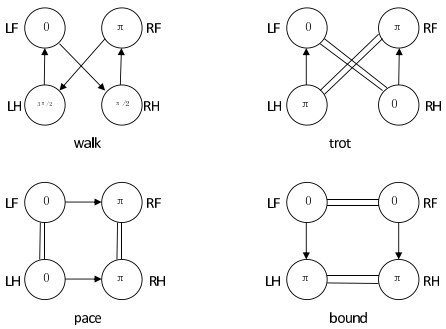
In a bottom-up approach, agent-based models have been extensively used in finance and economics in order to understand how macro-level phenomena can emerge from myriads of micro-level behaviours of individual agents. Moreover, in the absence of (big) data there is still the need to test economic theories and understand how macro-level laws can be materialized as the aggregate of a multitude of interactions of discrete agents. We exemplify how we can solve this problem in a particular instance: We introduce an agent-based method in order to generate data with Monte Carlo and then we interpolate the data with machine learning methods in order to derive multi-parametric demand functions. In particular, the model we construct is implemented in a simulated economy with 1000 consumers and two products, where each consumer is characterized by a unique set of preferences and available income. The demand for each product is determined by a stochastic process, incorporating the uncertainty in consumer preferences. By interpolating the data for the demands for various scenarios and types of consumers we derive poly-parametric demand functions. These demand functions are partially in tension with classical demand theory since on certain occasions they imply that the demand of a product increases as its price increases. Our proposed method of generating data from discrete agents with Monte Carlo and of interpolating the data with machine learning methods can be easily generalized and applied to the assessment of economic theories and to the derivation of economic laws in a bottom-up approach.
Citation: Georgios Alkis Tsiatsios, John Leventides, Evangelos Melas, Costas Poulios. A bounded rational agent-based model of consumer choice[J]. Data Science in Finance and Economics, 2023, 3(3): 305-323. doi: 10.3934/DSFE.2023018
[1] | Liqin Liu, Chunrui Zhang . A neural network model for goat gait. Mathematical Biosciences and Engineering, 2024, 21(8): 6898-6914. doi: 10.3934/mbe.2024302 |
[2] | Van Dong Nguyen, Dinh Quoc Vo, Van Tu Duong, Huy Hung Nguyen, Tan Tien Nguyen . Reinforcement learning-based optimization of locomotion controller using multiple coupled CPG oscillators for elongated undulating fin propulsion. Mathematical Biosciences and Engineering, 2022, 19(1): 738-758. doi: 10.3934/mbe.2022033 |
[3] | Jiacan Xu, Donglin Li, Peng Zhou, Chunsheng Li, Zinan Wang, Shenghao Tong . A multi-band centroid contrastive reconstruction fusion network for motor imagery electroencephalogram signal decoding. Mathematical Biosciences and Engineering, 2023, 20(12): 20624-20647. doi: 10.3934/mbe.2023912 |
[4] | Yong Yao . Dynamics of a delay turbidostat system with contois growth rate. Mathematical Biosciences and Engineering, 2019, 16(1): 56-77. doi: 10.3934/mbe.2019003 |
[5] | Changyong Xu, Qiang Li, Tonghua Zhang, Sanling Yuan . Stability and Hopf bifurcation for a delayed diffusive competition model with saturation effect. Mathematical Biosciences and Engineering, 2020, 17(6): 8037-8051. doi: 10.3934/mbe.2020407 |
[6] | Ranjit Kumar Upadhyay, Swati Mishra, Yueping Dong, Yasuhiro Takeuchi . Exploring the dynamics of a tritrophic food chain model with multiple gestation periods. Mathematical Biosciences and Engineering, 2019, 16(5): 4660-4691. doi: 10.3934/mbe.2019234 |
[7] | Qianqian Zheng, Jianwei Shen, Lingli Zhou, Linan Guan . Turing pattern induced by the directed ER network and delay. Mathematical Biosciences and Engineering, 2022, 19(12): 11854-11867. doi: 10.3934/mbe.2022553 |
[8] | Shunyi Li . Hopf bifurcation, stability switches and chaos in a prey-predator system with three stage structure and two time delays. Mathematical Biosciences and Engineering, 2019, 16(6): 6934-6961. doi: 10.3934/mbe.2019348 |
[9] | Jinhu Xu, Yicang Zhou . Bifurcation analysis of HIV-1 infection model with cell-to-cell transmission and immune response delay. Mathematical Biosciences and Engineering, 2016, 13(2): 343-367. doi: 10.3934/mbe.2015006 |
[10] | Ranjit Kumar Upadhyay, Swati Mishra . Population dynamic consequences of fearful prey in a spatiotemporal predator-prey system. Mathematical Biosciences and Engineering, 2019, 16(1): 338-372. doi: 10.3934/mbe.2019017 |
In a bottom-up approach, agent-based models have been extensively used in finance and economics in order to understand how macro-level phenomena can emerge from myriads of micro-level behaviours of individual agents. Moreover, in the absence of (big) data there is still the need to test economic theories and understand how macro-level laws can be materialized as the aggregate of a multitude of interactions of discrete agents. We exemplify how we can solve this problem in a particular instance: We introduce an agent-based method in order to generate data with Monte Carlo and then we interpolate the data with machine learning methods in order to derive multi-parametric demand functions. In particular, the model we construct is implemented in a simulated economy with 1000 consumers and two products, where each consumer is characterized by a unique set of preferences and available income. The demand for each product is determined by a stochastic process, incorporating the uncertainty in consumer preferences. By interpolating the data for the demands for various scenarios and types of consumers we derive poly-parametric demand functions. These demand functions are partially in tension with classical demand theory since on certain occasions they imply that the demand of a product increases as its price increases. Our proposed method of generating data from discrete agents with Monte Carlo and of interpolating the data with machine learning methods can be easily generalized and applied to the assessment of economic theories and to the derivation of economic laws in a bottom-up approach.
With the development of neuroscience, the controlling mechanism and mode of biological motion have been paid much attention by biologists [1,2,3,4], and rhythmic movement is a common mode of motion in biology. Rhythmic movement refers to periodic movement with symmetry of time and space, such as walking, running, jumping, flying, swimming and so on. Biologists have shown that rhythmic movement is not related to the consciousness of the brain, but to the self-excitation of the lower nerve centers. It is a spatiotemporal motion mode controlled by a central pattern generator located in the spinal cord of vertebrates or in the thoracic and abdominal ganglia of invertebrates [5]. They have the ability to automatically generate complex high dimensional control signals for the coordination of the muscles during rhythmic movements [6,7,8,9].
In engineering, CPG can be regarded as a distributed system consisting of a group of coupled nonlinear oscillators. The generation of rhythmic signals can be realized by phase coupling. Changing the coupling relationship of oscillators can produce spatiotemporal sequence signals with different phase relations, and realize different movement modes. CPG of animals lays a foundation for the research of bionic robots. For example, in [10,11] the gait control of quadruped robots based on CPG is studied. Mathematically, there are several common types of CPG oscillators systems, such as Hopf oscillators systems [12,13], Kimura oscillators systems, Rayleigh oscillators systems, Matsuoa oscillators systems and VDP oscillator systems [14,15], etc.
Quadrupedal gait is a kind of gait that people are very concerned. The gait of quadruped is an important type described by a symmetrical system [16,17,18]. For example, in [17,18], base on the symmetry property, the primary and secondary gait modes of quadruped are described, respectively. In animal gait movement, the legs are coupled with each other, and the coupling strength affects the complexity of animal gait. In this paper, the delay of leg signal is considered according to CPG model, the basic gait CPG model of a class of quadruped is constructed by using VDP oscillators, and the ranges of coupling strength between legs under four basic gaits are given. This paper is organized as follows. Firstly, a kind of delay CPG network system is constructed by using VDP oscillator. Secondly, the conditions of Hopf bifurcation in VDP-CPG network corresponding to the four basic gaits are given, and the coupling ranges between legs in four basic gaits are given. Finally, the theoretical results are supported by numerical simulations.
CPG, as the control center of rhythmic motion, is a kind of neural network that can generate the output of rhythmic mode without sensor feedback. It sends out motion instructions from the high-level center to control the initial state of rhythmic motion, and integrates the feedback information and perception information of CPG to regulate the motion organically. The CPG network in this paper adopts the following network structure [14].
In Figure 1, LF, RF, LH and RH represent the animal's left foreleg, right foreleg, left hind leg and right hind leg, respectively. The black arrows represent the leg raising sequence, and the numbers in the circles are the phase difference between other legs and LF leg. In order to generate the rhythmic signal of each leg, the VDP oscillator used in this paper can refer to [14], the equation is as follows.
{˙x=y,˙y=α(p2−x2)˙x−w2x, |
where x is the output signal from oscillator, α, p and w are variable parameters which can influence the character of oscillators. Commonly, the shape of the wave is affected by parameter α, and the amplitude of an output counts on the parameter p mostly. The output frequency is mainly relying on the parameter w when the amplitude parameter p is fixed. But the alteration of parameter p can lightly change the frequency of the signal, and α also can effect the output frequency.
Four-legged muscle groups are regarded as VDP oscillators for feedback motion signals, respectively. The animal's left foreleg, right foreleg, right hind leg and left hind leg are recorded as oscillator x1,x2,x3 and x4, respectively.
Then the oscillator of the ith leg is as follows
{˙xi=yi,˙yi=αi(p2i−x2ki)yi−w2ixki,i=1,2,3,4, |
where xki=xi+4∑j=1,j≠iKijxj denotes the coupling variable. Here Kij is the coupling coefficient, which represents strength of coupling from j oscillator to i oscillator.
Because the motion state of each leg depends on the motion state of the other three legs in the past short time, the time delay is introduced as follows
xki=xi(t)+4∑j=1,j≠iKijxj(t−τ). |
Assuming that the biological mechanism of each leg is similar and the degree of excitation or inhibition is the same between legs, and the excitation is positive coupling, then the inhibition is negative coupling. Therefore,
α1=α2=α3=α4=α, |
p1=p2=p3=p4=p, |
w1=w2=w3=w4=w, |
Kij={K,whenthejlegexcitestheileg,−K,whenthejlegrestrainstheileg.K>0. |
Thus, we study the following VDP-CPG system
{˙xi=yi,˙yi=α(p2−(xi(t)+4∑j=1,j≠iKijxj(t−τ))2)yi−w2(xi(t)+4∑j=1,j≠iKijxj(t−τ)), | (1) |
where i=1,2,3,4. It is clear that the origin (0, 0, 0, 0, 0, 0, 0, 0) is an equilibrium of Eq (1).
In this section, we construct a VDP-CPG network which is used for generation four basic gaits patterns (walk, trot, pace and bound). Then we analyze the conditions for four gait systems to produce Hopf bifurcation.
In order to analyses the four basic gaits, we make the following assumptions.
(H1) h<0,
(H2) 2s−h2>0,19m<K2<19,
(H3) K2<m,
where h=αp2,s=w2,m=4h2s−h44s2.
In walking gait, one leg is inhibited by the other three legs, then there are
Kij=−K,i,j=1,2,3,4,i≠j. |
So the VDP-CPG network in walking gait is as follows
{˙xi=yi,˙yi=α(p2−(xi(t)+4∑j=1,j≠i(−K)xj(t−τ))2)yi−w2(xi(t)+4∑j=1,j≠i(−K)xj(t−τ)). | (2) |
This is a symmetric system. We first explore the symmetry of system (2), then study the existence of Hopf bifurcation of system (2).
Let Yi=(xiyi)∈R2,i=1,2,3,4, system (2) can be written in block form as follows
˙Yi=MYi(t)+NYi+1(t−τ)+NYi+2(t−τ)+NYi+3(t−τ)+g(Yi(t)),i=1,2,3,4(mod4), | (3) |
where
M=(01−w2αp2),N=(00Kw20), |
g(xiyi)=(0−α(xi−Kxi+1(t−τ)−Kxi+2(t−τ)−Kxi+3(t−τ))2yi). |
Let Γ be a compact Lie group. It follows from [19], system ˙u(t)=G(ut) is said to be Γ− equivariant if G(γut)=γG(ut) for all γ∈Γ. Let Γ=D4 be the dihedral group of order 8, which is generated by the cyclic group Z4 of order 4 together with the flip of order 2. Denote by ρ the generator of the cyclic subgroup Z4 and k the flip. Define the action of D4 on R8 by
(ρU)i=Ui+1,(kU)i=U6−i,Ui∈R2,i=1,2,3,4(mod4). |
Then it is easy to get the following lemma.
Lemma 3.1. System (3) is D4− equivariant.
The linearization of Eq (3) at the origin is
˙Yi=MYi(t)+NYi+1(t−τ)+NYi+2(t−τ)+NYi+3(t−τ),i=1,2,3,4(mod4). | (4) |
The characteristic matrix of Eq (4) is given by
A(τ,λ)=(λI2−M−Ne−λτ−Ne−λτ−Ne−λτ−Ne−λτλI2−M−Ne−λτ−Ne−λτ−Ne−λτ−Ne−λτλI2−M−Ne−λτ−Ne−λτ−Ne−λτ−Ne−λτλI2−M), |
where I2 is a 2×2 identity matrix. This is a block circulant matrix, from [20], we have
det(A(τ,λ))=3∏j=0det(λI2−M−χjNe−λτ−(χj)2Ne−λτ−(χj)3Ne−λτ), |
where χj=eπj2i,i is the imaginary unit. The characteristic equation of Eq (4) at the zero solution is
Δ(τ,λ)=det(A(τ,λ))=Δ1(Δ2)3, | (5) |
with
Δ1=λ(λ−h)+s(1−3Ke−λτ)),Δ2=λ(λ−h)+s(1+Ke−λτ),h=αp2,s=w2. |
Lemma 3.2. If (H1) and (H2) hold, for the equation Δ1=0, we have the following results.
(1) when τ=0, all roots of equation Δ1=0 have negative real parts,
(2) when τ>0, there exist τj, such that when τ=τj(j=0,1,2,…), Δ1(±iβ)=0 holds,
(3) the transversality condition:
Re(dλdτ)|λ=iβ+,τ=τjwalk+>0,Re(dλdτ)|λ=iβ−,τ=τjwalk−<0, |
where
β=β±=√2s−h2±√(h2−2s)2−4s2(1−9K2)2, |
τj=τjwalk±=1β±(−arccoss−β2±3Ks+2jπ+2π),j=0,1,2,…. |
Proof. (1) When τ=0, equation Δ1=0 becomes λ(λ−h)+s(1−3K)=0, and the solution is obtained as follows
λ=h±√h2−4s(1−3K)2. |
By (H1) and (H2), the roots of equation Δ1=0 have negative real parts.
(2) When τ>0, let λ=iβ(β>0) be a root of Δ1=0. Substituting iβ into Δ1=0, then we have
−β2−iβh+s(1−3Ke−iβτ)=0. |
Separating the real and imaginary parts, we get the following form
{s−β2=3Kscos(βτ),βh=3Kssin(βτ). | (6) |
If (H2) holds, by solving the above equation, we have
β±=β=√2s−h2±√(h2−2s)2−4s2(1−9K2)2, | (7) |
τjwalk±=τj=1β(−arccoss−β23Ks+2jπ+2π),j=0,1,2,…. |
(3) Let λ(τ)=α(τ)+iβ(τ) be the root of equation Δ1=0, satisfying α(τj)=0 and β(τj)=β. Taking the derivative of the equation Δ1=0 with respect to τ, we can get
dλdτ=−3Ksλeλτ2λ−h+3Ksτeλτ. |
Then
Re(dλdτ)|λ=iβ,τ=τj=3Ksβhsin(βτj)−6Ksβ2cos(βτj)(−h+3Ksτcos(βτj))2+(2β−3Ksτjsin(βτj))2, |
by (6) and (7), we have
Re(dλdτ)|λ=iβ+,τ=τjwalk+>0,Re(dλdτ)|λ=iβ−,τ=τjwalk−<0, |
which means that the transversality condition holds at τjwalk±,j=0,1,2,….
The lemma 3.2 holds.
Lemma 3.3. For Δ2=0, we have the following results.
(1) if (H1) holds, when τ=0 all roots of equation Δ2=0 have negative real parts,
(2) if (H3) holds, when τ>0 equation Δ2=0 has no pure imaginary root.
Proof. (1) When τ=0, equation Δ2=0 becomes λ(λ−h)+s(1+K)=0, and the solution is obtained as follows
λ=h±√h2−4s(1+K)2. |
By (H1), the roots of equation Δ2=0 have negative real parts.
(2) When τ>0, let λ=iβ(β>0) be a root of Δ2=0. Substituting iβ into Δ2=0 then we have
−β2−iβh+s(1+Ke−iβτ)=0. |
The real and imaginary parts of the above equation are separated, then we obtain
{s−β2=−Kscos(βτ),βh=−Kssin(βτ). |
By solving the above equation, we have
β=√2s−h2±√(h2−2s)2−4s2(1−K2)2. |
By (H3), we obtain (h2−2s)2−4s2(1−K2)<0, then the formula above is not valid. So the lemma 3.3 holds.
From lemma 3.2 and 3.3, we have following theorem.
Theorem 3.1. If (H1), (H2) and (H3) hold, then we have the following results.
(1) all roots of Eq (5) have negative real parts for 0≤τ<τ0walk, and at least a pair of roots with positive real parts for τ∈(τ0walk,τ0walk+ε), for some ε>0,
(2) zero equilibrium of system (2) is asymptotically stable for 0≤τ<τ0walk, and unstable for τ∈(τ0walk,τ0walk+ε), for some ε>0,
(3) when τ=τ0walk, system (2) undergoes a Hopf bifurcation at zero equilibrium, where τ0walk=min{τ0walk+,τ0walk−}.
Remark 3.1. Near the critical value τ=τ0walk, the periodic solution of system (2) at the origin accords with walking gait.
In a trot, a leg on the same diagonal as the current leg stimulates the current leg, and two legs on the other diagonal suppress the current leg, thus
K12=−K,K13=K,K14=−K,K21=−K,K23=−K,K24=K,K31=K,K32=−K,K34=−K,K41=−K,K42=K,K43=−K. |
The VDP-CPG network for trotting is as follows.
{˙xi=yi,˙yi=αp2yi−w2(xi(t)+(−K)xi+1(t−τ)+Kxi+2(t−τ)+(−K)xi+3(t−τ))−α(xi(t)+(−K)xi+1(t−τ)+Kxi+2(t−τ)+(−K)xi+3(t−τ))2yi. | (8) |
This is also a symmetric system. Similarly, by lemma 3.1, we have
Lemma 3.4. System (8) is D4− equivariant.
The characteristic matrix of linearization of Eq (8) is given by
A1(τ,λ)=(λI2−M−Ne−λτNe−λτ−Ne−λτ−Ne−λτλI2−M−Ne−λτNe−λτNe−λτ−Ne−λτλI2−M−Ne−λτ−Ne−λτNe−λτ−Ne−λτλI2−M). |
This is a block circulant matrix, and we have
det(A1(τ,λ))=3∏j=0det(λI2−M−χjNe−λτ+(χj)2Ne−λτ−(χj)3Ne−λτ), |
with χj=eπj2i.
The characteristic equation of linearization of Eq (8) at zero solution is
Δ(τ,λ)=det(A1(τ,λ))=Δ3(Δ4)3, | (9) |
where
Δ3=λ(λ−h)+s(1+3Ke−λτ), |
Δ4=λ(λ−h)+s(1−Ke−λτ). |
Similarly, by lemma 3.2 and 3.3, we have following lemmas.
Lemma 3.5. For the equation Δ3=0, we have the following results.
(1) if (H1) holds, when τ=0, all roots of equation Δ3=0 have negative real parts,
(2) if (H2) holds, when τ>0, there exist τj, such that when τ=τj(j=0,1,2,…), Δ3(±iβ)=0 holds,
(3) the transversality condition:
Re(dλdτ)|λ=iβ+,τ=τjtrot+>0,Re(dλdτ)|λ=iβ−,τ=τjtrot−<0, |
where
β=β±=√2s−h2±√(h2−2s)2−4s2(1−9K2)2, |
τj=τjtrot±=1β±(arccoss−β2±−3Ks+2jπ),j=0,1,2,… |
Lemma 3.6. For Δ4=0, we have the following results.
(1) if (H1)and K<1 hold, when τ=0, all roots of equation Δ4=0 have negative real parts,
(2) if (H3) holds, when τ>0, equation Δ4=0 has no pure imaginary root.
From lemma 3.5 and 3.6, we have following theorem.
Theorem 3.2. If (H1), (H2) and (H3) hold, we have the following results.
(1) all roots of Eq (9) have negative real parts for 0≤τ<τ0trot, and at least a pair of roots with positive real parts for τ∈(τ0trot,τ0trot+ε), for some ε>0,
(2) zero equilibrium of Eq (8) is asymptotically stable for 0≤τ<τ0trot, and unstable for τ∈(τ0trot,τ0trot+ε), for some ε>0,
(3) when τ=τ0trot, system (8) undergoes a Hopf bifurcation at zero equilibrium, where τ0trot=min{τ0trot+,τ0trot−}
Remark 3.2. Near the critical value τ=τ0trot, the periodic solution of system (8) at the origin accords with trotting gait.
In a pace, the leg on the same side (left or right) of the current leg stimulates the current leg, and the other two legs inhibit the current leg, thus
K12=−K,K13=−K,K14=K,K21=−K,K23=K,K24=−K,K31=−K,K32=K,K34=−K,K41=K,K42=−K,K43=−K. |
Thus Eq (1) becomes the following VDP-CPG pacing system.
{˙xi=yi,˙yi=α(p2−(xi(t)+(−K)xi+1(t−τ)+(−K)xi+2(t−τ)+Kxi+3(t−τ))2)yi−w2(xi(t)+(−K)xi+1(t−τ)+(−K)xi+2(t−τ)+Kxi+3(t−τ)),i=1,3(mod4) | (10) |
{˙xi=yi,˙yi=α(p2−(xi(t)+(−K)xi−1(t−τ)+Kxi+1(t−τ)+(−K)xi+2(t−τ))2)yi−w2(xi(t)+(−K)xi−1(t−τ)+Kxi+1(t−τ)+(−K)xi+2(t−τ)),i=2,4(mod4) |
and the linearization of Eq (10) at the origin is
{˙xi=yi,˙yi=αp2yi−w2(xi(t)+(−K)xi+1(t−τ)+(−K)xi+2(t−τ)+Kxi+3(t−τ))i=1,3(mod4) | (11) |
{˙xi=yi,˙yi=αp2yi−w2(xi(t)+(−K)xi−1(t−τ)+Kxi+1(t−τ)+(−K)xi+2(t−τ)),i=2,4(mod4) |
the characteristic equation of system (11) is
|Rm−m−m+m−Rm+m−m−m+Rm−m+m−m−R|=Δ5(Δ6)3=0, | (12) |
where
Δ5=λ(λ−h)+s(1+3Ke−λτ),Δ6=λ(λ−h)+s(1−Ke−λτ). |
R=(λ−1w2λ−αp2),m+=(00Kw2e−λτ0),m−=(00−Kw2e−λτ0), |
Similarly, by theorem 3.1, we have following theorem.
Theorem 3.3. If (H1), (H2) and (H3) hold, we have the following results.
(1) all roots of Eq (12) have negative real parts for 0≤τ<τ0pace, and at least a pair of roots with positive real parts for τ∈(τ0pace,τ0pace+ε), for some ε>0,
(2) zero equilibrium of system (10) is asymptotically stable for 0≤τ<τ0pace, and unstable for τ∈(τ0pace,τ0pace+ε), for some ε>0,
(3) when τ=τ0pace, system (10) undergoes a Hopf bifurcation at zero equilibrium,
where
τ0pace=min{τ0pace+,τ0pace−}, |
τjpace±=1β±(arccoss−β2±−3Ks+2jπ),j=0,1,2,…, |
β±=√2s−h2±√(h2−2s)2−4s2(1−9K2)2. |
Remark 3.3. Near the critical value τ=τ0pace, the periodic solution of system (10) at the origin accords with pacing gait.
In a bound, legs on the same side (front or hind) as the current leg stimulate the current leg, and the other two legs inhibit the current leg, thus
K12=K,K13=−K,K14=−K,K21=K,K23=−K,K24=−K, |
K31=−K,K32=−K,K34=K,K41=−K,K42=−K,K43=K. |
Eq (1) becomes the following bounding VDP-CPG system.
{˙xi=yi,˙yi=α(p2−(xi(t)+Kxi+1(t−τ)+(−K)xi+2(t−τ)+(−K)xi+3(t−τ))2)yi−w2(xi(t)+Kxi+1(t−τ)+(−K)xi+2(t−τ)+(−K)xi+3(t−τ)),i=1,3(mod4) | (13) |
{˙xi=yi,˙yi=α(p2−(xi(t)+Kxi−1(t−τ)+(−K)xi+1(t−τ)+(−K)xi+2(t−τ))2)yi−w2(xi(t)+Kxi−1(t−τ)+(−K)xi+1(t−τ)+(−K)xi+2(t−τ)),i=2,4(mod4) |
and the linearization of Eq (13) at the origin is
{˙xi=yi,˙yi=αp2yi−w2(xi(t)+Kxi+1(t−τ)+(−K)xi+2(t−τ)+(−K)xi+3(t−τ)),i=1,3(mod4) | (14) |
{˙xi=yi,˙yi=αp2yi−w2(xi(t)+Kxi−1(t−τ)+(−K)xi+1(t−τ)+(−K)xi+2(t−τ)),i=2,4(mod4) |
the characteristic equation of system (14) is
|Rm+m−m−m+Rm−m−m−m−Rm+m−m−m+R|=Δ7(Δ8)3=0, | (15) |
where
Δ7=λ(λ−h)+s(1+3Ke−λτ),Δ8=λ(λ−h)+s(1−Ke−λτ). |
Similarly, by theorem 3.1, we have following theorem.
Theorem 3.4. If (H1), (H2) and (H3) hold, we have the following results.
(1) all roots of Eq (15) have negative real parts for 0≤τ<τ0bound, and at least a pair of roots with positive real parts for τ∈(τ0bound,τ0bound+ε), for some ε>0,
(2) zero equilibrium of system (13) is asymptotically stable for 0≤τ<τ0bound, and unstable for τ∈(τ0bound,τ0bound+ε), for some ε>0,
(3) when τ=τ0bound, system (13) undergoes a Hopf bifurcation at zero equilibrium,
where
τ0bound=min{τ0bound+,τ0bound−}, |
τjbound±=1β±(arccoss−β2±−3Ks+2jπ),j=0,1,2,…, |
β±=√2s−h2±√(h2−2s)2−4s2(1−9K2)2. |
Remark 3.4. Near the critical value τ=τ0bound , the periodic solution of system (13) at the origin accords with bounding gait.
In this section, the numerical simulation of model is carried out to verify the results obtained in the previous sections. Let α=−1.5, p=1,w=4, K=0.3, according to the calculation, we obtain the h=−1.5,s=16,m=0.1357,K2=0.09,19m=0.0151. Thus 2s−h2=29.7500>0,19m<K2<min{m,19} and the critical value τ0walk=0.7039, τ0trot=τ0pace=τ0bound=0.1103 are obtained. Basing on Theorem 3.2, we know the zero equilibrium is asymptotically stable when τ<τ0trot (shown in Figure 2a), when τ>τ0trot, the zero equilibrium of system (8) is unstable, and the periodic solution corresponding to the trot gait occurs (see Figure 2b). From theorem 3.3, we know the zero equilibrium is asymptotically stable when τ<τ0pace (shown in Figure 3a), when τ>τ0pace, the zero equilibrium of system (10) is unstable, and the periodic solution corresponding to the pace gait occurs (see Figure 3b). From theorem 3.4, we know the zero equilibrium is asymptotically stable when τ<τ0bound (shown in Figure 4a), when τ>τ0bound, the zero equilibrium of system (13) is unstable, and the periodic solution corresponding to the bound gait occurs (see Figure 4b).
In this paper, a kind of CPG network system is constructed by using VDP oscillators, and a VDP-CPG network system with four basic gaits (walk, trot, pace and bound) is presented. By studying the corresponding characteristic equations of four gaits systems, it is found that the conditions for the periodic solutions of four gaits systems are h<0,2s−h2>0 and 19m<K2<min{m,19} and the critical values τjwalk,τjtrot,τjpace andτjbound,j=0,1,2⋯. Thus, the range of coupling strength between legs in four gaits is 19m<K2<min{m,19}. Finally, the numerical simulations show that the gait systems (trot, pace and bound) produce corresponding gaits near the corresponding critical value.
This research is supported by the Fundamental Research Funds for the Central Universities (No.2572019BC12). The authors wish to express their gratitude to the editors and the reviewers for the helpful comments.
The authors declare there is no conflict of interest
[1] |
Akerlof GA (2002) Behavioral macroeconomics and macroeconomic behavior. Am Econ Rev 92: 411–433. https://doi.org/10.1257/00028280260136192 doi: 10.1257/00028280260136192
![]() |
[2] | Anderson TW, Goodman LA (1957) Statistical inference about Markov chains. Ann Math Stat 28: 89–110. |
[3] | Arrow KJ (1971) Essays in the Theory of Risk-Bearing. Markham Pub Co |
[4] |
Assenza T, Delli Gatti D, Grazzini J (2015) Emergent dynamics of a macroeconomic agent-based model with capital and credit. J Econ Dyn Control 50: 5–28. https://doi.org/10.1016/j.jedc.2014.07.001 doi: 10.1016/j.jedc.2014.07.001
![]() |
[5] | Axtell RL, Farmer JD (2022) Agent-Based Modeling in Economics and Finance: Past, Present, and Future. INET Oxford Working Paper No. 2002-10. 21st June 2022. |
[6] |
Barbu VS, D'Amico G, De Blasis R (2017) Novel advancements in the Markov chain stock model: Analysis and inference. Ann Financ 13: 125–152. https://doi.org/10.1007/s10436-017-0297-9 doi: 10.1007/s10436-017-0297-9
![]() |
[7] |
Battiston S, Farmer JD, Flache A, et al. (2016) Complexity theory and financial regulation. Science 351: 818–819. https://doi.org/10.1126/science.aad0299 doi: 10.1126/science.aad0299
![]() |
[8] | Billingsley P (1961) Statistical methods in Markov chains. Ann Math Stat 32: 12–40. |
[9] |
Bonabeau (2002) Agent-based modeling: Methods and techniques for simulating human systems. P Natl Acad Sci 99: 7280–7287. https://doi.org/10.1073/pnas.0820808 doi: 10.1073/pnas.0820808
![]() |
[10] |
Bookstaber R (2017) Agent-Based Models for Financial Crises. Ann Rev Financ Econ 9: 85–100. https://doi.org/10.1146/annurev-financial-110716-032556 doi: 10.1146/annurev-financial-110716-032556
![]() |
[11] | Buchanan M (2009) Meltdown modelling: could agent-based computer models prevent another financial crisis? Nature 460: 680–683. |
[12] |
Delli G, Gaffeo E, Gallegati M (2010) Complex agent-based macroeconomics: a manifesto for a new paradigm. J Econ Interact Coor 5: 111–135. https://doi.org/10.1007/s11403-010-0064-8 doi: 10.1007/s11403-010-0064-8
![]() |
[13] | Ehrenberg AS (1988) Repeat-Buying: Facts, Theory and Applications. Oxford University Press. |
[14] | Epstein JM (2006) Generative social science: Studies in agent-based computational modeling. Princeton University Press. |
[15] |
Farmer JD, Foley D (2009) The economy needs agent–based modelling. Nature 460: 685–686. https://doi.org/10.1038/460685a doi: 10.1038/460685a
![]() |
[16] |
Gary JG, Irwin PL, Goutam C, et al. (1991) Consumer evaluation of multi-product bundles: An information integration analysis. Market Lett 2: 47–57. https://doi.org/10.1007/BF00435195 doi: 10.1007/BF00435195
![]() |
[17] |
Grazzini J, Richiardi MG (2015) Estimation of ergodic agent-based models by simulated minimum distance. J Econ Dyn Control 51: 148–165. https://doi.org/10.1016/j.jedc.2014.10.006 doi: 10.1016/j.jedc.2014.10.006
![]() |
[18] | Hamill L, Gilbert N (2015) Agent–based modelling in economics. John Wiley & Sons. |
[19] | Kahneman D (2003) Maps of Bounded Rationality: Psychology for Behavioral Economics. Am Econ Rev 93: 1449–1475. |
[20] |
Kaplow L (2008) Optimal policy with heterogeneous preferences. BE J Econ Anal Policy 8. https://doi.org/10.2202/1935-1682.1947 doi: 10.2202/1935-1682.1947
![]() |
[21] | Leijonhufvud A (1996) Towards a not-too-rational macroeconomics, In: Colander, D. (Ed.), Beyond Microfoundations: Post Walrasian Macroeconomics. Cambridge University Press, Cambridge, MA., 39–55. |
[22] | Macal CM, North MJ (2005) Tutorial on agent−based modeling and simulation. Proceedings of the Winter Simulation Conference 14, IEEE. |
[23] |
Maravilha D, Silva S, Laranjeira E (2022) Consumer's behavior determinants after the electricity market liberalization: the Portuguese case. Green Financ 4: 436–449. https://doi.org/10.3934/GF.2022021 doi: 10.3934/GF.2022021
![]() |
[24] |
Mandel A, Landini S, Gallegati M, et al. (2015) Price dynamics, financial fragility and aggregate volatility. J Econ Dyn Control 51: 257–277. https://doi.org/10.1016/j.jedc.2014.11.001 doi: 10.1016/j.jedc.2014.11.001
![]() |
[25] |
Manout O, Ciari F (2021) Assessing the Role of Daily Activities and Mobility in the Spread of COVID-19 in Montreal with an Agent-Based Approach. Front Built Enviroment 7: 2021. https://doi.org/10.3389/fbuil.2021.654279 doi: 10.3389/fbuil.2021.654279
![]() |
[26] | McFadden D (1974) Conditional Logit Analysis of Qualitative Choice Behavior. Front Econometrics Zarembka (Ed.) 105–142. New York: Academic Press. |
[27] |
Mullainathan S, Spiess J (2017) Machine Learning: An Applied Econometric Approach. J Econ Perspect 31: 87–106. https://doi.org/10.1257/jep.31.2.87 doi: 10.1257/jep.31.2.87
![]() |
[28] |
Peña G (2020) A new trading algorithm with financial applications. Quant Financ Econ 4: 596–607. https://doi.org/10.3934/QFE.2020027 doi: 10.3934/QFE.2020027
![]() |
[29] |
Predelus W, Amine S (2022) The insolvency choice during an economic crisis: the case of Canada. Quant Financ Econ 6: 658–668. https://doi.org/10.3934/QFE.2022029 doi: 10.3934/QFE.2022029
![]() |
[30] |
Reisch LA, Zhao M (2017) Behavioural economics, consumer behaviour and consumer policy: state of the art. Behav Public Policy 1: 190–206. https://doi.org/10.1017/bpp.2017.1 doi: 10.1017/bpp.2017.1
![]() |
[31] |
Seetharaman PB (2004) Modeling multiple sources of state dependence in random utility models: A distributed lag approach. Market Sci 23: 263–271. https://doi.org/10.1287/mksc.1030.0024 doi: 10.1287/mksc.1030.0024
![]() |
[32] | Simon HA (1955) A Behavioral Model of Rational Choice. Q J Econ 69: 99–118. |
[33] | Simudyne (2023) A Complete-Guide to Agent-Based Modeling for Financial Services. Simudyne 2023. |
[34] |
Tesfatsion L (2006) Agent-Based Computational Economics: A Constructive Approach to Economic Theory. Handb Computat Econ 2: 831–880. https://doi.org/10.1016/S1574-0021(05)02016-2 doi: 10.1016/S1574-0021(05)02016-2
![]() |
[35] | Tesfatsion L, Judd KL (2006) Handbook of computational economics: agent-based computational economics. Elsevier. |
[36] | Thaler RH (2015) Misbehaving: The Making of Behavioral Economics. W. W. Norton & Company. |
[37] | Thurner S, Farmer JD, Geanakoplos J (2012) Using agent-based simulations, this work discusses the role of leverage in financial markets, explaining phenomena like fat-tailed asset returns. Quant Financ 12: 695–707. |
[38] | Tsiatsios GA, Kollias I, Melas E, et al. (2022) Some first results from an agent–based model of consumer demand. submitted. |
[39] | Tsiatsios GA, Leventides I, Melas E, et al. (2023a) A bounded rational agent-based model of consumer choice. submitted. |
[40] | Tsiatsios GA, Leventides I, Toudas K, et al. (2023b) An agent–based study on the dynamic distribution and firms concentration in a closed economy. submitted. |
[41] | Turell A (2016) Agent-based models: Understanding the economy from the bottom up. Bank England Quart Bull 2016. |
[42] | Varian HR (2014) Intermediate Microeconomics: A Modern Approach. W. W. Norton & Company. |
[43] |
Zhong Li Z (2020) Impact of economic policy uncertainty shocks on China's financial conditions. Financ Res Lett 35: 101303. https://doi.org/10.1016/j.frl.2019.101303 doi: 10.1016/j.frl.2019.101303
![]() |
1. | Liqin Liu, Xiaoxiao Liu, Chunrui Zhang, REALIZATION OF NEURAL NETWORK FOR GAIT CHARACTERIZATION OF QUADRUPED LOCOMOTION, 2022, 12, 2156-907X, 455, 10.11948/20210005 | |
2. | Mingfang Chen, Kangkang Hu, Yongxia Zhang, Fengping Qi, Motion coordination control of planar 5R parallel quadruped robot based on SCPL-CPG, 2022, 14, 1687-8140, 168781402110709, 10.1177/16878140211070910 | |
3. | Zigen Song, Jiayi Zhu, Jian Xu, Gaits generation of quadruped locomotion for the CPG controller by the delay-coupled VDP oscillators, 2023, 111, 0924-090X, 18461, 10.1007/s11071-023-08783-2 | |
4. | Yangyang Han, Guoping Liu, Zhenyu Lu, Huaizhi Zong, Junhui Zhang, Feifei Zhong, Liyu Gao, A stability locomotion-control strategy for quadruped robots with center-of-mass dynamic planning, 2023, 24, 1673-565X, 516, 10.1631/jzus.A2200310 | |
5. | Zigen Song, Fengchao Ji, Jian Xu, Is there a user-friendly building unit to replicate rhythmic patterns of CPG systems? Synchrony transition and application of the delayed bursting-HCO model, 2024, 182, 09600779, 114820, 10.1016/j.chaos.2024.114820 | |
6. | Liqin Liu, Chunrui Zhang, A neural network model for goat gait, 2024, 21, 1551-0018, 6898, 10.3934/mbe.2024302 |