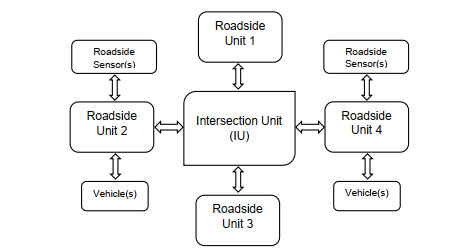
Reverse data delivery is one of the key operations in vehicular ad hoc networks which allows data delivery from roadside units (infrastructure) to vehicles. In the past, a number of data dissemination schemes have been proposed by researchers for the vehicular ad hoc networks to tackle the problem of data forwarding from vehicles and roadside units to data concentration points or Access Points (APs). In contrast, schemes for reverse data forwarding which allows a data concentration point to apprise the on-road vehicles about any eventuality or developing hazardous conditions are very few. This paper proposes a reverse data forwarding scheme which utilizes the characteristics of the road networks to inform passing vehicles of important information related to any accident, crash, or hazardous road conditions. The proposed scheme takes into account the geographical proximity factor by considering a localized sub-region/segment in the greater road network which is most adversely affected by the occurrence of some unfortunate road event. For its implementation, the scheme is dependent on DSRC-enabled roadside equipment to relay road-safety messages from AP to vehicles. In case if the passing vehicles are not fitted with DSRC on-board units, the scheme has the capability to directly interact with the variable-message signs on intersections with the objective of reaching as many vehicles as possible to increase the chances of message delivery. Simulation results using a small road segment confirm that the proposed scheme is more effective when the localized sub-region is smaller or in other words when more vehicles in the vicinity of the accident location are provided with pre-warning messages.
Citation: Farhan Khan, Sing Kiong Nguang. Location-based reverse data delivery between infrastructure and vehicles[J]. AIMS Electronics and Electrical Engineering, 2021, 5(2): 158-175. doi: 10.3934/electreng.2021009
[1] | Cherechi Ndukwe, M. Tariq Iqbal, Xiaodong Liang, Jahangir Khan, Lawrence Aghenta . LoRa-based communication system for data transfer in microgrids. AIMS Electronics and Electrical Engineering, 2020, 4(3): 303-325. doi: 10.3934/ElectrEng.2020.3.303 |
[2] | Efthymios N. Lallas . A survey on key roles of optical switching and labeling technologies on big data traffic of Data Centers and HPC environments. AIMS Electronics and Electrical Engineering, 2019, 3(3): 233-256. doi: 10.3934/ElectrEng.2019.3.233 |
[3] | Shahzad Ashraf, Arshad Ahmad, Adnan Yahya, Tauqeer Ahmed . Underwater routing protocols: Analysis of link selection challenges. AIMS Electronics and Electrical Engineering, 2020, 4(3): 234-248. doi: 10.3934/ElectrEng.2020.3.234 |
[4] | Boualem Djehiche, Alain Tcheukam, Hamidou Tembine . Mean-Field-Type Games in Engineering. AIMS Electronics and Electrical Engineering, 2017, 1(1): 18-73. doi: 10.3934/ElectrEng.2017.1.18 |
[5] | Farhan Khan, Sing Kiong Nguang . Dual sensing scheduling algorithm for WSN based road network surveillance. AIMS Electronics and Electrical Engineering, 2021, 5(1): 38-54. doi: 10.3934/electreng.2021003 |
[6] | Vuong Quang Phuoc, Nguyen Van Dien, Ho Duc Tam Linh, Nguyen Van Tuan, Nguyen Van Hieu, Le Thai Son, Nguyen Tan Hung . An optimized LSTM-based equalizer for 100 Gigabit/s-class short-range fiber-optic communications. AIMS Electronics and Electrical Engineering, 2024, 8(4): 404-419. doi: 10.3934/electreng.2024019 |
[7] | Asim Anwar, Boon-Chong Seet, Xue Jun Li . NOMA for V2X under similar channel conditions. AIMS Electronics and Electrical Engineering, 2018, 2(2): 48-58. doi: 10.3934/ElectrEng.2018.2.48 |
[8] | Deven Nahata, Kareem Othman . Exploring the challenges and opportunities of image processing and sensor fusion in autonomous vehicles: A comprehensive review. AIMS Electronics and Electrical Engineering, 2023, 7(4): 271-321. doi: 10.3934/electreng.2023016 |
[9] | B Naresh Kumar, Jai Sukh Paul Singh . Intelligence-based optimized cognitive radio routing for medical data transmission using IoT. AIMS Electronics and Electrical Engineering, 2022, 6(3): 223-246. doi: 10.3934/electreng.2022014 |
[10] | Minglong Zhang, Iek Cheong Lam, Arun Kumar, Kin Kee Chow, Peter Han Joo Chong . Optical environmental sensing in wireless smart meter network. AIMS Electronics and Electrical Engineering, 2018, 2(3): 103-116. doi: 10.3934/ElectrEng.2018.3.103 |
Reverse data delivery is one of the key operations in vehicular ad hoc networks which allows data delivery from roadside units (infrastructure) to vehicles. In the past, a number of data dissemination schemes have been proposed by researchers for the vehicular ad hoc networks to tackle the problem of data forwarding from vehicles and roadside units to data concentration points or Access Points (APs). In contrast, schemes for reverse data forwarding which allows a data concentration point to apprise the on-road vehicles about any eventuality or developing hazardous conditions are very few. This paper proposes a reverse data forwarding scheme which utilizes the characteristics of the road networks to inform passing vehicles of important information related to any accident, crash, or hazardous road conditions. The proposed scheme takes into account the geographical proximity factor by considering a localized sub-region/segment in the greater road network which is most adversely affected by the occurrence of some unfortunate road event. For its implementation, the scheme is dependent on DSRC-enabled roadside equipment to relay road-safety messages from AP to vehicles. In case if the passing vehicles are not fitted with DSRC on-board units, the scheme has the capability to directly interact with the variable-message signs on intersections with the objective of reaching as many vehicles as possible to increase the chances of message delivery. Simulation results using a small road segment confirm that the proposed scheme is more effective when the localized sub-region is smaller or in other words when more vehicles in the vicinity of the accident location are provided with pre-warning messages.
In the past two decades, considerable research efforts have enabled the actual implementation and deployment of wireless ad hoc networks tailored to the unique requirements of certain sensing and monitoring applications. Vehicular ad hoc network (VANET) used for road traffic safety is one of those applications [16]. Road traffic safety refers to the methods and measures used to prevent road users from being killed or seriously injured. Typical road users include pedestrians, cyclists, motorists, vehicle passengers, and passengers of on-road public transport (mainly buses and trams) [1]. For many years, the main objective of road safety measures was to facilitate survivors of road accidents but after the introduction of DSRC in 2006 [2] and its subsequent standardization under the name of IEEE WAVE (Wireless Access in Vehicular Environments) in 2010 [3], the focus of road safety has been shifted to the concept of accident avoidance [4].
DSRC is without any doubt one of the most promising technologies of the future and it is already under implementation phase in countries like US [5] and in testing phase in countries like Australia [6]. DSRC provides vehicle-to-vehicle (V2V) as well as vehicle-to-infrastructure (V2I) communication. To some extent, the infrastructure-to-vehicle communication is also present in DSRC e.g., when a traffic light tells all the DSRC capable vehicles waiting at the traffic signal about the amount of time in seconds after which the signal will turn green [13]. The proposed work enhances this advantage of DSRC to a localized sub-region of the road network referred to as region-of-interest in this work. The proposed Location-based Reverse Forwarding (LRF) scheme (which was initially proposed with some basic results in an earlier work [20]) has the capability to utilize DSRC technology without any additional hardware requirements. The proposed scheme makes use of the data collected by wireless sensors. It is based around the concept of Region-of-Interest (RoI), an idea which has roots in data sets and has applications in medical imaging, geographic information system, and document analysis.
In the near future, a typical wireless sensor network based urban traffic management system (UTMS) will look like the one shown in Figure 1 [7]. This system includes sensors, roadside units (RSUs), on-board units (OBUs) on vehicles and traffic management centers (TMCs) which communicate with all nearby RSUs and other TMCs either using Internet connectivity or multi-hop communication through sensors and RSUs. The sensors first forward the data to Roadside Units (RSUs). The RSUs collect the data from both sensor nodes and the OBUs and forward it to TMC. TMC may be thought of as an Access Point with Internet connectivity to the central TMC of a city and to other TMCs. The TMC analyzes the data for accident or hazardous condition and in such case identifies the region-of-interest (RoI) and then forwards announcements to all TMCs in that RoI, which send such notifications to concerned RSUs as well as to controllers managing variable-message signs. The RSUs in turn send these announcements to vehicle OBUs which are approaching the accident/hazard site from all directions. It should be kept in mind that the LRF scheme does not rely on vehicles for carrying data to the designated entrance points in the RoI. Instead, it completely relies on multi-hop data forwarding between sensors and RSUs and direct connectivity of RSUs with TMCs and if possible direct connectivity of TMCs with other TMCs. This type of communication will be considered as an example of reverse data forwarding from infrastructure-to-vehicle (I2V).
The main contributions of the work presented in this paper in both theoretical and practical aspects are as follows:
● The proposed LRF scheme exploits the concept of region-of-interest in a road network to ensure reverse forwarding of road condition information to vehicles entering into an accident-affected area of road network through designated intersections.
● This work proposes to create tables of intersections inside TMCs that can be used in case a need for accident related message dissemination arises.
● The proposed work can be readily applied to dense urban settings with DSRC-capable RSUs and OBUs.
The rest of this paper is organized as follows. A brief overview of related work in the area of reverse data forwarding is presented in Section 2. DSRC is discussed very briefly in Section 3. Problem formulation is discussed in Section 4. Basic concept of RoI and LRF protocol are discussed in Section 5 and Section 6 respectively. A performance analysis of the scheme is presented in Section 7 followed by discussion in Section 8 and the paper is concluded in Section 9.
There is plenty of work reported in literature on schemes which provide data forwarding for V2V and V2I scenarios specifically in the context of vehicular ad hoc networks utilizing the layout of road network and traffic statistics. These schemes include VADD (Vehicle-Assisted Data Delivery) [8], DBR (Delay-Bounded Routing) [9], SADV (Static-Node Assisted Adaptive Routing) [10], TBD (Trajectory-based Data Forwarding) [11], MDDV (Mobility-centric Data Dissemination) [18], and LDF (Location-based Data Forwarding) [19].
VADD is primarily based on the idea of aggressive carry-and-forward in such a way that a mobile vehicle continues to move with a packet for delivery to a stationary destination (e.g. AP) until it finds a new vehicle in its neighborhood that provides a forwarding path with the smallest packet delivery delay. VADD continues to utilize this dynamic path selection and handover approach during the entire data dissemination step until the packet reaches its destination. VADD utilizes predictable vehicular mobility, which takes into account the layout of the road network as well as patterns of traffic. In contrast to the VADD approach, DBR is based on the idea of data dissemination to satisfy a delay bound which is user-defined (or application-defined) rather than the smallest packet delivery delay as used in VADD. DBR is also a carry-and-forward approach that leverages knowledge of traffic and alternates between multi-hop forwarding of data and data muling. SADV can be referred to as a carry-store-and-forward approach which stores (rather buffers) data packets for some time in static roadside nodes until the most optimal vehicle to forward the packet further toward its destination comes under the communication range of the static node. TBD improves forwarding performance by computing the expected data delivery delay (EDD) metric at individual vehicles with AP as the destination. This EDD metric utilizes trajectory information and available traffic data. The computed EDD is shared with neighbors and the vehicle with the smallest EDD is chosen as the next forwarder of the packet. MDDV proposes a forwarding scheme in VANET utilizing opportunistic forwarding to allow a predefined packet forwarding trajectory for the packet from source to destination. The packet forwarding trajectory in MDDV is the path which the packets follow, and so it may be different from the vehicular trajectory especially when there is no vehicle found on the chosen path. LDF combines Q-learning for environment exploration with k-shortest path algorithm to ensure adaptive routing from static roadside sensors to a remote AP.
There is comparatively less work reported for schemes which provide I2V reverse data forwarding. One of the earliest schemes for reverse data forwarding for sharing road condition information tailored for the infrastructure-to-vehicle data delivery in vehicular networks is TSF (Trajectory-based Statistical Forwarding) [12]. This type of reverse data forwarding was proposed to deliver road condition information from infrastructure nodes to moving vehicles for the driving safety. TSF forwards packets to a multi-hop away target point where the vehicle is expected to pass by, which is defined as rendezvous point of the packet and the destination vehicle. Such a target point is optimally selected to minimize the packet delivery delay while satisfying the required packet delivery probability. The optimality is analytically achieved by utilizing both distributions of the packet delay and the destination vehicle delay, which are obtained from the vehicular traffic density and the vehicle trajectory. One limitation of TSF is that it requires vehicles to report their trajectory information to the Access Points (APs) using V2I schemes like VADD, DBR, SADV, TBD, MDDV, or LDF, therefore, preventing those devices without a DSRC OBU to take advantage of the available information. In comparison, LRF will allow I2V communication for vehicles fitted with DSRC OBUs as well as prompt announcements using variable-message signs for vehicles without the OBUs fitted in them.
Recently, there has been an interest in applying information-centric networking (ICN)-based approaches in the VANET forwarding scenarios [21]. Among the ICN-based approaches, named data networking (NDN) [22] is one of the approaches which has received considerable attention in the last few years [23]. NDN approach is a data centric approach in which the data is sought using its name (or a reference to contents) instead of using an address (like IP address) for the destination host. The data content is the most important component of an NDN-based network (whether it be a VANET or any other wireless ad hoc network). The field representing the user's request for a particular data item is referred to as an "interest''. The working of an NDN-based network involves three main components in its forwarding devices which are namely pending interests table(PIT), forwarding information base (FIB), and content store (CS). PIT holds interest requests while they are waiting for the corresponding data to arrive. FIB is a forwarding table/database to hold prefix/data pairs as well as routes to interests. CS is a small local cache to hold data for a later use. Forwarding mechanism in an NDN-based VANETs begins with a requester vehicle sending an interest request to an NDN-based routing vehicle which relays the interest request all the way to a data providing vehicle which replies with the data and additionally reverse forwards it to the requester vehicle using either the same relay path used by the interest request or a more optimal one. The forwarding vehicles on the reverse path can cache the interest/data pair for a possible later use. A very comprehensive classification of forwarding strategies in NDN-based VANETs is given in [24].
A number of comparatively recent techniques have augmented the basic mechanism of NDN-based VANETs with location information as well as link stability [25,26,27,28]. The basic idea behind these technique is to estimate a suitable location for a data providing vehicle before sending out an interest request. Once the location information about the producing vehicle is known, the interest request follows a greedy geographical forwarding mechanism to forward the interest to the provider location.
In [25], a location-aware ICN-based content management technique called LOICEN has been proposed in an NDN-based VANET. LoICen contains a location management service for the content providers. The location management service has a lookup table to hold the position of all the provider vehicles that have the highest probability for holding the requested data content in their local content store cache. This positioning data is then utilized to steer the interest packets in the proximity of a provider vehicle holding desired data content. The only downside of this proposal is its limitation in not identifying a newer position if it becomes available as the proposed technique is designed to steer an interest request to the last available position of a provider vehicle holoding the desired data content in its local content store. Both [26] and [27] employ link stability for forwarding an interest request. Their proposals employ factors like position, speed, and orientation to calculate the transmission delay between the requester vehicle and the routing vehicle. A priority-based mechanism for transmission of interest requests has been used which is based on a deference timer. The receiver utilizes the connection time to sender to compute a value for deference timer. The receiver uses this deference timer to send the desired data content along reverse path to the sender when the waiting time for the sender is shorter than the deference timer. This comes handy in choosing a more stable reverse path. Both the proposed scheme are suitable in urban scenarios where the vehicle density is sufficiently higher.
In [28], another technique utilizing the link stability has been proposed which is called link stability-based interest forwarding (LSIF). LSIF employs information like speed, orientation, and present location of a vehicle to predict link stability. The predicted link stability value is propagated along with the interest request. It also computes a local threshold value for the routing process and only initiates the transmission of content request if the two vehicles are within a suitable signal range. A vehicle receiving an interest request also checks the predicted link stability value in the interest request and computes a link lifetime value. Interest request is only forwarded if the computed link lifetime value is greater than the computed local threshold value. In case the threshold value is higher, the data content searching is not initiated. One downside of LSIF is that it does not take non-linear geometry of the trajectory in consideration.
DSRC uses RF with a 75 MHz of licensed spectrum in the 5.9 GHz band and has been regulated by the IEEE 802.11p standard for Wireless Access in Vehicular Environment (WAVE) [2]. DSRC targets to achieve a communication range of 1000m, however, range of 300m is the most common. A major motivation for utilizing DSRC is to allow accident avoidance. This can be accomplished by requiring vehicles to exchange data with other vehicles and roadside units. This exchange of data enables vehicles to have a better idea of neighbor's trajectory and look for potential threats by comparing it with their own path [13]. In addition to this data exchange (V2V), vehicles can also exchange data with RSUs which serves as a pre-warning mechanism. The proposed LRF scheme aims to further improve this mechanism. Utilizing the aforementioned V2V and I2V data exchanges, several safety messages can be sent, some of them are:
● Forward collision warning
● Hard-braking vehicle
● Blind spot caution
● Intersection warning
In US, DSRC utilizes a number of standards which afford interoperability to devices from different vendors. The PHY and MAC layers of the DSRC use IEEE 802.11p WAVE which is a modified version of IEEE 802.11 (Wi-Fi). The network and transport layers support TCP/UDP and IPv6 which is preferred when multi-hop messaging is desired. In addition, WAVE Short Message Protocol (WSMP) is also provided which is most suitable for single-hop messaging. An important standard used at application layer is the SAE J2735 message set dictionary standard which defines the syntax of very useful message formats for V2V communication. Among them, the most significant is the BSM (Basic Safety Message) which is used to communicate state information of vehicles in a V2V scenario.
The reverse data forwarding in vehicular networks can be formulated as: Given a road network with sensors, RSUs and TMCs, the objective of reverse forwarding is to forward pre-warning messages from TMCs to RSUs (and subsequently to vehicle OBUs) after identifying a region-of-interest containing all intersections from where the approaching vehicles will be most affected. As mentioned in Section 1, following are the assumptions for the WSN based urban traffic management system.
● Most vehicles are installed with OBUs. Those vehicles which do not have OBUs installed can take advantage of the messages shown on variable message signs at the intersections.
● Sensors are installed at sides of roads to monitor passing vehicles.
● RSUs are installed at intersections of the road networks and have DSRC capability in order to communicate with OBUs of vehicles as well as communication capability with sensors and corresponding TMC.
● TMCs are present at intersections and can communicate with corresponding RSUs as well as other TMCs and central TMC using DSRC.
● TMCs can use Internet connectivity to communicate with other TMCs or they can alternatively use multi-hop communication through sensors and RSUs.
There are few characteristics of road networks which put some stringent demands on the design of data forwarding schemes in vehicular networks. Three of the characteristics as discussed in [12] are:
● Vehicles move on physical roadways and hence are constrained by the physical limits of roadways.
● Vehicles are also constrained by speed limit.
● Communication shortest paths are mostly not equal to physical shortest paths.
Another characteristic which is a ramification of first characteristic above is that a certain point anywhere in the road network is only approachable or accessible by a specific number of intersections and road segments leading to that point. In civilian work or military operations, sometimes those intersections are also cordoned off or warned against to completely stop any inward flow of vehicles to reach that point. Since in civilian applications, road maps are mostly known, therefore, identifying these entrance points is straightforward. Such an identification will be highly beneficial in case of any eventuality (like accident, submerging, sink hole, or land sliding). If we consider that the set of all such entrance points are located within a certain radius of the point of accident, then that region encompassing those intersections (and corresponding TMCs and RSUs) will be referred to as region-of-interest (RoI). As an example of the concept of RoI, consider a small portion of a road network shown in Figure 2.
In order to reach any area which is affected by accident and marked with black diamond, all the entrance points are located at intersections marked with red stars. Therefore, for this simple segment of road network, the region-of-interest has a radius of approximately 1.5 km and contains five important entrance points. In case of any accident anywhere in this road network, the sensors and RSUs nearest to the accident site can report the accident to corresponding TMC which will use reverse forwarding to issue pre-warning messages to RSUs located at these five entrance points. The OBU of every vehicle trying to enter this region from these entrance points will receive these pre-warning messages and will act based on the severity of the situation. The motorist will have ample time to decide whether to slow down, choose an alternate path, or completely abandon the journey by taking a U-turn at these intersections.
One possible implementation of this idea will require every TMC to maintain a simple lookup table containing list of TMCs corresponding to sets of sensor locations. The lookup process will involve several steps. The sensors will forward sensor data to RSUs which will be a four-tuple containing sensor location, type of accident (like crash, tire skidding or others) or other measurement, time-stamp, and priority. RSUs will forward sensor data to TMC. TMC will perform a lookup in its lookup table to retrieve a list of remote TMCs (or remote RSUs). TMC will perform data forwarding using either direct connectivity with target TMC (if available) or multi-hop forwarding using other sensors and RSUs. Once the warning message is received at target TMCs (or RSUs), the TMCs will forward it to all of their connected RSUs which in turn will forward it to OBU of every passing vehicle. A possible structure of a lookup table is shown in Table 1. The rest of the details of this scheme are presented in the next section.
Reported sensor location/Accident site | TMCs/Intersections |
Set of Locations |
Another possible implementation will be to provide a small sub-region digital map to every TMC. Using the map and some analysis, the TMC should be able to infer itself which intersections will be most affected by the present accident/skidding and should forward the pre-warning messages to them. As will be discussed in the next section, both the LDF protocol and the simulation presently use the first implementation involving a lookup table of affected TMCs/intersections corresponding to sensor/accident sites.
In this section, the LRF protocol is explained. In its simplest form, the LRF protocol entails three steps. The first step is straightforward and involves sensors sharing their data with RSUs which forward that data to respective and nearest TMC. The second step is the most important and it involves forwarding pre-warning messages to all those RSUs which are located at intersections constituting the region-of-interest. Third step is the simplest and involves RSUs forwarding the received messages to all vehicles passing through the intersections.
In the first step, the sensors will constantly collect data from roadside and periodically share it with local RSUs which are closest to their own location. The forwarded data will be at least a four-tuple containing (Sensor Location, Sensor Observation, Timestamp, Priority). The RSUs will forward this data to TMC. Not only sensor data, but RSUs will also forward their own measurements/observations to the TMCs as well. The TMC will store these observations, analyze them based on the received priority and will try to extract all useful information from the received data.
In the second step, in case the local TMC discovers any eventuality or accident reported by sensors, it will perform a lookup in its lookup table using sensor location as an index to determine the list of remote TMCs (or RSUs) where pre-warning messages are to be delivered. After getting this list of TMCs, the local TMC will start the process of reverse forwarding to remote TMCs. Since TMCs are DSRC capable, therefore, they can use IPv6 routing for multi hop communication through the network of RSUs and sensors. The LRF packet sent from local TMC to remote TMC will have the format shown in Figure 3. If the IPv6 routing capability is not available, the LRF packet can also contain a source route to the remote TMCs.
The third step will involve the remote TMC forwarding warning message to all of their connected RSUs. The RSUs after receiving the warning messages start forwarding it to the OBUs of all the vehicles which are passing through that intersection. One additional step can involve the remote TMC to display an electronic warning message on the variable-message sign under its control (if any).
In this section, some results are reported with a small segment of road network with a focus on the inclusion of all intersections (and corresponding TMCs/RSUs) in a specified region-of-interest. The performance analysis includes percentage of vehicles to which the DSRC pre-warning messages are conveyed successfully as they try to approach the accident site from all possible directions. At this stage, the variable-message signs display option is not considered in this analysis because the assumption is that all passing vehicles are fitted with OBUs. But it is assumed that the TMCs have the capability to display electronic messages. Since the proposed work is the first work to include the concept of region-of-interest in DSRC-based vehicular networks, therefore, it is compared with:
Random selection of intersections in the specified region-of-interest (RANDOM)
Most distant (most outward) intersections in the specified region-of-interest (DISTANT).
For the performance analysis, the effect of number of chosen intersections (and corresponding RSUs) on the percentage of cars with the received pre-warning messages is studied. For the road network, a segment of road network is simulated with 50 intersections. Every exit going out of the intersection is fitted with an RSU, so if there are 4 exits, 4 RSUs are assumed. In comparison, the number of TMCs is equal to the number of intersections. A communication range of 300 meters is assumed which is a conservative estimate given that the DSRC has a communication range of up to 1000 meters. The number of vehicles moving within the road network is assumed to be 500. The vehicles are assumed to move at a speed of . Since LRF uses stationary TMCs, RSUs and sensors with multi-hop communication, therefore a Time-to-Live (TTL) value of 255 hops is used after which the LRF pre-warning messages will be purged from the TMCs (or RSUs). An accident site is simulated at almost the center of the road network and there is a maximum of 10 intersections which allow access to that accident site. Vehicles are allowed to choose any starting point or ending point in the road network at random. Additionally, the simulation is repeated with a region-of-interest having a radius of 2 km, 4 km and 6 km. Those vehicles which intend to pass from the area of accident are also marked as they arrive at intersections. Therefore, those vehicles which do not include the accident site in their path will be excluded from consideration for the purpose of simulation only.
City section mobility model [14] is used to perform the simulation using Python scripting. Using this model, the vehicles are randomly placed at one intersection as starting point on the road network and randomly select another intersection as ending point. The vehicles move according to the roadways from their starting point to their ending point. Also, the vehicles wait for a random waiting time (0 to 10 seconds) at intersections in order to allow for the effect of traffic signal. The simulation is continued until all of the pre-warning messages are delivered to the approaching vehicles or the messages are purged due to TTL expiration [12]. Most of the simulation parameters are chosen to match a typical scenario in a DSRC environment [15] as shown in Table 2.
Parameter Name | Value Used |
Area | |
DSRC Communication Range | |
Number of Vehicles | |
Vehicle Speed | |
Mobility Model | City Section |
Time-to-Live |
Figures 4 and 5 show the percentages of cars which have received accident pre-warning messages from the RSUs in a region-of-interest having a radius of 2, 4, and 6 km respectively. All the shown results are an average of 10000 runs of the same simulation scenario. There is an interesting trend after looking at Figures 4 and 5 which shows that the LRF protocol will provide the most optimal performance with higher numbers of messages delivered when the region-of-interest is smaller or in other words the chosen intersections are geographically closer to the accident site. In that case, the pre-warning messages will be delivered to most number of vehicles.
As pointed out in Section 5, a possible future direction for LRF will be to provide a digital map of the sub-region or RoI in consideration to all of the TMCs. In case of any reported accident/skidding, the concerned TMC will perform some analysis using the digital map in order to come up with a list of locations of the intersections which will be adversely affected with this accident. This type of data analysis will remove the need for establishing a lookup table at the time of network initialization. The lookup table can be created on-the-fly and could be cached/updated for later use.
A quick look at Figure 5 reveals that the percentage of vehicles receiving messages is comparatively small. This is due to the reason that the percentage is calculated for all the 500 vehicles in the simulation without taking the vehicle destination in consideration. A look inside the vehicle destinations revealed that around 255 out of 500 vehicles chose to pass through the single accident site in the middle of the network and the other did not pass through those intersections which had the pre-warning messages through LRF scheme. In order to depict the actual performance of the LRF, a new performance metric called packet delivery percentage was defined which only calculated percentages for those vehicles which had the destination with accident site included in their trajectory. The new metric for RoI radii of , , and is shown in Figures 6 and 7. The best performance of LRF is with an RoI radius of as depicted in Figure 6.
After looking at high delivery percentage in Figure 5, another relevant question arises that whether it is theoretically possible to achieve 100% delivery percentage or not. The answer to this question depends on the DSRC capability as well as absence of channel congestion in DSRC based networks. If DSRC-capable OBUs are fitted in all vehicles and sufficient bandwidth is available in the region-of-interest, there is a possibility of achieving 99% or higher percentage of message delivery.
The effect of increase in the number of roadside sensors on the mean packet delivery delay was studied by increasing the number of roadside sensors in all road segments from 10 to 20 while keeping the number of RSUs and intersections same as initial settings. This was repeated for the three chosen region-of-interest radii of 2, 4, and 6 km. It was observed that after increasing the number of roadside sensors, initially the mean packet delivery delay decreased with increase from 10 to 15 but leveled out between 15 and 20. This trend is shown in Figures 8 and 9. This is due to the fact that a higher number of sensors started contributing more communication delay as well as queuing delay in forwarding from sender TMC to remote TMC.
Initially the time-to-live counter value was set at a value of 255 hops, which was quite favourable for the simulated road network. In order to study the effect of variation in time-to-live counter value on packet reception percentages, the TTL value was gradually changed from 32 to 64, then to 128 and then to 255 hops (which was the initial setting) and the packet reception percentages were observed. The packet reception percentages were more profoundly affected for most distant intersections scenario because more pre-warning messages were purged due to TTL expiration. The reception percentage in the road network with a smaller RoI radius of 2 km was the least affected as the number of hops were less and most of the messages were successfully received by the remote TMCs and subsequently by the passing vehicles. The 2 km scenario is shown in Figure 10 and 6 km in Figure 11. The higher radii RoIs were affected more but similar trend was observed. Therefore, a medium to higher TTL value is recommended otherwise more messages will be purged affecting reception percentages.
Another very important question which might arise is that since all the simulations of LRF were done with a fixed vehicle speed of , whether an increase in speed will affect the performance of LRF or not. In order to answer this question, all the simulations presented in Section 7.1 were repeated for higher vehicle speeds of and . It was observed that there was no considerable decrease in the delivery percentages and there was no adverse effect of vehicle speed on the LRF performance. There is a reason behind this behavior as the LRF is using node-to-node packet delivery as opposed to carry-and-forward mechanism in VANETs. In VANETs, when the carry-and-forward mechanism is used, the carry delay becomes the most dominant part of the delivery delay or latency. For example, if there is a road segment of 1 km and a vehicle speed of 50 km/hr is used, it will take around 72 seconds if carry-and-forward approach is used. But, if node-to-node delivery using DSRC wireless interface is used, it will only take 30 msec or a little higher even after errors and retransmissions are taken into consideration as the DSRC allows 27 Mbps data rate.
The effect of vehicle speed deviation is left as a future extension of this work.
As pointed out earlier in Section 5 that the LRF scheme in its present form is creating a lookup table to correctly forward messages to concerned entrance points/TMCs in the road network but a digital map if provided to TMC will greatly benefit the LRF scheme in automating most of the lookup table creation tasks. A quick look in the literature revealed that there is a very attractive technique which has been proposed by Máttyus et al. and it is being referred to as DeepRoadMapper [17]. DeepRoadMapper can create road networks using aerial images. Since this technique was found after the simulation of the LRF scheme work was almost complete, therefore, it could not be included into the LRF scheme in its present form and is left as a possible future enhancement of the LRF scheme. The salient features of the DeepRoadMapper are given below:
● The road mapper algorithm extracts a graph representation of the road network from aerial images.
● This graph's nodes represent end points of street segments and the graph's edges represent the connections between these points, defining the road segment center lines.
● The road mapper algorithm exploits convolutional neural networks to segment the aerial images into the categories of interest.
● After segmentation, a simple process based on thinning extracts the road center lines from the segmentation output.
● The errors in the segmentation output can result in discontinuities, which might translate into topological errors of the extracted road network.
● To alleviate the problem of discontinuities, the road mapper further reasons about the presence or absence of edges in an augmented road graph which also contains a connection hypotheses covering the discotinuities.
The application of DeepRoadMapper in LRF will be straightforward as follows:
● The TMCs are loaded with aerial images of the region-of-interest.
● The TMCs execute the road mapper algorithm.
● From the extracted road network graph in a particular region of road network with a given radius, the TMCs find out entrance and exit points.
● These entrance and exit points are used by TMCs to update/create the lookup tables of the corresponding region-of-interest.
● The TMCs use these endpoints in their lookup tables to report to remote TMCs in case of any accident.
Additionally, source coding can also be combined with LRF to compress the DSRC data packets. The emergency packets can be left uncompressed.
In this paper, a location-based reverse forwarding scheme for I2V data delivery in vehicular networks has been proposed which is based around the concept of region-of-interest which is an area comprising intersections in a specific radius of the accident site. The proposed scheme uses stationary TMCs, RSUs and sensors with multi-hop communication to alert approaching vehicles at chosen intersections using DSRC communication. Simulation with a small segment of road network has shown that a smaller radius region-of-interest closer to the accident site is optimal in terms of number of messages delivered to the passing vehicles.
The authors wish to acknowledge the support provided by the Systems & Control Group at the Department of Electrical, Computer, & Software Engineering of the University of Auckland.
The authors declare that they have no conflict of interest.
[1] | Road traffic safety (2017) In Wikipedia, The Free Encyclopedia. Available from: https://en.wikipedia.org/w/index.php?title=Road_traffic_safety&oldid=783832588 |
[2] | Vehicle safety communications project final report HS-810-591. US Department of Transortation National Highway Traffic Safety Administration, Washington, DC, USA, 2006, pp. 1-10. |
[3] | IEEE Standard 802.11p: IEEE standard for information technology telecommunications and information exchange between systems local and metropolitan area networks specific requirements; Part 11: Wireless LAN medium access control (MAC) and physical layer (PHY) specifications; Amendment 6: Wireless access in vehicular environments, 2010. |
[4] | Cailean AM, Cagneau B, Chassagne L, et al. (2014) A survey on the usage of DSCR and VLC in communication-based vehicle safety applications. Proceedings of the 21st IEEE Symposium on Communications and Vehicular Technology in the Benelux (SCVT), 69-74 |
[5] | Abuelsamid S (2016) New cars could be required to talk to each other as soon as 2020. Retrieved from https://www.forbes.com/sites/samabuelsamid/2016/12/13/nhtsa-finally-issues-draft-v2v-communications-rule-could-be-mandatory-from-2021 |
[6] | Farquhar P (2016) There's one major obstacle to a driverless car future that tech can't solve -- and that's you. Retrieved from https://www.businessinsider.com.au/theres-one-major-obstacle-to-a-driverless-car-future-that-tech-cant-solve-and-thats-you-2016-12 |
[7] | Nellore K, Hancke GP (2016) A survey on urban traffic management system using wireless sensor networks. Sensors 16: 1-25. |
[8] | Zhao J, Cao G (2008) VADD: Vehicle-assisted data delivery in vehicular ad hoc networks. IEEE T Veh Technol 57: 1910-1922. |
[9] | Skordylis A, Trigoni N (2008) Delay-bounded routing in vehicular ad-hoc networks'. Proceedings of the 9th ACM International Symposium on Mobile Ad Hoc Networking and Computing, 341-350. |
[10] | Ding Y, Wang C, Xiao L (2007) A static-node assisted adaptive routing protocol in vehicular networks. Proceedings of the 4th ACM Workshop on Vehicular Ad Hoc Networks, 59-68. |
[11] | Jeong J, Guo S, Gu Y, et al. (2009) TBD: Trajectory-based data forwarding for light-traffic vehicular networks. Proceedings of the 29th IEEE International Conference on Distributed Computing Systems, 231-238. |
[12] | Jeong J, Guo S, Gu Y, et al. (2010) TSF: Trajectory-based statistical forwarding for infrastructure-to-vehicle data delivery in vehicular networks. Proceedins of the 30th IEEE International Conference on Distributed Computing Systems, 557-566. |
[13] | Kenney JB (2011) Dedicated short-range communications (DSRC) standards in the united states. P IEEE 99: 1162-1182. |
[14] | Camp T, Boleng J, Davies V (2002) A survey of mobility models for ad hoc network research. Wireless Communications and Mobile Computing 2: 483-502. |
[15] | Carter A (2005) The status of vehicle-to-vehicle communication as a means of improving crash prevention performance. Tech. Rep. 05-0264. |
[16] | Eze EC, Zhang S, Liu E (2014) Vehicular ad hoc networks (VANETs): Current state, challenges, potentials and way forward. Proceedings of the 20th International Conference on Automation and Computing, 176-181. |
[17] | Máttyus G, Luo W, Urtasun R (2017) DeepRoadMapper: Extracting road topology from aerial images. Proceedings of the 2017 IEEE International Conference on Computer Vision, 3458-3466. |
[18] | Wu H, Fujimoto R, Guensler R, et al. (2004) MDDV: A mobility-centric data dissemination algorithm for vehicular networks. Proceedings of the 1st ACM International Workshop on Vehicle Ad Hoc Network, 47-56. |
[19] | Khan F, Nguang SK (2020) Location-based data delivery between vehicles and infrastructure. IET Intell Transp Sy 14: 288-296. |
[20] | Khan F, Nguang SK (2017) LRF: Location-based reverse forwarding for infrastructure-to-vehicle data delivery. Proceedings of the 9th International Conference on Signal Processing Systems, 32-36. |
[21] | Li Y, Shi X, Lindgren A, et al. (2018) Context-aware data dissemination for ICN-based vehicular ad hoc networks. Information 9: 263. |
[22] | Zhang L, Afanasyev A, Burke J, et al. (2014) Named Data Networking. ACM SIGCOMM Comput Commun Rev 44: 66-73. |
[23] | Xu Y, Tong S, Zhang T, et al. (2020) Compass: Directing named data transmission in VANETs by dynamic directional interfaces. IEEE Access 8: 8418-8435. |
[24] | Ahed K, Benamar M, Lahcen AA, et al. (2020) Forwarding strategies in vehicular named data networks: A survey. Journal of King Saud University - Computer and Information Sciences. |
[25] | Boukerche A, Coutinho R (2019) LoIcen: A novel location-based and information-centric architecture for content distribution in vehicular networks. Ad Hoc Networks 93: 101899. |
[26] | Boukerche A, Coutinho R, Yu X (2017) LISIC: A link stability based protocol for vehicular information-centric networks. IEEE 14th International Conference on Mobile Ad Hoc and Sensor Systems (MASS), 233-240. |
[27] | Coutinho R, Boukerche A, Yu X (2018) A novel location-based content distribution protocol for vehicular named-data networks. IEEE Symposium on Computers and Communications (ISCC), 01007-01012. |
[28] | Sousa M, Arajo FR, Sampaio L (2018) A link-stability based interest-forwarding strategy for vehicular named data networks. IEEE Internet Comput 22: 16-26. |
1. | Dingmi Sun, Yimin Chen, Hao Li, Intelligent Vehicle Computation Offloading in Vehicular Ad Hoc Networks: A Multi-Agent LSTM Approach with Deep Reinforcement Learning, 2024, 12, 2227-7390, 424, 10.3390/math12030424 |
Reported sensor location/Accident site | TMCs/Intersections |
Set of Locations |
Parameter Name | Value Used |
Area | |
DSRC Communication Range | |
Number of Vehicles | |
Vehicle Speed | |
Mobility Model | City Section |
Time-to-Live |