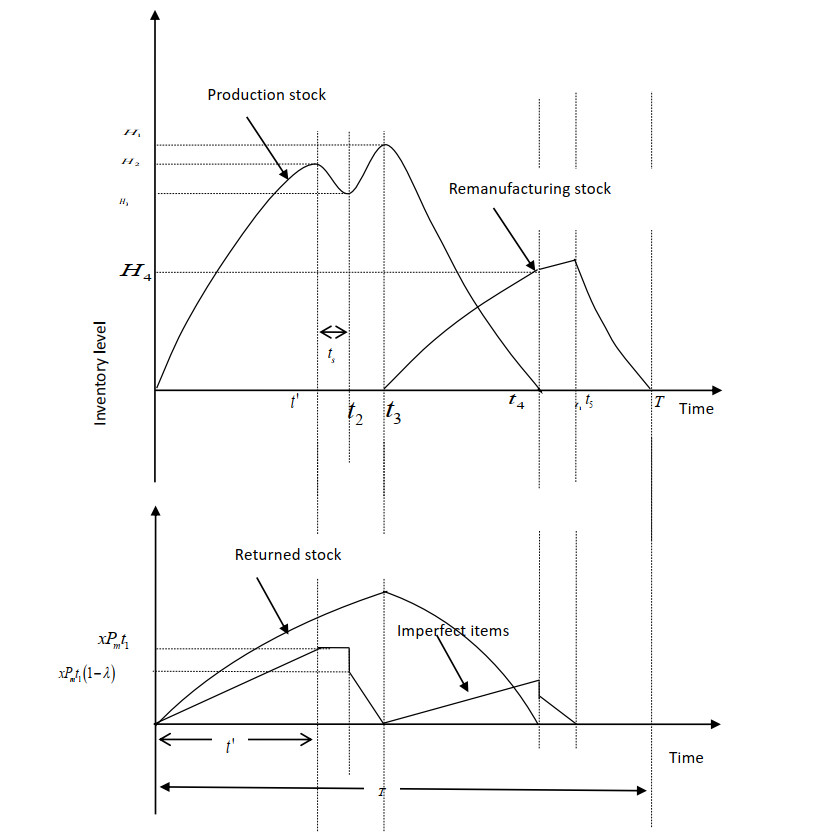
Machine breakdown usually implies unexpected physical damage to machinery due to any reason which requires fixing or replacement to continue the process. This article presentsan investigation of a sustainable model with stochastic machine breakdown. To reduce the risk of disruption,a smart manufacturing system is used. Considering the environmental issues faced by people,the model is developed under a circular economy through end-of-life treatment to recapture the value of the product,labor and resources. The used buyback products are collected,out of which items in good condition are remanufactured and sold in another market while the rest are salvaged. As the production process is not perfectly reliable,the serviceable products go through an automated inspection process,and imperfect items are reworked. A mathematical model is developed for deterioration of items to analyze the optimal replenishment policies,and the results have been illustrated with numerical verification. Based on the analysis,some managerial insights have been provided for decision-makers.
Citation: Neha Saxena, Jitendra Kumar, Shib Sankar Sana. Sustainable production model with stochastic machine breakdown using smart manufacturing under circular economy[J]. Green Finance, 2023, 5(4): 479-511. doi: 10.3934/GF.2023019
[1] | Sa Xu . International comparison of green credit and its enlightenment to China. Green Finance, 2020, 2(1): 75-99. doi: 10.3934/GF.2020005 |
[2] | Brojeswar Pal, Anindya Mandal, Shib Sankar Sana . A closed-loop green supply chain with retailers' competition and product recycling in the green environment under the cap-and-trade policy. Green Finance, 2024, 6(1): 117-161. doi: 10.3934/GF.2024006 |
[3] | Wei-Bin Zhang . Growth, environmental change and business cycles in a multi-regional economy. Green Finance, 2019, 1(2): 205-220. doi: 10.3934/GF.2019.2.205 |
[4] | Blagica Novkovska . Relationship between shadow and green economy: less shadow more green. Green Finance, 2019, 1(2): 130-138. doi: 10.3934/GF.2019.2.130 |
[5] | Rizwan Ullah Khan, Hina Arif, Noor E Sahar, Arif Ali, Munir A. Abbasi . The role of financial resources in SMEs' financial and environmental performance; the mediating role of green innovation. Green Finance, 2022, 4(1): 36-53. doi: 10.3934/GF.2022002 |
[6] | Ana Ramos, Filipa Magalhães, Diogo Neves, Nelson Gonçalves, António Baptista, Teresa Mata, Nuno Correia . Wind energy sustainability in Europe—A review of knowledge gaps, opportunities and circular strategies. Green Finance, 2023, 5(4): 562-602. doi: 10.3934/GF.2023022 |
[7] | Lars Carlsen . Responsible consumption and production in the European Union. A partial order analysis of Eurostat SDG 12 data. Green Finance, 2021, 3(1): 28-45. doi: 10.3934/GF.2021003 |
[8] | Mukul Bhatnagar, Sanjay Taneja, Ercan Özen . A wave of green start-ups in India—The study of green finance as a support system for sustainable entrepreneurship. Green Finance, 2022, 4(2): 253-273. doi: 10.3934/GF.2022012 |
[9] | Rui M. Pereira, Alfredo M. Pereira . Financing a renewable energy feed-in tariff with a tax on carbon dioxide emissions: A dynamic multi-sector general equilibrium analysis for Portugal. Green Finance, 2019, 1(3): 279-296. doi: 10.3934/GF.2019.3.279 |
[10] | Jordi Capó Vicedo, José-Vicente Tomás-Miquel . Decoding sustainable finance: A Multi-Criteria Approach to financial inclusion barriers in High-Income Economies. Green Finance, 2025, 7(1): 83-116. doi: 10.3934/GF.2025004 |
Machine breakdown usually implies unexpected physical damage to machinery due to any reason which requires fixing or replacement to continue the process. This article presentsan investigation of a sustainable model with stochastic machine breakdown. To reduce the risk of disruption,a smart manufacturing system is used. Considering the environmental issues faced by people,the model is developed under a circular economy through end-of-life treatment to recapture the value of the product,labor and resources. The used buyback products are collected,out of which items in good condition are remanufactured and sold in another market while the rest are salvaged. As the production process is not perfectly reliable,the serviceable products go through an automated inspection process,and imperfect items are reworked. A mathematical model is developed for deterioration of items to analyze the optimal replenishment policies,and the results have been illustrated with numerical verification. Based on the analysis,some managerial insights have been provided for decision-makers.
Abbreviations: EPQ: Economic production quantity; RL: Reverse logistics (RL); JIT: Just-in-time; EMQ: Economic manufacturing quantity; CE: Circular economy; EOQ: Economic order quantity
The main focuses of manufacturing firms are production, quality and maintenance of the product. In a cutthroat competitive atmosphere, the decision makers face different challenges day to day to manufacture a superior product and to give better service than others. Technological advancement and growing scientific research change manufacturing infrastructure rapidly. While manufacturing services are gradually becoming more complicated, current facilities can deteriorate. Consequently, machines can randomly shift from an in-control state to an out-of-control state, resulting in machine breakdown. Hence, the study of economic production quantity (EPQ) models for unreliable systems is quite considerable and significant.
Most of the studies developed with EPQ models pay no attention to the reliability factor, thus implicitly assuming completely reliable systems. Machine failures, however, do arise and could be really troublesome, mostly in an extremely mechanized manufacturing environment. Current study efforts attempt to integrate the shortcomings of the manufacturing process and equipment into the classical sizing conclusion structure. This article deals with a manufacturing inventory system for an unreliable system under the assumptions that the machine is subjected to stochastic random breakdown.
The research gap in this context can be articulated as follows:
In the appropriate literature, a substantial body of research has been dedicated to addressing the challenges posed by unreliable systems, particularly those subjected to machine breakdowns. Similarly, extensive work has been presented in the realm of green supply chain inventory, with a focus on sustainability and environmental considerations. However, a significant research gap exists where the joint effects of these two critical issues, namely, unreliable systems due to machine breakdowns and green supply chain inventory control, have not received adequate attention.
While prior research has recognized the impact of machine breakdowns on inventory management and explored strategies to mitigate their disruptive effects, it often overlooks the potential for proactive measures to prevent machine breakdowns. Specifically, there is a lack of investigation into how assessments and maintenance protocols can be employed to transition of a machine from an "in-control" state to an "out-of-control" state. Such proactive measures have the potential to not only reduce the number of imperfect products but also delay the occurrence of machine breakdowns during production runs.
The motivation behind this paper is to bridge this research gap by developing integrated production, inventory and maintenance models that comprehensively consider the joint effects of process decay, machine breakdowns and proactive assessments on optimal lot sizing decisions. This holistic approach acknowledges the dynamic nature of production systems and aims to optimize decision-making in a manner that enhances both system reliability and sustainability, aligning with the principles of green supply chain management. By exploring the synergy between these factors, this research seeks to contribute valuable insights to the field of production and supply chain management, offering a more comprehensive understanding of how to effectively manage inventory under conditions of uncertainty and unreliability while minimizing environmental impact.
The objective of this paper is to address a critical research gap in the field of production and supply chain management by investigating the joint impacts of unreliable systems due to machine breakdowns and green supply chain inventory control. While previous research has extensively examined each of these issues in isolation, there is a lack of comprehensive understanding regarding how they interact and influence inventory control decisions. Therefore, the primary objective of this paper is to develop integrated models that encompass production, inventory management and maintenance, taking into account the combined effects of process decay, machine breakdowns and proactive assessments on optimal lot sizing decisions.
One key objective is to create a holistic framework that considers the dynamic nature of production systems. Specifically, we aim to explore how proactive assessments can transition a machine from an "in-control" state to an "out-of-control" state in a controlled manner, thereby reducing the likelihood of machine breakdowns during production runs. This proactive approach holds the potential to not only enhance system reliability but also optimize inventory management by minimizing the production of imperfect products.
Another central objective of this paper is to align our research with the principles of green supply chain management. We seek to develop strategies that not only improve system reliability but also contribute to sustainability and environmental responsibility. By exploring the synergy between mitigating machine breakdowns and green inventory practices, we aim to provide decision-makers with insights on how to make inventory control decisions that are not only efficient but also environmentally conscious.
Furthermore, this research aims to validate the proposed models and strategies through numerical verification. By applying our models to practical scenarios and conducting numerical analyses, we aim to demonstrate the feasibility and effectiveness of the integrated approach in real-world applications. This verification process is essential in providing empirical support for our proposed strategies and ensuring their practical relevance.
In summary, the primary objectives of this paper are to develop integrated models that consider the joint impacts of process decay, machine breakdowns and proactive assessments on optimal lot sizing decisions. Additionally, we aim to align our research with green supply chain principles and validate our models through numerical verification. By achieving these objectives, we seek to provide a comprehensive framework for enhancing both the reliability and sustainability of inventory control systems in manufacturing and supply chain operations.
This study's novelty lies in its unique approach that combines machine breakdown management, green supply chain principles and proactive assessments within a single framework. It introduces proactive measures to prevent machine breakdowns, aligns inventory control with sustainability goals and validates its strategies through practical testing. This integrated approach offers valuable insights and practical solutions for real-world production and supply chain challenges, making it distinct from existing research. Items are presented in this paper subjected to the stochastic machine breakdown. The failure rate of the production machine is taken to be probabilistic in nature. It is assumed that the manufacturing process is not perfectly reliable. Thus, some non-conforming units are also produced during the manufacturing.
Machine unavailability is an unavoidable phenomenon in many production industries, resulting in three main things: preventive maintenance, corrective maintenance and machine breakdown. A lot of investigation has been made by researchers in this line of research. Groenevelt et al., (1992) developed the EPQ model subjected to stochastic machine breakdown. They studied their model with two replenishment policies. In the first policy, they considered that the machine cannot be repaired after breakdown. In the second one, they assumed that the machine will be fixed after the breakdown. The effects of machine breakdowns and corrective maintenance on the optimal policies were discussed. Liu and Cao (1999) presented an EPQ model with machine failure assuming that the demand rate is probabilistic in nature. They considered the period of failure by Gamma and Weibull distributions and resolved it rapidly and consistently. Al-khateeb (1999) studied an unreliable EPQ model under machine breakdown with general lifetime distribution. It was considered that the manufacturing process is subjected to a stochastic decay from a controllable situation to an uncontrollable situation, follow a stochastic breakdown. Chung (2003) developed a production lot sizing model for a failure-prone machine. He obtained the lower and upper bounds for the optimal lot size. He demonstrated that the optimal cost function is neither convex nor concave. In a later study, Giri and Dohi (2005) also presented a mathematical model of a stochastic economic manufacturing quantity (EMQ) framework. Zhang et al., (2007) formulated inventory levels as a major focus for supply chain management. In their model, they assumed that demand and production rates are constant. Lin (2010) studied a stochastic integrated supplier-retailer inventory problem. Singh and Prasher (2014) examined the role of inventory in a production process with flexible manufacturing, random breakdowns and stochastic repair time. Darma Wangsa and Wee (2018) developed a two-echelon supply chain involving a single vendor and single buyer with stochastic demand. They studied transportation cost, which is a function of shipping weight, distance and transportation modes. Poursoltan et al., (2020) derived an economic production quantity (EPQ) model with partial backlogging. Stochastic inventory deterioration and stochastic machine breakdown were considered. Jauhari et al., (2021) established a two-echelon supply chain inventory model containing a manufacturer and a retailer under a stochastic environment with carbon emission reductions. Emissions from transportation, production and storage activities are included in the model with reductions through carbon tax regulation. Pal and Adhikari (2021) developed an economic production quantity (EPQ) model where production is performed mainly by the original machine. Its buffer production continues when the system encounters a disruption. Deiranlou et al., (2022) introduced the general machine breakdown and repair time distributions. Shortages caused by long exponential repair time were considered. For instance, the manufacturer may procure certain quantities from an available supplier with a non-zero lead time. Sana's (2022) model consisted of a production-inventory model where preventive maintenance takes place at the end of a cycle to ensure smooth performance in the next cycle. The study examined how production systems produce defective products at different rates when "in-control" and when "out-of-control." The defective products are then reworked for costs in parallel systems.
Kelle and Schneider (1992) formulated reliability-type inventory models with minimal safety stock. They studied just-in-time (JIT) production. Panda and Maiti (2009) developed multi-item EPQ models. They studied flexibility and reliability in production process. Demand selling price and production stock dependence were considered. Manna et al., (2018) examined an imperfect production inventory model with production system reliability under two-layer supply chain management. In the model, the reliability parameter depends on the production rate. Islam et al., (2022) worked an inventory model to solve problems of supply uncertainty in response to a demand which expresses a Poisson distribution. One positive aspect of this model is the consideration of random inventory, delivery efficiencies and supplier reliability".
Increasingly, smart production is receiving attention, and its importance is paramount in smooth functioning of the production process in order to ensure the quality of the final product, which directly affects the business. Due to the manufacturer's preference for handling products manually during the entire production process from the raw material to the final product, finding defective products is not uncommon. Automating the process of smart manufacturing reduces the need for manual handling, and monitoring the production processes leaves no room for human error. By minimizing human errors, manufacturers are able to ensure food safety and quality through the use of digital technologies to improve their systems. Moreover, smart production processes improve plant efficiency by providing real-time material, sourcing, production and human resources. With smart manufacturing, all aspects of the system can be analyzed to ensure that the energy efficiency of the whole process is maximized. All of the factory's operations, from turning on and off the lights to scheduling production, can be guided by real-time information regarding energy consumption. Smart manufacturing has been gaining attention in recent years. De Giovanni (2011) presented a vendor managed inventory model with revenue sharing. Artificial intelligence was used for the production process, thereby increasing reliance on and improving performance of the supply chain. A vendor-buyer integration for a probabilistic inventory model was presented by Dey et al., (2021a). The supply chain model was developed for stochastic demand considering smart manufacturing and uniformly distributed lead time. In a subsequent study, Dey et al., (2021b) investigated a cost-effective production policy for a centralized production and remanufacturing model. Smart production was considered to deal with the imperfect production, which is a random variable. Recently, a mathematical model was provided by Kugele et al., (2022) with smart manufacturing. The model was developed with controllable carbon emissions and optimized by using geometric programming. Sarkar et al., (2022a) investigated the use of smart manufacturing processes for energy optimization during biofuel production.
Product life cycles are gradually shrinking, and the shorter life spans force practitioners to adapt to a circular economy (CE). Bringing the product back into the system allows the organization to recapture the value of the product, labor, material and resources. The circular economy has been identified as one of the most effective methods for an industry to ensure environmental sustainability through recycling and remanufacturing (Haupt and Hellweg, 2019). Practices and research have demonstrated that reverse logistics is an intricate procedure that complicates administrative choices trying to accomplish more noteworthy financial advantages (Srivastava, 2007). One of the complexities of remanufacturing is managing the two-supply arrangement of parts, i.e., the forward flow of material and the reverse flow. Reverse Logistics (RL) activities deal with the collection of used material; however, the quantity of buyback products is highly uncertain, which particularly influences the accumulation and replenishment policies (Dekker et al., 2004). The life cycle designing of a consumable product is vital at its earliest phase in order to maximize the life-cycle value of a product. The review presented by Sasikumar and Kannan (2009) emphasized the growing awareness of legislation and customers toward environmental issues. They portrayed two sorting proposals and recommended the research potential of end-of-life treatment. Alinovi et al., (2012) evaluated a stochastic order quantity inventory control model with a mixed manufacturing and remanufacturing strategy such that all manufacturing and procurement processes are integrated with reuse and remanufacturing to meet the demand in a timely and complete manner. The replenishment policies for the production and remanufacturing were provided to get the optimum results. Agrawal et al., (2015) provided a literature review and perspectives in RL. Up to 242 distributed articles were chosen, classified and broken down, and holes in the literature were identified to propose future research openings. Agrawal et al., (2015) additionally strengthened the finding of Sasikumar and Kannan (2009) with respect to the growth in significance of RL in environmental and sustainability concerns. Govindan et al., (2015) developed a review paper for RL and a closed-loop system for exploring future aspects. The point of the paper was to audit recent distributed papers in reverse strategic and closed-loop systems. In the review paper, the holes in the literature were identified to elucidate and to recommend future research openings. Also, a literature review of mathematical modeling was given by Bazan et al., (2016) for RL inventory models. The paper surveyed the literature on the RL stock frameworks that depend on the economic order quantity (EOQ) and EPQ with breakdown which are the principal attributes of related procedures. The literature was studied and reviewed by the particular issues confronted and modeling presumptions. Modak et al., (2018) introduced a two-tier RL model to assess the effects of reprocessing and product quality on pricing strategies. Chen et al., (2019) presented a supply chain model with a closed-loop structure involving a manufacturer, retailer and remanufacturer. They proposed a long-term collection strategy wherein the manufacturer must collaborate with the retailer to improve the remanufacturing process. Recently, Sanni et al., (2020) proposed a model for maximizing profit by tracing the reverse flow of used items. Lu et al., (2021) developed a green manufacturing-recycling network by developing a closed-loop logistics dual-objective optimization model. The study examined several methods to minimize the adverse effects on the environment by implementing environmentally friendly production techniques. To quantify the impact on the environment, the model incorporates a pollution equivalent number. Furthermore, Rentizelas et al., (2021) investigated whether wind turbine blades at the end of their life may be recycled to manufacture composite materials.
In practice, a machine cannot always work well because its spare parts may do malfunctioning at some point of time. It may cause out of stock amid its working time if breakdown period is longer. At the point when the stock level becomes less than the demand, the management unit completely sits out of gear. As a result, a green supply chain inventory model is essential for continuation of manufacturing systems without disruption of supply.
The model is developed here based on the following presumptions and notations.
1. Demand rate is assumed to be constant (Zhang et al., 2007).
2. Machine failure takes place at random during a production run (Poursoltan et al., 2020). We have assumed that the random number1 of breakdowns1 per unit time1 follows the Poisson1 distribution1, with the1 mean equal to β per1 unit time. Thus1, the random1 production time1 to break down should1 obey the1 exponential1 distribution1, with the1 density1 function 1 f(t)=βe−βt and the cumulative1 density1 function 1 F(t)=(1−e−βt).
3. Various studies have found that the reliability of manufacturing processes can be improved through the adoption of smart production technologies, which is regarded as being highly durable (Sarkar and Sarkar, 2022b). Therefore, to reduce the risk of disruption, smart technology is used with technology investment. Smart production is considered, and the cost of smart production is assumed to be
C(P,β,x)={π+γβP+(r1+r2β)+(s1+s2xy)+CpP} |
4. Imperfect production is taken into account and x portion of produced items and y portion of remanufactured items are assumed to be defective. These items are reworked at a rate P1.
5. Among these imperfect items, a small portion λ of the remanufactured items are totally scraped and will not be remanufactured further. Hence, scraped of the first time products and the other imperfect quality products can be repaired.
β | Production rate |
P, | Remanufacturing rate |
D1 | Demand rate |
D2 | Demand rate for remanufactured items |
P1 | Repairing rate of the imperfect items |
R | Returned rate |
x | Reliability of the production process |
y | Reliability of the remanufacturing process |
λ1 | Scrap from the produced items |
λ2 | Scrap from the remanufactured items |
θ | Deterioration rate |
Km | Set up cost $ per unit cycle for the production process |
K | Set up cost $ per unit cycle for the remanufacturing process |
hm | Holding cost $ per unit per month for the newly produced items |
h | Holding cost $ per unit per month for the remanufactured items |
hA | Holding cost $ per unit per month for the returned items |
h | Holding cost $ per unit per month for the reworked items |
Sm | Production cost$ per unit item |
S, | Remanufacturing cost$ per unit item |
Sm | Rework cost$ per unit item |
Cm | Procurement cost$ per unit item |
Cr | Remanufacturing cost $ per unit item |
Ci | Inspection cost $ per unit item |
Crw | Rework cost$ per unit item |
Cn | Acquisition cost $ per unit item |
cd | Disposable cost$ per unit item |
t1 | Production time |
t∗ | Time at which machine fails |
t8 | Machine repairing time |
This paper is intended to capture and describe a reverse logistics model in which the productivity of a manufacturer is directly related to the degree of its reliability. As is widely recognized in the manufacturing sector, the physical output realized by the firm is determined by a variety of factors that are associated with both performance and quality. In order to measure the effectiveness of a manufacturing system, it must be assessed in terms of its reliability and productivity. Various studies have found that the reliability of manufacturing processes can be improved through the adoption of smart production technologies, which is regarded as being highly durable. Whether a manufacturing system will fail or not will probably depend on its inherent reliability.
In this case, it is assumed that the machine breakdown takes place at the time t' before completing the actual production time t1, i.e., in this case we have taken that t′<t1. Apart from the breakdown, the production process is also not perfectly reliable. Hence, some imperfect items are also produced during this period and stored separately, out of which a small portion is scrapped. The machine is repaired by the time t2, and the machine starts to repair the defective items to the time t3. Thereafter, the level of inventory starts to decrease due to the demand and deterioration, at the same time remanufacturing starts. Imperfect items also are generated during the remanufacturing run and repaired in the period of t4 to t5. 1Returned items are collected from1 the starting of the cycle to the time when remanufacturing stops. Figure 1 depicts the behavior of the on-hand inventory level.
The differential1 equations governing1 the stock level1 during the period 1 0≤t≤T can be written as follows.
dIm(t)dt+θIm(t)=xP−D1 0≤t≤t′ Im(0)=0 | (1) |
dIm(t)dt+θIm(t)=−D1 t′≤t≤t2 Im(t′−)=Im(t′+) | (2) |
dIm(t)dt+θIm(t)=P1−D1 t2≤t≤t3 Im(t−3)=Im(t−3) | (3) |
dIm(t)dt+θIm(t)=−D1 t3≤t≤t4 Im(t4)=0 | (4) |
dIr(t)dt+θIr(t)=yPr−D2 t3≤t≤t4 Ir(t3)=0 | (5) |
dIr(t)dt+θIr(t)=P1−D2 t4≤t≤t5 Ir(t−4)=Ir(t+4) | (6) |
dIr(t)dt+θIr(t)=−D2 t5≤t≤T Ir(T)=0 | (7) |
dIR(t)dt+θIR(t)=R 0≤t≤t3 IR(0)=0 | (8) |
dIR(t)dt+θIR(t)=R−Pr t3≤t≤t4 IR(t4)=0 | (9) |
The solutions of the above differential equations are
Im(t)=(xP−D1θ)(1−e−θt) 0≤t≤t′ | (10) |
Im(t)=xPθ(e−θ(t−t′)−e−θt)−D1θ(1−e−θt) t′≤t≤t2 | (11) |
Im(t)=(P1θ)(1−eθ(t3−t))−D1θ(1−eθ(t4−t)) t2≤t≤t3 | (12) |
Im(t)=D1θ(eθ(t4−t)−1) t3≤t≤t4 | (13) |
Ir(t)=yPrθ(1−eθ(t3−t)) t3≤t≤t4 | (14) |
Ir(t)=P1−D2θ(1−eθ(t4−t))+yPrθ(eθ(t4−t)−eθ(t3−t)) t4≤t≤t5 | (15) |
Ir(t)=D2θ(eθ(T−t)−1) t5≤t≤T | (16) |
IR(t)=Rθ(1−e−θt) 0≤t≤t3 | (17) |
IR(t)=(R−Pr)θ(1−eθ(t4−t)) t3≤t≤t4 | (18) |
The per cycle cost components for the given inventory model are as follows.
i). Expected procurement and acquisition cost
The cost of purchasing the raw material (included deterioration cost) from the supplier can be calculated as
(CmPt′+CRRt4) | (19) |
Thus, the expected purchasing cost would be
∫t10β(CmPt′+CRRt4)e−βxdt′ | (20) |
ii). Expected production cost
Productivity of an automation-based production process fluctuates with replenishment strategies. In the event of a failure in an integral component of the machine, the component malfunctions adversely affect the system's productivity, product quality and production costs. For a manufacturing system, reliability is the measurement of output per unit of time in terms of the productivity of the whole system, whereas for a service system, productivity is the measure of output per unit of time. A controllable manufacturing system is one that has the ability to vary production rate to meet the demands of the market so that the productivity of manufacturing systems can be increased. 1Due to the fact that this model allows flexibility in the production rate of an item, the unit production cost becomes a function of three variables: the variable production rate m, the variable manufacturing yield Y and the variable manufacturing performance equality l.
The per unit production cost is
C(P,β,x)={π+γβP+(r1+r2β)+(s1+s2xy)+CpP} | (21) |
Therefore, the expected production would be
∫t10β{π+γβP+(r1+r2β)+(s1+s2xy)+CpP}Pt′e−βxdt′ | (22) |
iii). Expected remanufacturing cost
One of the complexities of remanufacturing is completely managing the two-supply arrangement of parts, i.e., the forward flow of material and the reverse flow. RL activities deal with the collection of used material; however, the quantity of buyback products is highly uncertain, which particularly influences the accumulation and replenishment policies. The remanufacturing starts at time t3, to the time t4. Therefore, the expected cost of remanufacturing is as follows:
∫t10βCrPr(t4−t3)e−βxdt′ | (23) |
iv). Expected inspection cost
During the production and remanufacturing process, items are inspected via automation policy. Therefore, they can be removed from the stock of serviceable items instantly. The expected cost of inspection is as follows:
∫t10βCi{Pt′+Pr(t4−t3)}e−βxdt′ | (24) |
v). Expected rework cost
x portion of produced items and y portion of remanufactured items are assumed to be defective per unit time of production. These items are reworked at a rate P1. The expected cost to rework those items can be calculated as follows:
∫t10βCrwP1(t3−t2+t5−t4)e−βxdt′ | (25) |
vi). Expected holding cost for newly produced material
The items are stored in the warehouse, and the manufacturer needs to spend some investment. The holding cost of carrying the newly produced inventory during the time period zero to t4 can be calculated as follows:
hm[∫t′0(xP−D1θ)(1−e−θt)dt+∫t2t′{xPθ(e−θ(t−t′)−e−θt)−D1θ(1−e−θt)}dt+∫t3t2{(P1θ)(1−eθ(t3−t))−D1θ(1−eθ(t4−t))}dt+∫t4t3D1θ(eθ(t4−t)−1)dt] |
=hm[(xP−D1θ)((e−θt′−1)θ+t′)+xPθ2(1−e−θt′−e−θ(t2−t′)+e−θt2)−D1θ(e−θt2−e−θt′θ+(t2−t′))+D1θ(eθ(t4−t2)−eθ(t4−t3)θ+(t3−t2))+P1θ((t3−t2)+(1−eθ(t3−t2))θ)−D1θ((t4−t3)+(1−eθ(t4−t3))θ)] | (26) |
The expected holding cost of carrying the newly produced inventory during the time period zero to t4 can be calculated as follows:
∫t10βhm[(xP−D1θ)((e−θt′−1)θ+t′)+xPθ2(1−e−θt′−e−θ(t2−t′)+e−θt2)−D1θ(e−θt2−e−θt′θ+(t2−t′))+D1θ(eθ(t4−t2)−eθ(t4−t3)θ+(t3−t2))+P1θ((t3−t2)+(1−eθ(t3−t2))θ)−D1θ((t4−t3)+(1−eθ(t4−t3))θ)]e−βxdt′ | (27) |
vii). Expected holding cost for remanufactured material
The cost of carrying the remanufactured inventory during the time period t3 to T is
hr[∫t4t3yPrθ(1−eθ(t3−t))dt+∫t5t4{P1−D2θ(1−eθ(t4−t))+yPrθ(eθ(t4−t)−eθ(t3−t))}dt+∫Tt5D2θ(eθ(T−t)−1)dt] |
=hr[yPrθ(eθ(t3−t4)−1θ+(t4−t3))+(P1−D2)θ(e−θ(t5−t4)−1θ+(t5−t4))+yPrθ(1−e−θ(t5−t4)+e−θ(t5−t3)−e−θ(t4−t3)θ)−D2θ(1−eθ(T−t5)θ+(T−t5))] | (28) |
The expected cost of carrying the remanufactured inventory during the time period t3 to T is
∫t10βhr[yPrθ(eθ(t3−t4)−1θ+(t4−t3))+(P1−D2)θ(e−θ(t5−t4)−1θ+(t5−t4))+yPrθ(1−e−θ(t5−t4)+e−θ(t5−t3)−e−θ(t4−t3)θ)−D2θ(1−eθ(T−t5)θ+(T−t5))]e−βxdt′ | (29) |
viii). Expected holding cost for returned items
The holding cost for carrying the collected returned items during the time period zero to t4 is
hR[∫t30Rθ(1−e−θt)dt+∫t4t3(R−Pr)θ(1−eθ(t4−t))dt] |
=hR{Rθ(t3+(e−θt3−1)θ)+(R−Pr)θ((t4−t3)+(1−eθ(t4−t3))θ)} | (30) |
The expected holding cost for carrying the collected returned items during the time period zero to t4 is
∫t10βhR{Rθ(t3+(e−θt3−1)θ)+(R−Pr)θ((t4−t3)+(1−eθ(t4−t3))θ)}e−βxdt′ | (31) |
ix). Expected holding cost for imperfect items
Due to the automation policy of inspection, imperfect items are instantly removed from the serviceable items and carried at a separated place. The holding cost for carrying the imperfect items during the time period zero to t5 is
hrw{(1−x)P(t′t2−t′22+t′λ1(t3−t2)2)+(1−y)Pr((t4−t3)22+λ2(t4−t3)(t5−t4))} | (32) |
The expected holding cost for carrying the imperfect items during the time period zero to t5 is.
∫t10βhrw{(1−x)P(t′t2−t′22+t′λ1(t3−t2)2)+(1−y)Pr((t4−t3)22+λ2(t4−t3)(t5−t4))}e−βxdt′ | (33) |
x). Expected setup cost
The system has to invest at the starting of the cycle to setup the manufacturing process, and the cost increases as the number of failures increases. The failure rate dependent setup cost can be calculated as follows:
(Km(1+Rsβ)+Kr) | (34) |
The expected setup cost can be calculated as follows:
∫t10β(Km(1+Rsβ)+Kr)e−βxdt′ | (35) |
xi). Expected disposal cost
As the production and remanufacturing process are not perfectly reliable, imperfect items are found, but all the items are not supposed to be in the condition to be reworked. Those items are disposed of. The cost of disposing these items is
cd{(1−x)(1−λ1)Pt′+(1−y)(1−λ2)Pr(t4−t3)} | (36) |
Thus, the expected cost of disposing these items is
∫t10βcd{(1−x)(1−λ1)Pt′+(1−y)(1−λ2)Pr(t4−t3)}e−βxdt′ | (37) |
Hence, the 1total cost per unit time1 of the given inventory model 1during the cycle [0,T] as a function of t1,t2,t3,t4,t5,T and TC1(t1,t2,t3,t4,t5,T) is given by
TC1=∫t10β(CmPt′+Rt4)e−βxdt′+∫t10β{π+γβP+(r1+r2β)+(s1+s2xy)+CpP}Pt′e−βxdt′+∫t10βCrPr(t4−t3)e−βxdt′+∫t10βCrwP1(t3−t2+t5−t4)e−βxdt′+∫t10βCi{Pt′+Pr(t4−t3)}e−βxdt′+∫t10βhm[(xP−D1θ)((e−θt′−1)θ+t′)+xPθ2(1−e−θt′−e−θ(t2−t′)+e−θt2)−D1θ(e−θt2−e−θt′θ+(t2−t′))+D1θ(eθ(t4−t2)−eθ(t4−t3)θ+(t3−t2))+P1θ((t3−t2)+(1−eθ(t3−t2))θ)−D1θ((t4−t3)+(1−eθ(t4−t3))θ)]e−βxdt′+∫t10βhr[yPrθ(eθ(t3−t4)−1θ+(t4−t3))+(P1−D2)θ(e−θ(t5−t4)−1θ+(t5−t4))+yPrθ(1−e−θ(t5−t4)+e−θ(t5−t3)−e−θ(t4−t3))θ)]−D2θ(1−eθ(T−t5)θ+(T−t5))]e−βxdt′+∫t10βhR{Rθ(t3+(e−θt3−1)θ)+(R−Pr)θ((t4−t3)+(1−eθ(t4−t3))θ)}e−βxdt′+∫t10βhrw{(1−x)P(t′t2−t′22+t′λ1(t3−t2)2)+(1−y)Pr((t4−t3)22+λ2(t4−t3)(t5−t4))}e−βxdt′+∫t10β(Km(1+Rsβ)+Kr)e−βxdt′+∫t10βcd{(1−x)(1−λ1)Pt′+(1−y)(1−λ2)Pr(t4−t3)}e−βxdt′ | (38) |
Based on the arrangement of inventory depicted in Figure 1, certain relations between the variables can derived, such as
ts=(t2−t′) | (39) |
xPθ(e−θ(t2−t′)−e−θt2)−D1θ(1−e−θt2)=(P1θ)(1−eθ(t3−t2))−D1θ(1−eθ(t4−t2)) | (40) |
P1−D2θ(1−e−θ(t5−t4))+yPrθ(e−θ(t5−t4)−e−θ(t5−t3))=−D2θ(1−eθ(T−t5)) | (41) |
Rθ(1−e−θt3)=(R−Pr)θ(1−eθ(t4−t3)) | (42) |
(1−x)λ1Pt′=P1(t3−t2) | (43) |
(1−y)λ2Pr(t4−t3)=P1(t5−t4) | (44) |
Therefore, from the above relations, the values of t2,t3,t4,t5 and T can be determined as functions of t′, say,
t2=f12(t′)=(ts+t′) | (45) |
t3=f13(t′)=(1−x)λ1Pt′+P1(ts+t′)P1 | (46) |
t4=f14(t′)=PPrt′(−1+x)λ1−P1Pr(ts+t′)(Pr−R){P1((ts+t′)θ−1)−Pt′(x−1)θλ1} | (47) |
t5=f15(t′)=−{Pr(P1(ts+t′)−Pt′(x−1)λ1){P12+(1−y){−P1R−P1(Pr−R)(ts+t′)θ+P(Pr−R)t′(x−1)θλ1}λ2}}{P12(Pr−R){P1((ts+t′)θ−1)−Pt′(x−1)θλ1}} | (48) |
T=f16(t′)=(Pr(−P(x−1)λ1t′+P1(ts+t′))(−P3(x−1)3(−1+y)yθ3Pr(−R+Pr)2λ31λ2(t′)3+θP51Pr(ts+t′)+P2(x−1)2(y−1)yθ2P1Pr(−R+Pr)λ21λ2(t′)2(2R+3θ(−R+Pr)(t′s+t′))−P(x−1)θP21λ1t′(P(x−1)θ(−R+Pr)λ1(−Ry+2yPr+R(−1+y)λ2)t′+(y−1)yPrλ2(R+θ(−R+Pr)(ts+t′))(R+3θ(−R+Pr)(ts+t′)))+D2P1(P2(x−1)2(−1+y)θ2Pr(−R+Pr)λ21λ2(t′)2−P(x−1)(−1+y)θP1Prλ1λ2t′(R+2θ(−R+Pr)(ts+t′))+P31(Pr−2θPr(ts+t′)+R(−1+θ(ts+t′)))+θP21(P(x−1)(−R+2Pr)λ1t′+(−1+y)Prλ2(ts+t′)(R+θ(−R+Pr)(ts+t′))))+θP31((−1+y)yPrλ2(ts+t′)(R+θ(−R+Pr)(ts+t′))2+P(−1+x)λ1t′((−1+y)λ2(2R2−2RPr+P2r+2Rθ(−R+Pr)(ts+t′))+y(−2R2+4RPr−P2r+2θ(R2−3RPr+2P2r)(ts+t′))))+P41(R2(y(−1+λ2)−λ2)(−1+θ(ts+t′))2−θP2r(ts+t′)(−λ2+y(−1+λ2+2θ(ts+t′)))+Pr(−P(−1+x)θλ1t′−R(−1+θ(ts+t′))(y−3yθ(ts+t′)+(−1+y)λ2(−1+θ(ts+t′)))))))((D2P1(−R+Pr)(−P(−1+x)θλ1t′+P1(−1+θ(ts+t′))(−(−1+y)θPrλ2(−P(−1+x)λ1t′+P1(ts+t′))(P(−1+x)θ(R−Pr)λ1t′+P1(R+θ(−R+Pr)(ts+t′)))+P21(−P(−1+x)θ(−R+2Pr)λ1t′+P1(R−Pr+θ(−R+2Pr)(ts+t′)))) | (49) |
Based on the above results, the expected cost function can be written as
TC1=∫t10β(CmPt′+CRRf14)e−βxdt′+∫t10β{π+γβP+(r1+r2β)+(s1+s2xy)+CpP}Pt′e−βxdt′+∫t10βCrPr(f14−f13)e−βxdt′+∫t10βCrwP1(f13−f12+f15−f14)e−βxdt′+∫t10βCi{Pt′+Pr(f14−f13)}e−βxdt′+∫t10βhm[(xP−D1θ)((e−θt′−1)θ+t′)+xPθ2(1−e−θt′−e−θ(f12−t′)+e−θf12)−D1θ(e−θf12−e−θt′θ+(f12−t′))+D1θ(eθ(f14−f12)−eθ(f14−f13)θ+(f13−f12))+P1θ((f13−f12)+(1−eθ(f13−f12))θ)−D1θ((f14−f13)+(1−eθ(f14−f13))θ)]e−βxdt′+∫t10βhr[yPrθ(eθ(f13−f14)−1θ+(f14−f13))+(P1−D2)θ(e−θ(f15−f14)−1θ+(f15−f14))+yPrθ(1−e−θ(f15−f14)+e−θ(f15−f13)−e−θ(f14−f13)θ)−D2θ(1−eθ(f16−f15)θ+(f16−f15))]e−βxdt′+∫t10βhR{Rθ(f13+(e−θf13−1)θ)+(R−Pr)θ((f14−f13)+(1−eθ(f14−f13))θ)}e−βxdt′+∫t10βhrw{(1−x)P(t′f12−t′22+t′λ1(f13−f12)2)+(1−y)Pr((f14−f13)22+λ2(f14−f13)(f15−f14))}e−βxdt′+∫t10β(Km(1+RSβ)+Kr)e−βxdt′+∫t10βcd{(1−x)(1−λ1)Pt′+(1−y)(1−λ2)Pr(f14−f13)}e−βxdt′ | (50) |
In this case, it is considered that the machine breakdown does not occur during the production run. The behavior of the on-hand inventory is depicted in Figure 2.
The differential equations governing the stock level during the period 0≤t≤T can be written as
dIm(t)dt+θIm(t)=xP−D1 0≤t≤t1 Im(0)=0 | (51) |
dIm(t)dt+θIm(t)=P1−D1 t1≤t≤t2 Im(t−1)=Im(t−1) | (52) |
dIm(t)dt+θIm(t)=−D1 t2≤t≤t3 Im(t3)=0 | (53) |
dIr(t)dt+θIr(t)=yPr−D2 t2≤t≤t3 Ir(t2)=0 | (54) |
dIr(t)dt+θIr(t)=P1−D2t3≤t≤t4Ir(t−3)=Ir(t+3) | (55) |
dIr(t)dt+θIr(t)=−D2 t4≤t≤t5 Ir(T)=0 | (56) |
dIR(t)dt+θIR(t)=R0≤t≤t2 IR(0)=0 | (57) |
dIR(t)dt+θIR(t)=R−Pr t2≤t≤t3 IR(t3)=0 | (58) |
The solutions of the above differential equations are
Im(t)=(xP−D1θ)(1−e−θt) 0≤t≤t1 | (59) |
Im(t)=(P1−D1θ)(1−eθ(t1−t))+(xP−D1θ)(eθ(t1−t)−e−θt) t1≤t≤t2 | (60) |
Im(t)=D1θ(eθ(t3−t)−1) t2≤t≤t3 | (61) |
Ir(t)=yPrθ(1−eθ(t2−t)) t2≤t≤t3 | (62) |
Ir(t)=P1−D2θ(1−eθ(t3−t))+yPrθ(eθ(t3−t)−eθ(t2−t)) t3≤t≤t4 | (63) |
Ir(t)=D2θ(eθ(T−t)−1) t4≤t≤t5 | (64) |
IR(t)=Rθ(1−e−θt) 0≤t≤t2 | (65) |
IR(t)=(R−Pr)θ(1−eθ(t3−t)) t2≤t≤t3 | (66) |
The per cycle cost components for the given inventory model are as follows.
i). Expected procurement and acquisition cost
The cost of purchasing the raw material (including deterioration cost) from the supplier can be calculated as
(CmPt1+CRRt3) | (67) |
Thus, the expected purchasing cost would be
∫∞t1β(CmPt1+Rt3)e−βxdt′ | (68) |
ii). Expected production cost
The per unit expected production cost is
∫∞t1β{π+γβP+(r1+r2β)+(s1+s2xy)+CpP}Pt1e−βxdt′ | (69) |
iii). Expected repairing cost
The per unit expected repairing cost is
∫∞t1βCrPr(t3−t2)e−βxdt′ | (70) |
iv). Expected rework cost
The per unit expected rework cost is
∫∞t1βCrwP1(t2−t1+t4−t3)e−βxdt′ | (71) |
v). Expected inspection cost
The per unit expected inspection cost is
∫∞t1βCi{Pt1+Pr(t3−t2)}e−βxdt′ | (72) |
vi). Expected holding cost for newly produced material
The items are stored in the warehouse, and the manufacturer needs to spend some investment. The expected holding cost of carrying the newly produced inventory during the time period zero to t3 can be calculated as follows:
hm[∫t10(xP−D1θ)(1−e−θt)dt+∫t2t1{(P1−D1θ)(1−eθ(t1−t))+(xP−D1θ)(eθ(t1−t)−e−θt)}dt+∫t3t2D1θ(eθ(t3−t)−1)dt] |
=hm[(xP−D1θ)((e−θt1−1)θ+t1)+(xP−D1θ2)(1−e−θt1−e−θ(t2−t1)+e−θt2)+(P1−D1)θ(e−θ(t2−t1)−1θ+(t2−t1))−D1θ(1−eθ(t3−t2)θ+(t3−t2))] | (73) |
The expected holding cost of carrying the newly produced inventory during the time period zero to t3 can be calculated as follows:
∫∞t1βhm[(xP−D1θ)((e−θt1−1)θ+t1)+(xP−D1θ2)(1−e−θt1−e−θ(t2−t1)+e−θt2)+(P1−D1)θ(e−θ(t2−t1)−1θ+(t2−t1))−D1θ(1−eθ(t3−t2)θ+(t3−t2))]e−βxdt′ | (74) |
vii). Expected holding cost for remanufactured material
hr[∫t3t2yPrθ(1−eθ(t2−t))dt+∫t4t3{P1−D2θ(1−eθ(t3−t))+yPrθ(eθ(t3−t)−eθ(t2−t))}dt+∫Tt4D2θ(eθ(T−t)−1)dt] |
=hr[yPrθ(e−θ(t3−t2)−1θ+(t3−t2))+(P1−D2)θ(e−θ(t4−t3)−1θ+(t4−t3))+yPrθ(1−e−θ(t4−t3)+e−θ(t4−t2)−e−θ(t3−t2)θ)−D2θ(1−eθ(T−t4)θ+(T−t4))]=∫∞t1βhr[yPrθ(e−θ(t3−t2)−1θ+(t3−t2))+(P1−D2)θ(e−θ(t4−t3)−1θ+(t4−t3))+yPrθ(1−e−θ(t4−t3)+e−θ(t4−t2)−e−θ(t3−t2)θ)−D2θ(1−eθ(T−t4)θ+(T−t4))]e−βxdt′ | (75) |
viii). Expected holding cost for returned items
hR[∫t20Rθ(1−e−θt)dt+∫t3t2(R−Pr)θ(1−eθ(t3−t))dt] |
=hR{Rθ(t2+(e−θt2−1)θ)+(R−Pr)θ((t3−t2)+(1−eθ(t3−t2))θ)} |
=∫∞t1βhR{Rθ(t2+(e−θt2−1)θ)+(R−Pr)θ((t3−t2)+(1−eθ(t3−t2))θ)}e−βxdt′ | (76) |
ix). Expected holding cost for reworked items
hrw{(1−x)P(t212+t1λ1(t2−t1)2)+(1−y)Pr((t3−t2)22+λ2(t3−t2)(t4−t3))} | (77) |
=∫∞t1βhrw{(1−x)P(t212+t1λ1(t2−t1)2)+(1−y)Pr((t3−t2)22+λ2(t3−t2)(t4−t3))}e−βxdt′ |
x). Expected setup cost
Km(1+Rsβ)+Kr=∫∞t1β(Km(1+Rsβ)+Kr)e−βxdt′ | (78) |
xi). Expected disposal cost
cd{(1−x)(1−λ1)Pt1+(1−y)(1−λ2)Pr(t3−t2)} |
=∫∞t1βcd{(1−x)(1−λ1)Pt1+(1−y)(1−λ2)Pr(t3−t2)}e−βxdt′ | (79) |
Hence, the total cost per unit time of the given inventory model during the cycle [0,T] as a function of t1,t2,t3,t4 and T, i.e., TC2(t1,t2,t3,t4,T), is Total cost, given by
Total cost = Procurement and acquisition cost+ Production and remanufacturing cost+ Holding cost for remanufactured material + Holding cost for produced material + Holding cost for returned items+ Holding cost for reworked items+ Disposal cost +Setup cost
=∫∞t1β(CmPt1+CRRt3)e−βxdt′+∫∞t1β{π+γβP+(r1+r2β)+(s1+s2xy)+CpP}Pt1e−βxdt′+∫∞t1βCrPr(t3−t2)e−βxdt′+∫∞t1βCrwP1(t2−t1+t4−t3)e−βxdt′+∫∞t1βCi{Pt1+Pr(t3−t2)}e−βxdt′+∫∞t1βhm[(xP−D1θ)((e−θt1−1)θ+t1)+(xP−D1θ2)(1−e−θt1−e−θ(t2−t1)+e−θt2)+(P1−D1)θ(e−θ(t2−t1)−1θ+(t2−t1))−D1θ(1−eθ(t3−t2)θ+(t3−t2))]e−βxdt′+∫∞t1βhr[yPrθ(e−θ(t3−t2)−1θ+(t3−t2))+(P1−D2)θ(e−θ(t4−t3)−1θ+(t4−t3))+yPrθ(1−e−θ(t4−t3)+e−θ(t4−t2)−e−θ(t3−t2)θ)−D2θ(1−eθ(T−t4)θ+(T−t4))]e−βxdt′+∫∞t1βhR{Rθ(t2+(e−θt2−1)θ)+(R−Pr)θ((t3−t2)+(1−eθ(t3−t2))θ)}e−βxdt′+∫∞t1βhrw{(1−x)P(t212+t1λ1(t2−t1)2)+(1−y)Pr((t3−t2)22+λ2(t3−t2)(t4−t3))}e−βxdt′+∫∞t1β(Km(1+Rsβ)+Kr)e−βxdt′+∫∞t1βcd{(1−x)(1−λ1)Pt1+(1−y)(1−λ2)Pr(t3−t2)}e−βxdt′ | (80) |
The above can be rewritten as
TC2=[(CmPt1+CRRt3)+{π+γβP+(r1+r2β)+(s1+s2xy)+CpP}Pt1+CrPr(t3−t2)+CrwP1(t2−t1+t4−t3)+Ci{Pt1+Pr(t3−t2)}+hm[(xP−D1θ)((e−θt1−1)θ+t1)+(xP−D1θ2)(1−e−θt1−e−θ(t2−t1)+e−θt2)+(P1−D1)θ(e−θ(t2−t1)−1θ+(t2−t1))−D1θ(1−eθ(t3−t2)θ+(t3−t2))]+hr[yPrθ(e−θ(t3−t2)−1θ+(t3−t2))+(P1−D2)θ(e−θ(t4−t3)−1θ+(t4−t3))+yPrθ(1−e−θ(t4−t3)+e−θ(t4−t2)−e−θ(t3−t2)θ)−D2θ(1−eθ(T−t4)θ+(T−t4))]+hR{Rθ(t2+(e−θt2−1)θ)+(R−Pr)θ((t3−t2)+(1−eθ(t3−t2))θ)}+hrw{(1−x)P(t212+t1λ1(t2−t1)2)+(1−y)Pr((t3−t2)22+λ2(t3−t2)(t4−t3))}+Km(1+Rsβ)+Kr+cd{(1−x)(1−λ1)Pt1+(1−y)(1−λ2)Pr(t3−t∞t1βe−βxdt | (81) |
Here, the cost function of the system is given in terms of t1,t2,t3,t4 and T. To find the optimum solution, we have to find the optimum values of t1,t2,t3,t4 and T that minimize TC2(t1,t2,…,T), but we have some relations between the variables as follows.
(P1−D1θ)(1−e−θ(t2−t1))+(xP−D1θ)(e−θ(t2−t1)−e−θt2)=D1θ(eθ(t3−t2)−1) | (82) |
P1−D2θ(1−e−θ(t4−t3))+yPrθ(e−θ(t4−t3)−e−θ(t4−t2))=D2θ(eθ(T−t4)−1) | (83) |
Rθ(1−e−θt2)=(R−Pr)θ(1−eθ(t3−t2)) | (84) |
(1−x)λ1Pt1=P1(t2−t1) | (85) |
(1−y)λ2Pr(t3−t2)=P1(t4−t3) | (86) |
Therefore, from the above equations, the values of t2,t3,t4 and T can be determined as functions of t1, say,
t2=f21(t1)=((1−x)λ1PP1+1)t1 | (87) |
t3=f22(t1)=(PrPr−R)((1−x)λ1PP1+1)t1 | (88) |
t4=f23(t1)=(PrPr−R){(1−y)λ2R+P1}((1−x)λ1PP1+1)t1 | (89) |
t4=f24(t1)=(1−x)λ1Pt′+P1(ts−t′)P1 | (90) |
T=f25(t1)=−t1Pr(P1−P(−1+x)λ1)(D2+P21+y(R+Pr)−P1(R(−1+y)λ2))D2P1(R−Pr) | (91) |
Therefore, the total variable cost function will be a function of a single variable, say, t1.The problem will be converted into the following problem with one variable Z2(t1) :
TC2=[(CmPt1+CRRf23)+{π+γβP+(r1+r2β)(s1+s2β)+CpP}Pt1+CrPr(f23−f22)+CrwP1(f22−t1+f24−f23)+Ci{Pt1+Pr(f23−f22)}+hm[(xP−D1θ)((e−θt1−1)θ+t1)+(xP−D1θ2)(1−e−θt1−e−θ(f22−t1)+e−θf22)+(P1−D1)θ(e−θ(f22−t1)−1θ+(f22−t1))−D1θ(1−eθ(f23−f22)θ+(f23−f22))]+hr[yPrθ(e−θ(f23−f22)−1θ+(f23−f22))+(P1−D2)θ(e−θ(f24−f23)−1θ+(f24−f23))+yPrθ(1−e−θ(f24−f23)+e−θ(f24−f22)−e−θ(f23−f22)θ)−D2θ(1−eθ(f25−f24)θ+(f25−f24))]+hR{Rθ(f22+(e−θf22−1)θ)+(R−Pr)θ((f23−f22)+(1−eθ(f23−f22))θ)}+hrw{(1−x)P(t212+t1λ1(f22−t1)2)+(1−y)Pr((f23−f22)22+λ2(f23−f22)(f24−f23))}+Km(1+RSβ)+Kr+cd{(1−x)(1−λ1)Pt1+(1−y)(1−λ2)Pr(f23−f22)}]∫∞t1βe−βxdt | (92) |
In this model, we have developed a green supply chain model with stochastic machine breakdown, that is, the failure can take place anytime during the production process. In the last section, we have derived the cost functions for both cases: The inventory cost function for case 1 when breakdown occurs is E[Z1(t′)], and the cost function for case 2 when the breakdown does not occur is E[Z2(t1)]. Here, we have 1assumed that the random1 number of 1breakdowns per unit 1time follows the 1Poisson distribution, with1 the mean 1equal to β per unit1 time. Thus, the 1random production 1time to breakdown1 should obey1 the exponential1 distribution1, with the1 density1 function f(t)=βe−βt and the cumulative1 density1 function F(t)=(1−e−βt). Then, 1 the expected 1production-inventory1 cost per 1unit time (whether a 1breakdown 1takes place or not), E[Z(t1)] is
E[Z(t1)]=∫t10E[Z1(t′)]f(t)dt+∫∞t1E[Z2(t1)]f(t)dtE[T] | (93) |
where
E[T]=∫t10E(T′)f(t)dt+∫∞t1E(T)f(t)dt | (94) |
Alternatively, it can be written as
E[T]=∫t10f15(t′)f(t)dt+∫∞t1f24(t1)f(t)dt | (95) |
Now, substituting E[Z1(t′)],E[Z2(t1)] and E[T] from Eqs. (50), (92) and (95), in Eq. (93), one can obtain the expected inventory cost per unit time.
Now, the necessary conditions for having a minimum for the problem is dE[Z(t1)]dt1=0 | (96) |
Using Equation 96, one can find the optimal value of t1 and hence evaluate the minimum inventory cost E[Z(t1)].
To illustrate the proposed model, we have considered the following input parameters in appropriate units:
Pr=400 items per month, P=500 items per month, D1=200 items per month, D2=100 items per month, P1=200 items per month, R=50 items per month, x=0.2,y=0.15,λ1=0.25,λ2=0.2, θ=0.01,Km=5000 $ percycle, Kr=2000 $ percycle, hm=1 $ peritems per month, hr=0.5 $ peritems per month, hR=0.5 $ peritems per month, h1=0.5 $ peritems per month, Sm=5 items per month, Sr=1 items per month, Srw=0.2 items per month, Cm=2 items per month, CR=0.5 items per month, Cs=5 items per month, Cd=0.1 items per month, β=0.5,ts=0.5 items per month, π=1500 $ perproduction lot, r1=12.5$ per cycle, r2=18.5 $ peritems, s1=15 $ percycle, s2=10 $ peritem, Cp=20 $ peritem, Cr=45 $ peritem, Crw=10 $ peritem, Ci=5 $ peritem, Rs=150 $ peritem, γ=45
Applying the procedure proposed in the last section (with the help of the software Mathematica) we find the optimal value of the production time period t1=7.36573 and hence the corresponding minimum total expected cost E[Z(t1)]=817840 The convexity of the inventory model is shown in Figure 3. The two-dimensional graph shows that the integrated expected total annual cost is convex.
As one can see from the example above, the numerical technique can be used to analyze the effect of parameters in order to determine the optimal values of the parameters as well as the minimum cost to be incurred by the system. The results of the investigation are obtained by changing the values of one parameter in turn while leaving the other parameters at their original values.
1. The analysis, as shown in Table 1 and Figures 4 to 9, highlights that the total cost exhibits a decreasing trend as the demand rate, return rate, β and repairing rate increase. This trend is reasonable, as it corresponds to a reduction in holding costs due to higher values of these parameters.
2. Conversely, the results demonstrate that the total cost increases with an increment in the production rate and remanufacturing rate. This is attributed to the fact that higher production and remanufacturing rates lead to elevated on-hand inventory levels per unit time, subsequently increasing holding costs and, consequently, the total cost.
3. The study reveals that the total cost is highly sensitive to variations in the production rate, remanufacturing rate, demand rate and β. Small changes in these parameters can significantly impact the total cost.
4. In contrast, the total cost displays only moderate sensitivity to changes in the return rate.
Parameter | t1 | TC | |
Pr | 300 | 7.37257 | 399069 |
350 | 7.36863 | 583824 | |
400 | 7.36573 | 817840 | |
450 | 7.36350 | 1106890 | |
500 | 7.36173 | 1456730 | |
P | 400 | 7.39605 | 507074 |
450 | 7.37931 | 653559 | |
500 | 7.36573 | 817840 | |
550 | 7.35451 | 1001650 | |
600 | 7.34985 | 1258420 | |
D | 80 | 7.32658 | 1102455 |
90 | 7.34665 | 936542 | |
100 | 7.36573 | 817840 | |
110 | 7.38787 | 651225 | |
120 | 7.40995 | 523109 | |
Pr | 160 | 7.37722 | 821022 |
180 | 7.37085 | 819733 | |
200 | 7.36573 | 817840 | |
220 | 7.36153 | 815702 | |
240 | 7.35801 | 813503 | |
R | 40 | 7.37233 | 874138 |
45 | 7.36889 | 845055 | |
50 | 7.36573 | 817840 | |
55 | 7.36284 | 792320 | |
60 | 7.36019 | 768341 | |
β | 0.40 | 9.20792 | 1595220 |
0.45 | 8.18451 | 1121040 | |
0.50 | 7.36573 | 817840 | |
0.55 | 6.69576 | 615000 | |
0.60 | 5.36548 | 483235 |
Decision-makers should consider optimizing the demand rate, return rate and repairing rate to reduce total costs. Increasing these parameters can lead to more efficient inventory management and lower holding costs. On the other hand, managers need to carefully assess and balance production and remanufacturing rates. Incremental increases in these rates may boost production but can also lead to higher inventory holding costs. Therefore, a well-calibrated production and remanufacturing strategy is essential. When making decisions related to production, remanufacturing and its demand, the managers should be aware of the high sensitivity of total costs to changes in these parameters. This underscores the importance of precise parameter estimation and management to minimize overall costs. While the return rate has a relatively moderate impact on total costs compared to other factors, it should not be neglected. Careful management of the return rate can still contribute to cost savings and improved efficiency in inventory control.
In summary, the observations and managerial insights from this analysis provide valuable guidance for optimizing inventory management strategies in the context of varying demand, returns, production and remanufacturing rates. Effective decision-making in these areas can significantly impact the overall cost efficiency of the system.
In this paper, we have introduced a reverse logistics inventory model that addresses the management of imperfect materials in the presence of stochastic machine breakdowns. Furthermore, we have considered the deterioration of products over time, adding another layer of complexity to the inventory control problem. Our analysis focused on two distinct scenarios: one where breakdowns occur and another where they do not. For each scenario, we derived mathematical formulas representing the total cost.
However, it is important to acknowledge certain limitations in our current study. We have assumed a Poisson distribution for the random number of breakdowns per unit time, simplifying the stochastic aspect of the breakdowns. Future research could explore more complex scenarios involving multiple breakdowns during both production and the remanufacturing cycle, providing a more realistic representation of the operational challenges faced in manufacturing and supply chain contexts.
Additionally, this model does not account for volume flexibility or probabilistic demand, which is prevalent in dynamic production environments. Investigating how our model can be extended to accommodate these factors would be a valuable avenue for future research. The incorporation of volume flexibility could enhance the model's adaptability to varying production needs, while addressing probabilistic demand would further improve its applicability to real-world situations.
In conclusion, while this paper offers a foundational framework for reverse logistics inventory management in the presence of machine breakdowns and product deterioration, it is by no means exhaustive. Future research endeavors should explore the nuances of multiple breakdown occurrences, volume flexibility and probabilistic demand, providing a more comprehensive and practical understanding of inventory control in dynamic and uncertain manufacturing environments. By addressing these limitations and extending the model, researchers and practitioners can make more informed decisions to enhance the efficiency and sustainability of their supply chain operations.
The authors declare they have not used artificial intelligence (AI) tools in the creation of this article.
The authors declare no conflict of interest.
All data used to justify the proposed model are given in the manuscript.
[1] |
Agrawal S, Sinhg RK, Murtaza Q (2015) A literature review and perspectives in reverse logistics. Res Con Recy 97: 76–92. https://doi.org/10.1016/j.resconrec.2015.02.009 doi: 10.1016/j.resconrec.2015.02.009
![]() |
[2] | Al-khateeb FB (1999) Quantitative modeling and information system support for just-in-time partnership. Louisiana State University and Agricultural & Mechanical College. https://doi.org/10.31390/qradschool_disstheses.6969 |
[3] |
Alinovi A, Bottani E, Montanari R (2012) Reverse logistics: a stochastic eoq-based inventory control model for mixed manufacturing/remanufacturing systems with return policies. Int J Prod Res 50: 1243–1264. https://doi.org/10.1080/00207543.2011.571921 doi: 10.1080/00207543.2011.571921
![]() |
[4] |
Bazan E, Jaber MY, Zanoni S (2016) A review of mathematical inventory models for reverse logistics and the future of its modeling: An environmental perspective. Appl Math Modell 40: 4151–4178. https://doi.org/10.1016/j.apm.2015.11.027 doi: 10.1016/j.apm.2015.11.027
![]() |
[5] |
Chen C, Zhang G, Shi J, et al. (2019) Remanufacturing network design for dual-channel closed-loop supply chain. Proce CIRP 83: 479–484. https://doi.org/10.1016/j.procir.2019.04.132 doi: 10.1016/j.procir.2019.04.132
![]() |
[6] |
Chung KJ (2003) Approximations to production lot sizing with machine breakdowns. Comp Oper Res 30: 1499–1507. https://doi.org/10.1016/S0305-0548(02)00079-5 doi: 10.1016/S0305-0548(02)00079-5
![]() |
[7] |
Darma Wangsa I, Wee HM (2018) An integrated vendor–buyer inventory model with transportation cost and stochastic demand. Int J Sys Sci Oper Logis 5: 295–309. https://doi.org/10.1080/23302674.2017.1296601 doi: 10.1080/23302674.2017.1296601
![]() |
[8] |
De Giovanni P (2011) Quality improvement vs. advertising support: Which strategy works better for a manufacturer? Eur J Oper Res 208: 119–130. https://doi.org/10.1016/j.ejor.2010.08.003 doi: 10.1016/j.ejor.2010.08.003
![]() |
[9] | Deiranlou M, Dehghanian F, Pirayesh M (2022) Joint determination of purchasing and production lot sizes in an unreliable production system. Scien Iran, Available from: https://scientiairanica.sharif.edu/article_22677_6974c69a20e846205a364e45cd93996e.pdf. |
[10] | Dekker R, Fleischmann M, Inderfurth K, et al. (2004) Reverse logistics: quantitative models for closed-loop supply chains. Springer Science & Business Media. |
[11] |
Dey BK, Bhuniya S, Sarkar B (2021a) Involvement of controllable lead time and variable demand for a smart manufacturing system under a supply chain management. Exp Sys Appl 184: 115464. https://doi.org/10.1016/j.eswa.2021.115464 doi: 10.1016/j.eswa.2021.115464
![]() |
[12] |
Dey BK, Sarkar B, Seok H (2021b) Cost-effective smart autonomation policy for a hybrid manufacturing-remanufacturing. Comp Ind Eng 162: 107758. https://doi.org/10.1016/j.cie.2021.107758 doi: 10.1016/j.cie.2021.107758
![]() |
[13] |
Giri BC, Dohi T (2005) Exact formulation of stochastic EMQ model for an unreliable production system. J Oper Res Soc 56: 563–575. https://doi.org/10.1057/palgrave.jors.2601840 doi: 10.1057/palgrave.jors.2601840
![]() |
[14] |
Groenevelt H, Pintelon L, Seidmann A (1992) Production lot sizing with machine breakdowns. Manag Sci 38: 104–123. https://doi.org/10.1287/mnsc.38.1.104 doi: 10.1287/mnsc.38.1.104
![]() |
[15] |
Haupt M, Hellweg S (2019) Measuring the environmental sustainability of a circular economy. Env Sustain Indic 1: 100005. https://doi.org/10.1016/j.indic.2019.100005 doi: 10.1016/j.indic.2019.100005
![]() |
[16] | Islam M, Azeem A, Jabir M, et al. (2022) An inventory model for a three-stage supply chain with random capacities considering disruptions and supplier reliability. Ann Oper Res 315: 1703–1728. https://link.springer.com/article/10.1007/s10479-020-03639-z |
[17] |
Jauhari WA, Pujawan IN, Suef M (2021) A closed-loop supply chain inventory model with stochastic demand, hybrid production, carbon emissions, and take-back incentives. J Clean Prod 320: 128835. https://doi.org/10.1016/j.jclepro.2021.128835 doi: 10.1016/j.jclepro.2021.128835
![]() |
[18] |
Govindan K, Soleimani H, Kannan D (2015) Reverse logistics and closed-loop supply chain: A comprehensive review to explore the future. Eur J Oper Res 240: 603–626. https://doi.org/10.1016/j.ejor.2014.07.012 doi: 10.1016/j.ejor.2014.07.012
![]() |
[19] |
Kelle P, Schneider H (1992) Extension of a reliability-type inventory model to just-in-time systems. Int J Prod Econ 26: 319–326. https://doi.org/10.1016/0925-5273(92)90082-I doi: 10.1016/0925-5273(92)90082-I
![]() |
[20] |
Kugele ASH, Ahmed W, Sarkar B (2022) Geometric programming solution of second degree difficulty for carbon ejection controlled reliable smart production system. RAIRO-Oper Res 56: 1013–1029. https://doi.org/10.1051/ro/2022028 doi: 10.1051/ro/2022028
![]() |
[21] |
Lin YJ (2010) A stochastic periodic review integrated inventory model involving defective items, backorder price discount, and variable lead time. 4 OR 8: 281–297. https://doi.org/10.1007/s10288-010-0124-x doi: 10.1007/s10288-010-0124-x
![]() |
[22] |
Liu B, Cao J (1999) Analysis of a production–inventory system with machine breakdowns and shutdowns. Comp Oper Res 26: 73–91. https://doi.org/10.1016/S0305-0548(98)00040-9 doi: 10.1016/S0305-0548(98)00040-9
![]() |
[23] |
Lu S, Hou W, Li Z, et al. (2021) Design and optimisation of a green manufacturing-recycling network considering heavy metal pollutants – an electronic assembly case, Int J Prod Res 60: 2830–2849. https://doi.org/10.1080/00207543.2021.1904160 doi: 10.1080/00207543.2021.1904160
![]() |
[24] |
Manna AK, Dey JK, Mondal SK (2018) Two layers supply chain in an imperfect production inventory model with two storage facilities under reliability consideration. J Ind Prod Eng 35: 57–73. https://doi.org/10.1080/21681015.2017.1415230 doi: 10.1080/21681015.2017.1415230
![]() |
[25] |
Modak NM, Modak N, Panda S, et al. (2018) Analyzing structure of two-echelon closed-loop supply chain for pricing, quality and recycling management. J Clean Prod 171: 512–528. https://doi.org/10.1016/j.jclepro.2017.10.033 doi: 10.1016/j.jclepro.2017.10.033
![]() |
[26] |
Pal B, Adhikari S (2021) Random machine breakdown and stochastic corrective maintenance period on an economic production inventory model with buffer machine and safe period. RAIRO-Oper Res 55: S1129–S1149. https://doi.org/10.1051/ro/2020069 doi: 10.1051/ro/2020069
![]() |
[27] |
Panda D, Maiti M (2009) Multi-item inventory models with price dependent demand under flexibility and reliability consideration and imprecise space constraint: A geometric programming approach. Math Comp Model 49: 1733–1749. https://doi.org/10.1016/j.mcm.2008.10.019 doi: 10.1016/j.mcm.2008.10.019
![]() |
[28] |
Poursoltan L, Seyedhosseini SM, Jabbarzadeh A (2020) An extension to the economic production quantity problem with deteriorating products considering random machine breakdown and stochastic repair time. Int J Eng 33: 1567–1578. https://doi.org/10.5829/ije.2020.33.08b.15 doi: 10.5829/ije.2020.33.08b.15
![]() |
[29] |
Rentizelas A, Trivyza N, Oswal S, et al. (2021) Reverse supply network design for circular economy pathways of wind turbine blades in Europe. Int J Prod Res 60: 1795–1814. https://doi.org/10.1080/00207543.2020.1870016 doi: 10.1080/00207543.2020.1870016
![]() |
[30] |
Sana SS (2022) Optimum buffer stock during preventive maintenance in an imperfect production system. Math Meth Appl Sci 45: 8928–8939. https://doi.org/10.1002/mma.8246 doi: 10.1002/mma.8246
![]() |
[31] | Sarkar B, Sarkar M, Dolgui A (2022a) An automated smart production with system reliability under a leader-follower strategy of supply chain management. IFIP Adv Infor Comm Tech 663: 459–567. https://link.springer.com/chapter/10.1007/978-3-031-16407-1_54 |
[32] |
Sarkar B, Mridha B, Pareek S (2022b) A sustainable smart multi-type biofuel manufacturing with the optimum energy utilization under flexible production. J Clean Prod 332: 129869. https://doi.org/10.1016/j.jclepro.2021.129869 doi: 10.1016/j.jclepro.2021.129869
![]() |
[33] |
Sasikumar P, Kannan G (2009) Issues in reverse supply chain, part III: Classification and simple analysis. Int J Sus Eng 2: 2–27. https://doi.org/10.1080/19397030802673374 doi: 10.1080/19397030802673374
![]() |
[34] |
Singh S, Prasher L (2014) A production inventory model with flexible manufacturing, random machine breakdown and stochastic repair time. Int J Ind Eng Comp 5: 575–588. https://doi.org/10.5267/j.ijiec.2014.7.003 doi: 10.5267/j.ijiec.2014.7.003
![]() |
[35] |
Sanni S, Jovanaski Z, Sidhu HS (2020) An economic order quantity model with reverse logistics program Oper Res Pers 7: 100133. https://doi.org/10.1016/j.orp.2019.100133 doi: 10.1016/j.orp.2019.100133
![]() |
[36] |
Srivastava SK (2007) Green supply‐chain management: a state‐of‐the‐art literature review. Int J Manag Rev 9: 53–80. https://doi.org/10.1111/j.1468-2370.2007.00202.x doi: 10.1111/j.1468-2370.2007.00202.x
![]() |
[37] |
Zhang T, Liang L, Yu Y, et al. (2007) An integrated vendor-managed inventory model for a two-echelon system with order cost reduction. Int J Prod Econ 109: 241–253. https://doi.org/10.1016/j.ijpe.2006.12.051 doi: 10.1016/j.ijpe.2006.12.051
![]() |
Parameter | t1 | TC | |
Pr | 300 | 7.37257 | 399069 |
350 | 7.36863 | 583824 | |
400 | 7.36573 | 817840 | |
450 | 7.36350 | 1106890 | |
500 | 7.36173 | 1456730 | |
P | 400 | 7.39605 | 507074 |
450 | 7.37931 | 653559 | |
500 | 7.36573 | 817840 | |
550 | 7.35451 | 1001650 | |
600 | 7.34985 | 1258420 | |
D | 80 | 7.32658 | 1102455 |
90 | 7.34665 | 936542 | |
100 | 7.36573 | 817840 | |
110 | 7.38787 | 651225 | |
120 | 7.40995 | 523109 | |
Pr | 160 | 7.37722 | 821022 |
180 | 7.37085 | 819733 | |
200 | 7.36573 | 817840 | |
220 | 7.36153 | 815702 | |
240 | 7.35801 | 813503 | |
R | 40 | 7.37233 | 874138 |
45 | 7.36889 | 845055 | |
50 | 7.36573 | 817840 | |
55 | 7.36284 | 792320 | |
60 | 7.36019 | 768341 | |
β | 0.40 | 9.20792 | 1595220 |
0.45 | 8.18451 | 1121040 | |
0.50 | 7.36573 | 817840 | |
0.55 | 6.69576 | 615000 | |
0.60 | 5.36548 | 483235 |
Parameter | t1 | TC | |
Pr | 300 | 7.37257 | 399069 |
350 | 7.36863 | 583824 | |
400 | 7.36573 | 817840 | |
450 | 7.36350 | 1106890 | |
500 | 7.36173 | 1456730 | |
P | 400 | 7.39605 | 507074 |
450 | 7.37931 | 653559 | |
500 | 7.36573 | 817840 | |
550 | 7.35451 | 1001650 | |
600 | 7.34985 | 1258420 | |
D | 80 | 7.32658 | 1102455 |
90 | 7.34665 | 936542 | |
100 | 7.36573 | 817840 | |
110 | 7.38787 | 651225 | |
120 | 7.40995 | 523109 | |
Pr | 160 | 7.37722 | 821022 |
180 | 7.37085 | 819733 | |
200 | 7.36573 | 817840 | |
220 | 7.36153 | 815702 | |
240 | 7.35801 | 813503 | |
R | 40 | 7.37233 | 874138 |
45 | 7.36889 | 845055 | |
50 | 7.36573 | 817840 | |
55 | 7.36284 | 792320 | |
60 | 7.36019 | 768341 | |
β | 0.40 | 9.20792 | 1595220 |
0.45 | 8.18451 | 1121040 | |
0.50 | 7.36573 | 817840 | |
0.55 | 6.69576 | 615000 | |
0.60 | 5.36548 | 483235 |