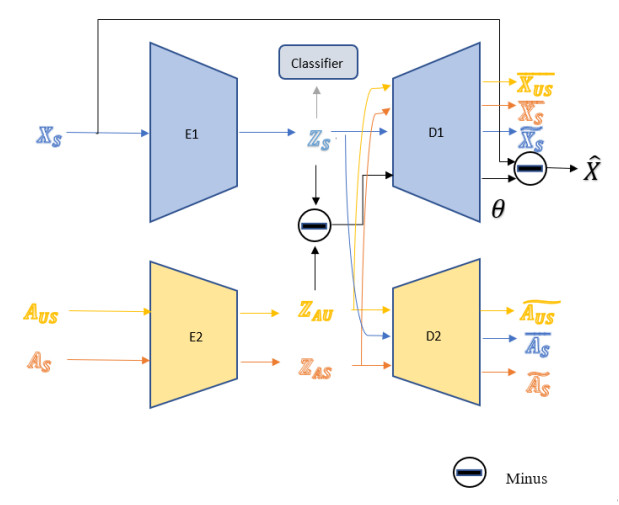
An entropically-driven binding interaction at a certain temperature may change to an enthalpically-driven process at another temperature, depending on the polarization state of the groups that are involved in binding. The streptavidin-biotin complex has been extensively studied across biological, medical, chemical and material science fields using various techniques, however, not much has been reported on this interaction across a broad temperature range, between 2 °C and 40 °C using biophysical techniques. In this study, we determined how the forces involved in the streptavidin-biotin complex formation are affected by the reaction temperature using the Affinity ITC (TA Instruments). We observed that this complex formation is a spontaneous binding process, indicated by a negative Gibbs energy (ΔG) at all temperatures tested. The observed negative heat capacity (ΔCp) ∼ −459.9 cal/mol K highlights the polar solvation of the interaction that corresponds to a decreasing enthalpy (more negative) (ΔH) with increasing reaction temperature. The stoichiometry (n) of 0.98 was estimated at 25 °C. An increase in reaction temperature resulted in an almost two-fold increase or more in n, notably from 1.59 to 3.41 between 30 °C and 40 °C. Whereas, at lower reaction temperatures, 2 °C to 10 °C, higher molar binding ratios were recorded, i.e. 2.74 to 5.76. We report an enthalpically-driven interaction between 30 °C and 40 °C whereas, an entropically-favourable interaction is observed at lower temperatures, suggestive of an interaction dominated by nonpolar interactions at lower temperatures and polar interactions at higher temperatures. Consequently, alterations in the polarisation state of streptavidin result in moderate binding affinity of biotin to streptavidin at higher reaction temperatures, KD 10−4 ≤ 10−5 M.
Citation: Keleabetswe L. Mpye, Samantha Gildenhuys, Salerwe Mosebi. The effects of temperature on streptavidin-biotin binding using affinity isothermal titration calorimetry[J]. AIMS Biophysics, 2020, 7(4): 236-247. doi: 10.3934/biophy.2020018
[1] | Ting-Huai Ma, Xin Yu, Huan Rong . A comprehensive transfer news headline generation method based on semantic prototype transduction. Mathematical Biosciences and Engineering, 2023, 20(1): 1195-1228. doi: 10.3934/mbe.2023055 |
[2] | Wu Zeng, Zheng-ying Xiao . Few-shot learning based on deep learning: A survey. Mathematical Biosciences and Engineering, 2024, 21(1): 679-711. doi: 10.3934/mbe.2024029 |
[3] | Hao Zhang, Lina Ge, Guifen Zhang, Jingwei Fan, Denghui Li, Chenyang Xu . A two-stage intrusion detection method based on light gradient boosting machine and autoencoder. Mathematical Biosciences and Engineering, 2023, 20(4): 6966-6992. doi: 10.3934/mbe.2023301 |
[4] | Depei Wang, Lianglun Cheng, Tao Wang . Fairness-aware genetic-algorithm-based few-shot classification. Mathematical Biosciences and Engineering, 2023, 20(2): 3624-3637. doi: 10.3934/mbe.2023169 |
[5] | Guoli Wang, Pingping Wang, Jinyu Cong, Benzheng Wei . MRChexNet: Multi-modal bridge and relational learning for thoracic disease recognition in chest X-rays. Mathematical Biosciences and Engineering, 2023, 20(12): 21292-21314. doi: 10.3934/mbe.2023942 |
[6] | Yevgeniy Bodyanskiy, Olha Chala, Natalia Kasatkina, Iryna Pliss . Modified generalized neo-fuzzy system with combined online fast learning in medical diagnostic task for situations of information deficit. Mathematical Biosciences and Engineering, 2022, 19(8): 8003-8018. doi: 10.3934/mbe.2022374 |
[7] | Jia Yu, Huiling Peng, Guoqiang Wang, Nianfeng Shi . A topical VAEGAN-IHMM approach for automatic story segmentation. Mathematical Biosciences and Engineering, 2024, 21(7): 6608-6630. doi: 10.3934/mbe.2024289 |
[8] | Hanjiang Wu, Jie Huang, Kehan Wu, António M. Lopes, Liping Chen . Precise tracking control via iterative learning for one-sided Lipschitz Caputo fractional-order systems. Mathematical Biosciences and Engineering, 2024, 21(2): 3095-3109. doi: 10.3934/mbe.2024137 |
[9] | Peng Wang, Shiyi Zou, Jiajun Liu, Wenjun Ke . Matching biomedical ontologies with GCN-based feature propagation. Mathematical Biosciences and Engineering, 2022, 19(8): 8479-8504. doi: 10.3934/mbe.2022394 |
[10] | Shi Liu, Kaiyang Li, Yaoying Wang, Tianyou Zhu, Jiwei Li, Zhenyu Chen . Knowledge graph embedding by fusing multimodal content via cross-modal learning. Mathematical Biosciences and Engineering, 2023, 20(8): 14180-14200. doi: 10.3934/mbe.2023634 |
An entropically-driven binding interaction at a certain temperature may change to an enthalpically-driven process at another temperature, depending on the polarization state of the groups that are involved in binding. The streptavidin-biotin complex has been extensively studied across biological, medical, chemical and material science fields using various techniques, however, not much has been reported on this interaction across a broad temperature range, between 2 °C and 40 °C using biophysical techniques. In this study, we determined how the forces involved in the streptavidin-biotin complex formation are affected by the reaction temperature using the Affinity ITC (TA Instruments). We observed that this complex formation is a spontaneous binding process, indicated by a negative Gibbs energy (ΔG) at all temperatures tested. The observed negative heat capacity (ΔCp) ∼ −459.9 cal/mol K highlights the polar solvation of the interaction that corresponds to a decreasing enthalpy (more negative) (ΔH) with increasing reaction temperature. The stoichiometry (n) of 0.98 was estimated at 25 °C. An increase in reaction temperature resulted in an almost two-fold increase or more in n, notably from 1.59 to 3.41 between 30 °C and 40 °C. Whereas, at lower reaction temperatures, 2 °C to 10 °C, higher molar binding ratios were recorded, i.e. 2.74 to 5.76. We report an enthalpically-driven interaction between 30 °C and 40 °C whereas, an entropically-favourable interaction is observed at lower temperatures, suggestive of an interaction dominated by nonpolar interactions at lower temperatures and polar interactions at higher temperatures. Consequently, alterations in the polarisation state of streptavidin result in moderate binding affinity of biotin to streptavidin at higher reaction temperatures, KD 10−4 ≤ 10−5 M.
Deep learning has significantly succeeded in image recognition, image segmentation and target detection. However, deep learning models often require a large amount of labeled data to train, and labeling the data will take more time. Some scholars propose zero-shot learning. Zero-shot learning uses the model obtained from the seen classes to infer the unseen class of samples, which can largely reduce the labeling of samples [1,2]. Zero-shot learning mimics the process of human cognition of new things. For example, a person knows an animal, like a horse, through pictures and their linguistic descriptions; when knowing about the linguistic description of zebras, zebras look like horses and have black and white stripes on its body, then the person can still recognize zebras by this linguistic description when seeing zebras even though he or she has never seen a zebra [3]. In zero-shot learning, semantic features are needed in addition to using sample features. Semantic features are linguistic descriptions of the samples, such as the color, size and other characteristics. Word vectors extracted by Word2Vec [4] are usually used as semantic features. In zero-shot learning, the model is trained by the seen class samples with semantic features to find the relationship between semantic features and the seen class samples, and then transfers the model to unseen class samples to infer the categories of the unseen class samples.
There are two categories of zero-shot learning: generalized zero-shot learning and conventional zero-shot learning. The test set contains only the unseen class samples for conventional zero-shot learning. While for generalized zero-shot learning, the test set contains not only the unseen class samples but also the seen class samples. In zero-shot learning, there are many approaches devoted to finding the relationship between the training set samples and the training set semantic features, such as mapping the training set samples to the semantic feature space, mapping the semantic features to the sample space [5], mapping the semantic features and samples to the common space [6,7] and mapping the semantic features and samples to each other's space [8]. However, the training set contains only the seen class samples, and the classes in the training set are not the same as those in the unseen class, which can lead to the inaccurate classification of the unseen class samples when the classification models are used in the test set.
To address these problems, some scholars use generative models, such as Variational Autoencoder (VAE) [9] and Generative Adversarial Network (GAN) [10], to generate the unseen class pseudo samples, and input them to the classifier for training, which can alleviate the problem of inaccurate classification of samples in the unseen classes. It has been proposed in the literature [11,12] that the use of VAE and GAN leads to the problems of posterior collapse and training instability, and the use of Wasserstein Auto-Encoder (WAE) and Wasserstein Generative Adversarial Network (WGAN) to generate pseudo samples can alleviate this problem. However, the unseen class samples generated by these methods are easily biased to the features of the seen class samples, leading to inaccurate classification results when classifying the real unseen class samples. To address these problems, we propose the following methods:
1) Different from the above generation models, we use autoencoder to generate the unseen class samples. To make the sample features in the latent space more distinguishable and representative, a classifier is used for the sample features in the latent space.
2) In order to reduce the unseen class pseudo sample features that are biased to the seen class sample features, we propose new sample features and use them together with unseen class semantic features for cross-reconstruction loss function.
3) The proposed method is validated for three datasets, AWA1, AWA2 and aPY and have good results.
The structure of this paper is organized as follows. First, we thoroughly review the related works in Section 2. The proposed method is illustrated in Section 3. In Section 4, we discuss the experiments, and we conclude the paper in Section 5.
For zero-shot learning, embedding-based zero-shot learning is a common approach. However, embedding-based zero-shot learning can produce domain shift problems and misclassification in unseen class samples [13].
There are three solutions to alleviate the unseen class samples that are easily misclassified into the seen classes in zero-shot learning: calibrated stacking, generative models and detection of the unseen class samples. Calibrated stacking [14] added a calibrated term to the classifier so that the score of the seen class is reduced during classification, and the score of the unseen class samples can be increased. The calibrated stacking equation is as follows:
ˆy=argmaxc∈Tfc(x)−γI[c∈S] |
where γ is the calibrated factor and the indicator function. I[⋅] indicates whether c belongs to a seen class, if c is a seen class, the value of the indicator function is 1, otherwise the value of the indicator function is 0. The classifier of APN [15] used class embedding and added calibrated stacking in the classifier to alleviate misclassification of the unseen class samples.
The method of generative models is to use generative models to generate pseudo samples substitute real unseen class samples [16]. The training set and pseudo samples are used in training the classifier, so that the unseen class samples can avoid bias to the seen classes. Multi-modal Feature Fusion algorithm (MFF) [17] used visual principal component features to compensate for the lack of descriptive information using only semantic features, and then combined GAN and VAE to generate high-quality pseudo-samples. Cross- and Distribution Aligned VAE (CADA-VAE) [18] was the VAE method. The latent space distributional alignment and cross-alignment were used to ensure the alignment between the two different modalities of sample features and semantic features. To make the generated samples close to the real samples, Over-Complete Distribution using Conditional Variational Autoencoder (OCD-CVAE) [19] used over-complete distribution to generate pseudo samples. Chen et al. [12] proposed to use WAE to generate pseudo samples and used an aggregated posterior distribution in the latent space to align the manifold structure of the sample features and the semantic features. f-CLSWGAN [20] used WGAN to generate pseudo samples and used a classifier to make the generated pseudo samples more discriminative. Based on f-CLSWGAN, Adaptive Bias-Aware GAN (ABA-GAN) [21] proposed adaptive adversarial loss and domain loss functions to make the generated pseudo samples more meaningful and to distinguish the seen classes from the unseen classes. Li et al. [11] used WGAN to generate pseudo samples and used multimodal cyclic loss function and bi-directional autoencoder. In response to the fact that GAN is not easy to train, and the pseudo samples generated by VAE are of low quality, Dual VAEGAN [22] used a combination of GAN and VAE.
Detection of samples of the unseen class. This method first distinguishes whether the samples belong to the seen classes or the unseen classes and then classifies the samples into specific class. GatingAE [3] first used the latent space and the cross-reconstruction space to detect samples belonging to the unseen class, and then used a linear classifier to classify the samples in the seen classes and a nearest neighbor classifier for the samples belonging to the unseen classes. Chen et al. [23] proposed determining whether a sample belongs to the seen class or the unseen class by calculating the cosine similarity between the latent space features of the samples and the mean value of each class. However, the models in these methods are obtained by training the samples from the seen classes; when migrating to the samples from the unseen classes, the classification results of the samples from the unseen classes are still biased to the seen classes.
Cao et al. [24] achieved recognition of zero shot traffic signs using autoencoder. Different from the literature [24], we use autoencoder to generate the unseen samples to alleviate the misclassification of the unseen class samples. To prevent the generated unseen class samples biased towards the features of seen class samples and improve the classification accuracy of the unseen class samples, we add the information of both unseen class semantic features and the proposed sample features.
In zero-shot learning, the training set can be denoted as S={XS,AS,YS}, and the unseen class can be denoted as U={XU,AU,YU}, where X denotes sample features, A denotes semantic features and Y denotes labels. For conventional zero-shot learning, the class of XU is predicted by the classifier: XU→YU; for generalized zero-shot learning, the class of X is predicted by the classifier: X→YS∪YU.
In this study, we use autoencoder to generate the pseudo samples of unseen classes, and the model is shown in Figure 1. In the Figure 1, E1 and E2 represent the encoder, D1 and D2 represent the decoder. The sample features and semantic features are encoded to obtain the same dimensional latent space features.
According to the autoencoder, for the training set, the generated sample features ~XS and the semantic features of the seen classes ~AS need to approximate the input features XS and AS.Assuming that there are m samples, the reconstruction loss function can be written as:
Lrecon1=1m∑mi=1|xsi−~xsi|+1m∑mi=1|asi−~asi| | (1) |
We use the lowercase xsi, ~xsi, asi and asi to denote one sample feature in XS, one generate sample feature in ~XS, one semantic feature in AS and one generated semantic feature in ~AS respectively. We want these two modality features to be aligned in the latent space. We use ZS to represent the sample features of the latent space and ZAS to represent the seen class semantic features of the latent space.
Llatent−recon=1m∑mi=1|zSi−zASi| | (2) |
In Eq (2), we use the lowercase zSi to denote one sample feature in ZS, and use the zASi to denote one seen class semantic feature in ZAS. In addition to the reconstruction loss function shown in Eq (1), zero-shot learning contains two different modalities, sample features and semantic features. Aligning different modalities can reduce the domain shift problem [25]. Inspired by GatingAE [3], Chen et al. [12], CADA-VAE [18] and Discriminative Cross-Aligned Variational Autoencoder(DCA-VAE) [25], we use the cross-reconstruction loss function. The features ¯XS and ¯AS are obtained by passing the semantic features and sample features of the latent space through D2 and D1 decoders, respectively, the cross-reconstruction loss function is as follows:
Lcross−recon1=1m∑mi=1|xsi−¯xsi|+1m∑mi=1|asi−¯asi| | (3) |
Here, ¯xsi denotes one feature in ¯XS, and ¯asi denotes one feature in ¯AS. Although we can use Eqs (1), (2) and (3) to train the model and then to generate samples of the unseen classes, Eqs (1), (2) and (3) only contains samples of the seen classes and semantic features, which will lead to the pseudo samples being biased to the seen classes. To address this problem, we add the unseen class semantic features AUS to the model and propose new sample features ˆX.
The unseen class semantic features AUS can be obtained by the following method. The sample features of the training set are mapped to the semantic feature space using the following equation:
minW||XS−WTAS||2F+α||W||2F | (4) |
||⋅||F in Eq (4) denotes Frobenius norm. The mapping matrix W is obtained as follows:
W=XTSAS(ATSAS+αI)−1 | (5) |
where I in Eq (5) represents the unit matrix and α denotes an adjustable parameter. The sample features in the training set are then mapped to the semantic feature space through the mapping matrix W and find the nearest unseen class semantic features, which constitute AUS.
After obtaining AUS, we input AUS to the autoencoder to obtain the generated unseen class semantic features ~AUS, and the reconstruction loss between ~AUS and AUS is:
Lrecon2=1m∑mi=1|ausi−~ausi| | (6) |
Here, we use ausi to denote one unseen class semantic feature in AUS and ~ausi to denote one generated unseen class semantic feature in ~AUS. Except the reconstruction loss for AUS. We also want to align different modalities between the unseen class semantic features and sample features, but there is a lack of unseen class samples in the training set. In this paper, we take the following approach to get the cross-reconstruction loss function: Find the difference between the unseen class semantic features and the seen class sample features in the latent space, the difference represents the relationship between the unseen class semantic features and the seen class sample features in the latent space. Then, pass the difference through the decoder D1 to get θ. We use ZS and ZAU to represent the latent features of the seen class sample features and the latent features of the unseen class semantic features. The formula is as follows:
θ=D1(ZS−ZAU) | (7) |
Then subtract θ from the sample features of the training set to obtain the feature ˆX:
ˆX=XS−θ | (8) |
The cross-reconstruction loss function for unseen class semantic features can be written as:
Lcross−recon2=1m∑mi=1|^xi−¯xusi|+β1m∑mi=1|ausi−¯asi| | (9) |
β in the above equation is an adjustable parameter. ^xi denotes one feature in ˆX, ¯xusi denotes one feature obtained by passing one unseen class semantic feature through the decoder D1. The reason for using the feature ˆX instead of XS is that ˆX can reduce the information of the seen class samples in the loss function, which can alleviate the similarity between the unseen class pseudo samples and the seen class samples.
To better find the relationship between the semantic features of unseen classes and the training set samples in Eq (7), and also make the sample features in the latent space distinguishable and representative, the sample features of the latent space are classified using the cross-entropy loss function:
Lclassifier=−∑mi=1ysilog~ysi | (10) |
~ysi in Eq (10) is the predicted label and ysi is the true label of the sample features of the latent space.
Combining Eqs (1), (2), (3), (6), (9) and (10), the objective function is:
L=Lrecon1+Llatent−recon+Lrecon2+Lcross−recon1+Lcross−recon2+Lclassifier | (11) |
After the model is trained according to Eq (11), the samples are generated with the sample features XS and semantic features AUS. For generalized zero-shot classification, all the generated seen class samples and unseen class samples need to be input to the classifier for training. For conventional zero-shot classification, only the generated unseen class samples need to be input to the classifier for training.
Three datasets, AWA1, AWA2 and aPY, are used in our study.
1) AWA1 [26]: The seen class contains 40 categories, and the unseen class contains 10 categories. The number of samples in the seen class is 19832, the number of samples in the unseen class is 5685 and the dimension of the semantic features is 85.
2) AWA2 [27]: The seen class contains 40 categories, and the unseen class contains 10 categories. The number of samples in the seen class is 23527, the number of samples in the unseen class is 7913 and the dimension of the semantic features is 85.
3) aPY [28]: The seen class contains 20 categories, and the unseen class contains 12 categories. The number of samples in the seen class is 5932, the number of samples in the unseen class is 7924, and the dimension of the semantic features is 64.
The sample features and semantic features used in our study are taken from the literature [27]. Following the literature [12], the input dimension of encoder E1 is 2048 dimensions, the output of the first layer is 512 dimensions, and the dimension of the latent space is 128; the dimension of the output of the first layer of encoder E2 is 128.The dimension of the output of the first layer of decoder D1 is 256 and the dimension of output is 2048; the dimension of output of the first layer of decoder D2 is 256. We use the Adam algorithm for optimization, the learning rate is 0.001 and the batch size is 256.
We use the evaluation criteria proposed in the literature [27]. For the conventional zero-shot classification, only the accuracy of classification needs to be calculated:
acc=1|C|∑|C|i#correct predictions in isamples in i |
For generalized zero-shot classification, not only the classification accuracy of the seen class and the unseen class should be calculated, but also the harmonic mean. Assuming that the classification accuracy of the samples of seen classes is denoted as acctr and the classification accuracy of the samples of unseen classes is denoted as accts, the harmonic mean can be written as:
H=2×acctr×acctsacctr+accts |
The generalized zero-shot classification results and the conventional zero-shot classification results are shown in Tables 1 and 2, where the results of Semantic Autoencoder (SAE) [8], Direct Attribute Prediction (DAP) [26], Indirect Attribute Prediction (IAP) [26] and Structured Joint Embedding (SJE) [29] are from the literature [27]. In Table 1, "ts" represents the classification results of unseen classes and "tr" represents the classification results of the seen classes. From Table 1, the proposed method is 1% less than CADA-VAE [18] for the AWA1 dataset. For the AWA2 dataset, the proposed method is 0.5% better than Chen et al. [23]. For the aPY dataset, the proposed method is 3.1% higher than DAP [26], while it is 4.9% higher than the generative model Chen et al. [12]. The accuracy of the proposed method on unseen class is higher than other methods.
AWA1 | AWA2 | aPY | |||||||
ts | tr | H | ts | tr | H | ts | tr | H | |
SAE [8] | 1.8 | 77.1 | 3.5 | 1.1 | 82.2 | 2.2 | 0.4 | 80.9 | 0.9 |
DAP [26] | 46.5 | 68.5 | 55.4 | 43.7 | 70.2 | 53.3 | 27.6 | 55.8 | 37.0 |
IAP [26] | 2.1 | 78.2 | 4.1 | 0.9 | 87.6 | 1.8 | 5.7 | 65.6 | 10.4 |
SJE [29] | 11.3 | 74.6 | 19.6 | 8.0 | 73.9 | 14.4 | 3.7 | 55.7 | 6.9 |
Preserving Semantic Relations (PSR) [30] | 20.7 | 73.8 | 32.3 | 13.5 | 51.4 | 21.4 | |||
f-CLSWGAN [20] | 57.9 | 61.4 | 59.6 | ||||||
Zhang et al. [31] | 20.7 | 67.9 | 38.6 | 16.1 | 66.9 | 25.9 | |||
Li et al. [11] | 54.9 | 71.7 | 62.2 | ||||||
CADA-VAE [18] | 57.3 | 72.8 | 64.1 | 55.8 | 75.0 | 63.9 | |||
Chen et al. [23] | 54.7 | 72.7 | 62.4 | 55.6 | 76.9 | 64.2 | |||
Chen et al. [12] | 54.5 | 72.8 | 62.3 | 55.2 | 73.5 | 63.0 | 26.7 | 51.5 | 35.2 |
The proposed method | 62.4 | 63.9 | 63.1 | 60.6 | 69.5 | 64.7 | 31.5 | 55.3 | 40.1 |
Table 2 shows the conventional zero-shot classification results. For the AWA1 dataset, the proposed method is slightly lower than f-CLSWGAN [20] and Li et al. [11], which used the GAN model. For the aPY dataset, the method in this paper is slightly lower than the method of Zhang et al. [31] and more accurate than the other methods. The accuracy of the method in this paper is higher than the other methods on the AWA2 dataset.
AWA1 | AWA2 | aPY | |
SAE [8] | 53.0 | 54.1 | 8.3 |
DAP [26] | 44.1 | 46.1 | 33.8 |
IAP [26] | 35.9 | 35.9 | 36.6 |
SJE [29] | 65.6 | 61.9 | 32.9 |
PSR [30] | 63.8 | 38.4 | |
Cross-Class Sample Synthesis (CCSS) [32] | 56.3 | 63.7 | 35.5 |
f-CLSWGAN [20] | 69.9 | ||
Zhang et al. [31] | 68.8 | 41.3 | |
Li et al. [11] | 69.9 | ||
CADA-VAE [18] | 58.8 | 60.3 | |
Chen et al. [12] | 65.2 | 65.5 | 32.7 |
The proposed method | 67.1 | 66.1 | 39.8 |
The parameters involved in the model are α, β and the dimensionality of the latent space, where we denote the dimensionality of the latent space as d. The effects of taking different values of α, β and d on the generalized zero-shot classification and the conventional zero-shot classification are shown in Figures 2, 3 and 4.
Figure 2 shows the effects of the parameter α on the zero-shot classification results. The parameter α is used to prevent overfitting. Taking the values of α as 0.1, 1, 10 and 100. It can be seen from Figure 2 that the classification results of the aPY dataset are decreasing and then increasing as α keeps increasing. The results of the AWA2 dataset on the conventional zero-shot classification are increasing all the time, while the values of the generalized zero-shot classification are decreasing and then increasing. The results of AWA1 dataset on the conventional zero-shot classification is decreasing and then increasing, and the harmonic mean is always increasing.
The values of β are taken as 0.001, 0.01, 0.1 and 1. β is used to regulate the relationship between the training set samples and the generated unseen class semantic features, and the value of β is taken small because the training set samples are not the real unseen class samples. From Figure 3, the accuracy of conventional zero-shot classification on the aPY dataset is almost unaffected by the value of β, but the value in the generalized zero-shot classification decreases with increasing of β. The classification accuracy of AWA1 and AWA2 on conventional zero-shot classification is also almost unaffected by the value of β, but the harmonic mean value increases and then decreases with increasing of β.
Figure 4 shows the effects of dimension d on the zero-shot classification results, with d taking values of 64,128 and 256. For the aPY dataset, the accuracy of conventional zero-shot classification increases first and then decreases as d increases, the harmonic mean value keeps decreasing. For the AWA1 dataset, the results increase and then decrease with increasing d, except for the classification results of the seen classes. For the AWA2 dataset, most of the zero-shot classification results show a trend of increasing and then decreasing with increasing d.
Figure 5 shows the tSNE for generalized zero-shot classification of the aPY dataset, where (a) and (b) denote the training set samples and unseen class samples, respectively, and (c) and (d) are the generated training set samples and unseen class samples.
For the training set samples, the distribution between the generated samples and the original samples is almost the same, and the generated samples are more dispersed between different categories and more concentrated within classes than the original samples. For the unseen class samples, there are more samples presenting orange color in the original samples, while in the generated samples, since AUS is chosen for generating the unseen class samples in this paper, it will lead to the number of some classes will be more and the number of some other classes will be less in the generated samples. Except for the inconsistent number of samples, most of the generated samples are similar to the distribution of the real samples.
The ablation experiments are divided into the following cases: a. Only Eq (1) is retained as the loss function of the model. b. Add Llatent−recon to Eq(1). c. Lcross−recon1≠0 on the basis of b. d. Lclassifier≠0 on the basis of c; e. Based on d, the second term in Lcross−recon2 is not 0; f. Based on e, Lrecon2≠0. The proposed method is to add the first term in Lcross−recon2, on the basis of f. The harmonic mean H and the accuracy acc of the conventional zero-shot classification are shown in Table 3.
AWA1 | AWA2 | aPY | ||||
acc | H | acc | H | acc | H | |
a | 45.1 | 30.0 | 45.1 | 17.9 | 30.7 | 22.0 |
b | 52.6 | 32.3 | 59.4 | 38.4 | 38.8 | 25.0 |
c | 56.5 | 33.3 | 57.6 | 23.4 | 35.2 | 26.3 |
d | 59.7 | 48.3 | 59.7 | 40.2 | 36.8 | 34.4 |
e | 61.1 | 51.4 | 60.2 | 43.8 | 36.9 | 35.1 |
f | 61.1 | 49.8 | 61.0 | 45.7 | 37.0 | 36.4 |
The proposed method | 67.1 | 63.1 | 66.1 | 63.1 | 39.8 | 40.1 |
As can be seen in Table 3, most of the classification results are increased as the term increased in the loss function. However, for the AWA1 dataset when changing from e to f, the accuracy does not change for the conventional zero-shot classification, and the harmonic mean decreases slightly. The proposed method does not increase particularly much in the conventional zero-shot classification compared to other methods, especially in the aPY dataset, and for aPY dataset, the method b is larger than other methods except the proposed method. For AWA2 when changing from b to c, the results decreases, especially in harmonic mean. The seen class information increased when we add the Lcross−recon1 and the accuracy of the unseen classes decreases. However, for generalized zero-shot classification, the proposed method can provide some information about the unseen classes when training the model, and reduce the similarity between the generated unseen class samples and the seen class samples.
By replacing ˆX in the loss function Lcross−recon2 with XS, the results are shown in Table 4 numbered as (1), and the results of the proposed model numbered as (2). From Table 4, when XS is used instead of ˆX, the results of zero-shot classification are all decreased, especially for generalized zero-shot classification. This is because the loss function contains more information about the samples of the seen classes, making the results easily biased to the seen classes.
AWA1 | AWA2 | aPY | ||||||||||
acc | ts | tr | H | acc | ts | tr | H | acc | ts | tr | H | |
(1) | 55.1 | 37.0 | 71.8 | 48.8 | 55.6 | 34.8 | 73.0 | 47.1 | 35.8 | 27.0 | 52.1 | 35.6 |
(2) | 67.1 | 62.3 | 63.9 | 63.1 | 66.1 | 60.6 | 69.5 | 64.7 | 39.8 | 31.5 | 55.3 | 40.1 |
In this study, an autoencoder approach is used for generating samples of unseen classes in zero-shot learning. For the problem that the generated unseen class sample features are always biased to the seen class features, we add the semantic features of the unseen class with the proposed new sample features to the cross-reconstruction loss function. This can reduce the information of the seen class samples and make the generated unseen class samples closer to the real unseen class samples, and improve the classification accuracy of the unseen class samples. The experimental results on three datasets verify that the proposed method can achieve good results.
The authors declare they have not used Artificial Intelligence (AI) tools in the creation of this article.
The authors declare there is no conflict of interest.
[1] | González M, Bagatolli LA, Echabe I, et al. (1997) Interaction of biotin with streptavidin. Thermostability and conformational changes upon binding. J Biol Chem 272: 11288-11294. |
[2] | Wilchek M, Bayer EA (1990) Introduction to avidin-biotin technology. Methods Enzymol 184: 5-13. |
[3] | Kurzban GP, Gitlin G, Bayer EA, et al. (1990) Biotin binding changes the conformation and decreases tryptophan accessibility of streptavidin. J Protein Chem 9: 673-682. |
[4] | Green NM (1990) Streptomyces avidinii. Methods Enzymol 184: 51-67. |
[5] | Delgadillo RF, Mueser TC, Zaleta-Rivera K, et al. (2019) Detailed characterization of the solution kinetics and thermodynamics of biotin, biocytin and HABA binding to avidin and streptavidin. Plos One 14: e0204194. |
[6] | Hyre DE, Stayton PS, Trong IL, et al. (2000) Ser45 plays an important role in managing both the equilibrium and transition state energetics of the streptavidin-biotin system. Protein Sci 9: 878-885. |
[7] | Chilkoti A, Tan PH, Stayton PS (1995) Site-directed mutagenesis studies of the high-affinity streptavidin-biotin complex: Contributions of tryptophan residues 79, 108, and 120. Proc Natl Acad Sci 92: 1754-1758. |
[8] | Klumb LA, Chu V, Stayton PS (1998) Energetic roles of hydrogen bonds at the ureido oxygen binding pocket in the streptavidin-biotin complex. Biochemistry 37: 7657-7663. |
[9] | Qureshi MH, Yeung JC, Wu SC, et al. (2001) Development and characterization of a series of soluble tetrameric and monomeric streptavidin muteins with differential biotin binding affinities. J Biol Chem 276: 46422-46428. |
[10] | Laitinen OH, Nordlund HR, Hytönen VP, et al. (2003) Rational design of an active avidin monomer. J Biol Chem 278: 4010-4014. |
[11] | Diamandis EP, Christopoulos TK (1991) The biotin-(strept) avidin system: principles and applications in biotechnology. Clin Chem 37: 625-636. |
[12] | Sano T, Glazer AN, Cantor CR (1992) A streptavidin-metallothionein chimera that allows specific labeling of biological materials with many different heavy metal ions. Proc Natl Acad Sci 89: 1534-1538. |
[13] | Chaiet L, Miller TW, Tausig F, et al. (1963) Antibiotic MSD-235. II. separation and purification of synergistic components. Antimicrob Agents Chemother 161: 28-32. |
[14] | Green NM (1975) Avidin. Adv Protein Chem 29: 85-133. |
[15] | Kuo TC, Tsai CW, Lee PC, et al. (2015) Revisiting the streptavidin-biotin binding by using an aptamer and displacement isothermal calorimetry titration. J Mol Recognit 28: 125-128. |
[16] | Pérez-Luna VH, O'Brien MJ, Opperman KA, et al. (1999) Molecular recognition between genetically engineered streptavidin and surface-bound biotin. J Am Chem Soc 121: 6469-6478. |
[17] | Lo YS, Simons J, Beebe TP (2002) Temperature dependence of the biotin−avidin bond-rupture force studied by atomic force microscopy. J Phys Chem B 106: 9847-9852. |
[18] | Neish CS, Martin IL, Henderson RM, et al. (2002) Direct visualization of ligand-protein interactions using atomic force microscopy. Br J Pharmacol 135: 1943-1950. |
[19] | Liu F, Zhang JZH, Mei Y (2016) The origin of the cooperativity in the streptavidin-biotin system: A computational investigation through molecular dynamics simulations. Sci Rep 6: 27190. |
[20] | Green N (1966) Thermodynamics of the binding of biotin and some analogues by avidin. Biochem J 101: 774-780. |
[21] | Du X, Li Y, Xia YL, et al. (2016) Insights into protein–ligand interactions: Mechanisms, models, and methods. Int J Mol Sci 17: 1-34. |
[22] | Bouchemal K (2008) New challenges for pharmaceutical formulations and drug delivery systems characterization using isothermal titration calorimetry. Drug Discov Today 13: 960-972. |
[23] | Roselin LS, Lin M-S, Lin PH, et al. (2010) Recent trends and some applications of isothermal titration calorimetry in biotechnology. Biotechnol J 5: 85-98. |
[24] | Velazquez-Campoy A, Ohtaka H, Nezami A, et al. (2004) Isothermal titration calorimetry. Curr Protoc Cell Biol 23: 17.81-17.8.24. |
[25] | Velazquez-Campoy A, Freire E (2006) Isothermal titration calorimetry to determine association constants for high-affinity ligands. Nat Protoc 1: 186-191. |
[26] | Kuo TC, Lee PC, Tsai CW, et al. (2013) Salt bridge exchange binding mechanism between streptavidin and its DNA aptamer--thermodynamics and spectroscopic evidences. J Mol Recognit 26: 149-159. |
[27] | Rajarathnam K, Rosgen J (2014) Isothermal titration calorimetry of membrane proteins-progress and challenges. Biochim Biophys Acta 1838: 69-77. |
[28] | Laemmli UK (1970) Cleavage of structural proteins during the assembly of the head of bacteriophage T4. Nature 227: 680-685. |
[29] | Gill SC, von Hippel PH (1989) Calculation of protein extinction coefficients from amino acid sequence data. Anal Biochem 182: 319-326. |
[30] | Swamy MJ (1995) Thermodynamic analysis of biotin binding to avidin. A high sensitivity titration calorimetric study. Biochem Mol Biol Int 36: 219-225. |
[31] | Privalov PL, Gill SJ (1988) Stability of protein structure and hydrophobic interaction. Adv Protein Chem 39: 191-234. |
[32] | Katz BA (1997) Binding of biotin to streptavidin stabilizes intersubunit salt bridges between Asp61 and His87 at low pH. J Mol Biol 274: 776-800. |
[33] | Hyre DE (2006) Cooperative hydrogen bond interactions in the streptavidin-biotin system. Protein Sci 15: 459-467. |
[34] | Prabhu NV, Sharp KA (2005) Heat capacity in proteins. Annu Rev Phys Chem 56: 521-548. |
[35] | Kauzmann W (1959) Some factors in the interpretation of protein denaturation. Adv Protein Chem 14: 1-63. |
[36] | Huang B, Muy S, Feng S, et al. (2018) Non-covalent interactions in electrochemical reactions and implications in clean energy applications. Phys Chem Chem Phys 20: 15680-15686. |
[37] | Yumura K, Ui M, Doi H, et al. (2013) Mutations for decreasing the immunogenicity and maintaining the function of core streptavidin. Protein Sci 22: 213-221. |
[38] | Sano T, Cantor CR (1990) Expression of a cloned streptavidin gene in Escherichia coli. PNAS 87: 142-146. |
1. | Tianshu Wei, Jinjie Huang, A Dual Discriminator Method for Generalized Zero-Shot Learning, 2024, 79, 1546-2226, 1599, 10.32604/cmc.2024.048098 |
AWA1 | AWA2 | aPY | |||||||
ts | tr | H | ts | tr | H | ts | tr | H | |
SAE [8] | 1.8 | 77.1 | 3.5 | 1.1 | 82.2 | 2.2 | 0.4 | 80.9 | 0.9 |
DAP [26] | 46.5 | 68.5 | 55.4 | 43.7 | 70.2 | 53.3 | 27.6 | 55.8 | 37.0 |
IAP [26] | 2.1 | 78.2 | 4.1 | 0.9 | 87.6 | 1.8 | 5.7 | 65.6 | 10.4 |
SJE [29] | 11.3 | 74.6 | 19.6 | 8.0 | 73.9 | 14.4 | 3.7 | 55.7 | 6.9 |
Preserving Semantic Relations (PSR) [30] | 20.7 | 73.8 | 32.3 | 13.5 | 51.4 | 21.4 | |||
f-CLSWGAN [20] | 57.9 | 61.4 | 59.6 | ||||||
Zhang et al. [31] | 20.7 | 67.9 | 38.6 | 16.1 | 66.9 | 25.9 | |||
Li et al. [11] | 54.9 | 71.7 | 62.2 | ||||||
CADA-VAE [18] | 57.3 | 72.8 | 64.1 | 55.8 | 75.0 | 63.9 | |||
Chen et al. [23] | 54.7 | 72.7 | 62.4 | 55.6 | 76.9 | 64.2 | |||
Chen et al. [12] | 54.5 | 72.8 | 62.3 | 55.2 | 73.5 | 63.0 | 26.7 | 51.5 | 35.2 |
The proposed method | 62.4 | 63.9 | 63.1 | 60.6 | 69.5 | 64.7 | 31.5 | 55.3 | 40.1 |
AWA1 | AWA2 | aPY | |
SAE [8] | 53.0 | 54.1 | 8.3 |
DAP [26] | 44.1 | 46.1 | 33.8 |
IAP [26] | 35.9 | 35.9 | 36.6 |
SJE [29] | 65.6 | 61.9 | 32.9 |
PSR [30] | 63.8 | 38.4 | |
Cross-Class Sample Synthesis (CCSS) [32] | 56.3 | 63.7 | 35.5 |
f-CLSWGAN [20] | 69.9 | ||
Zhang et al. [31] | 68.8 | 41.3 | |
Li et al. [11] | 69.9 | ||
CADA-VAE [18] | 58.8 | 60.3 | |
Chen et al. [12] | 65.2 | 65.5 | 32.7 |
The proposed method | 67.1 | 66.1 | 39.8 |
AWA1 | AWA2 | aPY | ||||
acc | H | acc | H | acc | H | |
a | 45.1 | 30.0 | 45.1 | 17.9 | 30.7 | 22.0 |
b | 52.6 | 32.3 | 59.4 | 38.4 | 38.8 | 25.0 |
c | 56.5 | 33.3 | 57.6 | 23.4 | 35.2 | 26.3 |
d | 59.7 | 48.3 | 59.7 | 40.2 | 36.8 | 34.4 |
e | 61.1 | 51.4 | 60.2 | 43.8 | 36.9 | 35.1 |
f | 61.1 | 49.8 | 61.0 | 45.7 | 37.0 | 36.4 |
The proposed method | 67.1 | 63.1 | 66.1 | 63.1 | 39.8 | 40.1 |
AWA1 | AWA2 | aPY | ||||||||||
acc | ts | tr | H | acc | ts | tr | H | acc | ts | tr | H | |
(1) | 55.1 | 37.0 | 71.8 | 48.8 | 55.6 | 34.8 | 73.0 | 47.1 | 35.8 | 27.0 | 52.1 | 35.6 |
(2) | 67.1 | 62.3 | 63.9 | 63.1 | 66.1 | 60.6 | 69.5 | 64.7 | 39.8 | 31.5 | 55.3 | 40.1 |
AWA1 | AWA2 | aPY | |||||||
ts | tr | H | ts | tr | H | ts | tr | H | |
SAE [8] | 1.8 | 77.1 | 3.5 | 1.1 | 82.2 | 2.2 | 0.4 | 80.9 | 0.9 |
DAP [26] | 46.5 | 68.5 | 55.4 | 43.7 | 70.2 | 53.3 | 27.6 | 55.8 | 37.0 |
IAP [26] | 2.1 | 78.2 | 4.1 | 0.9 | 87.6 | 1.8 | 5.7 | 65.6 | 10.4 |
SJE [29] | 11.3 | 74.6 | 19.6 | 8.0 | 73.9 | 14.4 | 3.7 | 55.7 | 6.9 |
Preserving Semantic Relations (PSR) [30] | 20.7 | 73.8 | 32.3 | 13.5 | 51.4 | 21.4 | |||
f-CLSWGAN [20] | 57.9 | 61.4 | 59.6 | ||||||
Zhang et al. [31] | 20.7 | 67.9 | 38.6 | 16.1 | 66.9 | 25.9 | |||
Li et al. [11] | 54.9 | 71.7 | 62.2 | ||||||
CADA-VAE [18] | 57.3 | 72.8 | 64.1 | 55.8 | 75.0 | 63.9 | |||
Chen et al. [23] | 54.7 | 72.7 | 62.4 | 55.6 | 76.9 | 64.2 | |||
Chen et al. [12] | 54.5 | 72.8 | 62.3 | 55.2 | 73.5 | 63.0 | 26.7 | 51.5 | 35.2 |
The proposed method | 62.4 | 63.9 | 63.1 | 60.6 | 69.5 | 64.7 | 31.5 | 55.3 | 40.1 |
AWA1 | AWA2 | aPY | |
SAE [8] | 53.0 | 54.1 | 8.3 |
DAP [26] | 44.1 | 46.1 | 33.8 |
IAP [26] | 35.9 | 35.9 | 36.6 |
SJE [29] | 65.6 | 61.9 | 32.9 |
PSR [30] | 63.8 | 38.4 | |
Cross-Class Sample Synthesis (CCSS) [32] | 56.3 | 63.7 | 35.5 |
f-CLSWGAN [20] | 69.9 | ||
Zhang et al. [31] | 68.8 | 41.3 | |
Li et al. [11] | 69.9 | ||
CADA-VAE [18] | 58.8 | 60.3 | |
Chen et al. [12] | 65.2 | 65.5 | 32.7 |
The proposed method | 67.1 | 66.1 | 39.8 |
AWA1 | AWA2 | aPY | ||||
acc | H | acc | H | acc | H | |
a | 45.1 | 30.0 | 45.1 | 17.9 | 30.7 | 22.0 |
b | 52.6 | 32.3 | 59.4 | 38.4 | 38.8 | 25.0 |
c | 56.5 | 33.3 | 57.6 | 23.4 | 35.2 | 26.3 |
d | 59.7 | 48.3 | 59.7 | 40.2 | 36.8 | 34.4 |
e | 61.1 | 51.4 | 60.2 | 43.8 | 36.9 | 35.1 |
f | 61.1 | 49.8 | 61.0 | 45.7 | 37.0 | 36.4 |
The proposed method | 67.1 | 63.1 | 66.1 | 63.1 | 39.8 | 40.1 |
AWA1 | AWA2 | aPY | ||||||||||
acc | ts | tr | H | acc | ts | tr | H | acc | ts | tr | H | |
(1) | 55.1 | 37.0 | 71.8 | 48.8 | 55.6 | 34.8 | 73.0 | 47.1 | 35.8 | 27.0 | 52.1 | 35.6 |
(2) | 67.1 | 62.3 | 63.9 | 63.1 | 66.1 | 60.6 | 69.5 | 64.7 | 39.8 | 31.5 | 55.3 | 40.1 |