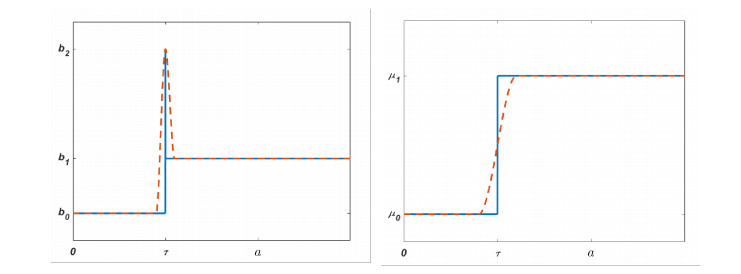
Citation: Cristeta U. Jamilla, Renier G. Mendoza, Victoria May P. Mendoza. Explicit solution of a Lotka-Sharpe-McKendrick system involving neutral delay differential equations using the r-Lambert W function[J]. Mathematical Biosciences and Engineering, 2020, 17(5): 5686-5708. doi: 10.3934/mbe.2020306
[1] | Sun Yi, Patrick W. Nelson, A. Galip Ulsoy . Delay differential equations via the matrix lambert w function and bifurcation analysis: application to machine tool chatter. Mathematical Biosciences and Engineering, 2007, 4(2): 355-368. doi: 10.3934/mbe.2007.4.355 |
[2] | Bo Zheng, Lihong Chen, Qiwen Sun . Analyzing the control of dengue by releasing Wolbachia-infected male mosquitoes through a delay differential equation model. Mathematical Biosciences and Engineering, 2019, 16(5): 5531-5550. doi: 10.3934/mbe.2019275 |
[3] | Jinliang Wang, Ran Zhang, Toshikazu Kuniya . A note on dynamics of an age-of-infection cholera model. Mathematical Biosciences and Engineering, 2016, 13(1): 227-247. doi: 10.3934/mbe.2016.13.227 |
[4] | Yanfeng Liang, David Greenhalgh . Estimation of the expected number of cases of microcephaly in Brazil as a result of Zika. Mathematical Biosciences and Engineering, 2019, 16(6): 8217-8242. doi: 10.3934/mbe.2019416 |
[5] | Andrei Korobeinikov, Philip K. Maini . A Lyapunov function and global properties for SIR and SEIR epidemiological models with nonlinear incidence. Mathematical Biosciences and Engineering, 2004, 1(1): 57-60. doi: 10.3934/mbe.2004.1.57 |
[6] | Tyler Cassidy, Morgan Craig, Antony R. Humphries . Equivalences between age structured models and state dependent distributed delay differential equations. Mathematical Biosciences and Engineering, 2019, 16(5): 5419-5450. doi: 10.3934/mbe.2019270 |
[7] | B. Spagnolo, D. Valenti, A. Fiasconaro . Noise in ecosystems: A short review. Mathematical Biosciences and Engineering, 2004, 1(1): 185-211. doi: 10.3934/mbe.2004.1.185 |
[8] | Mohsen Dlala, Sharifah Obaid Alrashidi . Rapid exponential stabilization of Lotka-McKendrick's equation via event-triggered impulsive control. Mathematical Biosciences and Engineering, 2021, 18(6): 9121-9131. doi: 10.3934/mbe.2021449 |
[9] | Saralees Nadarajah . Remark on the Paper by Rao And Kakehashi (2005). Mathematical Biosciences and Engineering, 2006, 3(2): 385-387. doi: 10.3934/mbe.2006.3.385 |
[10] | Dongxue Yan, Xingfu Zou . Dynamics of an epidemic model with relapse over a two-patch environment. Mathematical Biosciences and Engineering, 2020, 17(5): 6098-6127. doi: 10.3934/mbe.2020324 |
Delay differential equation (DDE) is a type of differential equation (DE) where the time derivatives at the present time are dependent on the solution and its derivatives at a previous time. A k-th order DDE takes the form
y(k)(t)=f(t,y(t),…,y(k−1)(t),y(d0),…,y(k)(dk)), | (1.1) |
where dj=dj(t,y(t)) is called the delay satisfying dj≤t for all times t on the interval [t0,t1], j=0,…,k. A DDE is said to have discrete delays if the delays are constant. DDEs can have a single delay or multiple delays. A DDE is of retarded type (RDDE) when there is no time-delay in the derivative terms of the DDE. Its simplest form is
˙y(t)=f(t,y(t),y(d0)). |
A DDE is of neutral type (NDDE) if there are delays in the derivatives or in the solution of the DDE. Its first-order form can be written as
˙y(t)=f(t,y(t),y(d0),˙y(d1)). |
Recall that the unique solution to an ordinary differential equation (ODE) is dependent on an initial condition. Analogously, the unique solution to a DDE is dependent on some initial function ϕ(t) defined on a previous time, say [−h,0], for some delay h∈R+. The function ϕ(t) is called the preshape or history function.
Asl and Ulsoy showed an approach for obtaining analytical solutions to systems of DDEs, particularly, first-order, linear, constant-coefficient RDDEs with constant delays using the Lambert W function [1]. This was then extended by Yi to solve nonhomogeneous systems [2,3,4]. These results on DDEs are notable since there are few studies on explicit solutions to these types of differential equations.
The Lambert W function, also known as the omega function, is defined to be the function W(a) satisfying
W(a)eW(a)−a=0. | (1.2) |
If a is a nonzero real number, then there are at most two real values of W(a) satisfying (1.2). If a is a complex number, then there is an infinite number of complex values of W(a) satisfying (1.2). An in-depth discussion on the Lambert W function, as well as the branch cut, asymptotes, and other properties, are found in [5].
A generalization of the Lambert W function, introduced by Mező [6,7,8], is defined as a multi-valued inverse of the transcendental function
e−x=a(x−t1)(x−t2)…(x−tn)(x−s1)(x−s2)…(x−sm) |
at a, where a∈C, a≠0 and n,m∈N. We consider a simple form of the generalization,
e−x=a(x−t1)(x−s1), | (1.3) |
with n and m equal to 1. Equation (1.3) can be transformed into the form as xex+rx=a, where r∈R, and hence, is coined as the r-Lambert W function. Therefore, the r-Lambert W function is defined to be the function Wr(a) satisfying the equation
Wr(a)eWr(a)+rWr(a)−a=0. | (1.4) |
In (1.4), we note the presence of the term rWr(a), which does not appear in the definition of the Lambert W function in (1.2). If a is a nonzero real number, then depending on the value of r, there can be one, two, or three real solutions of (1.4) with respect to Wr(a). This means that there are at most three branches of the r-Lambert W function, denoted W(r,0)(x),W(r,−1)(x), and W(r,−2)(x), having nonempty intersection with the real line. Extending to the complex plane, if a is a complex number, then (1.4) has infinitely many complex solutions Wr(a). This is similar to the solutions of (1.2) in the complex plane. However, Mező suggests that there are different structures of the branches of the r-Lambert W function depending on the value of r [8]. For a discussion on the solutions of (1.4), the branch structures, and other properties of the r-Lambert W function, we refer the readers to [7,8]. The generalized Lambert W function appears in the study of the solution to the Bose-Fermi mixtures [6] and on the asymptotic estimation of the Bell and generalized Bell numbers [9]. In [10], it was shown how the generalized Lambert W function can be used to obtain a closed-form of the inverse of Langevin and Brillouin functions. These functions are used in several fields of physics. In [11], it was illustrated how the r-Lambert W function can be used to characterize the equilibrium strategy in the symmetric two-player Hirshleifer contest. In [12], the r-Lambert function was used to show the asymptotic equivalence of the proximity operator of the logistic function and a composition of polynomial and exponential functions. For other applications of the generalized Lambert W function in engineering, physics, and biology, we refer the readers to [13,14,15,16,17,18,19,20,21,22,23,24,25,26,27,28].
In [29], Jamilla et. al showed an approach for obtaining an analytical solution to first-order, linear, constant-coefficient NDDEs with constant delays using the r-Lambert W function. The solution takes the form of a series whose terms are dependent on the parameters and the preshape function of the NDDE. In this paper, we adopt an age-structured population model described by a system of ODEs and NDDEs. We utilize the r-Lambert W function to provide an explicit solution to the model using the approach proposed in [29]. One advantage of this method is that its form is similar to the form of the solution of ODEs [1]. Moreover, as stated above, the terms in the obtained solution are dependent on the parameters of the NDDE, and hence, one can analyze the effect on the solution of varying parameter values.
The outline of this paper is as follows. We discuss in section 2 the Lotka-Sharpe-McKendrick age-structured population model presented in [30]. We show how the model is reduced to a system of ODEs and NDDEs, along with the necessary conditions and relationships of the parameters of the reduced and original model. In section 3, we solve the reduced system using the r-Lambert W function. An approach to numerically approximate the solution obtained in section 3 is detailed in section 4. Because the true solution of the model is not available, we compare our method to the MATLAB built-in solvers. We test our method by varying essential parameters and initial conditions, which we illustrate in three examples. The summary, conclusion, and future works are in section 5.
Bocharov and Hadeler in [30] considered a linear Lotka-Sharpe-McKendrick system of an age-structured population given by
nt+na+μ(a)n=0, | (2.1) |
n(t,0)=∫∞0b(a)n(t,a)da, | (2.2) |
n(0,a)=n0(a), | (2.3) |
where n(t,a) is a non-normalized age distribution of the population at time t, a is the chronological age, μ(a) is the mortality rate, and b(a) is the fertility rate. Both μ(a) and b(a) are functions dependent on age, and are assumed to be sums of step functions and delta peaks of the following forms:
μ(a)=μ0+(μ1−μ0)Hτ(a)+μ2δτ(a), | (2.4) |
b(a)=b0+(b1−b0)Hτ(a)+b2δτ(a), | (2.5) |
where
Hτ(a)={0 for a<τ1 for a≥τ, |
δτ(a) is the Dirac delta function, and μk and bk, k=0,1,2, represent death and birth rates, respectively. We assume that μk and bk are nonnegative. The coefficients μk and bk are chosen so that they mimic the qualitative behavior of real populations such as non-constant mortality, reproductive window, etc. [30]. In Figure 1, we see three coefficients b0, b1, b2 that constitute the fertility rate b(a). When a<τ, then b(a)=b0, while if a>τ, b(a)=b1. Notice that b(a) has a peak at a=τ. Similarly, the coefficients μ0 and μ1 appear in μ(a), and the graph of μ(a) has a jump at a=τ. The functions for the mortality and fertility rates are chosen such that the rates are different when a≤τ and when a>τ. Based on this, the population is divided into two classes, the juveniles (a≤τ) and the adults (a>τ), each with corresponding mortality and fertility rates.
The following new variables are then introduced:
U(t)=∫τ0n(t,a)da,V(t)=∫∞τn(t,a)da, |
where U(t) is the population of the juveniles and V(t) is the population of the adults at time t. We assume that the juveniles do not reproduce (b0=0). For example, the nymph or larval stage of most insects lacks functional reproductive organs [31]. We also assume that there is no sudden increase in the death rate at the age τ (μ2=0). Using the functions U(t) and V(t), the partial differential Eq (2.1) is transformed into a system of the form
˙U(t)={−μ0U(t)+b1V(t)+(b2−1)n0(τ−t)e−μ0t,0≤t≤τ,c0U(t)+c1V(t)+c2V(t−τ)+c3˙V(t−τ),t>τ, | (2.6) |
˙V(t)={n0(τ−t)e−μ0t−μ1V(t),0≤t≤τ,a1V(t−τ)+a2˙V(t−τ)−a3V(t),t>τ, | (2.7) |
with initial conditions
U(0)=∫τ0n(0,a)da=∫τ0n0(a)da, | (2.8) |
V(0)=∫∞τn(0,a)da=∫∞τn0(a)da, | (2.9) |
dependent on n0(a).
The parameters ai and cj are related to the parameters μk and bk in the following manner:
c0=−μ0,c1=b1,c2=(b2−1)(b1+b2μ1)e−μ0τ,c3=(b2−1)b2e−μ0τ,a1=(b1+b2μ1)e−μ0τ,a2=b2e−μ0τ,a3=μ1, | (2.10) |
where i=1,2,3 and j=0,1,2,3. Moreover, from [30], it is necessary that ai≥0 for all i and that a1≥a2a3.
We see that the partial differential equation in (2.1) is reduced to a system involving ODEs and NDDEs. Throughout this paper, we consider the age-structured population model (2.6)–(2.9). We present an explicit solution to this model and illustrate numerically its solution using the r-Lambert W function.
In this section, we consider system (2.6)–(2.7), along with the initial conditions (2.8) and (2.9). First, we determine the solutions U(t) and V(t) to the model for t∈[0,τ]. The solutions on [0,τ] are the preshape functions necessary to find the solutions U(t) and V(t) for t>τ.
We begin by solving for U(t) and V(t), t∈[0,τ], from the ODEs in (2.6) and (2.7). Note that V(t) is independent of U(t), and thus, can be solved separately. Applying techniques in solving ODEs, we get
V(t)=[∫n0(τ−t)e(μ1−μ0)tdt+C1]e−μ1t, | (3.1) |
where C1 is an arbitrary constant. Next, we solve for C1 using the initial condition V(0). We let
X(t)=∫n0(τ−t)e(μ1−μ0)tdt. | (3.2) |
Substituting (3.2) to (3.1) and solving for C1 at t=0, we get
C1=V(0)−X(0). |
Therefore,
V(t)=[X(t)+V(0)−X(0)]e−μ1t for t∈[0,τ]. | (3.3) |
Next, we solve for U(t) for t∈[0,τ]. We plug-in the expression for V(t) from (3.3) to the first equation in (2.6). The resulting equation is a non-homogeneous ODE, and its solution is given by
U(t)=[∫(eμ0tb1V(t)+(b2−1)n0(τ−t))dt+C2]e−μ0t, | (3.4) |
where C2 is an arbitrary constant. We then solve for C2 by letting
Y(t)=b1∫V(t)eμ0tdt, |
and
Z(t)=(b2−1)∫n0(τ−t)dt. |
Using the initial condition U(0), we get
C2=U(0)−Y(0)−Z(0). |
Thus,
U(t)=[Y(t)+Z(t)+U(0)−Y(0)−Z(0)]e−μ0t for t∈[0,τ]. | (3.5) |
We now solve system (2.6)–(2.9) for t>τ. Since V(t) is independent of U(t), we solve V(t) first following the methodology in [29]. Suppose that the solution V(t) to the second equation in (2.7) is of the form eλt. Plugging in V(t)=eλt into the NDDE, we get
λeλt+a3eλt−a1eλ(t−τ)−a2λeλ(t−τ)=0. |
Multiplying both sides of the equation by τeτ(λ+a3)−λt yields
τ(λ+a3)eτ(λ+a3)−a1τeτa3−a2τλeτa3=0. |
Now, adding a constant term −a2τa3eτa3 to both sides of the equation and rearranging terms, we obtain
τ(λ+a3)eτ(λ+a3)−a2eτa3τ(λ+a3)=a1τeτa3−a2τa3eτa3, | (3.6) |
which is of the form xex+rx=a, with x=τ(λ+a3), a=a1τeτa3−a2τa3eτa3, and r=−a2eτa3. Using the r-Lambert W function, we can write λ as
λ=1τWr(a1τeτa3+ra3τ)−a3, |
where Wr is defined to be solution of the r-Lambert W function. Noting that there is an infinite number of solutions to the r-Lambert W function, we express V(t) as
V(t)=∞∑k=−∞Ckeλkt, | (3.7) |
where
λk=1τW(r,k)(a1τeτa3+ra3τ)−a3, | (3.8) |
W(r,k) is defined to be the kth branch of the r-Lambert W function with r=−a2eτa3, and Ck is dependent on V(t) for t∈[0,τ].
Next, we solve for U(t) for t>τ. From (3.7), we obtain
V(t−τ)=∞∑k=−∞Ckeλk(t−τ),˙V(t)=∞∑k=−∞λkCkeλkt, |
and
˙V(t−τ)=∞∑k=−∞λkCkeλk(t−τ). |
Substituting these expressions to (2.6), we get
˙U(t)=c0U(t)+c1∞∑k=−∞Ckeλkt+c2∞∑k=−∞Ckeλk(t−τ)+c3∞∑k=−∞λkCkeλk(t−τ). | (3.9) |
Suppose that a solution to the associated homogeneous DE ˙U(t)=c0U(t) is of the form U(t)=eλt. Then ˙U(t)=λeλt, and hence, λ=c0. Thus, the complementary function for (3.9) is
Ug(t)=Aec0t, | (3.10) |
for some constant A. To find a particular solution Up(t) to (3.9), suppose that
Up(t)=∞∑k=−∞αkeβkt. |
Then
˙Up(t)=∞∑k=−∞αkβkeβkt. |
From (3.9), we have
∞∑k=−∞αkβkeβkt=c0∞∑k=−∞αkeβkt+c1∞∑k=−∞Ckeλkt+c2∞∑k=−∞Ckeλk(t−τ)+c3∞∑k=−∞λkCkeλk(t−τ). |
Rearranging the terms, we get
∞∑k=−∞(βk−c0)αkeβkt=∞∑k=−∞γkCkeλkt, | (3.11) |
where
γk=c1+c2e−λkτ+c3λke−λkτ. |
Comparing coefficients on both sides of (3.11), it follows that for each k,
βk=λk |
and
(βk−c0)αk=γkCk=(c1+c2e−λkτ+c3λke−λkτ)Ck. |
Then
(λk−c0)αk=(c1+c2e−λkτ+c3λke−λkτ)Ckαk=(c1+c2e−λkτ+c3λke−λkτ)Ckλk−c0, |
and
U(t)=Ug(t)+Up(t) for t>τ. | (3.12) |
Now, we solve for A in (3.10). Since we are solving for U(t) for t>τ, then it follows from (3.12) that the initial condition is given by
U(τ)=Aec0τ+∞∑k=−∞αkeλkτ. |
Hence,
A=(U(τ)−∞∑k=−∞αkeλkτ)e−c0τ. |
Finally, the solution to system (2.6)–(2.9) is given by
U(t)={(Y(t)+Z(t)+U(0)−Y(0)−Z(0))e−μ0t,0<t≤τ,(U(τ)−∞∑k=−∞αkeλkτ)ec0(t−τ)+∞∑k=−∞αkeλkt,t>τ, | (3.13) |
V(t)={(V(0)−X(0)+X(t))e−μ1t,0<t≤τ,∞∑k=−∞Ckeλkt,t>τ, | (3.14) |
where
X(t)=∫n0(τ−t)e(μ1−μ0)tdt,Y(t)=b1∫V(t)eμ0tdt,Z(t)=(b2−1)∫n0(τ−t)dt,αk=(c1+c2e−λkτ+c3λke−λkτ)Ckλk−c0,λk=1τW(r,k)(a3τr+a1τea3τ)−a3,r=−a2eτa3, |
and Ck is dependent on V(t) on [0,τ].
Note that the explicit solution computed in the previous section for t>τ involves an infinite series, and thus, cannot be exactly solved. We can only approximate the solution by limiting the summations, i.e., limiting the number of solutions to the r-Lambert W function being considered. For N∈N sufficiently large, we set
{U(t)≈(U(τ)−N∑k=−Nαkeλkτ)ec0(t−τ)+N∑k=−Nαkeλkt,V(t)≈N∑k=−NCkeλkt, | (4.1) |
on the interval [τ,T], where T>τ. Also, note that the solution V(t) on (τ,∞) is dependent on a history function ϕ(t), which is V(t) on the interval [0,τ]. To solve for V(t) numerically on MATLAB, we need an approximate for the coefficient vector Ck. For this, we use the procedure discussed in [29]. The history function ϕ(t) can be approximated as follows:
ϕ(t)≈N∑k=−NCkζk(t), | (4.2) |
where ζk(t)=eλkt, with the same λk as in (3.8). Partitioning the interval [0,τ] to get 2N+1 points, equation (4.2) becomes
{ϕ(−h)ϕ(−h+h2N)ϕ(−h+2h2N)⋮ϕ(0)}=(ζ−N(−h)⋯ζN(−h)ζ−N(−h+h2N)⋯ζN(−h+h2N)ζ−N(−h+2h2N)⋯ζN(−h+2h2N)⋮⋮ζ−N(0)⋯ζN(0)){C−NC−N+1C−N+2⋮CN}. |
Solving the system above gives the coefficients Ck,k∈{−N,…,N} in (4.1).
We illustrate in the following three examples the solution obtained in section 3 using the approximation (4.1). In the first two examples, we vary the initial age distribution n0(a). In the third example, we investigate under certain conditions the long-term behavior of the solution. The parameter t represents time in months. Because we do not know the true solution to system (2.6)–(2.9), we compare our obtained solution with the solution using the MATLAB built-in functions ode23 and ddensd.
In the next two examples, we use the parameter values shown in Table 1.
parameter | b1 | b2 | μ0 | μ1 | τ |
value | 0.0133 | 0.045 | 0.0011 | 0.00056 | 36 |
We set T=144 and compute for the following parameters:
c0=−μ0=−0.0011,c1=b1=0.0133,c2=(b2−1)(b1+b2μ1)e−μ0τ=(0.045−1)(0.0133+0.045(0.00056))e−0.0011(36)=−0.0122,c3=(b2−1)b2e−μ0τ=(0.045−1)0.045e−0.0011(36)=−0.0413,a1=(b1+b2μ1)e−μ0τ=(0.0133+0.045(0.00056))e−0.0011(36)=0.0128,a2=b2e−μ0τ=0.045e−0.0011(36)=0.0433,a3=μ1=0.00056. |
Example 1. We set the initial age distribution to be uniform, that is,
n0(a)=1,0<a≤amax=50, |
where amax is the maximum age in the population. Thus, the initial conditions are
U(0)=∫τ0n(0,a)da=36,V(0)=∫∞τn(0,a)da=14. |
For t∈[0,36], the solutions V(t) and U(t) are given by
V(t)=[V(0)−X(0)+X(t)]e−μ1t=1865.9e−0.00056t−1851.9e−0.0011t, | (4.3) |
U(t)=[Y(t)+Z(t)+U(0)−Y(0)−Z(0)]e−μ0t=45956e−0.00056t−(25.5853t+45920)e−0.0011t, | (4.4) |
with
X(t)=∫n0(τ−t)e(μ1−μ0)tdt=−1851.9e−0.00054t,X(0)=−1851.9e−0.00054⋅0=−1851.9,Y(t)=b1∫V(t)eμ0tdt=45956e0.00054t−24.6303t,Y(0)=45956e0.00054⋅0−24.6303⋅0=45956,Z(t)=(b2−1)∫n0(τ−t)dt=−0.955t,Z(0)=−0.955⋅0=0. |
Moreover, the approximate solutions U(t) and V(t) on the interval [36, 144] are given by
U(t)=(U(36)−N∑k=−Nαkeλkτ)ec0(t−τ)+N∑k=−Nαkeλkt=(16.40079−N∑k=−Nαke36λk)e−0.0011(t−36)+N∑k=−Nαkeλkt, | (4.5) |
and
V(t)=N∑k=−NCkeλkt, | (4.6) |
where
αk=[c1+c2e−λkτ+c3λke−λkτ]Ckλk−c0=[0.0133−(0.0122+0.0413λk)e−36λk]Ckλk+0.0011,λk=1τW(r,k)(a3τr+a1τea3τ)−a3=0.0277W(r,k)(0.4693)−0.00056,r=−a2ea3τ=−0.0442, |
and Ck is a column vector dependent on ϕ(t)=V(t) for t∈[0,36].
We evaluate the explicit solutions U(t) and V(t) on the interval [0,144]. Note that we approximate the solutions U(t) and V(t) on the interval [36, 144] using N=50. We also compute for the solution using the built-in functions ode23 and ddensd from MATLAB with stepsize s=0.01. We then plot our proposed solutions, U(t) and V(t), together with the solutions obtained using the MATLAB built-in functions, denoted by UM(t) and VM(t), for 30 equally spaced points on [0,144]. Figures 2a and 2b show the plots of these results.
Notice that there is a decline in the juvenile population for the first 36 months, compared to the steady increase in the adult population. Moreover, there is an almost steady increase for both populations for t>36. Also, at around t=76 months, the total population is doubled, and at t=144 months, the total population is quadrupled.
Comparing our solutions U(t) and V(t), with the numerical solutions UM(t) and VM(t) using the built-in functions at 30 different points, we see that the two solvers obtain similar results. The relative differences in approximation are
RU=30∑i=1‖UM(ti)−U(ti)‖2‖UM(ti)‖2=9.20418×10−10 and RV=30∑i=1‖VM(ti)−V(ti)‖2‖VM(ti)‖2=9.30018×10−10. |
Going back to system (2.6)–(2.9) and the parameter values (2.10), notice that the absence of the parameter b2 will transform the NDDEs into RDDEs. Thus, we consider b2, together with the delay τ, as the two most significant parameters. To show that our method is robust, we implement our method by varying the parameter values of b2 and τ. We then compare our results with the MATLAB built-in functions using the following measure of relative difference
R(b2,τ)=30∑i=1‖UM(b2,τ,ti)−U(b2,τ,ti)‖2‖UM(b2,τ,ti)‖2+‖VM(b2,τ,ti)−V(b2,τ,ti)‖2‖VM(b2,τ,ti)‖2, | (4.7) |
where ti,∈[0,144],i=1,...,30. We use the same values in Table 1 for the parameters b1, μ0, and μ1, and choose b2 and τ such that b2∈[0.0013,0.0045] and τ∈[24,48]. In particular, we evaluate R(b2,τ) at 50 different values of b2 and τ, and create a heat map to compare the obtained results. Figures 3a and 3b show the values of R in (4.4) for different values of the parameters b2 and τ when N=10 and N=50, respectively.
Notice that the relative difference when N=50 is smaller than when N=10, for all b2 and τ. Also, the minimum value of R is 4.75501×10−8 when N=10, while the maximum value is 1.83404×10−5. When N=50, the maximum value of R is 6.96986×10−8, and the minimum value is 3.75354×10−10. This shows that when b2 varies between [0.0013,0.0045] and τ varies between [24,48], our proposed method can give a good approximate solution.
Example 2. We also consider a normal distribution as the initial age distribution, given by
n0(a)=25σ√2πe−(a−25)22σ2,0≤a≤amax=50, |
where μ and σ are the mean and standard deviation, respectively. The values of μ and σ are set to 25 and 5, respectively. This distribution follows a bell-shape structure and indicates that the age a with the highest number of initial population is 25. The plot of n0(a) is illustrated in Figure 4.
Again, we set the maximum age to be 50. Solving for the initial conditions, we have
U(0)=∫τ0n(0,a)da=∫τ0n0(a)da=∫360255√2πe−(a−25)22⋅52da, | (4.8) |
V(0)=∫∞τn(0,a)da=∫∞τn0(a)da=∫5036255√2πe−(a−25)22⋅52da. | (4.9) |
We let t=a−255√2. Then we have dt=da5√2. Transforming (4.8) and (4.9), we have
U(0)=25√π∫36−255√20−255√2e−t2dt=252(−2√π∫−255√20e−t2dt+2√π∫115√20e−t2dt), |
and
V(0)=25√π∫50−255√236−255√2e−t2dt=252(2√π∫255√20e−t2dt−2√π∫115√20e−t2dt). |
Note that the addends above for U(0) and V(0) are similar to the form of the error function defined as
erf(x)=2√π∫x0e−t2dt. |
The error function arises when integrating the normal distribution. Substituting erf(x) to U(0) and V(0), we get
U(0)=252(erf(5√2)+erf(115√2)), |
and
V(0)=252(erf(5√2)−erf(115√2)). |
For t∈[0,36], the solutions V(t) and U(t) are given by
V(t)=[V(0)−X(0)+X(t)]e−μ1t=[12.42601erf(√2(t−10.9865)10)+12.07809]e−0.00056t, | (4.10) |
U(t)=[Y(t)+Z(t)+U(0)−Y(0)−Z(0)]e−μ0t=(306.04815erf(√2(t−10.9865)10)+306.03984)e−0.00056t−(319.80787erf(√2(t−11)10)+294.82334)e−0.0011t, | (4.11) |
with
X(t)=∫n0(τ−t)e(μ1−μ0)tdt=252⋅e−0.0059363⋅erf[√2(−10.9865+t)10],X(0)=252⋅e−0.0059363⋅erf[√2(−10.9865+0)10]=−12.07809,Y(t)=b1∫V(t)eμ0tdt=25(12.24192erf(√2(t−10.9865)10)+12.24159)e0.00054t−25⋅12.31481⋅erf(√2(t−11)10),Y(0)=25(12.24192erf(√2(0−10.9865)10)+12.24159)e0.00054⋅0−25⋅12.31481⋅erf(√2(0−11)10)=307.87019, |
and
Z(t)=(b2−1)∫n0(τ−t)dt=−0.955⋅252erf(t−115√2)=−11.9375erf(√2(t−11)10),Z(0)=−11.9375erf(√2(0−11)10)=11.60555. |
Approximate solutions U(t) and V(t) on the interval [36,144] are given by
U(t)=(U(36)−N∑k=−Nαkeλkτ)ec0(t−τ)+N∑k=−Nαkeλkt=(9.10443−N∑k=−Nαke36λk)e−0.0011(t−36)+N∑k=−Nαkeλkt, | (12) |
and
V(t)=N∑k=−NCkeλkt, | (4.13) |
where
αk=[c1+c2e−λkτ+c3λke−λkτ]Ckλk−c0=[0.0133−(0.0122+0.0413λk)e−36λk]Ckλk+0.0011,λk=1τW(r,k)(a3τr+a1τea3τ)−a3=0.0277W(r,k)(0.4693)−0.00056,r=−a2ea3τ=−0.0442, |
and Ck is a column vector dependent on ϕ(t)=V(t) for t∈[0,36].
Again, we evaluate the solutions U(t) and V(t) on the interval [0,144] using the parameter values in Table 1. We approximate the solutions on the interval [36,144] with N=50. We also compute for the solutions using the MATLAB built-in functions ode23 and ddensd with stepsize s=0.01. We then compare our solutions U(t) and V(t), with the numerical solutions UM(t) and VM(t) obtained using the built-in functions, for 30 equally spaced points in [0,144]. We see in Figures 5a and 5b that the graphs of U(t) and V(t) are close to the graphs of UM(t) and VM(t), respectively. The relative differences in approximation are
RU=30∑i=1‖UM(ti)−U(ti)‖2‖UM(ti)‖2=1.7498×10−09 and RV=30∑i=1‖VM(ti)−V(ti)‖2‖VM(ti)‖2=3.8372×10−09. |
Similar to the previous example, there is a decline in the population of the juveniles during the first 36 months, while there is an increase in the population of the adults. We can also see that for t>36, both populations increase. In fact, at t=95, the total population is twice the initial total population. It takes more time to double the population in this example, compared to Example 1.
We also compare the difference in approximation of our proposed method and the MATLAB built-in functions. We calculate R(b2,τ) in (4.7) at 50 different values of b2 and τ, and plot the results when N=10 and N=50. Figures 6a and 6b show the results. As expected, the values are smaller when N=50 than when N=10. When N=10, the maximum value of R is 4.51858×10−7, while the minimum value is 1.79839×10−9. When N=50, the maximum value of R is 6.96986×10−8 and the minimum value is 3.75354×10−10. The results show that our proposed explicit formulation can be used to numerically solve (2.6)–(2.9).
Example 3. In this example, we consider the model studied by Gourley and Kuang in [34]. The model is a system of ODEs and NDDEs given by
˙ui(t)={(b2−1)u0(τ−t)e−μt+b0ui(t)+b1um(t)−μui(t),t≤τ,(b2−1){b2˙um(t−τ)+b2d(um(t−τ))+b0ui(t−τ)+b1um(t−τ)}e−μτ+b0ui(t)+b1um(t)−μui(t),t>τ, | (4.14) |
˙um(t)={u0(τ−t)e−μt−d(um(t)),t≤τ,{b2˙um(t−τ)+b2d(um(t−τ))+b0ui(t−τ)+b1um(t−τ)}e−μτ−d(um(t)),t>τ, | (4.15) |
where ui and um represent the immature (juvenile) and mature (adult) members of the population, respectively, and u0(a) represents the initial age distribution of the corresponding PDE system, similar to system (2.1). Moreover, they considered a similar birth rate function (2.5) and set μ as the constant linear death rate for the juveniles and d(um(t)) as the adult mortality function satisfying
d(0)=0 and d(um) strictly increasing in um. | (4.16) |
System (4.8)–(4.9) can be used to describe the population of insects with a very long larval stage and a short adult stage allotted for mating [34]. One example of such insects is the 17-year periodical cicadas, particularly the species Magicicada septendecim, M. cassini, and M. septendecula, which spend years of their life as nymphs, feeding underground on plant root xylems and resurfacing as adults only to mate for a few weeks [35]. Another example is the marine midges of genera Pontomyia and Clunio, which spend a month as benthic larvae and a few hours as adults [36]. Mayflies of order Ephemeroptera are another example, since they generally live from 3–4 weeks to more than 2 years as nymphs, and from a few hours to a few days as adults [37]. Note that the juveniles of these insects are not capable of reproduction and hence, we set the juvenile reproduction to be 0 (b0=0).
Assuming that the adult mortality function d(um) is linear, i.e.,
d(um(t))=kum(t), |
for some k>0, system (4.14)-(4.15) is reduced to
˙ui(t)={−μui(t)+b1um(t)+(b2−1)u0(τ−t)e−μt,t≤τ,−μui(t)+b1um(t)+(b2−1)(b1+b2k)e−μτum(t−τ)+(b2−1)b2e−μτ˙um(t−τ),t>τ, | (4.17) |
˙um(t)={u0(τ−t)e−μt−kum(t),t≤τ,(b1+b2k)e−μτum(t−τ)+b2e−μτ˙um(t−τ)−kum(t),t>τ. | (4.18) |
A theorem on the existence of a solution to system (4.14)-(4.15) is proven in [38], and this also holds for the reduced system. Note that system (4.17)–(4.18) is composed of linear ODEs and NDDEs and thus, using our method, a solution to this system is given by
ui(t)={(y(t)+z(t)+ui(0)−y(0)−z(0))e−μt,t≤τ,(U(τ)−∞∑j=−∞αjeλjτ)e−μ(t−τ)+∞∑j=−∞αjeλjt,t>τ, | (4.19) |
um(t)={(um(0)−x(0)+x(t))e−kt,t≤τ,∞∑j=−∞Ckeλjt,t>τ, | (4.20) |
where
x(t)=∫u0(τ−t)e(k−μ)tdt,y(t)=b1∫um(t)eμtdt,z(t)=(b2−1)∫u0(τ−t)dt,αj={b1+(b2−1)(b1+b2k)e−(μ+λj)τ+(b2−1)b2λje−(μ+λj)τ}Cjλj+μ,λj=1τW(r,j)(kτr+(b1+b2k)τe(k−μ)τ)−k,r=−b2e(k−μ)τ, |
and Cj is dependent on um(t) on [0,τ]. Table 2 lists the parameter values used in the simulations. Furthermore, we set k=0.5 and the initial age distribution to be
u0(a)=10,0≤a≤amax=0.6. | (4.21) |
parameter | b0 | b1 | b2 | μ | τ |
value | 0 | 0 | 1.2 | 0.7 | 0.5 |
The chosen parameter values for b0, b1 and b2 in Table 2 imply that reproduction occurs at a very short period when the insect reaches adulthood. Figures 7a and 7b show the plots of the immature and mature populations (labeled ui and um, respectively) using (4.19)–(4.20) on the interval [0,30] with N=50, and using the MATLAB built-in functions ode23 and ddensd with s=0.01 (labeled u∗i and u∗m, respectively).
The relative differences in approximation of the solutions for 30 equally spaced points in [0,30] are Rui=3.7641×10−07 and Rum=3.7869×10−06, which imply that ui(t) and um(t) are close to u∗i(t) and u∗m(t), respectively. Moreover, we observe in Figure 7b that as t→∞, um(t) converges to 0. Also, since the juvenile population is dependent on the adult population, ui(t) also converges to 0 (Figure 7a). This convergence agrees with Theorem 2 of [34], which states that if b0=b1=0 and b2eμt<1, and d(um(t)) is a continuous strictly monotonic increasing function of um satisfying d(0)=0, then um→0 as t→∞.
Next, we consider the case when b1≠0. We verify the asymptotic behavior of the resulting system using Theorem 3 in [34], which states that if b0=0, b1>0,
b1ume−μτ<d(um)(1−b2e−μτ) ∀um>0, |
and d(um) is a continuous strictly monotonic increasing function of um satisfying d(0)=0, then um→0 as t→∞. Using our assumption on d(um) and simplifying the inequality above, we infer that if we set
k>1−b2e−μτb1e−μτ, |
then um→0. Consequently, using the parameter values for b0,b2,μ and τ in Table 2 and setting b1=2, then um→0 if k>9.1296. Figures 8a and 8b show our solutions ui(t) and um(t) with k=13 and N=200, in comparison with the solutions u∗i(t) and u∗m(t) using the MATLAB built-in functions with s=0.01. We see in Figures 8a and 8b that ui→0 and um→0 as t→∞. Moreover, the relative differences of the solutions for 30 equally spaced points on [0,50] are Rui=8.0737×10−07 and Rum=1.1265×10−05. This implies that our solution is close to the solution obtained using the MATLAB built-in solvers.
In this example, we have shown how our proposed numerical method can be used to investigate the asymptotic behavior of the solution.
We have presented an explicit solution to an age-structured model. By assuming that the juveniles do not reproduce and that there is no peak in the death rate when juveniles become adults, the age-structured model is reduced to a system of ODEs and NDDEs. We have shown that the arising NDDE can be solved using a generalization of the Lambert W function. An explicit solution in the form of an infinite series was obtained. The terms of this series depend on the parameters of the NDDE. Hence, one can identify the effects of changing parameter values in the solution. We tested our approach numerically and compared our computed solution with that of the MATLAB built-in solvers.
For future work, one may study the solution of the problem when there is no assumption on the non-reproduction of juveniles and no peak in the death rate when juveniles become adults. In Example 3, we have shown that our proposed numerical approach can be used to study the long-term behavior of the solution. However, an in-depth theoretical analysis of the convergence of the solution of the model using our proposed explicit solution is not trivial. This would require a thorough analysis of the r-Lambert W function and would demand a separate study. The explicit solution derived in this work relies heavily on the structure of the solutions of (1.4). To deal with a nonlinear model, one needs to study the structure of the solutions of (1.4) that is not only dependent on the constant a but also on time t. This is an exciting direction for future research.
The authors acknowledge the Office of the Chancellor of the University of the Philippines, through the Office of Vice Chancellor for Research and Development, for funding support through the Outright Research Grant.
All authors declare no conflicts of interest in this paper.
[1] | F. M. Asl, G. A. Ulsoy, Analysis of a system of linear delay differential equations, ASME J. Dyn. Sys., Meas., Control, 125 (2003), 215-223. |
[2] | S. Yi, P. W. Nelson, A. G. Ulsoy, Solution of systems of linear delay differential equations via Laplace transformation, in Proceedings of the 45th IEEE Conference on Decision and Control, IEEE, (2006), 2535-2540. |
[3] | S. Yi, P. W. Nelson, A. G. Ulsoy, Survey on analysis of time delayed systems via the Lambert W function, Differ. Equations, 14 (2007), 296-301. |
[4] | S. Yi, A. G. Ulsoy, Solution of a system of linear delay differential equations using the matrix Lambert function, in Proceedings of 2006 American Control Conference, Minneapolis, IEEE, (2006), 2433-2438. |
[5] | R. M. Corless, G. H. Gonnet, D. E. G. Hare, D. J. Jeffrey, D. E. Knuth, On the Lambert w function, Adv. Comput. Math., 5 (1996), 329-359. |
[6] | I. Mezö, G. Keady, Some physical applications of generalized Lambert functions, Eur. J. Phys., 37 (2016), 065802. |
[7] | I. Mezö, A. Baricz, On the generalization of the lambert W function, Trans. Amer. Math. Soc., 369 (2017), 7917-7934. |
[8] | I. Mezö, On the structure of the solution set of a generalized Euler-Lambert equation, J. Math. Anal. Appl., 455, (2017), 538-553. |
[9] | C. B. Corcino, R. B. Corcino, An asymptotic formula for r-Bell numbers with real arguments, ISRN Discrete Math., 2013 (2013). |
[10] | V. Barsan, Inverses of Langevin, Brillouin and related functions: a status report, Rom. Rep. Phys., 72 (2020). |
[11] | C. Ewerhart, G. Z. Sun, Equilibrium in the symmetric two-player Hirshleifer contest: uniqueness and characterization, Econ. Lett., 169 (2018), 51-54. |
[12] | L. M. Briceño-Arias, G. Chierchia, E. Chouzenoux, J. C. Pesquet, A random block-coordinate Douglas-Rachford splitting method with low computational complexity for binary logistic regression, Comput. Optim. Appl., 72 (2019), 707-726. |
[13] | V. Barsan, Simple and accurate approximants of inverse Brillouin functions, J. Magn. Magn. Mater., 473 (2019), 399-402. |
[14] | V. Barsan, Siewert solutions of transcendental equations, generalized Lambert functions and physical applications, Open Phys., 16 (2018), 232-242. |
[15] | V. Barsan, New results concerning the generalized Lambert functions and their applications to solar energy conversion and nanophysics, CTP-Trieste, Spectroscopy and Dynamics of Photoinduced Electronic Excitations (Workshop Poster), 2017. Available from: https://www.researchgate.net. |
[16] | D. Belkić, All the trinomial roots, their powers and logarithms from the Lambert series, Bell polynomials and fox-wright function: illustration for genome multiplicity in survival of irradiated cells, J. Math. Chem., 57 (2019), 59-106. |
[17] | N. Bovenzi, Spin-momentum locking in oxide interfaces and in Weyl semimetals, Ph.D. thesis, University of Leiden, 2018. |
[18] | N. Bovenzi, M. Breitkreiz, T. E. O'Brien, J. Tworzydło, C. W. J. Beenakker, Twisted fermi surface of a thin-film Weyl semimetal, New J. Phys., 20 (2018), 023023. |
[19] | R. M. Digilov, Gravity discharge vessel revisited: an explicit Lambert W function solution, Am. J. Phys., 85 (2017) 510-514. |
[20] | J. Guo, Exact procedure for Einstein-Johnson's sidewall correction in open channel flow, J. Hydraul. Eng., 143 (2017), 06016027. |
[21] | R. Jedynak, A comprehensive study of the mathematical methods used to approximate the inverse Langevin function, Math. Mech. Solids., 24 (2019), 1992-2016. |
[22] | R. Jedynak, New facts concerning the approximation of the inverse Langevin function, J. Nonnewton Fluid Mech., 249 (2017), 8-25. |
[23] | I. Lopez-Garcia, C. S. Lopez-Monsalvo, E. Campero-Littlewood, F. Beltran-Carbajal, E. Campero-Littlewood, Alternative modes of operation for wind energy conversion systems and the generalised Lambert W-function, IET Gener. Transm. Distrib., 12 (2018), 3152-3157. |
[24] | B. C. Marchi, E. M. Arruda, Generalized error-minimizing, rational inverse Langevin approximations, Math. Mech. Solids., 24 (2019), 1630-1647. |
[25] | O. Olendski, Thermodynamic properties of the 1D Robin quantum well, Ann. Phys., 530 (2018). |
[26] | S. Rebollo-Perdomo, C. Vidal, Bifurcation of limit cycles for a family of perturbed Kukles differential systems, Am. Inst. Math. Sci. Discrete Contin. Dyn. Syst. A, 38 (2018), 4189-4202. |
[27] | H. Vazquez-Leal, M. A. Sandoval-Hernandez, J. L. Garcia-Gervacio, A. L. Herrera-May, U. A. Filobello-Nino, PSEM approximations for both branches of Lambert W function with applications, Discrete Dyn. Nat. Soc., 2019 (2019). |
[28] | M. Vono, P. Chainais, Sparse Bayesian binary logistic regression using the split-and-augmented Gibbs sampler, in Proceedings of 2018 IEEE International Workshop on Machine Learning for Signal Processing, IEEE, (2018). |
[29] | C. Jamilla, R. Mendoza, I. Mezö, Solutions of neutral delay differential equations using a generalized Lambert W function, Appl. Math. Comput., 382 (2020), 125334. |
[30] | G. Bocharov, K. P. Hadeler, Structured population models, conservation Laws, and delay equations, J. Differ. Equations, 168 (2000), 212-237. |
[31] | P. J. Gullan, P. S. Cranston, The Insects: An Outline of Entomology, 5th edition, John Wiley & Sons, Ltd, 2014. |
[32] | M. A. Zabek, Understanding population dynamics of feral horses in the Tuan and Toolara State Forest for successful long-term population management, Ph.D. thesis, The University of Queensland, 2015. |
[33] | M. A. Zabek, D. M. Berman, S. P. Blomberg, C. W. Collins, J. Wright, Population dynamics of feral horses (Equus caballus) in an exotic coniferous plantation in Australia, Wildlife Res., 43 (2016), 358-367. |
[34] | S. A. Gourley, Y. Kuang, Dynamics of a neutral delay equation for an insect population with long larval and short adult phases, J. Differ. Equations, 246 (2009), 4653-4669. |
[35] | K. S. Williams, C. Simon, The ecology, behavior and evolution of periodical cicadas, Annu. Rev. Entomol., 40 (1995), 269-295. |
[36] | K. Soong, G. F. Chen, J. R. Cao, Life history studies of the flightless marine midges Pontomyia spp. (Diptera: Chironomidae), Zool. Stud., 38 (1999), 466-473. |
[37] | J. E. Brittain, M. Sartori, Ephemeroptera:(Mayflies), in Encyclopedia of Insects, Academic Press, (2009), 33-75. |
[38] | B. Dorociaková, I. Ilavská, R. Olach, Existence of solutions for an age-structured insect population model with a larval stage, Electron. J. Qual. Theo., 65 (2017), 1-14. |
1. | J. Leonel Rocha, Abdel-Kaddous Taha, Generalized r-Lambert Function in the Analysis of Fixed Points and Bifurcations of Homographic 2-Ricker Maps, 2021, 31, 0218-1274, 2130033, 10.1142/S0218127421300330 | |
2. | Cristeta U. Jamilla, Renier G. Mendoza, Victoria May P. Mendoza, Parameter Estimation in Neutral Delay Differential Equations Using Genetic Algorithm With Multi-Parent Crossover, 2021, 9, 2169-3536, 131348, 10.1109/ACCESS.2021.3113677 | |
3. | Erika Antonette T. Enriquez, Renier G. Mendoza, Arrianne Crystal T. Velasco, Philippine Eagle Optimization Algorithm, 2022, 10, 2169-3536, 29089, 10.1109/ACCESS.2022.3158357 | |
4. | J. Leonel Rocha, Abdel-Kaddous Taha, Generalized Lambert functions in γ-Ricker population models with a Holling type II per-capita birth function, 2023, 120, 10075704, 107187, 10.1016/j.cnsns.2023.107187 | |
5. | Michelle Sherman, Gilbert Kerr, Gilberto González-Parra, Comparison of Symbolic Computations for Solving Linear Delay Differential Equations Using the Laplace Transform Method, 2022, 27, 2297-8747, 81, 10.3390/mca27050081 | |
6. | Mutaz Mohammad, Alexander Trounev, Fathalla A. Rihan, A New Technique for Solving Neutral Delay Differential Equations Based on Euler Wavelets, 2022, 2022, 1099-0526, 1, 10.1155/2022/1753992 | |
7. | C.Y. Chew, G. Teng, Y.S. Lai, Simulation of Erlang and negative binomial distributions using the generalized Lambert W function, 2024, 10, 27724158, 100092, 10.1016/j.jcmds.2024.100092 | |
8. | Michelle Sherman, Gilbert Kerr, Gilberto González-Parra, Analytic solutions of linear neutral and non-neutral delay differential equations using the Laplace transform method: featuring higher order poles and resonance, 2023, 140, 0022-0833, 10.1007/s10665-023-10276-5 | |
9. | Michelle Sherman, Gilbert Kerr, Gilberto González-Parra, Analytical solutions of linear delay-differential equations with Dirac delta function inputs using the Laplace transform, 2023, 42, 2238-3603, 10.1007/s40314-023-02405-8 | |
10. | J. Leonel Rocha, Abdel-Kaddous Taha, Bifurcation Structures of the Homographic γ-Ricker Maps and Their Cusp Points Organization, 2023, 33, 0218-1274, 10.1142/S0218127423300112 | |
11. | Gilbert Kerr, Nehemiah Lopez, Gilberto González-Parra, Analytical Solutions of Systems of Linear Delay Differential Equations by the Laplace Transform: Featuring Limit Cycles, 2024, 29, 2297-8747, 11, 10.3390/mca29010011 |
parameter | b0 | b1 | b2 | μ | τ |
value | 0 | 0 | 1.2 | 0.7 | 0.5 |