68.51778 | 27.79294 | 3.689283 |
8 | 0.20503 | 71.39459 | 24.14619 | 4.459221 |
9 | 0.218298 | 73.62662 | 21.30353 | 5.069856 |
10 | 0.23014 | 75.36009 | 19.18527 | 5.454636 |
Table 8 demonstrates the effects of variance decompositions for the model (GDP, EP, and EC). The short-run shock to GDP causes 95.87% variation of the fluctuation in GDP, usually is self-explained. Further shock to electricity consumption influences 1.78% in the fluctuation of GDP. However, electricity price affects 2.35% to GDP. However, the long-run shock to GDP causes 84.54% variation of the fluctuation is self-explained. But electricity consumption and electricity prices effects by 5.25% and 10.21% respectively.To summarize, the by-and-large findings of the variance decomposition endorse the results achieved by the VECM model. The primary effect that energy usage is influenced by electricity prices, whereas output and electricity consumption also positively affect real GDP.
Period | Variance Decomposition of GDP: | |||
S.E. | EC | EP | GDP | |
1 | 0.027913 | 7.08547 | 1.074455 | 91.84007 |
2 | 0.047862 | 2.562744 | 1.192117 | 96.24514 |
3 | 0.058702 | 1.780112 | 2.351816 | 95.86807 |
4 | 0.067107 | 2.679764 | 3.801723 | 93.51851 |
5 | 0.076742 | 4.208902 | 5.118915 | 90.67218 |
6 | 0.086717 | 4.775009 | 6.861578 | 88.36341 |
7 | 0.095723 | 5.065867 | 8.767336 | 86.1668 |
8 | 0.103643 | 5.248982 | 10.21453 | 84.53649 |
9 | 0.110786 | 5.330424 | 11.07375 | 83.59582 |
10 | 0.117053 | 5.316104 | 11.42037 | 83.26352 |
Pakistan's electricity generation from 1987 to 2019 was revised monthly at an average of 5,955 GWh. In 2018, the data hit an all-time peak of 16,946 GWh and a historic low of 2,313 GWh in 1989. The findings indicate that model coefficients met all predictions, and the electricity price is a reliable mediating component for the industrial sector that revealed a 1% change in electricity price decreases electricity consumption by 0.13%. The study found that as the rise in industrial electricity prices raised firms' energy costs, it could push firms to pursue technological innovation and enhance production performance. By reducing their conflicting duties, the institutional setup of energy divisions needs to be standardized. For instance, the NEPRA electricity regulator's role to advise the government on secure and reliable ways to meet electricity demands at manageable rates should be fulfilled as per law. As well a 1% change in GDP increase EC by 0.09%. Disagammaegated power use and economic development analyses would make it more useful for authorities to devise a robust energy-saving and environmental degradation strategy. The findings show the industries in which economic development over long periods is related to energy consumption. A 1% upsurge in electricity consumption decreases electricity price by 0.19%, which is quite useful for policymakers that managing industrial electricity prices can enhance manufacturing productivity. These prediction findings were correlated with related studies in the existing literature to demonstrate the accuracy of the present analysis. The findings differ from various studies focused on energy factors using different models and periods, such as [12,80,81,82,83,84,85]. In Pakistan's case, many studies concluded differently, such as [3,88–92]; however, none of them focused on these factors. The novel findings can be beneficial for the sustainable energy policy as per Pakistan's industrial sector's potential.
The current electricity provision rates in Pakistan are considered unsustainable due, in particular, to poor infrastructure. The widespread blackouts throughout the country have incredibly adverse effects on Pakistan's economic growth and social lives. The current study contributes to the emerging energy literature by investigating the causal link in the industrial sector in Pakistan between electricity consumption, prices, and GDP, by using a neoclassical development system. The empirical analysis was conducted using data for the period 1970–2018. The indication of co-integration between variables showed the long-run relationship. It ensures a short-term fluctuation in electricity consumption and GDP, which ultimately returns to long-run equilibrium. Most of the coefficients lagged in our analysis are significant at a 5% level except for GDP electricity prices. The outcomes show a causal relationship from electricity consumption to price and GDP that impact is long-term in the industrial sector, which is the foremost determinant of Pakistan's economic change.
Pakistan is a country where a lack of electricity and the electricity sector work bare capacity margin. Based on our findings obtained in this study. More interest should be given to policy recommendations as follows: (a) 1% rise in the price of energy reduces electricity usage by 0.13%, and a 1% increase in GDP raises EC by 0.09%. The result implies that the key driver is the energy price, which indicates an inverse impact on electricity usage that is visa-versa with the costs of industrial production. Also, an increase in EC was positively affecting GDP. The government should invest more in power projects to maintain energy demands and supply, which is beneficial for economic growth and boosts industrial productivity. (b) While a 1 percent rise in energy demand reduces electricity by 0.19%, the price of electricity reduces by 0.16 percent if GDP increases by 1 percent. (c) However, an increase in energy consumption by 1% raises GDP by 0.15%. The empirical results further suggest that electricity prices are the main factor in the use of industrial electricity. The findings suggest that officials should focus on relevant legislation, subsidy reforms, and tax relief in the industrial sector. Moreover, it will attract the business community; foreign investors also provide employment opportunities and boost Pakistan's GDP growth. As a whole, electricity consumption is essential for long-term economic stability and growth in the industrial sector. We suggest that infrastructure planning and investments are vital to meeting the growing electricity demand. Whereas any policies to ease the electricity market should stimulate productivity, the invention in generation and supply, resulting in lesser prices as autonomous suppliers, can stabilize the fluctuation that may positively affect the long term. Finally, we propose that planning and investing in infrastructure growth is essential to meeting the growing electricity demand.
We have some limitations concerning our studies. Based on the findings of multiple literature pieces, this study selects energy consumption, price, and GDP as useful for the industrial sector. This research was carried out in the industrial sector's specific context, so other Pakistan sectors need to be investigated.
The authors declare no conflict of interest.
[1] | Kraft J, Kraft A (1978) On the relationship between energy and GNP. J Energy Dev 3: 401-403. |
[2] | Bowden N, Payne JE (2010) Sectoral analysis of the causal relationship between renewable and non-renewable energy consumption and real output in the US. Energy Sources, Part B Econ Plan Policy 5: 400-408. |
[3] | Foon C, Shahbaz M (2013) Sectoral analysis of the causal relationship between electricity consumption and real output in Pakistan. Energy Policy 60: 885-891. |
[4] | Ameyaw B, Oppong A, Abruquah LA, et al. (2017) Causality nexus of electricity consumption and economic growth: An empirical evidence from Ghana. 1-10. |
[5] | Abosedra S, Dah A, Ghosh S (2009) Electricity consumption and economic growth, the case of Lebanon. Appl Energy 86: 429-432. |
[6] | Shahbaz M, Feridun M (2012) Electricity consumption and economic growth empirical evidence from Pakistan. 1583-1599. |
[7] | Mahmoodi M, Mahmoodi E (2015) Renewable energy consumption and economic growth: The case of 7 Asian developing countries. Am J Sci Res. |
[8] | Binh PT (2011) Energy consumption and economic growth in Vietnam: Threshold cointegration and causality analysis. Int J Energy Econ Policy 1: 1-17. |
[9] | Dimitropoulos J, Hunt L, Judge G (2004) Estimating underlying energy demand trends using UK annual data. Surrey Energy Econ Cent. |
[10] | Hunt, L. C H (2019) Unravelling trends and seasonality: A structural time series analysis of transport oil demand in the UK and Japan. 24: 6574. |
[11] | Dimitropoulos J, Hunt LC, Judge G (2005) Estimating underlying energy demand trends using UK annual data Estimating underlying energy demand trends using UK annual data. Appl Econ Lett 12: 37-41. |
[12] | Dilaver Z, Hunt LC (2011) Industrial electricity demand for Turkey: A structural time series analysis. Energy Econ 33: 426-436. |
[13] | Hunt LC, Ninomiya Y (2012) Unravelling trends and seasonality: A structural time series analysis of transport oil demand in the UK and Japan. Int Assoc Energy Econ 24: 63-96. |
[14] | Hunt LC (2003) Edward elgar, energy in a competitive market: essays in honour of colin robinson, 2003. Available from: https://iea.org.uk/. |
[15] | Harvey A (1997) Trends, cycles and autoregressions. Econ J 107: 192-201. |
[16] | Diebold FX (1992) Forecasting, structural time series models. Econom Theory 8: 293-299. |
[17] | Harvey A, Jan Koopman S, Shephard N (2004) State space and unobserved component models: Theory and applications. State Sp Unobserved Compon Model Theory Appl 1-380. |
[18] | PES (2019) Pakistan economic survey. Pakistan Econ Surv 479: 299. |
[19] | Bekhet HA, Abdullah TAR bin T, Yasmin T (2016) Measuring output multipliers of energy consumption and manufacturing sectors in Malaysia during the global financial crisis. Procedia Econ Financ 35: 179-188. |
[20] | Mohiuddin O, Asumadu-Sarkodie S, Obaidullah M (2016) The relationship between carbon dioxide emissions, energy consumption, and GDP: A recent evidence from Pakistan. Cogent Eng 3: 1-16. |
[21] | Mahmood Iqbal (2014) Residential demand for electricity and natural gas in Pakistan. Pakistan Inst Dev Econ Islam 22: 23-36. |
[22] | Atif SM, Siddiqi MW (2012) The electricity consumption and economic growth nexus in Pakistan: A new evidence. SSRN Electron J. |
[23] | Belaïd F, Youssef A Ben, Lazaric N (2020) Scrutinizing the direct rebound effect for French households using quantile regression and data from an original survey. Ecol Econ 176: 106755. |
[24] | Bélaïd F, Youssef M (2017) Environmental degradation, renewable and non-renewable electricity consumption, and economic growth: Assessing the evidence from Algeria. Energy Policy 102: 277-287. |
[25] | Chen Y, Fang Z (2018) Industrial electricity consumption, human capital investment and economic growth in Chinese cities. Econ Model 69: 205-219. |
[26] | Cialani C, Mortazavi R (2018) Household and industrial electricity demand in Europe. Energy Policy 122: 592-600. |
[27] | Altinay G, Karagol E (2005) Electricity consumption and economic growth: Evidence from Turkey. Energy Econ 27: 849-856. |
[28] | Karanfil F (2008) Energy consumption and economic growth revisited: Does the size of unrecorded economy matter? Energy Policy 36: 3029-3035. |
[29] | Lise W, Van Montfort K (2007) Energy consumption and GDP in Turkey: Is there a co-integration relationship? Energy Econ 29: 1166-1178. |
[30] | Bélaïd F, Abderrahmani F (2013) Electricity consumption and economic growth in Algeria: A multivariate causality analysis in the presence of structural change. Energy Policy 55: 286-295. |
[31] | Belaïd F (2018) Exposure and risk to fuel poverty in France: Examining the extent of the fuel precariousness and its salient determinants. Energy Policy 114: 189-200. |
[32] | Shahbaz M, Zeshan M, Afza T (2012) Is energy consumption effective to spur economic growth in Pakistan? New evidence from bounds test to level relationships and Granger causality tests. Econ Model 29: 2310-2319. |
[33] | Nazlioglu S, Kayhan S, Adiguzel U (2014) Electricity consumption and economic growth in Turkey: Cointegration, linear and nonlinear granger causality. Energy Sources, Part B Econ Plan Policy 9: 315-324. |
[34] | Al-bajjali SK, Yacoub A (2018) Estimating the determinants of electricity consumption in Jordan. Energy 147: 1311-1320. |
[35] | Ghafoor A, Rehman TU, Munir A, et al. (2016) Current status and overview of renewable energy potential in Pakistan for continuous energy sustainability. Renew Sustain Energy Rev 60: 1332-1342. |
[36] | Sidique R (2014) Impact of Fossil Fuel Energy Consumption on CO2 Emissions: Evidence from Pakistan (1980-2010). Pak Dev Rev 327-346. |
[37] | Shahbaz M, Hooi H (2012) The dynamics of electricity consumption and economic growth: A revisit study of their causality in Pakistan. Energy 39: 146-153. |
[38] | Javid M, Qayyum A (2014) Electricity consumption-GDP nexus in Pakistan: A structural time series analysis. Energy 64: 811-817. |
[39] | Jamil F, Ahmad E (2010) The relationship between electricity consumption, electricity prices and GDP in Pakistan. Energy Policy 38: 6016-6025. |
[40] | Khan MA, Abbas F (2016) The dynamics of electricity demand in Pakistan: A panel cointegration analysis. Renew Sustain Energy Rev 65: 1159-1178. |
[41] | Hussain A, Rahman M, Alam J (2016) Forecasting electricity consumption in Pakistan: the way forward. Energy Policy 90: 73-80. |
[42] | Lin B, Yousaf M (2021) Analysis of electricity consumption in Pakistan using index decomposition and decoupling approach. Energy 214: 118888. |
[43] | Lin B, Yousaf M (2020) Analysis of energy security indicators and CO2 emissions. A case from a developing economy. Energy 200: 117575. |
[44] | Lin B, Yousaf M (2020) Energy substitution effect on transport sector of Pakistan: A trans-log production function approach. J Clean Prod 251: 119606. |
[45] | Shiu A, Lam P (2004) Electricity consumption and economic growth in China. Energy Policy 32: 47-54. |
[46] | Dagher L, Yacoubian T (2012) The causal relationship between energy consumption and economic growth in Lebanon. Energy Policy 50: 795-801. |
[47] | Kasperowicz R (2014) Electricity consumption and economic growth: Evidence from Poland. J Int Stud 7: 46-57. |
[48] | Bayar Y, Özel H (2014) Electricity consumption and economic growth in emerging economies. J Knowl Manag Econ Inf Technol 4: 1-18. |
[49] | Lu WC (2017) Electricity consumption and economic growth: Evidence from 17 Taiwanese industries. Sustainability 9. |
[50] | Ben-Salha O, Hkiri B, Aloui C (2018) Sectoral energy consumption by source and output in the U.S.: New evidence from wavelet-based approach. Energy Econ 72: 75-96. |
[51] | Abokyi E, Appiah-Konadu P, Sikayena I, et al. (2018) Consumption of electricity and industrial growth in the case of Ghana. J Energy 2018: 1-11. |
[52] | Yousaf M, Muhammad R, Sultan T (2019) Analysis of coal-related energy consumption in Pakistan: an alternative energy resource to fuel economic development. Environ Dev Sustain. |
[53] | Abbasi K, Lv K, Nadeem MA, et al. (2020) Agricultural and manufacturing sector determinants consumption, price, and real GDP from Pakistan of electricity. North Am Acad Res 3: 21-44. |
[54] | GOP (2018) Ministry of finance, pakistan economic survey, 2018. Available from: http://121.52.153.178:8080/xmlui/handle/123456789/6541. |
[55] | NTDC (2018) National tansmission & despatch comapany limited Pakistan, 2018. Available from: https://www.ntdc.com.pk/misc-downloads. |
[56] | World-Bank (2018) World Bank Open Data, 2018. Available from: https://data.worldbank.org/. |
[57] | Phillips PCB, Perron P (1988) Testing for a unit root in time series regression. Biometrika 75: 335. |
[58] | Dickey DA, Fuller WA (1979) Distribution of the estimators for autoregressive time series with a unit root. J Am Stat Assoc 74: 427-431. |
[59] | Nyamdash B and D (2011) The economic impact of electricity conservation policies: A case study of Ireland. Munich Pers RePEc Arch. |
[60] | Mehmood Mirza F, Bergland O, Afzal N (2014) Electricity conservation policies and sectorial output in Pakistan: An empirical analysis. Energy Policy 73: 757-766. |
[61] | Johansen S, Juselius K (1990) Maximum likelihood estimation and inference on cointegration-with applications to the demand for money. Oxf Bull Econ Stat 52: 169-210. |
[62] | Johansen S (1988) Statistical analysis of cointegration vectors. J Econ Dyn Control 12: 231-254. |
[63] | Ismail A, Amjad S (2014) Determinants of terrorism in Pakistan: An empirical investigation. Econ Model 37: 320-331. |
[64] | Lee S, Chong WO (2016) Causal relationships of energy consumption, price, and CO2 emissions in the U.S. building sector. Resour Conserv Recycl 107: 220-226. |
[65] | Kang SH, Islam F, Kumar Tiwari A (2019) The dynamic relationships among CO2 emissions, renewable and non-renewable energy sources, and economic growth in India: Evidence from time-varying Bayesian VAR model. Struct Chang Econ Dyn 50: 90-101. |
[66] | Ahmad N, Du L (2017) Effects of energy production and CO2 emissions on economic growth in Iran: ARDL approach. Energy 123: 521-537. |
[67] | Soytas U, Sari R (2003) Energy consumption and GDP: causality relationship in G-7 countries and emerging markets. Energy Econ 33-37. |
[68] | Kashif Abbasi, Jiao Z, Khan A, et al. (2020) Asymmetric impact of renewable and non-renewable energy on economic growth in Pakistan: New evidence from a nonlinear analysis. Energy Explor Exploit. |
[69] | Engle RF, Granger CWJ, Mar N (1987) Co-integration and error correction: Representation, estimation, and testing. Econometrica 55: 251-276. |
[70] | Rehman A, Deyuan Z (2018) Pakistan's energy scenario: A forecast of commercial energy consumption and supply from different sources through 2030. Energy Sustain Soc 8: 0-4. |
[71] | Zaman M, Shaheen F, Haider A, et al. (2015) Examining relationship between electricity consumption and its major determinants in Pakistan. Int J Energy Econ Policy 5: 998-1009. |
[72] | Muhammad H, Siddique A, Majeed MT (2016) The relationship between energy consumption and economic growth in Pakistan. Bull Energy Econ 4: 329-334. |
[73] | Yuan J, Kang J, Zhao C, et al. (2008) Energy consumption and economic growth: Evidence from China at both aggregated and disaggregated levels. Energy Econ 30: 3077-3094. |
[74] | Sari R, Soytas U (2004) Disaggregate energy consumption, employment and income in Turkey. Energy Econ 26: 335-344. |
[75] | Lee CC (2005) Energy consumption and GDP in developing countries: A cointegrated panel analysis. Energy Econ 27: 415-427. |
[76] | Aqeel A (2001) The Relationship between energy consmption and economic growth in Pakistan. Asia-Pacific Dev J 8: 101-110. |
[77] | Koop G, Pesaran MH, Potter SM (1996) Impulse response analysis in nonlinear multivariate models. J Econom 74: 119-147. |
[78] | Hashem H, Pesaran YS (1998) Generalized impulse response analysis in linear multivariate models. Econ Lett 62: 85-90. |
[79] | Lorde T, Waithe K, Francis B (2010) The importance of electrical energy for economic growth in Barbados. Energy Econ 32: 1411-1420. |
[80] | Azadeh A, Ghaderi SF, Sohrabkhani S (2008) Annual electricity consumption forecasting by neural network in high energy consuming industrial sectors. Energy Convers Manag 49: 2272-2278. |
[81] | Junejo I, Khoso JR (2018) Impact of Electricity crisis on industrial growth in Pakistan: A multiple regression analysis approach. Int J Acad Res Bus Soc Sci 8: 851-862. |
[82] | Ateba BB, Prinsloo JJ, Gawlik R (2019) The significance of electricity supply sustainability to industrial growth in South Africa. Energy Reports 5: 1324-1338. |
[83] | Bulut U, Inglesi-Lotz R (2019) Which type of energy drove industrial growth in the US from 2000 to 2018? Energy Reports 5: 425-430. |
[84] | Ewing BT, Sari R, Soytas U (2007) Disaggregate energy consumption and industrial output in the United States. Energy Policy 35: 1274-1281. |
[85] | Pegels A, Lütkenhorst W (2014) Is Germany's energy transition a case of successful green industrial policy? Contrasting wind and solar PV. Energy Policy 74: 522-534. |
1. | Kashif Raza Abbasi, Muhammad Shahbaz, Zhilun Jiao, Muhammad Tufail, How energy consumption, industrial growth, urbanization, and CO2 emissions affect economic growth in Pakistan? A novel dynamic ARDL simulations approach, 2021, 221, 03605442, 119793, 10.1016/j.energy.2021.119793 | |
2. | Radosław Wolniak, Adam Wyszomirski, Marcin Olkiewicz, Anna Olkiewicz, Environmental Corporate Social Responsibility Activities in Heating Industry—Case Study, 2021, 14, 1996-1073, 1930, 10.3390/en14071930 | |
3. | Rabnawaz Khan, Bing Xue, Beta decoupling relationship between CO2 emissions by GDP, energy consumption, electricity production, value-added industries, and population in China, 2021, 16, 1932-6203, e0249444, 10.1371/journal.pone.0249444 | |
4. | Faik Bilgili, Sevda Kuşkaya, Masreka Khan, Ashar Awan, Oguzhan Türker, The roles of economic growth and health expenditure on CO2 emissions in selected Asian countries: a quantile regression model approach, 2021, 0944-1344, 10.1007/s11356-021-13639-6 | |
5. | Georgeta Soava, Anca Mehedintu, Mihaela Sterpu, Eugenia Grecu, The Impact of the COVID-19 Pandemic on Electricity Consumption and Economic Growth in Romania, 2021, 14, 1996-1073, 2394, 10.3390/en14092394 | |
6. | An Pan, Shuangshuang Feng, Xinyuan Hu, Yaya Li, Bing Xue, How environmental regulation affects China’s rare earth export?, 2021, 16, 1932-6203, e0250407, 10.1371/journal.pone.0250407 | |
7. | Jaffar Abbas, Riaqa Mubeen, Paul Terhemba Iorember, Saqlain Raza, Gulnara Mamirkulova, Exploring the impact of COVID-19 on tourism: transformational potential and implications for a sustainable recovery of the travel and leisure industry, 2021, 2, 26665182, 100033, 10.1016/j.crbeha.2021.100033 | |
8. | Jehn-Yih Wong, Ming-Lee Hsiung, Shu-Ju Lee, Chia-Ying ChouHuang, The Relationship between Endurance Involvement and Travel Behavior in Camping and the Moderating Effect of Place Attachment, 2021, 13, 2071-1050, 5016, 10.3390/su13095016 | |
9. | Jiajia Wang, Yue Teng, Zhe Chen, Jing Bai, Yongning Niu, Huabo Duan, Assessment of carbon emissions of building interior decoration and renovation waste disposal in the fast-growing Greater Bay Area, China, 2021, 798, 00489697, 149158, 10.1016/j.scitotenv.2021.149158 | |
10. | Mustafa Tevfik Kartal, Uzair Ali, Zhanar Nurgazina, Asymmetric effect of electricity consumption on CO2 emissions in the USA: analysis of end-user electricity consumption by nonlinear quantile approaches, 2022, 29, 0944-1344, 83824, 10.1007/s11356-022-21715-8 | |
11. | Vipin Jain, Carlos Samuel Ramos-Meza, Ejaz Aslam, Chanchal Chawla, Tabish Nawab, Malik Shahzad Shabbir, Ajay Bansal, Do Energy Resources matter for Growth Level? The dynamic effects of different strategies of renewable energy, carbon emissions on sustainable economic growth, 2022, 1618-954X, 10.1007/s10098-022-02432-9 | |
12. | Peng Wang, Yuting Yang, Cheng Ji, Lei Huang, Influence of built environment on building energy consumption: a case study in Nanjing, China, 2023, 1387-585X, 10.1007/s10668-023-02930-w | |
13. | Sarah Ahmed, Nazima Ellahi, Ajmal Waheed, Nida Aman, Policy Intervention and Financial Sustainability in an Emerging Economy: A Structural Vector Auto Regression Analysis, 2022, 13, 1664-1078, 10.3389/fpsyg.2022.924545 | |
14. | Muhammad Usman, Rakhshanda Kousar, Muhammad Sohail Amjad Makhdum, Muhammad Rizwan Yaseen, Abdul Majeed Nadeem, Do financial development, economic growth, energy consumption, and trade openness contribute to increase carbon emission in Pakistan? An insight based on ARDL bound testing approach, 2023, 25, 1387-585X, 444, 10.1007/s10668-021-02062-z | |
15. | Tao Huang, Mingtao Ding, Zemin Gao, Ricardo Delgado Téllez, Check dam storage capacity calculation based on high-resolution topogrammetry: Case study of the Cutou Gully, Wenchuan County, China, 2021, 790, 00489697, 148083, 10.1016/j.scitotenv.2021.148083 | |
16. | Chang-Qing Guo, Xin Wang, Dan-Dan Cao, Yong-Guang Hou, The Impact of Green Finance on Carbon Emission--Analysis Based on Mediation Effect and Spatial Effect, 2022, 10, 2296-665X, 10.3389/fenvs.2022.844988 | |
17. | Hongfang Lu, Dongmin Xi, Guojin Qin, Environmental risk of oil pipeline accidents, 2023, 874, 00489697, 162386, 10.1016/j.scitotenv.2023.162386 | |
18. | Kunsen Lin, Youcai Zhao, Xiaofeng Gao, Meilan Zhang, Chunlong Zhao, Lu Peng, Qian Zhang, Tao Zhou, Applying a deep residual network coupling with transfer learning for recyclable waste sorting, 2022, 29, 0944-1344, 91081, 10.1007/s11356-022-22167-w | |
19. | Charles Shaaba Saba, CO2 emissions-energy consumption-militarisation-growth nexus in South Africa: evidence from novel dynamic ARDL simulations, 2022, 30, 1614-7499, 18123, 10.1007/s11356-022-23069-7 | |
20. | Rongji Wang, Ume Laila, Rabia Nazir, Xibin Hao, Unleashing the influence of industrialization and trade openness on renewable energy intensity using path model analysis: A roadmap towards sustainable development, 2023, 202, 09601481, 280, 10.1016/j.renene.2022.11.035 | |
21. | Richard Cartland, Al-Mas Sendegeya, Jean de Dieu Khan Hakizimana, Performance Analysis of a Hybrid of Solar Photovoltaic, Genset, and Hydro of a Rural-Based Power Mini-Grid: Case Study of Kisiizi Hydro Power Mini-Grid, Uganda, 2023, 11, 2227-9717, 175, 10.3390/pr11010175 | |
22. | Abdul Rehman, Hengyun Ma, Magdalena Radulescu, Crenguta Ileana Sinisi, Zahid Yousaf, Energy Crisis in Pakistan and Economic Progress: Decoupling the Impact of Coal Energy Consumption in Power and Brick Kilns, 2021, 9, 2227-7390, 2083, 10.3390/math9172083 | |
23. | Alieu Gibba, Muhammad Kamran Khan, Modeling the causal dynamics among energy consumption, economic growth, and oil import prices: A panel co-integration analysis for EU economies, 2023, 2, 2813-2823, 10.3389/frevc.2023.1114175 | |
24. | Muhammad Sani Musa, Gylych Jelilov, Paul Terhemba Iorember, Ojonugwa Usman, Effects of tourism, financial development, and renewable energy on environmental performance in EU-28: does institutional quality matter?, 2021, 28, 0944-1344, 53328, 10.1007/s11356-021-14450-z | |
25. | Qiang Gao, Hengyuan Zeng, Guanglin Sun, Jianfeng Li, Extreme risk spillover from uncertainty to carbon markets in China and the EU—A time varying copula approach, 2023, 326, 03014797, 116634, 10.1016/j.jenvman.2022.116634 | |
26. | Aysha Zamir, Noreen Mujahid, Nexus among green energy consumption, foreign direct investment, green innovation technology, and environmental pollution on economic growth, 2022, 29, 0944-1344, 76501, 10.1007/s11356-022-23184-5 | |
27. | Rafia Afroz, Md Muhibbullah, Dynamic linkages between non-renewable energy, renewable energy and economic growth through nonlinear ARDL approach: evidence from Malaysia, 2022, 29, 0944-1344, 48795, 10.1007/s11356-022-19346-0 | |
28. | Akmal Shahzad, Bushra Zulfiqar, Mehmood ul Hassan, Naif Mansour Mathkur, Irfan Ahmed, Investigating the Effects of Capital Structure and Corporate Governance on Firm Performance: An Analysis of the Sugar Industry, 2022, 13, 1664-1078, 10.3389/fpsyg.2022.905808 | |
29. | Shama Nazneen, Xu Hong, Nizam Ud Din, Barkat Jamil, Kramat Hussain, The moderating role of technological innovation between tourism and carbon emission: short and long-run panel analysis, 2023, 1614-7499, 10.1007/s11356-023-25892-y | |
30. | Ying Feng, Ching-Cheng Lu, I-Fang Lin, Jia-Yan Lin, Dynamic assessment of agro-industrial sector efficiency and productivity changes among G20 nations, 2021, 0958-305X, 0958305X2110560, 10.1177/0958305X211056030 | |
31. | Talat Ulussever, Serpil Kılıç Depren, Mustafa Tevfik Kartal, Özer Depren, Estimation performance comparison of machine learning approaches and time series econometric models: evidence from the effect of sector-based energy consumption on CO2 emissions in the USA, 2023, 1614-7499, 10.1007/s11356-023-26050-0 | |
32. | Sheeba Babu, L Ashok Kumar, Voltage controller with energy management unit for microgrid with hybrid sources, 2021, 39, 0144-5987, 2126, 10.1177/01445987211015392 | |
33. | Chunlei Wang, Dake Wang, Jaffar Abbas, Kaifeng Duan, Riaqa Mubeen, Global Financial Crisis, Smart Lockdown Strategies, and the COVID-19 Spillover Impacts: A Global Perspective Implications From Southeast Asia, 2021, 12, 1664-0640, 10.3389/fpsyt.2021.643783 | |
34. | Muzaffar Iqbal, Junhai Ma, Navieed Ahmad, Kramat Hussain, Muhammad Waqas, Yanjie Liang, Sustainable construction through energy management practices: an integrated hierarchal framework of drivers in the construction sector, 2022, 29, 0944-1344, 90108, 10.1007/s11356-022-21928-x | |
35. | Vadim Zasko, Elena Sidorova, Vera Komarova, Diana Boboshko, Olesya Dontsova, Digitization of the Customs Revenue Administration as a Factor of the Enhancement of the Budget Efficiency of the Russian Federation, 2021, 13, 2071-1050, 10757, 10.3390/su131910757 | |
36. | Paul Terhemba Iorember, Bruce Iormom, Terungwa Paul Jato, Jaffar Abbas, Understanding the bearable link between ecology and health outcomes: the criticality of human capital development and energy use, 2022, 8, 24058440, e12611, 10.1016/j.heliyon.2022.e12611 | |
37. | Mustafa KEVSER, Bitcoin as an Alternative Financial Asset Class: Relations Between Geopolitical Risk, Global Economic Political Uncertainty, and Energy Consumption, 2022, 2148-8703, 10.34232/pjess.1159796 | |
38. | Fei Yang, Chunchen Wang, Clean energy, financial development, and economic growth: Evidence from spatial spillover effects and quasi-natural experiments, 2021, 322, 09596526, 129045, 10.1016/j.jclepro.2021.129045 | |
39. | Huang Fu, Kashif Abbass, Tehmina Fiaz Qazi, Abdul Aziz Khan Niazi, Monica Violeta Achim, Analyzing the barriers to putting corporate financial expropriations to a halt: A structural modeling of the phenomenon, 2022, 10, 2296-665X, 10.3389/fenvs.2022.967418 | |
40. | Gang Lin, Dong Jiang, Jingying Fu, Yitong Yin, Pathways for the carbon peak of resource-based cities under an energy-water coupling relationship: A case study of Taiyuan, Shanxi Province, 2022, 10, 2296-665X, 10.3389/fenvs.2022.994543 | |
41. | Riaqa Mubeen, Dongping Han, Jaffar Abbas, Susana Álvarez-Otero, Muhammad Safdar Sial, The Relationship Between CEO Duality and Business Firms’ Performance: The Moderating Role of Firm Size and Corporate Social Responsibility, 2021, 12, 1664-1078, 10.3389/fpsyg.2021.669715 | |
42. | Yue Zhu, Juntao Zhang, Caiquan Duan, How does green finance affect the low-carbon economy? Capital allocation, green technology innovation and industry structure perspectives, 2023, 36, 1331-677X, 3519, 10.1080/1331677X.2022.2110138 | |
43. | Emre Tepe, The impact of built and socio-economic environment factors on Covid-19 transmission at the ZIP-code level in Florida, 2023, 326, 03014797, 116806, 10.1016/j.jenvman.2022.116806 | |
44. | Sandylove Afrane, Jeffrey Dankwa Ampah, Emmanuel Mensah Aboagye, Investigating evolutionary trends and characteristics of renewable energy research in Africa: a bibliometric analysis from 1999 to 2021, 2022, 29, 0944-1344, 59328, 10.1007/s11356-022-20125-0 | |
45. | Yating Deng, Fengtao Guang, Shuifeng Hong, Le Wen, How does power technology innovation affect carbon productivity? A spatial perspective in China, 2022, 29, 0944-1344, 82888, 10.1007/s11356-022-21488-0 | |
46. | Zhu Weimin, Muhammad Zubair Chishti, Abdul Rehman, Manzoor Ahmad, A pathway toward future sustainability: Assessing the influence of innovation shocks on CO2 emissions in developing economies, 2022, 24, 1387-585X, 4786, 10.1007/s10668-021-01634-3 | |
47. | Mohammad Amin Edalatpour, Seyed Mohammad Javad Mirzapour Al-e-Hashem, Amir Mohammad Fathollahi-Fard, Combination of pricing and inventory policies for deteriorating products with sustainability considerations, 2023, 1387-585X, 10.1007/s10668-023-02988-6 | |
48. | Tomasz Chrulski, Mariusz Łaciak, Analysis of Natural Gas Consumption Interdependence for Polish Industrial Consumers on the Basis of an Econometric Model, 2021, 14, 1996-1073, 7649, 10.3390/en14227649 | |
49. | Qiang Liu, Xiaoli Qu, Dake Wang, Jaffar Abbas, Riaqa Mubeen, Product Market Competition and Firm Performance: Business Survival Through Innovation and Entrepreneurial Orientation Amid COVID-19 Financial Crisis, 2022, 12, 1664-1078, 10.3389/fpsyg.2021.790923 | |
50. | Segla Roch Cedrique Zossou, Patrice Ygue Adegbola, Brice Tiburce Oussou, Gustave Dagbenonbakin, Roch Mongbo, Carlos Alberto Zúniga-González, Modelling smallholder farmers’ preferences for soil fertility management technologies in Benin: A stated preference approach, 2021, 16, 1932-6203, e0253412, 10.1371/journal.pone.0253412 | |
51. | Nourhane Merabet, Lina Chouichi, Kaouther Kerboua, Numerical design and simulation of a thermodynamic solar solution for a pilot residential building at the edge of the sun-belt region, 2022, 24, 1387-585X, 12582, 10.1007/s10668-021-01956-2 | |
52. | Pobitra Halder, Ibrahim Gbolahan Hakeem, Savankumar Patel, Shaheen Shah, Hafijur Khan, Kalpit Shah, 2022, 9780323886680, 23, 10.1016/B978-0-323-88668-0.00011-5 | |
53. | Rania Seif, Fatma Zakaria Salem, Nageh K. Allam, E-waste recycled materials as efficient catalysts for renewable energy technologies and better environmental sustainability, 2023, 1387-585X, 10.1007/s10668-023-02925-7 | |
54. | Mohaddeseh Azimi, Zhengfu Bian, Provincial and regional analysis of carbon neutrality policy and the environmental Kuznets curve: examining their effect on CO2 emissions in China, 2023, 1614-7499, 10.1007/s11356-023-25419-5 | |
55. | Maria Conceição Costa, Mara Madaleno, Pedro Macedo, Generalized maximum entropy in electrical energy price modeling for households and non-households in Portugal, 2022, 8, 23524847, 448, 10.1016/j.egyr.2022.01.091 | |
56. | Qiyao Liu, Xiaodong Zhu, Incentive strategies for retired power battery closed-loop supply chain considering corporate social responsibility, 2023, 1387-585X, 10.1007/s10668-023-03017-2 | |
57. | Aimen Ayub, Abraiz Khattak, Ahmad Aziz Alahmadi, Abdul Kashif Janjua, Nasim Ullah, Subrata Kumar Sarker, Investigation of a Battery Storage System Aimed at Demand-Side Management of Residential Load, 2023, 2023, 2050-7038, 1, 10.1155/2023/8079997 | |
58. | Daniel Balsalobre-Lorente, Jaffar Abbas, Chang He, Ladislav Pilař, Syed Ale Raza Shah, Tourism, urbanization and natural resources rents matter for environmental sustainability: The leading role of AI and ICT on sustainable development goals in the digital era, 2023, 82, 03014207, 103445, 10.1016/j.resourpol.2023.103445 | |
59. | Faisal Faisal, Sami Ur Rahman, Awais Khan, Adnan Ali, Mahmoud Abdelkarim Irshaid, Muhammad Yusuf Amin, Exploring the N‐shaped EKC in the top tourist destinations. Empirical evidence from cross‐country analysis, 2023, 73, 0020-8701, 479, 10.1111/issj.12397 | |
60. | Mochammad Fahlevi, Fakhrul Hasan, Mohammad Raijul Islam, Exploring consumer attitudes and purchase intentions: Unraveling key influencers in China’s green agricultural products market, 2023, 4, 27084965, 74, 10.22495/cbsrv4i3art8 | |
61. | Jinhua Tian, Jiaru Zhang, Kai Han, Jianfeng Qiao, Ping Li, 2024, Chapter 34, 978-981-97-1974-7, 377, 10.1007/978-981-97-1975-4_34 | |
62. | Soumya Basu, Keiichi Ishihara, Takaya Ogawa, Hideyuki Okumura, Structural Effects of Economic Shocks on the Macroeconomic Economy–Electricity–Emissions Nexus in India via Long-Term Cointegration Approach, 2024, 17, 1996-1073, 4354, 10.3390/en17174354 | |
63. | Nurcan Kilinc-Ata, Abdulkadir Barut, Mücahit Citil, Do military expenditures have an impact on the adoption of renewable energy in OECD nations? Evidence from a panel cointegration test approach, 2024, 18, 1750-6220, 1745, 10.1108/IJESM-07-2023-0010 | |
64. | Prince Opoku, Huaming Song, Sustainability and affordability of Chinese-funded renewable energy project in sub-Saharan Africa: a hybridized solid oxide fuel cell, temperature sensors, and lithium-based solar system approach, 2023, 30, 1614-7499, 80768, 10.1007/s11356-023-27661-3 | |
65. | Mohammad Hossein Jahangir, Reza Mokhtari, Fatemeh Salmanpour, Hossein Yousefi, Urban energy planning towards achieving an economically and environmentally optimized energy flow by 2050 based on different scenarios (a case study), 2024, 1573-2975, 10.1007/s10668-024-04754-8 | |
66. | Zhonggen Sun, Furong Zhang, Yifei Wang, Ziting Shao, Literature review and analysis of the social impact of a just energy transition, 2023, 7, 2571-581X, 10.3389/fsufs.2023.1119877 | |
67. | T. S. Ratna, T. Akhter, A. Chowdhury, F. Ahmed, Unveiling the causal link between livestock farming, economic development, and methane emissions in Bangladesh: a VECM investigation, 2024, 21, 1735-1472, 773, 10.1007/s13762-023-04993-8 | |
68. | Adel BEN YOUSSEF, The role of NGOs in climate policies: The case of Tunisia, 2024, 220, 01672681, 388, 10.1016/j.jebo.2024.02.016 | |
69. | Sebastian Borja-Urbano, Fabián Rodríguez-Espinosa, Marco Luna-Ludeña, Theofilos Toulkeridis, Valuing the Impact of Air Pollution in Urban Residence Using Hedonic Pricing and Geospatial Analysis, Evidence From Quito, Ecuador, 2021, 14, 1178-6221, 10.1177/11786221211053277 | |
70. | Mohammad Musa, Yanhua Gao, Preethu Rahman, Ahmad Albattat, Muhammad Abu Sufyan Ali, Swapan Kumar Saha, Sustainable development challenges in Bangladesh: an empirical study of economic growth, industrialization, energy consumption, foreign investment, and carbon emissions—using dynamic ARDL model and frequency domain causality approach, 2024, 26, 1618-954X, 1799, 10.1007/s10098-023-02680-3 | |
71. | Jianwei Xu, Shuxin Liu, Current status, evolutionary path, and development trends of low-carbon technology innovation: a bibliometric analysis, 2023, 26, 1573-2975, 24151, 10.1007/s10668-023-03640-z | |
72. | Yadong Liu, Asma Salman, Kamran Khan, Ch Kamran Mahmood, Carlos Samuel Ramos-Meza, Vipin Jain, Malik Shahzad Shabbir, The effect of green energy production, green technological innovation, green international trade, on ecological footprints, 2023, 1387-585X, 10.1007/s10668-023-03399-3 | |
73. | José Rubio-León, José Rubio-Cienfuegos, Cristian Vidal-Silva, Jesennia Cárdenas-Cobo, Vannessa Duarte, Applying Fuzzy Time Series for Developing Forecasting Electricity Demand Models, 2023, 11, 2227-7390, 3667, 10.3390/math11173667 | |
74. | Ashfaq Ahmad, Muhammad Mobeen Shafqat, Muhammad Ilyas, Muhammad Umair Ashraf, Afshan Urooj, Zhao Yu huan, Validation of the environmental Kuznets curve and role of economic globalization: an aggregate and sectoral analysis of an Indian economy, 2023, 16, 1873-9318, 2035, 10.1007/s11869-023-01390-5 | |
75. | Chandrika Raghavendra, Rampilla Mahesh, Muhammad Kamran Khan, Vishal Dagar, Sanjeet Singh, Rafael Alvarado, Impact of carbon emissions, renewable and non-renewable energy consumption on inbound cross-border mergers and acquisition’s investment inflow, 2024, 1573-2975, 10.1007/s10668-024-04511-x | |
76. | Jiarui Tian, Kashif Raza Abbasi, Magdalena Radulescu, Mohammad Jaradat, Marinela Barbulescu, Reevaluating energy progress: An in-depth policy framework of energy, urbanization, and economic development, 2024, 191, 03014215, 114196, 10.1016/j.enpol.2024.114196 | |
77. | Arup Roy, Ranjan DasGupta, Economic Development, Energy Consumption, and Environmental Deterioration: A Non-Linear Evidence from India, 2024, 22, 0971-1554, 721, 10.1007/s40953-024-00405-x | |
78. | Soumya Basu, Keiichi Ishihara, A Cybernetic Delay Analysis of the Energy–Economy–Emission Nexus in India via a Bistage Operational Amplifier Network, 2024, 13, 2079-9292, 4434, 10.3390/electronics13224434 | |
79. | Subrata Saha, Mohammad Jahangir Alam, Al Amin Al Abbasi, Ismat Ara Begum, Maria Fay Rola‐Rubzen, Shifting Landscape of Rural Transformation in Bangladesh: Exploring the Interplay of Non‐Farm GDP, Infrastructure and Education, 2025, 12, 2050-2680, 10.1002/app5.70011 | |
80. | Ling Luo, Sanshan Zhao, Dan Wu, Lian Liu, Xingde Huang, 2024, Chapter 76, 978-3-031-78275-6, 779, 10.1007/978-3-031-78276-3_76 | |
81. | Soumya Basu, Keiichi Ishihara, On the resiliency of post-crisis decoupling in higher-order economy-energy-environment nexus in high-inflation developing economies, 2025, 0033-5177, 10.1007/s11135-024-01999-3 |
Author | Country (Period) | Variables | Methodology | Direction of Causality | |||
→ | ← | ↔ | ≠ | ||||
[45] | China (1971–2001) | EC, GDP | Granger Causality |
✔ | ✘ | ✘ | ✘ |
[37] | Pakistan (1972–2009) | EC, RGDP | ARDL, VECM | ✘ | ✔ | ✘ | ✘ |
[46] | Switzerland (1950–2010) | EC, RGDP | ARDL, UECM | ✘ | ✘ | ✔ | ✘ |
[46] | United Kingdom(1975–2010) | EC, RGDP | Panel bootstrap causality | ✔ | ✘ | ✔ | ✘ |
[46] | OECD(1990–2008) | EC, GDP | Panel Granger causality, | ✔ | ✘ | ✔ | ✘ |
[46] | Lebanon (1983–2014) | EC, GDP | Toda-Yamamoto | ✘ | ✘ | ✔ | ✘ |
[46] | Algeria (1971–2010) | EC, GDP | Cointegration, VECM | ✘ | ✘ | ✔ | ✘ |
[3] | Pakistan (1972–2010) | EC, RGDP | Granger Causality | ✔ | ✘ | ✘ | ✘ |
[47] | Poland (2000–2012) | EC, GDP | Granger causality | ✘ | ✘ | ✔ | ✘ |
[48] | Turkey (1970–2011) | EC, GDP | Granger causality | ✘ | ✘ | ✔ | ✘ |
[19] | Malaysia (2005–2010) | EC, RGDP | Multiplier Approach | ✔ | ✘ | ✘ | ✘ |
[24] | Algeria(1980–2012) | RE, NRE | Granger causality | ✔ | ✘ | ✔ | ✘ |
[49] | Taiwan (1998–2014) | EC, RGDP | Granger causality | ✘ | ✘ | ✔ | ✘ |
[50] | U.S.(2005–2015) | EC, RGDP | Wavelet | ✔ | ✘ | ✘ | ✘ |
[51] | Ghana (1971–2014) | EC, Industrial Growth | ARDL, ECM | ✘ | ✔ | ✘ | ✘ |
[52] | Pakistan(1981–2017) | RE, NRE | ARDL, VECM | ✘ | ✘ | ✔ | ✘ |
[53] | Pakistan (1970–2018) | EC, EP, GDP | Granger causality VECM | ✔ | ✘ | ✘ | ✘ |
Note: →, ←, ↔, and ≠ denotes unidirectional bidirectional and no causality, respectively. |
Industrial Variables | Philips-Perron (PP) | Augmented Dickey-Fuller (ADF) | Order of Integration | ||
Levels | First difference | Levels | First difference | ||
EC | (0.32) | (0.00)* | (0.35) | (0.00)* | I(1) |
EP | (0.49) | (0.00)* | (0.51) | (0.00)* | I(1) |
GDP | (0.31) | (0.00)* | (0.29) | (0.00)* | I(1) |
Notes: *, ** asterisk specifies significance at 1% and 5% level, respectively. p-value denotes in () parentheses. |
Hypothesized | r = 0 | r ≤ 1 | r ≤ 2 |
Trace Statistics | 35.40 | 12.83 | 3.29 |
P-Value | (0.01)* | (0.003)* | (0.12) |
Max-Eigen Statistics | 22.57 | 9.54 | 3.33 |
P-Value | (0.03)* | (0.002)* | (0.07) |
Notes: The numbers in the parentheses are p-values, r: indicates the number of co-integration hypothesis relationships, *, ** asterisk implies significance at 5% and 10% level, correspondingly. |
Industrial Sector | VECM (Long-run relationship effects) | ECT(t-Stats) | |||||
Coefficient(p-value) | Standard Error | Coefficient(p-value) | Standard error | Coefficient (p-value) | Standard error | ||
ΔEC | 1 | - | −0.19 | −0.39 | 0.15 | −0.21 | (−2.66)* |
(0.03)* | (0.01)* | ||||||
ΔEP | −0.13 | −0.12 | 1 | - | 0.05 | −0.12 | (−3.97)** |
(0.04)* | (0.12) | ||||||
ΔGDP | 0.09 | −0.14 | −0.16 | −0.24 | 1 | - | (−3.36)* |
(0.001)* | (0.00)** | ||||||
Notes: The number in the parentheses is the p-value. *, ** asterisks indicate significance at 5% and 1% level, respectively. |
Period | Variance Decomposition of EC | |||
S.E. | EC | EP | GDP | |
1 | 0.026154 | 100 | 0 | 0 |
2 | 0.040085 | 96.92866 | 2.657988 | 0.41335 |
3 | 0.055235 | 96.07497 | 3.657699 | 0.267331 |
4 | 0.066524 | 95.30433 | 4.487052 | 0.208615 |
5 | 0.076296 | 93.71978 | 6.059193 | 0.221029 |
6 | 0.084754 | 91.77491 | 7.982465 | 0.242629 |
7 | 0.092929 | 89.84477 | 9.908605 | 0.246629 |
8 | 0.100791 | 87.9735 | 11.77734 | 0.249163 |
9 | 0.108377 | 86.54688 | 13.1834 | 0.269722 |
10 | 0.11552 | 85.69038 | 13.99968 | 0.309941 |
Period | Variance Decomposition of EP: | |||
S.E. | EC | EP | GDP | |
1 | 0.048996 | 56.37411 | 43.62589 | 0 |
2 | 0.080503 | 47.46051 | 52.45839 | 0.081095 |
3 | 0.111427 | 54.85629 | 44.96984 | 0.173865 |
4 | 0.136671 | 58.00428 | 41.5015 | 0.49422 |
5 | 0.158198 | 61.49309 | 36.99908 | 1.507833 |
6 | 0.17542 | 65.03976 | 32.20885 | 2.751394 |
7 | 0.190837 | 68.51778 | 27.79294 | 3.689283 |
8 | 0.20503 | 71.39459 | 24.14619 | 4.459221 |
9 | 0.218298 | 73.62662 | 21.30353 | 5.069856 |
10 | 0.23014 | 75.36009 | 19.18527 | 5.454636 |
Period | Variance Decomposition of GDP: | |||
S.E. | EC | EP | GDP | |
1 | 0.027913 | 7.08547 | 1.074455 | 91.84007 |
2 | 0.047862 | 2.562744 | 1.192117 | 96.24514 |
3 | 0.058702 | 1.780112 | 2.351816 | 95.86807 |
4 | 0.067107 | 2.679764 | 3.801723 | 93.51851 |
5 | 0.076742 | 4.208902 | 5.118915 | 90.67218 |
6 | 0.086717 | 4.775009 | 6.861578 | 88.36341 |
7 | 0.095723 | 5.065867 | 8.767336 | 86.1668 |
8 | 0.103643 | 5.248982 | 10.21453 | 84.53649 |
9 | 0.110786 | 5.330424 | 11.07375 | 83.59582 |
10 | 0.117053 | 5.316104 | 11.42037 | 83.26352 |
Author | Country (Period) | Variables | Methodology | Direction of Causality | |||
→ | ← | ↔ | ≠ | ||||
[45] | China (1971–2001) | EC, GDP | Granger Causality |
✔ | ✘ | ✘ | ✘ |
[37] | Pakistan (1972–2009) | EC, RGDP | ARDL, VECM | ✘ | ✔ | ✘ | ✘ |
[46] | Switzerland (1950–2010) | EC, RGDP | ARDL, UECM | ✘ | ✘ | ✔ | ✘ |
[46] | United Kingdom(1975–2010) | EC, RGDP | Panel bootstrap causality | ✔ | ✘ | ✔ | ✘ |
[46] | OECD(1990–2008) | EC, GDP | Panel Granger causality, | ✔ | ✘ | ✔ | ✘ |
[46] | Lebanon (1983–2014) | EC, GDP | Toda-Yamamoto | ✘ | ✘ | ✔ | ✘ |
[46] | Algeria (1971–2010) | EC, GDP | Cointegration, VECM | ✘ | ✘ | ✔ | ✘ |
[3] | Pakistan (1972–2010) | EC, RGDP | Granger Causality | ✔ | ✘ | ✘ | ✘ |
[47] | Poland (2000–2012) | EC, GDP | Granger causality | ✘ | ✘ | ✔ | ✘ |
[48] | Turkey (1970–2011) | EC, GDP | Granger causality | ✘ | ✘ | ✔ | ✘ |
[19] | Malaysia (2005–2010) | EC, RGDP | Multiplier Approach | ✔ | ✘ | ✘ | ✘ |
[24] | Algeria(1980–2012) | RE, NRE | Granger causality | ✔ | ✘ | ✔ | ✘ |
[49] | Taiwan (1998–2014) | EC, RGDP | Granger causality | ✘ | ✘ | ✔ | ✘ |
[50] | U.S.(2005–2015) | EC, RGDP | Wavelet | ✔ | ✘ | ✘ | ✘ |
[51] | Ghana (1971–2014) | EC, Industrial Growth | ARDL, ECM | ✘ | ✔ | ✘ | ✘ |
[52] | Pakistan(1981–2017) | RE, NRE | ARDL, VECM | ✘ | ✘ | ✔ | ✘ |
[53] | Pakistan (1970–2018) | EC, EP, GDP | Granger causality VECM | ✔ | ✘ | ✘ | ✘ |
Note: →, ←, ↔, and ≠ denotes unidirectional bidirectional and no causality, respectively. |
Variables | Data Source | Scale Unit |
Industrial electricity consumption | [54] | Gigawatt hours (GWh) |
Electricity prices | [55] | Millions |
Real gross domestic product | [56] | $Millions |
Industrial Variables | Philips-Perron (PP) | Augmented Dickey-Fuller (ADF) | Order of Integration | ||
Levels | First difference | Levels | First difference | ||
EC | (0.32) | (0.00)* | (0.35) | (0.00)* | I(1) |
EP | (0.49) | (0.00)* | (0.51) | (0.00)* | I(1) |
GDP | (0.31) | (0.00)* | (0.29) | (0.00)* | I(1) |
Notes: *, ** asterisk specifies significance at 1% and 5% level, respectively. p-value denotes in () parentheses. |
Hypothesized | r = 0 | r ≤ 1 | r ≤ 2 |
Trace Statistics | 35.40 | 12.83 | 3.29 |
P-Value | (0.01)* | (0.003)* | (0.12) |
Max-Eigen Statistics | 22.57 | 9.54 | 3.33 |
P-Value | (0.03)* | (0.002)* | (0.07) |
Notes: The numbers in the parentheses are p-values, r: indicates the number of co-integration hypothesis relationships, *, ** asterisk implies significance at 5% and 10% level, correspondingly. |
Industrial Sector | VECM (Long-run relationship effects) | ECT(t-Stats) | |||||
Coefficient(p-value) | Standard Error | Coefficient(p-value) | Standard error | Coefficient (p-value) | Standard error | ||
ΔEC | 1 | - | −0.19 | −0.39 | 0.15 | −0.21 | (−2.66)* |
(0.03)* | (0.01)* | ||||||
ΔEP | −0.13 | −0.12 | 1 | - | 0.05 | −0.12 | (−3.97)** |
(0.04)* | (0.12) | ||||||
ΔGDP | 0.09 | −0.14 | −0.16 | −0.24 | 1 | - | (−3.36)* |
(0.001)* | (0.00)** | ||||||
Notes: The number in the parentheses is the p-value. *, ** asterisks indicate significance at 5% and 1% level, respectively. |
Period | Variance Decomposition of EC | |||
S.E. | EC | EP | GDP | |
1 | 0.026154 | 100 | 0 | 0 |
2 | 0.040085 | 96.92866 | 2.657988 | 0.41335 |
3 | 0.055235 | 96.07497 | 3.657699 | 0.267331 |
4 | 0.066524 | 95.30433 | 4.487052 | 0.208615 |
5 | 0.076296 | 93.71978 | 6.059193 | 0.221029 |
6 | 0.084754 | 91.77491 | 7.982465 | 0.242629 |
7 | 0.092929 | 89.84477 | 9.908605 | 0.246629 |
8 | 0.100791 | 87.9735 | 11.77734 | 0.249163 |
9 | 0.108377 | 86.54688 | 13.1834 | 0.269722 |
10 | 0.11552 | 85.69038 | 13.99968 | 0.309941 |
Period | Variance Decomposition of EP: | |||
S.E. | EC | EP | GDP | |
1 | 0.048996 | 56.37411 | 43.62589 | 0 |
2 | 0.080503 | 47.46051 | 52.45839 | 0.081095 |
3 | 0.111427 | 54.85629 | 44.96984 | 0.173865 |
4 | 0.136671 | 58.00428 | 41.5015 | 0.49422 |
5 | 0.158198 | 61.49309 | 36.99908 | 1.507833 |
6 | 0.17542 | 65.03976 | 32.20885 | 2.751394 |
7 | 0.190837 | 68.51778 | 27.79294 | 3.689283 |
8 | 0.20503 | 71.39459 | 24.14619 | 4.459221 |
9 | 0.218298 | 73.62662 | 21.30353 | 5.069856 |
10 | 0.23014 | 75.36009 | 19.18527 | 5.454636 |
Period | Variance Decomposition of GDP: | |||
S.E. | EC | EP | GDP | |
1 | 0.027913 | 7.08547 | 1.074455 | 91.84007 |
2 | 0.047862 | 2.562744 | 1.192117 | 96.24514 |
3 | 0.058702 | 1.780112 | 2.351816 | 95.86807 |
4 | 0.067107 | 2.679764 | 3.801723 | 93.51851 |
5 | 0.076742 | 4.208902 | 5.118915 | 90.67218 |
6 | 0.086717 | 4.775009 | 6.861578 | 88.36341 |
7 | 0.095723 | 5.065867 | 8.767336 | 86.1668 |
8 | 0.103643 | 5.248982 | 10.21453 | 84.53649 |
9 | 0.110786 | 5.330424 | 11.07375 | 83.59582 |
10 | 0.117053 | 5.316104 | 11.42037 | 83.26352 |