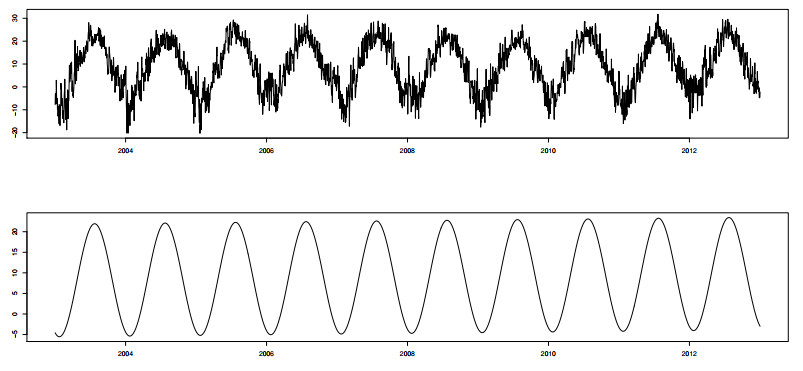
Citation: Kevin Z. Tong, Allen Liu. Modeling temperature and pricing weather derivatives based on subordinate Ornstein-Uhlenbeck processes[J]. Green Finance, 2020, 2(1): 1-19. doi: 10.3934/GF.2020001
[1] | Yilin Wu, Shiyu Ma . Impact of COVID-19 on energy prices and main macroeconomic indicators—evidence from China's energy market. Green Finance, 2021, 3(4): 383-402. doi: 10.3934/GF.2021019 |
[2] | Yuhang Zheng, Ziqing Du . A systematic review in crude oil markets: Embarking on the oil price. Green Finance, 2019, 1(3): 328-345. doi: 10.3934/GF.2019.3.328 |
[3] | Yoram Krozer . Financing of the global shift to renewable energy and energy efficiency. Green Finance, 2019, 1(3): 264-278. doi: 10.3934/GF.2019.3.264 |
[4] | Xuan Feng . The role of ESG in acquirers' performance change after M&A deals. Green Finance, 2021, 3(3): 287-318. doi: 10.3934/GF.2021015 |
[5] | Neha Saxena, Jitendra Kumar, Shib Sankar Sana . Sustainable production model with stochastic machine breakdown using smart manufacturing under circular economy. Green Finance, 2023, 5(4): 479-511. doi: 10.3934/GF.2023019 |
[6] | Memoona Kanwal, Hashim Khan . Does carbon asset add value to clean energy market? Evidence from EU. Green Finance, 2021, 3(4): 495-507. doi: 10.3934/GF.2021023 |
[7] | Tinghui Li, Junhao Zhong, Hai Zhang, Pierre Failler . Chinese financial cycle spillovers to developed countries. Green Finance, 2019, 1(4): 364-386. doi: 10.3934/GF.2019.4.364 |
[8] | Yaya Su, Gaoke Liao . The impact of macroeconomic news on stock returns of energy firms—evidence from China. Green Finance, 2019, 1(3): 297-311. doi: 10.3934/GF.2019.3.297 |
[9] | Hao Dong, Yue Liu, Jiaqi Chang . The heterogeneous linkage of economic policy uncertainty and oil return risks. Green Finance, 2019, 1(1): 46-66. doi: 10.3934/GF.2019.1.46 |
[10] | Patrick Hess . The supervision and regulation of climate risks for banks: overview from the perspective of a European practitioner. Green Finance, 2022, 4(3): 295-309. doi: 10.3934/GF.2022014 |
A weather derivative takes its value from an underlying measure of weather, such as temperature, precipitation, humidity, frost, stream flow or wind speed over a particular period of time, and permits the financial risk associated with climatic conditions to be managed. The first weather derivative transaction appeared over-the-counter (OTC) in US in 1997 and weather derivatives were officially introduced in the major financial markets like Chicago Mercantile Exchange (CME) in 1999. Over the recent years, weather derivatives market has grown rapidly and expanded to Europe and Asia.
Weather derivatives based on temperature are by far the most common type of weather derivatives, accounting for approximately 85% of all weather derivative transactions. Such contracts are typically written on the temperature indices including cumulative average temperature (CAT), heating degree days (HDD) and cooling degree days (CDD). CAT is defined as the sum of the daily average temperature over the period of the contract. HDD or CDD is a measure of the amount by which the daily average temperature during the period deviates from a threshold temperature (typically 18∘C or 65∘F). HDD and CDD are "one-side" metrics and they are critical for creating the risk management tools that utility, agriculture, construction and other firms can use to hedge their activities affected by outdoor climate. For example, a utility company might buy CDD futures if it expects higher temperatures and sell the futures if it expects significantly lower temperatures.
In this study we focus on pricing temperature-based derivatives whose underlying temperature process is modeled as a stochastic process. In the literature, the daily average temperature is often modeled as
S(t)=Λ(t)+Y(t), | (1) |
where Λ is a deterministic function that models the seasonal trend of the temperature process and Y, also known as deseasonalized temperature, is a stochastic process to model the random fluctuations from the trend. So far, the mean-reverting OU process is the most popular process used to model Y. Queruel (2000) develop a mean-reverting OU model for Y, where the diffusion term is a Brownian motion. Benth and Šaltytė-Benth (2005) propose a Lévy-driven OU model with seasonal mean and volatility, where the diffusion term is a Lévy process rather than a Brownian motion. Benth et al. (2007) generalize the OU model to the continuous-time autoregressive (CAR) models with seasonal variance. Swishchuk and Cui (2013) extend the Lévy-driven OU process to the Lévy-driven CAR process for Y. Other OU-based models for Y include e.g. fractional OU models of Brody et al. (2002) and Benth (2003), regime switching OU models of Elias et al. (2014) and Evarest et al. (2018).
Temperature-based derivatives pricing has been investigated e.g. in Brody et al. (2002), Benth (2003), Benth and Šaltytė-Benth (2005), Benth et al. (2007), Swishchuk and Cui (2013), Elias et al. (2014) and Evarest et al. (2018). Due to the complex nature of the temperature indices, numerical approximation or Monte Carlo simulations are typically used to obtain the temperature derivative prices. However, in some special cases, the analytical pricing formulas have been derived for some specific temperature derivative products. Benth et al. (2007) obtain the closed-form solutions to CAT future and future option prices when the temperature is modeled by the CAR process driven by Brownian motion. Applying the Fourier technique based on the characteristic function of the temperature process, Swishchuk and Cui (2013) derive the analytical formulas for CAT futures, CAT future options and HDD/CDD futures prices when the underlying temperature is modeled by the general Lévy-driven CAR process. However, for HDD/CDD future options, the analytical formulas for the prices are not available even for OU model driven by Brownian motion; see e.g. Benth et al. (2007) for further discussions.
In this paper, we propose a time-changed OU (TC-OU) model for the deseasonalized temperature process Y. We define the time change process T as T(t)=L(A(t)), where L is a Lévy subordinator and A(t)=∫t0b(u)du with b(u) being a deterministic function of time. Then Y is modeled by the subordinate OU process Y(t)=X(T(t)), where X is an OU process driven by a Brownian motion. Our model exhibits several distinct features. First, time changing a continuous time Markov process such as OU process can lead to a much wider class of models than the traditional jump-diffusion models. Depending on whether the Lévy subordinator L has drift or not, the new process will be a jump-diffusion or a pure jump process. Second, for the subordinate process Y, its jump measure is state-dependent and mean-reverting, making it ideal for modeling temperature. Third, through the deterministic function b(u), in our model the drift, volatility and jump measure of the process Y are all time dependent and seasonal. Thus, Our model has the potential to capture the salient characteristics of temperature time series. We need to mention that in the time-changed option pricing literature, Carr and Wu (2004) provide option pricing under time-changed Lévy processes, and Cui et al. (2019b) provide a framework for pricing options under general Markov processes, including the subordinate OU process. The subordinate OU process has been successfully applied for callable and putable bonds in Lim et al. (2012), commodities in Li and Linetsky (2014), electricity derivatives in Tong (2017), variance swaps in Tong and Liu (2017), Asian options in Tong and Liu (2018), dual-expiry exotics in Tong et al. (2019) and autocallables in Tong (2019). We also refer to Linetsky and Mitchell (2008) for a brief introduction of subordinate Markov processes and Mendoza-Arriaga et al. (2010), Arriaga and Linetsky (2013), Li et al. (2016b) and Li et al. (2017) for their further applications.
To price temperature derivatives, we assume that under the pricing measure, our model remains in the same form as in the physical measure, but with a different parameter set to account for risk premia. We are able to derive the analytical pricing formulas for CAT, HDD and CDD future and future option prices using eigenfunction expansion method. We need to emphasize that eigenfunction expansion method is particularly suitable for pricing contingent claims written on the subordinate processes. The subordinate process is as analytically tractable as the original process without time change. The subordinate process shares the same eigenfunctions with the original one and the only modification is the replacement of eigenvalues of the original process with the Laplace transform of the time change process. We refer to Linetsky and Mitchell (2008) for the surveys on the eigenfunction expansion method and Linetsky (2004), Mendoza-Arriaga et al. (2010), Lim et al. (2012), Mendoza-Arriaga and Linetsky (2013), Li and Linetsky (2014), Li et al. (2017), Tong (2017), Tong and Liu (2017), Tong and Liu (2018), Tong et al. (2019) and Tong (2019) for its various applications.
In this paper, we apply the eigenfunction expansion method for the pricing of temperature derivatives under the time-changed OU process. There are alternative analytical pricing methodologies that are applicable to the general case when the underlying stochastic process is a time-changed Markov process. A promising method is to approximate the underlying process by a continuous time Markov chain (CTMC). We refer to Cui et al. (2019a) for a review of this recent and growing literature and Li and Zhang (2018) for the relationship between CTMC approximation and the eigenfunction expansion approach applied in this work.
The rest of the paper is organized as follows. In Section 2, we introduce the general framework for modeling temperature as a time-changed OU process, where the time change process is modeled by a Lévy subordinator time changed by a deterministic clock with seasonable activity rate. In Section 3, we introduce the eigenfunction expansion method for our new model and also discuss how to calculate some important integrals that are essential for the determination of the eigenfunction expansion coefficients. In Section 4, we apply the eigenfunction expansion method to obtain the analytical pricing formulas for CAT, CDD and HDD futures and future options. In Section 5, we estimate our new model and compare its performance with the competing models using the temperature data. We also numerically study the impact of market price of risk on the temperature derivatives.
Let (Ω,F,P) be a probability space with an information filtration (Ft). Suppose under the physical measure P, the temperature process S is modeled as
S(t)=Λ(t)+Y(t), | (2) |
where Λ(t) is the seasonal trend term that is modeled by a combination of linear and cosine function:
Λ(t)=a0+a1t+a2cos(2π(t−a3)365). | (3) |
Similar forms can be found in Benth and Šaltytė-Benth (2005) and Swishchuk and Cui (2013).
The deseasonalized temperature process Y is specified as a time-changed OU process, that is
Y(t)=X(T(t)), | (4) |
where T is a time change process and X is an OU process
dX(t)=κ(θ−X(t))dt+σdB(t), | (5) |
where κ>0 and B(t) is a standard Brownian motion.
We choose the time change process T to be of the form T(t)=L(A(t)), where A(t)=∫t0b(u)du and the activity rate b(u) is modeled as a positive periodical function
b(u)=b0+b1cos(2π(u−b2)365), | (6) |
where b0≥|b1|. Then A(t) can be solved as
A(t)=b0t+b13652π[sin(2π(t−b2)365)−sin(−2πb2365)]. | (7) |
We remark that other specifications for b(u) are possible, as long as A(t) is easy to compute.
To introduce jumps into the temperature dynamics, we model the process L as a Lévy subordinator independent of B in (5). The Lévy subordinator L is a nondecreasing process with positive jumps and non-negative drift with the Laplace transform:
E[exp(−λL(t))]=exp(−tϕ(λ)), | (8) |
where ϕ is the Lévy exponent and given by the Lévy-Khintchine formula (see e.g. Sato, 1999)
ϕ(λ)=γλ+∫(0,∞)(1−exp(−λs))ν(ds), | (9) |
where γ≥0 and the Lévy measure ν must satisfy
∫(0,∞)(s∧1)ν(ds)<∞. | (10) |
An important sub-class of Lévy subordinators are the tempered stable subordinators. For such subordinators, the Lévy measure ν(s) is given by
ν(s)=Cs−1−pexp(−ηs), | (11) |
where C>0 and η>0. Important special cases are the Gamma subordinator with p=0, the IG subordinator with p=12 and the compound Poisson subordinator with p=−1. For such subordinators, the Lévy exponent is given by
ϕ(λ)={γλ−CΓ(−p)[(λ+η)p−ηp],p≠0γλ+Clog(1+λη),p=0. | (12) |
We can also reparameterize the exponent by setting
{ϑ=−CΓ(−p)pηp−1, ω=−CΓ(−p)p(1−p)ηp−2,p≠0ϑ=Cη, ω=Cη2,p=0, | (13) |
where ϑ=E(L(1))−γ and ω=Var(L(1)).
Then, the reparameterized exponent takes the following form:
ϕ(λ)={γλ+ϑp[ϑω(1−p)]−p+1[(λ+ϑω(1−p))p−(ϑω(1−p))p],p≠0γλ+ϑ2ωlog(1+λωϑ),p=0. | (14) |
From T(t)=L(A(t)), we know T is an additive subordinator, i.e. a non-negative and non-decreasing additive process (see e.g. Li et al., 2016b) and Y is an additive subordinate OU process (ASub-OU), whose drift, diffusion volatility and jumps are all time-dependent and seasonal. For more detailed discussions on general additive subordinators, we refer to Li et al. (2016a) and Li et al. (2017). For our model, we can calculate the Laplace transform of T as
E[exp(−λ(T(t)−T(s)))]=exp(−∫tsψ(λ,u)du)=exp[−ϕ(λ)∫tsb(u)du]=exp{−ϕ(λ)[b0(t−s)+365b12π(sin(2π(t−b2)365)−sin(2π(s−b2)365))]}, | (15) |
where ψ(λ,u) is called the density of the Laplace exponent and is given by
ψ(λ,u)=γb(u)λ+∫(0,∞)(1−exp(−λs))b(s)ν(ds). | (16) |
We note that T(t)=L(t) when b0=1 and b1=0. In this case, Y is a Lévy subordinate OU process (LSub-OU) and it will be a jump-diffusion process with mean-reverting diffusion drift and mean-reverting jumps if γ>0 or a pure jump process with mean-reverting jumps if γ=0. For this specification, the drift, diffusion volatility and jumps intensity are all time-independent.
To price temperature derivatives, we need to specify a risk-neutral probability measure Q. However, it is not possible to store or trade temperature and therefore, the temperature market is an incomplete market. Any probability measure Q equivalent to the physical measure P is a risk-neutral measure. In this paper, we will follow Li et al. (2016a) and Li et al. (2016b) and assume that the process for Y under measure Q remains the same as under P, but with possibly different parameters to account for risk premium. The generating tuples (κ, θ, σ, b(⋅), γ, ν(⋅)) for Y under the measure P then becomes (κ, ˉθ, σ, b(⋅), γ, ν(⋅)) under the measure Q. Therefore, we assume after the change of measure, θ becomes ˉθ and other parameters remain the same.
Under the risk-neutral measure Q, the OU process X defined in (5) becomes
dX(t)=κ(ˉθ−X(t))dt+σdBQ(t), | (17) |
where BQ is a standard Brownian motion, independent of the time change process T.
For the OU process X(t) defined in (17), its infinitesimal generator L is given by
Lf(x)=κ(ˉθ−x)f′(x)+12σ2f″(x), | (18) |
where f is a transformation function. f′ and f″ are the first- and the second-order derivatives of f, respectively.
The infinitesimal generator L with domain dom(L) is always self-adjoint on the Hilbert space L2((−∞,∞),m) of functions on (−∞,∞) square integrable with the speed measure m(dx)=m(x)dx and endowed with the inner product (f,g), 1 where
1An operator L with domain dom(L) is said to be self-adjoint if (Lf,g)=(f,Lg) ∀f,g∈dom(L).
m(x)=2σ2exp(−κ(ˉθ−x)2σ2), | (19) |
and
(f,g)=∫∞−∞f(x)g(x)m(x)dx. | (20) |
We are interested in the transition semigroup (Pt)t≥0 of X defined by
Ptf(x)=EQ[f(X(t))|X(0)=x]. | (21) |
We can use the eigenfunction expansion method for self-adjoint operators in Hilbert spaces to write down the spectral decomposition of Ptf(x) (see e.g. Linetsky and Mitchell, 2008).
Proposition 1. For any f∈L2((−∞,∞),m), the transition semigroup of the OU process X has an eigenfunction expansion of the form
Ptf(x)=∞∑n=0fnexp(−λnt)φn(x), | (22) |
where fn=(f,φn), {λn} are the eigenvalues of −L and {φn} are the corresponding eigenfunctions satisfying the following Sturm-Liouville equation
−Lφn=λnφn. | (23) |
The eigenvalues and eigenfunctions of OU process can be summarized in the following result (see e.g. Linetsky and Mitchell, 2008):
Proposition 2. For the OU process X defined in (17), the eigenvalues λn and the eigenfunction φn, n=0,1,…, are
λn=κn, | (24) |
and
φn(x)=NnHn(ξ), | (25) |
where ξ=√κσ(x−ˉθ), Nn=√σ√κ2n+1n!√π and Hn(x) is the Hermite polynomial defined as
Hn(x)=n!⌊n2⌋∑m=0(−1)mm!(n−2m)!(2x)n−2m. | (26) |
For the subordinate OU process Y defined in (4), we can also compute the transition semigroup (Pψt)t≥0 of Y, where ψ is the density of Laplace exponent for the additive subordinator T:
Pψtf(x)=EQ[f(Y(t))|Y(0)=x]. | (27) |
We can employ the eigenfunction expansion method again to compute the semigroup Pψtf(x) using the following result (see e.g. Li and Linetsky, 2014):
Proposition 3. For any f∈L2((−∞,∞),m), the transition semigroup of the subordinate OU process Y has an eigenfunction expansion of the form
Pψtf(x)=∞∑n=0fnexp(−∫t0ψ(λn,u)du)φn(x), | (28) |
where {λn} and {φn} are the eigenvalues and the eigenfunctions of OU process and can be obtained from (24) and (25), respectively. The exponential function exp(−∫t0ψ(λn,u)du) is the Laplace transform of the time change process T and can be calculated using (15).
From the above results, it is clear that a key feature of the eigenfunction expansion method is that the temporal and spatial variables are separated. The time variable t enters the expansion only through the exponential function exp(−λnt). The eigenfunction expansion of time-changed process Y has the same form as the original process X, but with exp(−λnt) replaced by exp(−∫t0ψ(λn,u)du). As long as the Laplace transform of the time change process T can be calculated in closed form, the time-changed model will be as tractable as the original model.
For our new pricing model, the eigenvalues and the eigenfunctions of the OU process can be calculated easily from Proposition 2. To employ the eigenfunction expansion method to calculate the temperature derivative prices, we still need to obtain the eigenfunction expansion coefficient fn. Here, we provide the formulas for several integrals that will be useful for calculating fn. The following integrals can be computed using the results of Prudnikov et al. (1986) and Lim et al. (2012):
Lemma 1.
(i)
ˉβn:=∫∞−∞exp(−x2)Hn(x)dx={√π,n=00,n≠0. | (29) |
(ii)
βn(u):=∫u−∞exp(−x2)Hn(x)dx={√πΦ(√2u),n=0−Hn−1(u)exp(−u2),n≠0, | (30) |
where Φ(x) is the CDF of standard normal distribution.
(iii)
ˉγm,n:=∫∞−∞exp(−x2)Hm(x)Hn(x)dx={√π2nn!,m=n0,m≠n. | (31) |
(iv) Define
γm,n(u):=∫u−∞exp(−x2)Hm(x)Hn(x)dx. | (32) |
Then, γm,n(u) can be calculated recursively as follows:
γ0,0(u)=√πΦ(√2u), γn,n(u)=2nγn−1,n−1(u)−Hn−1(u)Hn(u)exp(−u2), n≥1, | (33) |
and for m≠n,
γm,n(u)=Hm(u)Hn+1(u)−Hn(u)Hm+1(u)2(n−m)exp(−u2). | (34) |
We can also prove the following results:
Corollary 1.
(i)
ˉan:=∫∞−∞φnm(x)dx=2Nnσ√κˉβn. | (35) |
(ii)
ˉbn:=∫∞−∞xφnm(x)dx=2Nnσ√κ[σ2√κˉγn,1+ˉθˉβn]. | (36) |
(iii)
ˉcm,n:=∫∞−∞φmφnm(x)dx={1,m=n0,m≠n. | (37) |
(iv)
an(u):=∫u−∞φnm(x)dx=2Nnσ√κβn(√κσ(u−ˉθ)). | (38) |
(v)
bn(u):=∫u−∞xφnm(x)dx=2Nnσ√κ[σ2√κγn,1(√κσ(u−ˉθ))+ˉθβn(√κσ(u−ˉθ))]. | (39) |
(vi)
cm,n(u):=∫u−∞φmφnm(x)dx=2NmNnσ√κγm,n(√κσ(u−ˉθ)). | (40) |
Proof. (ⅰ)-(ⅵ) follow directly from Lemma 1.
The payoff of a temperature derivative is typically linked to an underlying temperature index such as CAT, HDD or CDD, which can be defined as
CAT(tN1,tN2)=N2∑k=N1S(tk), | (41) |
HDD(tN1,tN2)=N2∑k=N1max(c−S(tk),0), | (42) |
and
CDD(tN1,tN2)=N2∑k=N1max(S(tk)−c,0), | (43) |
where c is typically 18∘C or 65∘F.
Assume the time t value of temperature S(t)=x. Denote Ind∈{CAT,CDD,HDD} and let FInd(t,tN1,tN2,x) with t<tN1<tN2 be the time t price of temperature futures written on the corresponding index over period [tN1,tN2]. The future price can be calculated as:
FInd(t,tN1,tN2,x)=EQ[Ind(tN1,tN2)|Ft]. | (44) |
We have the following parity for the temperature futures.
Lemma 2. The prices of CAT, HDD and CDD futures have the following relationship
FCDD(t,tN1,tN2,x)=FHDD(t,tN1,tN2,x)+FCAT(t,tN1,tN2,x)−(N2−N1+1)c. | (45) |
Proof. The result follows from max(x−c,0)−max(c−x,0)=x−c.
Assume the time t value of temperature S(t)=x. Let PInd(t,K,tN0,tN1,tN2,x) be the time t price of a put option with strike price K and maturity tN0, written on a future contract with payoff FInd(tN0,tN1,tN2,S(tN0)). Then the put price can be calculated from:
PInd(t,K,tN0,tN1,tN2,x)=exp(−r(tN0−t))EQ[max(K−FInd(tN0,tN1,tN2,S(tN0)),0)|Ft], | (46) |
where r is the risk-free interest rate.
Let CInd(t,K,tN0,tN1,tN2,x) be the corresponding temperature call option. We have the following put-call parity for the temperature future options.
Lemma 3.
CInd(t,K,tN0,tN1,tN2,x)=PInd(t,K,tN0,tN1,tN2,x)+exp(−r(tN0−t))(FInd(t,tN1,tN2,x)−K). |
Proof. The result follows from max(x−K,0)−max(K−x,0)=x−K.
We can derive the analytical solutions for CAT, HDD and CDD future prices from the following results.
Proposition 4. Assume that the stochastic processes for the asset price S(t) is specified in (2), (4), (17) and the time change process T(t) is an additive subordinator with the density of Laplace transform ψ, then the time t prices of CAT, HDD and CDD futures can be calculated as
(i)
FCAT(t,tN1,tN2,x)=N2∑k=N1[Λ(tk)+1∑n=0fCATnexp(−∫tktψ(λn,u)du)φn(x−Λ(t))], | (47) |
where λn and φn are in (24) and (25), respectively. Furthermore, fCATn=ˉbn.
(ii)
FHDD(t,tN1,tN2,x)=N2∑k=N1∞∑n=0fHDDn,kexp(−∫tktψ(λn,u)du)φn(x−Λ(t)), | (48) |
where fHDDn,k=(c−Λ(tk))an(c−Λ(tk))−bn(c−Λ(tk)).
(iii)
FCDD(t,tN1,tN2,x)=N2∑k=N1∞∑n=0fCDDn,kexp(−∫tktψ(λn,u)du)φn(x−Λ(t)), | (49) |
where fCDDn,k=(Λ(tk)−c)(ˉan−an(c−Λ(tk)))+ˉbn−bn(c−Λ(tk)).
Proof.
We will focus on (ⅱ). (ⅰ) and (ⅲ) can be proved in a similar way.
FHDD(t,tN1,tN2,x)=N2∑k=N1EQ[(c−Λ(tk)−Y(tk))+|Ft]=N2∑k=N1EQ[(c−Λ(tk))1{Y(tk)<c−Λ(tk)}−Y(tk)1{Y(tk)<c−Λ(tk)}|Ft]. | (50) |
Using eigenfunction expansion and Corollary 1, we have
FHDD(t,tN1,tN2,x)=N2∑k=N1∞∑n=0fHDDn,kexp(−∫t0ψ(λn,u)du)φn(x−Λ(t)), | (51) |
where
fHDDn,k=(c−Λ(tk))∫c−Λ(tk)−∞φn(x)m(x)dx−∫c−Λ(tk)−∞xφn(x)m(x)dx=(c−Λ(tk))an(c−Λ(tk))−bn(c−Λ(tk)). | (52) |
We can also derive the analytical solutions for CAT, HDD and CDD future option prices from the following results.
Proposition 5. Assume that the stochastic processes for the asset price S(t) is specified in (2), (4), (17) and the time change process T(t) is an additive subordinator with the density of Laplace transform ψ, then the time t prices of CAT, HDD and CDD future options can be calculated as
(i)
PCAT(t,K,tN0,tN1,tN2,x)=exp(−r(tN0−t))∞∑m=0gCATmexp(−∫tN0tψ(λm,u)du)φm(x−Λ(t)), | (53) |
where
gCATm=(K−N2∑k=N1Λ(tk))am(x∗)−N2∑k=N11∑n=0fCATnexp(−∫tktN0ψ(λn,u)du)cm,n(x∗), | (54) |
where x∗ = x∗∗−Λ(tN0) and x∗∗ is the solution to the equation FCAT(tN0,tN1,tN2,x)=K.
(ii)
PHDD(t,K,tN0,tN1,tN2,x)=exp(−r(tN0−t))∞∑m=0gHDDmexp(−∫tN0tψ(λm,u)du)φm(x−Λ(t)), | (55) |
where
gHDDm=K(ˉam−am(x∗))−N2∑k=N1∞∑n=0fHDDn,kexp(−∫tktN0ψ(λn,u)du)(ˉcm,n−cm,n(x∗)), | (56) |
where x∗ = x∗∗−Λ(tN0) and x∗∗ is the solution to the equation FHDD(tN0,tN1,tN2,x)=K.
(iii)
PCDD(t,K,tN0,tN1,tN2,x)=exp(−r(tN0−t))∞∑m=0gCDDmexp(−∫tN0tψ(λm,u)du)φm(x−Λ(t)), | (57) |
where
gCDDm=Kam(x∗)−N2∑k=N1∞∑n=0fCDDn,kexp(−∫tktN0ψ(λn,u)du)cm,n(x∗), | (58) |
where x∗ = x∗∗−Λ(tN0) and x∗∗ is the solution to the equation FCDD(tN0,tN1,tN2,x)=K.
Proof. To prove (ⅰ), let x∗∗ be the solution to the equation FCAT(tN0,tN1,tN2,x)=K and x∗ = x∗∗−Λ(tN0), then we have
PCAT(t,K,tN0,tN1,tN2,x)=exp(−r(tN0−t))EQ[(K−FCAT(tN0,tN1,tN2,S(tN0)))+|Ft]=exp(−r(tN0−t))EQ[(K−FCAT(tN0,tN1,tN2,S(tN0)))1{Y(tN0)<x∗}|Ft]. | (59) |
Using eigenfunction expansion, Corollary 1 and Proposition 4.1, we get
PCAT(t,K,tN0,tN1,tN2,x)=exp(−r(tN0−t))∞∑m=0gCATmexp(−∫tN0tψ(λm,u)du)φm(x−Λ(t)), | (60) |
where
gCATm=(K−N2∑k=N1Λ(tk))∫x∗−∞φm(x)m(x)dx−N2∑k=N11∑n=0fCATnexp(−∫tktN0ψ(λn,u)du)∫x∗−∞φm(x)φn(x)m(x)dx=(K−N2∑k=N1Λ(tk))am(x∗)−N2∑k=N11∑n=0fCATnexp(−∫tktN0ψ(λn,u)du)cm,n(x∗). | (61) |
Similarly, we can prove (ⅱ) and (ⅲ).
We carry out an empirical study using the average daily temperature of Toronto, Canada, as measured at Pearson International Airport from January 1, 2003 to December 31, 2012. The data was collected from the website of National Climate Data and Information Archive of Canada. In Figure 1, we plot the average temperature for the whole sample. It is clear that the temperature has a seasonal pattern. As specified in Section 2, we model the temperature S(t) as the sum of the seasonal trend term Λ(t) and the deseasonalized stochastic component Y(t). The estimation procedure is performed in two steps.
In the first step, using linear regression we estimate Λ according to (3). The estimation results are in Table 1. All the parameters in (3) are statistically significant at the 1% level. In particular, the temperature has a weak but significantly positive linear trend. In Figure 1, we also plot the seasonal component Λ, which indicates that it captures the seasonal pattern in the temperature very well.
Parameters | Estimates | Standard error | t-statistics |
a0 | 8.15 | 0.14 | 57.40 |
a1 | 4.55e−4 | 6.74e−5 | 6.76 |
a2 | 13.70 | 0.10 | 136.63 |
a3 | −525.50 | 0.43 | −1232.67 |
In the second step, we estimate three models for the deseasonalized temperature Y. The first model is the OU model based on the process in (5) and Y(t)=X(t). We impose the restriction θ=0 for identification purpose. The other two models are the LSub-OU and the ASub-OU models. For both models, the Lévy subordinator is taken to be the Gamma subordinator with Lévy exponent given in (14). To uniquely identify the parameters of the models, we set ϑ=1. For the ASub-OU model, the activity rate function b is specified in (6), with the restrictions b0=1 and b0≥|b1|. Note if b1=0, the ASub-OU model reduces to the LSub-OU model. We also set the drift term of the Gamma subordinator γ=0, so Y will be a pure jump process with mean reverting jumps.
We choose maximum likelihood estimation (MLE) method for all the models. For a sample of size n of {S(ti)}ni=1, the log conditional likelihood function is given by
log(L(Θ))=n∑i=1log(p(ti,ti+1,S(ti),S(ti+1);Θ)), | (62) |
where Θ is the set of parameters to be estimated. p(ti,ti+1,S(ti),S(ti+1);Θ) is the transition density function.
Define
Ξ(u,i)=Λ(ti+1)+[S(ti)−Λ(ti)]exp(−κu)+θ(1−exp(−κu)), | (63) |
and
Σ(u)=σ22κ(1−exp(−2κu)). | (64) |
Then, the transition density function for the OU model is
p(ti,ti+1,S(ti),S(ti+1);Θ)=1√2πΣ(1)exp(−(S(ti+1)−Ξ(1,i))2Σ(1)). | (65) |
Let gt(du) be the transition probability distribution of a Gamma subordinator with zero drift, mean ϑ and variance ω, then gt(du) is given by
gt(du)=(ϑω)ϑ2t/ωΓ(ϑ2ωt)uϑ2t/ω−1exp(−ϑωu)du. | (66) |
Then, the transition density function for the ASub-OU model is given by
p(ti,ti+1,S(ti),S(ti+1);Θ)=∫[0,∞)1√2πΣ(u)exp(−(S(ti+1)−Ξ(u,i))2Σ(u))gA(ti+1)−A(ti)(du). | (67) |
This integral can be efficiently computed by the Gauss-Laguerre quadrature. Note if we impose the restriction b1=0, we can also obtain the transition density function for the LSub-OU model.
The MLE results for the deseasonalized temperature process Y are provided in Table 2. We find that all of the parameters of each model are estimated with high significance. Once the time change is introduced, the volatility parameter of the OU process drops significantly. This is not surprising since in the models with time change, the volatility is not only achieved through the diffusion term of diffusion process, but also can be realized through the contribution of the jumps. In Table 2, we also report the log-likelihood function values for three models. Since OU is nested in LSub-OU and LSub-OU is nested in ASub-OU, we can perform the likelihood ratio test to compare the goodness-of-fit. The likelihood ratio test statistics are 119.8 and 117.44 for OU versus LSub-OU and LSub-OU versus ASub-OU, respectively. Using the critical value of 3.84 for 95% quantile of the Chi-square distribution with one degree of freedom, we can clearly see that the two time-changed models are superior to the OU model and the ASub-OU model outperforms the LSub-OU model.
Parameters | OU | LSub-OU | ASub-OU |
κ | 0.36 | 0.39 | 0.34 |
(23.48) | (17.64) | (18.39) | |
σ | 3.64 | 0.38 | 0.37 |
(86.66) | (45.76) | (51.20) | |
ω | 0.59 | 0.41 | |
(8.59) | (6.72) | ||
b1 | 0.44 | ||
(12.46) | |||
b2 | 31.84 | ||
(6.43) | |||
Log likelihood | −9280.24 | −9220.34 | −9161.62 |
Note: The t-statistics are in the parentheses. |
In Figure 2, we plot the deseasonalized temperature Y together with the activity rate function b. It is clear that the seasonality still exists in the process Y. The diffusive volatility and jump intensity display seasonality, which are well captured by the function b in the ASub-OU model.
We also apply the eigenfunction expansion method to compute the temperature future and future option prices using the results in Section 4. In order to apply the eigenfunction expansion method to compute the derivative prices, we need to truncate eigenfunctions expansions after a finite number of terms. We price CAT and CDD futures and put options on the futures using the analytical formulas derived in Section 4. We take the model parameters from those reported in Tables 1 and 2. Other parameters are assumed to be the same as those in Figures 3 and 4. In Table 3, we demonstrate that only a small number of eigenfunctions are needed for the prices to converge.
Truncation terms | CAT futures | CAT future options | CDD futures | CDD future options |
1 | 689.854721 | 0.082439 | 145.118628 | 0.014278 |
2 | 689.854721 | 0.081924 | 145.118436 | 0.013929 |
3 | 689.854721 | 0.081908 | 145.118429 | 0.013911 |
4 | 689.854721 | 0.081906 | 145.118429 | 0.013911 |
5 | 689.854721 | 0.081906 | 145.118429 | 0.013911 |
Note: The parameters are taken from Tables 1 and 2. ˉθ=0.67. |
To take the risk premium into consideration, we need to specify the values of ˉθ for OU process X under the risk-neutral Measure Q. In Figures 3 and 4, we compare the futures and future options for different values of ˉθ under the OU, LSub-OU and ASub-OU models. As expected, both CAT and CDD future prices are a linear function of ˉθ, whereas CAT and CDD future option prices are a decreasing function of ˉθ. It is also clear that the market price of risk has a significant role for temperature derivatives. We also find different models can generate different values for temperature derivative prices. For CAT futures and future options, three models produce similar prices. However, the model prices from ASub-OU model can deviate from the other models significantly for CDD futures and future options.
This paper proposes a new model for temperature based on the time-changed OU process, where the time change process is a Lévy subordinator time changed by a deterministic clock with seasonal activity rate. Compared to the previous models, our model allows for state-dependent jumps. Furthermore, under our model, the drift, diffusion volatility and jumps are all seasonal which are empirically supported in the temperature data. Another advantage of our model is that we are able to employ the eigenfunction expansion technique to derive the analytical pricing formulas for the temperature futures and future options. From an empirical study using Toronto temperature data, we show that clearly our model with additive subordination provides a better fit than the competing models. We also demonstrate that the market price of risk has significant impact on the temperature derivative prices.
There are several future works that can be considered. We can introduce a stochastic volatility component into our model by a further time change based on the absolutely continuous time change processes. The combination of a subordinator and an integral of some positive functions of a Markov process with analytically tractable Laplace transform has been studied in Mendoza-Arriaga et al. (2010) and Li and Linetsky (2014). The model based on the combined time change will still be tractable since it still possesses the closed-form Laplace transform for the time change, which enables us to continue to apply the eigenfunction expansion method. In addition, we would like to calibrate our model using the real market data for the temperature derivatives and compare its performance with the existing popular models.
This research did not receive any specific grant from funding agencies in the public, commercial, or not-for-profit sectors.
All authors declare no conflicts of interest in this paper.
[1] | Benth FE (2003) On arbitrage-free pricing of weather derivatives based on fractional Brownian motion. Quant Financ 10: 303-324. |
[2] |
Benth FE, Šaltytė-Benth J (2005) Stochastic modeling of temperature variations with a view towards weather derivatives. Appl Math Financ 12: 53-85. doi: 10.1080/1350486042000271638
![]() |
[3] | Benth FE, Šaltytė-Benth J, Koekebakker S (2007) Putting a price on temperature. Scand J Stat 34: 746-767. |
[4] |
Brody DC, Syroka J, Zervos M (2002) Dynamical pricing of weather derivatives. Quant Financ 2: 189-198. doi: 10.1088/1469-7688/2/3/302
![]() |
[5] |
Carr P, Wu L (2004) Time-changed Lévy processes and option pricing. J Finan Econ 1: 113-141. doi: 10.1016/S0304-405X(03)00171-5
![]() |
[6] | Cui Z, Kirkby JL, Nguyen D (2019a) Continuous-time Markov chain and regime switching approximations with applications to options pricing, in Yin, G, Zhang, Q, Modeling, Stochastic Control, Optimization, and Applications, Springer, 115-146. |
[7] | Cui Z, Kirkby JL, Nguyen D (2019b) A general framework for time-changed Markov processes and applications. Eur J Oper Res 273: 785-800. |
[8] | Dornier F, Queruel M (2000) Caution to the wind. Risk 8: 30-32. |
[9] |
Elias RS, Wahab MIM, Fang L (2014) A comparison of regime-switching temperature modeling approaches for applications in weather derivatives. Eur J Oper Res 232: 549-560. doi: 10.1016/j.ejor.2013.07.015
![]() |
[10] |
Evarest E, Berntsson F, Singull M, et al. (2018) Weather derivatives pricing using regime switching model. Monte Carlo Methods Appl 24: 13-28. doi: 10.1515/mcma-2018-0002
![]() |
[11] | Li J, Li L, Mendoza-Arriaga R (2016a) Additive subordination and its applications in finance. Financ Stoch 20: 589-634. |
[12] |
Li J, Li L, Zhang G (2017) Pure jump models for pricing and hedging VIX derivatives. J Econ Dyn Contro 74: 28-55. doi: 10.1016/j.jedc.2016.11.001
![]() |
[13] |
Li L, Linetsky V (2014) Time-changed Ornstein-Uhlenbeck processes and their applications in commodity derivative models. Math Financ 24: 289-330. doi: 10.1111/mafi.12003
![]() |
[14] |
Li L, Mendoza-Arriaga R, Mo Z, et al. (2016b) Modelling electricity prices: a time change approach. Quant Financ 16: 1089-1109. doi: 10.1080/14697688.2015.1125521
![]() |
[15] |
Li L, Zhang G (2018) Error analysis of finite difference and Markov chain approximations for option pricing. Math Financ 28: 877-919. doi: 10.1111/mafi.12161
![]() |
[16] |
Lim D, Li L, Linetsky V (2012) Evaluating callable and putable bonds: an eigenfunction expansion approach. J Econ Dyn Contro 36: 1888-1908. doi: 10.1016/j.jedc.2012.06.002
![]() |
[17] |
Linetsky V (2004) The spectral decomposition of the option value. Int J Theor Appl Financ 7: 337-384. doi: 10.1142/S0219024904002451
![]() |
[18] | Linetsky V, Mitchell D (2008) Spectral methods in derivatives pricing, in Birge, JR, Handbook of Financial Engineering, Amsterdam: Elsevier, 223-299. |
[19] |
Mendoza-Arriaga R, Carr P, Linetsky V (2010) Time changed Markov processes in unified credit-equity modeling. Math Financ 20: 527-569. doi: 10.1111/j.1467-9965.2010.00411.x
![]() |
[20] |
Mendoza-Arriaga R, Linetsky V (2013) Time-changed CIR default intensities with two-sided meanreverting jumps. Ann Appl Probab 24: 811-856. doi: 10.1214/13-AAP936
![]() |
[21] | Prudnikov AP, Brychkov YA, Marichev OI (1986) Integrals and Series, Vol. 2, Gordon and Breach Science Publishers. |
[22] | Sato K (1999) Lévy Processes and Infinitely Divisible Distribution, Cambridge: Cambridge University Press. |
[23] |
Swishchuk A, Cui S (2013) Weather derivatives with applications to Canadian data. J Math Financ 3: 81-95. doi: 10.4236/jmf.2013.31007
![]() |
[24] |
Tong KZ, Hou D, Guan J (2019) The pricing of dual-expiry exotics with mean reversion and jumps. J Math Financ 9: 25-41. doi: 10.4236/jmf.2019.91003
![]() |
[25] |
Tong Z (2017) A nonlinear diffusion model for electricity prices and derivatives. Int J Bonds Deriv 3: 290-319. doi: 10.1504/IJBD.2017.091606
![]() |
[26] |
Tong Z (2019) A recursive pricing method for autocallables under multivariate subordination. Quant Financ Econ 3: 440-455. doi: 10.3934/QFE.2019.3.440
![]() |
[27] | Tong Z, Liu A (2017) Analytical pricing formulas for discretely sampled generalized variance swaps under stochastic time change. Int J Financ Eng 4: 1-24. |
[28] | Tong Z, Liu A (2018) Analytical pricing of discrete arithmetic Asian options under generalized CIR process with time change. Int J Financ Eng 5: 1-21. |
1. | Zhenzhen Wang, Hao Dong, Zhehao Huang, Carbon spot prices in equilibrium frameworks associated with climate change, 2023, 19, 1547-5816, 961, 10.3934/jimo.2021214 | |
2. | Shuaishuai Jia, Cunyi Yang, Mengxin Wang, Pierre Failler, Heterogeneous Impact of Land-Use on Climate Change: Study From a Spatial Perspective, 2022, 10, 2296-665X, 10.3389/fenvs.2022.840603 | |
3. | Meng Yang, Hujun Li, Fangzhao Deng, Qinchen Yang, Ning Ba, Yunxia Guo, Haitao Wu, Muhammad Irfan, Yu Hao, Inclusivity between internet development and energy conservation in Henan, China, 2023, 16, 1570-646X, 10.1007/s12053-023-10144-2 | |
4. | Michele Azzone, Roberto Baviera, Is (Independent) Subordination Relevant in Equity Derivatives?, 2024, 1524-1904, 10.1002/asmb.2904 | |
5. | Xin Yun, Yang Hu, An Overview of the Evolution in the Research Landscape of Green Finance, 2024, 5, 2673-4060, 1335, 10.3390/world5040068 |
Parameters | Estimates | Standard error | t-statistics |
a0 | 8.15 | 0.14 | 57.40 |
a1 | 4.55e−4 | 6.74e−5 | 6.76 |
a2 | 13.70 | 0.10 | 136.63 |
a3 | −525.50 | 0.43 | −1232.67 |
Parameters | OU | LSub-OU | ASub-OU |
κ | 0.36 | 0.39 | 0.34 |
(23.48) | (17.64) | (18.39) | |
σ | 3.64 | 0.38 | 0.37 |
(86.66) | (45.76) | (51.20) | |
ω | 0.59 | 0.41 | |
(8.59) | (6.72) | ||
b1 | 0.44 | ||
(12.46) | |||
b2 | 31.84 | ||
(6.43) | |||
Log likelihood | −9280.24 | −9220.34 | −9161.62 |
Note: The t-statistics are in the parentheses. |
Truncation terms | CAT futures | CAT future options | CDD futures | CDD future options |
1 | 689.854721 | 0.082439 | 145.118628 | 0.014278 |
2 | 689.854721 | 0.081924 | 145.118436 | 0.013929 |
3 | 689.854721 | 0.081908 | 145.118429 | 0.013911 |
4 | 689.854721 | 0.081906 | 145.118429 | 0.013911 |
5 | 689.854721 | 0.081906 | 145.118429 | 0.013911 |
Note: The parameters are taken from Tables 1 and 2. ˉθ=0.67. |
Parameters | Estimates | Standard error | t-statistics |
a0 | 8.15 | 0.14 | 57.40 |
a1 | 4.55e−4 | 6.74e−5 | 6.76 |
a2 | 13.70 | 0.10 | 136.63 |
a3 | −525.50 | 0.43 | −1232.67 |
Parameters | OU | LSub-OU | ASub-OU |
κ | 0.36 | 0.39 | 0.34 |
(23.48) | (17.64) | (18.39) | |
σ | 3.64 | 0.38 | 0.37 |
(86.66) | (45.76) | (51.20) | |
ω | 0.59 | 0.41 | |
(8.59) | (6.72) | ||
b1 | 0.44 | ||
(12.46) | |||
b2 | 31.84 | ||
(6.43) | |||
Log likelihood | −9280.24 | −9220.34 | −9161.62 |
Note: The t-statistics are in the parentheses. |
Truncation terms | CAT futures | CAT future options | CDD futures | CDD future options |
1 | 689.854721 | 0.082439 | 145.118628 | 0.014278 |
2 | 689.854721 | 0.081924 | 145.118436 | 0.013929 |
3 | 689.854721 | 0.081908 | 145.118429 | 0.013911 |
4 | 689.854721 | 0.081906 | 145.118429 | 0.013911 |
5 | 689.854721 | 0.081906 | 145.118429 | 0.013911 |
Note: The parameters are taken from Tables 1 and 2. ˉθ=0.67. |