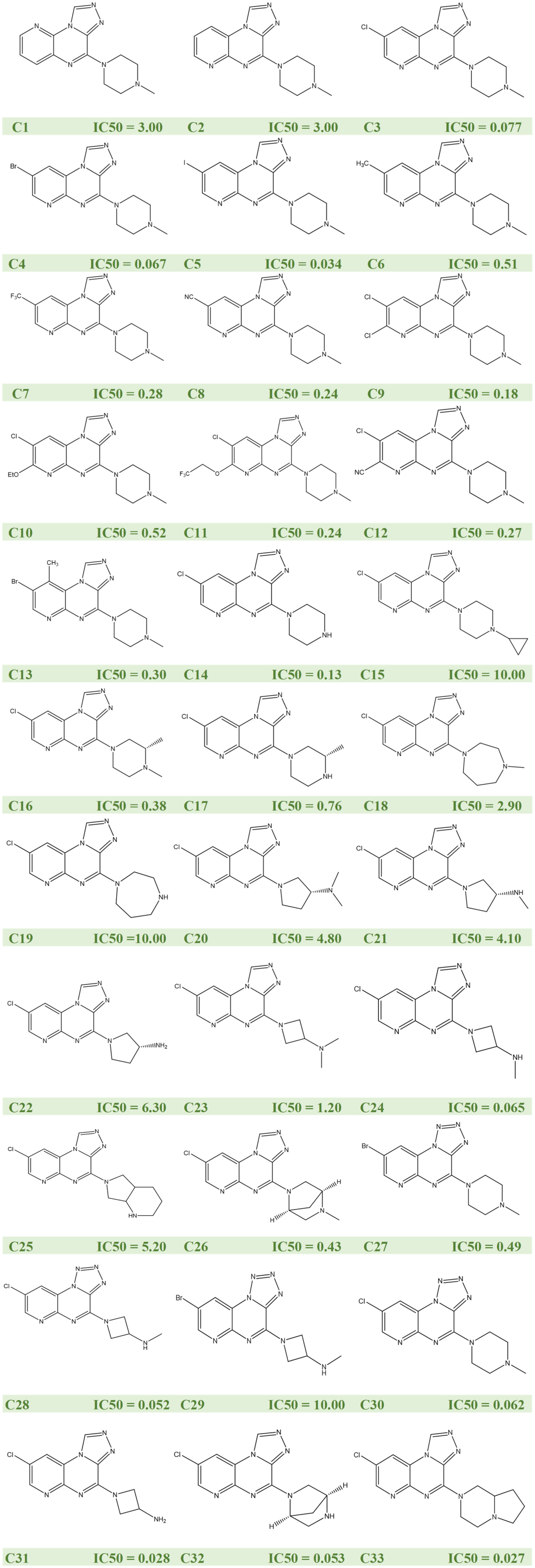
Atopic dermatitis (AD) is a prevalent inflammatory skin condition, primarily characterized by intense pruritus and chronic inflammation. Current therapeutic options targeting the histamine H4 receptor (H4R) have shown limited efficacy in addressing both pruritus and inflammation comprehensively. This study investigates pyridopyrazine derivatives as potential H4R antagonists with a focus on their suitability for AD treatment. To evaluate these compounds, we applied quantitative structure–activity relationship (QSAR) models and molecular docking techniques. A set of 33 pyridopyrazine derivatives was analyzed using principal component regression (PCR), multiple linear regression (MLR), and partial least squares (PLS) methodologies. Molecular descriptors were computed, and collinearity among descriptors was assessed through principal component analysis (PCA). Model performance was evaluated using the root mean square error (RMSE) and coefficient of determination (R2) values, providing insight into predictive accuracy. The PCR model emerged with strong predictive capabilities, showing an RMSE of 1.017 and an R2 of 0.897. Furthermore, molecular docking results indicated potent binding interactions with H4R, primarily through hydrophobic and hydrogen-bonding interactions. Notably, compound C11 demonstrated the highest binding affinity, underscoring its potential as a valuable candidate for anti-inflammatory development. In conclusion, pyridopyrazine derivatives, particularly compound C11, exhibit promising anti-inflammatory properties with specific binding efficacy to H4R, suggesting potential for advancing AD treatment options.
Citation: Mohamed El Yaqoubi, Mouad Lahyaoui, Yousra Seqqat, Taoufiq Saffaj, Bouchaib Ihssane, Nabil Saffaj, Rachid Mamouni, Fouad Ouazzani Chahdi, Fahad M Alshabrmi, Alaa Abdulaziz Alnahari, Saad M. Howladar, Ammar AL-Farga, Youssef Kandri Rodi. Pyridopyrazine derivatives as highly selective histamine H4 receptor antagonist for the treatment of atopic dermatitis: QSAR modeling and molecular docking studies[J]. AIMS Allergy and Immunology, 2024, 8(4): 303-323. doi: 10.3934/Allergy.2024019
[1] | Masahiro Seike . Histamine suppresses T helper 17 responses mediated by transforming growth factor-β1 in murine chronic allergic contact dermatitis. AIMS Allergy and Immunology, 2018, 2(4): 180-189. doi: 10.3934/Allergy.2018.4.180 |
[2] | Fatma Akpinar, Ayla Balci, Gulcan Ozomay, Ayca Sozen, Esra Kotan, Gulendam Kocak, Feyzullah Cetinkaya . Baby-skin care habits from different socio-economic groups and its impact on the development of atopic dermatitis. AIMS Allergy and Immunology, 2018, 2(1): 1-9. doi: 10.3934/Allergy.2018.1.1 |
[3] | Andrey Mamontov, Alexander Polevshchikov, Yulia Desheva . Mast cells in severe respiratory virus infections: insights for treatment and vaccine administration. AIMS Allergy and Immunology, 2023, 7(1): 1-23. doi: 10.3934/Allergy.2023001 |
[4] | Bouchra Sarah Boukhatem, Abd-Elmouneim Belhadj . In silico anti-inflammatory activity of lavender (Lavandula officinalis) essential oil bioactive compounds: Molecular docking analysis of COX-1 and COX-2, and ADMET prediction. AIMS Allergy and Immunology, 2023, 7(2): 132-153. doi: 10.3934/Allergy.2023009 |
[5] | Haiyan Guo, Mingsheng Lei, Jinan Ma, Hongchun Du, Youming Zhang . Allergy and ferroptosis. AIMS Allergy and Immunology, 2025, 9(1): 8-26. doi: 10.3934/Allergy.2025002 |
[6] | Kazunobu Baba, Akina Oomori, Tadaaki Miyazaki . Preventive effect of Enterococcus faecalis and β-glucan on the onset and exacerbation of symptoms of atopic dermatitis in mice. AIMS Allergy and Immunology, 2023, 7(3): 183-194. doi: 10.3934/Allergy.2023013 |
[7] | Manal Alkan, Fadel Sayes, Abdulraouf Ramadan, Francois Machavoine, Michel Dy, Elke Schneider, Nathalie Thieblemont . Basophil activation through TLR2 and TLR4 signaling pathways. AIMS Allergy and Immunology, 2018, 2(3): 126-140. doi: 10.3934/Allergy.2018.3.126 |
[8] | Moufag Mohammed Saeed Tayeb . Role of IgG food test in patients with allergic diseases. AIMS Allergy and Immunology, 2023, 7(2): 154-163. doi: 10.3934/Allergy.2023010 |
[9] | Ling Wang, Shunbin Ning . “Toll-free” pathways for production of type I interferons. AIMS Allergy and Immunology, 2017, 1(3): 143-163. doi: 10.3934/Allergy.2017.3.143 |
[10] | Ross M. Tanis, Piper A. Wedman-Robida, Alena P. Chumanevich, John W. Fuseler, Carole A. Oskeritzian . The mast cell/S1P axis is not linked to pre-lesional male skin remodeling in a mouse model of eczema. AIMS Allergy and Immunology, 2021, 5(3): 160-174. doi: 10.3934/Allergy.2021012 |
Atopic dermatitis (AD) is a prevalent inflammatory skin condition, primarily characterized by intense pruritus and chronic inflammation. Current therapeutic options targeting the histamine H4 receptor (H4R) have shown limited efficacy in addressing both pruritus and inflammation comprehensively. This study investigates pyridopyrazine derivatives as potential H4R antagonists with a focus on their suitability for AD treatment. To evaluate these compounds, we applied quantitative structure–activity relationship (QSAR) models and molecular docking techniques. A set of 33 pyridopyrazine derivatives was analyzed using principal component regression (PCR), multiple linear regression (MLR), and partial least squares (PLS) methodologies. Molecular descriptors were computed, and collinearity among descriptors was assessed through principal component analysis (PCA). Model performance was evaluated using the root mean square error (RMSE) and coefficient of determination (R2) values, providing insight into predictive accuracy. The PCR model emerged with strong predictive capabilities, showing an RMSE of 1.017 and an R2 of 0.897. Furthermore, molecular docking results indicated potent binding interactions with H4R, primarily through hydrophobic and hydrogen-bonding interactions. Notably, compound C11 demonstrated the highest binding affinity, underscoring its potential as a valuable candidate for anti-inflammatory development. In conclusion, pyridopyrazine derivatives, particularly compound C11, exhibit promising anti-inflammatory properties with specific binding efficacy to H4R, suggesting potential for advancing AD treatment options.
atopic dermatitis;
accessible surface area (positive charge);
accessible surface area (negative charge);
accessible surface area (polar);
atom site violation descriptor;
histamine H4 receptor;
inhibitory concentration 50%;
immunoglobulin E;
multiple linear regression;
negative logarithm of IC50;
principal component analysis;
principal component regression;
partial least squares;
quantitative structure–activity relationship;
coefficient of determination;
root mean square error;
resonance synthetic descriptor;
T-helper (e.g., TH1, TH17, TH22 cytokines);
Vertex Adjacency Equality Index;
molecular surface properties descriptor;
VSURF coefficient of polarizability;
VSURF hydrophobic constant (Cavity 4);
VSURF Hydrophilic–Lipophilic Index 1;
VSURF Hydrophilic–Lipophilic Index 2;
Wiener Polarity Index (descriptor of molecular branching);
software for statistical analysis;
Zagreb Index Descriptor (topological descriptor)
Atopic dermatitis (AD) is a common, long-term allergic skin condition affecting individuals of all ages. Characterized by pruritic and inflamed skin, AD develops through complex interactions between genetic predispositions, immune dysregulation, and environmental factors. The primary causes include abnormalities in skin cell development and an overactive immune response, which activate specific cytokine pathways [1], such as TH22, TH17/IL-23, and TH1, varying across AD subtypes [2]–[4]. Recent advancements in understanding AD's pathogenesis have shifted the focus from identifying causes to developing effective treatments [3]. Biological therapies targeting specific immune pathways have shown promise by addressing mechanisms such as skin barrier defects, skin dysbiosis [5], and abnormal immune responses [6],[7]. Due to AD's complex nature and its potential impact on overall health [8], accurate diagnosis and targeted therapeutic interventions are essential [9].
Pyridopyrazine derivatives display a diverse range of pharmacological activities. Studies have shown these compounds exhibit anti-inflammatory effects by modulating specific pathways [10], although the mechanisms are complex and can involve both anti-inflammatory and pro-inflammatory processes depending on the context [11]–[13]. Additionally, their antioxidant properties protect cells from oxidative stress, showcasing their therapeutic potential. Pyridopyrazines also have antimicrobial activity, neurological implications through neurotransmitter system modulation, and even anti-cancer effects, suggesting applications in oncology [14]–[16]. However, the exact pathways through which they influence cancer cells remain under investigation. With cardiovascular effects noted in several studies, pyridopyrazine compounds represent a pharmacologically versatile class with broad therapeutic implications [17]–[21].
This study investigates pyridopyrazine derivatives as potential antagonists of the histamine H4 receptor (H4R) for AD treatment. We applied quantitative structure–activity relationship (QSAR) analysis and molecular docking to assess their suitability [22]–[24]. In QSAR analysis, IC50 values measure the inhibitory activity of each compound, with values converted to pIC50 to facilitate correlation with molecular descriptors. Through statistical models—principal component regression (PCR), multiple linear regression (MLR), and partial least squares (PLS)—we analyzed descriptors to understand how structural factors influence activity. QSAR aims to streamline structural data analysis and pinpoint molecular features critical to activity, though accurately predicting the behavior of new compounds remains a significant challenge [25]–[28].
Molecular docking complements QSAR by modeling compound–receptor interactions, specifically examining binding modes and affinities with H4R at the active site of protein 7YFD [29]–[31]. Through this dual approach, combining QSAR's predictive capabilities with docking's structural insights, we aim to identify derivatives with enhanced efficacy as potential AD treatments [32],[33]. Compound C11 demonstrated a particularly high binding affinity to H4R, highlighting it as a promising candidate for anti-inflammatory drug development and advancing targeted AD therapies [34].
Ko et al. [35] designed a series of pyridopyrazine compounds to illustrate their potential as a treatment for atopic dermatitis (H4R) (Figure 1). The compounds were also evaluated for their effectiveness as a histamine H4 receptor antagonist.
Achieving a statistically robust model depends on the efficacy of the descriptors, which are based on a systematic and mathematical methodology [36] to describe the variation of activity with respect to the molecular structure. The kind of chemical representation and the technique designed to compute and predict the correlation within the 33 chemical derivatives and their inhibiting activity usually determine the information contained in the descriptors.
In order to establish a correlation between the inhibitory activity and the chemical structures of the investigated compounds, we utilized Python to compute additional molecular descriptors, the detailed results of which are presented in Table S1. In order to provide an accurate representation of the QSAR models, statistical techniques including PCR, MLR, and PLS were applied in the development of the QSAR relationship.
In the pursuit of constructing a QSAR model, a subset of 33 chemicals renowned for their potent inhibitory activity, as reported in previous studies, was chosen. The XLSTAT program was employed to randomly partition the entire set into a test set (7 compounds) for model validation and a training set (26 compounds) for model construction. The proposed methodology incorporates PCA to assess redundancy and collinearity among the studied descriptors [37]–[40]. To find a relationship between activity and molecular structure, three statistical models (PLS, PCR, and MLR) were compared statistically. Once the computations were completed using the 2014 version of the XLSTAT program, the selected descriptors were used to create statistical models that relate the activity of the chemicals to their chemical structures. The dataset was split into two subsets: a test set that was used to evaluate the performance of the derived models and a training set that was used to establish the three models.
The core step in a QSAR investigation is model computation; however, this alone cannot guarantee the model's validity. An external validation procedure is needed to ascertain the model's capacity to predict new compounds. Confirming that the model was not created at random is also crucial. For this test, we used the QSAR models that were created to estimate the activities of the chemicals in the test set. Although it was not involved in the creation of the QSAR models, the latter incorporates compounds from the series of molecules examined in this study. The external ability of the QSAR models to forecast the activity of the test set molecules was assessed by computing the R2 coefficient between the predicted and observed pIC50 values after the test set was included. Globarikh and Tropsha [41] stated that determining the result of the R2 test is useful for external validation of QSAR models. This explanation states that when the R2 test result is higher than 0.5, the model is statistically adequate for prediction and may be applied to additional external data [42].
The scoring results from the molecular docking procedure were easier to calculate and publish due to the modeling software. ChemDraw (18.2) was used to create molecular structures. The Protein Data Bank provided pertinent crystal structures for atopic dermatitis, including the Cryo-EM structure of the histamine H4 receptor and the Gq complex that is attached to it (PDB code: 7YFD) (https://www.rcsb.org/structure/7YFD). Water-binding ligands and cofactors were eliminated from the protein structure in order to improve the structure, and hydrogen atoms were subsequently introduced to stabilize it. Active sites were selectively excluded to create fictitious atoms. The MMFF94x force field was then used to assign the parameters and charges. The docking module made it easier to connect molecular models to the protein surface, and the alpha site search module was used to create alpha site spheres. Dock scoring within the program used the London dG scoring function, followed by refinement using two independent techniques. Self-turning docks were applied to the dock poses, which were selected based on high scores. Using the database browser, the docking postures were aligned with the co-crystallized structure's ligand, and the docking pose's root mean square deviation (RMSD) was calculated.
To assess the binding affinity, binding free energy, and formation of hydrogen bonds, calculations were performed for the synthesized molecules and the amino acid residues of the receptor. The default-docking model identified several forms of interactions, similar to the receptor structure's (native) ligand's RMSD [43].
PCA, a qualitative statistical technique, is utilized to reduce dimensionality and de-correlate extensive datasets. The resulting variables, referred to as principal components, enable the reduction of variables and reduce information redundancy. In this study, PCA was utilized on the 13 descriptors that characterize the molecular composition of 33 compounds. The resulting 13 principal components are shown in Figure 2.
Table 1 summarizes each descriptor's contribution to the principal components F1, F2, and F3. Consequently, the descriptors that contribute most to F1 are weinerPol, vsurf_CW4, vsurf_HL1, and vsurf_HL2. On the other hand, descriptors like vsurf_CP, VAdjEq, zagreb, and weinerPol contribute the most to F2. The three descriptors that contribute most to F3 are rsynth, ASA_P, and ast_violation.
When the variables are projected onto the plane defined by the first three principal components (F1, F2, and F3) and their percentage contributions are analyzed in the two correlation circles depicted in Figure 3, these axes collectively explain 73.96% of the variance. This percentage is considered sufficient to capture the information conveyed by the dataset.
F1 |
F2 |
F3 |
||||
correlation | contribution | correlation | contribution | correlation | contribution | |
PEOE_VSA_FPNEG | 0.692 | 8.298 | −0.037 | 0.052 | 0.270 | 5.836 |
weinerPol | −0.780 | 10.556 | 0.537 | 11.074 | 0.127 | 1.294 |
zagreb | −0.643 | 7.176 | 0.671 | 17.329 | 0.100 | 0.811 |
VAdjEq | 0.740 | 9.485 | −0.595 | 13.631 | −0.191 | 2.928 |
vsurf_CP | −0.633 | 6.936 | −0.585 | 13.156 | −0.075 | 0.447 |
vsurf_CW4 | 0.812 | 11.429 | 0.471 | 8.537 | 0.177 | 2.508 |
vsurf_HL1 | 0.856 | 12.708 | 0.477 | 8.746 | 0.041 | 0.133 |
vsurf_HL2 | 0.773 | 10.349 | 0.452 | 7.846 | 0.042 | 0.143 |
rsynth | −0.651 | 7.346 | −0.178 | 1.223 | 0.498 | 19.958 |
ASA_P | 0.060 | 0.063 | −0.337 | 4.359 | 0.684 | 37.583 |
ast_violation | −0.604 | 6.315 | 0.360 | 4.973 | −0.521 | 21.789 |
ASA+ | −0.642 | 7.137 | −0.145 | 0.813 | 0.123 | 1.212 |
ASA− | −0.356 | 2.202 | 0.464 | 8.262 | 0.258 | 5.358 |
After the PCA process, we identified two descriptors, including vsurf_CP, and ASA_P, for developing QSAR models. These descriptors were selected from a pool of 13 based on their correlation coefficients. Additionally, descriptors with the lowest correlation coefficients, as shown in Table 2 correlation matrix, were included. The database was then randomly divided into training and test sets using the XLSTAT program.
Variables | PEOE_VSA_FPNEG | weinerPol | zagreb | VAdjEq | vsurf_CP | vsurf_CW4 | vsurf_HL1 | vsurf_HL2 | rsynth | ASA_P | ast_violation | ASA+ | ASA− |
PEOE_VSA_FPNEG | 1 | ||||||||||||
weinerPol | −0.559 | 1 | |||||||||||
zagreb | −0.390 | 0.866 | 1 | ||||||||||
VAdjEq | 0.517 | −0.958 | −0.896 | 1 | |||||||||
vsurf_CP | −0.356 | 0.199 | 0.071 | −0.121 | 1 | ||||||||
vsurf_CW4 | 0.534 | −0.328 | −0.191 | 0.264 | −0.766 | 1 | |||||||
vsurf_HL1 | 0.529 | −0.401 | −0.223 | 0.320 | −0.802 | 0.940 | 1 | ||||||
vsurf_HL2 | 0.410 | −0.354 | −0.189 | 0.278 | −0.663 | 0.851 | 0.942 | 1 | |||||
rsynth | −0.300 | 0.504 | 0.276 | −0.474 | 0.497 | −0.476 | −0.614 | −0.518 | 1 | ||||
ASA_P | 0.061 | −0.127 | −0.161 | 0.094 | 0.107 | 0.021 | −0.056 | −0.039 | 0.149 | 1 | |||
ast_violation | −0.528 | 0.579 | 0.560 | −0.544 | 0.237 | −0.403 | −0.364 | −0.313 | 0.037 | −0.311 | 1 | ||
ASA+ | −0.447 | 0.345 | 0.343 | −0.408 | 0.359 | −0.589 | −0.546 | −0.462 | 0.398 | 0.055 | 0.204 | 1 | |
ASA− | 0.011 | 0.428 | 0.493 | −0.451 | -0.090 | −0.137 | −0.163 | −0.167 | 0.221 | −0.132 | 0.261 | 0.142 | 1 |
The quantitative evaluation of the chemicals to enhance the structure–activity relationship was conducted through partial least squares analysis. The correlation coefficient (R²), MSE, and RMSE were employed for the assessment and validation of the established model.
pIC50 = −11.12 + 0.27*weinerPol + 0.10*Zagreb − 7.91*VAdjEq + 20.01*vsurf_HL1 − 8.74*rsynth − 3.38*ast_violationn.
N = 26; R² = 0.744; MSE = 2.318; RMSE = 1.523.
The significance of the chosen descriptors in the developed model is crucial, and their representation is visually depicted in Figure 4.
The statistical metrics obtained for PLS affirm the reliability and predictive capability of the developed model, as delineated in Table 3. Furthermore, the symmetrical distribution of residuals around zero, illustrated in Figure 5, indicates the absence of systematic errors in the model. The calculated R2 for PLS signifies a close alignment between predicted and observed pIC50 values, validating the robustness of the developed model (Figure 6), Table 4.
The selected descriptors were used in a PCR to enhance the accuracy of forecasting the relationship between activity and molecular structure. The resulting equation, along with the statistical parameters obtained from the ANOVA table, is presented as follows:
pIC50 = 11.97 − 705.61*PEOE_VSA_FPNEG − 0.65*weinerPol − 0.27*Zagreb + 88.48*vsurf_CP − 15.93*vsurf_CW4 − 21.85*vsurf_HL2 − 12.87*rsynth + 4.89*ast_violation.
N = 26; R² = 0.897; R²adj = 0.826; MSE = 1.033; RMSE = 1.017.
The results of the analysis of variance are concisely presented in Table 3 of the ANOVA.
The statistical outcomes derived from the PCR demonstrate high quality and enhanced predictive capabilities comparable to PLS. This is substantiated by significantly high values of the R2 (=0.897) and R2adj (=0.826), coupled with a minimal MSE of 1.033. The expected pIC50 values correlate well with the experimental pIC50, as illustrated in Figure 7.
Source | DF | Sum of squares | Mean squares | F | Pr > F |
Model | 9 | 117.657 | 13.073 | 12.650 | 0.0001 |
Error | 13 | 13.435 | 1.033 | ||
Total | 22 | 131.092 |
Compounds | pIC50 | PLS model |
PCR model |
MLR model |
|||
Pred (pIC50) | Residual | Pred (pIC50) | Residual | Pred (pIC50) | Residual | ||
1 | 3 | 3.725 | −0.725 | 1.836 | 1.164 | 3.084 | −0.084 |
2 | 3 | −0.491 | 0.568 | 3.047 | −0.047 | −21.382 | 24.382 |
3 | 0.077 | 0.581 | −0.514 | 0.419 | −0.342 | 1.457 | −1.380 |
4 | 0.067 | 2.234 | −1.724 | 1.062 | −0.995 | 2.827 | −2.760 |
5 | 0.034 | −2.420 | 2.700 | 0.628 | −0.594 | −0.282 | 0.316 |
6 | 0.51 | −0.639 | 0.879 | 0.000 | 3.800 | 1.446 | −0.936 |
7 | 0.28 | −0.513 | 0.693 | −0.549 | 0.829 | −2.001 | 2.281 |
8 | 0.24 | 2.656 | −2.136 | 1.364 | −1.124 | 0.744 | −0.504 |
9 | 0.18 | 1.863 | −1.623 | 0.000 | 0.445 | 1.341 | −1.161 |
10 | 0.52 | −0.425 | 0.725 | −0.522 | 1.042 | 1.416 | −0.896 |
11 | 0.24 | −0.457 | 0.587 | 0.378 | −0.138 | 1.321 | −1.081 |
12 | 0.27 | 8.698 | 1.302 | 0.781 | −0.511 | −0.636 | 0.906 |
13 | 0.3 | 1.013 | −0.633 | −0.155 | 0.455 | −0.533 | 0.833 |
14 | 0.13 | 3.480 | −0.580 | 0.000 | −1.055 | 0.203 | −0.073 |
15 | 10 | 1.579 | 3.221 | 0.000 | 3.549 | 8.270 | 1.730 |
16 | 0.38 | 5.957 | 0.343 | 1.035 | −0.655 | 1.756 | −1.376 |
17 | 0.76 | −0.174 | 0.239 | 0.000 | −4.264 | 2.560 | −1.800 |
18 | 2.9 | 1.663 | −1.233 | 3.797 | −0.897 | 1.557 | 1.343 |
19 | 10 | 1.218 | −0.728 | 9.008 | 0.992 | 9.588 | 0.412 |
20 | 4.8 | 2.919 | −2.867 | 3.925 | 0.875 | 2.593 | 2.207 |
21 | 4.1 | 7.113 | 2.887 | 0.000 | 2.597 | 3.372 | 0.728 |
22 | 6.3 | 0.967 | −0.905 | 0.000 | 8.742 | 2.410 | 3.890 |
23 | 1.2 | 0.530 | −0.477 | 1.517 | −0.317 | −0.511 | 1.711 |
24 | 0.065 | −40.557 | 43.557 | −0.087 | 0.152 | 0.989 | −0.924 |
25 | 5.2 | −1.733 | 1.767 | 5.438 | −0.238 | 4.228 | 0.972 |
26 | 0.43 | −0.584 | 0.854 | 0.427 | 0.003 | −0.059 | 0.489 |
27 | 0.49 | −1.420 | 2.180 | 0.000 | −10.243 | 0.608 | −0.118 |
28 | 0.052 | −11.404 | 21.404 | −0.612 | 0.664 | 4.535 | −4.483 |
29 | 10 | 1.796 | 2.304 | 0.000 | 14.530 | 2.228 | 7.772 |
30 | 0.062 | −0.935 | 2.135 | 0.000 | 1.448 | 1.526 | −1.464 |
31 | 0.028 | 2.740 | 2.460 | 0.781 | −0.753 | 1.895 | −1.867 |
32 | 0.053 | 0.325 | −0.297 | −1.352 | 1.405 | 0.606 | −0.553 |
33 | 0.027 | −6.308 | 6.335 | 1.000 | −0.973 | −0.204 | 0.231 |
A novel QSAR model was created utilizing the MLR technique in order to improve the correlation between the set of 8 molecular descriptors and the anticipated activities generated through QSAR models created through PLS and PCR techniques.
Four criteria are considered when applying the MLR method: the Fisher ratio value (F), the root RMSE, the R², and the R²adj. The MLR results, including the correlation between observed and predicted activities and the standardized coefficients of the descriptors, are shown in Figures 8 and 9. Furthermore, the following equation represents the formulated model and the values of the statistical parameters:
pIC50 = 9.33 − 38.48*vsurf_CW4 + 167.69*vsurf_HL1 − 153.98*vsurf_HL2 + 9.40*rsynth − 1.79E − 7*ASA+ + 1.368E − 7*ASA− − 2.48E − 7*ASA_P.
N = 26; R² = 0.816; R²adj = 0.735; RMSE = 1.493; F = 10.124.
When analyzing the coefficient normalization diagram, it becomes clear that the constructed model highlights three outstanding descriptors (vsurf_CW4, vsurf_HL1, and vsurf_HL2) that correlate strongly with pIC50. The high R² (0.816), the reduced MSE (0.100), and the high statistical confidence (F = 10.124) confirm the statistical acceptability of the QSAR model. In addition, the achieved p-value of less than 0.05 (Pr < 0.0001) emphasizes the statistical significance of the QSAR model equation at a confidence level of over 95%. Figure 9 shows a significant correlation between observed and predicted pIC50 values, a consequence of the low MSE value achieved. This emphasizes the clear correlation that exists between the values found through experimentation and the values that the QSAR model predicts.
An external validation is performed to verify the validity of the prediction power of the obtained QSAR models. The purpose of the external validation test is to evaluate the QSAR models' ability to predict the pIC50 activity levels of the molecules in the test set. A critical criterion for assessing how well externally verified models perform in forecasting the activities of molecules unrelated to the model's creation is the R2 test coefficient calculation, which is part of this evaluation. The R2 test values achieved for the PLS, PCR, and MLR models are 0.636, 0.802, and 0.722 respectively, all above 0.5. These results verify the robust predictive ability of the QSAR models in estimating pIC50 values during external validation.
This study utilized molecular docking to predict interaction types, binding sites, and binding affinities of pyridopyrazine derivatives, particularly compound C11, against the histamine H4 receptor (H4R), which is implicated in inflammatory responses. Molecular docking provided valuable insights into binding affinities, with compound C11 showing the lowest binding energy at −5.80 kcal/mol, indicating a strong interaction with the H4R protein (7YFD). This binding affinity, among the highest in the compound library, supports the potential of C11 as a selective H4R antagonist for anti-inflammatory applications in conditions such as atopic dermatitis.
The results in Table 5 and Figure 10 detail these interactions, showing C11's significant hydrogen bonding with key residues (e.g., Thr 251 and Lys 27) and π-stacking interactions with Thr 219. These bonds are particularly relevant as they contribute to stabilizing the receptor–ligand complex. However, while the binding energy and interaction specifics suggest a strong affinity, it is essential to interpret these findings with caution. Docking studies are predictive, and factors like solubility, bioavailability, and off-target interactions in a physiological context need further validation through experimental assays.
compounds | Binding energy (Kcal/mol) | Ligand | receptor | interaction | distance | E (kcal/mol) |
1 | −5.38 | 6-ring | THR 219 | pi-H | 4.4 | −0.7 |
2 | −5.21 | 5-ring | THR 219 | pi-H | 3.31 | −0.7 |
6-ring | THR 219 | pi-H | 4.38 | −0.7 | ||
3 | −5.38 | 5-ring | THR 219 | pi-H | 3.29 | −0.8 |
6-ring | THR 219 | pi-H | 4.31 | −0.8 | ||
4 | −5.59 | N 24 | THR 251 | H-acceptor | 3.36 | −0.6 |
5 | −5.61 | I 35 | ARG 31 | H-donor | 4.19 | −0.5 |
N 24 | THR 251 | H-acceptor | 3.27 | −0.9 | ||
6 | −5.42 | 5-ring | THR 219 | pi-H | 3.29 | −0.8 |
6-ring | THR 219 | pi-H | 4.37 | −0.9 | ||
7 | −5.74 | F 38 | ARG 35 | H-acceptor | 3.23 | −0.7 |
8 | −5.53 | N 36 | LYS 27 | H-acceptor | 2.97 | −1.3 |
6-ring | THR 219 | pi-H | 4.62 | −1.2 | ||
5-ring | THR 219 | pi-H | 3.34 | −1.3 | ||
9 | −5.45 | 5-ring | THR 219 | pi-H | 3.29 | −0.8 |
6-ring | THR 219 | pi-H | 4.3 | −0.8 | ||
10 | −5.76 | 5-ring | THR 219 | pi-H | 3.28 | −0.8 |
6-ring | THR 219 | pi-H | 4.29 | −0.9 | ||
11 | −5.80 | CL 34 | THR 251 | H-donor | 3.2 | −0.6 |
N 23 | LYS 27 | H-acceptor | 3.39 | −0.7 | ||
5-ring | THR 219 | pi-H | 4.15 | −0.8 | ||
6-ring | THR 219 | pi-H | 4.82 | −0.8 | ||
12 | −5.75 | N 36 | SER 253 | H-acceptor | 3.81 | −0.8 |
13 | −5.64 | 6-ring | THR 219 | pi-H | 3.98 | −0.9 |
14 | −5.44 | 6-ring | THR 219 | pi-H | 3.98 | −0.9 |
15 | −5.55 | 6-ring | THR 219 | pi-H | 4.19 | −0.6 |
16 | −5.67 | N 24 | THR 251 | H-acceptor | 3.33 | −0.6 |
17 | −5.56 | N 24 | ARG 35 | H-acceptor | 3.26 | −4.4 |
5-ring | THR 219 | pi-H | 3.8 | −1 | ||
18 | −5.46 | 5-ring | THR 219 | pi-H | 3.3 | −1 |
6-ring | THR 219 | pi-H | 4.43 | −1 | ||
19 | −5.59 | N 28 | ARG 35 | H-acceptor | 3.09 | −5.2 |
20 | −5.6 | N 30 | ARG 35 | H-acceptor | 2.97 | −5.4 |
21 | −5.28 | 5-ring | THR 219 | pi-H | 3.77 | −0.6 |
22 | −5.26 | 6-ring | THR 219 | pi-H | 4.43 | −1.2 |
5-ring | THR 219 | pi-H | 3.29 | −1.1 | ||
23 | −5.59 | 5-ring | THR 219 | pi-H | 3.3 | −0.6 |
6-ring | THR 219 | pi-H | 4.3 | −0.7 | ||
24 | −5.23 | N 27 | ARG 35 | H-acceptor | 3.08 | −4.8 |
5-ring | THR 219 | pi-H | 3.77 | −1 | ||
25 | −5.48 | N 27 | THR 33 | H-donor | 2.94 | −1.2 |
26 | −5.66 | 6-ring | THR 219 | pi-H | 4.47 | −1 |
5-ring | THR 219 | pi-H | 3.31 | −1 | ||
27 | −5.53 | 6-ring | THR 219 | pi-H | 4.27 | −0.7 |
28 | −5.46 | N 17 | THR 219 | H-acceptor | 2.93 | 24.3 |
29 | −5.79 | N 29 | ARG 35 | H-acceptor | 2.98 | −3.9 |
30 | −5.38 | N 24 | THR 219 | H-acceptor | 2.9 | −2.7 |
31 | −5.3 | BR 17 | THR 251 | H-donor | 3.55 | −0.6 |
N 24 | LYS 27 | H-acceptor | 3.34 | −1 | ||
5-ring | THR 219 | pi-H | 3.4 | −0.6 | ||
6-ring | THR 219 | pi-H | 4.45 | −0.6 | ||
32 | −5.4 | N 26 | THR 219 | H-acceptor | 3.02 | −0.7 |
33 | −5.42 | N 26 | ARG 35 | H-acceptor | 3.21 | −1 |
In terms of structural interaction, the hydrogen bond distances (3.20–4.82 Å) and stabilizing energies (−0.6 to −0.8 kcal/mol) reveal that these interactions are consistent with moderate affinity, but further in vitro and in vivo testing is needed to confirm the stability and efficacy of C11 in a biological system. Additionally, the alignment of docking data with experimental LD10 values supports the model's reliability, though this correlation should be expanded by testing other promising compounds in the pyridopyrazine series to ensure broader applicability.
Simultaneously, QSAR modeling served as a predictive tool to understand structural determinants of activity. By identifying molecular descriptors with significant predictive power, such as vsurf_CW4 and weinerPol, the QSAR model allowed for efficient virtual screening of potential H4R antagonists, accelerating the identification of compounds with optimal pharmacological properties. However, one limitation observed is that QSAR models rely on known chemical libraries, and their predictions are limited when applied to novel structural frameworks. Future work should aim to refine QSAR models to include diverse compound libraries, allowing for a more robust selection process.
Overall, while molecular docking and QSAR provided strong preliminary data on compound C11 and other pyridopyrazine derivatives, these computational methods should be complemented with experimental validation. The observed binding affinities and structural insights are promising for advancing AD treatments, yet translating these findings into clinical applications will require further studies to confirm efficacy, selectivity, and safety profiles in biological systems.
In this study, we evaluated the inhibitory activity of pyridopyrazine derivatives against the histamine H4 receptor (H4R) as a potential treatment for atopic dermatitis. Through QSAR modeling and molecular docking, compound C11 emerged as a promising candidate, demonstrating strong binding affinity and favorable interactions with H4R. These findings highlight the potential of pyridopyrazine derivatives in anti-inflammatory therapy development for AD, with future studies needed to confirm their therapeutic viability.
The authors declare they have not used Artificial Intelligence (AI) tools in the creation of this article.
[1] |
Jolliffe IT, Cadima J (2016) Principal component analysis: A review and recent developments. Philos Trans R Soc A 374: 20150202. https://doi.org/10.1098/rsta.2015.0202 ![]() |
[2] |
Kim J, Kim BE, Leung DYM (2019) Pathophysiology of atopic dermatitis: Clinical implications. Allergy Asthma Proc 40: 84-92. https://doi.org/10.2500/aap.2019.40.4202 ![]() |
[3] |
David Boothe W, Tarbox JA, Tarbox MB (2017) Atopic dermatitis: Pathophysiology. Management of Atopic Dermatitis. Cham: Springer International Publishing 21-37. https://doi.org/10.1007/978-3-319-64804-0_3 ![]() |
[4] |
Sroka-Tomaszewska J, Trzeciak M (2021) Molecular mechanisms of atopic dermatitis pathogenesis. Int J Mol Sci 22: 4130. https://doi.org/10.3390/ijms22084130 ![]() |
[5] |
Maintz L, Bieber T, Simpson HD, et al. (2022) From skin barrier dysfunction to systemic impact of atopic dermatitis: Implications for a precision approach in dermocosmetics and medicine. J Pers Med 12: 893. https://doi.org/10.3390/jpm12060893 ![]() |
[6] | Santamaria-Babí LF (2022) Atopic dermatitis pathogenesis: Lessons from immunology. Dermatol Pract Concept 12. https://doi.org/10.5826/dpc.1201a152 |
[7] |
Guttman-Yassky E, Dhingra N, Leung DYM (2013) New era of biologic therapeutics in atopic dermatitis. Expert Opin Biol Ther 13: 549-561. https://doi.org/10.1517/14712598.2013.758708 ![]() |
[8] |
Mitchell PM, Al-Janabi H, Richardson J, et al. (2015) The relative impacts of disease on health status and capability wellbeing: A multi-country study. PLoS ONE 10: e0143590. https://doi.org/10.1371/journal.pone.0143590 ![]() |
[9] |
Brunner PM, Guttman-Yassky E, Leung DYM (2017) The immunology of atopic dermatitis and its reversibility with broad-spectrum and targeted therapies. J Allergy Clin Immunol 139: S65-S76. https://doi.org/10.1016/j.jaci.2017.01.011 ![]() |
[10] |
Mathew B, Srivastava S, Ross LJ, et al. (2011) Novel pyridopyrazine and pyrimidothiazine derivatives as FtsZ inhibitors. Bioorg Med Chem 19: 7120-7128. https://doi.org/10.1016/j.bmc.2011.09.062 ![]() |
[11] |
Lobo V, Patil A, Phatak A, et al. (2010) Free radicals, antioxidants and functional foods: Impact on human health. Pharmacogn Rev 4: 118-126. https://doi.org/10.4103/0973-7847.70902 ![]() |
[12] | Patra AK (2012) An overview of antimicrobial properties of different classes of phytochemicals. Dietary Phytochemicals and Microbes. Cham: Springer 1-32. https://doi.org/10.1007/978-94-007-3926-0_1 |
[13] | Pizzino G, Irrera N, Cucinotta M, et al. (2017) Oxidative stress: Harms and benefits for human health. Oxid Med Cell Longevity . https://doi.org/10.1155/2017/8416763 |
[14] |
Pettersson M, Johnson DS, Humphrey JM, et al. (2015) Design of pyridopyrazine-1,6-dione γ-secretase modulators that align potency, MDR efflux ratio, and metabolic stability. ACS Med Chem Lett 6: 596-601. https://doi.org/10.1021/acsmedchemlett.5b00070 ![]() |
[15] |
Pettersson M, Johnson DS, Rankic DA, et al. (2017) Discovery of cyclopropylchromane-derived pyridopyrazine-1,6-dione γ-secretase modulators with robust central efficacy. Med Chem Comm 8: 730-743. https://doi.org/10.1039/c6md00406g ![]() |
[16] |
Kékesi L, Sipos A, Németh G, et al. (2013) Synthesis and biological evaluation of novel pyrido [2,3-b] pyrazines inhibiting both erlotinib-sensitive and erlotinib-resistant cell lines. Bioorg Med Chem Lett 23: 6152-6155. https://doi.org/10.1016/j.bmcl.2013.09.005 ![]() |
[17] | Sahu R, Shah K, Gautam Y, et al. (2023) Pyrazine moiety: Recent developments in cancer treatment. Curr Org Chem 27: 6152-6155. http://dx.doi.org/10.2174/1385272827666230816105317 |
[18] |
Niaz L, Saddique FA, Aslam S, et al. (2020) Recent synthetic methodologies for pyridopyrazines: An update. Synth Commun 50: 2755-2786. https://doi.org/10.1080/00397911.2020.1786123 ![]() |
[19] |
Mahmud AW, Shallangwa GA, Uzairu A (2020) QSAR and molecular docking studies of 1,3-dioxoisoindoline-4-aminoquinolines as potent antiplasmodium hybrid compounds. Heliyon 6: e03449. https://doi.org/10.1016/j.heliyon.2020.e03449 ![]() |
[20] |
Rosell-Hidalgo A, Young L, Moore AL, et al. (2021) QSAR and molecular docking for the search of AOX inhibitors: A rational drug discovery approach. J Comput-Aided Molr Des 35: 245-260. https://doi.org/10.1007/s10822-020-00360-8 ![]() |
[21] |
Daoui O, Elkhattabi S, Chtita S, et al. (2021) QSAR, molecular docking and ADMET properties in silico studies of novel 4,5,6,7-tetrahydrobenzo[D]-thiazol-2-Yl derivatives derived from dimedone as potent anti-tumor agents through inhibition of C-Met receptor tyrosine kinase. Heliyon 7: e07463. https://doi.org/10.1016/j.heliyon.2021.e07463 ![]() |
[22] |
Liu J, Li Y, Zhang HX, et al. (2012) Studies of H4R antagonists using 3D-QSAR, molecular docking and molecular dynamics. J Mol Model 18: 991-1001. https://doi.org/10.1007/s00894-011-1137-x ![]() |
[23] |
Mehta P, Miszta P, Filipek S (2021) Molecular modeling of histamine receptors—recent advances in drug discovery. Molecules 26: 1778. https://doi.org/10.3390/molecules26061778 ![]() |
[24] |
Lahyaoui M, El-Idrissi H, Saffaj T, et al. (2023) QSAR modeling, molecular docking and molecular dynamic simulation of phosphorus-substituted quinoline derivatives as topoisomerase I inhibitors. Arabian J Chem 16: 104783. https://doi.org/10.1016/j.arabjc.2023.104783 ![]() |
[25] |
Lahyaoui M, Diane A, El-Idrissi, et al. (2023) QSAR modeling and molecular docking studies of 2-oxo-1, 2-dihydroquinoline-4- carboxylic acid derivatives as p-glycoprotein inhibitors for combating cancer multidrug resistance. Heliyon 9: e13020. https://doi.org/10.1016/j.heliyon.2023.e13020 ![]() |
[26] |
Li J, Luo D, Wen T, et al. (2021) Representative feature selection of molecular descriptors in QSAR modeling. J Mol Struct 1244: 131249. https://doi.org/10.1016/j.molstruc.2021.131249 ![]() |
[27] |
Frimayanti N, Yam ML, Lee HB, et al. (2011) Validation of quantitative structure-activity relationship (QSAR) model for photosensitizer activity prediction. Int Mol Sci 12: 8626-8644. https://doi.org/10.3390/ijms12128626 ![]() |
[28] |
Roy K, Ambure P, Kar S (2018) How precise are our quantitative structure-activity relationship derived predictions for new query chemicals?. ACS Omega 3: 11392-11406. https://doi.org/10.1021/acsomega.8b01647 ![]() |
[29] |
Khamouli S, Belaidi S, Bakhouch M, et al. (2022) QSAR modeling, molecular docking, ADMET prediction and molecular dynamics simulations of some 6-arylquinazolin-4-amine derivatives as DYRK1A inhibitors. J Mol Struct 1258: 132659. https://doi.org/10.1016/j.molstruc.2022.132659 ![]() |
[30] |
Li J, Fu A, Zhang L (2019) An overview of scoring functions used for protein–ligand interactions in molecular docking. Interdiscip Sci: Computa Life Sci 11: 320-328. https://doi.org/10.1007/s12539-019-00327-w ![]() |
[31] |
Abdullahi M, Shallangwa GA, Uzairu A (2020) In silico QSAR and molecular docking simulation of some novel aryl sulfonamide derivatives as inhibitors of H5N1 influenza a virus subtype. Beni-Suef Univ J Basic Appl Sci 9: 2. https://doi.org/10.1186/s43088-019-0023-y ![]() |
[32] |
El Fadili M, Er-Rajy M, Kara M, et al. (2022) QSAR, ADMET in silico pharmacokinetics, molecular docking and molecular dynamics studies of novel bicyclo (aryl methyl) benzamides as potent glyt1 inhibitors for the treatment of schizophrenia. Pharmaceuticals 15: 670. https://doi.org/10.3390/ph15060670 ![]() |
[33] |
Lahyaoui M, Haoudi A, Kartah BE, et al. (2023) Crystal structure, Hirshfeld surface analysis, intermolecular interaction energies, energy frameworks and DFT calculations of 4-amino-1-(prop-2-yn-1-yl)pyrimidin-2(1H)-one. Acta Crystallogr Sec E: Crystallogr Commun 79: 1183-1189. https://doi.org/10.1107/S2056989023009933 ![]() |
[34] |
Stoean B, Rugina D, Focsan M, et al. (2021) Novel (Phenothiazinyl)vinyl-pyridinium dyes and their potential applications as cellular staining agents. Int J Mol Sci 22: 2985. https://doi.org/10.3390/ijms22062985 ![]() |
[35] |
Ko K, Kim HJ, Ho PS, et al. (2018) Discovery of a novel highly selective histamine h4 receptor antagonist for the treatment of atopic dermatitis. J Med Chem 61: 2949-2961. https://doi.org/10.1021/acs.jmedchem.7b01855 ![]() |
[36] |
Danishuddin, Khan AU (2016) Descriptors and their selection methods in QSAR analysis: Paradigm for drug design. Drug Discovery Today 21: 1291-1302. https://doi.org/10.1016/j.drudis.2016.06.013 ![]() |
[37] | (1987) Statitcf SoftwareTechnical Institute of Cereals and Fodder. Paris, France: . |
[38] | Hmamouchi R, Larif M, Adad A, et al. (2014) Structure activity and prediction of biological activities of compound (2-methyl-6-phenylethynylpyridine) derivatives relationships rely on electronic and topological descriptors. J Comput Methods Mol Des 4: 61-71. |
[39] |
Wold S, Esbensen K, Geladi P (1987) Principal component analysis. Chemom Intell Lab Sys 2: 37-52. https://doi.org/10.1016/0169-7439(87)80084-9 ![]() |
[40] |
David CC, Jacobs DJ (2014) Principal component analysis: A method for determining the essential dynamics of proteins. Protein Dynamics. Cham: Springer 193-226. https://doi.org/10.1007/978-1-62703-658-0_11 ![]() |
[41] |
Golbraikh A, Tropsha A (2002) Beware of q2!. J Mol Graphics Modell 20: 269-276. https://doi.org/10.1016/S1093-3263(01)00123-1 ![]() |
[42] |
Chtita S, Belhassan A, Bakhouch M, et al. (2021) QSAR study of unsymmetrical aromatic disulfides as potent avian SARS-CoV main protease inhibitors using quantum chemical descriptors and statistical methods. Chemom Intell Lab Syst 210: 104266. https://doi.org/10.1016/j.chemolab.2021.104266 ![]() |
[43] |
Lahyaoui M, Filali M, Sghyar R, et al. (2024) Development of novel antibiotics derived from pyridazine: Synthesis, spectroscopic characterization, in vitro antimicrobial activity and molecular docking studies. Results Chem 10: 101699. https://doi.org/10.1016/j.rechem.2024.101699 ![]() |
![]() |
![]() |
F1 |
F2 |
F3 |
||||
correlation | contribution | correlation | contribution | correlation | contribution | |
PEOE_VSA_FPNEG | 0.692 | 8.298 | −0.037 | 0.052 | 0.270 | 5.836 |
weinerPol | −0.780 | 10.556 | 0.537 | 11.074 | 0.127 | 1.294 |
zagreb | −0.643 | 7.176 | 0.671 | 17.329 | 0.100 | 0.811 |
VAdjEq | 0.740 | 9.485 | −0.595 | 13.631 | −0.191 | 2.928 |
vsurf_CP | −0.633 | 6.936 | −0.585 | 13.156 | −0.075 | 0.447 |
vsurf_CW4 | 0.812 | 11.429 | 0.471 | 8.537 | 0.177 | 2.508 |
vsurf_HL1 | 0.856 | 12.708 | 0.477 | 8.746 | 0.041 | 0.133 |
vsurf_HL2 | 0.773 | 10.349 | 0.452 | 7.846 | 0.042 | 0.143 |
rsynth | −0.651 | 7.346 | −0.178 | 1.223 | 0.498 | 19.958 |
ASA_P | 0.060 | 0.063 | −0.337 | 4.359 | 0.684 | 37.583 |
ast_violation | −0.604 | 6.315 | 0.360 | 4.973 | −0.521 | 21.789 |
ASA+ | −0.642 | 7.137 | −0.145 | 0.813 | 0.123 | 1.212 |
ASA− | −0.356 | 2.202 | 0.464 | 8.262 | 0.258 | 5.358 |
Variables | PEOE_VSA_FPNEG | weinerPol | zagreb | VAdjEq | vsurf_CP | vsurf_CW4 | vsurf_HL1 | vsurf_HL2 | rsynth | ASA_P | ast_violation | ASA+ | ASA− |
PEOE_VSA_FPNEG | 1 | ||||||||||||
weinerPol | −0.559 | 1 | |||||||||||
zagreb | −0.390 | 0.866 | 1 | ||||||||||
VAdjEq | 0.517 | −0.958 | −0.896 | 1 | |||||||||
vsurf_CP | −0.356 | 0.199 | 0.071 | −0.121 | 1 | ||||||||
vsurf_CW4 | 0.534 | −0.328 | −0.191 | 0.264 | −0.766 | 1 | |||||||
vsurf_HL1 | 0.529 | −0.401 | −0.223 | 0.320 | −0.802 | 0.940 | 1 | ||||||
vsurf_HL2 | 0.410 | −0.354 | −0.189 | 0.278 | −0.663 | 0.851 | 0.942 | 1 | |||||
rsynth | −0.300 | 0.504 | 0.276 | −0.474 | 0.497 | −0.476 | −0.614 | −0.518 | 1 | ||||
ASA_P | 0.061 | −0.127 | −0.161 | 0.094 | 0.107 | 0.021 | −0.056 | −0.039 | 0.149 | 1 | |||
ast_violation | −0.528 | 0.579 | 0.560 | −0.544 | 0.237 | −0.403 | −0.364 | −0.313 | 0.037 | −0.311 | 1 | ||
ASA+ | −0.447 | 0.345 | 0.343 | −0.408 | 0.359 | −0.589 | −0.546 | −0.462 | 0.398 | 0.055 | 0.204 | 1 | |
ASA− | 0.011 | 0.428 | 0.493 | −0.451 | -0.090 | −0.137 | −0.163 | −0.167 | 0.221 | −0.132 | 0.261 | 0.142 | 1 |
Source | DF | Sum of squares | Mean squares | F | Pr > F |
Model | 9 | 117.657 | 13.073 | 12.650 | 0.0001 |
Error | 13 | 13.435 | 1.033 | ||
Total | 22 | 131.092 |
Compounds | pIC50 | PLS model |
PCR model |
MLR model |
|||
Pred (pIC50) | Residual | Pred (pIC50) | Residual | Pred (pIC50) | Residual | ||
1 | 3 | 3.725 | −0.725 | 1.836 | 1.164 | 3.084 | −0.084 |
2 | 3 | −0.491 | 0.568 | 3.047 | −0.047 | −21.382 | 24.382 |
3 | 0.077 | 0.581 | −0.514 | 0.419 | −0.342 | 1.457 | −1.380 |
4 | 0.067 | 2.234 | −1.724 | 1.062 | −0.995 | 2.827 | −2.760 |
5 | 0.034 | −2.420 | 2.700 | 0.628 | −0.594 | −0.282 | 0.316 |
6 | 0.51 | −0.639 | 0.879 | 0.000 | 3.800 | 1.446 | −0.936 |
7 | 0.28 | −0.513 | 0.693 | −0.549 | 0.829 | −2.001 | 2.281 |
8 | 0.24 | 2.656 | −2.136 | 1.364 | −1.124 | 0.744 | −0.504 |
9 | 0.18 | 1.863 | −1.623 | 0.000 | 0.445 | 1.341 | −1.161 |
10 | 0.52 | −0.425 | 0.725 | −0.522 | 1.042 | 1.416 | −0.896 |
11 | 0.24 | −0.457 | 0.587 | 0.378 | −0.138 | 1.321 | −1.081 |
12 | 0.27 | 8.698 | 1.302 | 0.781 | −0.511 | −0.636 | 0.906 |
13 | 0.3 | 1.013 | −0.633 | −0.155 | 0.455 | −0.533 | 0.833 |
14 | 0.13 | 3.480 | −0.580 | 0.000 | −1.055 | 0.203 | −0.073 |
15 | 10 | 1.579 | 3.221 | 0.000 | 3.549 | 8.270 | 1.730 |
16 | 0.38 | 5.957 | 0.343 | 1.035 | −0.655 | 1.756 | −1.376 |
17 | 0.76 | −0.174 | 0.239 | 0.000 | −4.264 | 2.560 | −1.800 |
18 | 2.9 | 1.663 | −1.233 | 3.797 | −0.897 | 1.557 | 1.343 |
19 | 10 | 1.218 | −0.728 | 9.008 | 0.992 | 9.588 | 0.412 |
20 | 4.8 | 2.919 | −2.867 | 3.925 | 0.875 | 2.593 | 2.207 |
21 | 4.1 | 7.113 | 2.887 | 0.000 | 2.597 | 3.372 | 0.728 |
22 | 6.3 | 0.967 | −0.905 | 0.000 | 8.742 | 2.410 | 3.890 |
23 | 1.2 | 0.530 | −0.477 | 1.517 | −0.317 | −0.511 | 1.711 |
24 | 0.065 | −40.557 | 43.557 | −0.087 | 0.152 | 0.989 | −0.924 |
25 | 5.2 | −1.733 | 1.767 | 5.438 | −0.238 | 4.228 | 0.972 |
26 | 0.43 | −0.584 | 0.854 | 0.427 | 0.003 | −0.059 | 0.489 |
27 | 0.49 | −1.420 | 2.180 | 0.000 | −10.243 | 0.608 | −0.118 |
28 | 0.052 | −11.404 | 21.404 | −0.612 | 0.664 | 4.535 | −4.483 |
29 | 10 | 1.796 | 2.304 | 0.000 | 14.530 | 2.228 | 7.772 |
30 | 0.062 | −0.935 | 2.135 | 0.000 | 1.448 | 1.526 | −1.464 |
31 | 0.028 | 2.740 | 2.460 | 0.781 | −0.753 | 1.895 | −1.867 |
32 | 0.053 | 0.325 | −0.297 | −1.352 | 1.405 | 0.606 | −0.553 |
33 | 0.027 | −6.308 | 6.335 | 1.000 | −0.973 | −0.204 | 0.231 |
compounds | Binding energy (Kcal/mol) | Ligand | receptor | interaction | distance | E (kcal/mol) |
1 | −5.38 | 6-ring | THR 219 | pi-H | 4.4 | −0.7 |
2 | −5.21 | 5-ring | THR 219 | pi-H | 3.31 | −0.7 |
6-ring | THR 219 | pi-H | 4.38 | −0.7 | ||
3 | −5.38 | 5-ring | THR 219 | pi-H | 3.29 | −0.8 |
6-ring | THR 219 | pi-H | 4.31 | −0.8 | ||
4 | −5.59 | N 24 | THR 251 | H-acceptor | 3.36 | −0.6 |
5 | −5.61 | I 35 | ARG 31 | H-donor | 4.19 | −0.5 |
N 24 | THR 251 | H-acceptor | 3.27 | −0.9 | ||
6 | −5.42 | 5-ring | THR 219 | pi-H | 3.29 | −0.8 |
6-ring | THR 219 | pi-H | 4.37 | −0.9 | ||
7 | −5.74 | F 38 | ARG 35 | H-acceptor | 3.23 | −0.7 |
8 | −5.53 | N 36 | LYS 27 | H-acceptor | 2.97 | −1.3 |
6-ring | THR 219 | pi-H | 4.62 | −1.2 | ||
5-ring | THR 219 | pi-H | 3.34 | −1.3 | ||
9 | −5.45 | 5-ring | THR 219 | pi-H | 3.29 | −0.8 |
6-ring | THR 219 | pi-H | 4.3 | −0.8 | ||
10 | −5.76 | 5-ring | THR 219 | pi-H | 3.28 | −0.8 |
6-ring | THR 219 | pi-H | 4.29 | −0.9 | ||
11 | −5.80 | CL 34 | THR 251 | H-donor | 3.2 | −0.6 |
N 23 | LYS 27 | H-acceptor | 3.39 | −0.7 | ||
5-ring | THR 219 | pi-H | 4.15 | −0.8 | ||
6-ring | THR 219 | pi-H | 4.82 | −0.8 | ||
12 | −5.75 | N 36 | SER 253 | H-acceptor | 3.81 | −0.8 |
13 | −5.64 | 6-ring | THR 219 | pi-H | 3.98 | −0.9 |
14 | −5.44 | 6-ring | THR 219 | pi-H | 3.98 | −0.9 |
15 | −5.55 | 6-ring | THR 219 | pi-H | 4.19 | −0.6 |
16 | −5.67 | N 24 | THR 251 | H-acceptor | 3.33 | −0.6 |
17 | −5.56 | N 24 | ARG 35 | H-acceptor | 3.26 | −4.4 |
5-ring | THR 219 | pi-H | 3.8 | −1 | ||
18 | −5.46 | 5-ring | THR 219 | pi-H | 3.3 | −1 |
6-ring | THR 219 | pi-H | 4.43 | −1 | ||
19 | −5.59 | N 28 | ARG 35 | H-acceptor | 3.09 | −5.2 |
20 | −5.6 | N 30 | ARG 35 | H-acceptor | 2.97 | −5.4 |
21 | −5.28 | 5-ring | THR 219 | pi-H | 3.77 | −0.6 |
22 | −5.26 | 6-ring | THR 219 | pi-H | 4.43 | −1.2 |
5-ring | THR 219 | pi-H | 3.29 | −1.1 | ||
23 | −5.59 | 5-ring | THR 219 | pi-H | 3.3 | −0.6 |
6-ring | THR 219 | pi-H | 4.3 | −0.7 | ||
24 | −5.23 | N 27 | ARG 35 | H-acceptor | 3.08 | −4.8 |
5-ring | THR 219 | pi-H | 3.77 | −1 | ||
25 | −5.48 | N 27 | THR 33 | H-donor | 2.94 | −1.2 |
26 | −5.66 | 6-ring | THR 219 | pi-H | 4.47 | −1 |
5-ring | THR 219 | pi-H | 3.31 | −1 | ||
27 | −5.53 | 6-ring | THR 219 | pi-H | 4.27 | −0.7 |
28 | −5.46 | N 17 | THR 219 | H-acceptor | 2.93 | 24.3 |
29 | −5.79 | N 29 | ARG 35 | H-acceptor | 2.98 | −3.9 |
30 | −5.38 | N 24 | THR 219 | H-acceptor | 2.9 | −2.7 |
31 | −5.3 | BR 17 | THR 251 | H-donor | 3.55 | −0.6 |
N 24 | LYS 27 | H-acceptor | 3.34 | −1 | ||
5-ring | THR 219 | pi-H | 3.4 | −0.6 | ||
6-ring | THR 219 | pi-H | 4.45 | −0.6 | ||
32 | −5.4 | N 26 | THR 219 | H-acceptor | 3.02 | −0.7 |
33 | −5.42 | N 26 | ARG 35 | H-acceptor | 3.21 | −1 |
F1 |
F2 |
F3 |
||||
correlation | contribution | correlation | contribution | correlation | contribution | |
PEOE_VSA_FPNEG | 0.692 | 8.298 | −0.037 | 0.052 | 0.270 | 5.836 |
weinerPol | −0.780 | 10.556 | 0.537 | 11.074 | 0.127 | 1.294 |
zagreb | −0.643 | 7.176 | 0.671 | 17.329 | 0.100 | 0.811 |
VAdjEq | 0.740 | 9.485 | −0.595 | 13.631 | −0.191 | 2.928 |
vsurf_CP | −0.633 | 6.936 | −0.585 | 13.156 | −0.075 | 0.447 |
vsurf_CW4 | 0.812 | 11.429 | 0.471 | 8.537 | 0.177 | 2.508 |
vsurf_HL1 | 0.856 | 12.708 | 0.477 | 8.746 | 0.041 | 0.133 |
vsurf_HL2 | 0.773 | 10.349 | 0.452 | 7.846 | 0.042 | 0.143 |
rsynth | −0.651 | 7.346 | −0.178 | 1.223 | 0.498 | 19.958 |
ASA_P | 0.060 | 0.063 | −0.337 | 4.359 | 0.684 | 37.583 |
ast_violation | −0.604 | 6.315 | 0.360 | 4.973 | −0.521 | 21.789 |
ASA+ | −0.642 | 7.137 | −0.145 | 0.813 | 0.123 | 1.212 |
ASA− | −0.356 | 2.202 | 0.464 | 8.262 | 0.258 | 5.358 |
Variables | PEOE_VSA_FPNEG | weinerPol | zagreb | VAdjEq | vsurf_CP | vsurf_CW4 | vsurf_HL1 | vsurf_HL2 | rsynth | ASA_P | ast_violation | ASA+ | ASA− |
PEOE_VSA_FPNEG | 1 | ||||||||||||
weinerPol | −0.559 | 1 | |||||||||||
zagreb | −0.390 | 0.866 | 1 | ||||||||||
VAdjEq | 0.517 | −0.958 | −0.896 | 1 | |||||||||
vsurf_CP | −0.356 | 0.199 | 0.071 | −0.121 | 1 | ||||||||
vsurf_CW4 | 0.534 | −0.328 | −0.191 | 0.264 | −0.766 | 1 | |||||||
vsurf_HL1 | 0.529 | −0.401 | −0.223 | 0.320 | −0.802 | 0.940 | 1 | ||||||
vsurf_HL2 | 0.410 | −0.354 | −0.189 | 0.278 | −0.663 | 0.851 | 0.942 | 1 | |||||
rsynth | −0.300 | 0.504 | 0.276 | −0.474 | 0.497 | −0.476 | −0.614 | −0.518 | 1 | ||||
ASA_P | 0.061 | −0.127 | −0.161 | 0.094 | 0.107 | 0.021 | −0.056 | −0.039 | 0.149 | 1 | |||
ast_violation | −0.528 | 0.579 | 0.560 | −0.544 | 0.237 | −0.403 | −0.364 | −0.313 | 0.037 | −0.311 | 1 | ||
ASA+ | −0.447 | 0.345 | 0.343 | −0.408 | 0.359 | −0.589 | −0.546 | −0.462 | 0.398 | 0.055 | 0.204 | 1 | |
ASA− | 0.011 | 0.428 | 0.493 | −0.451 | -0.090 | −0.137 | −0.163 | −0.167 | 0.221 | −0.132 | 0.261 | 0.142 | 1 |
Source | DF | Sum of squares | Mean squares | F | Pr > F |
Model | 9 | 117.657 | 13.073 | 12.650 | 0.0001 |
Error | 13 | 13.435 | 1.033 | ||
Total | 22 | 131.092 |
Compounds | pIC50 | PLS model |
PCR model |
MLR model |
|||
Pred (pIC50) | Residual | Pred (pIC50) | Residual | Pred (pIC50) | Residual | ||
1 | 3 | 3.725 | −0.725 | 1.836 | 1.164 | 3.084 | −0.084 |
2 | 3 | −0.491 | 0.568 | 3.047 | −0.047 | −21.382 | 24.382 |
3 | 0.077 | 0.581 | −0.514 | 0.419 | −0.342 | 1.457 | −1.380 |
4 | 0.067 | 2.234 | −1.724 | 1.062 | −0.995 | 2.827 | −2.760 |
5 | 0.034 | −2.420 | 2.700 | 0.628 | −0.594 | −0.282 | 0.316 |
6 | 0.51 | −0.639 | 0.879 | 0.000 | 3.800 | 1.446 | −0.936 |
7 | 0.28 | −0.513 | 0.693 | −0.549 | 0.829 | −2.001 | 2.281 |
8 | 0.24 | 2.656 | −2.136 | 1.364 | −1.124 | 0.744 | −0.504 |
9 | 0.18 | 1.863 | −1.623 | 0.000 | 0.445 | 1.341 | −1.161 |
10 | 0.52 | −0.425 | 0.725 | −0.522 | 1.042 | 1.416 | −0.896 |
11 | 0.24 | −0.457 | 0.587 | 0.378 | −0.138 | 1.321 | −1.081 |
12 | 0.27 | 8.698 | 1.302 | 0.781 | −0.511 | −0.636 | 0.906 |
13 | 0.3 | 1.013 | −0.633 | −0.155 | 0.455 | −0.533 | 0.833 |
14 | 0.13 | 3.480 | −0.580 | 0.000 | −1.055 | 0.203 | −0.073 |
15 | 10 | 1.579 | 3.221 | 0.000 | 3.549 | 8.270 | 1.730 |
16 | 0.38 | 5.957 | 0.343 | 1.035 | −0.655 | 1.756 | −1.376 |
17 | 0.76 | −0.174 | 0.239 | 0.000 | −4.264 | 2.560 | −1.800 |
18 | 2.9 | 1.663 | −1.233 | 3.797 | −0.897 | 1.557 | 1.343 |
19 | 10 | 1.218 | −0.728 | 9.008 | 0.992 | 9.588 | 0.412 |
20 | 4.8 | 2.919 | −2.867 | 3.925 | 0.875 | 2.593 | 2.207 |
21 | 4.1 | 7.113 | 2.887 | 0.000 | 2.597 | 3.372 | 0.728 |
22 | 6.3 | 0.967 | −0.905 | 0.000 | 8.742 | 2.410 | 3.890 |
23 | 1.2 | 0.530 | −0.477 | 1.517 | −0.317 | −0.511 | 1.711 |
24 | 0.065 | −40.557 | 43.557 | −0.087 | 0.152 | 0.989 | −0.924 |
25 | 5.2 | −1.733 | 1.767 | 5.438 | −0.238 | 4.228 | 0.972 |
26 | 0.43 | −0.584 | 0.854 | 0.427 | 0.003 | −0.059 | 0.489 |
27 | 0.49 | −1.420 | 2.180 | 0.000 | −10.243 | 0.608 | −0.118 |
28 | 0.052 | −11.404 | 21.404 | −0.612 | 0.664 | 4.535 | −4.483 |
29 | 10 | 1.796 | 2.304 | 0.000 | 14.530 | 2.228 | 7.772 |
30 | 0.062 | −0.935 | 2.135 | 0.000 | 1.448 | 1.526 | −1.464 |
31 | 0.028 | 2.740 | 2.460 | 0.781 | −0.753 | 1.895 | −1.867 |
32 | 0.053 | 0.325 | −0.297 | −1.352 | 1.405 | 0.606 | −0.553 |
33 | 0.027 | −6.308 | 6.335 | 1.000 | −0.973 | −0.204 | 0.231 |
compounds | Binding energy (Kcal/mol) | Ligand | receptor | interaction | distance | E (kcal/mol) |
1 | −5.38 | 6-ring | THR 219 | pi-H | 4.4 | −0.7 |
2 | −5.21 | 5-ring | THR 219 | pi-H | 3.31 | −0.7 |
6-ring | THR 219 | pi-H | 4.38 | −0.7 | ||
3 | −5.38 | 5-ring | THR 219 | pi-H | 3.29 | −0.8 |
6-ring | THR 219 | pi-H | 4.31 | −0.8 | ||
4 | −5.59 | N 24 | THR 251 | H-acceptor | 3.36 | −0.6 |
5 | −5.61 | I 35 | ARG 31 | H-donor | 4.19 | −0.5 |
N 24 | THR 251 | H-acceptor | 3.27 | −0.9 | ||
6 | −5.42 | 5-ring | THR 219 | pi-H | 3.29 | −0.8 |
6-ring | THR 219 | pi-H | 4.37 | −0.9 | ||
7 | −5.74 | F 38 | ARG 35 | H-acceptor | 3.23 | −0.7 |
8 | −5.53 | N 36 | LYS 27 | H-acceptor | 2.97 | −1.3 |
6-ring | THR 219 | pi-H | 4.62 | −1.2 | ||
5-ring | THR 219 | pi-H | 3.34 | −1.3 | ||
9 | −5.45 | 5-ring | THR 219 | pi-H | 3.29 | −0.8 |
6-ring | THR 219 | pi-H | 4.3 | −0.8 | ||
10 | −5.76 | 5-ring | THR 219 | pi-H | 3.28 | −0.8 |
6-ring | THR 219 | pi-H | 4.29 | −0.9 | ||
11 | −5.80 | CL 34 | THR 251 | H-donor | 3.2 | −0.6 |
N 23 | LYS 27 | H-acceptor | 3.39 | −0.7 | ||
5-ring | THR 219 | pi-H | 4.15 | −0.8 | ||
6-ring | THR 219 | pi-H | 4.82 | −0.8 | ||
12 | −5.75 | N 36 | SER 253 | H-acceptor | 3.81 | −0.8 |
13 | −5.64 | 6-ring | THR 219 | pi-H | 3.98 | −0.9 |
14 | −5.44 | 6-ring | THR 219 | pi-H | 3.98 | −0.9 |
15 | −5.55 | 6-ring | THR 219 | pi-H | 4.19 | −0.6 |
16 | −5.67 | N 24 | THR 251 | H-acceptor | 3.33 | −0.6 |
17 | −5.56 | N 24 | ARG 35 | H-acceptor | 3.26 | −4.4 |
5-ring | THR 219 | pi-H | 3.8 | −1 | ||
18 | −5.46 | 5-ring | THR 219 | pi-H | 3.3 | −1 |
6-ring | THR 219 | pi-H | 4.43 | −1 | ||
19 | −5.59 | N 28 | ARG 35 | H-acceptor | 3.09 | −5.2 |
20 | −5.6 | N 30 | ARG 35 | H-acceptor | 2.97 | −5.4 |
21 | −5.28 | 5-ring | THR 219 | pi-H | 3.77 | −0.6 |
22 | −5.26 | 6-ring | THR 219 | pi-H | 4.43 | −1.2 |
5-ring | THR 219 | pi-H | 3.29 | −1.1 | ||
23 | −5.59 | 5-ring | THR 219 | pi-H | 3.3 | −0.6 |
6-ring | THR 219 | pi-H | 4.3 | −0.7 | ||
24 | −5.23 | N 27 | ARG 35 | H-acceptor | 3.08 | −4.8 |
5-ring | THR 219 | pi-H | 3.77 | −1 | ||
25 | −5.48 | N 27 | THR 33 | H-donor | 2.94 | −1.2 |
26 | −5.66 | 6-ring | THR 219 | pi-H | 4.47 | −1 |
5-ring | THR 219 | pi-H | 3.31 | −1 | ||
27 | −5.53 | 6-ring | THR 219 | pi-H | 4.27 | −0.7 |
28 | −5.46 | N 17 | THR 219 | H-acceptor | 2.93 | 24.3 |
29 | −5.79 | N 29 | ARG 35 | H-acceptor | 2.98 | −3.9 |
30 | −5.38 | N 24 | THR 219 | H-acceptor | 2.9 | −2.7 |
31 | −5.3 | BR 17 | THR 251 | H-donor | 3.55 | −0.6 |
N 24 | LYS 27 | H-acceptor | 3.34 | −1 | ||
5-ring | THR 219 | pi-H | 3.4 | −0.6 | ||
6-ring | THR 219 | pi-H | 4.45 | −0.6 | ||
32 | −5.4 | N 26 | THR 219 | H-acceptor | 3.02 | −0.7 |
33 | −5.42 | N 26 | ARG 35 | H-acceptor | 3.21 | −1 |