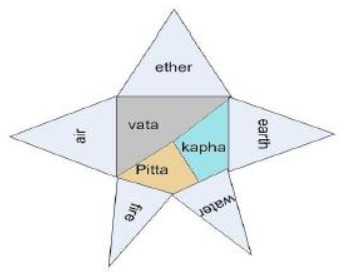
Pulse diagnosis, also known as Nadi Pariksha, is one of the various diagnostic modalities used in Ayurveda. Nadi Pariksha is a way of determining the underlying cause of a sickness that needs extensive knowledge of the Tridosha signals (i.e. Vata, Pitta and Kapha), as well as the peculiarities of each pulse signal and their relationship to each dominant signal. A Nadi expert can gain a sense of the patient's health status by using this approach and then provide treatment based on that information. In the present day, the health monitoring of people has become an essential requirement. A system which keeps track of the patient's health and continuously captures pulse signals will be helpful. In this work a healthcare monitoring system that uses sensors was developed, and the analysis of Vata, Pitta and Kapha for various patients is discussed, as well as the uploading of the same data to a self-made IoT cloud. The mean values of Vata, Pita and Kapha were compared for different age groups; we found that it is more significant for the age group of 41‒50.
Citation: Sanjay Dubey, M. C. Chinnaiah, I. A. Pasha, K. Sai Prasanna, V. Praveen Kumar, R. Abhilash. An IoT based Ayurvedic approach for real time healthcare monitoring[J]. AIMS Electronics and Electrical Engineering, 2022, 6(3): 329-344. doi: 10.3934/electreng.2022020
[1] | Mostafa A.R. Eltokhy, Mohamed Abdel-Hady, Ayman Haggag, Hisham A. Hamad, Tarek Hosny, Ahmed A. F. Youssef, Ali M. El-Rifaie . An indoor IoT-based LiFi system using LEDs for sensor data transfer. AIMS Electronics and Electrical Engineering, 2025, 9(2): 118-138. doi: 10.3934/electreng.2025007 |
[2] | Lawrence O. Aghenta, M. Tariq Iqbal . Design and implementation of a low-cost, open source IoT-based SCADA system using ESP32 with OLED, ThingsBoard and MQTT protocol. AIMS Electronics and Electrical Engineering, 2020, 4(1): 57-86. doi: 10.3934/ElectrEng.2020.1.57 |
[3] | Cherechi Ndukwe, M. Tariq Iqbal, Xiaodong Liang, Jahangir Khan, Lawrence Aghenta . LoRa-based communication system for data transfer in microgrids. AIMS Electronics and Electrical Engineering, 2020, 4(3): 303-325. doi: 10.3934/ElectrEng.2020.3.303 |
[4] | Edwin Collado, Euribiel Valdés, Antony García, Yessica Sáez . Design and implementation of a low-cost IoT-based agroclimatic monitoring system for greenhouses. AIMS Electronics and Electrical Engineering, 2021, 5(4): 251-283. doi: 10.3934/electreng.2021014 |
[5] | B Naresh Kumar, Jai Sukh Paul Singh . Intelligence-based optimized cognitive radio routing for medical data transmission using IoT. AIMS Electronics and Electrical Engineering, 2022, 6(3): 223-246. doi: 10.3934/electreng.2022014 |
[6] | Efe Francis Orumwense, Khaled Abo-Al-Ez . Internet of Things for smart energy systems: A review on its applications, challenges and future trends. AIMS Electronics and Electrical Engineering, 2023, 7(1): 50-74. doi: 10.3934/electreng.2023004 |
[7] | K.V. Dhana Lakshmi, P.K. Panigrahi, Ravi kumar Goli . Machine learning assessment of IoT managed microgrid protection in existence of SVC using wavelet methodology. AIMS Electronics and Electrical Engineering, 2022, 6(4): 370-384. doi: 10.3934/electreng.2022022 |
[8] | Minglong Zhang, Iek Cheong Lam, Arun Kumar, Kin Kee Chow, Peter Han Joo Chong . Optical environmental sensing in wireless smart meter network. AIMS Electronics and Electrical Engineering, 2018, 2(3): 103-116. doi: 10.3934/ElectrEng.2018.3.103 |
[9] | Abdelkrim Abanay, Lhoussaine Masmoudi, Mohamed El Ansari, Javier Gonzalez-Jimenez, Francisco-Angel Moreno . LIDAR-based autonomous navigation method for an agricultural mobile robot in strawberry greenhouse: AgriEco Robot. AIMS Electronics and Electrical Engineering, 2022, 6(3): 317-328. doi: 10.3934/electreng.2022019 |
[10] | Abhijeet Kumar, Arpit Kumar . Contactless temperature and distance measuring device: A low-cost, novel infrared ―based "Badge"-shaped structural model for measuring physical distance and body temperature. AIMS Electronics and Electrical Engineering, 2022, 6(1): 43-60. doi: 10.3934/electreng.2022004 |
Pulse diagnosis, also known as Nadi Pariksha, is one of the various diagnostic modalities used in Ayurveda. Nadi Pariksha is a way of determining the underlying cause of a sickness that needs extensive knowledge of the Tridosha signals (i.e. Vata, Pitta and Kapha), as well as the peculiarities of each pulse signal and their relationship to each dominant signal. A Nadi expert can gain a sense of the patient's health status by using this approach and then provide treatment based on that information. In the present day, the health monitoring of people has become an essential requirement. A system which keeps track of the patient's health and continuously captures pulse signals will be helpful. In this work a healthcare monitoring system that uses sensors was developed, and the analysis of Vata, Pitta and Kapha for various patients is discussed, as well as the uploading of the same data to a self-made IoT cloud. The mean values of Vata, Pita and Kapha were compared for different age groups; we found that it is more significant for the age group of 41‒50.
Ayurveda is a method of determining the fundamental cause of any problem. In Ayurveda, health is defined differently than in the biomedical concept. Ayurveda defines health as the balance of biological entities (dosha), digestive fluids, enzymes, hormones (agni), body tissues (dhatu) and normal waste excretion (mala), as well as a pleasant condition of the soul (atma), sensory and motor organs (indriya) and mind (manas). Some unhealthy factors, such as irregular food, stress and a weather change, tend to disrupt this equilibrium. As a result, even in a reasonably "healthy" patient, the doctor can detect small subclinical changes in the dosha [1].
The Ayurvedic Panchamahabhootas system is made up of five elements: Vayu, Jala, Aakash, Prithvi and Teja. Ether (space), Fire, Water, Air and Earth are the translations for these elements.
Five elements, according to Ayurveda, have a corresponding sensory signal:
Smell = Earth
Taste = Water
Sight = Fire
Touch = Air
Hearing = Space [2]
The Vata doshas, which act on the principles of Air, are formed by the conjunction of Ether and Air. The Pitta doshas, which act on the principle of Fire, are formed by the union of Fire and Water. The Kapha doshas, which act on the principle of Water, are formed by the union of Earth and Water Pranavayu, Udanavayu, Samanavayu, Vyanavayu and Apana are the five sub-doshas of the Vata doshas. Pachak, Alochaka, Ranjaka, Sadhaka, Bhrajaka, and Bhrajaka are the five sub-doshas of the Pitta dosha, while Bodhaka, Kledaka, Shleshaka, Tarpaka and Avalambaka are the five sub-doshas of the Kapha dosha. The Vata signal has a higher amplitude than Pitta and Kapha. Vata Pitta and Kapha have frequency ranges of 80‒95 Hz, 70‒80 Hz and 55‒70 Hz, respectively [3].
Nadi Pariksha (pulse diagnosis) is the most convenient, noninvasive, painless, affordable and side effect-free of the eight diagnosis procedures.
Tridoshas are felt with the index, middle and ring fingers, in those order. The positions of Vata, Pitta and Kapha are identified on the thumb radial artery on the wrist, which is used to assess a person's health. Despite the fact that the pulse signals are controlled by the heart, they are carried by blood flow though arteries.
Nutrients are delivered on the molecular level through an uninterrupted flow of communication between cells and blood through arteries [4]. The Vata pulse signal has a snake-like shape, the Pitta pulse signal has a frog-like shape and the Kapha pulse signal has a swarn-like shape [5]
On the left wrist of the female and the right wrist of the male, the Nadi Pariksha is performed. catabolism, metabolism and anabolism are the biological terms for Vata, Pitta and Kapha, respectively. Catabolism is the process of breaking down bigger molecules into smaller molecules in the digestive system. Metabolism is defined as the production of the chemical compounds necessary for the digestion of internal substances. Anabolism energy is stored in living organisms by the creation of complex molecules from simpler ones.
If the Vata, Pitta and Kapha do not have the right balance, the body will be affected by diseases or the subject will suffer from health problems such as rheumatoid arthritis, constipation, diarrhea and asthma. When the Vata, Pitta and Kapha energies are balanced, the subject is said to be healthy.
In order to improve the quality of human life by identifying disease at the correct moment and monitoring the patient, the design and development of remote patient monitoring systems is critical in the current industry. Many researchers prefer to use noninvasive approaches to monitor the patient's status in real time with a wearable low-cost real-time pulse monitoring system.
The scientific research of Ayurvedic science and technologies involved in the pulse acquisition system and the suggested tasks are explained in Section 3. The results and conclusions are discussed in Section 4.
Among everyone's busy daily schedules, healthcare is the biggest concern; it is an important perspective that the disease should be identified early by diagnosing properly; delay in the identification of the disease leads to major problems and makes the diagnosis process difficult. It requires extreme knowledge of the humans; since pulse values are different for each individual the proposed model is used to observe the physiological states of the human body.
According to Anuroopadevi et al., the human pulse can be collected using an XD58C sensor, which includes a noise reduction circuit. The sensor is connected to the MyRIO, where the MyRIO is a real-time embedded board manufactured by National Instruments that includes an FPGA and a microprocessor and requires LabVIEW software. This receives a pulse signal as input. The human pulse is displayed on the monitor during programming in LabVIEW. There are various periods of life, and the doshas alter with each stage. Children (0‒16 years old) are controlled by the Kapha dosha, adults (16‒50 years old) are affected by Pitta dosha disturbances and elders (above 50 years old) are affected by Kapha dosha disturbances [6].
People with the Vata dosha are creative, fast at comprehending information, and also quick to forget it. Headaches, hypertension, anxiety, dry cough, sore throat, earaches, sleeplessness, abdominal gas, diarrhea, abnormal heart rhythms, nervous stomach, constipation, muscular spasms, lower back discomfort, arthritis and nervous system issues are common among Vata people.
People with the Pitta dosha might be assertive, self-assured, and Pitta people frequently have heartburn, ulcers and burning in the stomach or intestines.
Bowels, sleeplessness, rashes or swelling on the skin, skin cancer, anemia, gall bladder, problems and liver disease are some of the conditions that can affect the people. Similarly, those with the Kapha dosha have a moderate learning style but a good long-term memory. Obesity, allergies, colds, respiratory issues, atherosclerosis, congestion, and sinus pain affect Kapha persons [6].
According to Kalange and Gangal, Subject a was found to be Vata dominant, Subject b was found to be Pitta dominant and Subject c was found to be Kapha dominant. Vata, Pitta and Kapha pulse data on other subjects were taken. The pulse repetition frequency for Vata dominated persons was found to be in the range of 1.35 Hz to 1.58 Hz, where it was 1.16 Hz to 1.33 Hz for Pitta dominated persons, and 0.833 Hz to 1.2 Hz for Kapha dominated persons [7].
Pavana MG, Shashikala N, Joshi stated that the amplitude of the three pulse signals in healthy individuals are in the ration of 4:2:1, and that this ratio may change based on the environment's temperature, humidity and time.
The frequency and amplitude spectra were applied to the signal collected from the pulse sensor. The acquired noise signal was passed through the filter using LabVIEW, and spectral analysis was performed on 10 subjects. A Vata signal with a cutoff frequency of 60 Hz was required, which is a high-pass filter, and a Kapha signal with a cutoff frequency of 80 Hz was required, which is a low-pass filter [8].
The Nadi Pariksha Yantra, in which three Vata, Pitta, and Kapha pressure points are obtained at the radial artery. Three pressure sensors with a diameter of 10 mm were employed, with a gap of 6 mm between each sensor. Pressure sensors (MLT 1010) were placed on the wrist using Velcro tape. The data acquisition system was connected to the pressure sensors through coaxial cables. The pulse data gathered using three channels was shown on the PC. Nadi-Parikshan Yantra was used to collect pulse data from a variety of patients, and the data were examined in terms of frequency and time, as explained by Kalange et al. [9].
Applying more pressure to the epidermis increased the radial pulse amplitudes, particularly the harmonics. When the pressure exerted exceeded a particular level, the pulse amplitudes and wave velocities all dropped as per Yoon et al. [10]. The two test series of pressure transducer arrays and their prototypes based on EMFi were suggested by Sorvoja et al. [11].
Chaudhari et al. suggested work on Nadi Pariksha, in where the system used a photoplethysmography (PPG) sensor, and the difference between the incident and reflected light was used to calculate the signal at specific time intervals [12]. The PPG heart rate sensor was connected to the NODE MCU ESP8266 module. The sensor output was in analogue format, and it was amplified and filtered before being fed into the NODEMCU's ADC0 PIN. The integrated WIFI (Wireless Fidelity) on the ESP 8266 allowed it to connect to a wireless router. On the phone, raw data, BPM (Beats per Minute) and IBI (Ischemic Brain Infarction) were observed. When the signal processing and the graphical user interface are finished, it can be extended to new devices by using Java Script and HTML [13].
According to Khaire NN, Joshi YV, the pulse signal microphone was employed for sensing. The signal collected from the microphone had a millivolt range, which is extremely low. In order to gain a better signal, the signal was amplified using a quad op-amp IC LM 324 amplifier, and the frequency of the pulse signal ranged from 0.7 Hz to 12 Hz; a second-order low-pass filter with a cut off frequency of 12.12 Hz was utilized.
The amplifier output was connected to the National Instruments BNC-2120 (via) the DAQ card PCI-6281 and then to the computer to convert the signal from analog to digital. Simulink in MATLAB was used to obtain digital data. The digital signal, which contains high-frequency noise, was retrieved from the DAQ. In MATLAB [14], a low pass filter was designed to eliminate noise.
As per Khandai SK, Jain SK, the pulse signal was acquired by using two types of sensors: a piezoelectric sensor and a piezoresistive pressure sensor. The pulse acquisition system was coupled to piezoelectric and piezoresistive pressure sensors. Sensors, signal conditioning circuits, and a digitizer were the three primary components of the system. The Muratas 7BB-15-6LO piezoelectric sensor had a diameter of 15 mm and a resonance frequency of 6 kHz. The analog output of the Piezoresistive pressure sensor of type MPX5010G6U was in the range of 0.2 to 4.7 V, the pressure range was 0 to 10 kPa and the sensitivity was 450 mV/ kPa [15].
The construction of a digital pulse diagnosis system and analysis of an oriental pulse waveform were presented by Lee et al. The data collected by the pulse diagnosis system were compared to the physician's clinical findings, and both yielded equivalent results. By using a pulse wave signal as an input, this system performed the necessary signal processing for the recognition method, filtered the signals to remove noise, and extracted the diagnostic parameter. Finally, the signals were identified and evaluated. The size of the amplitude was estimated for several waveforms as part of the analysis. The amplitude size range for several waveforms was found to be 10.08‒22.41 mm [16].
The radial artery pulse can be used to assess pain. A differential mode pressure sensor based on a strain gauge was placed on the wrist to sense the pressure imposed by the radial artery. The amplitudes of percussion and dicrotic waves were used to assess the collected pulse signals. The dominance of doshas was validated based on those waves, and a pain treatment strategy was advised [17].
The fingertip detects Nadi pulses, which are actually pressure measurements delivered by the artery. Because the number of pulse beats in pressure units is so small, obtaining data is particularly challenging. These pulses were recorded with a 1 mV output medium pressure Sensor and processed with a DAQ card [18].
Measurement and analyses of the arterial pulse waveform (APW) to help with cardiovascular risks was explained by Pereira et al. For the APW assessment, optical sensors were employed, which did not come into contact with the skin and allowed for the detection of skin surface displacement. After labeling and filtering the noisy data, 37 features were retrieved from a dataset of 213 patients. Amplitude, time, frequency, wavelet, and cross-correlation characteristics were among the subgroups of the features collected. K-nearest neighbors and support vector machine (SVM) methods were used to classify the extracted features. SVM feature elimination was used to identify the most important feature. The best results came from the SVM classifier. By incorporating features from multiple domains and also by employing feature extraction in arterial waveform by applying machine learning algorithms, this approach of classifying the collected data provides promising methods to recognize the APW and assists the patient diagnosis system [19].
As Rao and Rao proposed that wrist pulse assessment could be extended for blood pressure monitoring. Wrist pulses were collected by using piezoelectric film sensors (MEAS-LDT0-028 K) near the radial artery, and wave properties such as spatial pulse transit Time (SPTT) and spatial pulse wave velocity (SPWV) were retrieved. The signal conditioning circuit amplified and filtered the signals generated by the sensors, and the signals were then analyzed in LabVIEW utilizing the USB 6003DAQ module. The SPTT and SPWV acquired from the wrist pulse were used to predict blood pressure. To determine the link between the SPTT and BP, the human subject correlation coefficient was determined. The use of an empirical model to calculate the SBP and DBP resulted in a 35 mmHg inaccuracy. The Hagen-Poiseuilli equation has been shown to be more effective in calculating mean artery pressure (MAP) in the radial artery. To validate the BP monitoring equipment, the MAP readings were compared to the clinical readings. The MAP readings were varied between 4.5 and 2.3 mmHg [20].
Health is an important concern in everyone's life. Sensors were implanted on the patient's body to detect their temperature and heartbeat. Two extra sensors were set at the home to sense the humidity and temperature of the room where the patient was staying. When all four sensors were linked to the control unit, the values of these four sensors were calculated. Because the readings must be accessed by the doctor from any location, the sensors' data is sent to the base station via the IoT cloud. Now, based on the temperature and heartbeat measurements of the patients, as well as the room, the doctor can determine their condition and take appropriate action [21]. A device called Nadi Tarangini is already on the market, the system also generates a report based on the pulse signals variation and it can be viewed by the Nadi Vaidya on the Nadi Tarangini app [25].
Das and Namasudra stated that the security of healthcare data and privacy of the users are major concerns in IoT enabled health-care system. To protect the data there are two major categories of encryption they are symmetric and asymmetric cryptographic techniques. For encrypting the data of health-care AES symmetric, serpent symmetric and elliptic curve cryptography (ECC) based asymmetric encryption schemes were used for enhancing the security of the healthcare data [26].
In [27], Gupta and Namasudra proposed that virtual machines (VMs) are virtualized versions of computers that can do practically any computer-related functions in a cloud computing environment. Moving VMs from one physical machine to another is referred to as VM migration. The suggested approach takes into account the host selection migration (HSMT). VM reallocation migration time (VMRMT), and VM reallocation bandwidth use three primary techniques. By reducing the migration time, these strategies help to improve the performance of cloud computing system.
According to Das S, Namasudra S, ciphertext policy attribute-based encryption (CP-ABE) technology is frequently used in an IoT-based healthcare system to encrypt patient healthcare data in order to retain confidentiality and accomplish fine-grained access control. The attribute revocation problem is solved by the novel CP-ABE-based fine-grained access control mechanism proposed in there paper. To reduce the work overhead associated with having a single authority in the conventional CP-ABE systems, the suggested technique comprises several attribute authorities. In order to decrease end-user decryption overhead, the suggested approach also outsources the decryption operation to a decryption helper entity [28].
Kumar et al. stated that recently, distributed electric power transmission frameworks have drawn attention to smart grid (SG) communication. These device's data security and validation features, which also include lightweight operation with simple computations, significantly contribute to the SG technologies. In this study, an ECC-based validation method was suggested for safe guarding the SG. This procedures formal verification was carried out using the ProVerif tool and Burrows-Abadi-Needham logic (BAN logic), which confirmed its security in the presence of potential intruder [29].
In [30], Bawankar et al. stated that all details of the patients had been registered, and following registering, the patient's wrist was fitted with pulse sensors, which were utilized to capture the patient's pulse signal. After the data collection, the data were safely fetched for cloud preprocessing, and after preprocessing the classification algorithms were used to validate.
Kashyap and Jain have proposed a new diagnostic system to study the effect of gender on the wrist pulse, The diagnostic system helps in identifying different diseases through the detection of pulses [31].
This section discussed the scientific study of Ayurvedic science, the pulse acquisition system methodologies and the basic characteristics of the three doshas (Vata, Pitta and Kapha). The types of sensors utilized for pulse acquisition, as well as the intermediate devices required for pulse signal processing, have been discussed. The proposed research method is discussed in the following section.
Three optical pulse sensors, a CC3200 Launchpad XL, a personal computer, and a developed IoT cloud make up the proposed system. Three optical pulse sensors (SEN11574) were used to collect three pulse signals: Vata, Pitta and Kapha, which were captured by the CC3200 Launchpad XL. The obtained signals were amplified and transferred to the created IoT server using an Energia program. The data collected were saved on a personal computer for additional processing and analysis. Figures 3 and 6 illustrate a block diagram of the pulse acquisition system and snapshots of the subjects' data acquisition.
The following steps were involved in developing the pulse acquisition system for healthy and unhealthy persons from the different age groups including both males and females.
Step 1: In order to get the noise free signals, pulse sensors (SEN115754) were used for the pulse acquisitions.
Step 2: To acquire the signals with no delay, sensors were interfaced with the CC3200 Launchpad XL board to acquire and process the same signals.
Step 3: Preprocessing and analyzing the acquired data.
Step 4: Analyzed data were stored to the cloud (database) for patient health records and data were sent to doctors for the remote health monitoring system.
At different sessions, the studies were carried out on different people based on their age group, which was likely 21‒30 years, 31‒40 years, and more than 41 years. The data of 40 subjects, both males and females, from each age group were recorded, evaluated, and compared. All of the participants were mentally stable and in good health. All of the subjects were explicitly informed about the goal of the research and the experimental protocol, and then an informed permission form was obtained from each of them, which was duly signed by the subjects.
The sensor had two sides, one with the design soldered on the sensor and the other with the LED sensor. The LED sensor was positioned on the subject's finger/wrist over a blood vein. When the heart pumps the blood flow inside the radial artery, the light sensor senses the flow and bounces back the light; therefore the analysis was taken with respect to time and amplitude.
The Avago (APDS-9008) pulse sensor with an ambient light sensor, a diode for power line safety, and an active filter that brightens the pulse waveform and prevents backward propagation makes a significant difference. In the output waveform, a tiny sawtooth wave is supported by a DC voltage. The signal fluctuates as the blood flows through the tissues and the reflected light changes. When fresh blood flows from capillary tissues, an analogue signal from the pulse sensor rises or falls, depending on the subject's conditions.
A photodiode is generally employed as a source current in op-amp designs, and the source current is well-known as a starting point for building the Vata, Pitta and Kapha signal monitors. A well-known op-amp design is a current-to-voltage converter. For the output, a universal low-pass filter with an RC resistance of 100 and a capacitance of 4.7 µF was used. Ambient sensor light was utilized in the phones and laptops to change the LCD brightness, and this light was integrated in the photodiode/feedback network/op-amp.
The op-amp was included in the advanced CMOS microchip. With a 500 pF capacitive load and a 1 MHz gain bandwidth product, this family offers 90° phase margin and 45° phase margins. The ability of the dual-input, balanced-output differential amplifier's supply range to suppress unwanted disturbances that could be amplified together with the intended signal is an essential feature. When a matched pair of transistors is employed in a differential amplifier, undesirable signals such as 60 Hz noise or vibration may appear as common to both input bases, resulting in a theoretical net output of zero. The degree of matching between the two common-emitter stages that make up the differential amplifier determines the practical efficacy of rejecting the common signal. In other words, the stronger the common mode signal rejection, the closer the currents in the input transistors Q1 and Q2 are to being identical.
Although the pulse starts from the heart, the thickness and flexibility of the skin, the composition of blood, and other factors all impact the fluctuation. As a result, some of the healthy states that are linked to these characteristics show up in the pulse. Above the radial artery, the pulse sensor was positioned. The output was created with the aid of the TI-CC3200, and it showed a rapid rise in the percussion wave owing to the velocity of blood flows inside the radial artery. Blood flow is linked to the heartbeat, which has two peaks: systolic and diastolic. Systolic refers to the contraction of the heart that occurs as air is inhaled into the body. Diastolic refers to the heart's relaxation. The percussion wave (P), tidal wave (T), valley wave (V), and dicrotic wave (D) are some of the features of the arterial pulse signal.
The percussion wave's functionality shows a peak level, the tidal wave suggests a deep level, the valley wave represents an abrupt rise in level, and the dicrotic wave indicates a modest peak level in waveform. These properties must be identified on the wrist for pulse analysis, and features can also be retrieved from waveforms by using the first derivative [23], [24].
This research has been approved by the Institutional Ethics committee of BVRIT (IEC_BVRIT) and the persons have been explained clearly about the research that the data has been taken in a non-invasive approach and the consent form duly signed by each subject have been taken.
Experiments were carried out with a prototype that had been developed. To detect Vata, Pitta, and Kapha signals, optical pulse sensors were placed on the wrist in three distinct locations. On the radial artery, the pulse values generated by the optical pulse sensors are perceived in relation to time. For each individual, pulse data were collected for a period of 2 minutes at three separate times on the same day, namely, in the morning, afternoon and evening. The CC3200 Launchpad XL was then used to handle the pre-amplified pulse data. Pulse data from the CC3200 were kept on a personal computer linked via USB, and the same data were transferred to the cloud.
The Vata, Pitta and Kapha pulse signals were gathered at different times throughout the day, such as the morning, afternoon, and evening. Data were gathered in the morning session before a meal. Data samples from the morning session were collected between 9:00 and 10:00 am.
Afternoon data samples were obtained between 1:00 pm and 2:00 pm, i.e., one and a half hours after the participants had their meal. Data samples were obtained between 4:00 and 5:00 pm in the evening for last session. The Vata, Pitta and Kapha waveforms for Male Subject1 (Age: 32 years) in the morning, afternoon, and evening are shown in Figures 7‒9.
The collected pulse signals from the pulse sensors are shown in Figure 7. The acquired Vata signal appears to be moderate, the Pitta appears to be moderate, and the Kapha appears to be strong.
Figure 8 depicts a Vata signal that is moderate, a Pitta signal that is strong and a Kapha signal that is moderate.
Figure 9 demonstrates that the Vata signal appears to be strong, the Pitta appears to be moderate, and the Kapha appears to be moderate.
The collected signals were then subjected to peak detection analysis. The peaks of all samples, as well as their minimal peak distance, were recognized. The difference between identified peaks, mean values were then computed. The featured mean values differs for different doshas as well as distinct subjects. For Tridoshas, the mean of peak differences was computed as shown in Figures 10‒12.
All of the participants' Vata, Pitta and Kapha mean values were computed by using the same procedure.
For Subject 1: the Vata had a mean value of 4.300 mV, the Pitta has a mean value of 7.20 mV, and the Kapha had a mean value of 6.50 mV.
For Subject 2: the featured Vata mean value was 6 mV, the Pitta mean value was 9 mV, and the Kapha mean value was 11 mV.
For Subject 3: the featured Vata mean value was 6.5 mV, the Pitta mean value was 8.1 mV, and the Kapha mean value was 9.5 mV. The following table contains the mean of differences of Vata, Pitta, and Kapha values for all age groups.
Age group | Mean of peak difference for Vata (mV) | Mean of peak difference for Pitta (mV) | Mean of peak difference for Kapha (mV) |
21‒30 | 4.63‒6.5 | 6.6‒7.5 | 6.7‒7.79 |
31‒40 | 7.5‒8.19 | 7.5‒8.01 | 7.9‒8.65 |
41‒50 | 10.26‒11.05 | 9.88‒10.52 | 9.6‒10.32 |
51‒60 | 6.6‒7.31 | 6.5‒7.35 | 6.7‒6.98 |
According to the findings, for the age group 21‒30, the Vata values were between 4.63 and 6.5 mV, the Pitta values were 6.6‒7.5 mV, and the Kapha values were 6.7‒7.79 mV, with a difference for each age group.
For the same subject Subject 1, the area occupied for the sample pulse signal at the morning session was calculated as follows.
Vata pulse signal:
Area of occurred pulse signal = (Area of internal geometrical shapes of a signal)
F(x) = Area of Triangle 1 + Area of Triangle 2
F(x) = 72. 9 Sq.mt
Continuous time function of a cycle = 72.9 Sq.mt.
Similarly, for the same typical subject, the area of the occurred pulse signal of Pitta was found to be as follows:
F(x) = 62.75 Sq.mt for the Kapha pulse signal, it was F(x) = 92.875 Sq.mt.
Subject 1's frequency range for Vata was 1.321‒1.430 Hz, for Pitta was 1.265‒1.428 Hz and for Kapha was 1.328‒1.428 Hz. Subject 2's repetitive frequency range for Vata was 1.718‒1.811 Hz, for Pitta was 1.612‒1.945 Hz and Kapha was 1.718‒1.865 Hz.
These findings will be beneficial when the future for Nadi Vaidya in determining if the person is healthy or sick. A report will be created based on the numbers, and the same data will be communicated to the physicians through the IoT. The Nadi Vaidya receives the real-time pulse signals and sends them to a remote health monitoring system.
The data collected was kept in the IoT cloud. The table was first established in the IoT database with the appropriate number of tables. The table was then immediately filled with the uploaded information. Every one seconds, the IoT cloud was updated. We monitored real-time data through the cloud as data were being uploaded.
A physician monitored the relationship between Vata, Pitta and Kapha in real time, and the resulting reports were reviewed before therapy began.
The created IoT cloud has been used to upload real-time pulse signals. The Nadi Vaidya will recommend therapy based on signals, which will be analyzed and compared to old recorded data of individuals. As shown in Figure 13, the data collected from the pulse sensor linked to the CC3200 Launch pad can be transferred to the self-developed IoT cloud.
Using a designed pulse capture device, data were gathered from 40 participants from each age group (a total of 120 subjects) at various times such as the morning, afternoon, and evening. By comparing the amplitudes of Vata, Pitta, and Kapha signals with regard to time, dominant doshas can be determined. At each session, the behavior of the Vata, Pitta, and Kapha doshas were evaluated for three subjects and uploaded to the IoT cloud for Nadi Vaidhya consultation in order to track the subject's health. For two participants, the repetitive frequencies of the Vata, Pitta, and Kapha doshas were computed. As a result, the biological clock was used to match dominant doshas for the same subjects at various sessions. This work may be extended to expand the knowledge of the subjects' healthy and ill states.
We would like to thank the Department of Science and Technology, New Delhi, India for supporting this work under their Technology Interventions for Disabled and Elderly (TIDE) scheme, (Vide Ref. No. SEED/TIDE/048).
The authors have no conflict of interest regarding this study.
[1] |
Kurande V, Waagepetersen R, Toft E, et al. (2012) Repeatability of Pulse Diagnosis and Body Constitution Diagnosis in Traditional Indian Ayurveda Medicine. Global advances in health and medicine 1: 36–42. https://doi.org/10.7453/gahmj.2012.1.5.011 doi: 10.7453/gahmj.2012.1.5.011
![]() |
[2] |
Roopini N, Shivaram JM, Shridhar D (2015) Design & Development of a System for Nadi Pariksha. International Journal of Engineering Research Technology (IJERT) 4: 465‒470. http://dx.doi.org/10.17577/IJERTV4IS060509 doi: 10.15623/ijret.2015.0406080
![]() |
[3] | Narayanan C, Kumar AD, Priyadharshini S, et al. (2015) Cardiac Disorder Diagnosis through Nadi (Pulse) using Piezo Electric Sensors. International Journal of Multidisciplinary Research and Modern Education (IJMRME) 1: 209‒214. |
[4] | Lad V (2007) Secrets of the Pulse: The ancient art of Ayurvedic pulse diagnosis. 2 Eds., Delhi: Motilal Banarsidass Publishing House. |
[5] |
Navghare S, Bajaj P (2018) Design of Non-Invasive Pulse Rate Detector using LabVIEW. International Journal of Computer Applications 181: 19‒24. http://dx.doi.org/10.5120/ijca2018917811 doi: 10.5120/ijca2018917811
![]() |
[6] |
Anu S, Devi R, Keerthana R, et al. (2015) PC based Monitoring of Human Pulse Signal using LabVIEW. International Journal of Innovative Research in Electrical, Electronics, Instrumentation and Control Engineering 3: 186‒187. http://dx.doi.org/10.17148/IJIREEICE.2015.3344 doi: 10.17148/IJIREEICE.2015.3344
![]() |
[7] |
Kalange AE, Gangal SA (2007) Piezoelectric Sensor for Human Pulse Detection. Defence Sci J 57: 109‒114. https://doi.org/10.14429/dsj.57.1737 doi: 10.14429/dsj.57.1737
![]() |
[8] |
Pavana MG, Shashikala N, Joshi M (2016) Design, development and comparative performance analysis of Bessel and Butterworth filter for Nadi Pariksha Yantra. 2016 IEEE International Conference on Engineering and Technology (ICETECH), 1068‒1072. https://doi.org/10.1109/ICETECH.2016.7569413 doi: 10.1109/ICETECH.2016.7569413
![]() |
[9] |
Kalange AE, Mahale BP, Aghav ST, et al. (2012) Nadi Parikshan Yantra and analysis of radial pulse. 1st International Symposium on Physics and Technology of Sensors (ISPTS-1), 165‒168. https://doi.org/10.1109/ISPTS.2012.6260910 doi: 10.1109/ISPTS.2012.6260910
![]() |
[10] |
Yoon YZ, Lee MH, Soh KS (2000) Pulse type classification by varying contact pressure. IEEE Eng Med Biol 19: 106‒110. https://doi.org/10.1109/51.887253 doi: 10.1109/51.887253
![]() |
[11] |
Sorvoja H, Kokko VM, Myllyla R, et al. (2005) Use of EMFi as a blood pressure pulse transducer. IEEE Transactions on Instrumentation and Measurement 54: 2505‒2512. https://doi.org/10.1109/TIM.2005.853345 doi: 10.1109/TIM.2005.853345
![]() |
[12] |
Chaudhari S, Mudhalwadkar R (2017) Nadi pariksha system for health diagnosis. International Conference on Intelligent Computing and Control (I2C2), 1‒4. https://doi.org/10.1109/I2C2.2017.8321935 doi: 10.1109/I2C2.2017.8321935
![]() |
[13] |
Škraba A, Koložvari A, Kofjač D, et al. (2019) Prototype of Group Heart Rate Monitoring with ESP32. 8th Mediterranean Conference on Embedded Computing (MECO), 1‒4. https://doi.org/10.1109/MECO.2019.8760150 doi: 10.1109/MECO.2019.8760150
![]() |
[14] |
Khaire NN, Joshi YV (2015) Diagnosis of Disease Using Wrist Pulse Signal for classification of pre-meal and post-meal samples. International Conference on Industrial Instrumentation and Control (ICIC), 866‒869. https://doi.org/10.1109/IIC.2015.7150864 doi: 10.1109/IIC.2015.7150864
![]() |
[15] |
Khandai SK, Jain SK (2017) Comparison of sensors performance for the development of wrist pulse acquisition system. TENCON 2017 IEEE Region 10 Conference, 2870‒2875. https://doi.org/10.1109/TENCON.2017.8228351. doi: 10.1109/TENCON.2017.8228351
![]() |
[16] |
Lee J, Kim J, Lee M (2001) Design of Digital Hardware System for Pulse Signals. J Med Syst 25: 385–394. https://doi.org/10.1023/A:1011975727571 doi: 10.1023/A:1011975727571
![]() |
[17] | Krishnan S, Abudhahir A (2016) Development of system to acquire Radial Artery Pulse for Objective Pain Measurement. Biomedicine 36: 35‒40. |
[18] |
Joshi A, Kulkarni A, Chandran S, et al. (2007) Nadi Tarangini: A Pulse Based Diagnostic System. 29th Annual International Conference of the IEEE Engineering in Medicine and Biology Society, 2207‒2210. https://doi.org/10.1109/IEMBS.2007.4352762 doi: 10.1109/IEMBS.2007.4352762
![]() |
[19] |
Pereira T, Paiva JS, Correia C, et al. (2016) An automatic method for arterial pulse waveform recognition using KNN and SVM classifiers. Med Biol Eng Comput 54: 1049–1059. https://doi.org/10.1007/s11517-015-1393-5 doi: 10.1007/s11517-015-1393-5
![]() |
[20] |
Rao S, Rao R (2015) Investigation on pulse reading using flexible pressure sensor. International Conference on Industrial Instrumentation and Control (ICIC), 213‒216. https://doi.org/10.1109/IIC.2015.7150740 doi: 10.1109/IIC.2015.7150740
![]() |
[21] |
Valsalan P, Baomar TA, Baabood AH (2020) IOT Based Health Monitoring System. Journal of Critical Reviews 7: 739‒743. https://doi.org/10.31838/jcr.07.04.137 doi: 10.31838/jcr.07.04.137
![]() |
[22] | Murphy J, Gitman Y, Pulse Sensor. Open Hardware. Available from: https://pulsesensor.com/pages/open-hardware |
[23] |
Sareen M, Kumar M, Santhosh J, et al. (2009) Nadi Yantra: a robust system design to capture the signals from the radial artery for assessment of the autonomic nervous system non-invasively. Journal of Biomedical Science and Engineering 2: 471‒479. https://doi.org/10.4236/jbise.2009.27068 doi: 10.4236/jbise.2009.27068
![]() |
[24] | Thakker B, Vyas AL (2009) Outlier Pulse Detection and Feature Extraction for Wrist Pulse Analysis. International Journal of Biomedical and Biological Engineering 3: 127‒130. |
[25] | Highlights of Nadi Tarangini. Available from: https://www.naditarangini.com/nadi-tarangini-device/ |
[26] |
Das S, Namasudra S (2022) A novel hybrid encryption method to secure healthcare data in IoT-enabled healthcare infrastructure. Comput Electr Eng 101: 107991. https://doi.org/10.1016/j.compeleceng.2022.107991 doi: 10.1016/j.compeleceng.2022.107991
![]() |
[27] |
Gupta A, Namasudra S (2022) A novel technique for accelerating live migration in cloud computing, Automat Softw Eng 29: 1‒21. https://doi.org/10.1007/s10515-022-00332-2 doi: 10.1007/s10515-021-00310-0
![]() |
[28] |
Das S, Namasudra S (2022) MACPABE: Multi authority-based CP-ABE with efficient attribute revocation for IoT-enabled healthcare infrastructure. Int J Netw Manag, e2200. https://doi.org/10.1002/nem.2200 doi: 10.1002/nem.2200
![]() |
[29] |
Kumar A, Abhishek K, Namasudra S, et al. (2021) A novel elliptic curve cryptography based system for smart grid communication. Int J Web Grid Serv 17: 321‒342. https://doi.org/10.1504/IJWGS.2021.10040914 doi: 10.1504/IJWGS.2021.118398
![]() |
[30] | Bawankar BU, Dharmik RC, Telrandhe S (2021) Nadi pariksha: IOT-based patient monitoring and disease prediction system. InJournal of Physics: Conference Series 1913: 012124. IOP Publishing. https://doi.org/10.1088/1742-6596/1913/1/012124 |
[31] | Kashyap M, Jain S (2022) Importance of Pulse Examination and Its Diagnostic System. In Recent Innovations in Computing, 189‒199. Springer, Singapore. https://doi.org/10.1007/978-981-16-8248-3_16 |
1. | Mrunal Fatangare, Sukhada Bhingarkar, A comprehensive review on technological advancements for sensor-based Nadi Pariksha: An ancient Indian science for human health diagnosis, 2024, 15, 09759476, 100958, 10.1016/j.jaim.2024.100958 | |
2. | J Thamil Selvi, E Priya, V Dhanush Kumar, K Kishore, Sriram Santhosh Rajkumar, 2024, Non-Invasive Device to Predict Human Well Being from Wrist Radial Artery, 979-8-3503-5268-9, 1, 10.1109/IC3IoT60841.2024.10550317 | |
3. | Kuldeep Vayadande, Chudaman D. Sukte, Yogesh Bodhe, Tanishka Jagtap, Atharv Joshi, Palash Joshi, Arushi Kadam, Sai Kadam, Heart Disease Diagnosis and Diet Recommendation System Using Ayurvedic Dosha Analysis, 2024, 11, 2414-1399, 10.4108/eetiot.6016 | |
4. | Mrunal Fatangare, Sukhada Bhingarkar, Investigating an Efficient Filter to Implement Automated Nadi Pariksha by Analysing Time and Frequency Domain Features with Machine Learning Approach, 2025, 2582-1040, 223, 10.54392/irjmt25216 |
Age group | Mean of peak difference for Vata (mV) | Mean of peak difference for Pitta (mV) | Mean of peak difference for Kapha (mV) |
21‒30 | 4.63‒6.5 | 6.6‒7.5 | 6.7‒7.79 |
31‒40 | 7.5‒8.19 | 7.5‒8.01 | 7.9‒8.65 |
41‒50 | 10.26‒11.05 | 9.88‒10.52 | 9.6‒10.32 |
51‒60 | 6.6‒7.31 | 6.5‒7.35 | 6.7‒6.98 |