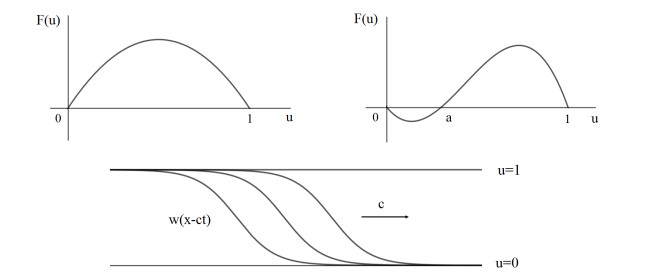
Citation: Tiziana M. Florio. Stereotyped, automatized and habitual behaviours: are they similar constructs under the control of the same cerebral areas?[J]. AIMS Neuroscience, 2020, 7(2): 136-152. doi: 10.3934/Neuroscience.2020010
[1] | Xiao-Min Huang, Xiang-ShengWang . Traveling waves of di usive disease models with time delay and degeneracy. Mathematical Biosciences and Engineering, 2019, 16(4): 2391-2410. doi: 10.3934/mbe.2019120 |
[2] | Maryam Basiri, Frithjof Lutscher, Abbas Moameni . Traveling waves in a free boundary problem for the spread of ecosystem engineers. Mathematical Biosciences and Engineering, 2025, 22(1): 152-184. doi: 10.3934/mbe.2025008 |
[3] | Danfeng Pang, Yanni Xiao . The SIS model with diffusion of virus in the environment. Mathematical Biosciences and Engineering, 2019, 16(4): 2852-2874. doi: 10.3934/mbe.2019141 |
[4] | Tiberiu Harko, Man Kwong Mak . Travelling wave solutions of the reaction-diffusion mathematical model of glioblastoma growth: An Abel equation based approach. Mathematical Biosciences and Engineering, 2015, 12(1): 41-69. doi: 10.3934/mbe.2015.12.41 |
[5] | Xixia Ma, Rongsong Liu, Liming Cai . Stability of traveling wave solutions for a nonlocal Lotka-Volterra model. Mathematical Biosciences and Engineering, 2024, 21(1): 444-473. doi: 10.3934/mbe.2024020 |
[6] | Ming Mei, Yau Shu Wong . Novel stability results for traveling wavefronts in an age-structured reaction-diffusion equation. Mathematical Biosciences and Engineering, 2009, 6(4): 743-752. doi: 10.3934/mbe.2009.6.743 |
[7] | M. B. A. Mansour . Computation of traveling wave fronts for a nonlinear diffusion-advection model. Mathematical Biosciences and Engineering, 2009, 6(1): 83-91. doi: 10.3934/mbe.2009.6.83 |
[8] | Tong Li, Zhi-An Wang . Traveling wave solutions of a singular Keller-Segel system with logistic source. Mathematical Biosciences and Engineering, 2022, 19(8): 8107-8131. doi: 10.3934/mbe.2022379 |
[9] | Dong Liang, Jianhong Wu, Fan Zhang . Modelling Population Growth with Delayed Nonlocal Reaction in 2-Dimensions. Mathematical Biosciences and Engineering, 2005, 2(1): 111-132. doi: 10.3934/mbe.2005.2.111 |
[10] | Bogdan Kazmierczak, Zbigniew Peradzynski . Calcium waves with mechano-chemical couplings. Mathematical Biosciences and Engineering, 2013, 10(3): 743-759. doi: 10.3934/mbe.2013.10.743 |
Reaction-diffusion waves arise in various applications such as ecological invasions [1], tumor growth [2], cardiovascular events [3], combustion process [4] and many others. All these very different applications can be considered as some particular cases of a general mathematical theory developing during already almost one century and studying waves existence, stability, speed of propagation, and their nonlinear dynamics. In this review, we will speak about the state of the art in one of more recent developments of this theory related to delayed reaction-diffusion equations.
In this section we will briefly recall the main results about reaction-diffusion waves for the scalar equation without delay. They will help us to present the results for the delay equations in the following sections. We consider the equation
∂u∂t=∂2u∂x2+F(u) | (1.1) |
on the whole axis x∈R with a sufficiently smooth function F(u) such that F(0)=F(1)=0. Traveling wave solution of this equation is a solution of the form u(x,t)=w(x−ct), where c is the wave speed. It is an unknown constant which should be found together with the function w>0 satisfying the equation
w″+cw′+F(w)=0 | (1.2) |
and such that
w(−∞)=1,w(∞)=0. | (1.3) |
Systematic theory of reaction-diffusion waves begins in the 1930s with the works by Fisher [5] and Kolmogorov-Petrovskii-Piskunov [1] in population dynamics, by Zeldovich and Frank-Kamenetskii in combustion theory [6], and by Semenov in chemical kinetics [7], but the first works by Mikhelson [8] and Luther [9] appeared several decades earlier.
Existence and stability of reaction-diffusion waves depend on the nonlinearity F(u). It is convenient to introduce the classification of the functions F(u) according to the stability of the stationary points u=0 and u=1 of the equation du/dt=F(u). In the bistable case, both of them are stable; in the monostable case, one point is stable while another one is unstable; in the unstable case, both of them are unstable, see Figure 1, upper row. A further classification of monostable and bistable nonlinearities can be found in section 2.1 of [10].
Wave existence. Existence of solutions of problem (1.2), (1.3) can be studied by the phase plane analysis for the corresponding system of first-order equations. In the monostable case, under the assumption that F(u)>0 for 0<u<1, monotonically decreasing solutions of this problem exist for all values of speed c greater than or equal to some minimal speed c0>0. We will see below that the monotone waves are stable, while non-monotone waves are unstable. The latter exist for all positive c. If in addition F(u)≤F′(0)u, u≥0, then c0=2√F′(0). Without the assumption that the monostable nonlinearity F(u) is positive in the interval [0,1], a solution of problem (1.2), (1.3) may not exist. If it exists, then it is possible to affirm that the [0,1]-waves, that is, solutions of Eq (1.2) with limits (1.3), exist in some interval [c0,c1) of speeds. If such waves do not exist, then the solution of the Cauchy problem for equation (1.1) is described by systems of waves (see below).
In the simple bistable case where F(u)<0 for 0<u<u0, F(u)>0 for u0<u<1, and some u0∈(0,1), there exists a solution of problem (1.2), (1.3) for a unique value of speed c. In the general bistable case, if the [0,1]-wave exists, then the speed is unique. If it does not exist, then, as before, there are systems of waves. Finally, in the unstable case, such wave does not exist.
Wave stability. There are different types of wave stability. Solution u(x,t) of Eq (1.1) with some initial condition u(x,0)=u0(x) converges to a wave w(x) in form and in speed if there exists a function m(t) such that u(x,t)→w(x−m(t)) as t→+∞, uniformly in R, and m′(t)→c. This convergence (in other terms) and the corresponding method to study it were introduced in KPP [1]. Uniform convergence implies that u(x,t)→w(x−ct−h) uniformly in R for some constant h. Convergence in form and in speed follows from the uniform convergence but the opposite may not be true.
In the monostable case, solution of the Cauchy problem converges to one of the [0,1]-waves depending on the initial condition u0(x). Namely, if u′0(x)/u0(x)→−λ as x→+∞, where λ=c/2−√c2/4−F′(0), and lim_x→−∞u0(x)>0, then the solution converges in form and in speed to the wave with the speed c≥c0. If λ≤c0/2−√c20/4−F′(0), then the convergence occurs to the wave with the minimal speed c0. More general results are also known (see [11] and the references therein). In applications, initial conditions such that u0(x)≡0 for x sufficiently large are often considered. In this case, the solution converges to the wave with the minimal speed. The uniform convergence in the monostable case occurs under some additional conditions.
In the bistable case, the [0,1]-wave is globally asymptotically stable in the sense of uniform convergence if F′(0)<0,F′(1)<0 and for a large class of initial conditions. The convergence in form and in speed occurs without the last condition on the derivatives.
In general, monotone waves for the scalar reaction-diffusion equations are stable, and non-monotone waves are unstable. This can be seen from the analysis of the spectrum. In the bistable case, it can be easily verified that the linearized operator Lv=v″+cv′+F′(w(x))v has a zero eigenvalue with the corresponding eigenfunction v0(x)=−w′(x). If w(x) is a monotonically decreasing function, then the eigenfunction v0(x) is positive. Therefore, 0 is the eigenvalue with the maximal real part (the principal eigenvalue) [12], and all other spectrum lies in the left-half plane of the complex plane. Such structure of the spectrum provides asymptotic stability of waves with shift with respect to small perturbations. If the wave is non-monotone, then the eigenfunction v0(x) has variable sign. Hence, 0 is not the principal eigenvalue, and there is a positive eigenvalue of the operator L. Thus, the wave is unstable. In the monostable case, the situation is more complex because of the essential spectrum but the result about stability of monotone waves (in certain sense) and instability of non-monotone waves remains valid.
Systems of waves. If the [0,1]-waves do not exist, then behavior of solutions of the Cauchy problem is determined by systems of waves. In order to explain this notion, consider the following example. Suppose that F(0)=F(u0)=F(1)=0 for some u0∈(0,1), and F′(0),F′(u0),F′(1)<0. Hence, we can consider the bistable [0,u0]-wave and another bistable [u0,1]-wave assuming that they exist. Denote by c1 the speed of the former and by c2 of the latter. If c1>c2, then there are two waves propagating one after another with different speeds, and the solution u(x,t) converges to a two-step function formed by these waves. If c1<c2, then the two waves merge, and there is a single [0,1]-wave. Such solutions were first studied in combustion theory. In the mathematical context they were introduced and studied in [13,14] (called there minimal decomposition of waves). For general functions F(u) they were studied in [15,16].
Monotone systems. Consider now equation (1.1) with the vector variables u=(u1,...,un), F=(F1,...,Fn). This system of equations is called a monotone system if the following inequalities
∂Fi∂uj>0,i,j=1,...,n,i≠j | (1.4) |
are satisfied for all u∈Rn. This is a class of systems for which the maximum principle and comparison theorems, conventionally used for the scalar equation, remain valid. These properties of monotone systems provide the results on the wave existence and stability similar to the results presented above for the scalar equation [12,11]. Furthermore, the minimax representation of the wave speed and the results on the systems of waves are also applicable for the monotone systems.
For a more general class of locally monotone systems, the inequalities (1.4) are supposed to hold only on the surfaces Fi=0. In this case, the maximum principle is not applicable but it is still possible to prove wave existence in the bistable case.
The fundamentals of the theory of parabolic functional differential equations (including reaction-diffusion equations with delays) were developed in the 1970s [19], with the earlier contributions by Artola [17,18] and later extensive studies in [20,21] (among many other papers: see the aforementioned works for more history and references).
Particularly, the existence and uniqueness of traveling waves for scalar equations with non-local diffusion and with reaction allowing spatiotemporal delays were already considered in 1980 by Schumacher [22]. Even so, the first systematic and comprehensive studies of wave solutions for scalar reaction-diffusion equations with discrete delays were realized by Schaaf [23] in 1987.
It is worth mentioning that the biggest part of mathematical models given by parabolic functional differential equations is related to biological and ecological applications. In fact, the celebrated Kolmogorov-Petrovskii-Piskunov-Fisher studies of propagation of advantageous genes [5,1] are often taken as a starting point for further improvements considering elaborated spatiotemporal interactions, cf. recent studies in [24,25,26,27,28]. In continuation, we touch briefly on the variety of parabolic functional differential equations that appear in the theory of traveling waves.
In the next sections, we are considering biological systems* whose evolution is determined by the interaction of a linear diffusion D and appropriate nonlinear response R incorporating delayed effects:
* Evolving in a continuous environment; note that there exists a vast bibliography on traveling waves for delayed reaction-diffusion equations describing biological models in patchy habitats [30]
∂∂tu(t,x)=Du+Ru,u∈R, x∈Rn. | (2.1) |
In the ecological models, the term Du describes random motion of individuals. The derivation of the diffusion term in (2.1) was analyzed by many authors, e.g. see [31,1,32]. In particular, Kolmogorov et al. [1] explain how random spatial walk can be naturally represented by non-local diffusion operators of the form
(Du)(t,x)=(K∗u)(t,x)−u(t,x), |
where K∗u is the space-convolution and K≥0 is some normalized (probabilistic) kernel,
(K∗u)(t,x)=∫RnK(x−y)u(t,y)dy,∫RnK(y)dy=1. |
If non-local diffusion is isotropic and short-ranged, then it can be approximated [1] by the local diffusion operator given by the Laplacian
(Du)(t,x)=kΔu(t,x), | (2.2) |
where k>0 is some diffusion coefficient. In Chapter 11 of [32], J. Murray presented a simplified random walk derivation of the diffusion operator (2.2). Furthermore, the Fokker-Planck theory provides a rigorous justification for the form (2.2).
In most biological applications, delayed Eq (2.1) is considered with Fickian diffusion (2.2) and therefore (Du)(t,x) depends only on the current values of u(t,x) (see [28,29] for other examples). Delayed continuous reaction-diffusion equation with delay-independent nonlocal diffusion were studied by many authors, see for more references [33,30,34,35,36,37].
Next, as it was first suggested by Britton [38,39], nonlocal space interactions (or, more generally, spatio-temporal competitive effects in a population) can be taken into account by considering the delayed reaction term
(Ru)(t,x)=f(u(t,x),(K∗g(u))(t−h,x)). | (2.3) |
Here f(u,v) and g(u) are some appropriate continuous functions of real variables, h>0 is time delay and K∗g(u) is the space-convolution of g(u) and some normalized non-negative kernel K. Instead, one can also consider more general spatio-temporal convolution K∗∗g(u),
(K∗∗g(u))(t,x)=∫t−∞∫RnK(x−y,t−s)g(u(s,y))dyds,∫+∞0∫RnK(y,s)dyds=1. |
There are also some other biological applications related to the analysis of age-structured populations and leading to the same form of reaction term (2.3). Based on earlier work by Smith and Thieme [40,41], corresponding theory was developed in [31,42] to analyze evolution of the total matured sub-community of a single species population with age structure and a fixed maturation period h.
In the simplified but nonetheless representative and non-trivial case when the spatial intraspecific interaction is ignored in the reaction term and the influence of the past history on the current evolution at each moment t is expressed only by the values of u(t−h,x), we obtain
(Ru)(t,x)=h(u(t,x),u(t−h,x)). |
The surveys [31,43,44] discussed a series of specific biological situations which can be modeled by equation (2.1) with D and R of the types (2.2), (2.3), respectively. Moreover, the question of traveling waves and their properties was studied in [31,44]. After the publication of the aforementioned papers, an important piece of work has been done by different authors. It includes studies of key questions of the theory concerning existence, stability, uniqueness, genesis of waves etc, by different methods and for various families of equations. Since almost each such family incorporates either Kolmogorov type equation with some appropriate specific rate of increase f(u,v) and normalized non-negative kernel K(x)
∂∂tu(t,x)=Δu(t,x)+u(t,x)f(u(t,x),(K∗u)(t−h,x)) | (2.4) |
or the diffusive Mackey-Glass type equations
∂∂tu(t,x)=Δu(t,x)−g(u(t,x))+f((K∗u)(t−h,x)), |
∂∂tu(t,x)=Δu(t,x)−g(u(t,x))+(K∗f(u))(t−h,x)) | (2.5) |
with some birth and mortality rates f(u),g(v), it is both natural and instructive to illustrate results obtained in last decade or so on the above models. This will be our main goal in the next sections, where we will be concerned with both bistable and monostable plane traveling waves u=ϕ(ν⋅x+ct), where ν∈Rn, |ν|=1, is direction of wave propagation. Planarity of wave motion simplifies its analysis which actually shows that the spatial dimension n is irrelevant in various aspects. Therefore in subsequent sections we will always assume that n=1 and x∈R.} The exposition below reflects the personal interests of the authors taking into account that it would be rather difficult to mention all recent valuable contributions to the theory of traveling waves in Eqs (2.4) and (2.5).
Equations (2.4) and (2.5) are called quasi-monotone in the case when the birth functions f(u,v),f(v) are monotonically increasing, with respect to the variable v, between the equilibria connected by the traveling wave u=ϕ(x+ct). Similarly to (1.4), this property enables direct application of the comparison principle and sliding techniques for the analysis of waves in (2.4), (2.5) and the related equations, including the following profile equations:
ϕ″(t)−cϕ′(t)+ϕ(t)f(ϕ(t),(K∗ϕ)(t−ch))=0, | (3.1) |
ϕ″(t)−cϕ′(t)−g(ϕ(t))+f((K∗ϕ)(t−ch))=0. | (3.2) |
Actually, the use of comparison arguments led to the development of general theories of wave propagations in abstract monotone evolution systems which include conveniently the quasi-monotone models (2.4), (2.5). Within an abstract setting, the bistable case was considered in [45,46] and [47] while the monostable one was studied in [48,50] (see also in [51,52] and references therein for an abstract recursive approach). In the next subsections, we discuss basic properties of our quasi-monotone models.
Assume that g,f are increasing C1-smooth functions on some interval [0,κ] and that the following monostability conditions are satisfied:
f(u)−g(u)>0,u∈(0,κ), f(0)=g(0)=0, f(κ)=g(κ), f′(0)>g′(0), f′(κ)<g′(κ). |
Depending on the boundary conditions imposed on the wave profile at ±∞, we will consider the leftward traveling waves (wavefronts)
u(t,x)=ϕ(x+ct), ϕ(−∞)=0, ϕ(+∞)=κ, |
and the rightward traveling waves
u(t,x)=ϕ(x+ct), ϕ(−∞)=κ, ϕ(+∞)=0. |
The first fundamental result concerns the structure of the set of all admissible wave speeds:
Proposition 3.1. There exist two extended real numbers (called rightward and leftward minimal speeds) c∗−,c∗+∈R∪{±∞}, c∗−<c∗+, such that (2.5) has a leftward [respectively, rightward] traveling wave with monotone profile ϕ if and only if c≥c∗+ [respectively, if c≤c∗−] [50]. Furthermore, c∗−,c∗+ are the spreading speeds in the following sense: if u(t,x,u0) solves, for Eq (2.5), the initial value problem u(s,x,u0)=u0(s,x), s∈[−τ,0], x∈R, with continuous and compactly supported initial datum u0:[−τ,0]×R→[0,κ), then
limt→+∞sup{u(t,x,u0),x∈(−∞,−c′t]∪[−c″t,+∞)}=0whenever c′>c∗+, c″<c∗−. |
On the other hand, for each ϵ∈(0,κ) and each pair (c″,c′), satisfying c∗−<c″<c′<c∗+, there exists positive rϵ such that if the initial function u0 satisfies the inequality u0(s,x)≥ϵ for all s∈[−τ,0], x∈[a,a+rϵ] and some real a, then uniformly in x
limt→+∞, x∈[−c′t,−c″t]u(t,x,u0)=κ. |
Proof. In view of Remark 4.1 in [50], the above result follows from Theorems 3.4 and 4.4 (proved by means of an abstract recursive approach) of the same paper. The waves propagating with the minimal speeds are called the minimal (or critical) waves.
Remark 3.2. In Eq (2.5), the properties of the extended numbers c∗± depend essentially on the normalized non-negative kernel K. For example, if the bilateral Laplace transform K(λ)=∫Re−λsK(s)ds is defined for all real λ, both numbers c∗± are finite and c∗−<c∗+. In general, both of these numbers can be nonpositive (or nonnegative). This means that a rightward wave can actually propagate in the the left direction (in such a case, we obtain an extinction wavefront instead of an invasion wave). However, if K is an even (symmetric) function this situation can not happen since c∗−=−c∗+<0. Now, if K(s) converges slowly to 0 at ∞ (for example, the convergence is algebraic and K(λ) is defined only for λ=0), a different type of waves must be considered.
The earlier (weaker) versions of Proposition 3.1 were established under the additional sub-tangency inequality
f(u)≤f′(0)u. | (3.3) |
The non-negativity of the function f′(0)u−f(u) simplifies essentially the respective proofs and allows the explicit calculation of the speeds c∗± from the characteristic equation
χ(z,c):=z2−cz−g′(0)+f′(0)e−zchK(z)=0. |
It is easy to see that there exists a unique value c#− [respectively, c#+] of parameter c for which χ(⋅,c) has a negative [respectively, positive] double zero. If (3.3) is assumed then c∗±=c#± and it may happen that c∗−<c#− and/or c∗+>c#+ in the opposite case. The minimal wavefront propagating with the speed different from c#± is called pushed [53].
The nonlinear stability (see Section 7 [54] for a formal definition of this concept) of the monostable wavefronts (including monotone wavefronts as a particular case) to Eq (2.5) with n=1 was analyzed by means of the energy methods developed by Ming Mei et al. in a series of papers [55,56,57,58,59,60]. See also [61,62] where additionally quasi-monotonicity condition was assumed. Since the energy approach can be equally used for non-quasi-monotone nonlinearities, respective stability results will be discussed later, in section 4. Instead, in Theorem 3.3 below we present one global convergence result from [63] proved for the quasi-monotone delayed equation
∂∂tu(t,x)=∂2∂x2u(t,x)−u(t,x)+f(u(t−h,x)), | (3.4) |
by means of the Chen's [45] squeezing techniques (the same techniques were also used in [64] for establishing the global stability of non-critical monotone waves for the general Eq (2.1) with the monostable quasi-monotone non-local nonlinearity (2.3). However, in contrast to the previous works, the sub-tangency condition (3.3) was not assumed in the mentioned theorem from [63]).
We start by observing that χ(z,c) has exactly two positive zeros (counting multiplicity) λ1=λ1(c)≤λ2=λ2(c) if and only if c≥c#+. Set λ∗:=λ1(c∗+), then 0<λ∗≤λ1(c#+).
Proposition 3.3. Assume that the initial function w0 satisfies [63, Theorem 1]
(IC1) 0≤w0(s,x)≤|w0|∞:=sup(s,x)∈Π0w0(s,x)<∞,(s,x)∈[−h,0]×R⊂R2;
(IC2) lim infx→+∞mins∈[−h,0]w0(s,x)>0,
and, for some A>0 and c>c∗,
limx→−∞w0(s,x)e−λ1(c)(x+cs)=A |
uniformly on s∈[−h,0]. If, in addition, the birth function g∈C1,γ[0,κ] is strictly increasing then the solution u(t,x) of the initial value problem u(s,x)=w0(s,x), s∈[−h,0], x∈R, for Eq (3.4) satisfies
limt→∞supx∈R|u(t,x)−ϕ(x+ct+a)|ηλ1(x+ct)=0,ηλ(x):=min{eλx,1}, | (3.5) |
where a=(λ1(c))−1lnA and the front profile ϕ (existing in virtue of the assumption c>c∗) is normalised by limx→−∞e−λ1(c)xϕ(x)=1.
Clearly, Theorem 3.3 also allows to answer the velocity selection question for solutions with initial data possessing exponential decay at −∞.
The pushed wavefronts (i.e. when c=c∗+>c#+) for the quasi-monotone Eq (3.4) were further analyzed in [35] and their global attractivity properties were established in [65] by means of the squeezing approach combined with the Berestycki and Nirenberg method of the sliding solutions [35,66] as well as some ideas developed by Ogiwara and Matano in [46]:
Proposition 3.4. Let g∈C1,γ[0,κ] be monotone and the initial data w0 satisfy (IC1), (IC2) together with [65, Theorem 1.5]
w0(s,x)≤Keμx,x∈R,s∈[−h,0]; |
for some μ>λ1(c∗) and K>0. Next, fix a positive number λ<μ such that λ∈(λ1(c∗),λ2(c∗)). Then the solution of the initial value problem u(s,x)=w0(s,x), s∈[−h,0], x∈R, for Eq (3.4) asymptotically converges to a shifted pushed front: for some s0∈R,
limt→∞supx∈R|u(t,x)−ϕ(x+c∗t+s0)|/ηλ(x+c∗t)=0. | (3.6) |
Observe that Theorems 3.3 and 3.4 do not give any estimation of the rate of convergence of the solution u(t,x) to its associated limit wavefront. It was conjectured in [63,65] that the convergence rate in (3.5) and (3.6) is of the exponential type. In the particular case of the pushed waves, by enhancing the squeezing method with some ideas of Chen [45] (Theorem 3.1) and Smith et al. [67] (Theorem 3.3), this conjecture was affirmatively solved by Wu et al. in [68] while the case of the pulled wavefronts (c>c∗+) still remains open.
The above stability results imply that two wavefronts to (3.4) propagating at the same speed c>c∗+ and having the same rate of exponential growth at −∞ should coincide (possibly, after a translation). See also Theorem 1.2 of [35] for an alternative proof (based on the sliding solution method of Berestycki and Nirenberg) of this uniqueness property of wavefronts (which are necessarily monotone under the quasi-monotonicity assumption). If the birth function f meets the following special Lipschitz condition (which implies (3.3))
|f(u)−f(v)|≤f′(0)|u−v|,u,v∈[0,κ], | (3.7) |
then the uniqueness of wavefronts (including the minimal ones) for the non-local equations (2.5) is assured by the Diekmann-Kaper theory [33,69]. Without condition (3.7), as far as we know, the uniqueness of wavefronts for the non-local equations is an open question even if the birth function is assumed to be monotone. A weaker uniqueness property within the class of monotone wavefronts for (2.5) was shown in [70] for the monotone C1-smooth nonlinearities satisfying
f′(u)≥f′(κ), u∈[0,κ], |
(importantly, the latter inequality does not exclude the weak Allee effect [71,72]).
Finally, it is worth mentioning than several results proved for non-quasi-monotone equations and mentioned in section 4 are also valid for more simple monotone case.
Consider the reaction term Ru defined in (2.3),
(Ru)(t,x)=f(u(t,x),(K∗g(u))(t−h,x)). |
We will say that the diffusive Eq (2.1) is of the bistable type if C1-smooth function F(u):=f(u,g(u)), u∈[0,κ], is such that
F(u)(u−a)>0,u∈(0,κ)∖{a}, F(0)=F(κ)=F(a)=0, F′(e)≠0 for e∈{0,a,κ}. |
The bistable Eq (2.1) is called quasi-monotone if, in addition, f(u,g(v)) is an increasing function of v. The definition of the bistable wavefront for bistable quasi-monotone Eq (2.1) is the same as given at the beginning of section 3.1. It should be noted that (2.1) also can have monostable wavefronts connecting the equilibria a and κ.
In 1987, for the particular quasi-monotone nonlinearity (Ru)(t,x)=f(u(t,x),u(t−h,x)), Schaaf [23] established the existence and uniqueness of the bistable wavefront. What is not very typical for the delayed systems, the phase space arguments were used in the cited work. This wave solution (propagating at some speed c∗(h)) has strictly increasing profile ϕh. Furthermore, ϕh and c∗(h) depend continuously on h, c∗(h) is monotone decreasing and sign c∗(h)= sign∫κ0F(u)du. As it was showed by Ogiwara and Matano in Theorem 7.2 of [46] on the base of their general convergence theorem for abstract order preserving systems under a group action, obtained bistable wavefront is necessarily locally asymptotically stable with phase shift. Moreover, by using the squeezing technique within the general framework of the theory of abstract functional differential equations [20], Smith and Zhao [67] proved that this bistable wave is globally and exponentially asymptotically stable with phase shift.
Next, in 1997, Chen [45] considered an abstract scalar evolution equation of the bistable quasi-monotone type. By introducing new ideas and partially motivated by the seminal work of Fife and McLeod [13], Chen successfully approached the questions of the existence, uniqueness and asymptotic stability of the bistable wavefronts for his abstract (non-delayed but generally non-local) evolution equation. For non-delayed Eq (2.1) with bistable quasi-monotone nonlinearity (2.3), Theorem 5.1 [45] guarantees the existence and uniqueness of the bistable wavefront (which, in addition, should be monotonic). Moreover, an explicit a priori estimate for the absolute value |c∗(h)| (not depending on h) can be drawn from Theorem 3.5 [45]. In 2007, developing further the comparison and squeezing technique of [45], Ma and Wu [73] proved the global exponential stability with phase shift of the unique monotone wavefront in the bistable quasi-monotone Eq (2.5). For a family of nonlocal delayed advection-diffusion equations (which include the model (2.1) with (2.3)) the existence, uniqueness and stability results of similar kind were obtained by Wang et al. [74].
Finally, a completely different approach for proving wave exponential stability (in the case of quasi-monotone non-local but non-delayed bistable equation) was developed by Ducrot et al. in [75]. It requires a careful analysis of the spectral properties of some associated linear elliptic operator with a nonlocal term. As it was noted by authors of [75], their method may also apply to reaction–diffusion equations with delay.
Suppose now that the birth function f(u,v) is not monotone increasing with respect to the second variable. For example, such situation happens when f(u,v)=u(1−v) (the case of the KPP-Fisher delayed or nonlocal equations) or when f(v) in the Mackey-Glass type Eq (3.4) is not increasing between equilibria. This lack of the monotonicity has at least three following immediate negative (and partially related among them) consequences for the studies of Eq (2.1) with non-monotone nonlinearity (2.3):
i) Restricted application of the comparison arguments;
ii) Limitations in the use of various efficient approaches (e.g. the monotone iterative technique [76] and the sliding solution method);
iii) More complex spectral theory for the linearized equation at the positive equilibrium κ (on the other hand, for the above mentioned biological models, there are not qualitative changes in the spectral analysis of the linearized equation at the zero equilibrium). In particular, the spectrum Σ(κ) of the mentioned equation can have an empty intersection with the real negative semi-axis and therefore generally wave profiles are oscillating around the positive equilibrium [26,28,56,77,78,79,80] (each positive wave developing non-decaying oscillations around the positive equilibrium and converging to 0 at the opposite end of the real line will be referred to as a semi-wavefront). But even when Σ(κ) contains negative eigenvalues, a new class of non-oscillating and non-monotone stable wavefronts appear in several relevant biological models as a consequence of delayed effects [81,82,83]. Observe that stable wavefronts for scalar non-delayed bistable equations are necessarily monotone, cf. [46].
As a result, till the present moment the theory of traveling waves for non-monotone delayed equations did not obtained such a finished form as in the case of quasi-monotone equations. In general, even the basic questions concerning the existence and uniqueness of waves are not completely answered (surprisingly, even adequate definition of the minimal speeds of propagation for monostable equations including (3.4) is an open question [84,85]). Nevertheless, there are a series of rather satisfactory results concerning the existence, uniqueness, stability, geometric and convergence properties of wavefronts for some particular subclasses of non-monotone delayed reaction-diffusion equations. Let us mention some of these findings:
Existence of monostable semi-wavefronts. The abstract recursive approach [50] mentioned in section 3.1 is an efficient tool to study the spreading properties of solutions to the nonlocal delayed diffusive equations. Its extension in [37,86] for some classes of non-monotone systems also allows to solve the wave existence problem. In contrast with [50], the sub-tangency assumption (3.3) was assumed in these works (cf. [37] inequality (4.4) and [86] condition (GC)) to prove the existence of the minimal speed for the semi-wavefronts propagation. By amplifying the Diekmann-Kaper theory of a nonlinear convolution equation [69], an alternative abstract approach to the semi-wavefront existence problem was proposed in [87]. It also requires the dominance condition (3.3). For Eq (3.4), the abstract settings of [37,86] and [87] yield essentially the same conclusions as the earlier remarkable contribution [37,86,88] by Ma (see also [87,89,90,] for the further references).
Quasi-monotonicity in the sense of Wu and Zou: existence of monostable monotone wavefronts. Even if the Eq (2.1) has a non-monotone nonlinearity (2.3), it can still have the monotone wavefronts for some range of the propagation speeds. In particular, this happens if the non-monotone differential wave profile equation can be rewritten in the equivalent integral form possessing good monotonicity properties. As it was indicated in the pioneering work [76] by Wu and Zou, sometimes it is possible to impose a special quasi-monotonicity condition on the birth function (which is not monotone increasing with respect to delayed variable) which allows to implement such a transformation [28]. Remarkably, further developments of the Wu and Zou idea allowed to obtain simple criteria for the existence of the monotone wavefronts in the non-local delayed (including some neutral types) KPP-Fisher and Mackey-Glass equations [28,70,91,92]. It should be noted here that for the latter equation, apart of inequality (3.3), a similar condition at the positive equilibrium was required in [70].
Hale-Lin functional analytic-approach and global continuation of monotone wavefronts. Assuming that Eq (2.1) has a wave solution for certain parameters (e.g., for some fixed speed and delay), sometimes it is possible to use the Lyapunov-Schmidt reduction method in appropriate functional spaces to extend this solution for close values of parameters. For instance, since the wave existence problem simplifies for the parameters ϵ=1/c=0 (and some fixed delay τ>0) or for τ=0 (and some fixed admissible speed), it is convenient to the extend wave solutions existing either for non-delayed equations (when τ=0) or for simpler first-order delay differential equations (when the propagation speed c=∞). The idea behind this method goes back to the Hale and Lin studies [34,93] of the heteroclinic connections for the delayed equations. As a perturbation technique for establishing the persistence of fast monostable wave solutions in general delayed systems, it was invoked for the first time by Faria et al. in [49]. Application of this method requires a detailed analysis of the Fredholm properties of the linearization about the original wave solution. The presence of positive delay complicates this analysis considerably. In a series of subsequent works, the functional analytic approach was successfully applied to study the existence of periodic waves and semi-wavefronts periodically oscillating around the positive equilibrium [95,96], the persistence of wavefronts in nonlocal reaction-diffusion equations for small delays [97,98], the positivity and oscillation properties of the wavefronts [77,99], the uniqueness of fast wavefronts in the Mackey-Glass type equations [100], the non-uniqueness of semi-wavefronts in the non-local KPP-Fisher equations [78], the existence of non-oscillating non-monotone wavefronts in the Kolmogorov ecological equation [82]. Moreover, as it was shown in [101] for the unimodal monostable equations and in [102] for the unimodal bistable equations, the Hale-Lin approach allows to obtain rather optimal global continuation results for the case of monotone wavefronts.
Leray-Schauder continuation principle. The main idea of this method is to transform continuously a non-quasi-monotone model to a quasi-monotone one while keeping track of traveling wave solutions. It was successfully applied to prove the existence: a) of bistable wavefronts for a series of non-delayed models (see [11,103,104] for more references and further discussion); b) of the minimal pulled monostable semi-wavefronts in the non-local KPP-Fisher equation [105]; c) of the wavefronts to non-delayed combustion models [66]. Recent works [106,107] show that the version of the Leray-Schauder theory elaborated in [103,104] allows to prove the existence of monotone wavefronts for the non-quasi-monotone model
∂∂tu(t,x)=∂2∂x2u(t,x)+u(t,x)(1−u(t,x)−g((K∗u)(t−h,x)),u≥0, (t,x)∈R2, | (4.1) |
proposed in [108] in order to understand spatiotemporal dynamics of virus infection spreading in tissues (see section 5 for more details). According to its biological meaning explained in [106,107,108] it was assumed in [106,107] that smooth function g has a unique critical (global maximum) point and g(0)>1, g′(0)>0,
equationg(w)=1−whasasinglesolutionw0for0<w<1, |
g(w)>0for0≤w<1,g(1)=0,g′(1)>−1. |
Observe that in the spatially local case with single discrete delay, Eq (4.1) was also analyzed in [108] (by using the phase plane method) and in [102] (under more realistic conditions on g and inside the Hale-Lin functional-analytical framework).
Now, for the bistable types of Eq (3.4) and non-local Eq (2.4) (with f(u,v)=(u−θ)(1−v)), Alfaro et al. [109,110] appealed to the Leray-Schauder topological degree argument to prove the existence of both monotone fronts and non-monotone semi-wavefronts. In contrast to [103,104,111] (where topological degree was constructed for general elliptic problems in unbounded domains) but similarly to [66,105], the continuation method in [109,110] was applied to establish the existence of a 'truncated semi-wavefronts' ϕa on finite intervals [−a,a], then the required wave was obtained by letting a→+∞.
Uniqueness of semi-wavefronts. For a broad series of autonomous delayed and non-local equations, the Diekmann-Kaper theory of a nonlinear convolution equation [69] and its versions developed in [28,33,91,92] allowed to answer the uniqueness question in the case of the monostable semi-wavefronts, both critical and non-critical: each semi-wavefront propagating with a fixed velocity c is unique up to translation. Application of this theory, however, requires a rather strong linear dominance condition, like (3.7) for the model (3.4). Now, as it was recently shown in [112], the condition (3.7) can be weaken for a broad class of reaction-diffusion models with distributed but finite delays. For example, by Corollary 4 in [112], for the particular case of Eq (3.4), the inequality
f(y)−f(x)≤f′(0)(y−x), for all non-negative y>x, |
implies the uniqueness. An analog of this condition also holds for the delayed logistic (i.e. KPP-Fisher) model that allowed to prove the uniqueness of semi-wavefronts for this equation.
In the case of both bistable and monostable pushed semi-wavefronts, the above-mentioned dominance type conditions are not longer available: as a consequence, even for such simple equation as (3.4), the uniqueness of either bistable or pushed semi-wavefront remains to be an open question [110].
Importantly, as the studies [78,113,114] reveal, for some non-local monostable models, including the non-local KPP-Fisher equation, the wave's uniqueness property fails to hold. It would be interesting to find an adequate biological interpretation of such a non-uniqueness phenomenon.
Stability of semi-wavefronts. The stability of monostable wavefronts (both monotone and oscillating) to non-quasimonotone version of Eq (2.5) with n=1 was an object of investigation in several recent papers by Mei and his collaborators [59,60,115,116]. The technical weighted energy approach was combined with other analytical tools in these works. For example, for Eq (3.4) satisfying (3.7), Theorem 1.1 in [116] presents a rather optimal global stability result, showing that all non-critical wavefronts are globally exponentially stable and all critical waves are globally algebraically stable (with the convergence rate O(t−1/2) as t→+∞ in the usual sup-norm for C(R,R)). It is unclear at this point whether a similar stability result could be obtained: a) for the proper (not converging) semi-wavefronts; b) in situation when (3.7) is not assumed and the wave speed is close to the minimal one.
Recently, a different approach to the stability of oscillating monostable semi-wavefronts (both critical and non-critical) was proposed by Solar et al. in [54,63,117,118]. It is based on maximum principle arguments and requires the dominance of the linearization of (2.5) at zero equilibrium over the whole Eq (2.5). The obtained results complement the studies in [59,60,115,116] and can be considered as the first steps in the solution of the above mentioned problems a) and b). Furthermore, Benguria and Solar [24] developed a technique which allowed to demonstrate local stability (on infinite semi-axes) of each semi-wavefront propagating with the velocity c≥2√2 to delayed version of the celebrated KPP-Fisher equation
ut(t,x)=∂2∂x2u(t,x)+u(t,x)(1−u(t−τ,x)), u≥0, x∈Rm,τ≥0, | (4.2) |
(see [27,76,92,94,101,119,120] for more references about this model). Since the minimal speed c∗ in (4.2) is equal to c∗=2, the stability problem for this model remains open in the case of slow semi-wavefronts.
Finally, we refer to [37,54,63,71] for related asymptotic properties of solutions to non-quasimonotone delayed monostable equations. In should be noted that the stability (and even a weaker question of uniqueness) of both bistable and pushed semi-wavefronts for non-quasimonotone delayed equations seems to be unexplored in the existing literature.
Virus density distribution in the tissue u(x,t) can be described by the delayed reaction-diffusion equation [108,121,122]:
∂u∂t=D∂2u∂x2+ku(1−u)−f(uτ)u, | (5.1) |
where the first term in the right-hand side characterizes its random motion, the second term determines virus reproduction considered in the logistic form, and the last term its elimination by the immune cells. Time delay τ in the function f(uτ), where uτ(x,t)=u(x,t−τ), characterizes clonal expansion of immune cells. Parameters D and k are positive constants, the function f(u) describes the intensity of immune response depending on viral load. It is a non-negative sufficiently smooth function defined for u≥0, it is growing for sufficiently small values of u and decaying for u large enough. Its characteristic form can be qualitatively described by the function f(u)=(a1u+a2)e−a3u, where a1,a2,a3 are some positive constants. It will be used below for convenience of presentation. Let us note that f(0) corresponds to immune response in the absence of pathogen, that is, to the level of acquired immunity.
RDE without time delay. The function F(u)=ku(1−u)−f(u)u vanishes at u0=0 and it has up to three positive zeros. For a single positive zero u=u1, there exist [u0,u1]-waves, that is, the waves with the limits u(−∞)=u1,u(∞)=0, for all values of the speed c≥c0, where c0=2√k−f(0) is the minimal speed (monostable case). These waves are stable in appropriate weighted norms.
If there are two positive zeros, u1 and u2, u1<u2, and u0 and u2 are stable stationary points of the equation du/dt=F(u) (bistable case), then the [u0,u2]-wave exists for a single value of c. This wave is globally asymptotically stable.
In the case of three positive zeros, u1,u2,u3, u1<u2<u3, there are monostable [u0,u1]-waves and a bistable [u1,u3]-wave. If the speed c1 of the [u1,u3]-wave is greater than the minimal speed c0 of the [u0,u1]-waves, then there are [u0,u3]-waves. Otherwise, such waves do not exist, and there are two consecutive waves propagating with different speeds. Convergence of the solution of the Cauchy problem to waves and systems of waves in known [13,14,15,16].
Time delay. If the function f(u) is decreasing, then the existence of waves in the monostable and bistable cases can be proved using the methods based on the maximum principle and comparison theorems [45,73]. For a non-monotone function f(u) such methods are not applicable. In the bistable case, the wave existence is proved in [106] by the Leray-Schauder method and in [102] by the Hale-Lin approach (see section 4).
If f′(u1)>0, where u1 is the first positive zero of the function F(u), then the stationary solution u=u1 of the delay differential equation
dudt=F(uτ) | (5.2) |
loses it stability for the values of τ greater than some critical value τc. Periodic time oscillations, period doubling bifurcations and transition to chaos occur as τ increases [123].
Periodic time oscillations of solutions of Eq (5.2) influence the propagation of monostable [u0,u1]-waves and monostable-bistable waves. Figure 1 shows some examples of wave propagation in the monostable-bistable case [108]. The monostable wave with the minimal speed in this example propagates faster than the bistable wave. The monostable wave becomes non-monotone with decaying or persistent oscillations behind the wave front. The speed of the bistable wave increases with the increase of time delay, and for τ sufficiently large, the two waves merge forming a single wave with the speed c0 of the monostable wave.
Another mechanism of the emergence of spatiotemporal patterns described by Eq (5.1) is related to secondary bifurcations of the homogeneous in space periodic time oscillations. The loss of stability of such oscillations with respect to spatial perturbations leads to the emergence of standing waves, periodic waves, and more complex aperiodic regimes (Figure 3) [123,124].
Behavior of solutions of this equation is different for the initial condition of u(x,0)=u0(x) considered as a piece-wise constant function, u0(x)=u1 for 0≤x≤x0 and u0(x)=u2 for x0<x≤L, where L is the length of the interval, x0∈(0,L), u1 and u2 are some constants. Two different time-oscillating solutions of Eq (5.2) with the initial conditions u(0)=u1 and u(0)=u2 are observed in the left and in the right parts of the interval [0,L] separated by a transition zone between them. The width of this transition zone grows in time with a decaying rate (Figure 4, left) or with a constant rate (Figures 4, middle and right). The former is determined by diffusion, and the latter by a wave propagating between a constant in space and periodic in time solution and spatiotemporal oscillations in the middle of the interval. These oscillations can be close to periodic with a single spike moving back and forth along the transition zone (Figure 4, left) or they can have a more complex structure with multiple spikes (Figures 4, middle and right). Though such oscillations are not periodic, they have some characteristic space and time scales which can be identified in the patterns formed by the maxima of solutions (Figure 4, lower row).
Multi-dimensional equation. The 2D equation
∂u∂t=Dx∂2u∂x2+Dy∂2u∂y2+ku(1−bu)−f(I(uτ))u−σ(y)u | (5.3) |
is introduced in [125] (without time delay) in order to study dynamics of virus distribution with respect to the space variable x∈R and genotype variable y∈[−L,L]. Here I(u)=∫L−Lu(x,y,t)dy is the total virus density for all genotypes. Dependence of the function f(I(u)) on this integral signifies that the strength of immune response depends on the total virus density for all genotypes. A non-negative function σ(y) characterized genotype-dependent virus mortality.
If σ(y)≡0, then for the boundary condition
∂u∂y|y=±L=0, | (5.4) |
and the initial condition independent of y, dynamics of this solution is similar to the dynamics for the 1D equation described above.
The corresponding 1D equation with respect to the genotype variable y and the function σ(y) different from 0 was studied in [126,127]. Positive stationary solutions of this equation decaying at infinity correspond to virus quasi-species. The condition of their existence is determined by the properties of the genotype-dependent mortality σ(y) and by the mutation rate Dy.
If σ(y)≠0, then the solution of Eq (5.3) is essentially two-dimensional. However, in the particular case b=0 it can be reduced to 1D equation by the methods of separation of variables. Set u(x,y,t)=u(x,t)ϕ(y). Then problem (5.3), (5.4) can be reduced to the 1D equation for the function v(x,t):
∂v∂t=Dx∂2v∂x2+kv(1−f(vτ))+λv, | (5.5) |
where λ is the principal eigenvalue of the problem
Dyϕ″−σ(y)ϕ=λϕ,ϕ′(±L)=0, | (5.6) |
with ∫L−Lϕ(y)dy=1. Equation (5.5) is similar to Eq (5.1) with a modified nonlinearity. Dynamics of its solutions can be analyzed by the same methods.
Systems of equations. A more complete model of infection development consists of the equations for the virus density and for the concentration of immune cells:
∂u∂t=Du∂2u∂x2+ku(1−u)−σcu, | (5.7) |
∂c∂t=Dc∂2c∂x2+ϕ(uτ)c(1−c)−ψ(uτ)c, | (5.8) |
where Du,Dc,k, and σ are positive parameters, ϕ(u) and ψ(u) are some non-negative functions. Equation (5.7) is similar to Eq (5.1) where the concentration c of immune cells is replaced by the function f(uτ) (see below). The right-hand side of Eq (5.8) describes random motion of immune cells, their reproduction, and death. The last two terms depend on the virus density with time delay. Under the quasi-stationary approximation in Eq (5.8), from the equality ϕ(uτ)c(1−c)−ψ(uτ)c=0, we get c=f(uτ), where f(u)=1−ψ(u)/ϕ(u). This approximation allows us to reduce system (5.7), (5.8) to Eq (5.1).
If the function f(u) is strictly decreasing, then system (5.7), (5.8) with τ=0 can be reduced to a locally monotone system. The existence of waves in this case is proved by the Leray-Schauder method based on the topological degree for elliptic problems in unbounded domains and a priori estimates in some properly chosen weighted spaces [12]. If this function is not monotonically decreasing, this reduction cannot be done. The wave existence in the bistable case without time delay is proved in [128] and with time delay in [111]. Dynamics of waves is studied numerically in [108,129]. Depending on the values of parameters, these waves convert the disease-free equilibrium either in the virus-free equilibrium, corresponding to infection elimination, or to virus-positive equilibrium corresponding to persistent infection.
Neural field models describe the distribution of electric potential in the brain cortex. Ensemble of neurons in these models is considered as a continuous medium. Neurons receive signals from other neurons and fire if this signal exceeds some threshold level. Neural field models describe various waves and patterns observed in different types of brain activity [130,131,132,134].
The investigation of neural field models begins with the work by Wilson and Cowan [133] who introduced delay integro-differential equations for the densities of activating and inhibiting neurons. This work was followed by many others with a variety of models and results. Typically, neural field model can be considered as a system of two equations
∂u∂t=D1∂2u∂x2+W11−W12−σ1u, | (6.1) |
∂v∂t=D2∂2v∂x2+W21−W22−σ2v, | (6.2) |
where the integrals
Wi1(x,t)=∫∞−∞ϕi1(x−y)Si1(u(y,t−|x−y|q1−τ1))dy,i=1,2, |
Wi2(x,t)=∫∞−∞ϕi2(x−y)Si2(v(y,t−|x−y|q2−τ2))dy,i=1,2, |
describe total signals coming from all points y to the point x, W11 and W21 correspond to the activating signals and W12 and W22 to inhibiting signals. Time delay |x−y|/qi corresponds to the delay in signal transmission, where its speed qi can be different for activating and inhibiting signals, and τi is time delay in the response function Sij due to signal processing. The connectivity functions ϕij characterize the intensity of neuron connection as a function of distance between them. They are usually considered as exponentially decaying functions of the argument |x−y|, while the neuron response functions Sij are sigmoid-type functions. The last terms in right-hand sides in Eqs (6.1), (6.2) describe signal decay. Neural field models are often considered without the diffusion terms [135]. In this case, signal transmission occurs along the axons, and it is taken into account in the integral terms. For the sake of generality and taking into other possible mechanisms (molecular diffusion), we consider the case of nonzero diffusion coefficient. Signal transmission speed qi are sufficiently fast [136], and the terms |x−y|/qi can be neglected.
We will consider various particular cases of the model (6.1), (6.2) and will describe their main properties from the point of view of the existence of wave fronts and periodic waves.
Activation without inhibition. Assuming that the coefficients in Eqs (6.1) and (6.2), as well as connectivity and response functions are equal to each other, we get u(x,t)≡v(x,t) for all x and t, if this equality is satisfied for the initial condition. Therefore, the system of two equations can be reduced to a single equation. Next, if the response function for inhibiting neurons is set 0, then we obtain the scalar equation for the density of activating neurons (signals):
∂u∂t=D∂2u∂x2+∫∞−∞ϕ(x−y)S(u(y,t−τ))dy−σu. | (6.3) |
The subscripts are omitted here for simplicity of notation. Existence and stability of wave fronts for a similar equation in the context of population dynamics was studied in [73]. The wave speed in a partially damaged tissue due to stroke, where the connectivity and response function are modified, decreases with respect to the normal tissue [137]. If the damage of the tissue is not too strong, the wave speed can be restored by the external stimulation.
A modified equation, where the integral and the nonlinearity are inverted, S(J(u)) with J(u)=∫∞−∞ϕ(x−y)u(y,t−τ)dy, instead of J(S(u)) in (6.3), are also considered in neural field models and in other applications. It is shown that the wave speed for this equation can be determined either by diffusion or by neuron connectivity depending on their relative contribution [138].
Activation and inhibition. In the presence of inhibition, system (6.1), (6.2) can be reduced to the single equation
∂u∂t=D∂2u∂x2+∫∞−∞ϕ1(x−y)S1(u(y,t−τ1))dy−∫∞−∞ϕ2(x−y)S2(u(y,t−τ2))dy−σu |
(with the corresponding subscripts). Assuming that S1(0)=S2(0)=0, then u=0 is a homogeneous in space stationary solution of this equation. Linear stability analysis shows that this solution can lose its stability leading to the bifurcation of homogeneous in space periodic time oscillations or of stationary periodic in space solutions. Periodic traveling waves bifurcate from the unstable solution u=0. Therefore, they are unstable near the bifurcation point but they can become stable for sufficiently large time delay τ2 [139]. It is interesting to note that different waves can coexist for the same values of parameters. This result corresponds to the existence of periodic waves with different frequencies and speeds observed in the brain cortex.
Reduction of system (6.1), (6.2) to the single equation imposes some constraints on parameters and dynamics of solutions. Bifurcation of stable periodic waves can occur for the system of two equations, various other patterns and waves are observed [135].
Other models. Models with distributed time delay can also manifest complex dynamics with periodic traveling waves, stationary and time-dependent patterns [140,141]. Neural field model with refractoriness [142] takes into account time delay after neuron firing. Traveling waves in delay reaction-diffusion equations for some other applications are considered in [143,144].
Delayed reaction-diffusion equations arise in various biological and biomedical applications where time delay in the reaction term takes into account duration of some production or elimination processes, such as duration of cell cycle in cell proliferation or virus replication inside cell, and so on. The derivation of these models should be done with certain precaution because time delay in negative terms can lead to the loss of positivity of solutions. Furthermore, the combination of delay with random motion (diffusion) can false the density distribution because the 'particle' moves during delay period. Therefore, the reaction term f(u(x,t−τ)) taken at the space point x at time t−τ does not necessarily correspond to the density variation ∂u∂t(x,t) at the same space point but some time later. In this case, the assumption that motion and proliferation are mutually exclusive, as it is the case in some models of tumor growth, can be appropriate.
Introduction of time delay in biological models allows us sometimes to simplify the model excluding some processes occurring at a lower space scale. If the model concerns, for example, a cell population, then we can possibly bypass complex and often not sufficiently well known intracellular regulation. On the other hand, if the characteristic diffusion time is much larger than time delay in the reaction term, then the introduction of time delay is not justified. As such, in the evolutionary models with time scale measured in dozens of generations, we do not take into account the duration of gestation.
From the mathematical point of view, delayed equations represent an interesting development of the classical theory of reaction-diffusion equations. We can identify two sub-classes of such equations, satisfying or not the maximum principle (quasi-monotonicity). If it is satisfied, then conventional methods to study existence and stability of traveling waves remain usually applicable, though they can be technically more involved. Much less can be done if quasi-monotonicity is not satisfied. In this case, some other methods, such as various continuation methods (including Leray-Schauder method), are developed for some particular types of equations.
Similar to ODEs where time delay can lead to time oscillations, time delay in reaction-diffusion equations makes their dynamics more complex and interesting. Numerical simulations show the emergence of periodic waves and other spatiotemporal patterns. Complex nonlinear dynamics of delayed reaction-diffusion equation is not yet well investigated. The same is true for many other questions including distributed and state dependent delay in reaction-diffusion equations, systems of delayed equations, multi-dimensional equations.
S. Trofimchuk was partially supported by FONDECYT (Chile), project 1190712 (Sections 2–4). V. Volpert is supported by the Ministry of Science and Higher Education of the Russian Federation: agreement no. 075-03-2020-223/3 (FSSF-2020-0018) (Sections 1, 5–7).
The authors declare no conflict of interest.
[1] |
Anholt RRC (2020) Evolution of epistatic networks and the genetic basis of innate behaviors. Trends Genet 36: 24-29. doi: 10.1016/j.tig.2019.10.005
![]() |
[2] |
Arber S (2012) Motor circuits in action: specification, connectivity, and function. Neuron 74: 975-989. doi: 10.1016/j.neuron.2012.05.011
![]() |
[3] |
Adolph KE (2002) Babies' steps make giant strides toward a science of development. Infant Behav Dev 25: 86-90. doi: 10.1016/S0163-6383(02)00106-6
![]() |
[4] |
Simion F, Regolin L, Bulf H (2008) A predisposition for biological motion in the newborn baby. PNAS 105: 809-813. doi: 10.1073/pnas.0707021105
![]() |
[5] |
Slater A, Quinn PC (2001) Face recognition in the newborn infant. Inf Child Dev 10: 21-24. doi: 10.1002/icd.241
![]() |
[6] |
Ekman P, Friesen W (1971) Constants across cultures in the face and emotion. J Pers Social Psychol 17: 124-129. doi: 10.1037/h0030377
![]() |
[7] | Miellet S, Vizioli L, He L, et al. (2013) Mapping face recognition information use across cultures. Front Pshycol 4. |
[8] |
Grillner S, Robertson B (2016) The basal ganglia over 500 million years. Curr Biol 26: R1088-R1100. doi: 10.1016/j.cub.2016.06.041
![]() |
[9] |
Stephenson-Jones M, Samuelsson E, Ericsson J, et al. (2011) Evolutionary conservation of the basal ganglia as a common vertebrate mechanism for action selection. Curr Biol 21: 1081-1091. doi: 10.1016/j.cub.2011.05.001
![]() |
[10] |
Romo R, de Lafuente V (2013) Conversion of sensory signals into perceptual decisions. Prog Neurobiol 103: 41-75. doi: 10.1016/j.pneurobio.2012.03.007
![]() |
[11] |
Boettiger CA, D'Esposito M (2005) Frontal networks for learning and executing arbitrary Stimulus–Response associations. J Neurosci 25: 2723-2732. doi: 10.1523/JNEUROSCI.3697-04.2005
![]() |
[12] |
Garr E (2019) Contributions of the basal ganglia to action sequence learning and performance. Neurosci Biobehav Rev 107: 279-295. doi: 10.1016/j.neubiorev.2019.09.017
![]() |
[13] |
Wu T, Hallett M, Chana P (2015) Motor automaticity in Parkinson's disease. Neurobiol Dis 82: 226-234. doi: 10.1016/j.nbd.2015.06.014
![]() |
[14] |
Marinelli L, Quartarone A, Hallett M, et al. (2017) The many facets of motor learning and their relevance for Parkinson's disease. Clin Neurophysiol 128: 1127-1141. doi: 10.1016/j.clinph.2017.03.042
![]() |
[15] |
Balleine BW, Liljeholm M, Ostlund SB (2009) The integrative function of the basal ganglia in instrumental conditioning. Behav Brain Res 199: 43-52. doi: 10.1016/j.bbr.2008.10.034
![]() |
[16] |
Kobesova A, Kolar P (2014) Developmental kinesiology: Three levels of motor control in the assessment and treatment of the motor system. J Bodyw Mov Ther 18: 23-33. doi: 10.1016/j.jbmt.2013.04.002
![]() |
[17] |
Alexander GE, Crutcher MD (1990) Functional architecture of basal ganglia circuits: Neural substrates of parallel processing. Trends Neurosci 13: 266-271. doi: 10.1016/0166-2236(90)90107-L
![]() |
[18] |
Yin HH, Knowlton BJ (2006) The role of the basal ganglia in habit formation. Nat Rev Neurosci 7: 464-76. doi: 10.1038/nrn1919
![]() |
[19] |
Hiebert NM, Vo A, Hampshire A, et al. (2014) Striatum in stimulus–response learning via feedback and in decision making. Neuroimage 101: 448-457. doi: 10.1016/j.neuroimage.2014.07.013
![]() |
[20] |
Jenkins IH, Jahanshahi M, Jueptner M, et al. (2000) Self-initiated versus externally triggered movements. II. The effect of movement predictability on regional cerebral blood flow. Brain 123: 1216-1228. doi: 10.1093/brain/123.6.1216
![]() |
[21] |
Gasbarri A, Sulli A, Packard MG (1997) The dopaminergic mesencephalic projections to the hippocampal formation in the rat. Prog Neuropsychopharmacol Biol Psychiatry 21: 1-22. doi: 10.1016/S0278-5846(96)00157-1
![]() |
[22] |
Shu SY, Jiang G, Zeng QY, et al. (2015) The marginal division of the striatum and hippocampus has different role and mechanism in learning and memory. Mol Neurobiol 51: 827-839. doi: 10.1007/s12035-014-8891-6
![]() |
[23] |
Seger CA (2008) How do the basal ganglia contribute to categorization? Their roles in generalization, response selection, and learning via feedback. Neurosci Biobehav Rev 32: 265-278. doi: 10.1016/j.neubiorev.2007.07.010
![]() |
[24] |
Arroyo-García LE, Rodríguez-Moreno A, Flores G (2018) Apomorphine effects on the hippocampus. Neural Regen Res 13: 2064-2066. doi: 10.4103/1673-5374.241443
![]() |
[25] | Bissonette GB, Roesch MR (2016) Neurophysiology of rule switching in the corticostriatal circuit. Neuroscience 14: 64-76. |
[26] |
Shu SY, Jiang G, Zeng QY, et al. (2019) A New neural pathway from the ventral striatum to the nucleus basalis of Meynert with functional implication to learning and memory. Mol Neurobiol 56: 7222-7233. doi: 10.1007/s12035-019-1588-0
![]() |
[27] | Goodman J, Packard MG (2017) Memory Systems of the Basal Ganglia. Handbook of Basal Ganglia Structure and Function Academic Press, 725-740. |
[28] |
Peall KJ, Lorentzos MS, Heyman I, et al. (2017) A review of psychiatric co-morbidity described in genetic and immune mediated movement disorders. Neurosci Biobehav Rev 80: 23-25. doi: 10.1016/j.neubiorev.2017.05.014
![]() |
[29] |
Crittenden JR, Graybiel AM (2011) Basal ganglia disorders associated with imbalances in the striatal striosome and matrix compartments. Front Neuroanat 5: 59. doi: 10.3389/fnana.2011.00059
![]() |
[30] |
Graybiel AM (1997) The basal ganglia and cognitive pattern generators. Schizophr Bull 23: 459-69. doi: 10.1093/schbul/23.3.459
![]() |
[31] |
Leisman G, Moustafa AA, Shafir T (2016) Thinking, walking, talking: integratory motor and cognitive brain function. Front Public Health 4: 94. doi: 10.3389/fpubh.2016.00094
![]() |
[32] |
Nobili A, Latagliata EC, Viscomi MT, et al. (2017) Dopamine neuronal loss contributes to memory and reward dysfunction in a model of Alzheimer's disease. Nat Commun 8: 14727. doi: 10.1038/ncomms14727
![]() |
[33] |
Intzandt B, Beck EN, Silveira CRA (2018) The effects of exercise on cognition and gait in Parkinson's disease: A scoping review. Neurosci Biobeh Rev 95: 136-169. doi: 10.1016/j.neubiorev.2018.09.018
![]() |
[34] |
Crowley EK, Nolan YM, Sullivan AM (2019) Exercise as a therapeutic intervention for motor and non-motor symptoms in Parkinson's disease: Evidence from rodent models. Prog Neurobiol 172: 2-22. doi: 10.1016/j.pneurobio.2018.11.003
![]() |
[35] |
Feng YS, Yang SD, Tan ZX, et al. (2020) The benefits and mechanisms of exercise training for Parkinson's disease. Life Sci 245: 117345. doi: 10.1016/j.lfs.2020.117345
![]() |
[36] |
Cunnington R, Iansek R, Thickbroom GW, et al. (1996) Effects of magnetic stimulation over supplementary motor area on movement in Parkinson's disease. Brain 119: 815-822. doi: 10.1093/brain/119.3.815
![]() |
[37] |
Grieb B, von Nicolai C, Engler G, et al. (2013) Decomposition of abnormal free locomotor behavior in a rat model of Parkinson's disease. Front Syst Neurosci 7: 95. doi: 10.3389/fnsys.2013.00095
![]() |
[38] |
Bouchekioua Y, Tsutsui-Kimura I, Sanob H, et al. (2018) Striatonigral direct pathway activation is sufficient to induce repetitive behaviors. Neurosci Res 132: 53-57. doi: 10.1016/j.neures.2017.09.007
![]() |
[39] |
Smith KS, Graybiel AM (2016) Habit formation. Dialogues Clin Neurosci 18: 33-43. doi: 10.31887/DCNS.2016.18.1/ksmith
![]() |
[40] |
Jin X, Costa RM (2015) Shaping action sequences in basal ganglia circuits. Curr Opin Neurobiol 33: 188-196. doi: 10.1016/j.conb.2015.06.011
![]() |
[41] |
Graybiel AM (1998) The basal ganglia and chunking of action repertoires. Neurobiol Learn Mem 70: 119-136. doi: 10.1006/nlme.1998.3843
![]() |
[42] |
Thorn CA, Atallah H, Howe M, et al. (2010) Differential Dynamics of activity changes in dorsolateral and dorsomedial striatal loops during learning. Neuron 66: 781-795. doi: 10.1016/j.neuron.2010.04.036
![]() |
[43] | Smith KS, Graybiel AM (2014) Investigating habits: strategies, technologies and models. Front Behav Neurosci 8: 39. |
[44] |
Iachini T, Ruggiero G, Ruotolo F, et al. (2014) Motor resources in peripersonal space are intrinsic to spatial encoding: Evidence from motor interference. Acta Psychol 153: 20-27. doi: 10.1016/j.actpsy.2014.09.001
![]() |
[45] |
Wang C, Chen X, Knierim JJ (2020) Egocentric and allocentric representations of space in the rodent brain. Curr Opin Neurobiol 60: 12-20. doi: 10.1016/j.conb.2019.11.005
![]() |
[46] |
Glover S, Bibby E, Tuomi E (2020) Executive functions in motor imagery: support for the motor-cognitive model over the functional equivalence model. Exp Brain Res 238: 931-944. doi: 10.1007/s00221-020-05756-4
![]() |
[47] |
Soriano M, Cavallo A, D'Ausilio A, et al. (2018) Movement kinematics drive chain selection toward intention detection. PNAS 115: 10452-10457. doi: 10.1073/pnas.1809825115
![]() |
[48] |
Del Vecchio M, Caruana F, Sartori I, et al. (2020) Action execution and action observation elicit mirror responses with the same temporal profile in human SII. Commun Biol 3: 80. doi: 10.1038/s42003-020-0793-8
![]() |
[49] |
McBride SD, Parker MO (2015) The disrupted basal ganglia and behavioural control: An integrative cross-domain perspective of spontaneous stereotypy. Behav Brain Res 276: 45-58. doi: 10.1016/j.bbr.2014.05.057
![]() |
[50] | Guzulaitis R, Alaburda A, Hounsgaard J (2013) Increased activity of pre-motor network does not change the excitability of motoneurons during protracted scratch initiation. J Physiol 120: 2542-2554. |
[51] |
Grillner S, McClellan A, Perret C (1981) Entrainment of the spinal pattern generators for swimming by mechano-sensitive elements in the lamprey spinal cord in vitro. Brain Res 217: 380-386. doi: 10.1016/0006-8993(81)90015-9
![]() |
[52] |
Grillner S (1991) Recombination of motor pattern generators. Curr Biol 1: 231-3. doi: 10.1016/0960-9822(91)90066-6
![]() |
[53] |
Kalueff AV, Stewart AM, Song C, et al. (2015) Neurobiology of rodent self-grooming and its value for translational neuroscience. Nat Rev Neurosci 17: 45-59. doi: 10.1038/nrn.2015.8
![]() |
[54] |
Ashby FG, Turner BO, Horvitz JC (2010) Cortical and basal ganglia contributions to habit learning and Automaticity. Trends Cogn Sci 14: 208-215. doi: 10.1016/j.tics.2010.02.001
![]() |
[55] |
Redgrave R, Rodriguez M, Smith Y, et al. (2011) Goal-directed and habitual control in the basal ganglia: implications for Parkinson's disease. Nat Rev Neurosci 11: 760-772. doi: 10.1038/nrn2915
![]() |
[56] |
Dezfouli A, Balleine BW (2012) Habits, action sequences and reinforcement learning. Eur J Neurosci 35: 1036-1051. doi: 10.1111/j.1460-9568.2012.08050.x
![]() |
[57] | Hernández LF, Redgrave P, Obeso JA (2015) Habitual behaviour and dopamine cell vulnerability in Parkinson disease. Front Neuroanat 9: 99. |
[58] |
Helie S, Chakravarthy S, Moustafa AA (2013) Exploring the cognitive and motor functions of the basal ganglia: an integrative review of computational cognitive neuroscience models. Front Comput Neurosci 7: 174. doi: 10.3389/fncom.2013.00174
![]() |
[59] |
Smith KS, Graybiel AM (2013) A dual operator view of habitual behavior reflecting cortical and striatal dynamics. Neuron 79: 361-374. doi: 10.1016/j.neuron.2013.05.038
![]() |
[60] |
Hart G, Leung BK, Balleine BW (2014) Dorsal and ventral streams: The distinct role of striatal subregions in the acquisition and performance of goal-directed actions. Neurobiol Learn Mem 108: 104-118. doi: 10.1016/j.nlm.2013.11.003
![]() |
[61] |
Hinman JR, Chapman GW, Hasselmo ME (2019) Neural representation of environmental boundaries in egocentric coordinates. Nat Commun 10: 2772. doi: 10.1038/s41467-019-10722-y
![]() |
[62] | Florio TM (2017) The 6-Hydroxydopamine Hemiparkinsonian Rat Model: Evidence of Early Stage Degeneration of the Nigrostriatal Pathway. Xjenza Online Available from: https://www.xjenza.org/JOURNAL/OLD/5-2-2017/05.pdf. |
[63] |
Poldrack RA, Packard MG (2003) Competition among multiple memory systems: converging evidence from animal and human brain studies. Neuropsychologia 41: 245-251. doi: 10.1016/S0028-3932(02)00157-4
![]() |
[64] |
Calabresi P, Maj R, Pisani A, et al. (1992) Longterm synaptic depression in the striatum: physiological and pharmacological characterization. J Neurosci 12: 4224-4233. doi: 10.1523/JNEUROSCI.12-11-04224.1992
![]() |
[65] |
Calabresi P, Centonze D, Gubellini P, et al. (2000) Synaptic transmission in the striatum: from plasticity to neurodegeneration. Prog Neurobiol 61: 231-265. doi: 10.1016/S0301-0082(99)00030-1
![]() |
[66] |
Calabresi P, Picconi B, Tozzi A, et al. (2007) Dopamine mediated regulation of corticostriatal synaptic plasticity. Trends Neurosci 30: 211-219. doi: 10.1016/j.tins.2007.03.001
![]() |
[67] |
Pisani A, Centonze D, Bernardi G, et al. (2005) Striatal synaptic plasticity: Implications for motor learning and Parkinson's disease. Movement Disord 20: 395-402. doi: 10.1002/mds.20394
![]() |
[68] |
Centonze D, Picconi B, Gubellini P, et al. (2001) Dopaminergic control of synaptic plasticity in the dorsal striatum. Eur J Neurosci 13: 1071-1077. doi: 10.1046/j.0953-816x.2001.01485.x
![]() |
[69] |
Grahn JA, Parkinson JA, Owen AM (2009) The role of the basal ganglia in learning and memory: Neuropsychological studies. Behav Brain Res 199: 53-60. doi: 10.1016/j.bbr.2008.11.020
![]() |
[70] |
Atallah HE, Frank MJ, O'Reilly RC (2004) Hippocampus, cortex, and basal ganglia: Insights from computational models of complementary learning systems. Neurobiol Learn Mem 82: 253-267. doi: 10.1016/j.nlm.2004.06.004
![]() |
[71] |
Brown JW, Bullock D, Grossberg S (2004) How laminar frontal cortex and basal ganglia circuits interact to control planned and reactive saccades. Neural Networks 17: 471-510. doi: 10.1016/j.neunet.2003.08.006
![]() |
[72] |
Aron AR, Behrens TE, Smith S, et al. (2007) Triangulating a cognitive control network using diffusion-weighted magnetic resonance imaging (MRI) and functional MRI. J Neurosci 27: 3743-52. doi: 10.1523/JNEUROSCI.0519-07.2007
![]() |
[73] |
Baunez C, Nieoullon A, Amalric M (1995) Dopamine and complex sensorimotor integration: further studies in a conditioned motor task in the rat. Neuroscience 65: 375-384. doi: 10.1016/0306-4522(94)00498-T
![]() |
[74] |
Lehéricy S, Benali H, Van de Moortele PF, et al. (2005) Distinct basal ganglia territories are engaged in early and advanced motor sequence learning. Proc Natl Acad Sci 102: 12566-12571. doi: 10.1073/pnas.0502762102
![]() |
[75] |
Alexander GE, Crutcher MD (1990) Functional architecture of basal ganglia circuits: neural substrates of parallel processing. Trends Neurosci 13: 266-271. doi: 10.1016/0166-2236(90)90107-L
![]() |
[76] |
Lawrence AD, Sahakian BJ, Robbins TW (1998) Cognitive functions and corticostriatal circuits: insights from Huntington's disease. Trends Cogn Sci 2: 379-388. doi: 10.1016/S1364-6613(98)01231-5
![]() |
[77] |
Bédard P, Sanes JN (2009) On a basal ganglia role in learning and rehersing visual-MPTPr associations. Neuroimage 47: 1701-1710. doi: 10.1016/j.neuroimage.2009.03.050
![]() |
[78] |
Delgado MR, Miller MM, Inati S, et al. (2005) An fMRI study of reward-related probability learning. Neuroimage 24: 862-873. doi: 10.1016/j.neuroimage.2004.10.002
![]() |
[79] |
Grol MJ, de Lange FP, Verstraten FA, et al. (2006) Cerebral changes during performance of overlearned arbitrary visuomotor associations. J Neurosci 26: 117-125. doi: 10.1523/JNEUROSCI.2786-05.2006
![]() |
[80] |
Seger CA, Cincotta CM (2006) Dynamics of frontal, striatal, and hippocampal systems during rule learning. Cereb Cortex 16: 1546-1555. doi: 10.1093/cercor/bhj092
![]() |
[81] |
Boettiger CA, D'Esposito M (2005) Frontal networks for learning and executing arbitrary stimulus–response associations. J Neurosci 25: 2723-2732. doi: 10.1523/JNEUROSCI.3697-04.2005
![]() |
[82] |
Buch ER, Brasted PJ, Wise SP (2006) Comparison of population activity in the dorsal premotor cortex and putamen during the learning of arbitrary visuomotor mappings. Exp Brain Res 169: 69-84. doi: 10.1007/s00221-005-0130-y
![]() |
[83] |
Charpier S, Mahon S, Deniau JM (1999) In vivo induction of striatal long-term potentiation by low-frequency stimulation of the cerebral cortex. Neuroscience 91: 1209-1222. doi: 10.1016/S0306-4522(98)00719-2
![]() |
[84] |
Bonsi P, Florio T, Capozzo A, et al. (2003) Behavioural learning-induced increase in spontaneous GABAA-dependent synaptic activity in rat striatal cholinergic interneurons. Eur J Neurosci 17: 174-178. doi: 10.1046/j.1460-9568.2003.02410.x
![]() |
[85] |
Frank MJ, Loughry B, O'Reilly RC (2001) Interactions between the frontal cortex and basal ganglia in working memory: A computational model. Cogn Affect Behav Neurosci 1: 137-160. doi: 10.3758/CABN.1.2.137
![]() |
[86] |
Fino E, Glowinski J, Venance L (2005) Bidirectional activity-dependent plasticity at corticostriatal synapses. J Neurosci 25: 11279-11287. doi: 10.1523/JNEUROSCI.4476-05.2005
![]() |
[87] |
Fino E, Deniau JM, Venance L (2008) Cell-specific spike-timing-dependent plasticity in GABAergic and cholinergic interneurons in corticostriatal rat brain slices. J Physiol 586: 265-282. doi: 10.1113/jphysiol.2007.144501
![]() |
[88] |
Fino E, Deniau JM, Venance L (2009) Brief subthreshold events can act as Hebbian signals for long-term plasticity. PloS ONE 4: e6557. doi: 10.1371/journal.pone.0006557
![]() |
[89] |
Bliss TV, Collingridge GL (1993) A synaptic model of memory: long-term potentiation in the hippocampus. Nature 361: 31-39. doi: 10.1038/361031a0
![]() |
[90] |
Martin SJ, Grimwood PD, Morris RG (2000) Synaptic plasticity and memory: an evaluation of the hypothesis. Annu Rev Neurosci 23: 649-711. doi: 10.1146/annurev.neuro.23.1.649
![]() |
[91] |
Martin SJ, Morris RG (2002) New life in an old idea: the synaptic plasticity and memory hypothesis revisited. Hippocampus 12: 609-636. doi: 10.1002/hipo.10107
![]() |
[92] |
Lynch MA (2004) Long-term potentiation and memory. Physiol Rev 84: 87-136. doi: 10.1152/physrev.00014.2003
![]() |
[93] |
Giordano N, Iemolo A, Mancini M, et al. (2018) Motor learning and metaplasticity in striatal neurons: relevance for Parkinson's disease. Brain 141: 505-520. doi: 10.1093/brain/awx351
![]() |
[94] |
Cameron IGM, Coe CB, Watanabe M, et al. (2009) Role of the basal ganglia in switching a planned response. Eur J Neurosci 29: 2413-2425. doi: 10.1111/j.1460-9568.2009.06776.x
![]() |
[95] |
Hikosaka O, Isoda M (2010) Switching from automatic to controlled behavior: cortico-basal ganglia mechanisms. Trends Cogn Sci 14: 154-161. doi: 10.1016/j.tics.2010.01.006
![]() |
[96] |
Obeso JA, Rodriguez-Oroz MC, Benitez-Temino B, et al. (2008) Functional organization of the basal ganglia: Therapeutic implications for Parkinson's Disease. Movement Disord 23: S548-S559. doi: 10.1002/mds.22062
![]() |
[97] |
Mink JW (1996) The basal ganglia: focused selection and inhibition of competing motor programs. Prog Neurobiol 50: 381-425. doi: 10.1016/S0301-0082(96)00042-1
![]() |
[98] |
Reynolds JNJ, Hyland BI, Wickens JR (2019) A cellular mechanism of reward-related learning. Nature 413: 67-70. doi: 10.1038/35092560
![]() |
[99] |
Vandaele Y, Mahajan NR, Ottenheimer DJ, et al. (2019) Distinct recruitment of dorsomedial and dorsolateral striatum erodes with extended training. eLife 8: e49536. doi: 10.7554/eLife.49536
![]() |
[100] |
Klaus A, Martins GJ, Paixao VB, et al. (2017) The spatiotemporal organization of the striatum encodes action space. Neuron 95: 1171-1180. doi: 10.1016/j.neuron.2017.08.015
![]() |
[101] |
Florio TM, Scarnati E, Rosa I, et al. (2018) The basal ganglia: More than just a switching device. CNS Neurosci Ther 24: 677-684. doi: 10.1111/cns.12987
![]() |
[102] |
Florio TM, Confalone G, Sciarra A, et al. (2013) Switching ability of over trained movements in a Parkinson's disease rat model. Behav Brain Res 250: 326-333. doi: 10.1016/j.bbr.2013.05.020
![]() |
[103] |
Patel N, Jankovic J, Hallett M (2014) Sensory aspects of movement disorders. Lancet Neurol 13: 100-112. doi: 10.1016/S1474-4422(13)70213-8
![]() |
[104] |
Williams-Gray CH, Worth PF (2016) Parkinson's disease. Movement Disorders. Medicine (Baltimore) 44: 542-546. doi: 10.1016/j.mpmed.2016.06.001
![]() |
[105] |
Asakawa T, Fang H, Sugiyama K, et al. (2016) Human behavioural assessments in current research of Parkinson's disease. Neurosci Biobehav Rev 68: 741-772. doi: 10.1016/j.neubiorev.2016.06.036
![]() |
[106] |
Obeso JA, Stamelou M, Goetz CG, et al. (2017) Past, present, and future of Parkinson's disease: A special essay on the 200th Anniversary of the Shaking Palsy. Mov Disord 32: 1264-1310. doi: 10.1002/mds.27115
![]() |
[107] |
Berganzo K, Tijero B, González-Eizaguirre A, et al. (2016) Motor and non-motor symptoms of Parkinson's disease and their impact on quality of life and on different clinical subgroups. Neurologia 31: 585-591. doi: 10.1016/j.nrl.2014.10.010
![]() |
[108] |
Cameron IGM, Watanabe M, Pari G, et al. (2010) Executive impairment in Parkinson's disease: Response automaticity and task switching. Neuropsychologia 48: 1948-57. doi: 10.1016/j.neuropsychologia.2010.03.015
![]() |
[109] | Magrinelli F, Picelli A, Tocco P, et al. (2016) Pathophysiology of Motor Dysfunction in Parkinson's Disease as the Rationale for Drug Treatment and Rehabilitation. Parkinsons Dis 2016: 9832839. |
[110] |
Mancini M, Carlson-Kuhta P, Zampieri C, et al. (2012) Postural sway as a marker of progression in Parkinson's disease: A pilot longitudinal study. Gait Posture 36: 471-476. doi: 10.1016/j.gaitpost.2012.04.010
![]() |
[111] |
Rosa I, Di Censo D, Ranieri B, et al. (2020) Comparison between Tail Suspension Swing Test and Standard Rotation Test in revealing early motor behavioral changes and neurodegeneration in 6-OHDA hemiparkinsonian rats. Int J Mol Sci 21: 2874. doi: 10.3390/ijms21082874
![]() |
[112] |
Siragy T, Nantel J (2020) Absent arm swing and dual tasking decreases trunk postural control and dynamic balance in people with Parkinson's disease. Front Neurol 11: 213. doi: 10.3389/fneur.2020.00213
![]() |
[113] |
Fox ME, Mikhailova MA, Bass CE, et al. (2016) Cross-hemispheric dopamine projections have functional significance. Proc Natl Acad Sci 113: 6985-6990. doi: 10.1073/pnas.1603629113
![]() |
[114] |
Lisman JE, Grace AA (2005) The Hippocampal-VTA loop: Controlling the entry of information into long-term memory. Neuron 46: 703-713. doi: 10.1016/j.neuron.2005.05.002
![]() |
[115] |
Cordella A, Krashia P, Nobili A, et al. (2018) Dopamine loss alters the hippocampus-nucleus accumbens synaptic transmission in the Tg2576 mouse model of Alzheimer's disease. Neurobiol Dis 116: 142-154. doi: 10.1016/j.nbd.2018.05.006
![]() |
[116] |
Casarrubea M, Di Giovanni G, Crescimanno G, et al. (2019) Effects of Substantia Nigra pars compacta lesion on the behavioural sequencing in the 6-OHDA model of Parkinson's disease. Behav Brain Res 362: 28-35. doi: 10.1016/j.bbr.2019.01.004
![]() |
[117] |
Gilat M, Bell PT, Ehgoetz Martens KA, et al. (2017) Dopamine depletion impairs gait automaticity by altering cortico-striatal and cerebellar processing in Parkinson's disease. Neuroimage 152: 207-220. doi: 10.1016/j.neuroimage.2017.02.073
![]() |
[118] | Wissner-Gross AD, Freer CE (2013) Causal entropic forces. Phys Rev Lett 110: 168702-1-168702-5. |
[119] |
Merchant H, Averbeck BB (2017) The computational and neural basis of rhythmic timing in medial premotor cortex. J Neurosci 37: 4552-4564. doi: 10.1523/JNEUROSCI.0367-17.2017
![]() |
[120] |
Athalye VR, Carmena JM, Costa RM (2020) Neural reinforcement: re-entering and refining neural dynamics leading to desirable outcomes. Curr Opin Neurobiol 60: 145-154. doi: 10.1016/j.conb.2019.11.023
![]() |
1. | Wei-Jian Bo, Junfeng He, Guo Lin, Qualitative properties of traveling wave solutions in delayed reaction-diffusion equations with degenerate monostable nonlinearity, 2022, 514, 0022247X, 126318, 10.1016/j.jmaa.2022.126318 | |
2. | Gaetano Campi, Antonio Bianconi, Periodic recurrent waves of Covid-19 epidemics and vaccination campaign, 2022, 160, 09600779, 112216, 10.1016/j.chaos.2022.112216 | |
3. | M. Banerjee, M. Kuznetsov, O. Udovenko, V. Volpert, Nonlocal Reaction–Diffusion Equations in Biomedical Applications, 2022, 70, 0001-5342, 10.1007/s10441-022-09436-4 | |
4. | Changchun Liu, Ming Mei, Jiaqi Yang, Global stability of traveling waves for nonlocal time-delayed degenerate diffusion equation, 2022, 306, 00220396, 60, 10.1016/j.jde.2021.10.027 | |
5. | Latifa Ait Mahiout, Nikolai Bessonov, Bogdan Kazmierczak, Georges Sadaka, Vitaly Volpert, Infection spreading in cell culture as a reaction-diffusion wave, 2022, 56, 2822-7840, 791, 10.1051/m2an/2022019 | |
6. | Gaetano Campi, Andrea Perali, Augusto Marcelli, Antonio Bianconi, Sars-Cov2 world pandemic recurrent waves controlled by variants evolution and vaccination campaign, 2022, 12, 2045-2322, 10.1038/s41598-022-22816-7 | |
7. | Domenico Mezzanotte, Donatella Occorsio, Ezio Venturino, Analysis of a line method for reaction-diffusion models of nonlocal type, 2024, 203, 01689274, 255, 10.1016/j.apnum.2024.05.011 | |
8. | Jui-Pin Tseng, Uniform ultimate boundedness and approximate synchronization of coupled reaction-diffusion systems with parameter mismatches, 2024, 533, 0022247X, 127993, 10.1016/j.jmaa.2023.127993 | |
9. | Ezio Venturino, Sebastian Aniţa, Domenico Mezzanotte, Donatella Occorsio, A high order numerical scheme for a nonlinear nonlocal reaction–diffusion model arising in population theory, 2024, 451, 03770427, 116082, 10.1016/j.cam.2024.116082 | |
10. | Abraham Solar, A Simple Approach to Stability of Semi-wavefronts in Parabolic-Difference Systems, 2024, 1040-7294, 10.1007/s10884-024-10371-w | |
11. | V. Volpert, S. Petrovskii, Reaction-diffusion waves in biology: new trends, recent developments, 2025, 52, 15710645, 1, 10.1016/j.plrev.2024.11.007 |