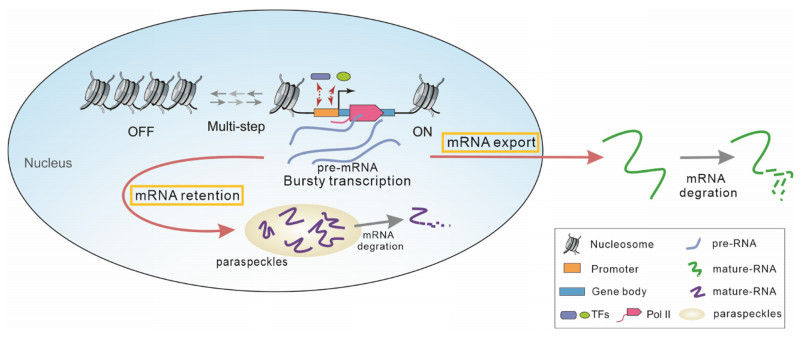
The emergence of cooperative quasi-species consortia (QS-C) thinking from the more accepted quasispecies equations of Manfred Eigen, provides a conceptual foundation from which concerted action of RNA agents can now be understood. As group membership becomes a basic criteria for the emergence of living systems, we also start to understand why the history and context of social RNA networks become crucial for survival and function. History and context of social RNA networks also lead to the emergence of a natural genetic code. Indeed, this QS-C thinking can also provide us with a transition point between the chemical world of RNA replicators and the living world of RNA agents that actively differentiate self from non-self and generate group identity with membership roles. Importantly the social force of a consortia to solve complex, multilevel problems also depend on using opposing and minority functions. The consortial action of social networks of RNA stem-loops subsequently lead to the evolution of cellular organisms representing a tree of life.
Citation: Luis P. Villarreal, Guenther Witzany. Social Networking of Quasi-Species Consortia drive Virolution via Persistence[J]. AIMS Microbiology, 2021, 7(2): 138-162. doi: 10.3934/microbiol.2021010
The emergence of cooperative quasi-species consortia (QS-C) thinking from the more accepted quasispecies equations of Manfred Eigen, provides a conceptual foundation from which concerted action of RNA agents can now be understood. As group membership becomes a basic criteria for the emergence of living systems, we also start to understand why the history and context of social RNA networks become crucial for survival and function. History and context of social RNA networks also lead to the emergence of a natural genetic code. Indeed, this QS-C thinking can also provide us with a transition point between the chemical world of RNA replicators and the living world of RNA agents that actively differentiate self from non-self and generate group identity with membership roles. Importantly the social force of a consortia to solve complex, multilevel problems also depend on using opposing and minority functions. The consortial action of social networks of RNA stem-loops subsequently lead to the evolution of cellular organisms representing a tree of life.
Transcription is not only the most important but also the most complex step in gene expression. This double characteristic makes gene transcription receive lasting and extensive attention. With the development of measurement technologies (e.g., single-cell and single-molecule technologies), more molecular details of transcription have been experimentally uncovered. Nevertheless, some intermediate processes would not have been specified due to the complexity of gene transcription. Thus, traditional Markov models of gene transcription such as extensively studied ON-OFF models [1,2,3,4] would nor interpret experimental phenomena nor reveal the stochastic mechanisms of transcription. More biologically reasonable mathematical models need to be developed.
It is well known that gene transcription involves RNA nuclear retention (RNR) and nuclear RNA export (NRE). However, these two important processes were often ignored in previous studies [1,2,3,4,5,6,7,8]. Main reasons include that 1) NRE was before considered to be a transient process, compared to other processes occurring in transcription. It was reported that the NRE phase lasted about 20min on average and were gene-specific [9,10]; 2) For RNR, less than 30% poly (A+) RNA is nuclear-retained and undetectable in the cytoplasm [11]. Currently, more and more experimental evidences have indicated that RNR play a key role in biological processes, e.g., in S. cerevisiae cells, RNR may play a precautionary role during stress situations [12]; in plants, the RNR process of NLP7 can orchestrate the early response to nitrate [13]; and in the signaling pathway of antiviral innate immunity, the RNA helicase DDX46 acts like a negative regulator to induce nuclear retention of antiviral innate transcripts [14]. These experimental facts suggest that RNR or NRE cannot be neglected when one makes theoretical predictions of gene expression (including expression levels and noise).
Several works have revealed the respective roles of RNE and RNR in modulating stochastic gene expression [15,16,17,18,19]. One report exhibited that transcriptional burst attributed to promoter state switching could be substantially attenuated by the transport of mRNA from nucleus to cytoplasm [17]. Another report showed that slow pre-mRNA export from the nucleus could be an effective mechanism to attenuate protein variability arising from transcriptional burst [15]. In addition, RNR was also identified to buffer transcriptional burst in tissues and mammalian cells [16,18]. However, it has been experimentally confirmed that NRE and RNR can occur simultaneously in eukaryotes [20]. How these two dynamic processes cooperatively affect gene expression remains elusive and even unexplored.
As a matter of fact, gene activation, NRE and RNR are multistep processes. In general, transcription begins only when the chromatin template accumulates over time until the promoter becomes active [21,22], where the accumulating process is a multistep biochemical process in which some intermediate steps would not have been specified due to experimental technologies. A representative example is that inactive phases of the promoter involving the prolactin gene in a mammalian cell are differently distributed, showing strong memory [23]. Similarly, both the export of mRNAs generated in the nucleus to the cytoplasm through nuclear pores and the retention of mRNAs among nuclear speckles or paraspeckles are in general also multistep reaction processes [24]. All these multistep processes can create memories between reaction events, leading to non-Markov dynamics. Traditional Markov models are no longer suitable to the modeling of gene transcription with molecular memory, and non-Markov models can well model multistep processes involved in gene transcription [7].
In this paper, we introduce a non-Markov model of stochastic gene transcription. It considers not only RNR and NRE but also molecular memories created due to the multistep NRE process, due to RNR process, or due to the multistep activation process, thus including previous transcription models [1,2,3,4] as its special case. In order to solve this non-Markov model, we introduce effective transition rates, which explicitly decode the effect of molecular memory and by which we can transform the original non-Markov issue into an equivalent yet mathematical tractable Markov one. Based on this useful technique, we derive analytical results, which extend previous results [3,8,24,25] and provide insights into the role of molecular memory in affecting the nuclear and cytoplasmic mRNA mean and noise. The overall modeling and analysis provide a paradigm for studying more complex stochastic transcription processes.
Most previous studies [15,20,26] of gene transcription focused on the dynamics of NRE processes, where mature mRNAs were released to the cytoplasm with the help of nuclear pore complex (NPC) (Figure 1) [16,27]. The number of NPCs or the count of those assistant proteins that controlled NPC determined the speed of exporting mRNA. Measuring the exporting rate was often replaced by measuring the retention time in the nucleus, which however may vary with environmental changes [16,17,18,19]. Other previous studies of gene transcription [9,10,11,28,29] focused on the dynamics of transcription initiation and elongation, where elongation time (T) was measured by the length of a gene using the length of bases (L) and the average rate of elongation (v), i.e., T=L/Lvv. These studies assumed that all mature mRNAs were first exported to the cytoplasm and then translated into proteins. However, biological experiments indicated that there were a considerable part of mature mRNAs that stayed in the nucleus in a probabilistic manner and lasted a long period (Figure 1) [24].
Here, we consider two cases: one case where NRE dominates over RNR and the other case where RNR dominates over NRE. For both cases, the gene is assumed to have one "off" state (corresponding to the inactive form of the promoter) and one "on" state (corresponding to the active form), and the promoter is assumed to switch randomly between these two states. Only in "on" state, can the gene generate pre-mRNA. After an alternative splicing (AS) process or an alternative polyadenylation (APA) process, which occurs frequently at the 3' UTR, a portion of mature mRNAs (one type of transcripts) may be transported to the cytoplasm through NPC wherein they execute translation tasks. The rest mature mRNAs (another type of transcripts) may be remained in the nucleus for a long time, possibly assembling on the sub-cellular region (wherein they form nuclear speckles or paraspeckles [30,31,32]) with the assistance of proteins, some of which would have been unspecified. When the intracellular environment is changed, most of mature mRNAs will release to the cytoplasm in response to this change. In addition, most genes (especially in eukaryotic cells) are expressed in a bursty manner [1,2,3,4].
As pointed out in the introduction, gene transcription, NRE and RNR are all multistep reaction processes. In order to model these processes, we introduce a non-exponential waiting-time distribution for each intermediate reaction as done in ref. [7,33]. Since non-exponential waiting times lead to non-Markovian dynamics, the existing Markov theory cannot be used directly.
Assume that burst size in gene transcription follows a distribution described by prob{B=i}=αi, where each αi is a nonnegative constant and i=0,1,2,⋯. Let M1, M2 and M3 represent pre-mRNA, mature mRNA (one type of transcripts) transported to the cytoplasm and mature mRNA (another type of transcripts) retained in the nucleus respectively, and denote by m1, m2 and m3 their molecular numbers respectively. Thus, m=(m1,m2,m3)T represents the micro-state of the underlying system. Let W1(t;m), W2(t;m) and W3(t;m) be waiting-time distributions for the syntheses of pre-mRNAs, mature mRNA transported to the cytoplasm, and mature mRNA retained in the nucleus, respectively. Let W4(t;m) and W5(t;m) be waiting-time distributions for degradations of M2 and M3, respectively. To that end, the gene-expression model to be studied are described by the following five biochemical reactions labelled by Ri (1⩽i⩽5)
R1:DNAW1(t;m)→DNA+B×M1, R2:M1W2(t;m)→M2,R3: M1W3(t;m)→M3, R4:M2W4(t;m)→∅, R5:M3W5(t;m)→∅. | (1) |
Let ⟨B⟩ represent the mean burst size. Note that if α1=1 and αk=0 for all k≠1, this implies⟨B⟩≡1. In this case, the promoter is in the ON state all the time, and Eq (1) describes the constitutive gene expression. The other cases correspond to bursty gene expression. This is because B=0 implies that the promoter is in the OFF state (meaning that pre-mRNAs are not generated), whereas B>0 implies that the promoter is at the ON state (meaning that pre-mRNAs are generated).
For each reaction, there is a memory function [7,33]. Denote by Mi(t;m) memory function for reaction Ri (1⩽i⩽5). These memory functions can be expressed by waiting-time distributions in Eq (1). In fact, if we let ˜Mi(s;m) be the Laplace transform of memory function Mi(t;m), then ˜Mi(s;m) can be expressed as ˜Mi(s;m)=s˜φi(s;m)/s˜φi(s;m)[1−∑5i=1˜φi(s;m)][1−∑5i=1˜φi(s;m)], where ˜φi(s;m) is the Laplace transform of function φi(t;m) that can be expressed as φi(t;m)=Wi(t;m)∏k≠i[1−∫t0Wk(t′;m)dt′] (1⩽i⩽5) [7]. Let P(m;t) be the probability that the system is in state m at time t and ˜P(m;s) be the Laplace transform of P(m;t). With ˜Mi(s;m), we can show that the chemical master equation in the sense of Laplace transform takes the form
s˜P(m;s)−P(0;s)=(m1∑i=0αiE−i1−I)[˜M1(s;m)˜P(m;s)]+(E1E−12−I)[˜M2(s;m)˜P(m;s)] +(E1E−13−I)[˜M3(s;m)˜P(m;s)]+5∑j=4(Ej−I)[˜Mj(s;m)˜P(m;s)], | (2) |
where E is the step operator and E−1 is the inverse of E, and I is the unit operator.
Interestingly, we find that limit lims→0˜Mi(s;m) always exists, and if the limit function is denoted by Ki(m), then Ki(m) can be explicitly expressed by given waiting-time distributions Wk(t;m) (1⩽k⩽5), that is,
Ki(m)=∫ + ∞0Wi(t;m)[∏j≠i∫∞tWj(t′;m)dt′]dt∫ + ∞0[∏5j=1∫∞tWj(t′;m)dt′]dt,1⩽i⩽5. | (3) |
Note that s→0 corresponds to t→+∞ according to the definition of Laplace transform. However, t→+∞ corresponds to the steady-state case, which is our interest. We point out that function Ki(m), which will be called effective transition rate for reaction Ri, explicitly decodes the effect of molecular memory, where 1⩽i⩽5. More importantly, using these effective transition rates, we can construct a Markov reaction network with the same topology as the original non-Markov reaction network:
DNAK1(m)→DNA+B×M1, M1K2(m)→M2, M1K3(m)→M3, M2K4(m)→∅, M3K5(m)→∅. | (4) |
Moreover, two reaction networks have exactly the same chemical master equation at steady state:
(m1∑i=0αiE−i1−I)[K1(s;m)P(m)]+(E1E−12−I)[K2(m)P(m)] +(E1E−13−I)[K3(m)P(m)]+∑j∈{4,5}(Ej−I)[Kj(m)P(m)]=0, | (5) |
implying that both stationary behaviors are exactly the same (referring to Figure 2), where P(m) is the stationary probability density function corresponding to dynamic density probability function P(m;t).
In summary, by introducing an effective transition rate (Ki(m)) for each reaction Ri, given by Eq (3), a mathematically difficult non-Markov issue is transformed into a mathematical tractable Markov issue. This brings us convenience for theoretical analysis. In the following, we will focus on analysis of Eq (5).
Note that Gamma functions can well model multistep processes [34,35]. This is because the convolution of several exponential distributions is an Erlang distribution (a special case of Gamma distribution). Therefore, in order to model the effect of molecular memory on the mRNA expression, we assume that waiting-time distributions for gene activation, NRE and RNR processes are Gamma distributions: W1(t;m)=[Γ(L0)]−1tL0−1(k0)L0e−k0t, W2(t;m)=[Γ(Lc)]−1tLc−1(m1kc)Lce−m1kct andW3(t;m)=[Γ(Lr)]−1tLr−1(m1kr)Lre−m1krt, and that waiting-time distributions for the other processes are exponential ones, W4(t;m)=m2dce−m2dct, and W5(t;m)=m3dre−m3drt. Here Γ(⋅) is the common Gamma function, k0 is the mean transcription rate, kc and kr denote the mean synthesis rates for mRNAs in the nucleus and mRNAs in the cytoplasm respectively, dc and dr are the mean degradation rates of mRNA in the nucleus and mRNA in the cytoplasm respectively. Throughout this paper, L0, Lc and Lr are called memory indices since, e.g., L0=1 corresponds to the memoryless case whereas L0≠1 to the memory case.
Let k1 be the total synthesis rate of mRNAs in the cytoplasm, which is composed of two parts: one part is the rate at which pre-mRNA generates a transcript through the AS process or the APA process, and the other is the rate at which the mature mRNA is finally exported to the cytoplasm with the help of NPC. The rate of generating mature mRNAs, determined by the gene length, is generally fixed. In contrast, the rate of exporting mRNAs to the cytoplasm may change in a large range, depending on cellular environments. This is because some types of mRNAs are exported in a fast manner due to RNA binding proteins or linked splicing factors, and other types of mRNAs are exported in a slow manner and the corresponding genes are most intron-containing ones [19]. Thus, we can use k1 to characterize the export rate indirectly. Similarly, if we let k2 be the synthesis rate of mRNAs in the nucleus, then it also includes two parts: one part is the rate of pre-mRNA produced through an AS or APA process, and the other is the rate at which transcripts are transported to some sub-cellular regions (e.g., nuclear speckles or paraspeckles) under the assistance of some proteins. Here, we assume that k2 changes a little so that the involved processes are simplified. Owing to AS or APA processes, the lengths of mature mRNAs of the two kinds can be significantly different. Usually, the rate k1 is faster than the rate k2. The retention and export of transcripts are random, we introduce another parameter pr, called remaining probability throughout this paper, to characterize this randomness. Then, the practical export rate and the practical retention rate should be kc=k1(1−pr) and kr=k2pr respectively, where pr∈(0,1).
Based on the experimental data from Halpern's group [26] that measured the whole genome-wide catalogue of nuclear and cytoplasmic mRNA from MIN6 cells, we know that most genes (~70%) has more cytoplasmic transcripts than nuclear transcripts. Thus, we can get an approximate formula for remaining probability pr: pr=Nn/Nn(Nn+Nc)(Nn+Nc) where Nn is the number of transcripts in the nucleus and Nc the number of transcripts in the cytoplasm. By considering gene INS1 for which the value of ratio Nc/NcNnNn is the maximal (13.2±4.6), we can know that the value of pr is about 5%. In that paper, the authors also mentioned that about 30% of the genes in MIN6 cells have more transcripts in the nucleus than in the cytoplasm. By considering gene ChREBP for which the value of ratio cytoplasm/nucleus is about 0.05, we can know that the value of pr is about 95%. Therefore, the range of remaining probability (pr) in the whole genome is about 5~95%. It is reasonable that the 50% value of pr is set as a threshold. For convenience, we categorize models of eukaryotic gene expression into two classes: one class where the RNE process is dominant and the other class where the RNR process is dominant. For the former, pr<0.5 holds, implying that most mRNAs are exported to the cytoplasm through nuclear pores, whereas for the latter, pr>0.5 holds, implying that most mRNAs retain in the nucleus.
In the following analysis, memory indices Li (i=0,c,r), and remaining probability pr will be taken as key parameters while the other parameter values will be kept fixed. Without loss of generality, we assume that two degradation rates for mRNAs in the nucleus and cytoplasm are equal and denote by d the common degradation rate, i.e., dc=dr=d.
First, if we let xi represent the concentration of reactive species Mi, i.e., xi=limmi→∞Ω→∞mi/miΩΩ (i=1,2,3) where Ω represents the volume of the system, then the rate equations corresponding to the constructed-above Markov reaction network can be expressed as
dxdt=SK(x), | (6) |
where x=(x1,x2,x3)T is a column vector, S=(Sij)3×5=(⟨B⟩−1−100010−100010−1) is a matrix, and K(x)=(K1(x),K2(x),K3(x),K4(x),K5(x))T is a column vector of effective transition rates. The stead states or equilibriums of the system described by Eq (6), denote by xS, are determined by solving the algebraic equation group, i.e., SK(xS)=0.
Second, if denoting by ⟨X⟩ the mean of random variable X and taking approximation ⟨Mi⟩≈xSi (i=1,2,3), then we can derive the following matrix equation (see Appendix A):
ASΣS+ΣSATS+ΩDS=0, | (7) |
where two square matrices AS=(Aij)3×3 and DS=(Dij)3×3 evaluated at steady state are known, and covariance matrix ΣS=(σij) with σij=⟨(Mi−⟨Mi⟩)(Mj−⟨Mj⟩)⟩ is unknown. Note that diagonal elements σ22 and σ33 represent variances for random variables M2 (corresponding to mRNA in the cytoplasm) and M3 (corresponding to mRNA in the nucleus), which are our interest. In addition, we can also derive formulae similar to Eq (3) in the case of continuous variables.
In order to show the explicit effect of molecular memory on the mRNA expression in different biological processes, we consider two special cases: 1) The process of gene activation with memory and other processes are memoryless. 2) The process of nuclear RNA export with memory and other processes are memoryless. In other cases, there are in general no analytical results.
Case 1 L0≠1, Lc=1 and Lr=1. In this case, the five effect transition rates become: K1(x)=(kcx1+krx1+dx2+dx3)(k0)L0(kcx1+krx1+dx2+dx3+k0)L0−(k0)L0, K2(x)=kcx1, K3(x)=krx1, K4(x)=dx2, and K5(x)=dx3. Note that in the case of continuous variables, the corresponding effect transition rates Ki(x) (1⩽i⩽5) have the same expressions except for variable notations.
We can show that the means of mRNAs in the nucleus and in the cytoplasm are given respectively by (see Appendix B)
⟨M2⟩=˜k0˜kc,⟨M3⟩=˜k0˜kr, | (8) |
where ˜k0=12[(1+2⟨B⟩)1/1L0L0−1]k0kc+kr>0 with ⟨B⟩ being the expectation of burst size B (random variable), ˜kc=kcd and ˜kr=krd. Apparently, ⟨Mi⟩ (i=2,3) is a monotonically decreasing function of memory index L0, implying that molecular memory always reduces the mRNA expression level in the nucleus and cytoplasm. In addition, by noting kc=k1(1−pr) and kr=k2pr, we can know that if k2/k2k1k1 is fixed, then ⟨M3⟩ (i.e., the mean of mRNAs in the nucleus) is a monotonically increasing function of remaining probability pr whereas ⟨M2⟩ (i.e., the mean of mRNAs in the cytoplasm) is a monotonically decreasing function of pr. In addition, ⟨M3⟩ is a monotonically decreasing function of ρ=kc/kckrkr whereas ⟨M2⟩ is a monotonically increasing function of ρ. These results are in agreement with intuition.
Interestingly, we find that σ22 and σ33, the variances for mRNAs in the nucleus and in the cytoplasm resepctively, have the following relationship (see Appendix B)
σ22=(˜kr˜kc)2σ33+˜k0(˜kc+˜kr)˜kc˜kr, | (9) |
indicating that the mRNA variance in the cytoplasm, σ22, is larger than that in the nucleus, σ33.
From the viewpoint of experiments, the cytoplasmic mRNAs are easy to measure whereas the cytoplasmic mRNAs are difficult to measure. Therefore, we are interested in the cytoplasmic mRNA expression (including the level and noise). By complex calculations, we can further show that the cytoplasmic mRNA variance is given by
σ22=˜k0˜kc˜k3r˜k3c+˜k3r{2+˜kc˜kr+122˜b+[γ−(1+˜b)2](˜kc+˜kr)1+(1+˜b)(1+2˜b)(˜kc+˜kr)}. | (10) |
where ˜b=14⟨B⟩[L0+2(L0−1)⟨B⟩−L0(1+2⟨B⟩)(L0−1)/(L0−1)L0L0]>0 and γ=⟨B2⟩+⟨B⟩⟨B⟩ with ⟨B2⟩ being the second-order raw moment of burst size B. Furthermore, if we define the noise intensity as the ratio of the variance over the squared mean, then the noise intensity for the cytoplasmic mRNA, denoted by ηc, can be analytically expressed as
ηc=1˜k0˜kc˜k3r˜k3c+˜k3r{2+˜kc˜kr+122˜b+[γ−(1+˜b)2](˜kc+˜kr)1+(1+˜b)(1+2˜b)(˜kc+˜kr)}. | (11) |
Note that if L0=1, which correspond to the Markov case, then ˜k0=k0⟨B⟩kc+kr and ˜b=0. Thus, the cytoplasmic mRNA noise in the Markov case, denoted by ηc|L0=1, is given by
ηc|L0=1=1˜k0˜kc˜k3r˜k3c+˜k3r(˜kc+2˜kr˜kr+γ2˜kc+˜kr1+˜kc+˜kr). | (12) |
Therefore, the ratio of the noise in non-Markov (L0≠1) case over that in the Markov (L0=1) case
ηcηc|L0=1={˜kc+2˜kr˜kr+122˜b+[γ−(1+˜b)2](˜kc+˜kr)1+(1+˜b)(1+2˜b)(˜kc+˜kr)}(˜kc+2˜kr˜kr+γ2˜kc+˜kr1+˜kc+˜kr)−1, | (13) |
which may be more than the unit but may also be less than the unit, depending on the size of remaining probability. However, if L0 is large enough (e.g., L0>2), then the ratio in Eq (13) is always larger than the unit, implying that molecular memory amplifies the cytoplasmic mRNA noise.
Case 2 L0=1, Lc≠1 and Lr=1. In this case, five effect transition rates reduce to K1(x)=k0, K2(x)=(k0+krx1+dx2+dx3)(kcx1)Lc(k0+kcx1+krx1+dx2+dx3)Lc−(kcx1)Lc, K3(x)=kcx1, K4(x)=dx2, and K5(x)=dx3. It seems to us there are no analytical results as in Case 1. However, if pr=0 (i.e., if we do not consider nuclear retention), then we can show that the steady state is given by x1=k0k1ω,x2=k0⟨B⟩d,x3=0, where ω=(1+⟨B⟩)⟨B⟩1/1LcLc(1+2⟨B⟩)1/1LcLc−⟨B⟩1/1LcLc is a factor depending on both transcriptional burst and molecular memory. Moreover, the mRNA noise in the cytoplasm is given by (see Appendix C for derivation)
ηc=d(1+⟨B⟩)2k0⟨B⟩2dω(1+ω+⟨B⟩)+γLck1⟨B⟩k1(1+2⟨B⟩)dω(1+ω+⟨B⟩)+Lc⟨B⟩(1+2⟨B⟩)[dω+k1(1+⟨B⟩)]. | (15) |
In order to see the contribution of molecular memory to the cytoplasmic mRNA noise, we calculate the ratio of the noise in non-Markov (Lc≠1) case over that in the Markov (Lc=1) case
ηcηc|Lc=1=d+k12d+γk1(1+⟨B⟩)[2dω(1+ω+⟨B⟩)+γLck1⟨B⟩k1(1+2⟨B⟩)]dω(1+ω+⟨B⟩)+Lc⟨B⟩(1+2⟨B⟩)[dω+k1(1+⟨B⟩)], | (16) |
which is in general larger than the unit for large Lc>1 (corresponding to strong memory), indicating that molecular memory in general enlarges the cytoplasmic mRNA noise.
Here we numerically investigate the effect of molecular memory (Lc) from nuclear RNA export on the cytoplasm mRNA (M2) in the cases that the other model parameter values are fixed. Numerical results are demonstrated in Figure 3. From Figure 3(a), we observe that the mean level of the cytoplasm mRNA is a monotonically decreasing function of Lc, independent of the value choice of remaining probability (pr) (even though we only show the two cases of pr values). This is in agreement with our intuition since a more reaction step for mRNA synthesis inevitably leads to less mRNA molecules. On the other hand, we observe from Figure 3(b) that molecular memory reduces the cytoplasm mRNA noise (ηc) for smaller values of Lc but enlarges ηc for larger values of Lc, implying that there is an optimal Lc such that ηc arrives at the minimum. We emphasize that the dependences shown in Figure 3(a), (b) are qualitative since they are independent of the value choice of remaining probability.
Importantly, Figure 3 indicates that the results obtained by the linear noise approximation (solid lines) are well in agreement with the results obtained by the Gillespie algorithm [36]. Therefore, the linear noise approximation can be used in fast evaluation of the expression noise, and in the following, we will focus on results obtained by the linear noise approximation. In addition, we point out that most results obtained here and thereafter are qualitative since they are independent of the choice of parameter values. However, to demonstrate interesting phenomena clearly, we will choose special values of some model parameters.
Here we focus on numerically investigating joint effects of molecular memory (Lc) and remaining probability (pr) on the cytoplasm mRNA (M2). Figure 4(a) demonstrates effects of pr on the M2 noise for three values of Lc. We observe that with the increase of remaining probability, the cytoplasmic mRNA noise (ηc) first decreases and then increases, implying that there is a critical pr such that ηc arrives at the minimum (referring to empty circles in Figure 4(a)) or that remaining probability can minimize the cytoplasmic mRNA noise. Moreover, this minimum is independent of the values of memory index Lc. In addition, we find that the minimal ηc first increases and then decreases with increasing Lc (the inset of Figure 4(a)). In other words, noise of cRNA can derive a optimal value with the decrease of remaining probability and the increase of memory index. Figure 4(b) shows the dependences of ηc on Lc for three different values of remaining probability. We find that molecular memory can also make cytoplasmic mRNA noise reach the minimum (referring to empty circles in Figure 4(b)), and this minimal noise is monotonically increasing function of pr.
Here we focus on numerically analyzing joint effects of memory index L0 and remaining probability pr on the cytoplasm mRNA (M2). Figure 5(a) demonstrates effects of pr on the M2 noise for two representative values of L0 (note: L0=1 corresponds to the memoryless case whereas L0=2 corresponds to the memory case). We observe that with the increase of remaining probability, the cytoplasmic mRNA noise (ηc) first decreases and then increases, implying that there is a critical pr such that ηc reaches the minimum (referring to empty circles in Figure 5(a)) or that remaining probability can minimize the cytoplasmic mRNA noise. Moreover, this minimum (referring to empty circles) is a monotonically increasing function of memory index L0.
Figure 5(b) demonstrates that the cytoplasmic mRNA noise (ηc) is always a monotonically increasing function of memory index L0, independent of remaining probability. In addition, we observe that ηc is a monotonically increasing function of remaining probability (this can be seen by comparing three lines).
Here we consider the case that RNR is a multistep process, i.e., Lr≠1. Numerical results are demonstrated in Figure 6. We observe from Figure 6(a) that except for the case of Lr=1, which corresponds to the Markov process and for which the cytoplasmic mRNA noise (ηc) is a monotonically increasing function of remaining probability (pr), the dependences of ηc on pr are not monotonic in the cases of Lr≠1 (corresponding to non-Markov processes) but there is a threshold of pr such that ηc reaches the minimum (referring to empty circles), similarly to the case of Figure 5(a). Moreover, this minimal noise is a monotonically decreasing function of memory index Lr (referring to the inset of Figure 6(a)) but the monotonicity is opposite to that in the case of Figure 5(a).
Figure 6(b) shows how the cytoplasmic mRNA noise (ηc) depends on memory index Lr for two different values of remaining probability. Interestingly, we observe that there is an optimal value of Lr such that the cytoplasmic mRNA noise reaches the minimum. Moreover, the minimal ηc is a monotonically decreasing function of remaining probability (pr), referring to the inset in the bottom right-hand corner.
Gene transcription in eukaryotes involve many molecular processes, some of which are well known and others are little known and even unknown [37,38]. In this paper, we have introduced a non-Markov model of stochastic transcription, which simultaneously considers RNA nuclear retention and nuclear RNA export processes and in which we have used non-exponential waiting-time distributions (e.g., Gamma distributions) to model some unknown or unspecified molecular processes involved in, e.g., the synthesis of pre-mRNA and the export of mRNAs generated in the nucleus to the cytoplasm and the retention of mRNA in the nucleus. Since non-exponential waiting times can lead to non-Markov kinetics, we have introduced effective transition rates for the reactions underlying transcription to transform a mathematically difficult issue to a mathematically tractable one. As a result, we have derived the analytical expressions of mRNA means and noise in the nucleus and cytoplasm, which revealed the importance of molecular memory in controlling or fine-tuning the expressions of two kinds of mRNA. Our modeling and analysis provided a heuristic framework for studying more complex gene transcription processes.
Our model considered main events occurring in gene transcription such as bursty expression (burst size follows a general distribution), alternative splicing (by which two kinds of transcripts are generated), RNR (a part of RNA molecules that are kept in the nucleus) and RNE (another part of RNA molecules that are exported to the cytoplasm). Some popular experimental technologies such as single-cell sequence data [39], single-molecule fluorescence in-situ hybridization (FISH) [40] and electron micrographs (EM) of fixed cells [41] have indicated that RNR and NRE are two complex biochemical processes, each involving regulation by a large number of proteins or complexes [42]. In particular, the mRNAs exported to the cytoplasm involve the structure of nuclear pore complex (NPC) [43]. A number of challenging questions still remain unsolved, e.g., how do RNR and NRE cooperatively regulate the expressions of nuclear and cytoplasmic mRNAs? Why are these two dynamical processes necessary for the whole gene-expression processes when the cells survive in complex environments? And what advantages do they have in contrast to a single NRE process?
Despite simple, our model can not only reproduce results for pre-mRNA (nascent mRNA) means at steady state in previous studies but also give results in agreement with experimental data on the mRNA Fano factors (define as the ratio of variance over mean) of some genes. However, we point out that some results of Fano factors obtained using our model is not always in agreement with the experimental data, e.g., for five genes, RBP3, TAF5, TAF6, TAF12 and KAP104, results obtained by our model seem not in agreement with experimental data but results obtained by a previous theoretical model [44] seems better (data are not shown). In addition, for the PRB8 gene, results on Fano factor, obtained by our model and the previous model, are poorly in agreement with experimental data (data are not shown). This indicates that constructing a theoretical model for the whole transcription process still needs more work.
In spite of differences, our results are wholly in agreement with some experimental data or observations. First, the qualitative result that RNR always reduces the nuclear pre-mRNA noise and always amplifies the cytoplasmic mRNA noise is in agreement with some experimental observations [28,42,45] and also with intuition since the retention naturally increases the mean number of the nuclear pre-mRNAs but decreases the mean number of the cytoplasmic mRNAs. Second, we compare our theoretical predictions with experimental results [28,45]. Specifically, we use previously published experimental data for two yeast genes, RBP2 and MDN1 [28,45] to calculate the cytoplasmic mRNA Fano factors. Parameter k1 is set as k1≈0.29±0.013/min, which is based on experimental data [28] and the degradation rates of the cytoplasmic mRNAs for RBP2 and MDN1 are set according to dc=ln2/ln2t1/2t1/2, where t1/2 is an experimental mRNA half-life. Then, we can find that the results on the Fano factors of genes RBP2 and MDN1 are well in agreement with the experimental data [45].
At the whole genome scale, about 70% mRNAs in the nucleus are transported to the cytoplasm whereas about 30% mRNAs are retained in the nucleus [26]. This fact implies that the changing range of remaining probability is moderate or small. In addition, the nuclear export rate of a different gene is in general different. If this rate is not too large, then following the increase of remaining probability, the increase in the cytoplasmic mRNA noise is inevitable. This result indirectly interprets the reason why the noise at the protein level is quite large as shown in previous studies of gene expression [46].
Finally, for some genes, the relative changing ranges of remaining probability and nuclear export rate may be large at the transcription level. In this case, it is in theory sufficient that adjusting one of nuclear export rate and remaining probability can fine-tune the cytoplasmic mRNA noise if the mean burst size is fixed, but differences would exist between theoretical and experimental results since NRE and RNR occur simultaneously in gene expression and are functionally cooperative. In addition, since biological regulation may be different from the theoretical assumption made here, the nuclear or cytoplasmic mRNA noise predicted in theory may be overestimated.
This work was partially supported by National Nature Science Foundation of China (11931019), and Key-Area Research and Development Program of Guangzhou (202007030004).
All authors declare no conflicts of interest in this paper.
First, the chemical master equation for the constructed Markov reaction network reads
∂P(m;t)∂t=(m1∑i=0αiE−i1−I)[K1(m)P(m;t)]+(E1E−12−I)[K2(m)P(m;t)] +(E1E−13−I)[K3(m)P(m;t)]+∑j∈{4,5}{(Ej−I)[Kj(m)P(m;t)]}, | (A1) |
Second, the stead state or equilibrium of the system described by Eq (6) in the main text, denoted by xS=(xS1,xS2,xS3)T, can be obtained by solving the algebraic equation group
⟨B⟩K1(xS)−K2(xS)−K3(xS)=0K2(xS)−K4(xS)=0K3(xS)−K5(xS)=0 | (A2) |
Then, we perform the Ω-expansions [47] to derive a Lyapunov matrix equation for covariance matrix between Mi and Mj with i,j=1,2,3, i.e., for matrix Σ=(⟨(Mi−⟨Mi⟩)T(Mj−⟨Mj⟩)⟩)≡(σij). Note that
Ki(n)=Ki(x+Ω−1/−122z)=Ki(x)+Ω−1/−122∑j=p,c,rzj∂Ki(x)∂xj+o(Ω−1),i=1,2,3 | (A3a) |
m1∑i=0αiE−i1−I=−Ω−1/−122m1∑i=0iαi∂∂z1+12Ω−1m1∑i=0i2αi∂2∂z21+o(Ω−3/−322), | (A3b) |
E1E−12−I=−Ω−1/−122(∂∂z2−∂∂z1)+12Ω−1(∂2∂z21+∂2∂z22−2∂2∂z1∂z2)+o(Ω−3/−322), | (A3c) |
E1E−13−I=−Ω−1/−122(∂∂z3−∂∂z1)+12Ω−1(∂2∂z21+∂2∂z23−2∂2∂z1∂z3)+o(Ω−3/−322), | (A3d) |
Ej−I=−Ω−1/−122∂∂zj+12Ω−1∂2∂z2j+o(Ω−3/−322),j=2,3. | (A3e) |
Hereafter o(y) represents the infinitestmal quantity of the same order as y→0. We denote by Π(z;t) the probability density function for new random variable z. Then, the relationship between variables P(m;t) and Π(z;t) is
∂P(m;t)∂t=∂Π(z;t)∂t−Ω1/122∑i=1,2,3dxidt∂Π(z;t)∂zi. | (A4) |
By substituting Eqs (A3) and (A4) into Eq (A1) and comparing the coefficients of Ω1/122, we have
∑i=1,2,3dxidt∂Π(z;t)∂zi=⟨B⟩K1(x)∂Π(z;t)∂z1+K2(x)(∂Π(z;t)∂z2−∂Π(z;t)∂z1) +K3(x)(∂Π(z;t)∂z3−∂Π(z;t)∂z1)+∑j∈{2,3}Kj∂Π(z;t)∂zj, | (A5) |
which naturally holds due to Eq (6) in the main text, where ⟨B⟩=m1∑i=0iαi is the mean burst size. Comparing the coefficients of Ω0, we have
∂Π(z;t)∂t=−⟨B⟩∑j=1,2,3∂K1(x)∂xj∂[zjΠ(z;t)]∂z1−∑j=1,2,3∂K2(x)∂xj(∂[zjΠ(z;t)]∂z2−∂[zjΠ(z;t)]∂z1)−∑j=1,2,3∂K3(x)∂xj(∂[zjΠ(z;t)]∂z3−∂[zjΠ(z;t)]∂z1)−∑j∈{2,3}∑k=1,2,3∂Kj(x)∂xk∂[zkΠ(z;t)]∂zj+12⟨B2⟩K1(x)∂2Π(z;t)∂z21+12K2(x)(∂2Π(z;t)∂z21+∂2Π(z;t)∂z22−2∂2Π(z;t)∂z1∂z2)+12K3(x)(∂2Π(z;t)∂z21+∂2Π(z;t)∂z23−2∂2Π(z;t)∂z1∂z3)+12∑j∈{2,3}Kj∂2Π(z;t)∂z2j | (A6) |
where ⟨B2⟩=m1∑i=0i2αi is the second moment of burst size. Since Ki(x) is independent of z, Eq (A6) can be rewritten as
∂Π(z;t)∂t=−∑i,j∈{1,2,3}Aij∂[zjΠ(z;t)]∂zi+12∑i,j∈{1,2,3}Dij∂2Π(z;t)∂zi∂zj, | (A7) |
where the elements of matrix A=(Aij) take the form
A=(Aij)=(⟨B⟩∂K1∂x1−∂K2∂x1−∂K3∂x1⟨B⟩∂K1∂x2−∂K2∂x2−∂K3∂x2⟨B⟩∂K1∂x3−∂K2∂x3−∂K3∂x3∂K2∂x1−∂K4∂x1∂K2∂x2−∂K4∂x2∂K2∂x3−∂K4∂x3∂K3∂x1−∂K5∂x1∂K3∂x2−∂K5∂x2∂K3∂x3−∂K5∂x3) | (A8a) |
and matrix D=(Dij) takes the form
D=(⟨B2⟩K1+K2+K3−K2−K3−K2K2+K40−K30K3+K5). | (A8b) |
If we consider the stationary equation of Eq (A7), denote by AS and DS the corresponding matrices.
Third, the steady-state Fokker Planck equation allows a solution of the following form
Π(z)=1√(2π)3det(ΣS)exp(−12zTΣ−1Sz). | (A9) |
Here, matrix ΣS=(⟨(M−xS)T(M−xS)⟩)≡(σij) (covariance matrix) is determined by solving the following Lyapunov matrix equation
ASΣS+ΣSATS+DS=0. | (A10) |
Note that the diagonal elements of matrix ΣS are just the variances of the state variables, and the vector of the mean concentrations of the reactive species is given approximately by ⟨M⟩≈xS. Eq (A10) is an extension of the linear noise approximation in the Markov case [48].
In this case, we can show that effective transition rates are given by K1(x)=(x1kc+x1kr+x2d+x3d)(k0)L0(x1kc+x1kr+x2d+x3d+k0)L0−(k0)L0, K2(x)=x1kc, K3(x)=x1kr, K4(x)=x2d, and K5(x)=x3d, where x=(x1,x2,x3)T. Thus, accoridng to Eq (A2), we know that the steady state is given by
xs1=ak0kc+kr,xs2=kcdxs1,xs3=krdxs1, | (B1) |
where a=(1+2⟨B⟩)1/1L0L0−12. Note that
∂K1(x)∂x1=(kc+kr)(k0)L0[k0(1+2a)]L0−(k0)L0−2ak0L0(kc+kr)(k0)L0[k0(1+2a)]L0−1{[k0(1+2a)]L0−(k0)L0}2 |
Therefore,
∂K1(x)∂x1|x=xS=∂K1(xS)∂x1=(kc+kr)2⟨B⟩−L0[(1+2⟨B⟩)−(1+2⟨B⟩)(L0−1)/(L0−1)L0L0]4⟨B⟩2. | (B2a) |
Completely similarly, we have
∂K1(x)∂x2=∂K1(x)∂x3=d2⟨B⟩−L0[(1+2⟨B⟩)−(1+2⟨B⟩)(L0−1)/(L0−1)L0L0]4⟨B⟩2. | (B2b) |
Thus, matrix AS reduces to
A=(Aij)=((b⟨B⟩−1)(kc+kr)bd⟨B⟩bd⟨B⟩kc−d0kr0−d), | (B3) |
where b=−14⟨B⟩2[L0+2(L0−1)⟨B⟩−L0(1+2⟨B⟩)(L0−1)/(L0−1)L0L0]. Meanwhile, the matrix DS in Eq (A10) becomes
DS=˜k0(γ(kc+kr)−kc−kr−kc2kc0−kr02kr), | (B4) |
where ˜k0=ak0kc+kr and γ=⟨B2⟩+⟨B⟩⟨B⟩. We can directly derive the following relationships from Eq (A10):
(b⟨B⟩−1)(kc+kr)σ11+bd⟨B⟩(σ12+σ13)=−˜k02γ(kc+kr) | (B5a) |
(b⟨B⟩−1)(kc+kr)σ12+bd⟨B⟩σ22+bd⟨B⟩σ23+kcσ11−dσ12=˜k0kc | (B5b) |
(b⟨B⟩−1)(kc+kr)σ13+bd⟨B⟩σ23+bd⟨B⟩σ33+krσ11−dσ13=˜k0kr | (B5c) |
and obtain the following relationships
σ12=dkcσ22−˜k0, σ13=dkrσ33−˜k0 and σ23=12(krkcσ22+kckrσ33)−kc+kr2d˜k0. |
Substituting these relationships into Eq (B4a)–Eq (B4c) yields
(b⟨B⟩−1)(kc+kr)σ11+bd⟨B⟩(dkcσ22+dkrσ33)=2bd⟨B⟩˜k0−˜k02γ(kc+kr), | (B6a) |
kcdσ11+(2b⟨B⟩−1+3b⟨B⟩−22krkc−dkc)σ22+b⟨B⟩2kckrσ33=kcd˜k0+[3b⟨B⟩−22kc+krd−1]˜k0, | (B6b) |
krdσ11+b⟨B⟩2krkcσ22+(2b⟨B⟩−1+3b⟨B⟩−22kckr−dkr)σ33=krd˜k0+[3b⟨B⟩−22kc+krd−1]˜k0. | (B6c) |
The combination of Eq (B6a), (B6b) gives
σ22=(krkc)2σ33+kckrkc+krd˜k0, or σ33=(kckr)2(σ22−kc+krdkckr˜k0). | (B7a) |
The sum of Eq (B6b), (B6c) gives
kc+krdσ11+[(2b⟨B⟩−1)kc+krd−1](dkcσ22+dkrσ33)=[3b⟨B⟩−12kc+krd−1]˜k0. | (B7b) |
The combination of Eq (B7b) and (B6a) yields
dkcσ22+dkrσ33=1−b⟨B⟩+12[(b⟨B⟩−1)2−γ]kc+krd1+(b⟨B⟩−1)(2b⟨B⟩−1)kc+krd | (B7c) |
By substituting this equation into Eq (B7a), we finally obtain
σ22=˜k0˜kc˜k3r˜k3c+˜k3r{2+˜kc˜kr+122˜b+[γ−(1+˜b)2](˜kc+˜kr)1+(1+˜b)(1+2˜b)(˜kc+˜kr)}, | (B8a) |
and further
σ33=˜k0˜kr˜k3c˜k3c+˜k3r{1−˜k3c˜k3r−˜k4c˜k4r+122˜b+[γ−(1+˜b)2](˜kc+˜kr)1+(1+˜b)(1+2˜b)(˜kc+˜kr)}. | (B8b) |
where ˜b=−b⟨B⟩=14⟨B⟩[L0+2(L0−1)⟨B⟩−L0(1+2⟨B⟩)(L0−1)/(L0−1)L0L0]>0 with b<0, ˜kc=kcd, ˜kr=krd, γ=⟨B2⟩+⟨B⟩⟨B⟩.
Thus, the cytoplasmic mRNA noise is given by
ηc=σ22⟨M2⟩2=1˜k0˜kc˜k3r˜k3c+˜k3r{1+˜kc+˜kr˜kr+122˜b+[γ−(1+˜b)2](˜kc+˜kr)1+(1+˜b)(1+2˜b)(˜kc+˜kr)}, | (B9a) |
and the nuclear mRNA noise in the nucleus by
ηr=σ33⟨M3⟩2=1˜k0˜kr˜k3c˜k3c+˜k3r{1−˜k3c˜k3r−˜k4c˜k4r+122˜b+[γ−(1+˜b)2](˜kc+˜kr)1+(1+˜b)(1+2˜b)(˜kc+˜kr)}. | (B9b) |
In this case, we can show that five effect transition rates take the forms: K1(x)=k0, K2(x)=(k0+x1kr+dx2+dx3)(x1kc)Lc(k0+x1kc+x1kr+dx2+dx3)Lc−(x1kc)Lc, K3(x)=x1kr, K4(x)=dx2, and K5(x)=dx3. In order to derive analytical results, we assume that remaining probability is so small that pr≈0, implying kr=0, kc=k1 and K3(x)=0. By solving the steady-state deterministic equation
{⟨B⟩K1−K2−K3=0K2−K4=0K3−K5=0, | (C1) |
we obtain the analytical expression of steady state (xS) given by
xS1=k0(1+⟨B⟩)⟨B⟩1/1LcLck1[(1+2⟨B⟩)1/1LcLc−⟨B⟩1/1LcLc], xS2=k0⟨B⟩d and xS3=0. | (C2) |
Note that the elements of Jacob matrix in the linear noise approximation reduce to
a11=−∂K2(xS)∂x1, a12=−∂K2(xS)∂x2, a13=−∂K2(xS)∂x3, a21=∂K2(xS)∂x1, a22=∂K2(xS)∂x2−d, a23=∂K2(xS)∂x3, a31=0, a32=0, and a33=−d. Defferentiating function K2(x) with regard to x1 yields
∂K2(x)∂x1=Lc(k0+x2d)(k1)Lc(x1)Lc−1(k0+x1k1+x2d)Lc−(x1k1)Lc−Lc(k0+x2d)(x1k1)Lc[k1(k0+x1k1+x2d)Lc−1−(k1)Lc(x1)Lc−1][(k0+x1k1+x2d)Lc−(x1k1)Lc]2. |
Therefore,
∂K2(x)∂x1|x=xS=−∂K2(xS)∂x1=Lck0⟨B⟩x1[1+2⟨B⟩1+⟨B⟩−⟨B⟩1+⟨B⟩(1+2⟨B⟩⟨B⟩)(Lc−1)/(Lc−1)LcLc]. | (C3) |
Completely similarly, we have
∂K2(x)∂x2=∂K2(x)∂x3=d⟨B⟩1+⟨B⟩−dLc⟨B⟩21+⟨B⟩k0k1x1(1+2⟨B⟩⟨B⟩)(Lc−1)/(Lc−1)LcLc. | (C4) |
Furthermore, the Jacob matrix becomes
As=(a11a12a12−a11−a12−d−a1200−d), | (C5) |
where a11 = −∂K2(xS)∂x1 and a12 = −∂K2(xS)∂x2 are given by Eqs (C3) and (C4).
Meanwhile, the matrix Ds in the linear noise approximation is given by
Ds=(k0⟨B2⟩+k0⟨B⟩−K20−K22K20000). | (C6) |
It follows from matrix equation ASΣS+ΣSATS+DS=0 that
σ11=−k0⟨B2⟩+k0⟨B⟩2a11−a12a211K2+a12(a12+d)a211σ22, | (C7a) |
σ22=k0−a11(⟨B2⟩+⟨B⟩)+2d⟨B⟩2d(a12+d−a11). | (C7b) |
Substituting the expressions of a11 and a12 into Eq (C7b) yields
σ22=k0⟨B⟩2dLck1ω1+2⟨B⟩1+ω+⟨B⟩(⟨B2⟩+⟨B⟩)+2dd1+⟨B⟩+Lc⟨B⟩(1+2⟨B⟩)1+ω+⟨B⟩(d1+⟨B⟩+k1ω), | (C8) |
where ω=(1+⟨B⟩)⟨B⟩1/1LcLc(1+2⟨B⟩)1/1LcLc−⟨B⟩1/1LcLc.
[1] |
Lehman N (2015) The RNA World: 4,000,000,050 years old. Life 5: 1583-1586. doi: 10.3390/life5041583
![]() |
[2] |
Díaz Arenas C, Lehman N (2010) Quasispecies-like behavior observed in catalytic RNA populations evolving in a test tube. BMC Evol Biol 10: 80. doi: 10.1186/1471-2148-10-80
![]() |
[3] |
Yeates JAM, Lehman N (2016) RNA networks at the origins of life. Biochemist 38: 8-12. doi: 10.1042/BIO03802008
![]() |
[4] |
Di Mauro E, Saladino R, Trifonov EN (2014) The path to life's origins. Remaining hurdles. J Biomol Struct Dyn 32: 512-522. doi: 10.1080/07391102.2013.783509
![]() |
[5] |
Adamski P, Eleveld M, Sood A, et al. (2020) From self-replication to replicator systems en route to de novo life. Nat Rev Chem 4: 386-403. doi: 10.1038/s41570-020-0196-x
![]() |
[6] |
Vaidya N, Walker SI, Lehman N (2013) Recycling of informational units leads to selection of replicators in a prebiotic soup. Chem Biol 20: 241-252. doi: 10.1016/j.chembiol.2013.01.007
![]() |
[7] |
French RK, Holmes EC (2020) An ecosystems perspective on virus evolution and emergence. Trends Microbiol 28: 165-175. doi: 10.1016/j.tim.2019.10.010
![]() |
[8] |
Villarreal LP, Witzany G (2013) Rethinking quasispecies theory: From fittest type to cooperative consortia. World J Biol Chem 4: 79-90. doi: 10.4331/wjbc.v4.i4.79
![]() |
[9] |
Janzen E, Blanco C, Peng H, et al. (2020) Promiscuous ribozymes and their proposed role in prebiotic evolution. Chem Rev 120: 4879-4897. doi: 10.1021/acs.chemrev.9b00620
![]() |
[10] |
Furubayashi T, Ueda K, Bansho Y, et al. (2020) Emergence and diversification of a host-parasite RNA ecosystem through Darwinian evolution. Elife 9: e56038. doi: 10.7554/eLife.56038
![]() |
[11] |
Witzany G (2011) The agents of natural genome editing. J Mol Cell Biol 3: 181-189. doi: 10.1093/jmcb/mjr005
![]() |
[12] | Witzany G (2019) Communication as the Main Characteristic of Life. Handbook of Astrobiology Boka Raton: CrC Press, 91-105. |
[13] |
Villarreal LP, Witzany G (2019) That is life: communicating RNA networks from viruses and cells in continuous interaction. Ann NY Acad Sci 1447: 5-20. doi: 10.1111/nyas.14040
![]() |
[14] |
Harish A, Caetano-Anollés G (2012) Ribosomal history reveals origins of modern protein synthesis. PLoS One 7: e32776. doi: 10.1371/journal.pone.0032776
![]() |
[15] |
Root-Bernstein M, Root-Bernstein R (2015) The ribosome as a missing link in the evolution of life. J Theor Biol 367: 130-158. doi: 10.1016/j.jtbi.2014.11.025
![]() |
[16] |
Pak D, Root-Bernstein R, Burton ZF (2017) tRNA structure and evolution and standardization to the three nucleotide genetic code. Transcription 8: 205-219. doi: 10.1080/21541264.2017.1318811
![]() |
[17] |
Mizuno CM, Guyomar C, Roux S, et al. (2019) Numerous cultivated and uncultivated viruses encode ribosomal proteins. Nat Commun 10: 752. doi: 10.1038/s41467-019-08672-6
![]() |
[18] | Villarreal LP (2009) Origin of Group Identity: Viruses, Addiction and Cooperation New York: Springer. |
[19] |
Villarreal LP, Witzany G (2015) When competing viruses unify: evolution, conservation, and plasticity of genetic identities. J Mol Evol 80: 305-318. doi: 10.1007/s00239-015-9683-y
![]() |
[20] | Hayden EJ, Lehman N, Unrau P (2019) Transitions. RNA and Ribozymes in the Development of Life. Handbook of Astrobiology Boka Raton: CrC Press, 379-394. |
[21] | Villarreal LP (2004) Can Viruses Make Us Human? Proc Am Phl Soc 148: 296-323. |
[22] | Villarreal LP (2015) Can Virolution Help Us Understand Recent Human Evolution? Astrobiology. An Evolutionary Approach Boca Raton: CRC Press, 441-472. |
[23] |
Gemmell P, Hein J, Katzourakis A (2019) The exaptation of HERV-H: evolutionary analyses reveal the genomic features of highly transcribed elements. Front Immunol 10: 1339. doi: 10.3389/fimmu.2019.01339
![]() |
[24] |
Larouche JD, Trofimov A, Hesnard L, et al. (2020) Widespread and tissue-specific expression of endogenous retroelements in human somatic tissues. Genome Med 12: 40. doi: 10.1186/s13073-020-00740-7
![]() |
[25] |
Witzany G (2006) Natural genome-editing competences of viruses. Acta Biotheor 54: 235-253. doi: 10.1007/s10441-006-9000-7
![]() |
[26] |
Witzany G (2009) Noncoding RNAs: persistent viral agents as modular tools for cellular needs. Ann NY Acad Sci 1178: 244-267. doi: 10.1111/j.1749-6632.2009.04989.x
![]() |
[27] |
Villarreal LP, Witzany G (2010) Viruses are essential agents within the roots and stem of the tree of life. J Theor Biol 262: 698-710. doi: 10.1016/j.jtbi.2009.10.014
![]() |
[28] |
Manrubia SC, Briones C (2007) Modular evolution and increase of functional complexity in replicating RNA molecules. RNA 13: 97-107. doi: 10.1261/rna.203006
![]() |
[29] |
Villarreal LP (2005) Viruses and The Evolution of Life Washington: ASM Press. doi: 10.1128/9781555817626
![]() |
[30] |
Villarreal LP (2009) Persistence pays: how viruses promote host group survival. Curr Opin Microbiol 12: 467-472. doi: 10.1016/j.mib.2009.06.014
![]() |
[31] |
Gómez J, Ariza-Mateos A, Cacho I (2015) Virus is a Signal for the Host Cell. Biosemiotics 8: 483-491. doi: 10.1007/s12304-015-9245-0
![]() |
[32] |
Feschotte C (2008) Transposable elements and the evolution of regulatory networks. Nat Rev Genet 9: 397-405. doi: 10.1038/nrg2337
![]() |
[33] |
Moelling K, Broecker F (2019) Viruses and Evolution - Viruses First? A Personal Perspective. Front Microbiol 10: 523. doi: 10.3389/fmicb.2019.00523
![]() |
[34] | Ryan F (2009) Virolution London: William Collins. |
[35] | Villarreal LP (2006) Virus-host symbiosis mediated by persistence. Symbiosis 44: 1-9. |
[36] |
Villarreal LP (2008) The Widespread Evolutionary Significance of Viruses. Origin and Evolution of Viruses New York: Academic Press, 477-516. doi: 10.1016/B978-0-12-374153-0.00021-7
![]() |
[37] | Villareal LP, Ryan F (2011) Viruses in host evolution: General principles and future extrapolations. Curr Top Virol 9: 79-90. |
[38] |
Pereira L, Rodrigues T, Carrapiço F (2012) A Symbiogenic Way in the Origin of Life. In The Beginning: Precursors of Life, Chemical Models and Early Biological Evolution, Cellular Origin, Life in Extreme Habitats and Astrobiology Dordrecht: Springer, 723-742. doi: 10.1007/978-94-007-2941-4_36
![]() |
[39] |
Villarreal LP, Witzany G (2018) Editorial: Genome Invading RNA-Networks. Frontiers Microbiol 9: 581. doi: 10.3389/fmicb.2018.00581
![]() |
[40] |
Domingo E, Sheldon J, Perales C (2012) Viral quasispecies evolution. Microbiol Mol Biol Rev 76: 159-216. doi: 10.1128/MMBR.05023-11
![]() |
[41] |
Vaidya N, Manapat ML, Chen IA, et al. (2012) Spontaneous network formation among cooperative RNA replicators. Nature 491: 72-77. doi: 10.1038/nature11549
![]() |
[42] |
Higgs PG, Lehman N (2015) The RNA World: molecular cooperation at the origins of life. Nat Rev Genet 16: 7-17. doi: 10.1038/nrg3841
![]() |
[43] |
Nelson JW, Breaker RR (2017) The lost language of the RNA World. Sci Signal 10: eaam8812. doi: 10.1126/scisignal.aam8812
![]() |
[44] |
Villarreal LP (2015) Force for ancient and recent life: viral and stemloop RNA consortia promote life. Ann NY Acad Sci 1341: 25-34. doi: 10.1111/nyas.12565
![]() |
[45] |
Krupovic M, Bamford DH (2009) Does the evolution of viral polymerases reflect the origin and evolution of viruses? Nat Rev Microbiol 7: 250. doi: 10.1038/nrmicro2030-c1
![]() |
[46] |
Forterre P, Prangishvili D (2013) The major role of viruses in cellular evolution: facts and hypotheses. Curr Opin Virol 3: 558-565. doi: 10.1016/j.coviro.2013.06.013
![]() |
[47] | Wolf YI, Kazlauskas D, Iranzo J, et al. (2018) Origins and evolution of the Global RNA virome. mBio 9: e02329-18. |
[48] |
Feschotte C, Gilbert C (2012) Endogenous viruses: insights into viral evolution and impact on host biology. Nat Rev Genet 13: 283-296. doi: 10.1038/nrg3199
![]() |
[49] |
Vignuzzi M, López CB (2019) Defective viral genomes are key drivers of the virus-host interaction. Nat Microbiol 4: 1075-1087. doi: 10.1038/s41564-019-0465-y
![]() |
[50] |
Koonin EV, Dolja VV, Krupovic M (2015) Origins and evolution of viruses of eukaryotes: The ultimate modularity. Virology 479–480: 2-25. doi: 10.1016/j.virol.2015.02.039
![]() |
[51] |
Briones C, Stich M, Manrubia SC (2009) The dawn of the RNA world: toward functional complexity through ligation of random RNA oligomers. RNA 15: 743-749. doi: 10.1261/rna.1488609
![]() |
[52] |
Tjhung KF, Shokhirev MN, Horning DP, et al. (2020) An RNA polymerase ribozyme that synthesizes its own ancestor. Proc Natl Acad Sci USA 117: 2906-2913. doi: 10.1073/pnas.1914282117
![]() |
[53] |
Petrov AS, Bernier CR, Hsiao C, et al. (2014) Evolution of the ribosome at atomic resolution. Proc Natl Acad Sci USA 111: 10251-10256. doi: 10.1073/pnas.1407205111
![]() |
[54] | Villarreal LP, Witzany G (2013) The DNA Habitat and its RNA Inhabitants: At the Dawn of RNA Sociology. Genomics Ins 6: 1-12. |
[55] | Bertalanffy L, Laviolette PA (1981) A Systems View of Man New York: Routledge. |
[56] |
Schudoma C, Larhlimi A, Walther D (2011) The influence of the local sequence environment on RNA loop structures. RNA 17: 1247-1257. doi: 10.1261/rna.2550211
![]() |
[57] |
Witzany G (2020) What is Life? Front Astron Space Sci 7: 7. doi: 10.3389/fspas.2020.00007
![]() |
[58] |
Root-Bernstein RS, Merrill SJ (1997) The necessity of cofactors in the pathogenesis of AIDS: a mathematical model. J Theor Biol 187: 135-146. doi: 10.1006/jtbi.1997.0449
![]() |
[59] |
Witzany G (2014) RNA sociology: group behavioral motifs of RNA consortia. Life 4: 800-818. doi: 10.3390/life4040800
![]() |
[60] |
Witzany G (2014) Pragmatic turn in biology: From biological molecules to genetic content operators. World J Biol Chem 5: 279-285. doi: 10.4331/wjbc.v5.i3.279
![]() |
[61] |
Schudoma C (2011) It's a loop world—single strands in RNA as structural and functional elements. Biomol Conc 2: 171-181. doi: 10.1515/bmc.2011.016
![]() |
[62] |
Bergthaler A, Menche J (2017) The immune system as a social network. Nat Immunol 18: 481-482. doi: 10.1038/ni.3727
![]() |
[63] |
Witzany G (2016) Crucial steps to life: From chemical reactions to code using agents. Biosystems 140: 49-57. doi: 10.1016/j.biosystems.2015.12.007
![]() |
[64] |
Koonin EV (2016) Viruses and mobile elements as drivers of evolutionary transitions. Philos Trans R Soc Lond B Biol Sci 371: 20150442. doi: 10.1098/rstb.2015.0442
![]() |
[65] |
Sullivan MB, Weitz JS, Wilhelm S (2017) Viral ecology comes of age. Environ Microbiol Rep 9: 33-35. doi: 10.1111/1758-2229.12504
![]() |
[66] |
Bushman FD (2003) Targeting survival: Integration site selection by retroviruses and LTR-retrotransposons. Cell 115: 135-138. doi: 10.1016/S0092-8674(03)00760-8
![]() |
[67] |
Hayden EJ, Lehman N (2006) Self-assembly of a group I intron from inactive oligonucleotide fragments. Chem Biol 13: 909-918. doi: 10.1016/j.chembiol.2006.06.014
![]() |
[68] |
Bartel DP (2009) MicroRNAs: target recognition and regulatory functions. Cell 136: 215-233. doi: 10.1016/j.cell.2009.01.002
![]() |
[69] |
Belfort M, Curcio MJ, Lue NF (2011) Telomerase and retrotransposons: reverse transcriptases that shaped genomes. Proc Natl Acad Sci USA 108: 20304-20310. doi: 10.1073/pnas.1100269109
![]() |
[70] |
Pyle AM (2016) Group II Intron Self-Splicing. Annu Rev Biophys 45: 183-205. doi: 10.1146/annurev-biophys-062215-011149
![]() |
[71] |
Villarreal LP (2011) Viruses and host evolution: virus-mediated self identity. Adv Exp Med Biol 738: 185-217. doi: 10.1007/978-1-4614-1680-7_12
![]() |
[72] |
Villarreal LP (2012) The addiction module as a social force. Viruses: Essential Agents of Life Dordrecht: Springer Science + Business Media, 107-146. doi: 10.1007/978-94-007-4899-6_6
![]() |
[73] |
Villarreal LP (2016) Persistent virus and addiction modules: an engine of symbiosis. Curr Opin Microbiol 31: 70-79. doi: 10.1016/j.mib.2016.03.005
![]() |
[74] |
Kobayashi I (2001) Behavior of restriction-modification systems as selfish mobile elements and their impact on genome evolution. Nucleic Acids Res 29: 3742-3756. doi: 10.1093/nar/29.18.3742
![]() |
[75] |
Mruk I, Kobayashi I (2014) To be or not to be: regulation of restriction-modification systems and other toxin-antitoxin systems. Nucleic Acids Res 42: 70-86. doi: 10.1093/nar/gkt711
![]() |
[76] |
Gerdes K, Wagner EG (2007) RNA antitoxins. Curr Opin Microbiol 10: 117-124. doi: 10.1016/j.mib.2007.03.003
![]() |
[77] |
Witzany G (2020) Evolution of Genetic Information without Error Replication. Theoretical Information Studies: Information in the World Singapore: World Scientific, 295-320. doi: 10.1142/9789813277496_0014
![]() |
[78] |
Schuster P (2011) Mathematical modeling of evolution. Solved and open problems. Theory Biosci 130: 71-89. doi: 10.1007/s12064-010-0110-z
![]() |
[79] |
Eigen M (1971) Selforganization of matter and the evolution of biological macromolecules. Naturwissenschaften 58: 465-523. doi: 10.1007/BF00623322
![]() |
[80] |
Koonin EV (2009) On the origin of cells and viruses: primordial virus world scenario. Ann N Y Acad Sci 1178: 47-64. doi: 10.1111/j.1749-6632.2009.04992.x
![]() |
[81] |
Comeau AM, Hatfull GF, Krisch HM, et al. (2008) Exploring the prokaryotic virosphere. Res Microbiol 159: 306-313. doi: 10.1016/j.resmic.2008.05.001
![]() |
[82] |
Zhang YZ, Chen YM, Wang W, et al. (2019) Expanding the RNA Virosphere by Unbiased Metagenomics. Annu Rev Virol 6: 119-139. doi: 10.1146/annurev-virology-092818-015851
![]() |
[83] |
Moelling K (2013) What contemporary viruses tell us about evolution: a personal view. Arch Virol 158: 1833-1848. doi: 10.1007/s00705-013-1679-6
![]() |
[84] |
Stedman KM (2015) Deep recombination: RNA and ssDNA virus genes in DNA virus and host genomes. Annu Rev Virol 2: 203-217. doi: 10.1146/annurev-virology-100114-055127
![]() |
[85] |
Orgel LE, Crick FH (1980) Selfish DNA: the ultimate parasite. Nature 284: 604-607. doi: 10.1038/284604a0
![]() |
[86] |
Doolittle WF, Sapienza C (1980) Selfish genes, the phenotype paradigm and genome evolution. Nature 284: 601-603. doi: 10.1038/284601a0
![]() |
[87] |
Volff JN (2006) Turning junk into gold: domestication of transposable elements and the creation of new genes in eukaryotes. Bioessays 28: 913-922. doi: 10.1002/bies.20452
![]() |
[88] |
Biémont C, Vieira C (2006) Genetics: junk DNA as an evolutionary force. Nature 443: 521-524. doi: 10.1038/443521a
![]() |
[89] |
Ryan F (2006) Genomic creativity and natural selection: a modern synthesis. Biol J Linn Soc 88: 655-672. doi: 10.1111/j.1095-8312.2006.00650.x
![]() |
[90] |
Root-Bernstein RS, Dillon PF (1997) Molecular complementarity I: the complementarity theory of the origin and evolution of life. J Theor Biol 188: 447-749. doi: 10.1006/jtbi.1997.0476
![]() |
[91] |
Stadler PF, Schuster P (1992) Mutation in autocatalytic reaction networks. An analysis based on perturbation theory. J Math Biol 30: 597-631. doi: 10.1007/BF00948894
![]() |
[92] |
Naville M, Volff JN (2016) Endogenous Retroviruses in Fish Genomes: From Relics of Past Infections to Evolutionary Innovations? Front Microbiol 7: 1197. doi: 10.3389/fmicb.2016.01197
![]() |
[93] |
Broecker F, Moelling K (2019) Evolution of Immune Systems. From Viruses and Transposable Elements. Front Microbiol 10: 51. doi: 10.3389/fmicb.2019.00051
![]() |
[94] |
Naville M, Warren IA, Haftek-Terreau Z, et al. (2016) Not so bad after all: retroviruses and long terminal repeat retrotransposons as a source of new genes in vertebrates. Clin Microbiol Infect 22: 312-323. doi: 10.1016/j.cmi.2016.02.001
![]() |
[95] | Cech TR (2012) The RNA worlds in context. Cold Spring Harb Perspect Biol 4: a006742. |
[96] |
Eigen M, Schuster P (1977) A principle of natural self-organization. Naturwissenschaften 64: 541-565. doi: 10.1007/BF00450633
![]() |
[97] |
Szathmáry E (1993) Coding coenzyme handles: a hypothesis for the origin of the genetic code. Proc Natl Acad Sci USA 90: 9916-9920. doi: 10.1073/pnas.90.21.9916
![]() |
[98] |
Domingo E, Schuster P (2016) Quasispecies: From Theory to Experimental Systems Cham: Springer. doi: 10.1007/978-3-319-23898-2
![]() |
[99] |
Domingo E, Grande-Pérez A, Martín V (2008) Future prospects for the treatment of rapidly evolving viral pathogens: insights from evolutionary biology. Expert Opin Biol Ther 8: 1455-1460. doi: 10.1517/14712598.8.10.1455
![]() |
[100] |
Villarreal LP (2016) Viruses and the placenta: the essential virus first view. APMIS 124: 20-30. doi: 10.1111/apm.12485
![]() |
[101] |
Chaudhari N, Hahn WE (1983) Genetic expression in the developing brain. Science 220: 924-928. doi: 10.1126/science.6189184
![]() |
[102] |
Khaitovich P, Muetzel B, She X, et al. (2004) Regional patterns of gene expression in human and chimpanzee brains. Genome Res 14: 1462-1473. doi: 10.1101/gr.2538704
![]() |
[103] |
Watanabe H, Fujiyama A, Hattori M, et al. (2004) DNA sequence and comparative analysis of chimpanzee chromosome 22. Nature 429: 382-388. doi: 10.1038/nature02564
![]() |
[104] |
Wetterbom A, Sevov M, Cavelier L (2006) Comparative genomic analysis of human and chimpanzee indicates a key role for indels in primate evolution. J Mol Evol 63: 682-690. doi: 10.1007/s00239-006-0045-7
![]() |
[105] |
Mills RE, Luttig CT, Larkins CE, et al. (2006) An initial map of insertion and deletion (INDEL) variation in the human genome. Genome Res 16: 1182-1190. doi: 10.1101/gr.4565806
![]() |
[106] | Mager DL, Stoye JP (2014) Mammalian endogenous retroviruses. Microbiol Spectrum 3: MDNA3-0009-2014. |
[107] |
Sakate R, Suto Y, Imanishi T, et al. (2007) Mapping of chimpanzee full-length cDNAs onto the human genome unveils large potential divergence of the transcriptome. Gene 399: 1-10. doi: 10.1016/j.gene.2007.04.013
![]() |
[108] |
Zeh DW, Zeh JA, Ishida Y (2009) Transposable elements and an epigenetic basis for punctuated equilibria. Bioessays 31: 715-726. doi: 10.1002/bies.200900026
![]() |
[109] |
Keightley PD, Lercher MJ, Eyre-Walker A (2005) Evidence for widespread degradation of gene control regions in hominid genomes. PLoS Biol 3: e42. doi: 10.1371/journal.pbio.0030042
![]() |
[110] |
Keightley PD, Lercher MJ, Eyre-Walker A (2006) Understanding the degradation of hominid gene control. PLoS Comput Biol 2: e19. doi: 10.1371/journal.pcbi.0020019
![]() |
[111] |
Toder R, Grützner F, Haaf T, et al. (2001) Species-specific evolution of repeated DNA sequences in great apes. Chromosome Res 9: 431-435. doi: 10.1023/A:1011605824530
![]() |
[112] |
Bird CP, Stranger BE, Liu M, et al. (2007) Fast-evolving noncoding sequences in the human genome. Genome Biol 8: R118. doi: 10.1186/gb-2007-8-6-r118
![]() |
[113] |
Bejerano G, Haussler D, Blanchette M (2004) Into the heart of darkness: large-scale clustering of human non-coding DNA. Bioinformatics 20: i40-48. doi: 10.1093/bioinformatics/bth946
![]() |
[114] |
Bush EC, Lahn BT (2005) Selective constraint on noncoding regions of hominid genomes. PLoS Comput Biol 1: e73. doi: 10.1371/journal.pcbi.0010073
![]() |
[115] |
Barry G, Mattick JS (2012) The role of regulatory RNA in cognitive evolution. Trends Cogn Sci 16: 497-503. doi: 10.1016/j.tics.2012.08.007
![]() |
[116] |
Mattick JS (2009) Deconstructing the dogma: a new view of the evolution and genetic programming of complex organisms. Ann N Y Acad Sci 1178: 29-46. doi: 10.1111/j.1749-6632.2009.04991.x
![]() |
[117] |
Mattick JS (2011) The central role of RNA in human development and cognition. FEBS Lett 585: 1600-1616. doi: 10.1016/j.febslet.2011.05.001
![]() |
[118] |
Mattick JS (2005) The functional genomics of noncoding RNA. Science 309: 1527-1528. doi: 10.1126/science.1117806
![]() |
[119] |
Mattick JS, Taft RJ, Faulkner GJ (2010) A global view of genomic information--moving beyond the gene and the master regulator. Trends Genet 26: 21-28. doi: 10.1016/j.tig.2009.11.002
![]() |
[120] |
Claverie JM (2005) Fewer genes, more noncoding RNA. Science 309: 1529-1530. doi: 10.1126/science.1116800
![]() |
[121] |
Khalil AM, Guttman M, Huarte M, et al. (2009) Many human large intergenic noncoding RNAs associate with chromatin-modifying complexes and affect gene expression. Proc Natl Acad Sci USA 106: 11667-11672. doi: 10.1073/pnas.0904715106
![]() |
[122] |
Mehler MF, Mattick JS (2007) Noncoding RNAs and RNA editing in brain development, functional diversification, and neurological disease. Physiol Rev 87: 799-823. doi: 10.1152/physrev.00036.2006
![]() |
[123] |
Mattick JS, Mehler MF (2008) RNA editing, DNA recoding and the evolution of human cognition. Trends Neurosci 31: 227-233. doi: 10.1016/j.tins.2008.02.003
![]() |
[124] |
Mercer TR, Dinger ME, Mariani J, et al. (2008) Noncoding RNAs in Long-Term Memory Formation. Neuroscientist 14: 434-445. doi: 10.1177/1073858408319187
![]() |
[125] |
Nghe P, Hordijk W, Kauffman SA, et al. (2015) Prebiotic network evolution: six key parameters. Mol Biosyst 11: 3206-3217. doi: 10.1039/C5MB00593K
![]() |
[126] |
Kaliatsi EG, Giarimoglou N, Stathopoulos C, et al. (2020) Non-Coding RNA-Driven Regulation of rRNA Biogenesis. Int J Mol Sci 21: 9738. doi: 10.3390/ijms21249738
![]() |
[127] |
Shepherd JD (2018) Arc-An endogenous neuronal retrovirus? Semin Cell Dev Biol 77: 73-78. doi: 10.1016/j.semcdb.2017.09.029
![]() |
[128] |
Pastuzyn ED, Day CE, Kearns RB, et al. (2018) The neuronal gene arc encodes a repurposed retrotransposon gag protein that mediates intercellular RNA transfer. Cell 172: 275-288. doi: 10.1016/j.cell.2017.12.024
![]() |
[129] |
Vignuzzi M, Stone JK, Arnold JJ, et al. (2006) Quasispecies diversity determines pathogenesis through cooperative interactions in a viral population. Nature 439: 344-348. doi: 10.1038/nature04388
![]() |
[130] |
Korboukh VK, Lee CA, Acevedo A, et al. (2014) RNA virus population diversity, an optimum for maximal fitness and virulence. J Biol Chem 289: 29531-29544. doi: 10.1074/jbc.M114.592303
![]() |
[131] |
Briones C, de Vicente A, Molina-París C, et al. (2006) Minority memory genomes can influence the evolution of HIV-1 quasispecies in vivo. Gene 384: 129-138. doi: 10.1016/j.gene.2006.07.037
![]() |
[132] | Briones C, Domingo E (2008) Minority report: hidden memory genomes in HIV-1 quasispecies and possible clinical implications. AIDS Rev 10: 93-109. |
[133] |
Bordería AV, Stapleford KA, Vignuzzi M (2011) RNA virus population diversity: implications for inter-species transmission. Curr Opin Virol 1: 643-648. doi: 10.1016/j.coviro.2011.09.012
![]() |
[134] |
Grande-Pérez A, Lázaro E, Lowenstein P, et al. (2005) Suppression of viral infectivity through lethal defection. Proc Natl Acad Sci USA 102: 4448-4452. doi: 10.1073/pnas.0408871102
![]() |
[135] |
Domingo E, Gomez J (2007) Quasispecies and its impact on viral hepatitis. Virus Res 127: 131-150. doi: 10.1016/j.virusres.2007.02.001
![]() |
[136] |
Ojosnegros S, Perales C, Mas A, et al. (2011) Quasispecies as a matter of fact: viruses and beyond. Virus Res 162: 203-215. doi: 10.1016/j.virusres.2011.09.018
![]() |
[137] |
Lauring AS, Andino R (2010) Quasispecies theory and the behavior of RNA viruses. PLoS Pathog 6: e1001005. doi: 10.1371/journal.ppat.1001005
![]() |
[138] |
Arbiza J, Mirazo S, Fort H (2010) Viral quasispecies profiles as the result of the interplay of competition and cooperation. BMC Evol Biol 10: 137. doi: 10.1186/1471-2148-10-137
![]() |
[139] |
Brooks K, Jones BR, Dilernia DA, et al. (2020) HIV-1 variants are archived throughout infection and persist in the reservoir. PLoS Pathog 16: e1008378. doi: 10.1371/journal.ppat.1008378
![]() |
[140] |
Levin SR, Gandon S, West SA (2020) The social coevolution hypothesis for the origin of enzymatic cooperation. Nat Ecol Evol 4: 132-137. doi: 10.1038/s41559-019-1039-3
![]() |
[141] | Atkins JF, Loughran G, Bhatt PR, et al. (2016) Ribosomal frameshifting and transcriptional slippage: From genetic steganog- raphy and cryptography to adventitious use. Nucleic Acids Res 44: 7007-7078. |
[142] |
Urtel GC, Rind T, Braun D (2017) Reversible Switching of Cooperating Replicators. Phys Rev Lett 118: 078102. doi: 10.1103/PhysRevLett.118.078102
![]() |
[143] | Bastet L, Turcotte P, Wade JT, et al. (2018) Maestro of regulation: Riboswitches orchestrate gene expression at the levels of translation, transcription and mRNA decay. RNA Biol 15: 679-682. |
[144] | Gesteland RF, Cech TR, Atkins JF (2005) The RNA World New York: Cold Spring Harbor Laboratory Press. |
[145] | Altman S Rna008-BioTheory (2013) .Available from: http://tbio.molpit.ru/main-results/rna-world/rna008. |
[146] |
Holmes EC (2013) What can we predict about viral evolution and emergence? Curr Opin Virol 3: 180-184. doi: 10.1016/j.coviro.2012.12.003
![]() |
[147] |
Wildschutte JH, Williams ZH, Montesion M, et al. (2016) Discovery of unfixed endogenous retrovirus insertions in diverse human populations. Proc Natl Acad Sci USA 113: E2326-2334. doi: 10.1073/pnas.1602336113
![]() |
[148] |
Randau L, Söll D (2008) Transfer RNA genes in pieces. EMBO Rep 9: 623-628. doi: 10.1038/embor.2008.101
![]() |
[149] |
Tamura K (2015) Origins and early evolution of the tRNA molecule. Life 5: 1687-1699. doi: 10.3390/life5041687
![]() |
[150] | DeFarias ST, Rêgo TG, José MV (2019) Origin of the 16S ribosomal molecule from ancestor tRNAs. Sciences 1: 8. |
[151] |
Daly T, Chen XS, Penny D (2011) How old are RNA networks? Adv Exp Med Biol 722: 255-273. doi: 10.1007/978-1-4614-0332-6_17
![]() |
[152] |
Frenkel-Pinter M, Haynes JW, Mohyeldin AM, et al. (2020) Mutually stabilizing interactions between proto-peptides and RNA. Nat Commun 11: 3137. doi: 10.1038/s41467-020-16891-5
![]() |
[153] |
Yarus M (2011) The meaning of a minuscule ribozyme. Philos Trans R Soc Lond B Biol Sci 366: 2902-2909. doi: 10.1098/rstb.2011.0139
![]() |
[154] |
Martin LL, Unrau PJ, Müller UF (2015) RNA synthesis by in vitro selected ribozymes for recreating an RNA world. Life 5: 247-268. doi: 10.3390/life5010247
![]() |
[155] |
Smit S, Yarus M, Knight R (2006) Natural selection is not required to explain universal compositional patterns in rRNA secondary structure categories. RNA 12: 1-14. doi: 10.1261/rna.2183806
![]() |
[156] |
Yarus M (2011) Getting past the RNA world: the initial Darwinian ancestor. Cold Spring Harb Perspect Biol 3: a003590. doi: 10.1101/cshperspect.a003590
![]() |
[157] |
Vitas M, Dobovišek A (2018) In the Beginning was a Mutualism - On the Origin of Translation. Orig Life Evol Biosph 48: 223-243. doi: 10.1007/s11084-018-9557-6
![]() |
[158] |
Müller S, Appel B, Krellenberg T, et al. (2012) The many faces of the hairpin ribozyme: structural and functional variants of a small catalytic RNA. IUBMB Life 64: 36-47. doi: 10.1002/iub.575
![]() |
[159] |
Cheng LK, Unrau PJ (2010) Closing the circle: replicating RNA with RNA. Cold Spring Harb Perspect Biol 2: a002204. doi: 10.1101/cshperspect.a002204
![]() |
[160] |
Gwiazda S, Salomon K, Appel B, et al. (2012) RNA self-ligation: from oligonucleotides to full length ribozymes. Biochimie 94: 1457-1463. doi: 10.1016/j.biochi.2012.03.015
![]() |
[161] |
Behrouzi R, Roh JH, Kilburn D, et al. (2012) Cooperative tertiary interaction network guides RNA folding. Cell 149: 348-357. doi: 10.1016/j.cell.2012.01.057
![]() |
[162] | Ferré-D'Amaré AR, Scott WG (2010) Small self-cleaving ribozymes. Cold Spring Harb Perspect Biol 2: a003574. |
[163] |
Breaker RR (2018) Riboswitches and Translation Control. Cold Spring Harb Perspect Biol 10: a032797. doi: 10.1101/cshperspect.a032797
![]() |
[164] |
Matzke MA, Mosher RA (2014) RNA-directed DNA methylation: an epigenetic pathway of increasing complexity. Nat Rev Genet 15: 394-408. doi: 10.1038/nrg3683
![]() |
[165] |
Perreault J, Weinberg Z, Roth A, et al. (2011) Identification of hammerhead ribozymes in all domains of life reveals novel structural variations. PLoS Comput Biol 7: e1002031. doi: 10.1371/journal.pcbi.1002031
![]() |
[166] |
Kumar RM, Joyce GF (2003) A modular, bifunctional RNA that integrates itself into a target RNA. Proc Natl Acad Sci USA 100: 9738-9743. doi: 10.1073/pnas.1334190100
![]() |
[167] |
Maizels N, Weiner AM (1994) Phylogeny from function: evidence from the molecular fossil record that tRNA originated in replication, not translation. Proc Natl Acad Sci USA 91: 6729-6734. doi: 10.1073/pnas.91.15.6729
![]() |
[168] |
de Farias ST, José MV (2020) Transfer RNA: The molecular demiurge in the origin of biological systems. Prog Biophys Mol Biol 53: 28-34. doi: 10.1016/j.pbiomolbio.2020.02.006
![]() |
[169] |
Demongeot J, Seligmann H (2019) More pieces of ancient than recent theoretical minimal proto-tRNA-like RNA rings in genes coding for tRNA synthetases. J Mol Evol 87: 152-174. doi: 10.1007/s00239-019-09892-6
![]() |
[170] |
Root-Bernstein R (2012) A modular hierarchy-based theory of the chemical origins of life based on molecular complementarity. Acc Chem Res 45: 2169-2177. doi: 10.1021/ar200209k
![]() |
[171] | Dick TP, Schamel WA (1995) Molecular evolution of transfer RNA from two precursor hairpins: implications for the origin of protein synthesis. J Mol Evol 41: 1-9. |
[172] |
Belfort M, Weiner A (1997) Another bridge between kingdoms: tRNA splicing in archaea and eukaryotes. Cell 89: 1003-1006. doi: 10.1016/S0092-8674(00)80287-1
![]() |
[173] |
Lambowitz AM, Zimmerly S (2011) Group II introns: mobile ribozymes that invade DNA. Cold Spring Harb Perspect Biol 3: a003616. doi: 10.1101/cshperspect.a003616
![]() |
[174] |
Fujishima K, Kanai A (2014) tRNA gene diversity in the three domains of life. Front Genet 5: 142. doi: 10.3389/fgene.2014.00142
![]() |
[175] |
Rodin AS, Szathmáry E, Rodin SN (2011) On origin of genetic code and tRNA before translation. Biol Direct 6: 14. doi: 10.1186/1745-6150-6-14
![]() |
[176] |
Wolf YI, Koonin EV (2007) On the origin of the translation system and the genetic code in the RNA world by means of natural selection, exaptation, and subfunctionalization. Biol Direct 2: 14. doi: 10.1186/1745-6150-2-14
![]() |
[177] |
Sun FJ, Caetano-Anollés G (2008) Transfer RNA and the origins of diversified life. Sci Prog 91: 265-284. doi: 10.3184/003685008X360650
![]() |
[178] |
Petrov AS, Gulen B, Norris AM, et al. (2015) History of the ribosome and the origin of translation. Proc Natl Acad Sci USA 112: 15396-15401. doi: 10.1073/pnas.1509761112
![]() |
[179] |
Di Giulio M (2013) A polyphyletic model for the origin of tRNAs has more support than a monophyletic model. J Theor Biol 318: 124-128. doi: 10.1016/j.jtbi.2012.11.012
![]() |
[180] |
Liow LH, Van Valen L, Stenseth NC (2011) Red Queen: from populations to taxa and communities. Trends Ecol Evol 26: 349-358. doi: 10.1016/j.tree.2011.03.016
![]() |
[181] |
Hayne CK, Schmidt CA, Haque MI, et al. (2020) Reconstitution of the human tRNA splicing endonuclease complex: insight into the regulation of pre-tRNA cleavage. Nucleic Acids Res 48: 7609-7622. doi: 10.1093/nar/gkaa438
![]() |
[182] |
Bowman JC, Petrov AS, Frenkel-Pinter M, et al. (2020) Root of the Tree: The Significance, Evolution, and Origins of the Ribosome. Chem Rev 120: 4848-4878. doi: 10.1021/acs.chemrev.9b00742
![]() |
[183] |
Root-Bernstein R, Root-Bernstein M (2019) The Ribosome as a Missing Link in Prebiotic Evolution III: Over-Representation of tRNA- and rRNA-Like Sequences and Plieofunctionality of Ribosome-Related Molecules Argues for the Evolution of Primitive Genomes from Ribosomal RNA Modules. Int J Mol Sci 20: 140. doi: 10.3390/ijms20010140
![]() |
[184] | Arenas CD, Lehman N (2010) The continuous evolution in vitro technique. Curr Protoc Nucleic Acid Chem Chapter 9: 9.7.1-9.7.17. |
[185] |
Dadon Z, Samiappan M, Wagner N, et al. (2012) Chemical and light triggering of peptide networks under partial thermodynamic control. Chem Commun (Camb) 48: 1419-1421. doi: 10.1039/C1CC14301H
![]() |
[186] | Ameta S, Arsène S, Foulon S, et al. (2019) Darwinian properties and their trade-offs in autocatalytic RNA reaction networks. bioRxiv Available from: https://doi.org/10.1101/726497. |
[187] |
Hordijk W, Steel M (2012) Predicting template-based catalysis rates in a simple catalytic reaction model. J Theor Biol 295: 132-138. doi: 10.1016/j.jtbi.2011.11.024
![]() |
[188] |
Jalasvuori M, Bamford JK (2008) Structural co-evolution of viruses and cells in the primordial world. Orig Life Evol Biosph 38: 165-181. doi: 10.1007/s11084-008-9121-x
![]() |
[189] |
Kun Á, Szilágyi A, Könnyű B, et al. (2015) The dynamics of the RNA world: insights and challenges. Ann NY Acad Sci 1341: 75-95. doi: 10.1111/nyas.12700
![]() |
[190] |
Rieckmann JC, Geiger R, Hornburg D, et al. (2017) Social network architecture of human immune cells unveiled by quantitative proteomics. Nat Immunol 18: 583-593. doi: 10.1038/ni.3693
![]() |
[191] | Caetano-Anollés G, Sun FJ (2014) The natural history of transfer RNA and its interactions with the ribosome. Front Genet 5: 127. |
[192] |
Thornlow BP, Armstrong J, Holmes AD, et al. (2020) Predicting transfer RNA gene activity from sequence and genome context. Genome Res 30: 85-94. doi: 10.1101/gr.256164.119
![]() |
[193] |
Withers M, Wernisch L, dos Reis M (2006) Archaeology and evolution of transfer RNA genes in the Escherichia coli genome. RNA 12: 933-942. doi: 10.1261/rna.2272306
![]() |
[194] |
Ariza-Mateos A, Briones C, Perales C, et al. (2019) The archaeology of coding RNA. Ann NY Acad Sci 1447: 119-134. doi: 10.1111/nyas.14173
![]() |
[195] |
Stevenson DS (2002) Co-evolution of the genetic code and ribozyme replication. J Theor Biol 217: 235-253. doi: 10.1006/jtbi.2002.3013
![]() |
[196] |
Witzany G (2012) From Molecular Entities to Competent Agents: Viral Infection-Derived Consortia Act as Natural Genetic Engineers. Viruses: Essential Agents of Life Dordrecht: Springer, 407-419. doi: 10.1007/978-94-007-4899-6_20
![]() |
[197] |
Hesselberth JR (2013) Lives that introns lead after splicing. Wiley Interdiscip Rev RNA 4: 677-691. doi: 10.1002/wrna.1187
![]() |
[198] |
Belfort M (2017) Mobile self-splicing introns and inteins as environmental sensors. Curr Opin Microbiol 38: 51-58. doi: 10.1016/j.mib.2017.04.003
![]() |
[199] |
Lennon CW, Belfort M (2017) Inteins. Curr Biol 27: 204-206. doi: 10.1016/j.cub.2017.01.016
![]() |
[200] | Villareal LP (2015) Virolution Can Help Us Understand the Origin of Life. Astrobiology. An Evolutionary Approach Boca Raton: CRC Press, 421-440. |
[201] | Villarreal LP, Witzany G (2021) Infectious thoughts: discovering biology as a social science. PrePrint . |
[202] |
Vandevenne M, Delmarcelle M, Galleni M (2019) RNA Regulatory Networks as a Control of Stochasticity in Biological Systems. Front Genet 10: 403. doi: 10.3389/fgene.2019.00403
![]() |
[203] |
Lozada-Chávez I, Stadler PF, Prohaska SJ (2011) Hypothesis for the modern RNA world: a pervasive non-coding RNA-based genetic regulation is a prerequisite for the emergence of multicellular complexity. Orig Life Evol Biosph 41: 587-607. doi: 10.1007/s11084-011-9262-1
![]() |
1. | Noreen Saba, Sana Maqsood, Muhammad Asghar, Ghulam Mustafa, Faheem Khan, An extended Mittag Leffler function in terms of extended Wright complex hypergeometric function, 2025, 117, 11100168, 364, 10.1016/j.aej.2024.12.065 |