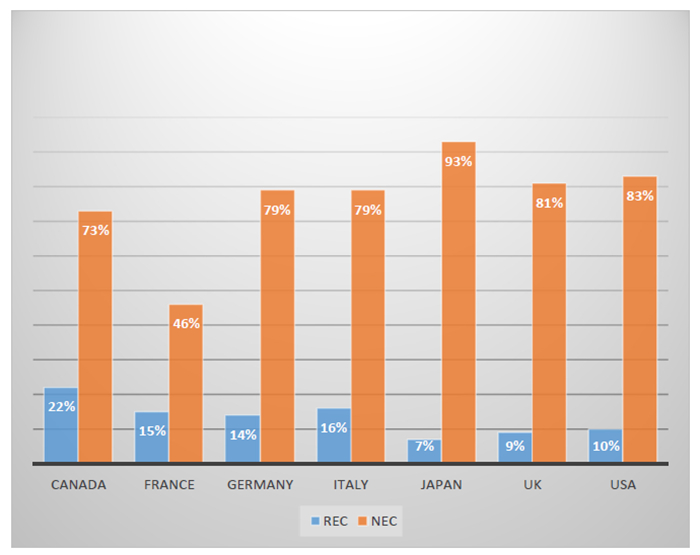
Many developed countries including G-7 became part of the Kyoto Protocol for improving their environmental quality in 2005. Its main goals were to develop national-level programs for energy conservation and the eradication of greenhouse gas emissions. To achieve such goals, certain policy measures including reduction in deforestation, urban population and promotion of renewable energy consumption were adopted. This study aims to examine the consequences of these policies on the environmental quality of G-7 from 1988 to 2018. LLC and IPS unit root tests were applied to check the stationarity of analyzed variables. The results of Pedroni and Kao's co-integration tests proved the long-run relationship between the dependent and explanatory variables. The application of multiple cross-sectional dependence tests revealed that the cross-sections are independent of each other. The findings of the panel Autoregressive distributed lag (ARDL) model exhibited that urbanization, economic growth and nonrenewable energy consumption are hampering the environmental quality. While renewable energy consumption and globalization are improving it in the long term. Urbanization, renewable and nonrenewable energy consumption significantly improve the environmental quality during the short term whereas globalization insignificantly deteriorates the environment. The study confirms the presence of reversed U environmental Kuznets curve between urbanization and carbon emissions with a turning point at 80 per cent of urbanization. The study suggests that the transformation of energy consumption from nonrenewable to renewable sources and strict compliance with environment management policies will prove prolific for improving the environmental quality of G-7.
Citation: Laila Khalid, Imran Hanif, Farhat Rasul. How are urbanization, energy consumption and globalization influencing the environmental quality of the G-7?[J]. Green Finance, 2022, 4(2): 231-252. doi: 10.3934/GF.2022011
[1] | Mihaela Onofrei, Bogdan Narcis Fîrțescu, Florin Oprea, Dana Claudia Cojocaru . The effects of environmental patents on renewable energy consumption. Green Finance, 2024, 6(4): 630-648. doi: 10.3934/GF.2024024 |
[2] | Paul Adjei Kwakwa, Frank Adusah-Poku . Determinants of electricity consumption and energy intensity in South Africa. Green Finance, 2019, 1(4): 387-404. doi: 10.3934/GF.2019.4.387 |
[3] | Yoram Krozer . Financing of the global shift to renewable energy and energy efficiency. Green Finance, 2019, 1(3): 264-278. doi: 10.3934/GF.2019.3.264 |
[4] | Xueying Xu, Peng Hou, Yue Liu . The impact of heterogeneous environmental regulations on the technology innovation of urban green energy: a study based on the panel threshold model. Green Finance, 2022, 4(1): 115-136. doi: 10.3934/GF.2022006 |
[5] | Clement Allan Tisdell . Renewable energy use and the renewable energy sector’s development: public finance, environmental externalities and sustainability. Green Finance, 2019, 1(2): 156-173. doi: 10.3934/GF.2019.2.156 |
[6] | Óscar Suárez-Fernández, José Manuel Maside-Sanfiz, Mª Celia López-Penabad, Mohammad Omar Alzghoul . Do diversity & inclusion of human capital affect ecoefficiency? Evidence for the energy sector. Green Finance, 2024, 6(3): 430-456. doi: 10.3934/GF.2024017 |
[7] | Jinhui Zhu, Zhehao Huang, Zhenghui Li, Khaldoon Albitar . The Impact of Urbanization on Energy Intensity — An Empirical Study on OECD Countries. Green Finance, 2021, 3(4): 508-526. doi: 10.3934/GF.2021024 |
[8] | Vitor Miguel Ribeiro . Pioneering paradigms: unraveling niche opportunities in green finance through bibliometric analysis of nation brands and brand culture. Green Finance, 2024, 6(2): 287-347. doi: 10.3934/GF.2024012 |
[9] | Manuel Padeira Navarro, Margarida Catalão-Lopes . A structured financial product applied to renewable energies. Green Finance, 2019, 1(1): 82-93. doi: 10.3934/GF.2019.1.82 |
[10] | Ali Omidkar, Razieh Es'haghian, Hua Song . Developing a machine learning model for fast economic optimization of solar power plants using the hybrid method of firefly and genetic algorithms, case study: optimizing solar thermal collector in Calgary, Alberta. Green Finance, 2024, 6(4): 698-727. doi: 10.3934/GF.2024027 |
Many developed countries including G-7 became part of the Kyoto Protocol for improving their environmental quality in 2005. Its main goals were to develop national-level programs for energy conservation and the eradication of greenhouse gas emissions. To achieve such goals, certain policy measures including reduction in deforestation, urban population and promotion of renewable energy consumption were adopted. This study aims to examine the consequences of these policies on the environmental quality of G-7 from 1988 to 2018. LLC and IPS unit root tests were applied to check the stationarity of analyzed variables. The results of Pedroni and Kao's co-integration tests proved the long-run relationship between the dependent and explanatory variables. The application of multiple cross-sectional dependence tests revealed that the cross-sections are independent of each other. The findings of the panel Autoregressive distributed lag (ARDL) model exhibited that urbanization, economic growth and nonrenewable energy consumption are hampering the environmental quality. While renewable energy consumption and globalization are improving it in the long term. Urbanization, renewable and nonrenewable energy consumption significantly improve the environmental quality during the short term whereas globalization insignificantly deteriorates the environment. The study confirms the presence of reversed U environmental Kuznets curve between urbanization and carbon emissions with a turning point at 80 per cent of urbanization. The study suggests that the transformation of energy consumption from nonrenewable to renewable sources and strict compliance with environment management policies will prove prolific for improving the environmental quality of G-7.
The concerns related to environmental quality have now become the centre of attention worldwide due to their serious and increasing impacts on planet life. It does not mean global warming only but unexpected weather changes, deforestation, shifts in flora and fauna, water pollution, and other factors are also part and parcel. All such happenings are attributed to human activities which raise the level of carbon dioxide and other heat trapped gases in the environment. The only way to improve the environmental quality lies in how the energy is produced and consumed. Energy use is the most essential factor for economic growth, however, it is also obligatory to make it harmless for the environment. On one side, the rapid increase in urban population and goals to achieve higher economic growth exert upward pressure on energy consumption which in turn spoils the environmental quality by adding carbon emissions. On the other side, increasing deforestation, lesser renewable energy use and more nonrenewable energy use are detrimental to the environmental quality. Moreover, due to intense globalization at all levels, it is the equal responsibility of the developed and developing countries to protect the environment.
There is extensive literature available over the last few decades describing energy-growth-environment linkage and can be grouped into three (Apergis and Ozturk, 2015; Behera and Dash, 2017). The first group of studies highlighted the role of economic growth in increasing or decreasing carbon emissions by inspecting the Kuznets curve1 hypothesis. The studies (Grossman and Krueger, 1991; Ozturk and Mulali, 2015; Dogan and Turkekul, 2016; Shahbaz et al., 2019) among others found the inverted U environmental Kuznets curve (EKC). However many studies including (Kaufmann et al., 1998; Diao, 1999) reported the existence of U shaped EKC. The next group related the energy use and environmental quality in the context of the IPAT or STIRPAT model. They established energy use and environmental quality linkage by using either the aggregate energy use or by decomposing the renewable energy use and nonrenewable energy use. The studies (Jebli et al., 2016; Dogan and Seker, 2016; Sharif et al., 2019) found that the use of renewable energy lessens emissions while nonrenewable energy worsens them. Apergis et al. (2010) found the contrary results with the same variables. So there is still ambiguity in the past literature and more research is required in this regard. The studies in the third category are a combination of the first two classifications and explained the energy-growth-environment linkage. Many other studies (Soytas and Sari, 2006; Sadorsky, 2009; Magazzino, 2014; Sharif et al., 2019; Raza et al., 2020) examined the association between growth-energy-environment by applying different techniques. But the majority of studies found varying empirical evidence thus raising the controversy in the existing literature. In this situation, it is impossible to recommend a uniform policy suited to all countries. Therefore, there is a need to further explore the nexus by using the countries that are working and spending huge amounts on renewable energy consumption to conserve the environment.
1Simon Kuznet (1955) developed this hypothesis to explain the causality between income and inequality. It reveals that an increase in income accompanies inequality and after a threshold level, this relationship turns to be negative.
Despite the efforts to reduce their emissions level, another reason for selecting the G-7 (Canada, France, Germany, Italy, Japan, the UK and the USA) is their global status and impact on the rest of the world. The International Energy Agency (IEA, 2019) report presents the usage of renewable and non-renewable energy in Canada (22%, 73%), France (15%, 46%), Germany (14%, 79%), Italy (16%, 79%), Japan (7%, 93%), The UK (9%, 81%) and the USA (10%, 83%) respectively in 2016 (Figure 1). Canada used more renewable energy than others due to its vast hydroelectric power generation system. The G-7 group being the world's largest exporter, nuclear energy producer and carbon dioxide emitter took important decisions to lower its carbon emissions under the Kyoto Protocol treaty of 2005. It was an extension of efforts started in 1992 by the United Nations Framework Convention on Climate Change (UNFCCC). The IEA, 2019 report reveals per capita carbon emissions in Canada (15 tons), France (5 tons), Germany (9 tons), Italy (5 tons), Japan (10 tons), the UK (7 tons) and the USA (16 tons) during 2018 (Fig.2). The G-7 contributes 26% of the world's economic output and 33% of global carbon emissions according to the World Bank report 2019.
The basic purpose of this study is to observe the effects of urbanization, economic growth, renewable and nonrenewable energy consumption and globalization on carbon emissions for G-7 using the STIRPAT2 model and EKC framework. The existing literature seems to be focused mostly on the panels like BRICS3 and European Union4 while G-7 is very limitedly considered for such kinds of investigations. Therefore this study selected a panel of G-7 to reveal the energy-growth-environment nexus. Many past studies used either aggregate energy or a single source of energy for exploring its contribution to carbon emissions and the individual role of renewable and nonrenewable energy sources at the same time is inadequately discussed. Thus the secondary purpose of this study is to break down the energy consumption into renewable and nonrenewable to obtain clarity about the effect of each source on emissions levels. In addition, the previous studies mostly used trade openness as a proxy for globalization to measure its effects on carbon emissions. Therefore the tertiary objective of this study is the use of the KOF index as a proxy for globalization to obtain ample insight into its impact on environmental quality.
2Stochastic impact for regression on population, affluence and technology. It is an ecological framework which incorporates three multiplicative components and their impact on the quality of the environment.
3Brazil, Russia, India, China, South Africa.
4Consists of 28 members of European countries like Austria, Belgium, Spain and Switzerland.
The further components of the study are as follows; a review of the literature is presented in section 2, while section 3 sheds light on data and methodology, section 4 comprises results and their discussion while section 5 displays the conclusion.
Previous literature discussed the significance of different determinants of environmental quality in developed and developing countries of the world. By considering the importance of major determinants of carbon emissions, some of the relevant past literature is summarized here.
Soytas and Sari (2006) examined the impact of economic growth and energy use on the environment in the G-7. The findings reported that energy conservation decreases growth in all economies except Germany. Narayan et al. (2007) employed dynamic OLS to analyze how variations in incomes and prices of electricity influence household usage and carbon emissions in G-7. The long-run analysis showed that electricity prices had a negative relationship with household energy use while income variations had no impact on electricity demand. Sadorsky (2009) found that economic growth is accompanied by more renewable energy use and CO2 emissions. However, a little decrease in renewable energy use is noticed due to the rise in oil prices. Magazzino (2014) confirmed the unidirectional relationship between CO2 emissions and energy use in the case of Italy from 1970 to 2006. He concluded that GDP growth and energy use harms the environment.
Mahalik and Mallick (2014) explored the energy- growth-urbanization nexus in India. The estimates of the ARDL revealed that urbanization increased energy use in the long-run. However, urbanization adversely affected economic growth but positively impacted energy use. Dogan and Turkekul (2016) found that economic growth and urbanization increase emissions while trade impedes emissions in the USA. Dogan and Seker (2016) and Sharif et al. (2019) selected twenty-three countries including G-6 from the renewable energy attractiveness index to analyze the impact of the main drivers of pollution. Their findings reveal that non-renewable energy use proves harmful to the environment whereas the use of wind and solar energy and trade prove good for the environment.
Zaman et al. (2016) conducted panel study of different sized economies for testing the EKC hypothesis. The findings of 2SLS revealed the existence of a linear relationship between income and carbon emissions up till the threshold level, then there exists a non-linear linkage. Furthermore, energy consumption and tourism are found responsible for hampering the environment. The findings of Nasreen et al. (2017) also confirmed the co-integration of GDP, urbanization and energy use with carbon emissions and the existence of EKC for South Asian countries. To test the N shaped EKC in the case of five EU economies, Lorente et al. (2017) considered economic growth, renewable energy consumption and innovations. The findings validated the existence of N shaped EKC. Renewable energy consumption and innovations were found to reduce carbon emissions. Duada et al. (2019) and Raza et al. (2020) also observed similar findings.
Behera and Dash (2017) studied the impact of urbanization and energy use on the environment of South Asian countries and found that energy consumption increased carbon emissions. However, the impact of urbanization varies among developed and developing economies. Khan et al. (2019) used globalization, urbanization and energy use to detect their effects on the environment of Pakistan. They found that energy consumption, globalization and urbanization significantly raise carbon emissions. Shahbaz et al. (2019) employed GMM on annual data for G-7 economies w.e.f 1980 to 2014 and revealed that globalization and urbanization impede environmental quality. Alkadiri et al. (2019) and Shahbaz et al. (2019) found that economic integration proved useful for advanced countries and has a prospect of reducing environmental degradation. However, a contrasting situation exists in developing nations. The studies revealed that globalization may positively, negatively, directly or indirectly impact CO2 emissions.
Zhang et al. (2018) examined the effect of land and population urbanization on carbon emissions in China for the period of 2005 to 2014. Using STIRPAT and the spatial econometric model, they found an insignificant impact of urbanization on carbon emissions. While urbanization significantly increased the carbon emissions spatially. On the contrary, Danish et al. (2019) explored that urbanization and renewable energy consumption reduced the carbon emissions and improved the environmental quality of BRICS from 1992 to 2016. They used dynamic OLS and fully modified OLS methods to validate the Kuznets curve hypothesis. Khalid et al. (2020) explored the significant and positive role of renewable energy on ecological footprints in all SAARC economies from the period 1990 to 2017. Therefore the policy of financial assistance from developed economies is suggested for preventing the SAARC countries from further climatic changes and to coop with an energy crisis.
By employing the panel data estimation strategy, Ulucak et al. (2021) also found that economic growth and conventional energy sources triggered environmental pollution in the Mediterranean region over the period 1995 to 2016. However, the urban sprawl and clean energy sources reduced carbon emissions. Therefore, it is suggested that urbanization should be properly planned and monitored to attain its benefits for the environment. Sahoo and Sethi (2021) investigated the impact of urbanization, population density, economic structure and innovations on the environmental quality of newly industrialized countries from 1990 to 2017. They used Mean Group and Pooled Mean Group techniques and found that urbanization, energy consumption and economic growth deteriorate the environmental quality. The study recommended the investments in the renewable energy sector to reduce environmental degradation.
The previous studies either focused on the association of growth and carbon emissions or energy use and carbon emissions in the presence of other variables. But the relationship between urbanization, economic growth, renewable energy consumption, non-renewable energy consumption, globalization and the effect of this relationship on carbon emissions are discussed very limitedly in the past literature. Many studies also found contradictory results using mentioned variables in the case of different or same countries. Moreover different econometric methods are used by different studies with different outcomes for the same indicators. So there is a need for more work to obtain clarity regarding the linkage of analyzed variables and to gauge the effectiveness of the specific sources of energy on the environment. Therefore to bridge this gap in existing literature, this study is empirically designed to reveal the relationship among underlying variables and their effects on the environmental quality of G-7. So it is the first time that these variables are used together in the case of a panel of G-7 using STIRPAT and EKC framework.
This study evaluates the impact of urbanization, economic growth, renewable energy consumption, nonrenewable energy consumption and globalization on the environmental quality of G-7 by using the STIRPAT model. The STIRPAT model allows for to add more variables in the base model along with squared terms to test the existence of EKC. (Dietz et al., 2007). The STIRPAT model specifications in the panel data scenario can be written as:
Iit=αPitβ1Aitβ2Titβ3ε | (1) |
Here, I reflect the impact on environmental quality, P stands for population size, A shows affluence, T displays technology, α and βs show constant and measurements of population, affluence and technology respectively while ɛ is an error term. The number of countries is reflected by i and t displays the time period. The STIRPAT model after insertion of additional variables is written as under:
EQi,t=αi,t+β1URBi,t+β2GDPi,t+β3RECi,t+β4NECi,t+β5GLOi,t+εi,t | (2) |
In Equation (2), EQ denotes environmental quality and this study uses per capita carbon dioxide emissions in metric tons to analyze it by pursuing (Dogan and Turkekul, 2016; Behera and Dash, 2017; Nasreen et al., 2017; Anser, 2019). The increase in carbon emissions means environmental quality is hurting while the decrease in carbon emissions reveals the improvement in environmental quality. Urban population (URB) as a percentage of the total population is used to examine its effects on the environmental quality by following (Mahalik and Mallick, 2014; Dogan and Turkekul, 2016; Anser et al., 2020). GDP depicts real gross domestic product per capita (2010, US$) which is also used by (Soytas and Sari, 2006; Mahalik et al., 2014; Nasreen et al., 2017; Raza et al., 2020). Renewable energy consumption (REC) and nonrenewable energy consumption (NEC) as a percentage of total energy consumption are utilized to test their impact on carbon emissions by following the footprints of (Dogan and Seker, 2016; Sharif et al., 2019). GLO shows globalization for which the KOF globalization index5 is used in this study. KOF index combines economic, social and political dimensions of globalization, similar indicator was used by (Shahbaz et al., 2019; Akadiri, 2019; Khan et al., 2019). Like (Anser et al., 2020) the present study also examines the presence of EKC between urbanization and carbon emissions for G-7. For this purpose, the square of urbanization is included in the selected model and written as follows:
EQi,t=αi,t+β1URBi,t+β2URB2i,t+β3GDPi,t+β4RECi,t+β5NECi,t+β6GLOi,t+εi,t | (3) |
In the light of existing literature, the expected sign of β1 is positive because urbanization leads to an increase in carbon emissions. The expectation of sign for β2 is negative if the existence of an inverted U curve is confirmed between urbanization and carbon emissions. The sign expectation for β3 is positive as more economic growth raises emissions and hurts the environment. The expected sign regarding β4 is negative while it is positive for β5. β6 can either be negative or positive depending on how globalization affects the carbon emissions. The annual time series data is transformed into a natural logarithm except for the variables whose data is already in percentage. Most data is obtained from World Development Indicators while the KOF globalization index is found in the Swiss Economic Institute database.
As Equation (3) demonstrates the long-run association of population, affluence, technology and globalization with carbon emissions. Following (Hanif et al., 2019; Anser, 2019) panel Autoregressive distributed lag model6 (ARDL) technique is used to attain efficient and consistent results during the long and short term with error correction representation. ARDL is a more robust method of estimation than the traditional approaches of co-integration such as Engel and Granger (1987) and Johansen and Juselius (1992). ARDL takes into account the small sample properties and controls the simultaneity issues among the variables of interest. Moreover, data on macroeconomic variables usually contains unit root and the use of the conventional OLS method for non-stationary data provides misleading results. ARDL is also flexible to host the mixed order of variables and capable to address the issues of endogeneity and autocorrelation due to optimal lag selection criteria (Ali et al., 2016). To fulfil the purpose, short-run dynamics are included in Equation (3) and written as Equation (4).
5It was presented by Dreher (2006) and elaborated by Dreher, Gaston and Martens (2008) and then Gygli et al. (2018).
6It was developed by Pesaran et al. (1999).
ΔEQit=α0+n∑m=1ϕ1mΔEQt−m+n∑m=0ϕ2mΔURBt−m+n∑m=0ϕ3mΔURB2t−m+∑nm=0ϕ4mΔGDPt−m+∑nm=0ϕ5mΔRECt−m+∑nm=0ϕ6mΔNECt−m+∑nm=0ϕ7mΔGLOt−m+β1EQi,t−1+β2URBi,t−1+β3URB2i,t−1+β4GDPi,t−1+β5RECi,t−1+β6NECi,t−1+β7GLOi,t−1+εi | (4) |
Here, Δ represents variables at the first difference, ϕs indicate short term coefficients, βs show the long term coefficients and ɛ is an error term. After establishing the short and long term relationship between the analyzed variables, the next step is to include ECM in the model. It provides the speed of correcting errors which emerged due to any internal or external shock during the short term. It can be written as:
ΔEQit=β0+k∑j=1ω1jΔEQt−j+k∑j=1γ2jΔURBt−j+k∑j=1δ3jΔURB2t−j+k∑j=1ψ4jΔGDPt−j+k∑j=1η5jΔRECt−j+k∑j=1τ6jΔNECt−j+k∑j=1φ7jΔGLOt−j+β1URBi,t+β2URB2i,t+β3GDPi,t+β4RECi,t+β5NECi,t+β6GLOi,t+θECi,t−1+εi,t | (5) |
Here, Ɵ is the coefficient of lagged error correction term showing the long term co-integration. It must be negative and statistically significant because it describes the speed of error adjustment.
The descriptive statistics of selected variables for the G-7 are reported in Table 1. The environmental quality of the USA shows the maximum deterioration during 2000 due to carbon emissions of 3.00 metric tons per capita. The heavy use of big cars, houses and air conditioners makes the USA one of the biggest emitters in history. Whereas carbon emissions in France remain at their lowest during 2014 with 1.52 metric tons per capita having a mean and standard deviation of 2.29 and 0.40 respectively. The attainment of more than 70 per cent of electricity from nuclear energy is the main reason behind it. Urbanization reveals the maximum value of 91.61 per cent for Japan in 2018 as it is experiencing rapid upward trends in rural-urban migration. The minimum described value of urbanization is 66.70 per cent for Italy in 1991 with a mean and standard deviation of 77.50 and 5.43. The economic growth of the USA is highest in 2018 at 10.90 US dollars per capita. While the economic growth of the UK during 1988 is reported as lowest as 10.22 US dollars per capita, having a mean and standard deviation equal to 10.56 and 0.15 US dollars per capita. Renewable energy consumption represents the highest number of 22.61 per cent and the lowest number of 0.85 per cent of total energy consumption with a mean and standard deviation of 8.88 and 6.44 for Canada and the UK during 2012 and 2001 respectively. The highest consumer of nonrenewable energy is Japan with 94.63 per cent in 2013 while France remains the lowest nonrenewable energy consumer with 46.22 per cent of total energy during 2017 with a mean and standard deviation of 79.34 and 12.33 respectively. Globalization remains highest in the UK in 2017 at 4.49 while the minimum value of globalization is 4.03 in the year 1988 for Japan with a mean and standard deviation of 4.36 and 0.09 respectively.
Variable | EQ | URB | GDP | REC | NEC | GLO |
Mean | 2.29 | 77.50 | 10.56 | 8.88 | 79.34 | 4.36 |
Median | 2.24 | 78.00 | 10.57 | 7.35 | 82.75 | 4.39 |
Maximum | 3.00 | 91.61 | 10.90 | 22.61 | 94.63 | 4.49 |
Minimum | 1.52 | 66.70 | 10.22 | 0.85 | 46.22 | 4.03 |
Std. Dev. | 0.40 | 5.43 | 0.15 | 6.44 | 12.33 | 0.09 |
Observations | 217 | 217 | 217 | 217 | 217 | 217 |
Note: EQ is the environmental quality for which CO2 emissions are used as a proxy, URB is the urbanization, GDP is the per capita economic growth, REC is renewable energy consumption, NEC is the non-renewable energy consumption and GLO is the globalization. |
The coefficients of correlation and variance inflation factor7 (VIF) are presented in Table 2. It is very important to check the nature of the pairwise relationship and the issue of multi-collinearity between all the variables before the further procedure. The magnitude of VIF between all analyzed variables is also positive and less than 10 which concludes that all regressors have a weak correlation with carbon emissions hence they are free from multi-collinearity issues.
7The variance inflation factor is calculated by using the formula [1 / (1 – r2)], where r denotes the coefficient of correlation. The value of VIF is less than 10 which indicates the absence of multi-collinearity between variables.
Variables | EQ | URB | GDP | REC | NEC | GLO |
EQ | 1 | - | - | - | - | - |
URB | 0.26 (1.07) |
1 | - | - | - | - |
GDP | 0.32 (1.11) |
0.61 (1.59) |
1 | - | - | - |
REC | 0.20 (1.04) |
0.01 (1.00) |
0.26 (1.07) |
1 | - | - |
NEC | 0.38 (1.16) |
0.04 (1.00) |
0.08 (1.00) |
0.46 (1.26) |
1 | - |
GLO | 0.18 (1.03) |
0.12 (1.01) |
0.28 (1.08) |
0.21 (1.04) |
0.24 (1.06) |
1 |
Note: Variance inflation factor is shown in parenthesis. |
At the initial stage, the data collected for underlying variables is analyzed for whether the cross-sections are dependent on each other or not. It should consider before applying the panel unit root test to avoid biased estimations. Moreover, it helps in choosing the appropriate panel unit root test. Multiple CD tests are used in this study having the same null hypothesis. Table 3 provides the outcomes of the CD test. The insignificant probability values of all tests do not allow the rejection of the null hypothesis and conclude that cross-sections have no dependence on each other. As cross sections are independent so the next step is to check the stationarity of data by using Levin, Lin, Chu (LLC) and Im, Pesaran and Shin (IPS) unit root tests. LLC unit root test provides efficient results when the panel is balanced and the time period is long. Whereas IPS testing procedure is less restrictive and comprehensive. It assumes that all cross-sections have different speeds of convergence towards equilibrium.
Test | Statistics | Probability |
Pesaran scaled LM | 0.395 | 0.69 |
Bias-corrected scaled LM | 0.265 | 0.79 |
Pesaran CD | 1.193 | 0.23 |
Ho: No cross-section dependence. |
Both tests show in Table 4 that carbon emissions, urbanization, GDP, the renewable and nonrenewable energy consumption are stationary at first difference while globalization is stationary at level. It concludes that variables are integrated of order I (0) and I (1).
LLC test | IPS test | ||||
Variable | At level | At first difference | At level | At first difference | |
EQ | −0.70 (0.24) |
−9.75 (0.00)* |
−0.02 (0.48) |
−12.30 (0.00)* |
|
URB | −1.46 (0.07) |
−6.84 (0.00)* |
−0.75 (0.22) |
−8.48 (0.00)* |
|
GDP | −1.15 (0.12) |
−8.71 (0.00)* |
1.35 (0.91) |
−10.18 (0.00)* |
|
REC | 2.50 (0.99) |
−8.56 (0.00)* |
2.34 (0.99) |
−9.69 (0.00)* |
|
NEC | 1.25 (0.89) |
−7.49 (0.00)* |
2.64 (0.99) |
−9.39 (0.00)* |
|
GLO | −8.38 (0.00)* |
− | −5.10 (0.00)* |
− | |
H0: Data series contain unit root. Note: Probabilities are shown in parenthesis. (*) shows at 1% significance level. |
In the next step, the Pedroni test(1999, 2004) and the Kao (1999) test are applied to ascertain whether co-integration exists between the analyzed variables or not. These tests are Engle and Granger (1987) based. Pedroni test displays estimates in two sets, the first set checks the co-integration status of a homogeneous panel while the second set tells about the co-integration of a heterogeneous panel. The significant p values of the majority of statistics decide to accept the alternative hypothesis and validate the long-term relationship of selected variables. The Kao co-integration test shows the ADF statistics and probability value, the significant probability value confirms the co-integration between selected variables by accepting an alternate hypothesis of co-integration.
The seven statistics of the Pedroni test are shown in Table 5. The four statistics out of seven indicate that selected variables have stable long term relationships. Then the Kao test is applied and its results are shown in Table 6. This result also indicates the existence of co-integration of explanatory variables with carbon emissions because a significant p-value indicates the acceptance of the alternate hypothesis of co-integration.
Common AR coefficients | ||
Estimates | Statistics | Weighted statistics |
Panel v-Statistic | 0.41 (0.33) |
−1.14 (0.87) |
Panel rho-Statistic | −0.43 (0.33) |
0.23 (0.59) |
Panel PP-Statistic | −6.86 (0.00)* |
−5.01 (0.00)* |
Panel ADF-Statistic | −7.70 (0.00)* |
−6.05 (0.00)* |
Individual AR coefficients | ||
Group rho-Statistic | 0.83 (0.79) |
− |
Group PP-Statistic | −7.92 (0.00)* |
− |
Group ADF-Statistic | −8.24 (0.00)* |
− |
Note: * denotes at 1% significance level. Probabilities are shown in parenthesis. |
ADF | t-statistics | probability |
−2.86* | 0.00 | |
Note: * denotes a 1% level of significance. |
After getting strong proof for co-integration between explained and explanatory variables, there is a way forward for long-run analysis and outcomes of ARDL estimation are reported in Table 7.
Variable | Coefficient | Standard Error | t-statistic |
URB | 0.2579** | 0.1096 | 2.3531 |
URB2 | −0.0016** | 0.0007 | −2.2857 |
GDP | 0.9047* | 0.1007 | 8.9841 |
REC | −0.0070** | 0.0030 | −2.3333 |
NEC | 0.0198* | 0.0026 | 7.6153 |
GLO | −1.2364* | 0.2156 | −5.7346 |
Source: Calculated by author. Note: The dependent variable is EQ. *, ** show at 1% and 5% significance levels respectively. |
The results reveal that urbanization has a positive and significant relationship with carbon emissions. It means that a one per cent increase in urbanization increases the carbon emissions by 0.2579 per cent on average in the long run assuming other factors are constant. This is similar to (Sadorsky, 2014; Mulali and Ozturk, 2015). Initially, when urbanization takes place, the needs of migrants for goods and construction multiply rapidly which raises the use of cars, public transport, air conditioners, refrigerators, materials and other appliances. All this requires heavy energy consumption which ultimately pollutes the environment. The empirical findings indicate that the urbanization square is negatively and significantly related to carbon emissions. In short, the reduction of 0.0016 per cent in carbon emissions is linked with a one per cent increase in urbanization square if the other elements are considered to be unchanged. These findings validate the reversed U-shape relationship between urbanization and carbon emissions. So this study confirms the Kuznets hypothesis in the case of G-7 which states that carbon emissions increase with the initial increase in urbanization up to a specific level after that carbon emissions decrease with further urbanization. These findings are in line with (Xu and Lin, 2015; Bekhet and Othman, 2017). Unorganized urbanization exerts upward pressure on non-renewable energy consumption and harms environmental quality. Over time, people become well aware of the health benefits of a clean environment and adopt energy-saving techniques like solar panels, inverters, LED technology, high rise buildings and renewable energy which curtail carbon emissions. This way environmental quality starts improving after a turning point and carbon emissions decline with the increase in urbanization. Another important reason for a reduction in carbon emissions due to increased urbanization is the strict policies implemented in developed countries regarding personal possession of electric appliances, usage of natural resources and renewable sources (Nejat et al., 2015). Following (Anser et al., 2020) the calculation of the turning point is mentioned as follows:
TurningPoint=−(0.5×CoefficientofurbanizationCoefficientofurbanizationsquare)TurningPoint=−(0.5×0.2579−0.0016)TurningPoint=80.59% |
It shows that urbanization in G-7 has a turning point at 80.59% of urbanization. It is the point up till which carbon emissions initially increase along with an increase in urbanization and when the urban population reaches 80.59%, then carbon emissions start declining with further urbanization. That's why the current study finds a reverse U shaped linkage between urbanization and carbon emissions. The turning point specifies that Canada, Japan, the UK and USA have entered their second phase of EKC where urbanization is non-linearly linked with carbon emissions. The remaining G-7 countries are still prevailing in phase first where urbanization and carbon emissions have linear linkage.
The empirical findings indicate that economic growth is increasing carbon emissions in long-run. One per cent increase in economic growth is associated with a 0.9047 per cent increase in carbon emissions if other things are assumed to be fixed. It means that the world's developed and industrialized G-7 economies have to bear environmental degradation as a cost of growth. This result is similar to (Magazzino, 2016; Duada et al., 2019; Shahbaz et al., 2019). It is evident that when economic growth increases, it increases the carbon emissions because of the increased use of fossil fuel energy due to its cheaper cost. It is historically proven that when countries are desirous of growth, their energy use increases due to supplementary economic activity which leads to pollution. So the transformation of energy sources is inevitable to attain economic growth without polluting the environment.
The results show that renewable energy consumption decreases carbon emissions and one per cent consumption of renewable energy reduces carbon emissions by 0.0070 per cent. This result endorses (Boluk and Mert, 2014; Ozturk and Mulali, 2016; Dogan and Seker, 2016). It is so because renewable energy sources are environmental-friendly as they emit no or very less carbon dioxide so their use is beneficial to improve the environmental quality. The long term findings exhibit that use of nonrenewable energy deteriorates the environmental quality and its one per cent addition is the source of a 0.0198 per cent increase in carbon emissions keeping other factors constant. This is in line with the results of (Farhani and Shahbaz, 2014; Jebli et al., 2016). The non-renewable energy being available at lower rates and easy access is widely used in the world, thus it increases the carbon emissions a lot. Now G-7 have entered into many environment protection agreements and regulations to shift most of its energy towards renewable energy.
Globalization has a negative and significant association with environmental quality and an increase of one per cent in globalization is linked with 1.2364 per cent decrease in carbon emissions. This is similar to (Christmann and Taylor, 2001; Lee and Min, 2014; You and Lv, 2018; Khan et al., 2019). Globalization is a multi-faceted phenomenon and mostly brings the countries closer through trade and foreign direct investment. Sometimes progressive countries like the G-7 transfer their high pollutant plants and machinery to developing countries due to globalization, by doing so they reduce their pollution. However, globalization also enables the nations to take benefit from each other's research and innovations to improve the environmental quality by reducing emission levels. The growing economic, political and social integration facilitates the countries to exchange environment-friendly technologies.
The relationship of explanatory variables with the explained variables for G-7 during the short term is explored through the ARDL technique and reported in Table 8.
Variable | Coefficient | Standard Error | t-statistic |
EQ(−1) | 0.4813* | 0.1786 | 2.6948 |
URB | 0.6969* | 0.0328 | 21.2469 |
URB(−1) | −1.5597** | 0.6358 | −2.4531 |
URB2 | −0.0044* | 0.00022 | −20.200 |
URB2(−1) | 0.0096** | 0.0039 | 2.4615 |
GDP | 0.5929* | 0.0761 | 7.7910 |
GDP(−1) | −0.0320 | 0.2930 | −0.1092 |
REC | 0.0293* | 0.0008 | 34.8809 |
REC(−1) | −0.0276* | 0.0105 | −2.6285 |
NEC | 0.0253* | 0.0005 | 51.2758 |
NEC(−1) | −0.0089*** | 0.0047 | −1.8936 |
GLO | −0.8986* | 0.1532 | −5.8655 |
GLO(−1) | 0.3077 | 0.5718 | 0.5381 |
C | −29.6259* | 1.9125 | −15.4906 |
Source: Calculated by author. Note: *, ** show at 1% and 5% significance level respectively. |
Table 8 reveals that a one per cent increase in carbon emissions in the previous period is linked with a 0.4813 per cent carbon emissions in the current period. In addition during the short term, 1.5597 per cent significant decrease in carbon emissions is evident due to one per cent increase in urbanization. The squared rate of urbanization is positively and significantly associated with carbon emissions by 0.0096 per cent. The renewable and nonrenewable energy consumption declines the emissions keeping other things fixed during the short term. However, GDP and globalization do not contribute significantly to carbon emissions during the same period.
Finally, Table 9 exhibits the outcomes of the error correction model and multiple diagnostics tests. The negative and significant coefficient of lagged error correction term (ECt-1) depicts the explanatory power of independent variables and the significance of the estimated model. The significant ECt-1 confirms the long term relationship between the explanatory and explained variables. Its negative sign indicates that the model can converge from disturbance to a stable state. Its coefficient shows the speed of correcting errors and concludes that 54.19 per cent of errors will be corrected annually. Thus it will take approximately 1.85 years (1/0.5419 = 1.845) to restore the equilibrium.
Variable | Coefficient | Standard Error | t-statistic |
URB | 0.2579** | 0.1096 | 2.3531 |
URB2 | −0.0016** | 0.0007 | −2.2857 |
GDP | 0.9047* | 0.1007 | 8.9841 |
REC | −0.0070** | 0.0030 | −2.333 |
NEC | 0.0198* | 0.0026 | 7.6153 |
GLO | −1.2364* | 0.2156 | −5.7346 |
ΔEQ | −0.2391** | 0.1141 | −2.0955 |
ΔURB | 4.2051 | 2.6673 | 1.5765 |
ΔURB2 | −0.0287 | 0.0180 | −1.5944 |
ΔGDP | 0.1258 | 0.1432 | 0.8784 |
ΔREC | −0.0075 | 0.0071 | −1.0563 |
ΔNEC | 0.0074 | 0.0048 | 1.5416 |
ΔGLO | 0.2227*** | 0.1192 | 1.8682 |
ECt−1 | −0.5419* | 0.2053 | −2.6395 |
Test statistics | LM version | F version | |
Serial Correlation | χ2 (1)=1.029(0.31) | F (1, 13)=0.478(0.50) | |
Functional Form | χ2 (1) =1.928(0.16) | F(1, 13)=0.925(0.35) | |
Normality | χ2 (2) =1.339(0.51) | _ | |
Heteroscedasticity | χ2 (1) =1.415(0.23) | F(1, 27)=1.385(0.25) | |
Source: Calculated by author. Note: Dependent variable=ΔEQ, Δ means first difference operator. *, **, *** show at 1%, 5% and 10% significance level respectively. |
The findings related to multiple diagnostic tests are reported at the bottom of the same table and prove that the residuals of the model are free from the issue of serial correlation. The functional form of the model is correctly specified. The residuals are following the normal distribution with zero mean and constant variance. The variance of the residuals is homoscedastic which verifies the absence of heteroscedasticity.
In the end, the stability of the estimated model is diagnosed by plotting the graphs of the cumulative sum of recursive residuals (CUSUM) and the cumulative sum of squares of recursive residuals (CUSUM squared). Figures 3 and 4 reflect that the CUSUM and CUSUM squared locate within the critical bounds and affirm the stability of the estimated model. So it concludes that the mean and variance of the disturbance term are structurally stable from 1988 to 2018.
The present study investigates the impact of urbanization, energy use, economic growth and globalization on the environmental quality of the G-7 group by using the STIRPAT and EKC framework from1988 to 2018. The study employed LLC and IPS unit root tests, CD test, Pedroni and Kao co-integration tests and panel ARDL approach for empirical analysis. The results based on the ARDL technique show that urbanization, economic growth and non-renewable energy consumption significantly escalate the carbon emissions while renewable energy consumption and globalization reduce the carbon emissions during the long term. Urbanization, renewable and nonrenewable energy consumption bring significant improvement in the environmental quality in the short term. The influence of economic growth and globalization on environmental quality remains insignificant at the same time. The Error Correction Model describes that 54% error will be corrected each year and converge towards stable equilibrium within 1.85 years approximately.
The study confirms an inverted U shaped EKC between urbanization and carbon emissions which means that unorganized urbanization hurts the environmental quality up to a certain threshold level but after that level, urbanization tends to improve the environmental quality. The findings also establish that Japan has crossed the turning point of 80.59% urbanization while other countries will take more time to enter the non-linear phase of urbanization and environmental quality. So it is concluded that urbanization should be systematic to avoid its harm to energy sources and the environment. The study highlights the positive impact of renewable energy use and the adverse influence of non-renewable energy use on environmental quality.
The findings reveal that increased urbanization and nonrenewable energy use are hurting the environment of the G-7 countries significantly because more urban population demands more transportation, residence and fossil fuels. Therefore the policymakers should promote the construction of high rise buildings instead of big houses to conserve agricultural lands and nonrenewable energy sources. The government should control the unorganized urbanization and provide subsidies and loans to people for transforming most of their energy consumption from nonrenewable to renewable sources to abstain from further environmental degradation. Through the vast use of renewable energy obtained from solar, wind and wave power, the G-7 group can continue to attain the highest economic growth without polluting the environment. As the role of globalization is also found quite encouraging to reduce carbon emissions in the developed countries so the administrations should continue to create global awareness of the need and importance of a clean environment and renewable energy consumption.
The present study explores the association between the variables of G-7 countries so the generalization of outcomes is not possible; although it provides an opportunity for further research either by conducting the same study considering a single country or comparative analysis of many countries. Through the application of twofold tests for unit root and co-integration and ARDL estimation, robust results are obtained. Moreover further study can be designed to achieve more robust results depending upon more advanced econometric methods and the availability of the longest and latest available data for the underlying variables in the case of G-7 economies. The impact of urbanization on the environment is complex so more research work can be carried out on the same pattern by including additional socioeconomic factors.
We hereby confirm that the manuscript has no actual or potential conflict of interest with any parties, including any financial, personal or other relationships with other people or organizations. We confirm that the paper has not been published previously, it is not under consideration for publication elsewhere, and the manuscript is not being simultaneously submitted elsewhere.
The authors received no financial support for the research, authorship, and publication of this article.
The data that support the findings of this study are available from the corresponding author, upon reasonable request.
All authors declare no conflicts of interest in this paper.
[1] |
Adusah-Poku F (2016) Carbon dioxide emissions, urbanization and population: empirical evidence from Sub-Saharan Africa. Energ Econ Lett 3: 1–16. https://doi.org/10.18488/journal.82/2016.3.1/82.1.1.16 doi: 10.18488/journal.82/2016.3.1/82.1.1.16
![]() |
[2] |
Ali HS, Law SH, Zannah TI (2016) Dynamic impact of urbanization, economic growth, energy consumption, and trade openness on CO2 emissions in Nigeria. Environ Sci Pollut R 23: 12435–12443. https://doi.org/10.1007/s11356-016-6437-3 doi: 10.1007/s11356-016-6437-3
![]() |
[3] |
Al-Mulali U, Ozturk I (2015) The effect of energy consumption, urbanization, trade openness, industrial output, and the political stability on the environmental degradation in the MENA (Middle East and North African) region. Energy 84: 382–389. https://doi.org/10.1016/j.energy.2015.03.004 doi: 10.1016/j.energy.2015.03.004
![]() |
[4] |
Al-Mulali U, Ozturk I (2016). The investigation of environmental Kuznets curve hypothesis in the advanced economies: the role of energy prices. Renew Sust Energ Rev 54: 1622–1631. https://doi.org/10.1016/j.rser.2015.10.131 doi: 10.1016/j.rser.2015.10.131
![]() |
[5] |
Anser MK (2019) Impact of energy consumption and human activities on carbon emissions in Pakistan: application of STIRPAT model. Environ Sci Pollut Res 26: 13453–13463. https://doi.org/10.1007/s11356-019-04859-y doi: 10.1007/s11356-019-04859-y
![]() |
[6] |
Anser MK, Alharthi M, Aziz B, et al. (2020) Impact of urbanization, economic growth, and population size on residential carbon emissions in the SAARC countries. Clean Technol Envir, 1–14. https://doi.org/10.1007/s10098-020-01833-y doi: 10.1007/s10098-020-01833-y
![]() |
[7] |
Apergis N, Ozturk I (2015) Testing environmental Kuznets curve hypothesis in Asian countries. Ecol Indic 52: 16–22. https://doi.org/10.1016/j.ecolind.2014.11.026 doi: 10.1016/j.ecolind.2014.11.026
![]() |
[8] |
Apergis N, Payne JE (2010) Renewable energy consumption and economic growth: evidence from a panel of OECD countries. Energ Policy 38: 656–660. https://doi.org/10.1016/j.enpol.2009.09.002 doi: 10.1016/j.enpol.2009.09.002
![]() |
[9] |
Apergis N, Payne JE, Menyah K, et al. (2010) On the causal dynamics between emissions, nuclear energy, renewable energy, and economic growth. Ecol Econ 69: 2255–2260. https://doi.org/10.1016/j.ecolecon.2010.06.014 doi: 10.1016/j.ecolecon.2010.06.014
![]() |
[10] |
Balsalobre-Lorente D, Shahbaz M, Roubaud D, et al. (2018) How economic growth, renewable electricity and natural resources contribute to CO2 emissions? Energ Policy 113: 356–367. https://doi.org/10.1016/j.enpol.2017.10.050 doi: 10.1016/j.enpol.2017.10.050
![]() |
[11] |
Behera SR, Dash D (2017) The effect of urbanization, energy consumption, and foreign direct investment on the carbon dioxide emission in the SSEA (South and Southeast Asian) region. Renew Sust Energ Rev 70: 96–106. https://doi.org/10.1016/j.rser.2016.11.201 doi: 10.1016/j.rser.2016.11.201
![]() |
[12] |
Bekhet HA, Othman NS (2017) Impact of urbanization growth on Malaysia CO2 emissions: evidence from the dynamic relationship. J Clean Prod 154: 374–388. https://doi.org/10.1016/j.jclepro.2017.03.174 doi: 10.1016/j.jclepro.2017.03.174
![]() |
[13] |
Bölük G, Mert M (2014) Fossil & renewable energy consumption, GHGs (greenhouse gases) and economic growth: Evidence from a panel of EU (European Union) countries. Energy 74: 439–446. https://doi.org/10.1016/j.energy.2014.07.008 doi: 10.1016/j.energy.2014.07.008
![]() |
[14] |
Christmann P, Taylor G (2001) Globalization and the environment: Determinants of firm self-regulation in China. J Int Bus Stud 32: 439–458. https://doi.org/10.1057/palgrave.jibs.8490976 doi: 10.1057/palgrave.jibs.8490976
![]() |
[15] |
Çoban S, Topcu M (2013) The nexus between financial development and energy consumption in the EU: A dynamic panel data analysis. Energ Econ 39: 81–88. https://doi.org/10.1016/j.eneco.2013.04.001 doi: 10.1016/j.eneco.2013.04.001
![]() |
[16] |
Dauda L, Long X, Mensah CN, et al. (2019) The effects of economic growth and innovation on CO2 emissions in different regions. Environ Sci Pollut Res 26: 15028–15038. https://doi.org/10.1007/s11356-019-04891-y doi: 10.1007/s11356-019-04891-y
![]() |
[17] |
Diao XD, Zeng SX, Tam CM, et al. (2009) EKC analysis for studying economic growth and environmental quality: a case study in China. J Clean Product 17: 541–548. https://doi.org/10.1016/j.jclepro.2008.09.007 doi: 10.1016/j.jclepro.2008.09.007
![]() |
[18] |
Dietz T, Rosa EA, York R (2007) Driving the human ecological footprint. Front Ecol Environ, 13–18. https://doi.org/10.1890/1540-9295 doi: 10.1890/1540-9295
![]() |
[19] |
Dogan E, Turkekul B (2016) CO2 emissions, real output, energy consumption, trade, urbanization and financial development: testing the EKC hypothesis for the USA. Environ Sci Pollut Res 23: 1203–1213. https://doi.org/10.1007/s11356-015-5323-8 doi: 10.1007/s11356-015-5323-8
![]() |
[20] |
Dogan E, Seker F (2016) Determinants of CO2 emissions in the European Union: The role of renewable and non-renewable energy. Renew Energ 94: 429–439. https://doi.org/10.1016/j.renene.2016.03.078 doi: 10.1016/j.renene.2016.03.078
![]() |
[21] |
Dreher A (2006) Does globalization affect growth? Evidence from a new index of globalization. Appl Econ 38: 1091–111. https://doi.org/10.1080/00036840500392078 doi: 10.1080/00036840500392078
![]() |
[22] | Dreher A, Gaston N, Martens P (2008) The Measurement of Globalization. In Measuring Globalization. Springer New York, 25–74. https://doi.org/10.1007/978-0-387-74069-0_3 |
[23] |
Duh JD, Shandas V, Chang H, et al. (2008) Rates of urbanization and the resiliency of air and water quality. Sci Total Environ 400: 238–256. https://doi.org/10.1016/j.scitotenv.2008.05.002 doi: 10.1016/j.scitotenv.2008.05.002
![]() |
[24] |
Engle RF, Yoo BS (1987) Forecasting and testing in co-integrated systems. J Econometrics 35: 143–159. https://doi.org/10.1016/0304-4076(87)90085-6 doi: 10.1016/0304-4076(87)90085-6
![]() |
[25] |
Farhani S, Shahbaz M (2014) What role of renewable and non-renewable electricity consumption and output is needed to initially mitigate CO2 emissions in MENA region? Renew Sust Energ Revi 40: 80–90. https://doi.org/10.1016/j.rser.2014.07.170 doi: 10.1016/j.rser.2014.07.170
![]() |
[26] | Grossman G, Krueger A (1991) Environmental Impacts of North American Free Trade Agreement. National Bureau of Economics Research Working Paper 3194. |
[27] | Gygli S, Haelg F, Potrafke N, et al. (2019) The KOF Globalization Index - Revisited. CESifo Working Paper No. 7430. Available from: https://ssrn.com/abstract=3338784 or http://dx.doi.org/10.2139/ssrn.3338784. |
[28] |
Hanif I (2017) Economics-energy-environment nexus in Latin America and the Caribbean. Energy 141: 170–178. https://doi.org/10.1016/j.energy.2017.09.054 doi: 10.1016/j.energy.2017.09.054
![]() |
[29] |
Hanif I, Raza SMF, Gago-de-Santos P, et al. (2019) Fossil fuels, foreign direct investment, and economic growth have triggered CO2 emissions in emerging Asian economies: some empirical evidence. Energy 171: 493–501. https://doi.org/10.1016/j.energy.2017.09.054 doi: 10.1016/j.energy.2017.09.054
![]() |
[30] |
Im KS, MH Pesaran, Shin Y (2003) Testing for unit roots in heterogeneous panels. J Econometrics 115: 53–74. https://doi.org/10.1016/S0304-4076(03)00092-7 doi: 10.1016/S0304-4076(03)00092-7
![]() |
[31] | International Energy Agency (2019) Available from: https://www.iea.org/geco/emissions/ (Accessed 28 March 2019). |
[32] |
Jebli MB, Youssef, SB, Ozturk I (2016) Testing environmental Kuznets curve hypothesis: The role of renewable and non-renewable energy consumption and trade in OECD countries. Ecol Indic 60: 824–831. https://doi.org/10.1016/j.ecolind.2015.08.031 doi: 10.1016/j.ecolind.2015.08.031
![]() |
[33] |
Kahn ME, Schwartz J (2008) Urban air pollution progress despite sprawl: the "greening" of the vehicle fleet. J Urban Econ 63: 775–787. https://doi.org/10.1016/j.jue.2007.06.004 doi: 10.1016/j.jue.2007.06.004
![]() |
[34] |
Kao Chihwa (1999) Spurious Regression and Residual-based Tests for Co-integration in Panel Data. J Econometrics 90: 1–44. https://doi.org/10.1016/S0304-4076(98)00023-2 doi: 10.1016/S0304-4076(98)00023-2
![]() |
[35] |
Khan MK, Teng JZ, Khan MI, et al. (2019) Impact of globalization, economic factors and energy consumption on CO2 emissions in Pakistan. Sci Total Environ 688: 424–436. https://doi.org/10.1016/j.scitotenv.2019.06.065 doi: 10.1016/j.scitotenv.2019.06.065
![]() |
[36] |
Khalid K, Usman M, Mehdi MA (2021) The determinants of environmental quality in the SAARC region: a spatial heterogeneous panel data approach. Environ Sci Pollut Res 28: 6422–6436. https://doi.org/10.1007/s11356-020-10896-9 doi: 10.1007/s11356-020-10896-9
![]() |
[37] | Kuznets S (1955) Economic growth and income inequality. Am Econ Rev 45: 1–28. https://www.jstor.org/stable/1811581 |
[38] |
Lee KH, Min B (2014) Globalization and carbon constrained global economy: a fad or a trend? J Asia-Pac Bus 15: 105–121. https://doi.org/10.1080/10599231.2014.904181 doi: 10.1080/10599231.2014.904181
![]() |
[39] |
Levin A, Lin CF, Chu C (2002) Unit Root Tests in Panel Data: Asymptotic and Finite. J Econometrics 108: 1–24. https://doi.org/10.1016/S0304-4076(01)00098-7 doi: 10.1016/S0304-4076(01)00098-7
![]() |
[40] |
Li B, Yao R (2009) Urbanization and its impact on building energy consumption and efficiency in China. Renew Energ 34: 1994–1998. https://doi.org/10.1016/j.renene.2009.02.015 doi: 10.1016/j.renene.2009.02.015
![]() |
[41] |
López-Menéndez AJ, Pérez R, Moreno B (2014) Environmental costs and renewable energy: Re-visiting the Environmental Kuznets Curve. J Environ Manage 145: 368–373. https://doi.org/10.1016/j.jenvman.2014.07.017 doi: 10.1016/j.jenvman.2014.07.017
![]() |
[42] |
Magazzino C (2016) The relationship between CO2 emissions, energy consumption and economic growth in Italy. Inter J Sust Energ 35: 844–857. https://doi.org/10.1080/14786451.2014.953160 doi: 10.1080/14786451.2014.953160
![]() |
[43] | Mahalik MK, Mallick H (2014) Energy consumption, economic growth and financial development: exploring the empirical linkages for India. J Dev Areas, 139–159. https://www.jstor.org/stable/24241254 |
[44] |
Martínez-Zarzoso I, Maruotti A (2011) The impact of urbanization on CO2 emissions: Evidence from developing countries. Ecol Econ 70: 1344–1353. https://doi.org/10.1016/j.ecolecon.2011.02.009 doi: 10.1016/j.ecolecon.2011.02.009
![]() |
[45] | McCoskey S, Kao C (1999) A Monte Carlo Comparison of Tests for Co-integration in Panel data. Available from: https://ssrn.com/abstract=1807953 or http://dx.doi.org/10.2139/ssrn.1807953 |
[46] | Meadows DL, Meadows DH, Randers J, et al. (1972) The limits to growth: a report for the Club of Rome's project on the predicament of ma universe books, New York. Available from: http://www.clubofrome.org/docs/limits.rtf. |
[47] |
Narayan PK, Smyth R, Prasad A (2007) Electricity consumption in G7 countries: A panel cointegration analysis of residential demand elasticities. Energ Policy 35: 4485–4494. https://doi.org/10.1016/j.enpol.2007.03.018 doi: 10.1016/j.enpol.2007.03.018
![]() |
[48] |
Nasreen S, Anwar S, Ozturk I (2017) Financial stability, energy consumption and environmental quality: Evidence from South Asian economies. Renew Sust Energ Rev 67: 1105–1122. https://doi.org/10.1016/j.rser.2016.09.021 doi: 10.1016/j.rser.2016.09.021
![]() |
[49] |
Nejat P, Jomehzadeh F, Taheri MM, et al. (2015) A global review of energy consumption, CO2 emissions and policy in the residential sector (with an overview of the top ten v2 emitting countries). Renew Sust Energ Rev 43: 843–862. https://doi.org/10.1016/j.rser.2014.11.066 doi: 10.1016/j.rser.2014.11.066
![]() |
[50] |
Pedroni P (1999) Critical values for co-integration tests in heterogeneous panels with multiple regressors. Oxford Bull Econ Stat 61: 653–670. https://doi.org/10.1111/1468-0084.0610s1653 doi: 10.1111/1468-0084.0610s1653
![]() |
[51] |
Pedroni P (2004) Panel co-integration: asymptotic and finite sample properties of pooled time series tests with an application to the PPP hypothesis. Econometric Theory 20: 597–625. https://doi.org/10.1017/S0266466604203073 doi: 10.1017/S0266466604203073
![]() |
[52] | Pesaran MH (2004) General diagnostic tests for cross section dependence in panels. University of Cambridge, England. |
[53] |
Pesaran MH, Shin, Y, Smith RP (1999) Pooled mean group estimation of dynamic heterogeneous panels. J Am Stat Assoc 94: 621–634. 10.1080/01621459.1999.10474156 doi: 10.1080/01621459.1999.10474156
![]() |
[54] |
Raza, SA, Shah, N, Khan KA (2020) Residential energy environmental Kuznets curve in emerging economies: the role of economic growth, renewable energy consumption, and financial development. Environ Sci Pollut Res 27: 5620–5629. https://doi.org/10.1007/s11356-019-06356-8 doi: 10.1007/s11356-019-06356-8
![]() |
[55] |
Sadorsky P (2014) The effect of urbanization on CO2 emissions in emerging economies. Energ Econ 41: 147–153. https://doi.org/10.1016/j.eneco.2013.11.007 doi: 10.1016/j.eneco.2013.11.007
![]() |
[56] |
Sadorsky P (2009) Renewable energy consumption, CO2 emissions and oil prices in the G7 countries. Energ Econ 31: 456–462. https://doi.org/10.1016/j.eneco.2008.12.010 doi: 10.1016/j.eneco.2008.12.010
![]() |
[57] |
Saint Akadiri S, Alola AA, Akadiri AC (2019) The role of globalization, real income, tourism in environmental sustainability target. Evidence from Turkey. Sci Total Environ 687: 423–432. https://doi.org/10.1016/j.scitotenv.2019.06.139 doi: 10.1016/j.scitotenv.2019.06.139
![]() |
[58] |
Sahoo M, Sethi N (2021) The dynamic impact of urbanization, structural transformation, and technological innovation on ecological footprint and PM2. 5: evidence from newly industrialized countries. Environ Dev Sust, 1–34. https://doi.org/10.1007/s10668-021-01614-7 doi: 10.1007/s10668-021-01614-7
![]() |
[59] |
Shafiei S, Salim RA (2014) Non-renewable and renewable energy consumption and CO2 emissions in OECD countries: a comparative analysis. Energ Policy 66: 547–556. https://doi.org/10.1016/j.enpol.2013.10.064 doi: 10.1016/j.enpol.2013.10.064
![]() |
[60] |
Shahbaz M, Loganathan K, Muzaffar AT, et al. (2016) How urbanization affects CO2 emissions in Malaysia? The application of STIRPAT model. Renew Sust Energ Rev 57: 83–93. https://doi.org/10.1016/j.rser.2015.12.096 doi: 10.1016/j.rser.2015.12.096
![]() |
[61] |
Shahbaz M, Balsalobre D, Shahzad SJH (2019) The influencing factors of CO2 emissions and the role of biomass energy consumption: statistical experience from G-7 countries. Environ Model Asses 24: 143–161. https://doi.org/10.1007/s10666-018-9620-8 doi: 10.1007/s10666-018-9620-8
![]() |
[62] |
Shahbaz M, Mahalik MK, Shahzad SJH, et al. (2019) Testing the globalization-driven carbon emissions hypothesis: international evidence. Int Econ 158: 25–38. https://doi.org/10.1016/j.inteco.2019.02.002 doi: 10.1016/j.inteco.2019.02.002
![]() |
[63] |
Sharif A, Raza SA, Ozturk I, et al. (2019) The dynamic relationship of renewable and nonrenewable energy consumption with carbon emission: A global study with the application of heterogeneous panel estimations. Renew Energ 133: 685–691. https://doi.org/10.1016/j.renene.2018.10.052 doi: 10.1016/j.renene.2018.10.052
![]() |
[64] |
Sharma SS (2011) Determinants of carbon dioxide emissions: Empirical evidence from 69 countries. Appl Energ 88: 376–382. https://doi.org/10.1016/j.apenergy.2010.07.022 doi: 10.1016/j.apenergy.2010.07.022
![]() |
[65] |
Soytas U, Sari R (2006) Energy consumption and income in G-7 countries. J Policy Model 28: 739–750. https://doi.org/10.1016/j.jpolmod.2006.02.003 doi: 10.1016/j.jpolmod.2006.02.003
![]() |
[66] |
Stock JH, Watson MW (1993) A simple estimator of co-integrating vectors in higher order integrated systems. Econometrica J Econometric Soc, 783–820. https://doi.org/10.2307/2951763 doi: 10.2307/2951763
![]() |
[67] |
Ulucak R, Khan SUD (2020) Determinants of the ecological footprint: role of renewable energy, natural resources, and urbanization. Sust Cities Soc 54: 101996. https://doi.org/10.1016/j.scs.2019.101996 doi: 10.1016/j.scs.2019.101996
![]() |
[68] |
Wang Q, Zeng YE, Wu BW (2016) Exploring the relationship between urbanization, energy consumption, and CO2 emissions in different provinces of China. Renew Sust Energ Rev 54: 1563–1579. https://doi.org/10.1016/j.rser.2015.10.090 doi: 10.1016/j.rser.2015.10.090
![]() |
[69] | WDI (2019) World Development Indicators | Database. www.data.worldbank.org. |
[70] |
Xu B, Lin B (2015) How industrialization and urbanization process impacts on CO2 emissions in China: evidence from nonparametric additive regression models. Energ Econ 48: 188–202. https://doi.org/10.1016/j.eneco.2015.01.005 doi: 10.1016/j.eneco.2015.01.005
![]() |
[71] |
You W, Lv Z (2018) Spillover effects of economic globalization on CO2 emissions: a spatial panel approach. Energ Econ 73: 248–257. https://doi.org/10.1016/j.eneco.2018.05.016 doi: 10.1016/j.eneco.2018.05.016
![]() |
[72] |
Zaman K, Shahbaz M, Loganathan N, et al. (2016) Tourism development, energy consumption and Environmental Kuznets Curve: Trivariate analysis in the panel of developed and developing countries. Tour Manage 54: 275–283. https://doi.org/10.1016/j.tourman.2015.12.001 doi: 10.1016/j.tourman.2015.12.001
![]() |
[73] |
Zhang G, Zhang N, Liao W (2018) How do population and land urbanization affect CO2 emissions under gravity centre change? A spatial econometric analysis. J Clean Prod 202: 510–523. https://doi.org/10.1016/j.jclepro.2018.08.146 doi: 10.1016/j.jclepro.2018.08.146
![]() |
1. | Asif Raihan, Shewly Bala, Afsana Akther, Mohammad Ridwan, Md. Eleais, Prattoy Chakma, Advancing environmental sustainability in the G-7: The impact of the digital economy, technological innovation, and financial accessibility using panel ARDL approach, 2024, 29499488, 10.1016/j.ject.2024.06.001 | |
2. | Shuja Iqbal, Hongyun Tian, Saqib Muneer, Abhishek Tripathi, Ahmad Y.A. Bani Ahmad, Mineral resource rents, fintech technological innovation, digital transformation, and environmental quality in BRI countries: An insight using panel NL-ARDL, 2024, 93, 03014207, 105074, 10.1016/j.resourpol.2024.105074 | |
3. | Omid Mansourihanis, Mohammad Javad Maghsoodi Tilaki, Tahereh Kookhaei, Ayda Zaroujtaghi, Shiva Sheikhfarshi, Nastaran Abdoli, Integrating geospatial intelligence and spatio-temporal modeling for monitoring tourism-related carbon emissions in the United States, 2024, 1477-7835, 10.1108/MEQ-04-2024-0156 | |
4. | Radu Ioan Petrariu, Marian Nastase, Gabriel Croitoru, Nicoleta Valentina Florea, Nicoleta Cristache, Mihaela Cristina Onica Ibinceanu, Analysis of Responsible Energy Consumers Behaviour in the Context of REPowerEU Plan , 2023, 25, 15829146, 743, 10.24818/EA/2023/64/743 | |
5. | Bartosz Jóźwik, Mesut Doğan, Samet Gürsoy, The Impact of Renewable Energy Consumption on Environmental Quality in Central European Countries: The Mediating Role of Digitalization and Financial Development, 2023, 16, 1996-1073, 7041, 10.3390/en16207041 | |
6. | Qingsheng Zhu, Changwen Xie, Jia-Bao Liu, The impact of population agglomeration on ecological resilience: Evidence from China, 2023, 20, 1551-0018, 15898, 10.3934/mbe.2023708 | |
7. | Iftikhar Ahmad, Arifa Saeed, Asra Jabbar, Marie G. Nakitende, The Impact of Renewable Energy; Financial Development and Economic Growth on Carbon Emission: Empirical Evidence from Pakistan, 2024, 10, 2410-8162, 90, 10.61506/02.00210 | |
8. | Laila Khalid, Farhat Rasul, Nabila Asghar, Exploring the Complementary and Nonlinear Effects of Financial Development and ICT on Inclusive Growth in South Asia, 2023, 18, 2010-4952, 10.1142/S2010495223500136 | |
9. | Fergül Özgün, KÜRESELLEŞME VE KENTLEŞMENİN ÇEVREYE ETKİSİ: TÜRKİYE ÜZERİNE BİR UYGULAMA, 2024, 25, 1303-0027, 130, 10.24889/ifede.1501231 | |
10. | Haider Mahmood, Maham Furqan, Renewable energy transition, urbanization, and environment nexus in the Middle East and North Africa: Cross-sectional dependence analyses, 2025, 16, 19986041, 89, 10.21511/ee.16(1).2025.07 |
Variable | EQ | URB | GDP | REC | NEC | GLO |
Mean | 2.29 | 77.50 | 10.56 | 8.88 | 79.34 | 4.36 |
Median | 2.24 | 78.00 | 10.57 | 7.35 | 82.75 | 4.39 |
Maximum | 3.00 | 91.61 | 10.90 | 22.61 | 94.63 | 4.49 |
Minimum | 1.52 | 66.70 | 10.22 | 0.85 | 46.22 | 4.03 |
Std. Dev. | 0.40 | 5.43 | 0.15 | 6.44 | 12.33 | 0.09 |
Observations | 217 | 217 | 217 | 217 | 217 | 217 |
Note: EQ is the environmental quality for which CO2 emissions are used as a proxy, URB is the urbanization, GDP is the per capita economic growth, REC is renewable energy consumption, NEC is the non-renewable energy consumption and GLO is the globalization. |
Variables | EQ | URB | GDP | REC | NEC | GLO |
EQ | 1 | - | - | - | - | - |
URB | 0.26 (1.07) |
1 | - | - | - | - |
GDP | 0.32 (1.11) |
0.61 (1.59) |
1 | - | - | - |
REC | 0.20 (1.04) |
0.01 (1.00) |
0.26 (1.07) |
1 | - | - |
NEC | 0.38 (1.16) |
0.04 (1.00) |
0.08 (1.00) |
0.46 (1.26) |
1 | - |
GLO | 0.18 (1.03) |
0.12 (1.01) |
0.28 (1.08) |
0.21 (1.04) |
0.24 (1.06) |
1 |
Note: Variance inflation factor is shown in parenthesis. |
Test | Statistics | Probability |
Pesaran scaled LM | 0.395 | 0.69 |
Bias-corrected scaled LM | 0.265 | 0.79 |
Pesaran CD | 1.193 | 0.23 |
Ho: No cross-section dependence. |
LLC test | IPS test | ||||
Variable | At level | At first difference | At level | At first difference | |
EQ | −0.70 (0.24) |
−9.75 (0.00)* |
−0.02 (0.48) |
−12.30 (0.00)* |
|
URB | −1.46 (0.07) |
−6.84 (0.00)* |
−0.75 (0.22) |
−8.48 (0.00)* |
|
GDP | −1.15 (0.12) |
−8.71 (0.00)* |
1.35 (0.91) |
−10.18 (0.00)* |
|
REC | 2.50 (0.99) |
−8.56 (0.00)* |
2.34 (0.99) |
−9.69 (0.00)* |
|
NEC | 1.25 (0.89) |
−7.49 (0.00)* |
2.64 (0.99) |
−9.39 (0.00)* |
|
GLO | −8.38 (0.00)* |
− | −5.10 (0.00)* |
− | |
H0: Data series contain unit root. Note: Probabilities are shown in parenthesis. (*) shows at 1% significance level. |
Common AR coefficients | ||
Estimates | Statistics | Weighted statistics |
Panel v-Statistic | 0.41 (0.33) |
−1.14 (0.87) |
Panel rho-Statistic | −0.43 (0.33) |
0.23 (0.59) |
Panel PP-Statistic | −6.86 (0.00)* |
−5.01 (0.00)* |
Panel ADF-Statistic | −7.70 (0.00)* |
−6.05 (0.00)* |
Individual AR coefficients | ||
Group rho-Statistic | 0.83 (0.79) |
− |
Group PP-Statistic | −7.92 (0.00)* |
− |
Group ADF-Statistic | −8.24 (0.00)* |
− |
Note: * denotes at 1% significance level. Probabilities are shown in parenthesis. |
ADF | t-statistics | probability |
−2.86* | 0.00 | |
Note: * denotes a 1% level of significance. |
Variable | Coefficient | Standard Error | t-statistic |
URB | 0.2579** | 0.1096 | 2.3531 |
URB2 | −0.0016** | 0.0007 | −2.2857 |
GDP | 0.9047* | 0.1007 | 8.9841 |
REC | −0.0070** | 0.0030 | −2.3333 |
NEC | 0.0198* | 0.0026 | 7.6153 |
GLO | −1.2364* | 0.2156 | −5.7346 |
Source: Calculated by author. Note: The dependent variable is EQ. *, ** show at 1% and 5% significance levels respectively. |
Variable | Coefficient | Standard Error | t-statistic |
EQ(−1) | 0.4813* | 0.1786 | 2.6948 |
URB | 0.6969* | 0.0328 | 21.2469 |
URB(−1) | −1.5597** | 0.6358 | −2.4531 |
URB2 | −0.0044* | 0.00022 | −20.200 |
URB2(−1) | 0.0096** | 0.0039 | 2.4615 |
GDP | 0.5929* | 0.0761 | 7.7910 |
GDP(−1) | −0.0320 | 0.2930 | −0.1092 |
REC | 0.0293* | 0.0008 | 34.8809 |
REC(−1) | −0.0276* | 0.0105 | −2.6285 |
NEC | 0.0253* | 0.0005 | 51.2758 |
NEC(−1) | −0.0089*** | 0.0047 | −1.8936 |
GLO | −0.8986* | 0.1532 | −5.8655 |
GLO(−1) | 0.3077 | 0.5718 | 0.5381 |
C | −29.6259* | 1.9125 | −15.4906 |
Source: Calculated by author. Note: *, ** show at 1% and 5% significance level respectively. |
Variable | Coefficient | Standard Error | t-statistic |
URB | 0.2579** | 0.1096 | 2.3531 |
URB2 | −0.0016** | 0.0007 | −2.2857 |
GDP | 0.9047* | 0.1007 | 8.9841 |
REC | −0.0070** | 0.0030 | −2.333 |
NEC | 0.0198* | 0.0026 | 7.6153 |
GLO | −1.2364* | 0.2156 | −5.7346 |
ΔEQ | −0.2391** | 0.1141 | −2.0955 |
ΔURB | 4.2051 | 2.6673 | 1.5765 |
ΔURB2 | −0.0287 | 0.0180 | −1.5944 |
ΔGDP | 0.1258 | 0.1432 | 0.8784 |
ΔREC | −0.0075 | 0.0071 | −1.0563 |
ΔNEC | 0.0074 | 0.0048 | 1.5416 |
ΔGLO | 0.2227*** | 0.1192 | 1.8682 |
ECt−1 | −0.5419* | 0.2053 | −2.6395 |
Test statistics | LM version | F version | |
Serial Correlation | χ2 (1)=1.029(0.31) | F (1, 13)=0.478(0.50) | |
Functional Form | χ2 (1) =1.928(0.16) | F(1, 13)=0.925(0.35) | |
Normality | χ2 (2) =1.339(0.51) | _ | |
Heteroscedasticity | χ2 (1) =1.415(0.23) | F(1, 27)=1.385(0.25) | |
Source: Calculated by author. Note: Dependent variable=ΔEQ, Δ means first difference operator. *, **, *** show at 1%, 5% and 10% significance level respectively. |
Variable | EQ | URB | GDP | REC | NEC | GLO |
Mean | 2.29 | 77.50 | 10.56 | 8.88 | 79.34 | 4.36 |
Median | 2.24 | 78.00 | 10.57 | 7.35 | 82.75 | 4.39 |
Maximum | 3.00 | 91.61 | 10.90 | 22.61 | 94.63 | 4.49 |
Minimum | 1.52 | 66.70 | 10.22 | 0.85 | 46.22 | 4.03 |
Std. Dev. | 0.40 | 5.43 | 0.15 | 6.44 | 12.33 | 0.09 |
Observations | 217 | 217 | 217 | 217 | 217 | 217 |
Note: EQ is the environmental quality for which CO2 emissions are used as a proxy, URB is the urbanization, GDP is the per capita economic growth, REC is renewable energy consumption, NEC is the non-renewable energy consumption and GLO is the globalization. |
Variables | EQ | URB | GDP | REC | NEC | GLO |
EQ | 1 | - | - | - | - | - |
URB | 0.26 (1.07) |
1 | - | - | - | - |
GDP | 0.32 (1.11) |
0.61 (1.59) |
1 | - | - | - |
REC | 0.20 (1.04) |
0.01 (1.00) |
0.26 (1.07) |
1 | - | - |
NEC | 0.38 (1.16) |
0.04 (1.00) |
0.08 (1.00) |
0.46 (1.26) |
1 | - |
GLO | 0.18 (1.03) |
0.12 (1.01) |
0.28 (1.08) |
0.21 (1.04) |
0.24 (1.06) |
1 |
Note: Variance inflation factor is shown in parenthesis. |
Test | Statistics | Probability |
Pesaran scaled LM | 0.395 | 0.69 |
Bias-corrected scaled LM | 0.265 | 0.79 |
Pesaran CD | 1.193 | 0.23 |
Ho: No cross-section dependence. |
LLC test | IPS test | ||||
Variable | At level | At first difference | At level | At first difference | |
EQ | −0.70 (0.24) |
−9.75 (0.00)* |
−0.02 (0.48) |
−12.30 (0.00)* |
|
URB | −1.46 (0.07) |
−6.84 (0.00)* |
−0.75 (0.22) |
−8.48 (0.00)* |
|
GDP | −1.15 (0.12) |
−8.71 (0.00)* |
1.35 (0.91) |
−10.18 (0.00)* |
|
REC | 2.50 (0.99) |
−8.56 (0.00)* |
2.34 (0.99) |
−9.69 (0.00)* |
|
NEC | 1.25 (0.89) |
−7.49 (0.00)* |
2.64 (0.99) |
−9.39 (0.00)* |
|
GLO | −8.38 (0.00)* |
− | −5.10 (0.00)* |
− | |
H0: Data series contain unit root. Note: Probabilities are shown in parenthesis. (*) shows at 1% significance level. |
Common AR coefficients | ||
Estimates | Statistics | Weighted statistics |
Panel v-Statistic | 0.41 (0.33) |
−1.14 (0.87) |
Panel rho-Statistic | −0.43 (0.33) |
0.23 (0.59) |
Panel PP-Statistic | −6.86 (0.00)* |
−5.01 (0.00)* |
Panel ADF-Statistic | −7.70 (0.00)* |
−6.05 (0.00)* |
Individual AR coefficients | ||
Group rho-Statistic | 0.83 (0.79) |
− |
Group PP-Statistic | −7.92 (0.00)* |
− |
Group ADF-Statistic | −8.24 (0.00)* |
− |
Note: * denotes at 1% significance level. Probabilities are shown in parenthesis. |
ADF | t-statistics | probability |
−2.86* | 0.00 | |
Note: * denotes a 1% level of significance. |
Variable | Coefficient | Standard Error | t-statistic |
URB | 0.2579** | 0.1096 | 2.3531 |
URB2 | −0.0016** | 0.0007 | −2.2857 |
GDP | 0.9047* | 0.1007 | 8.9841 |
REC | −0.0070** | 0.0030 | −2.3333 |
NEC | 0.0198* | 0.0026 | 7.6153 |
GLO | −1.2364* | 0.2156 | −5.7346 |
Source: Calculated by author. Note: The dependent variable is EQ. *, ** show at 1% and 5% significance levels respectively. |
Variable | Coefficient | Standard Error | t-statistic |
EQ(−1) | 0.4813* | 0.1786 | 2.6948 |
URB | 0.6969* | 0.0328 | 21.2469 |
URB(−1) | −1.5597** | 0.6358 | −2.4531 |
URB2 | −0.0044* | 0.00022 | −20.200 |
URB2(−1) | 0.0096** | 0.0039 | 2.4615 |
GDP | 0.5929* | 0.0761 | 7.7910 |
GDP(−1) | −0.0320 | 0.2930 | −0.1092 |
REC | 0.0293* | 0.0008 | 34.8809 |
REC(−1) | −0.0276* | 0.0105 | −2.6285 |
NEC | 0.0253* | 0.0005 | 51.2758 |
NEC(−1) | −0.0089*** | 0.0047 | −1.8936 |
GLO | −0.8986* | 0.1532 | −5.8655 |
GLO(−1) | 0.3077 | 0.5718 | 0.5381 |
C | −29.6259* | 1.9125 | −15.4906 |
Source: Calculated by author. Note: *, ** show at 1% and 5% significance level respectively. |
Variable | Coefficient | Standard Error | t-statistic |
URB | 0.2579** | 0.1096 | 2.3531 |
URB2 | −0.0016** | 0.0007 | −2.2857 |
GDP | 0.9047* | 0.1007 | 8.9841 |
REC | −0.0070** | 0.0030 | −2.333 |
NEC | 0.0198* | 0.0026 | 7.6153 |
GLO | −1.2364* | 0.2156 | −5.7346 |
ΔEQ | −0.2391** | 0.1141 | −2.0955 |
ΔURB | 4.2051 | 2.6673 | 1.5765 |
ΔURB2 | −0.0287 | 0.0180 | −1.5944 |
ΔGDP | 0.1258 | 0.1432 | 0.8784 |
ΔREC | −0.0075 | 0.0071 | −1.0563 |
ΔNEC | 0.0074 | 0.0048 | 1.5416 |
ΔGLO | 0.2227*** | 0.1192 | 1.8682 |
ECt−1 | −0.5419* | 0.2053 | −2.6395 |
Test statistics | LM version | F version | |
Serial Correlation | χ2 (1)=1.029(0.31) | F (1, 13)=0.478(0.50) | |
Functional Form | χ2 (1) =1.928(0.16) | F(1, 13)=0.925(0.35) | |
Normality | χ2 (2) =1.339(0.51) | _ | |
Heteroscedasticity | χ2 (1) =1.415(0.23) | F(1, 27)=1.385(0.25) | |
Source: Calculated by author. Note: Dependent variable=ΔEQ, Δ means first difference operator. *, **, *** show at 1%, 5% and 10% significance level respectively. |