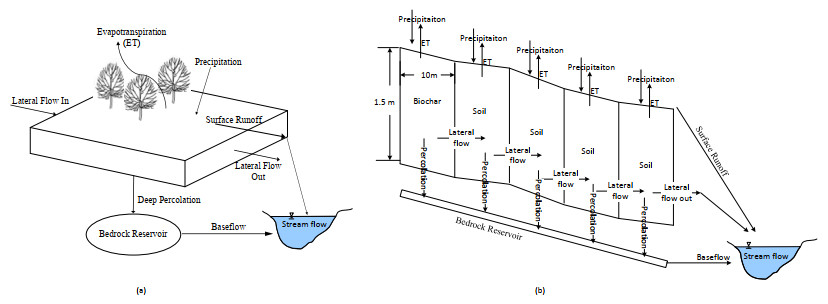
The effect of biochar on hydrologic fluxes was estimated using a single hillslope version of a gridded soil moisture routing (SMR) model. Five grid cells were aligned linearly with varied slopes to simulate a small undulating hillslope with or without a restrictive layer beneath the soil profile. Biochar amendments (redwood sawdust and wheat straw biochar) at concentrations of 0%, 4%, and 7% were applied to the topmost grid-cell by mass of dry soil. Simulated streamflow hydrographs for restricted and non-restricted soil profiles were manually calibrated with measured Palouse River streamflow data. Evapotranspiration, percolation, lateral flow, baseflow, and streamflow were all modeled yearly. Two generally reported field capacities (FC) in literature at −6 and −33 kPa were considered to assess the effect of biochar. Field capacity considered at −6 kPa corresponds to higher moisture content, and hence higher moisture storage capacity between FC and permanent wilting point than at −33 kPa. At −6 kPa FC, biochar effectively increased evapotranspiration and reduced the lateral flow of the system. Increased soil porosity from biochar amendment enhanced the water holding capacity of the soil and plant available water. These mechanisms impacted the streamflow generated from the system indicating positive outcomes from biochar amendment in both restricted and non-restricted soil profiles. Biochar amendment showed an order of magnitude smaller effects with −33 kPa FC compared to −6 kPa FC; the increased porosity appeared to be less influential at lower field capacity values. Additionally, the results showed that the over-application of coarse biochar might negatively affect retaining soil moisture. These findings point to positive results for using biochar as a water management strategy if applied less than 7% in this study, but further exploration is needed to find the optimum level of biochar with different biochar and soil properties.
Citation: Adam O'Keeffe, Erin Brooks, Chad Dunkel, Dev S. Shrestha. Soil moisture routing modeling of targeted biochar amendment in undulating topographies: an analysis of biochar's effects on streamflow[J]. AIMS Environmental Science, 2023, 10(4): 529-546. doi: 10.3934/environsci.2023030
[1] | Hashem Al-Mattarneh, Rabah Ismail, Adnan Rawashdeh, Hamsa Nimer, Mohanad Khodier, Randa Hatamleh, Dua'a Telfah, Yaser Jaradat . Development of new dielectric models for soil moisture content using mixture theory, empirical methods, and artificial neural network. AIMS Environmental Science, 2025, 12(1): 137-164. doi: 10.3934/environsci.2025007 |
[2] | Qianlan Li, Qingdan Wu, Xiaochen Zheng, Pengfei Wang, Dongsheng Zou, Fen Liu, Zhihua Xiao . The conversion and migration behavior of phosphorus speciation during pyrolysis of different sludges. AIMS Environmental Science, 2024, 11(1): 1-20. doi: 10.3934/environsci.2024001 |
[3] | Li Ma, Likun Zhan, Qingdan Wu, Longcheng Li, Xiaochen Zheng, Zhihua Xiao, Jingchen Zou . Optimization of liquefaction process based on global meta-analysis and machine learning approach: Effect of process conditions and raw material selection on remaining ratio and bioavailability of heavy metals in biochar. AIMS Environmental Science, 2024, 11(3): 342-359. doi: 10.3934/environsci.2024016 |
[4] | Diksha Puri, Parveen Sihag, Mohindra Singh Thakur, Mohammed Jameel, Aaron Anil Chadee, Mohammad Azamathulla Hazi . Analysis of data splitting on streamflow prediction using random forest. AIMS Environmental Science, 2024, 11(4): 593-609. doi: 10.3934/environsci.2024029 |
[5] | Adi Setiawan, Ananda Fringki, M. Iqbal Hifzi, Shafira Riskina, Jalaluddin, Eddy Kurniawan, Burhanuddin . The effect of feedstock densification on the process and product properties of sugarcane leaves pyrolysis. AIMS Environmental Science, 2024, 11(6): 866-882. doi: 10.3934/environsci.2024043 |
[6] | Carlos Garcia, Teresa Hernandez, Maria D Coll, Sara Ondoño . Organic amendments for soil restoration in arid and semiarid areas: a review. AIMS Environmental Science, 2017, 4(5): 640-676. doi: 10.3934/environsci.2017.5.640 |
[7] | Maja Radziemska, Agnieszka Bęś, Zygmunt M. Gusiatin, Jerzy Jeznach, Zbigniew Mazur, Martin Brtnický . Novel combined amendments for sustainable remediation of the Pb-contaminated soil. AIMS Environmental Science, 2020, 7(1): 1-12. doi: 10.3934/environsci.2020001 |
[8] | Benjamin Hersh, Amin Mirkouei, John Sessions, Behnaz Rezaie, Yaqi You . A review and future directions on enhancing sustainability benefits across food-energy-water systems: the potential role of biochar-derived products. AIMS Environmental Science, 2019, 6(5): 379-416. doi: 10.3934/environsci.2019.5.379 |
[9] | Voke O. Urhibo, Bernard O. Ejechi . Crude oil degradation potential of bacteria isolated from oil-polluted soil and animal wastes in soil amended with animal wastes. AIMS Environmental Science, 2017, 4(2): 277-286. doi: 10.3934/environsci.2017.2.277 |
[10] | Jerry R. Miller, John P. Gannon, Kyle Corcoran . Concentrations, mobility, and potential ecological risks of selected metals within compost amended, reclaimed coal mine soils, tropical South Sumatra, Indonesia. AIMS Environmental Science, 2019, 6(4): 298-325. doi: 10.3934/environsci.2019.4.298 |
The effect of biochar on hydrologic fluxes was estimated using a single hillslope version of a gridded soil moisture routing (SMR) model. Five grid cells were aligned linearly with varied slopes to simulate a small undulating hillslope with or without a restrictive layer beneath the soil profile. Biochar amendments (redwood sawdust and wheat straw biochar) at concentrations of 0%, 4%, and 7% were applied to the topmost grid-cell by mass of dry soil. Simulated streamflow hydrographs for restricted and non-restricted soil profiles were manually calibrated with measured Palouse River streamflow data. Evapotranspiration, percolation, lateral flow, baseflow, and streamflow were all modeled yearly. Two generally reported field capacities (FC) in literature at −6 and −33 kPa were considered to assess the effect of biochar. Field capacity considered at −6 kPa corresponds to higher moisture content, and hence higher moisture storage capacity between FC and permanent wilting point than at −33 kPa. At −6 kPa FC, biochar effectively increased evapotranspiration and reduced the lateral flow of the system. Increased soil porosity from biochar amendment enhanced the water holding capacity of the soil and plant available water. These mechanisms impacted the streamflow generated from the system indicating positive outcomes from biochar amendment in both restricted and non-restricted soil profiles. Biochar amendment showed an order of magnitude smaller effects with −33 kPa FC compared to −6 kPa FC; the increased porosity appeared to be less influential at lower field capacity values. Additionally, the results showed that the over-application of coarse biochar might negatively affect retaining soil moisture. These findings point to positive results for using biochar as a water management strategy if applied less than 7% in this study, but further exploration is needed to find the optimum level of biochar with different biochar and soil properties.
The Pacific Northwest United States, spanning from southeastern Washington to west-central Idaho, consists of over 2 million acres of agricultural land [1,2] known as the Palouse area. The area is characterized as gentle rolling hills dryland farming. Dryland agricultural systems rely upon stored subsurface water. Patterns of stored water are linked to the topography [3,4], cropping sequences [5], and tillage practices [6,7,8]. Throughout the year, stored soil water fluctuates spatially for various reasons, including climatic changes [9], vegetation[10], topography [11], and heterogeneous soil profiles [12]. The complexities surrounding the fluctuations of soil water create the need for model-based management practices to maximize soil water storage and availability.
Soil management practices are becoming increasingly important with extending drought conditions from climate change. Additionally, topographical attributes, erosion, and historical agricultural management practices have degraded topsoil in the region, hindering the soil's fertility, health, productivity, and water retention capacity [13]. Since the cultivation of the region began, all of the original topsoil has been lost from 10% of the region, and one-fourth to three-fourths of the original topsoil has been lost from another 60 percent of the cropland. From 1939 to 1977, the average annual erosion rate was 20.6 metric ton/hectare (9.2 tons/acre) of available cropland [14]. A study in Whitman County found an average annual erosion rate over 26 years varied from 23 to 77 kg of soil for each kg of wheat raised and that high erosion rates have created soils with shallow topsoil depth, low organic matter, poor water retention, and poor nutrient use and cycling [13].
The undulating topography creates site-specific microclimates from unique patterns of sunlight exposure, snowmelt, and wind [15]. Specific soils throughout the region (typically found in cooler wetter climates) form shallow argillic and fragipan restrictive layers that drive rapid subsurface lateral flows and accelerate the eluviation of clays in albic E-soil horizons leading to perched water tables in the winter months [15,16,17,18]. Shallow soils at the tops of ridges, clay knobs, generally have the most erosion and therefore the argillic layers are closest to the surface. Topography based surface and subsurface lateral flow is an influential determinant of water movement and storage in the winter and spring months [19,20]. While it is well documented that soil properties and topography influence water distribution in a soil profile [9], these relationships are site-specific [21,22]. Combined with diverse management legacies and irregular weather patterns, heterogeneous growing conditions are developed [23], contributing to patterns/variation of water and crop yields.
Winter wheat yields in the Palouse can vary from 335 kg/ha to as high as 7.4 t/ha in the same field [24]. Geostatistical analysis in the Palouse showed that the variability in wheat yields differed from field to field and between areas of a single field [25]. Using regression analysis, a similar study showed that topographic attributes including elevation, slope, and aspect could explain 13 to 35% of the wheat yield variability. Amending soil with biochar, a carbon-rich solid product from thermochemical conversion of biomass improves soil productivity and health [26]. Biochar has shown promise as a method for influencing soil hydrologic properties, soil water retention, and soil fertility [27,28,29,30]. Biochar application significantly increased available water in the coarse-textured soils (by 45%) compared to the medium-and fine-textured soils (by 21% and 14%, respectively), suggesting that biochar may have a greater benefit on coarse-textured soils [31]. It should be noted as described later, the soil used in this study is Palouse silt loam which is not coarse-textured soil.
Targeting areas of Palouse fields that would receive the greatest benefit from biochar amendment, theoretically the tops of hillslopes and with low organic matter areas, farmers could maximize return on investment by improving plant available water. An understanding of how biochar affects soil water distribution and hydrologic fluxes (percolation, lateral flow, baseflow, and streamflow) helps farmers better manage water throughout their fields. The objective of this work is to estimate and model the effects of biochar amendment, applied only at the hilltops of a typical Palouse field, to soil moisture and hydrologic water fluxes within the field.
The model analyzes a hillslope in the Palouse region outside Moscow, Idaho, United States. The Palouse follows precipitation patterns similar to Mediterranean climates with cold, wet winters and warm, dry summers[32] further characterized by a xeric moisture regime [33]. Roughly 60% of the annual precipitation accumulates from November through March, while roughly 5% occurs between July and August [13]. An additional 10% of moisture occurs between March and May [15]. The soil throughout the region is dominated by fine-silty, mixed, super active, mesic Pachic Ultic Haploxerolls [34]. The region is dominated by dryland cereal production with three-year wheat crop rotations, exp. winter wheat – spring wheat – wheat pulse crop.
In this work, the Soil Moisture Routing (SMR) model was used to simulate the effects of targeted biochar amendment on hydrologic processes in a small catchment. The SMR model is a distributed water balance model that operates on a daily time step to predict daily hydrologic responses at any point in a watershed [35]. This model utilizes a grid cell approach to simulate daily soil moisture storage changes under typical meteorological and hydrologic processes. Frankenberger et al. [35] first developed this model to operate in the US Army Corps of Engineers GRASS program and Brooks further modified and developed it for applicability to the Palouse region in the inland Pacific Northwest [35,36]. The physical representation and distributed nature of SMR models permit the assessment of watershed response to precipitation on both integrated and distributed levels [37].
The model tracks the flow into and out of grid cells using a basic mass balance:
(1) |
Where subscript is the cell address, the depth to a hydraulically restricting layer, is the volumetric moisture content of the cell, is the effective precipitation (rain plus snowmelt), is the actual evapotranspiration (ET), is the lateral inflow from surrounding upslope cells, is the lateral outflow to surrounding downslope cells, is the vertical leakage or percolation out of the surface soil layer, R is the surface runoff, A is the area of the grid cell, and t is the time step.
Five grid cells ( = 1 to 5) and area (A = 10 m by 10 m) were aligned linearly together to follow the topography of the selected site (Figure 1). Each grid cell has a different slope with an average slope of 18% across the five grids. Subsurface lateral flow out of a grid cell is simulated using Darcy's equation with hydraulic gradient equal to the land slope following the approach described in Brooks, Boll [36]. Soil depth is fixed to either the maximum rooting depth or to a hydrologically restrictive layer. Vertical leakage out of the bottom of the layer is calculated based upon the saturated hydraulic conductivity (Ksub) of the soil or bedrock matrix below the soil depth whenever the soil moisture content exceeds field capacity assuming a unit hydraulic gradient with Darcy's law. Biochar amendment was applied uniformly only to the top cell to full depth of 1.5m.
The Hargreaves model as described in Allen [38] was used to calculate the reference ET hillslope. Actual ET was modeled according to Brooks, Boll [36] work.
A single crop coefficient was implemented based upon the Food and Agriculture Organization's irrigation and drainage paper and reference evapotranspiration calculation software documentation for FAO and ASCE standardized equations [38,39]. Whether a plant root grows and by how much on a given day depends upon three considerations: the current day's growing degree day, the current day is during the growing season for winter wheat, and the current day is not in the dormancy period for winter wheat. The typical growing season for winter wheat throughout the Palouse is October 15th to August 15th. Winter wheat has a dormancy period throughout winter (assumed when temperatures dip below a base temperature of 4.4 ˚C (~39 ˚F)) [38,40]. During this time, the roots are assumed to not grow in length and will be stagnant.
The root development will begin again when the temperature is consistently above the base temperature of 4.4 ˚C the following spring. This varies from year to year. For simplicity, winter is assumed to begin on November first and end on March first. A growing degree day (a day with increased temperature above threshold) is determined consistent with guidelines by North Dakota Agricultural Weather Network [40]. Biochar's effects on crop yield are estimated from increases in the flux water out of the system from ET. It is assumed that this water goes to transpiration and translates to increases in crop yield. The ET depends on the soil moisture, permanent wilting point of the soil, and the saturated moisture content. Biochar amendment changes the wilting point and saturated soil moisture values for the soil, thus affecting ET. Additionally, biochar acts as mulching for clay soil, hence reducing ET. Baseflow is modeled using an unconfined linear reservoir.
(2) |
Where Q is the baseflow on any given day, a is the representative aquifer constant (0.04 cm/day from [36]; S is the reservoir storage. This model assumes that 100% of the percolation accumulates in and flows through a single linear reservoir which recharges streamflow and does not contribute to deeper aquifer storage (e.g., watertight basin). Percolation is set to either 0.5 cm/day or 20 cm/day depending on if the soil is restrictive or non-restrictive respectively. Streamflow is the summation of the base flow, lateral flow, and runoff.
In order to assess the impacts of biochar amendments we selected a Palouse silt loam soil (dominate soil type throughout the region) and amended the soil at two different biochar concentrations, 4% and 7% by mass of dry soil, corresponding to roughly 1 ton/acre and 5 ton/acre biochar amendments respectively if applied and incorporated within top 15 cm of soil. Two different biochars were used: Redwood Sawdust (RSD) and Wheat Straw (WS). Physical and hydraulic characteristics were measured for all samples with (both types) and without biochar (control, 4% biochar, and 7% biochar). This provided critical information for understanding the physical and soil hydraulic changes the biochar induced in the soil.
The physical properties of the soil samples are shown in Table 1 and measurement method is described in O'Keeffe [41]. The work by O'Keeffe [41] utilizes a HYPROP from METER group to measure biochar's effects on soil hydraulic properties. The HYPROP utilizes theory from Schindler et al. [42] to estimate the water retention curve for a soil [42]. Using fundamental soil physics equations presented in the original work of Van Genuchten [43], the hydraulic conductivity and soil diffusivity curves were determined [43]. These curves relate soil moisture content to hydraulic conductivity and matric potential. For a given matric potential, the curves allow determination of moisture content at field capacity, and permanent wilting point (PWP) to calculate plant available water (PAW).
Soil sample and desired properties | |||||
Control 100% soil | 4% RSD BC | 7% RSD BC | 4% WS BC | 7% WS BC | |
Bulk Density (g/cm3) | 1.22 (0.07) | 1.06 (0.01) | 0.94 (0.02) | 1.07 (0.01) | 0.96 (0.03) |
Porosity (%) | 54% (3%) | 60% (1%) | 65% (1%) | 60% (1%) | 64% (1%) |
Soil Organic Matter | 5.08% | 5.44% | 6.43% | 5.22% | 5.36% |
Field capacity (FC−33kPa) | 33.2% (2.3%) | 33.1% (2.2%) | 27.2% (0.9%) | 32.6% (0.7%) | 32.4% (1.8%) |
Field capacity (FC−6kPa) | 48% (1.3%) | 50.7% (2.1%) | 44% (0.8%) | 50.3% (0.9%) | 53.7% (4.2%) |
PWP (−1500 kPa) | 9.1% (0.5%) | 8.7% (0.5%) | 7.1% (0.5%) | 8.5% (0.1%) | 8.5% (0.8%) |
PAWlow=FC−33kPa− PWP | 24.1% (2%) | 24.4% (1.9%) | 20.1% (0.7%) | 24.1% (0.8%) | 23.9% (2.2%) |
PAWhigh= FC−6kPa− PWP | 39% (1.4%) | 42% (2%) | 37% (0.9%) | 41.8% (1%) | 45.2% (4.8%) |
Vertical Ksat (cm/day) | 49.4 | 102.3 | 148.0 | 101.2 | 157.3 |
Lateral Ksat (cm/day)* | 247.0 | 264.1 | 492.0 | 258.5 | 538.0 |
* Estimated from vertical Ksat as described in O'Keeffe [41] and outlined later in this section. |
Matric potential at FC has been reported between −6 to −33 kPa depending on the texture, structure and content of organic matter in the soil [44]. The reasons for evaluating two different FC values are twofold. First, the bounds for PAW in the soil can be variable. While Permanent Wilting Point PWP is more concrete (−1500 kPa), the upper limit, FC, is commonly reported at −33 kPa, −10 kPa or −6 kPa, which indicates different ranges for the upper limit of plant water uptake [45]. Secondly, FC, PWP, and soil depth are among the parameters with the greatest sensitivity (discussed in section 3.1 Model Calibration and Sensitivity Analysis). Of these parameters, FC displayed the highest sensitivity indicating that changes in FC resulted in more significant changes to model output (Table 4). We considered the bounds of PAW to assess the potential effects of biochar amendment; because PAW indicates when and how much soil water is available for crop use. Local measured soil water potential data were obtained from AgWeatherNet stations that are supported by Washington State University (weather.wsu.edu). Plotting soil water potential data over specified dates can provide insight into soil water potentials that plants may uptake water (Figure 2).
Figure 2 shows that the soil water potential depends on soil depth. The dates each depth drops below FC−33kPa provide some important information. The 12.7 cm depth occurred on April 13th, while the 61 cm depth dropped below FC−33kPa nearly a month later (15th of May). The 5.1 cm soil depth reduces past FC−33kPa on two occasions, the 11th and 29th of March. From the dates Soil water potential (SWP) crossed −33 kPa, it appears that the actual FC was closer to −6 kPa rather than −33 kPa and that SWP greater than −33 kPa during the growing season indicates that this water was likely available for plant use. This assumption provides support for the use of field capacity values greater than −33 kPa closer to −6 kPa. Although SWP in Figure 2 never reached −6 kPa (The highest SWP was near −8 kPa), we used −6 kPa from literature as one of the possible FCs. However, since −33 kPa is one of the reported SWPs in literature and for deeper soil the FC was frequently below −8 kPa, we included FC at −33 kPa in our analysis. From Figure 2, since SWP was near −6 kPa for up to the first five months of the year, the results involving field capacity values of −6 kPa presented in this study closely align with what farmers experience in the field in the Palouse.
PAW is deemed usable by the plant between FC and PWP. Throughout this work, PAW is presented at high and low values, which are indicative of matric potential for FC between −6 and −33 kPa. PAW is an important parameter to monitor the effect of biochar on crop growth. Plant growth is closely related to ET; a higher ET correlates with better plant growth. Higher PAW allows the soil to store more water that is accessible to plants and in a dryland cropping region this can lead to a greater overall seasonal ET. The potential biochar's effect (specifically regarding crop growth) is analyzed throughout this work by estimating change in actual ET.
Biochar amendment to soil affects grid cell's physical and hydrological parameters such as bulk density (BD), FC, PWP, θsat, residual moisture content (θr), soil depth, and Ksat (both vertical and lateral). Lateral saturated hydraulic conductivity (Ksat) can be 5 to 10 times larger than laboratory Ksat values [16]. The HYPROP experiment was used to measure the vertical Ksat. To calculate lateral Ksat, in Table 1, the 100% soil sample was scaled by 5 times in accordance with Brooks et al. (2004) [16]. Biochar samples were scaled based upon the percentage increase in vertical Ksat between the 100% soil sample and each biochar sample. Adding biochar adds to soil volume, and hence increases soil depth to the amendment area. This work assumes a 2 cm soil depth increase for a 4% biochar amendment and 4 cm soil depth increase a 7% amendment increases the soil depth.
The effects of biochar amendment on soil characteristics are linked to the key biochar properties (Table 2). They include the ash content, surface area, coefficient of uniformity (indicator of particle size uniformity in each sample), and the particle size. This was adapted from the O'Keeffe, Shrestha [41]. The RSD sample had higher percentage of larger particles than the WS sample indicated by a larger fraction above 3.35 mm and a smaller fraction below 0.589 mm. Additionally, the RSD sample had more surface area, while the WS sample was more uniform and had a higher ash content.
Particle Size (% mass) | |||||
Sample | Ash content (% mass) | Surface Area (m2/g) | Coefficient of Uniformity | > 3.35 mm | < 0.589 mm |
RSD | 1.94 | 47.12 | 11.6 | 7.62% | 58.45% |
WS | 24.34 | 7.08 | 27.9 | 0.39% | 70.57% |
The model uses data from a SNOTEL site (Site id# 989) near Moscow, Idaho for precipitation [46]. The Moscow Mountain SNOTEL site is deemed representative of the surrounding region. The meteorological inputs were scaled to account for differences in elevation between the SNOTEL and actual site (from 1430 m at SNOTEL site to 786 m which is representative of the surrounding region). Daily temperatures were scaled based on an adiabatic lapse rate of 0.6 ˚C increase with every 100 m decrease in elevation. Daily values for snow water equivalent (SWE), precipitation, observed temperature, max temperature, and minimum temperature were taken from the SNOTEL site for the 2013 to 2019 water years. Five 10 m by 10 m grid cells were aligned linearly. The soil depth was held constant at 1.5 m. The grid cells vary in slope, simulating an undulating topography. The slope of each grid cell from ridge to toe, respectively, was as follows: cell 1 = 5%, cell 2 = 30%, cell 3 = 40%, cell 4 = 10 %, and cell 5 = 3%, equaling an average slope of 18%. Biochar is added uniformly to the topmost grid cell throughout the entire cell. The soil moisture within each cell is assumed to be uniformly distributed at the end of each day. At the beginning of the simulation on October 1st, 2013, the initial storage of each grid cell is set to the PWP.
Two soil profiles were simulated: a soil profile with an argillic/fragipan horizon (restricted layer) and a non-restrictive Palouse silt loam soil. A restrictive layer is denoted by restricting percolation to a max of 0.5 cm/day, whereas in a non-restricted soil profile, percolation is set to a max of 20 cm/day. The topmost grid cell was amended with RSD and WS biochar samples at 4% and 7% concentrations by mass. Note that the FC is reported at two static tensions, −33 kPa and−6 kPa. These values are used in soil balance models to define the maximum water storage in a grid cell [45].
Each hydrologic process is tracked individually across all grid cells. Fluxes of water into and out of each grid cell occur in a quasi-steady state manner in the following order: lateral flow, root zone storage, crop coefficient and actual ET, percolation, moisture redistribution between the layers, runoff, aquifer storage, baseflow, and finally streamflow. This work relies upon fundamentals developed by Frankenberger, Brooks [35].
Simulated hydrographs were generated for the 100% soil profiles (i.e., soil profiles without biochar) (both restricted and non-restricted) for all five grid cells and then calibrated using statistical analysis against measured streamflow data (unit hydrographs) from the Palouse River outside of Potlatch, Idaho, USA. Predicting hydrographs is not the primary purpose of SMR modeling but streamflow integrates hydrologic response from across the watershed, which can then be used to assess the validity of model predictions [35]. Moriasi, Arnold [47] recommend a combination of graphical and statistical techniques for calibration. This paper uses two independent measurements, Nash-Sutcliffe efficiency (NSE), and percent bias (PBIAS) to determine each of the statistical tests for model assessment as follows:
(3) |
(4) |
NSE values above 0.5 were acceptable, with values exceeding 0.75 showing good model performance. PBIAS is deemed acceptable with bias being within ± 25%. Sensitivity analysis was performed on the individual variables of the model with a restricted and non-restricted soil profile by changing the baseline input value by ± 3% incrementally by 1%. The effects of FC, PWP, lateral Ksat, θsat, and soil depth were all tested with sensitivity analysis. Sensitivity analysis helps identify parameters that impact model output and, therefore, influence model response [48].
While the simulated hydrographs under predict the more significant streamflow events and over predict some areas of the measured data (Figure 3), the hydrographs track the yearly streamflow events well. Streamflow increases and decreases at similar times throughout the year, which indicates the model is performing adequately. The model appears to track the timing of the observed increase in rain and snow that hits the region in the late fall and winter months as well as capturing the timing of melt and wet spring months. Since the model area is much smaller than the entire catchment, the model is expected to capture only the general shape.
Based upon the guidelines set by Moriasi, Arnold [47], the simulated scenarios showed acceptable performance levels. Both simulated scenarios performed inadequately for PBIAS, and NSE. These results are summarized in Table 3.
Statistical test | Restricted | Non-restricted |
NSE | 0.36 | 0.37 |
PBIAS | −0.33 | −0.34 |
Table 4 displays the sensitivity analysis performed on each input parameter regarding ET and streamflow for each of restricted and non-restricted soil profiles. Sensitivity is defined as the change in response per unit change in input parameters to help identify important model parameters.
Restricted soil profile | Non- Restricted soil profile | |||
Input | Streamflow Sensitivity | ET Sensitivity | Streamflow Sensitivity | ET Sensitivity |
PWP | 0.17 | −0.13 | 0.17 | −0.13 |
FC | −0.62 | 0.46 | −0.62 | 0.47 |
Soil depth | −0.33 | 0.24 | −0.33 | 0.25 |
Θsat | −1.1 × 10−3 | 1.1 × 10−3 | −5.0 × 10−5 | 1.8 × 10−3 |
Lateral Ksat | 4.0 × 10−4 | −4.0 × 10−4 | 2.0 × 10−5 | −2.0 × 10−5 |
Initial Storage amount (cm) | 3.7 × 10−2 | 2.5 × 10−2 | −2.4 × 10−3 | −3.7 × 10−2 |
The sensitivity analysis showed that PWP, FC, and soil depth are the parameters that demonstrate the greatest influence on the model output. This is an important consideration because biochar amendment to a soil will influence each of these three parameters. Furthermore, streamflow and ET exhibit an inverse relationship, indicating that changes to each of these parameters would allow for either more or less water to be used as ET depending upon the sign convention presented in Table 4. Maximizing the desired influence of biochar (increasing the PAW as a total water depth) incorporates all three of these parameters.
Both RSD and WS biochar amendment increased the field capacity at the 4% amendment level. At 7%, WS biochar further increased the FC−6kPa whereas RSD biochar reduced FC−6kPa (Table 1). This difference is mainly attributed to the contrast in particle size distribution and coarse fraction of biochar (Table 2). RSD biochar has a much higher coarse fraction (7.62% with greater than 3.5mm) while WS biochar was much finer (only 0.39% with greater than 3.5 mm) with much higher uniformity coefficient. The trend was similar for field capacity at −33 kPa except the magnitude of change was much smaller.
The overall effect due to the increase in field capacity is an increase in plant water availability and more ET. Biochar amendment had variable effects in a non-restricted soil profile and at FC−33kPa. The change in each of the average annual simulated fluxes from biochar (amendment – control) is displayed in Figure 4. Further, this figure displays the outcomes from each of the simulated cases (restricted and non-restricted soil profiles and FC−33kPa and FC−6kPa). Error bars in each figure denote a 95% confidence interval (CI) for each hydrologic flux and amendment. A moving average was used to determine the 95% CI for average annual flux values based on daily changes in soil storage. The benefit of using a moving average lies in the preservation of the degrees of freedom compared to simply using the average annual values. This allows for the cumulative variation over the 6 years of data to be preserved and reflected in the 95% CI presented.
The benefit of biochar to crops is primarily determined by ET. An increase in ET implies that biochar amendment increases the water available for crops, which is desired. Literature suggests that every 2.54 cm (1 inch) increase in available moisture contributes to a 403 kg/ha (6 bu/acre) increase in wheat yield [49]. This estimate indicates the potential effect of biochar on crop yield just in terms of changes in soil storage. Lateral flow and baseflow are included in the results because, in this model, these hydrologic fluxes are two components of streamflow.
The results for non-restricted soil profiles are shown in Figure 4(a) and 4(b). In Figure 4 (a), there is minimal (almost zero) lateral flow and runoff at FC−33kPa. The average annual percolation and the baseflow are nearly identical when examined on an average annual scale. This is due to the model assumption that percolation is the only source of base flow, and they are closely related but lagging in time. Percolation occurs when the grid cell is above FC and set to 20 cm/day in the unrestricted soil profile and 0.5 cm/day for the restricted soil profile. Percolation of a max of 20 cm/day negates potential runoff and most lateral flow due to the soil rarely remaining near or above saturation after percolation. Therefore, the differences in lateral flow from biochar amendment are minimal for non-restricted soil profiles because most of the streamflow is generated entirely from the baseflow out of the grid cell.
At FC−33kPa, three biochar amendments (4% RSD, 4% WS, and 7% WS) showed increased ET, and reduction in percolation, baseflow, and streamflow (Figure 4a). At FC−6kPa, (Figure 4b), the effect was even larger. An increase in field capacity, from FC−33kPa, to FC−6kPa created more variation in the effect of biochar amendments. However, the 7% RSD amendment showed opposite results than the other amendments in non-restricted soil profiles and when FC was set to −33 kPa. This is likely because of the significant changes to the porosity and void space in the soil profile. While the RSD and WS samples changed the porosity of the soil in a similar manner, the RSD is coarser biochar with larger particle size Table 2, compared to the WS biochar. The larger particles resulted in larger pores, which facilitated easier pore drainage at higher water concentrations. Easier drainage with a 7% RSD amendment is displayed when evaluating the field capacity and plant available water values [24]. At 7% RSD amendment, the field capacity at −33 kPa reduced from 33.2% to 27.2%, significantly reducing the PAW. Other amendments had similar PAW compared to 100% soil.
The results suggest an optimal level of biochar amendment exists for maximum benefits such as 'maximum water holding capacity' or 'reduced streamflow and erosion'.. Specific biochar characteristics could be used to achieve desired results across various soil types. Biochar coarseness and porosity are important considerations when amending to a soil. While the WS sample showed increases in porosity it demonstrated higher retention at or near saturation, which points to higher effectiveness for the desired effect. Although the theories behind why the 7% RSD amendment oppositely affects the hydrologic fluxes are suggestive, more research is required to understand the complexities of this interaction.
The restricted soil profiles pertain to Figure 4(c) and 4(d).At FC−33kPa, 7% RSD, 4% WS, and 7% WS showed increases in average yearly ET and reductions in percolation, lateral flow, and baseflow culminating in a reduction in streamflow (Figure 4 c). The changes of each of these fluxes varied between samples, however the 4% WS and 7% WS samples did not significantly change percolation from each other. The RSD samples showed opposite effects when amended at 4 and 7%, denoting that a higher concentration of RSD biochar would reduce the ET while increasing the lateral flow and overall streamflow. This was consistent with the results in the non-restricted soil profile and likely due to the same particle size effects as discussed previously. The WS samples showed more consistent results at FC−33kPa and restricted soils. Increasing the concentration of WS biochar in the soil showed similar changes to ET while showing larger reductions in percolation compared to the 4% amendment.
However, in Figure 4(d), the 7% WS amendment demonstrated different effects than the 4% WS amendment. The 7% WS amendment demonstrated increased runoff while reducing percolation, lateral flow, and baseflow. The increase in runoff indicates that the 7% WS increased the FC−6kPa to the point that increased the susceptibility of the soil to saturation excess runoff. It is plausible that the increased susceptibility is due to the reduction in the difference between the saturated water content, θsat, and FC−6kPa. Whenever the cell storage is above field capacity a saturated thickness forms at the base of a cell and generates lateral flow. When the difference between FC−6kPa and θsat is relatively large most of the water will leave a cell as lateral flow (the primary reason that runoff is minimal when FC is set to −33 kPa). When the difference between FC and θsat is relatively small, a shallower saturated layer forms at the base of the cell and less daily lateral flow leaves the cell. There is a reduction in the potential lateral flow from a cell. Simply put, less water is required to reach θsat from FC when the difference between the two is small. When moderate or large rainfall events occur, more runoff will be generated due to the reduction in lateral flow. This outcome also affects the amount of percolation that is generated (which, like lateral flow, occurs whenever the cell is above FC). Overall, the 7% WS amendment showed the largest reduction in streamflow, which subsequently increased the yearly ET the most amongst all samples. These notions are demonstrated in the 7% WS results in Figure 4 d. All samples showed a reduction in lateral flow. The 4% RSD, 7% RSD, and 4% WS showed increases to percolation and baseflow.
Although statistical testing showed inadequate model performance predicting sharp peaks in Figure 3, graphical inspection demonstrates that the model is performing adequately by tracking the timing of moisture accumulation and discharge as well as general timing. The statistical analysis results are not surprising given the small test area data being used to predict the entire watershed flow. Despite the statistical testing the model is verified in its ability to track yearly streamflow events.
The developed SMR model is an effective tool that can be used to estimate biochar's effects on the greater hydrologic processes in a hillslope. The strength of a model relies not only upon consistent calibration and statistical testing [47] but clear and distinct presentation regarding the limitations of the model [50].
This model lacks the inclusion of parameters that may affect the output, mainly infiltration from rainfall and infiltration excess runoff. Incorporating these fluxes would provide a more complete model. Implementing a more standard reference ET model, Penman-Monteith's reference ET model [39] opposed to the Hargreaves ET model, would increase the accuracy of the daily actual ET flux out of the system. This model does not account for frozen soils in the wintertime, which would have some influence on the hydrologic fluxes of water, which is a limitation that may lead to poor simulation for some years, specifically during the winter months. Finally, deep percolation to an aquifer could be included to assess the potential influence of biochar on groundwater recharge.
The effectiveness of biochar amendment hinges upon biochar's ability to increase ET and PAW (crop use water) while reducing the hydrologic fluxes that facilitate streamflow and erosion (mainly lateral flow and runoff). Reductions in lateral flow and runoff indicate the possibility of using biochar as an erosion mitigation technique in agricultural soils. Conversely, increases to percolation from biochar amendment suggest the possibility of using biochar as a method of recharging aquifers. Pairing reduced streamflow with increased ET indicates more water is stored in the profile and is available for crop use, since winter wheat is growing throughout this simulation.
Adding biochar to soil had mixed effects on field capacity depending on biochar type and matric potential at field capacity. Soil porosity consistently increased by adding biochar, and with low level of biochar application, the plant available water consistently increased. However, because biochar has a lower affinity to water at field capacity (water draining more easily), plant available water was decreased for higher rate (7%) of redwood sawdust biochar application. The decrease in plant available water was more pronounced for redwood sawdust biochar than wheat straw biochar. For non-restricted soil profiles, biochar amendment showed an increase in evapotranspiration (ET) and a reduction in lateral flow and percolation, leading to an overall reduction in yearly streamflow derived from the hillslope. Increase in ET is good for crop production. In restricted soil profiles, biochar amendment reduces the saturation excess runoff and lateral flow while increasing the percolation and ET. This leads to an overall reduction in yearly streamflow from this simulated catchment. These results are more prominent if the upper limit of available water (FC) is, in fact, −6 kPa rather than −33kPa. However, we acknowledge the overall impact of biochar amendment to the soil was relatively small, 0.3 cm/year. Compared to the measured yearly ET values close to 50 cm/year, biochar's impact appears minimal. However, it is important to note that biochar's potential impact extends beyond affecting the water retention in a soil, which is not simulated here. This analysis shows promise for integrating biochar into precision agriculture and warrants further research and exploration in the field and continued model refinement.
We have not used Artificial Intelligence (AI) tools in the creation of this article.
Funding: This work was supported by the USDA National Institute of Food and Agriculture, Hatch project 1009342, and the US Department of Energy's Industrial Assessment Grant. Publication of this article was funded by the University of Idaho - Open Access Publishing Fund. Supplemental material (data and calculations used in this paper) can be found at https://data.mendeley.com/datasets/8c4n535x9z
The Authors have no conflict of interest in the results of this paper.
[1] | Hall M, Young D L, Walker D J (1999) Agriculture in the Palouse, a Portrait of Diversity. Moscow, ID: BUL 794. University of Idaho. |
[2] | Hartmans MA, Michalson EL (1998) Evaluating the Economic & Environmental Impacts of Farming Practices on the Palouse Using PLANETOR. Moscow, ID: Agricultural Experiment & UI Extension Publications, University of Idaho. |
[3] |
Beven KJ, Kirkby MJ (1979) A physically based, variable contributing area model of basin hydrology / Un modèle à base physique de zone d'appel variable de l'hydrologie du bassin versant. Hydrolog Sci Bull 24: 43–69. doi: https://doi.org/10.1080/02626667909491834. doi: 10.1080/02626667909491834
![]() |
[4] |
Wilson DJ, Western AW, Grayson RB (2005) A terrain and data-based method for generating the spatial distribution of soil moisture. Adv Water Resour 28: 43–54. doi: https://doi.org/10.1016/j.advwatres.2004.09.007. doi: 10.1016/j.advwatres.2004.09.007
![]() |
[5] |
Schlegel AJ, Assefa Y, Haag LA, et al. (2017) Yield and Soil Water in Three Dryland Wheat and Grain Sorghum Rotations. Agron J 109: 227–38. doi: https://doi.org/10.2134/agronj2016.07.0387. doi: 10.2134/agronj2016.07.0387
![]() |
[6] |
Fuentes JP, Flury M, Huggins DR, et al. (2003) Soil water and nitrogen dynamics in dryland cropping systems of Washington State, USA. Soil Till Res USA 71: 33–47. doi: https://doi.org/10.1016/S0167-1987(02)00161-7. doi: 10.1016/S0167-1987(02)00161-7
![]() |
[7] |
Jin K, Cornelis WM, Schiettecatte W, et al. (2007) Effects of different management practices on the soil–water balance and crop yield for improved dryland farming in the Chinese Loess Plateau. Soil Till Res 96: 131–44. doi: https://doi.org/10.1016/j.still.2007.05.002. doi: 10.1016/j.still.2007.05.002
![]() |
[8] |
Kühling I, Redozubov D, Broll G, et al. (2017) Impact of tillage, seeding rate and seeding depth on soil moisture and dryland spring wheat yield in Western Siberia. Soil Till Res 170: 43–52. doi: https://doi.org/10.1016/j.still.2017.02.009. doi: 10.1016/j.still.2017.02.009
![]() |
[9] |
Brown M, Heinse R, Johnson-Maynard J, et al. (2021) Time-lapse mapping of crop and tillage interactions with soil water using electromagnetic induction. Vadose Zone J 20: e20097. doi: https://doi.org/10.1002/vzj2.20097. doi: 10.1002/vzj2.20097
![]() |
[10] |
Eagleson PS (1978) Climate, soil, and vegetation: 1. Introduction to water balance dynamics. Water Resour Res 14: 705–12. doi: https://doi.org/10.1029/WR014i005p00705. doi: 10.1029/WR014i005p00705
![]() |
[11] |
Burt TP, Butcher DP (1985) Topographic controls of soil moisture distributions. J Soil Sci 36: 469–486. doi: https://doi.org/10.1111/j.1365-2389.1985.tb00351.x. doi: 10.1111/j.1365-2389.1985.tb00351.x
![]() |
[12] |
Sheets KR, Hendrickx JMH (1995) Noninvasive Soil Water Content Measurement Using Electromagnetic Induction. Water Resour Res 31: 2401–2419. doi: https://doi.org/10.1029/95WR01949. doi: 10.1029/95WR01949
![]() |
[13] | Kaiser VG (1967) Soil erosion and wheat yields in Whitman County, Washington. Northwest Sci. Soil erosion and wheat yields in Whitman County, Washington 41: 6. |
[14] | USDA (1978) Palouse Cooperative River Basin Study. Washington D.C.: 1978. Report No.: 719153. |
[15] | Brooks ES, Boll J, McDaniel PA (2012) Chapter 10 - Hydropedology in Seasonally Dry Landscapes: The Palouse Region of the Pacific Northwest USA. In: Lin H, editor. Hydropedology. Boston: Academic Press. p. 329–350. |
[16] |
Brooks ES, Boll J, McDaniel PA (2004) A hillslope-scale experiment to measure lateral saturated hydraulic conductivity. Water Resour Res 40. doi: https://doi.org/10.1029/2003WR002858. doi: 10.1029/2003WR002858
![]() |
[17] |
McDaniel PA, Gabehart RW, Falen AL, et al. (2001) Perched Water Tables on Argixeroll and Fragixeralf Hillslopes. Soil Sci Soc Am J s 65: 805–810. doi: https://doi.org/10.2136/sssaj2001.653805x. doi: 10.2136/sssaj2001.653805x
![]() |
[18] |
McDaniel PA, Regan MP, Brooks E, et al. (2008) Linking fragipans, perched water tables, and catchment-scale hydrological processes. Catena 73: 166–173. doi: https://doi.org/10.1016/j.catena.2007.05.011. doi: 10.1016/j.catena.2007.05.011
![]() |
[19] |
Western AW, Grayson RB, Blöschl G (2002) Scaling of Soil Moisture: A Hydrologic Perspective. Annu Rev Earth Pl Sc 30: 149–180. doi: https://doi.org/10.1146/annurev.earth.30.091201.140434. doi: 10.1146/annurev.earth.30.091201.140434
![]() |
[20] |
Zhu Q, Lin H, Doolittle J (2010) Repeated Electromagnetic Induction Surveys for Determining Subsurface Hydrologic Dynamics in an Agricultural Landscape. Soil Sci Soc Am J 74: 1750–1762. doi: https://doi.org/10.2136/sssaj2010.0055. doi: 10.2136/sssaj2010.0055
![]() |
[21] |
Corwin DL, Lesch SM (2003) Application of Soil Electrical Conductivity to Precision Agriculture. Agron J 95: 455–471. doi: https://doi.org/10.2134/agronj2003.4550. doi: 10.2134/agronj2003.4550
![]() |
[22] |
Corwin DL, Lesch SM (2005) Apparent soil electrical conductivity measurements in agriculture. Comput Electron Agr 46: 11–43. doi: https://doi.org/10.1016/j.compag.2004.10.005. doi: 10.1016/j.compag.2004.10.005
![]() |
[23] | Weddell B, Brown T, Borrelli K (2017) Chapter 8: Precision Agriculture. In: Yorgey G, Kruger C, editors. Advances in Dryland Farming in the Inland Pacific Northwest. Pullman, Washington: Washington State University. |
[24] | O'Keeffe AL, Shrestha D, Brooks E, et al. (2020) Modeling Palouse hills to quantify moisture redistribution from the selective non-uniform application of biochar. SABE Annual International Meeting; Omaha, Nebraska: American Society of Agricultural and Biological Engineers. p. pages 1–12. |
[25] |
Yang C, Peterson CL, Shropshire GJ, et al. (1998) Spatial variability of field topography and wheat yield in the Palouse region of the Pacific Northwest. T ASAE 41: 17–27. doi: https://doi.org/10.13031/2013.17147. doi: 10.13031/2013.17147
![]() |
[26] | Lehmann J, Joseph S (2009) Chapter 1- Biochar for Environmental Management: An Introduction. Biochar for Environmental Management: Routledge. |
[27] | Masiello CA, Dugan B, Brewer CE, et al. (2019) Biochar effects on soil hydrology. Biochar for Environmental Management 2nd Edition. |
[28] |
Aller D, Rathke S, Laird D, et al. (2017) Impacts of fresh and aged biochars on plant available water and water use efficiency. Geoderma 307: 114–121. doi: https://doi.org/10.1016/j.geoderma.2017.08.007. doi: 10.1016/j.geoderma.2017.08.007
![]() |
[29] |
Dokoohaki H, Miguez FE, Laird D, et al. (2017) Assessing the Biochar Effects on Selected Physical Properties of a Sandy Soil: An Analytical Approach. Commun Soil Sci Plan 48: 1387–1398. doi: https://doi.org/10.1080/00103624.2017.1358742. doi: 10.1080/00103624.2017.1358742
![]() |
[30] | Joint Research C, Institute for E, Sustainability, Bastos A, Verheijen F, Jeffery S (2010) Biochar application to soils: a critical scientific review of effects on soil properties, processes and functions: Publications Office. |
[31] |
Razzaghi F, Obour PB, Arthur E (2020) Does biochar improve soil water retention? A systematic review and meta-analysis. Geoderma 361: 114055. doi: https://doi.org/10.1016/j.geoderma.2019.114055. doi: 10.1016/j.geoderma.2019.114055
![]() |
[32] | McCool D, Huggins D, Saxton K, et al. (2001) Factors affecting agricultural sustainability in the Pacific Northwest, USA: An overview.. In: Mohtar RH, Steinhardt GC, Stott DE, editors. Sustaining the Global Farm: Selected Papers from the 10th International Soil Conservation Organization Meeting. West Lafayette: Purdue University. p. 255–260. |
[33] | NRCS (2022) Keys to soil taxonomy. 13th ed: United States Department of Agriculture, Natural Resources Conservation Service. |
[34] | NRCS (2023) Web Site for Official Soil Series Descriptions and Series Classification Washington D.C.: U.S. Department of Agriculture, National Cooperative Soil Survey. Available from: https://soilseries.sc.egov.usda.gov/OSD_Docs/P/PALOUSE.html. |
[35] |
Frankenberger JR, Brooks ES, Walter MT, et al. (1999) A GIS-based variable source area hydrology model. Hydrol Process 13: 805–822. doi: https://doi.org/10.1002/(SICI)1099-1085(19990430)13:6<805::AID-HYP754>3.0.CO;2-M. doi: 10.1002/(SICI)1099-1085(19990430)13:6<805::AID-HYP754>3.0.CO;2-M
![]() |
[36] |
Brooks ES, Boll J, McDaniel PA (2007) Distributed and integrated response of a geographic information system-based hydrologic model in the eastern Palouse region, Idaho. Hydrol Process 21: 110–122. doi: https://doi.org/10.1002/hyp.6230. doi: 10.1002/hyp.6230
![]() |
[37] |
Johnson MS, Coon WF, Mehta VK, et al. (2003) Application of two hydrologic models with different runoff mechanisms to a hillslope dominated watershed in the northeastern US: a comparison of HSPF and SMR. J Hydrol 284: 57–76. doi: https://doi.org/10.1016/j.jhydrol.2003.07.005. doi: 10.1016/j.jhydrol.2003.07.005
![]() |
[38] | Allen R (2013) Ref-ET: Reference Evapotranspiration Calculation Software for FAO and ASCE Standardized Equations Kimberly, Idaho: University of Idaho; [cited 2023 May, 28]. Version 3.1: [Available from: https://www.webpages.uidaho.edu/ce325bae355/references/manual_prn.pdf. |
[39] | Allen RG, Pereira LS, Raes D, et al. (1998) FAO Irrigation & drainage Paper No. 56: Crop evapotranspiration (guidelines for computing crop water requirements).Rome, Italy: FAO - Food and Agriculture Organization of the United Nations. |
[40] | NDAWN (2023) Wheat Growth Stage Prediction Using Growing Degree Days (GDD) Fargo, North Dakota: North Dakota Agricultural Weather Network; [cited 2023 May, 28]. Available from: https://ndawn.ndsu.nodak.edu/help-wheat-growing-degree-days.html. |
[41] |
O'Keeffe A, Shrestha D, Dunkel C, et al. (2023) Modeling moisture redistribution from selective non-uniform application of biochar on Palouse hills. Agr Water Manage 277. doi: https://doi.org/10.1016/j.agwat.2022.108026. doi: 10.1016/j.agwat.2022.108026
![]() |
[42] |
Schindler U, Durner W, von Unold G, et al. (2010) Evaporation Method for Measuring Unsaturated Hydraulic Properties of Soils: Extending the Measurement Range. Soil Sci Soc Am J 74: 1071–1083. doi: https://doi.org/10.2136/sssaj2008.0358. doi: 10.2136/sssaj2008.0358
![]() |
[43] |
Van Genuchten M (1980) A Closed-form Equation for Predicting the Hydraulic Conductivity of Unsaturated Soils Soil Sci Soc Am J 44. doi: https://doi.org/10.2136/sssaj1980.03615995004400050002x. doi: 10.2136/sssaj1980.03615995004400050002x
![]() |
[44] | de Oliveira RA, Ramos MM, de Aquino LA (2015) Chapter 8 - Irrigation Management. In: Santos F, Borém A, Caldas C, editors. Sugarcane. San Diego: Academic Press. p. 161–183. |
[45] |
de Jong van Lier Q (2017) Field capacity, a valid upper limit of crop available water? Agr Water Manage 193: 214–220. doi: https://doi.org/10.1016/j.agwat.2017.08.017. doi: 10.1016/j.agwat.2017.08.017
![]() |
[46] | NRCS (2023) National Water and Climate Center Data: United States Department of Agriculture, Natural Resources Conservation Service. Available from: https://wcc.sc.egov.usda.gov/nwcc/site?sitenum = 989. |
[47] |
Moriasi DN, Arnold JG, Van Liew MW, et al. (2007) Model Evaluation Guidelines for Systematic Quantification of Accuracy in Watershed Simulations. T ASABE 50: 885–900. doi: https://doi.org/10.13031/2013.23153. doi: 10.13031/2013.23153
![]() |
[48] |
Devak M, Dhanya CT (2017) Sensitivity analysis of hydrological models: review and way forward. J Water Clim Change 8: 557–575. doi: https://doi.org/10.2166/wcc.2017.149. doi: 10.2166/wcc.2017.149
![]() |
[49] | Koenig RT (2005) Dryland Winter Wheat : Eastern Washington Nutrient Management Guide. Department of Crop and Soil Sciences, Washington State University, Pullman. |
[50] |
Grayson RB, Moore ID, McMahon TA (1992) Physically based hydrologic modeling: 2. Is the concept realistic? Water Resour Res 28: 2659–2666. doi: https://doi.org/10.1029/92WR01259. doi: 10.1029/92WR01259
![]() |
1. | Qianlan Li, Qingdan Wu, Xiaochen Zheng, Pengfei Wang, Dongsheng Zou, Fen Liu, Zhihua Xiao, The conversion and migration behavior of phosphorus speciation during pyrolysis of different sludges, 2024, 11, 2372-0352, 1, 10.3934/environsci.2024001 |
Soil sample and desired properties | |||||
Control 100% soil | 4% RSD BC | 7% RSD BC | 4% WS BC | 7% WS BC | |
Bulk Density (g/cm3) | 1.22 (0.07) | 1.06 (0.01) | 0.94 (0.02) | 1.07 (0.01) | 0.96 (0.03) |
Porosity (%) | 54% (3%) | 60% (1%) | 65% (1%) | 60% (1%) | 64% (1%) |
Soil Organic Matter | 5.08% | 5.44% | 6.43% | 5.22% | 5.36% |
Field capacity (FC−33kPa) | 33.2% (2.3%) | 33.1% (2.2%) | 27.2% (0.9%) | 32.6% (0.7%) | 32.4% (1.8%) |
Field capacity (FC−6kPa) | 48% (1.3%) | 50.7% (2.1%) | 44% (0.8%) | 50.3% (0.9%) | 53.7% (4.2%) |
PWP (−1500 kPa) | 9.1% (0.5%) | 8.7% (0.5%) | 7.1% (0.5%) | 8.5% (0.1%) | 8.5% (0.8%) |
PAWlow=FC−33kPa− PWP | 24.1% (2%) | 24.4% (1.9%) | 20.1% (0.7%) | 24.1% (0.8%) | 23.9% (2.2%) |
PAWhigh= FC−6kPa− PWP | 39% (1.4%) | 42% (2%) | 37% (0.9%) | 41.8% (1%) | 45.2% (4.8%) |
Vertical Ksat (cm/day) | 49.4 | 102.3 | 148.0 | 101.2 | 157.3 |
Lateral Ksat (cm/day)* | 247.0 | 264.1 | 492.0 | 258.5 | 538.0 |
* Estimated from vertical Ksat as described in O'Keeffe [41] and outlined later in this section. |
Particle Size (% mass) | |||||
Sample | Ash content (% mass) | Surface Area (m2/g) | Coefficient of Uniformity | > 3.35 mm | < 0.589 mm |
RSD | 1.94 | 47.12 | 11.6 | 7.62% | 58.45% |
WS | 24.34 | 7.08 | 27.9 | 0.39% | 70.57% |
Statistical test | Restricted | Non-restricted |
NSE | 0.36 | 0.37 |
PBIAS | −0.33 | −0.34 |
Restricted soil profile | Non- Restricted soil profile | |||
Input | Streamflow Sensitivity | ET Sensitivity | Streamflow Sensitivity | ET Sensitivity |
PWP | 0.17 | −0.13 | 0.17 | −0.13 |
FC | −0.62 | 0.46 | −0.62 | 0.47 |
Soil depth | −0.33 | 0.24 | −0.33 | 0.25 |
Θsat | −1.1 × 10−3 | 1.1 × 10−3 | −5.0 × 10−5 | 1.8 × 10−3 |
Lateral Ksat | 4.0 × 10−4 | −4.0 × 10−4 | 2.0 × 10−5 | −2.0 × 10−5 |
Initial Storage amount (cm) | 3.7 × 10−2 | 2.5 × 10−2 | −2.4 × 10−3 | −3.7 × 10−2 |
Soil sample and desired properties | |||||
Control 100% soil | 4% RSD BC | 7% RSD BC | 4% WS BC | 7% WS BC | |
Bulk Density (g/cm3) | 1.22 (0.07) | 1.06 (0.01) | 0.94 (0.02) | 1.07 (0.01) | 0.96 (0.03) |
Porosity (%) | 54% (3%) | 60% (1%) | 65% (1%) | 60% (1%) | 64% (1%) |
Soil Organic Matter | 5.08% | 5.44% | 6.43% | 5.22% | 5.36% |
Field capacity (FC−33kPa) | 33.2% (2.3%) | 33.1% (2.2%) | 27.2% (0.9%) | 32.6% (0.7%) | 32.4% (1.8%) |
Field capacity (FC−6kPa) | 48% (1.3%) | 50.7% (2.1%) | 44% (0.8%) | 50.3% (0.9%) | 53.7% (4.2%) |
PWP (−1500 kPa) | 9.1% (0.5%) | 8.7% (0.5%) | 7.1% (0.5%) | 8.5% (0.1%) | 8.5% (0.8%) |
PAWlow=FC−33kPa− PWP | 24.1% (2%) | 24.4% (1.9%) | 20.1% (0.7%) | 24.1% (0.8%) | 23.9% (2.2%) |
PAWhigh= FC−6kPa− PWP | 39% (1.4%) | 42% (2%) | 37% (0.9%) | 41.8% (1%) | 45.2% (4.8%) |
Vertical Ksat (cm/day) | 49.4 | 102.3 | 148.0 | 101.2 | 157.3 |
Lateral Ksat (cm/day)* | 247.0 | 264.1 | 492.0 | 258.5 | 538.0 |
* Estimated from vertical Ksat as described in O'Keeffe [41] and outlined later in this section. |
Particle Size (% mass) | |||||
Sample | Ash content (% mass) | Surface Area (m2/g) | Coefficient of Uniformity | > 3.35 mm | < 0.589 mm |
RSD | 1.94 | 47.12 | 11.6 | 7.62% | 58.45% |
WS | 24.34 | 7.08 | 27.9 | 0.39% | 70.57% |
Statistical test | Restricted | Non-restricted |
NSE | 0.36 | 0.37 |
PBIAS | −0.33 | −0.34 |
Restricted soil profile | Non- Restricted soil profile | |||
Input | Streamflow Sensitivity | ET Sensitivity | Streamflow Sensitivity | ET Sensitivity |
PWP | 0.17 | −0.13 | 0.17 | −0.13 |
FC | −0.62 | 0.46 | −0.62 | 0.47 |
Soil depth | −0.33 | 0.24 | −0.33 | 0.25 |
Θsat | −1.1 × 10−3 | 1.1 × 10−3 | −5.0 × 10−5 | 1.8 × 10−3 |
Lateral Ksat | 4.0 × 10−4 | −4.0 × 10−4 | 2.0 × 10−5 | −2.0 × 10−5 |
Initial Storage amount (cm) | 3.7 × 10−2 | 2.5 × 10−2 | −2.4 × 10−3 | −3.7 × 10−2 |