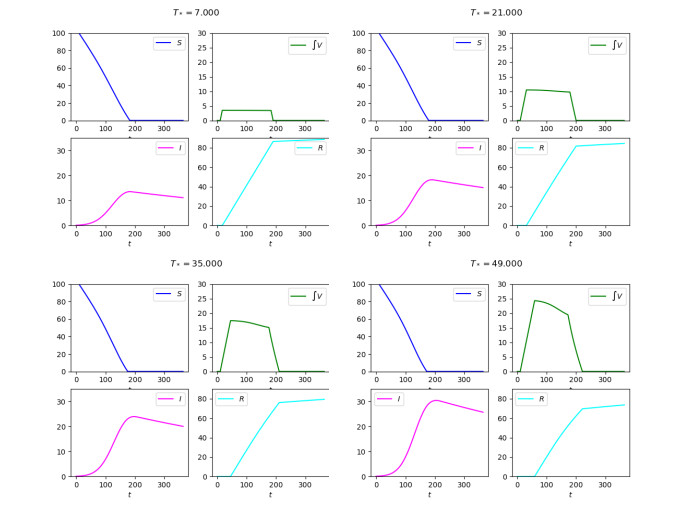
Solutions to (3)–(7)–(8) in the
This paper is devoted to a mathematical proof of the continuous dependence on the initial data for the discrete thermostatted kinetic framework, for all T > 0. This is a versatile model for describing the time-evolution of a biological complex system which is composed by a large number of interacting entities, called active particles, and is subjected to an external force field due to the environment. A thermostat term is introduced in order to keep the 2nd-order moment of the system, corresponding to the physical global activation energy, constant in time. This model is expressed by a system of nonlinear ordinary differential equations with quadratic nonlinearity.
Citation: Marco Menale, Bruno Carbonaro. The mathematical analysis towards the dependence on the initial data for a discrete thermostatted kinetic framework for biological systems composed of interacting entities[J]. AIMS Biophysics, 2020, 7(3): 204-218. doi: 10.3934/biophy.2020016
[1] | Qi Luo, Ryan Weightman, Sean T. McQuade, Mateo Díaz, Emmanuel Trélat, William Barbour, Dan Work, Samitha Samaranayake, Benedetto Piccoli . Optimization of vaccination for COVID-19 in the midst of a pandemic. Networks and Heterogeneous Media, 2022, 17(3): 443-466. doi: 10.3934/nhm.2022016 |
[2] | Prateek Kunwar, Oleksandr Markovichenko, Monique Chyba, Yuriy Mileyko, Alice Koniges, Thomas Lee . A study of computational and conceptual complexities of compartment and agent based models. Networks and Heterogeneous Media, 2022, 17(3): 359-384. doi: 10.3934/nhm.2022011 |
[3] | Xia Li, Chuntian Wang, Hao Li, Andrea L. Bertozzi . A martingale formulation for stochastic compartmental susceptible-infected-recovered (SIR) models to analyze finite size effects in COVID-19 case studies. Networks and Heterogeneous Media, 2022, 17(3): 311-331. doi: 10.3934/nhm.2022009 |
[4] | Eiman, Kamal Shah, Muhammad Sarwar, Thabet Abdeljawad . On rotavirus infectious disease model using piecewise modified $ ABC $ fractional order derivative. Networks and Heterogeneous Media, 2024, 19(1): 214-234. doi: 10.3934/nhm.2024010 |
[5] | Ryan Weightman, Temitope Akinode, Benedetto Piccoli . Optimal control of pandemics via a sociodemographic model of non-pharmaceutical interventions. Networks and Heterogeneous Media, 2024, 19(2): 500-525. doi: 10.3934/nhm.2024022 |
[6] | Guillaume Cantin, Cristiana J. Silva, Arnaud Banos . Mathematical analysis of a hybrid model: Impacts of individual behaviors on the spreading of an epidemic. Networks and Heterogeneous Media, 2022, 17(3): 333-357. doi: 10.3934/nhm.2022010 |
[7] | Xavier Blanc, Claude Le Bris, Frédéric Legoll, Tony Lelièvre . Beyond multiscale and multiphysics: Multimaths for model coupling. Networks and Heterogeneous Media, 2010, 5(3): 423-460. doi: 10.3934/nhm.2010.5.423 |
[8] | Richard Carney, Monique Chyba, Taylor Klotz . Using hybrid automata to model mitigation of global disease spread via travel restriction. Networks and Heterogeneous Media, 2024, 19(1): 324-354. doi: 10.3934/nhm.2024015 |
[9] | Yimamu Maimaiti, Zunyou Lv, Ahmadjan Muhammadhaji, Wang Zhang . Analyzing vegetation pattern formation through a time-ordered fractional vegetation-sand model: A spatiotemporal dynamic approach. Networks and Heterogeneous Media, 2024, 19(3): 1286-1308. doi: 10.3934/nhm.2024055 |
[10] | Xia Li, Andrea L. Bertozzi, P. Jeffrey Brantingham, Yevgeniy Vorobeychik . Optimal policy for control of epidemics with constrained time intervals and region-based interactions. Networks and Heterogeneous Media, 2024, 19(2): 867-886. doi: 10.3934/nhm.2024039 |
This paper is devoted to a mathematical proof of the continuous dependence on the initial data for the discrete thermostatted kinetic framework, for all T > 0. This is a versatile model for describing the time-evolution of a biological complex system which is composed by a large number of interacting entities, called active particles, and is subjected to an external force field due to the environment. A thermostat term is introduced in order to keep the 2nd-order moment of the system, corresponding to the physical global activation energy, constant in time. This model is expressed by a system of nonlinear ordinary differential equations with quadratic nonlinearity.
In this paper we propose an epidemiological compartmental model where the efficacy time of vaccinations, i.e., the time a dosed individual needs to become immune, plays a key role. Furthermore, we also account for the concurrent effect of different vaccines, differing, for instance, in the time they need to provide immunization. The role of age can also be accounted for, letting vaccines' efficacy and immunization times depend on age, as well as on the choice of the vaccine type. These features are well known to be relevant in the present Covid-19 pandemic.
Compartmental models are a formidable tool in the description of a variety of real situations. The techniques above suggest a general framework able to introduce a specific dynamic evolution within compartments. In these models, each individual is considered to be of one compartment at any given time, its evolution consisting in passing from one compartment to another one, based on the structure and on the parameters of the model. In other words, the global dynamics consist in individuals entering the system (e.g., newborns), others leaving the system (e.g., casualties) and, during the evolution, passing from one compartment to another (e.g., getting ill, being vaccinated, recovering,
In the context of epidemiological models describing the spread of infectious diseases, the SIR model, named after its three compartments (Susceptible, Infected and Recovered), is the traditional prototype. This epidemic model dates back to 1927, see [16], less than a decade after the 1918 influenza pandemic. In what is probably its simplest form [22,§10.2], a prototype SIR model reads
S→I→R↓{˙S=−ρIS˙I=ρIS−(ϑ+μ)I˙R=ϑI | (1) |
where
The popularity of (1) is due partly to its simplicity, which allows to describe the disease behavior by estimating a small number of parameters, and partly to its being amenable to a variety of extensions. The dynamics of the SIR epidemic model are widely considered, here we recall for instance [1,4,21,25], or [14,Chapter 6], [22,Chapter 10], and [23,§1.5.1].
The literature on SIR-type models, typically containing additional compartments, is indeed huge. For instance, SEIR and SEIRS-type models contain also the compartment E (Exposed) where infected individuals spend a latent/incubation period prior to become infective, see [12,13,18,19] and the references therein. Vaccination campaigns and effects are described in SVIR-type models which consider individuals that get vaccinated, see [9,17,20] or also the different approach in [5].
Recall also, for instance, the SIHR model proposed in [6], where infected individuals are either Infective (I) or Hospitalized (H), the former ones spreading the disease, while the latter ones being isolated, typically hospitalized or in quarantine, thus taking into account lockdown effects.
Since 2020, with the Covid-19 pandemic, the development and use of these models boomed. The need to explicitly introduce the immunization time of a vaccine and the concurrent use of different vaccines lead us to introduce a dynamics within compartments. This machinery allows to fix a priori the time an individual spends in a compartment, as in the case of the immunization time of a vaccine, see §2, allowing also this time to be age dependent, as in §3. This general framework is sufficiently flexible to account for the simultaneous adoption of different vaccines, as in model (4).
In other instances, it might be appropriate to smoothen the change of status related to the change of compartment, an example being the SEIR model as modified in §4. Indeed, pass from (15) to (16) allows to account for a somewhat continuous evolution from exposed to infective. In all these examples, key statistics, such as the basic reproduction number, are naturally extended to these new frameworks keeping their original meaning.
Worth mentioning is the recent SIDARTHE model from [11], consisting of
In §6, the SIHR model is modified to comprehend spatial (geographic) movements. The introduction of the age structure, where advisable, is in general possible, as shown in §7. These two steps, namely the introduction of space and age structures, are indeed doable in all the examples discussed.
Remark that the present framework indeed provides an extension to the current compartmental modeling habits. In fact, suitable choices of various functions or parameters allow to trivialize intra–compartmental dynamics, thus recovering known models.
Pandemic dynamics can clearly be described through many other tools. For instance, the recent work [2] proposes a multiscale approach and discusses the current kinetic literature on the subject. Cellular automata are used for instance in [10] in a probabilistic setting, still with a compartmental structure, see [10,Figure 1 (b)]. The literature also offers essays correlating pandemics to other human activities, primarily to economy, see for instance [8].
In the effective application of the models discussed below, a key issue is parameters' estimation. We defer for instance to [24] and to [27] for two entirely different approaches.
From the analytic point of view, a rigorous abstract approach to these classes of models is possible but at the cost of a quite intricate PDE based formalism, see a first attempt in [7]. Therefore, the sequel is devoted to show that intra-compartmental dynamics can both refine usual models, and also take into account new features, not captured by standard compartmental models.
The model presented in [20], here slightly modified, amounts to this extension of (1):
V↗↓↘S→I→R↓{˙S=−ρSIS−p(t,S)˙V=p(t,S)−ρVIV−ϑVV˙I=(ρSS+ρVV)I−(ϑI+μ)I˙R=ϑII+ϑVV. | (2) |
Here,
Before introducing the immunization time in (2), we remark that setting
The effect of doses, as is well known, is not immediate. On the contrary, the time
V(0)→V(T∗)↗↓↘S→I→R↓{˙S=−ρSIS−p(t,S)∂tV+∂τV=−ρVIV˙I=(ρSS+∫T∗0ρVV)I−ϑI−μI˙R=ϑI+V(t,T∗)V(t,0)=p(t,S(t)). | (3) |
Here, the number of individuals at time
In connection with the present Covid-19 pandemic, we remark that model (3) can be easily extended to the case of different, say
Vℓ(0)→Vℓ(Tℓ∗)ℓ=1,…,m↗↓↘S→I→R↓{˙S=−ρSIS−m∑ℓ=1pℓ(t,S)∂tVℓ+∂τVℓ=−ρVℓIVℓ˙I=(ρSS+m∑ℓ=1∫Tℓ∗0ρVℓVℓ)I−(ϑ+μ)I˙R=ϑI+m∑ℓ=1Vℓ(t,Tℓ∗)Vℓ(t,0)=pℓ(t,S(t)). | (4) |
Note that, as is to be expected, according to (4) the total number of individuals is affected only by mortality, in the sense that
ddt(S(t)+m∑ℓ=1∫Tℓ∗0Vℓ(t,τ)dτ+I(t)+R(t))=−μI(t). |
A parameter often used to describe the tendency of the pandemic is the (time dependent) basic reproduction number
Ro(t)=ρSS(t)+m∑ℓ=1∫Tℓ∗0ρVℓ(τ)Vℓ(t,τ)dτϑ+μ, | (5) |
so that the increasing in the infected individuals at time
ddtI(t)≷0⟺Ro(t)≷1. | (6) |
The above equivalence holds also in the case of the parameters
At first sight, the numerator in the right hand side of (5) is misleading, for it apparently implies that higher values of
A direct comparison between (2) and (3), though suggestive, is inevitably highly arbitrary. Clearly,
Thus, below, to investigate the role of
1
ρS=5×10−4ϑ=1×10−3μ=1×10−4ρV(τ)=ρS√1−τT∗p(t,S)=0.5χ[10,+∞[(t)χR+(S) | (7) |
and initial data
So=99.9,Vo(τ)≡0,Io=0.1,Ro=0. | (8) |
We consider
Times necessary for the vaccination to provide immunity and corresponding casualties according to model (3)–(7)–(8). The initial total population is
1 | 7 | 14 | 21 | 28 | 35 | 42 | 49 | |
Deaths: | 0.28 | 0.32 | 0.37 | 0.43 | 0.49 | 0.56 | 0.63 | 0.70 |
The relevant differences in the diagram of the map
In Figure 1, the differences in the
The evolutions in the
The flexibility introduced by dynamics internal to the dosed (
p(t,S)=0.5χ[10,30]∪[120,+∞[(t)χR+(S). | (9) |
Then, the number of casualties sharply grows with respect to the number of casualties in (3)–(7)–(8), as clearly shown when comparing Table 1 with Table 2.
Times necessary for the vaccination to provide immunity and corresponding casualties, according to model (3)–(7)–(8), in the case vaccinations are suspended as detailed in (9). The initial total population is
1 | 7 | 14 | 21 | 28 | 35 | 42 | 49 | |
Deaths: | 1.11 | 1.18 | 1.25 | 1.32 | 1.38 | 1.43 | 1.48 | 1.53 |
The diagrams in Figure 2 also confirm the negative effect in a suspension of the vaccination campaign.
As a further example, we consider the problem of choosing between two different vaccines. We integrate (4) with
T1∗=7ρV1(τ)=ρS√1−τT1∗p1(t,S)=ωχ[20,+∞[(t)χR+(S)T2∗=35ρV2(τ)=0.2ρS√1−τT2∗p2(t,S)=(0.5−ω)χ[20,+∞[(t)χR+(S), | (10) |
with the parameter
The insertion of an intra–compartmental dynamics is not limited to ODE models. As it is well known, age differences often have a role in the spreading of diseases. Therefore, we introduce the following extension of (3):
{∂tS+∂aS=−∫R+ρ(t,a,α)I(t,α)dαS−p(t,a,S)∂tV+∂aV+∂τV=−ρVIV∂tI+∂aI=∫R+ρ(t,a,α)I(t,α)dαS+∫R+∫T∗0ρV(t,a,τ,α)V(t,α,τ)dαdτI−ϑI−μI∂tR+∂aR=ϑI+V(t,a,T∗), | (11) |
Vaccination entering also as boundary datum in the evolution of the
V(t,a,0)=p(t,a,S(t,a)). | (12) |
In (11)–(12), we use the same symbols as in (3), allowing the various parameters to depend also on age. For instance
System (11) needs to be supplied with initial and boundary data, such as
{S(0,a)=So(a)V(0,a,τ)=Vo(a,τ)I(0,a)=Io(a)R(0,a)=Ro(a){S(t,0)=Sb(t)V(t,0,τ)=0I(t,0)=0R(t,0)=0 | (13) |
where, as it is realistic, we set to
Note that setting all data and parameters in (11)–(12)–(13) constant in
Extending the analogous property valid for system (3), note that the total number of individuals varies not only due to mortality but also due to the natality
ddt∫R+(S(t,a)+∫T∗0V(t,a,τ)dτ+I(t,a)+R(t,a))da=−∫R+μ(t,a)I(t,a)da+Sb(t). |
The present model realistically allows infection to be propagated across different ages. Therefore, we can only have an index
Ro(t)=∫R+∫R+(ρ(t,α,a)S(t,α)+∫T∗0ρV(t,a,τ,α)V(t,α,τ)dτ)dαI(t,a)da∫R+(ϑ(t,a)+μ(t,a))I(t,a)da |
we have the following extension of (6):
ddt∫R+I(t,a)da≷0⟺Ro(t)≷1. | (14) |
Remark that also model (11) can easily be extended to the case of different vaccines having different full efficacy times through the introduction of different vaccinated populations
The use of model (11)–(12)–(13) allows to tackle the key issue of optimizing the use of the available vaccines on the basis of their different efficacy on different age classes.
The description provided by (1) is often too approximate. For instance, it might be necessary to distinguish between exposed (
S→E→I→R↓↓{˙S=−ρIS˙E=ρIS−(ϑE+μE+κ)E˙I=κE−(ϑI+μI)I˙R=ϑEE+ϑII, | (15) |
where we introduced the term
Assume now that individuals infected at time
S→I(0)→I(τ)→→R↓{˙S=−∫R+ρ(τ)I(t,τ)dτS∂tI+∂τI=−ϑ(τ)I−μ(τ)I˙R=∫ϑ(τ)I(t,τ)dτI(t,0)=∫R+ρ(τ)I(t,τ)dτS(t). | (16) |
Observe that the last line above is in fact a boundary condition, prescribing how many individuals get infected at time
Remark that the dependence of
Observe that, as is to be expected, the variation in the total number of individuals resulting from (16) is due exclusively to mortality. Indeed
ddt(S(t)+∫R+I(t,τ)dτ+R(t))=−∫R+μ(τ)I(t,τ)dτ. | (17) |
Within the framework of (16), an explicit expression for the basic reproduction number
Ro(t)=∫R+ρ(τ)I(t,τ)dτS(t)∫R+(ϑ(τ)+μ(τ))I(t,τ)dτ |
and it is immediate to see that its exceeding unity is equivalent to the increase of the total number of infected individuals:
ddt(∫R+I(t,τ)dτ)≷0⟺Ro(t)≷1. | (18) |
It goes without saying that suitable choices of initial data and parameters in (16) allow to recover within (16) the solutions of (1). Here we only provide a quick example of a comparison among solutions to (1), (15) and (16), with data and parameters in (1) being an average of those in (15), while the choices in (16) are an interpolation of those in (15), as detailed in (19).
(1)(15)(16)ρ=0.1ρ=0.1ρ(τ)=0.1(1−e−0.2τ)ϑ=3(ϑE,ϑI)=(7,1)ϑ(τ)={7τ<21τ≥2μ=0.5(μE,μI)=(0.1,0.9)μ(τ)=0.9−0.8e−2τκ=5So=10So=10So=10Io=6.(Eo,Io)=(1,5)Io(τ)={0.5τ∈[0,2[2.5τ∈[2,4]Ro=0Ro=0Ro=0. | (19) |
Figure 4 shows sample integrations of (1), (15) and (16). In the situation considered, according to mortality, the mixed ODE–PDE system (16) can be seen in some senses in the middle between the other two classical purely ODE based compartmental models (1) and (15). Intra-compartmental dynamics can thus recover the dynamics described through standard compartmental models.
Above, the integrations of (1) and (15), below on the left that of (16) (19). The rightmost diagram on the second line displays the total number of living individuals in the three cases, showing that, with respect to mortality, the ODE–PDE model (16) can be seen in some senses in the middle between the ODE models (1) and (15)
.On the other hand, other scenarios can hardly be recovered through the use of only standard compartments. Indeed, for instance, it can be reasonable to assume that infected individuals are most infective in given time intervals after infection where, in general, these intervals depend on the specific disease under consideration. Such a situation, within Model (16), is easily described and we consider, for instance, the following choices:
ρ(τ)=ˉρte−α(t−ˉT)2 with ρ=0.1 and (i)α=1.0,ˉT=10.0,(ii)α=1.0,ˉT=4.0,(iii)α=0.25,ˉT=4.0. | (20) |
The sample choices (20) differ in the time
The results are summarized in Figure 5. The differences in the evolutions prescribed by (16) corresponding to the choices (20) are very intuitive, Case
Above, from left to right, the integrations of Case
We now consider the SIDARTHE model introduced in [11]. It consists of
Populations in model (21) from [11]
.Susceptible | healthy | can be infected | ||
Infected | asymptomatic | infective | undetected | |
Diagnosed | asymptomatic | infective | detected | |
Ailing | symptomatic | infective | undetected | |
Recognized | symptomatic | infective | detected | |
Threatened | acutely symptomatic | infected | detected | |
Healed | healthy | immune | ||
Extinct |
Its block diagram and the corresponding system of ordinary differential equations read:
S→I→D↙↓↓↘H←A→R↘↑↘↓↘↓↑E←T→↓↑↓←←←←←←←{˙S=−αIS−γAS−βDS−δRS˙I=αIS+γAS+βDS+δRS−(ε+ζ+λ)I˙D=εI−(η+ρ)D˙A=ζI−(ϑ+μ+κ)A˙R=ηD+ϑA−(ν+ξ)R˙T=μA+νR−(σ+τ)T˙H=λI+ρD+κA+ξR+σT˙E=τT. | (21) |
We refer to [11] for the specific meaning of each constant and for the motivations of this model.
Here, we note that both the evolutions
(22) |
Introduce now the populations
S→I(0)→I(s)→→R(0)→R(s)→→E↘↙ ⟶H⟵ | (23) |
{˙S=−∫α(s)I(t,s)dsS−∫δ(s)R(t,s)dsS∂tI+∂sI=∫α(s)I(t,s)dsS+∫δ(s)R(t,s)dsS−∫ε(s)I(t,s)ds−∫ϑ(s)I(t,s)ds∂tR+∂sR=∫ϑ(s)I(t,s)s−∫η(s)R(t,s)ds˙H=∫ε(s)Ids˙E=∫η(s)R(t,s)ds, | (24) |
All integrals being computed over
Note also that in model (24) the explicit expression of the basic reproduction number is immediately at hand. Indeed, defining
Ro(t)=∫(α(s)I(t,s)+δ(s)R(t,s))dsS(t)∫(ε(s)+ϑ(s))I(t,s)ds |
the key property (18) still holds.
As a further example of intra-compartmental dynamics we consider movements. Indeed, as a prototype for the description of the role of quarantine in the spreading of a pandemic, consider the model [6,Formula (9)]
S→I↘↓RH↗{∂tS+μSS=−ρIS∂tI+μII=ρIS−κI−ϑI∂tH+μHH=κI−ηH∂tR+μRR=ϑI+ηH, | (25) |
where we used the standard notation for the
In this connection, it can be relevant to take into consideration events that provoked relevant gatherings of crowds, a well known example being the Atalanta vs. Valencia football match played in Milan (Italy) on February 19th, 2020, see [26]. To this aim, following [6], we introduce a geographical movement as follows
S(x)↺→I(x)↺↘↓R(x)↺H↗{∂tS+div(vSS)+μSS=−ρIS∂tI+div(vII)+μII=ρIS−κI−ϑI∂tH+μHH=κI−ηH∂tR+div(vRR)+μRR=ϑI+ηH. | (26) |
Above, all populations are also space dependent, so that, for instance,
Introducing age structure in the SIS model [15], see also [3,Formula 10.1], we get
S⟷I{∂tS+∂aS=−ρIS+γI∂tI+∂aI=ρIS−γI. | (27) |
In this model, when infected individuals recover, they get back to being susceptible at a rate governed by the, possibly age dependent, parameter
S⟶⟶I↖↙R(0)→R(T∗){∂tS+∂aS=−ρIS+R(t,a,T∗(a))∂tI+∂aI=ρIS−γI∂tR+∂aR+∂τR=0R(t,a,0)=γI(t,a). | (28) |
Note however that the very simple nature of the third equation allows to rewrite (28) as a system of
{∂tS+∂aS=−ρIS+γI(t−T∗(a),a−T∗(a))∂tI+∂aI=ρIS−γI(t,a). | (29) |
The form (28) is more prone to extensions than (29), since it allows to specify various evolutions of the
From the analytic point of view, we remark that (28) provides a first minimal example of a "junction" in
[1] |
Bianca C, Riposo J (2015) Mimic therapeutic actions against keloid by thermostatted kinetic theory methods. Eur Phys J Plus 130: 159. doi: 10.1140/epjp/i2015-15159-4
![]() |
[2] |
Bonabeau E, Theraulaz G, Deneubourg JL (1996) Mathematical model of self-organizing hierarchies in animal societies. B Math Biol 58: 661-717. doi: 10.1007/BF02459478
![]() |
[3] |
Choi B, Rempala GA (2012) Inference for discretely observed stochastic kinetic networks with applications to epidemic modeling. Biostatistics 13: 153-165. doi: 10.1093/biostatistics/kxr019
![]() |
[4] |
Conte M, Gerardo-Giorda L, Groppi M (2020) Glioma invasion and its interplay with nervous tissue and therapy: A multiscale model. J Theor Biol 486: 110088. doi: 10.1016/j.jtbi.2019.110088
![]() |
[5] |
Gray A, Greenhalgh D, Hu L, et al. (2011) A stochastic differential equation SIS epidemic model. SIAM J Appl Math 71: 876-902. doi: 10.1137/10081856X
![]() |
[6] |
Hethcote HW (2000) The mathematics of infectious diseases. SIAM Rev 42: 599-653. doi: 10.1137/S0036144500371907
![]() |
[7] |
Masurel L, Bianca C, Lemarchand A (2018) On the learning control effects in the cancer-immune system competition. Physica A: Stat Mech Appl 506: 462-475. doi: 10.1016/j.physa.2018.04.077
![]() |
[8] | Krause J, Ruxton GD, Ruxton GD, et al. (2002) Living in Groups Oxford: Oxford University Press. |
[9] | Poleszczuk J, Macklin P, Enderling H (2016) Agent-based modeling of cancer stem cell driven solid tumor growth. Stem Cell Heterogeneity New York: Humana Press, 335-346. |
[10] |
Rybokonenko D, Balakhontceva M, Voloshin D, et al. (2015) Agent-based modeling of crowd dynamics on a moving platform. Procedia Comput Sci 66: 317-327. doi: 10.1016/j.procs.2015.11.037
![]() |
[11] |
Spina S, Giorno V, Román-Román P, et al. (2014) A stochastic model of cancer growth subject to an intermittent treatment with combined effects: Reduction in tumor size and rise in growth rate. B Math Biol 76: 2711-2736. doi: 10.1007/s11538-014-0026-8
![]() |
[12] |
Anderson PW (2011) More and Different: Notes from a Thoughtful Curmudgeon World Scientific. doi: 10.1142/8141
![]() |
[13] | Bar-Yam Y (1997) Dynamics of Complex Systems CRC Press. |
[14] |
Cilliers P, Spurrett D (1999) Complexity and postmodernism: Understanding complex systems. S Afr J Philos 18: 258-274. doi: 10.1080/02580136.1999.10878187
![]() |
[15] |
Holland JH (2006) Studying complex adaptive systems. J Syst Sci Complex 19: 1-8. doi: 10.1007/s11424-006-0001-z
![]() |
[16] |
Cercignani C (1988) The boltzmann equation. the Boltzmann Equation and its Applications New York: Springer. doi: 10.1007/978-1-4612-1039-9
![]() |
[17] |
Bianca C (2012) Thermostated kinetic equations as models for complex systems in physics and life sciences. Phys life Rev 9: 359-399. doi: 10.1016/j.plrev.2012.08.001
![]() |
[18] | Bianca C (2012) An existence and uniqueness theorem to the Cauchy problem for thermostatted-KTAP models. Int J Math Anal 6: 813-824. |
[19] | Bianca C (2012) Kinetic theory for active particles modelling coupled to Gaussian thermostats. Appl Math Sci 6: 651-660. |
[20] | Bianca C (2012) Modeling complex systems by functional subsystems representation and thermostatted-KTAP methods. Appl Math Inform Sci 6: 495-499. |
[21] |
Wennberg B, Wondmagegne Y (2006) The Kac equation with a thermostatted force field. J Stat Phys 124: 859-880. doi: 10.1007/s10955-005-9020-8
![]() |
[22] |
Hartwell LH, Hopfield JJ, et al. (1999) From molecular to modular cell biology. Nature 402: C47-C52. doi: 10.1038/35011540
![]() |
[23] |
Woese CR (2004) A new biology for a new century. Microbiol Mol Biol Rev 68: 173-186. doi: 10.1128/MMBR.68.2.173-186.2004
![]() |
[24] |
Augere P, de La Parra RB, et al. (2008) Aggregation methods in dynamical systems and applications in population and community dynamics. Phys Life Rev 5: 79-105. doi: 10.1016/j.plrev.2008.02.001
![]() |
[25] | Perthame B (2006) Transport Equations In Biology Springer Science & Business Media. |
[26] | Webb GF (1985) Theory of Nonlinear Age-Dependent Population Dynamics CRC Press. |
[27] |
Chowell G, Nishiura H (2008) Quantifying the transmission potential of pandemic influenza. Phys Life Rev 5: 50-77. doi: 10.1016/j.plrev.2007.12.001
![]() |
[28] |
Delitala M (2004) Generalized kinetic theory approach to modeling spread and evolution of epidemics. Math Comput Model 39: 1-12. doi: 10.1016/S0895-7177(04)90501-8
![]() |
[29] | Diekmann O, Heesterbeek JAP (2000) Mathematical epidemiology of infectious diseases. Model building, analysis 5. |
[30] |
Thieme HR (2018) Mathematics in Population Biology Princeton: Princeton University Press. doi: 10.2307/j.ctv301f9v
![]() |
[31] |
Becker N (1979) The uses of epidemic models. Biometrics 35: 295-305. doi: 10.2307/2529951
![]() |
[32] | Dietz K, Schenzle D (1985) Mathematical models for infectious disease statistics. A Celebration of Statistics New York: Springer. |
[33] | Hethcote HW (1994) A thousand and one epidemic models. Frontiers in Mathematical Biology Berlin: Springer. |
[34] |
Hethcote HW, Van Ark JW (1987) Epidemiological models for heterogeneous populations: proportionate mixing, parameter estimation, and immunization programs. Math Biosci 84: 85-118. doi: 10.1016/0025-5564(87)90044-7
![]() |
[35] | Hethcote HW, Stech HW, van den Driessche P (1981) Periodicity and stability in epidemiological models: a survey. Differential Equations and Applications in Ecology, Epidemiology, and Population Problems New York: Academic Press. |
[36] | Hethcote HW, Levin SA (1989) Periodicity in epidemiological models. Applied Mathematical Ecology Berlin: Springer. |
[37] |
Bertram D, Toscani G (2007) Hydrodynamics from kinetic models of conservative economies. Physica A 384: 493-506. doi: 10.1016/j.physa.2007.05.062
![]() |
[38] |
Naldi G, Pareschi L, Toscani G (2010) Mathematical Modeling of Collective Behavior in Socio-Economic and Life Sciences Springer Science & Business Media. doi: 10.1007/978-0-8176-4946-3
![]() |
[39] |
Bianca C, Mogno C (2018) Modelling pedestrian dynamics into a metro station by thermostatted kinetic theory methods. Math Comp Model Dyn 24: 207-235. doi: 10.1080/13873954.2018.1432664
![]() |
[40] |
Iannini MLL, Dickman R (2016) Kinetic theory of vehicular traffic. Am J Phys 84: 135-145. doi: 10.1119/1.4935895
![]() |
[41] |
Dogbé C (2010) Modeling crowd dynamics by the mean-field limit approach. Math Comp Model 52: 1506-1520. doi: 10.1016/j.mcm.2010.06.012
![]() |
[42] |
Carbonaro B, Serra N (2002) Towards mathematical models in psychology: A stochastic description of human feelings. Math Mod Meth Appl S 12: 1453-1490. doi: 10.1142/S0218202502002197
![]() |
[43] |
Carbonaro B, Giordano C (2005) A second step towards a stochastic mathematical description of human feelings. Math Comput Model 41: 587-614. doi: 10.1016/j.mcm.2003.05.021
![]() |
[44] |
Kacperski K (1999) Opinion formation model with strong leader and external impact: A mean field approach. Physica A 269: 511-526. doi: 10.1016/S0378-4371(99)00174-0
![]() |
[45] |
Bianca C, Mogno C (2018) Qualitative analysis of a discrete thermostatted kinetic framework modeling complex adaptive systems. Commun Nonlinear Sci 54: 221-232. doi: 10.1016/j.cnsns.2017.06.007
![]() |
[46] |
Bianca C, Menale M (2019) Existence and uniqueness of nonequilibrium stationary solutions in discrete thermostatted models. Commun Nonlinear Sci 73: 25-34. doi: 10.1016/j.cnsns.2019.01.026
![]() |
[47] |
Cooper EL (2010) Evolution of immune systems from self/not self to danger to artificial immune systems (AIS). Phys Life Rev 7: 55-78. doi: 10.1016/j.plrev.2009.12.001
![]() |
[48] |
Pappalardo F, Palladini A, Pennisi M, et al. (2012) Mathematical and computational models in tumor immunology. Math Model Nat Pheno 7: 186-203. doi: 10.1051/mmnp/20127312
![]() |
[49] |
Carbonaro B, Menale M (2019) Dependence on the initial data for the continuous thermostatted framework. Mathematics 7: 602. doi: 10.3390/math7070602
![]() |
[50] |
Dimarco G, Pareschi L (2013) Asymptotic preserving implicit-explicit Runge–Kutta methods for nonlinear kinetic equations. SIAM J Numer Anal 51: 1064-1087. doi: 10.1137/12087606X
![]() |
[51] |
Dimarco G, Pareschi L (2014) Numerical methods for kinetic equations. Acta Numer 23: 369-520. doi: 10.1017/S0962492914000063
![]() |
[52] |
Dogbe C (2008) On the numerical solutions of second order macroscopic models of pedestrian flows. Comput Math Appl 56: 1884-1898. doi: 10.1016/j.camwa.2008.04.028
![]() |
[53] |
Bianca C, Menale M (2020) Mathematical analysis of a thermostatted equation with a discrete real activity variable. Mathematics 8: 57. doi: 10.3390/math8010057
![]() |
[54] | Walter W (2012) Differential and Integral Inequalities Springer Science & Business Media. |
[55] |
Dogbe C (2012) On the modelling of crowd dynamics by generalized kinetic models. J Math Anal Appl 387: 512-532. doi: 10.1016/j.jmaa.2011.09.007
![]() |
[56] |
Gramani L (2009) On the modeling of granular traffic flow by the kinetic theory for active particles trend to equilibrium and macroscopic behavior. Int J Nonlin Mech 44: 263-268. doi: 10.1016/j.ijnonlinmec.2008.11.008
![]() |
[57] |
Derbel L (2004) Analysis of a new model for tumor-immune system competition including long-time scale effects. Math Mod Meth Appl S 14: 1657-1681. doi: 10.1142/S0218202504003738
![]() |
[58] | Gałach M (2003) Dynamics of the tumor—immune system competition—the effect of time delay. Int J Ap Mat Com-Pol 13: 395-406. |
[59] |
Kolev M (2003) Mathematical modelling of the competition between tumors and immune system considering the role of the antibodies. Math Comput Model 37: 1143-1152. doi: 10.1016/S0895-7177(03)80018-3
![]() |
1. | Rinaldo M. Colombo, Mauro Garavello, Infectious Disease Spreading Fought by Multiple Vaccines Having a Prescribed Time Effect, 2023, 71, 0001-5342, 10.1007/s10441-022-09452-4 | |
2. | Lihong Sun, Qiang He, Yueyang Teng, Qi Zhao, Xin Yan, Xingwei Wang, A complex network-based vaccination strategy for infectious diseases, 2023, 136, 15684946, 110081, 10.1016/j.asoc.2023.110081 | |
3. | R.M. Colombo, M. Garavello, M. Tandy, On the coupling of well posed differential models, 2023, 232, 0362546X, 113290, 10.1016/j.na.2023.113290 | |
4. | Rinaldo M. Colombo, Mauro Garavello, Francesca Marcellini, Elena Rossi, 2024, Chapter 28, 978-3-031-55263-2, 325, 10.1007/978-3-031-55264-9_28 |
Times necessary for the vaccination to provide immunity and corresponding casualties according to model (3)–(7)–(8). The initial total population is
1 | 7 | 14 | 21 | 28 | 35 | 42 | 49 | |
Deaths: | 0.28 | 0.32 | 0.37 | 0.43 | 0.49 | 0.56 | 0.63 | 0.70 |
Times necessary for the vaccination to provide immunity and corresponding casualties, according to model (3)–(7)–(8), in the case vaccinations are suspended as detailed in (9). The initial total population is
1 | 7 | 14 | 21 | 28 | 35 | 42 | 49 | |
Deaths: | 1.11 | 1.18 | 1.25 | 1.32 | 1.38 | 1.43 | 1.48 | 1.53 |
Populations in model (21) from [11]
.Susceptible | healthy | can be infected | ||
Infected | asymptomatic | infective | undetected | |
Diagnosed | asymptomatic | infective | detected | |
Ailing | symptomatic | infective | undetected | |
Recognized | symptomatic | infective | detected | |
Threatened | acutely symptomatic | infected | detected | |
Healed | healthy | immune | ||
Extinct |
1 | 7 | 14 | 21 | 28 | 35 | 42 | 49 | |
Deaths: | 0.28 | 0.32 | 0.37 | 0.43 | 0.49 | 0.56 | 0.63 | 0.70 |
1 | 7 | 14 | 21 | 28 | 35 | 42 | 49 | |
Deaths: | 1.11 | 1.18 | 1.25 | 1.32 | 1.38 | 1.43 | 1.48 | 1.53 |
Susceptible | healthy | can be infected | ||
Infected | asymptomatic | infective | undetected | |
Diagnosed | asymptomatic | infective | detected | |
Ailing | symptomatic | infective | undetected | |
Recognized | symptomatic | infective | detected | |
Threatened | acutely symptomatic | infected | detected | |
Healed | healthy | immune | ||
Extinct |